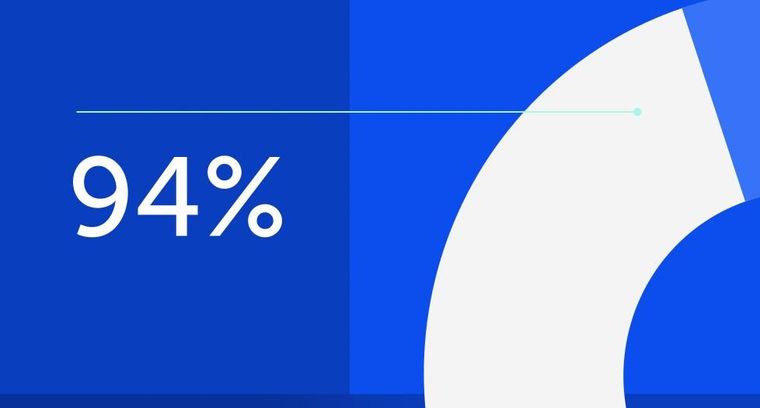
94% of researchers rate our articles as excellent or good
Learn more about the work of our research integrity team to safeguard the quality of each article we publish.
Find out more
ORIGINAL RESEARCH article
Front. For. Glob. Change, 20 November 2024
Sec. People and Forests
Volume 7 - 2024 | https://doi.org/10.3389/ffgc.2024.1495806
This article is part of the Research TopicRecreational forests for co-benefits: conservation, tourism and well-beingView all 5 articles
Urban scenic forests provide urban residents with various physical and mental health and wellbeing services. However, in the research on the color quantification and health services of scenic forests, it is still unclear how their color quantification characteristics feedback on the stress - attention of the adolescent group. In this study, visual color elements (green, red and yellow) of three landscape forests were used to generate 48 images of four groups of urban landscape forests according to color combinations and proportions. Virtual images were used to assess the stress indicators and attention indicators of participants before and after viewing. The results showed that the four groups of experimental groups showed varying degrees of α wave reduction and β/α ratio increase after viewing, G1 group showed an extremely significant increase in β wave after image stimulation, G1 and G3 group significantly decreased θ/β power, G4 group and G5 control group had no significant change. Among the 16 channels of absolute α wave and absolute β wave in the brain, the F3 and F4 channels corresponding to the frontal lobe of the G3 group showed the most prominent consistency of β wave in the frontal cortex during highly concentrated mental activities. Our study shows that positive EEG (Electroencephalogram) feedback of arousal and attention recovery can be obtained by using monochromatic or two-color changing color features in urban landscape forest. This study can provide references and methods for urban forest planning, design and visual evaluation.
The development of global densification has significantly increased the risk of anxiety and mood disorders among urban residents, especially with the increase of life pressure, urban people need more convenient ways to provide attention, cognitive recovery and stress reduction. Good urban natural planning is considered to be an effective means to address the cumulative benefits of public health (Norwood et al., 2019). A large number of experiments in cities based on the mediating effects of natural interventions or natural connections (Korpela et al., 2017) have demonstrated the important role of natural outdoor Spaces in improving general health and wellbeing, neurodevelopmental and cognitive skills, and social, emotional and behavioral development (Shoari et al., 2021). The WHO recommends that urban populations should reach green Spaces within 300 meters or a 5-min walk (Zhu et al., 2023). But ironically, in the limited space of the city, the tedious daily work greatly reduces the powerful potential of outdoor natural contact. If we can explore more efficient health interventions through the changeability of the urban green space environment, it will not only benefit the integrity of the ecological service system, but also protect the health of urban residents (Wolch et al., 2014). Urban forest is an important part of urban natural planning, and its ecological, health and welfare and social and economic benefits are highly valued by the society (Butt et al., 2021). Color change is an important source and reflection of urban forest diversity and experience. Although the potential health benefits of color in urban forests are well documented, more detailed mechanisms affecting health, such as color combinations and proportions, have not been fully studied. Therefore, the aim of this study is to evaluate and optimize the feedback of EEG indicators by controlling color quantification.
Forest is a three-dimensional space that changes with time, and forest exposure under different property changes is conducive to obtaining different degrees of health promotion. These studies contribute to the overall value of urban landscape forests, not only enabling them to function as part of an ecosystem, but also highlighting their importance as a potential public health resource by increasing their recovery potential. There are two main ways to study the health benefits of urban landscape forest based on color: First, the color of vegetation is limited to green, and different environmental characteristics [plant richness, area (Zhu et al., 2023), element arrangement and coordination (Tomasso et al., 2023), etc.] while emphasizing the important restoration role of natural landscape. For example, some studies have shown that the most suitable scene for human brain is the three-layer vegetation structure with a green landscape index of 60–80%, and the two-layer vegetation structure with a VGI of more than 80% has the largest instantaneous weakening effect (Butt et al., 2021; Zhu et al., 2021). The presence of 20–35 fully grown trees in the 200 m range had more positive emotional feedback (Hao et al., 2024). In the panoramic view measured at eye level, vegetation coverage should not be <41% to ensure moderate preference values and ecological benefits (Jiang et al., 2015). These studies have obtained more detailed quantitative information in the green environment, so as to carry out different dimensions of health promotion through landscape planning guidance, and are also the mainstream of current research to evaluate the physical characteristics of urban green space health support. Second, the measured values of greenness change under seasonal changes, and the seasonal differences in plant color display lead to different health benefits. Among them, with autumn studies as the mainstream, the quantitative values provided in urban forest management suggestions include the presentation of cold and warm leaf color to promote the increase of awakening consciousness (Neale et al., 2021), and the colorful flower cover has better aesthetic preference and psychological recovery than more than 27% (Wang et al., 2019). Psychopaths showed more positive facial expressions when viewing images of trees with autumn colors than green trees (Jang et al., 2014). Urban landscape forest is an important part of urban forest, and its color beauty is an important consideration for scientific management measures (Wang et al., 2020). In the existing studies to predict the possibility of its restoration, it can be seen that the color presentation of contact urban landscape forests is limited to the binary classification of “green” and “non-green,” and more detailed differentiation is lacking. The traditional value of urban landscape forests is recreation and landscape recreation, but the service function of improving human wellbeing reflects its indirect economic benefits, which are important for saving global public health costs, so the design decision of urban landscape forests should be based on detailed empirical data. To the best of our knowledge, more detailed quantification of the benefits of urban landscape forests is unclear, and this knowledge gap may hinder policymakers and professionals from quantifying the proportion of color in the conservation, design, and management of space, including in urban green space planning. One of the aims of this study was to provide a scientific basis for quantifying the health effects of landscape forest colors by investigating them.
Electroencephalography (EEG) is a non-invasive biofeedback tool for studying the electrical activity of the brain, which is characterized by accuracy and rapacity (Lin et al., 2020). It is a traditional method for studying the cognitive process of the brain, and is suitable for detecting users’ emotional recognition, stress level measurement, mental load and other applications in green space (Lin et al., 2023; Gibson et al., 2022). Most of the brain waves in the landscape field are realized by visual stimulation, and the environmental quality and landscape preference provided by visual stimulation can affect its feedback (Zhu et al., 2021). Electromagnetic waves generated by different frequencies and amplitudes can indicate brain activity and cortical arousal (Luo et al., 2023) for physiological assessment for different research purposes. The most relevant in greenfield health benefit studies are alpha (8–12.9 Hz) waves and beta (13–29.9 Hz) waves. Alpha waves are negatively correlated with physiological arousal, mainly occurring in a calm, relaxed state, including low frequency α (1.8–9.9 Hz) and high frequency α (2.10–12.9 Hz) depending on the frequency. Beta waves occur mainly in focus and concentration, divided into low frequency beta (1.13–17.9 Hz) and high frequency beta (2.18–29.9 Hz), and the higher arousal inherent in the beta band can also indicate emotional anxiety, anger, tension, and excitement. Some power values based on θ, α, and β can also represent pressure measurements, such as β/α can represent pressure indicators to describe the effect of stress on the nervous system, and higher β/α power indicates higher stress levels (Lin et al., 2023; Luo et al., 2023; Deng et al., 2020). Most of the studies on EEG feedback of urban landscape forest are combined with other factors. For example, Lin et al. (2023) discussed from the perspective of elevation therapy that when the proportion of leaves and sky elements is moderate, the lower the proportion of trunk elements is, the lower the β/α power will be. In the feedback differences of neural indicators of different behaviors under urban landscape forest, Wu et al. (2023) showed that in the six emotional parameters, the “Engagement” of sitting quietly was significantly lower than that of walking. Wang et al. (2021) show that color plays a major role in stimulating alpha waves in forest landscape types. These indicators convert EEG parameters into clinical biomarkers, and explore changes in EEG microstates to explore important links with landscape features with health benefits.
Because of the different EEG channels set up by different EEG devices, these channels may also explain the different EEG feedback information that can be provided. The neural parameters used for analysis are connected by these brain electrical channels to four brain regions, namely the frontal, temporal, parietal and occipital lobes, which are responsible for different behavioral functions and are involved in emotional feedback (Wu et al., 2023) (Figure 1). For example, when viewing natural scenery, the activation of the left parietal lobe and the right frontal lobe in the brain response to space, and the parietal activity related to goal orientation will change with different behavioral priorities (Wu et al., 2023). The P8 electrodes in the parietal lobe showed significant differences when the spatial processing of subjects’ classification was activated and the information was integrated under different behavior patterns (Wu et al., 2023). Zanzotto et al. found that α and β waves were most similar in the parietal and occipital lobes. Viewing high-density forest vegetation (100%) increased frontal brain activity (Tyrväinen et al., 2014). The brain wave channels F4 and F3 can be used as biomarkers to analyze the relaxation and arousal response in real space (Song et al., 2021). These EEG channels can also be combined with the bands described above to explore the feedback calculation of involuntary attention. For example, the arousal level and titer were calculated in the study of Paraskevopoulou et al. (2018) Arousal = (βF3 + βF4 + βAF3 + βAF4)/(αF3 + αF4 + αAF3 + αAF4); Titer = αF4-αF3. Although previous EEG feedback studies on urban green Spaces have a solid evidence base, a more direct understanding of how urban landscape forests affect mental recovery, and more detailed information relative to quantitative environmental control, is still lacking.
The purpose of this study was to investigate the effects of different color characteristics of urban landscape forest on stress and attention based on physiological EEG feedback. In the experiment, we used the mobile multi-channel Bitbrain wearable EEG system to monitor EEG data, and quantitatively analyzed the EEG feedback data by comparing the participants’ absolute α wave, α/β ratio, absolute β wave, θ/β ratio and the differences of 16 different EEG channels through the pre-test of pressure and distraction and the post-test of virtual image visual stimulation. It also provides reference for the color planning of urban landscape forest. The key research questions are: (1) whether urban landscape forests with different color characteristics have stress and attention recovery effects. (2) If it has recovery benefits, what are the differences between different experimental groups, and explore the causes and conditions causing the differences.
The vegetation composition of landscape forests in cities is often complex, often presenting different visual perception scenes due to structural (density, biodiversity level, location, etc.) or non-structural (season, nature of use, etc.) influences. Landscape forest is a three-dimensional space, which often presents different states from different perspectives. This study focuses on color quantification, so the spatial attribute of the research object is limited to carry out a more detailed discussion on color quantification. The first is to select a controllable vertical viewpoint to ensure the complete quantification after the superposition of elements; Second, the observation Angle is head-up, the vertical Angle between the line of sight and the visual plane is small, and people do not need to look up (look up) or arrive at a special observation point (look down) to see the complete landscape (Lin et al., 2023). Third, most of the research results support the restoration potential of higher biodiversity and vegetation structure (Martens et al., 2011), but overly complex vegetation structure will cause visual fatigue and poor processing (Wang et al., 2021). In this study, two layers of vegetation structure are selected: Ground cover and trees were used as the vegetation layer of urban landscape forest in this study. Since pure forest can help reduce non-structural confounding factors (Wu et al., 2023), the vegetation selection in this experiment was fixed and familiar to the participants.
The experimental image is composed of 30*60 rectangles and 18 10*10 cube images. Because different construction and non-construction factors may also affect health benefits, combined with previous studies (Lin et al., 2023; Zangeneh Soroush et al., 2017; Wang and Xu, 2021). We used 60% of urban landscape forest and 40% of random sky, architectural background and ground cover plants for virtual image composition, and added micro-dynamic effects to enhance realism. In order to facilitate quantitative calculation, we used six trees to form the image, and the quantified value of the total number of trees accounted for by one tree was 16.66%.Color Select the three most common colors: green (G), red (R); Yellow (Y) was quantitatively combined. These three colors are the main colors of urban forests in their evergreen, autumn-changing state. and a total of 8 color combinations were obtained after the combination of the three colors (Figure 1a). Since the collocation of green with other plants is the most common in nature, in order to increase the universality and quantification accuracy of the study, we only selected the color combination composed of green, namely group G1 (G:100%, R:0%, Y:0%) and group G2 (G:66.66%, R:33.33%, Y:0%). G3 group (G:66.66%, R:0%, Y:33.33%), G4 group (R:33.33%, G: 33.33%, Y: 33.33%) (Figure 1b). In addition, brightness, hue and saturation are the three elements of color. In order to better study the proportion of the three colors, we chose the three elements of color from low to high for the gradual change of three red colors (Figure 1c). Then, according to the arrangement and combination of the four groups based on the change of green, red and yellow, and referring to the quantification standard of color matching, 27 kinds of tree number color schemes corresponding to different proportions were generated, including seven color schemes of group G1, nine color schemes of group G2 and group G3, and 27 color schemes of group G4 (Figure 2). The quantification standard of tree and image composition is shown in Figure 3.
Adobe Photoshop CS6 is used for color control and image composition according to a quantified color scheme, where fir is used for green plants, ginkgo for yellow plants, metasoia for red plants, and color gradient is adjusted by color saturation. In PS software, we slightly adjusted the size of the trees at random to be closer to the actual urban landscape forest. Since the number of colors in the color scheme was not uniform, we changed the color order of trees, fine-tuned the size and randomly added them after the image combination to ensure that there were 30 original images in each group. Then we invited four researchers who had been in landscape health care for at least 3 years to conduct experimental image selection. The selection criteria were that they could represent the general characteristics of urban landscape forests. It is composed of trees of a certain area, and it is possible to see the maintenance or urban pruning and urban elements of the city. It has certain color quantification characteristics and differences; The image is clearly expressed. In the end, 12 images were selected for each group, and a total of 48 images were used for the final experiment. In addition, we selected the same 12 city street images as the control group (group G5), part of which are shown in Figure 4.
A total of 100 subjects (50 male, 50 female, mean age 23.42 ± 1.03) were recruited through campus posters and online publicity. All subjects were required to meet the following criteria: no history of major physical illness or mental illness, normal vision and hearing, and visual acuity ≥0.8 with corrected or uncorrected vision. There was no major surgery or hormone drug use during the first 6 months of the study. To rule out hemispheric differences, we required that all subjects be right-handed (Neale et al., 2017). In order to ensure the accuracy of the experiment results, alcohol, vigorous exercise and medication were prohibited the day before the experiment began. We established a total of four experimental groups and one control group based on color quantization. The color quantization classification group (G1, G2, G3, G4) in this study was named G1, G2, G3, G4, respectively, and the control group was named G5.Each experimental group was randomly assigned 20 experimenters (10 male, 10 female) on the premise of gender balance, and each subject only participated in one of the groups. Before the experiment began, the research staff sent the detailed experiment process to the volunteers, each subject signed the volunteer agreement before the experiment began and expressed their consent to participate in the experiment.
The experiment was carried out in a university laboratory in May 2024. During the experiment, the room temperature in the laboratory was kept at 26 degrees Celsius, the humidity was maintained at 20–30%, and the light intensity was 500 lux. The experimental process is divided into two stages: pre-test and post-test. Volunteers will take a 5-min rest after arriving at the experimental classroom in the designated time period, and then start the pre-test after the rest. The main purpose of the pre-test is to increase pressure and distract attention. Volunteers will take a 10-min TMT connection test and English writing test, during which brain wave recording will be performed, and brain wave recording will be stopped after the pre-test is completed. After that, the volunteers were asked to watch the simulated images for 7 min and record their brainwaves. After the experiments were completed, the brainwave instrument was removed. Figure 5 shows the steps of the experiment. The study was reviewed and approved by the ethics committee of the university College in the process of program formulation and implementation.(Ethics Committee of the School of Arts, Sichuan Tourism University), the entire experimental process is in line with the norms and standards of the Helsinki Declaration.
The mobile multi-channel Bitbrain wearable EEG system is used for EEG data monitoring. This instrument is a Semi-Dry EEG semi-dry electrode EEG system, which has the characteristics of lightweight and stable contact with the sensor and better data signal acquisition. The instrument does not require the practical advantage of using a conductive gel and is considered suitable for a large number of non-clinical participant studies (Wu et al., 2023). According to the channels and covered brain regions used in the present study, 14 electrodes (O1, O2, AF3, AF4, FC5, FC6, F3, F4, F7, F8, T7, T8, P7, P8) and 2 reference channels were selected for CMS/DRL noise reduction configuration (P3, P4 position), and a total of 16 channels were monitored. EEG sampling frequency is 256HZ-1000 Hz/channel, resolution is 24Bits, and includes 16Bit inertial motion Unit (IMU) (9-axis sensor) in addition to 16 EEG channels.
Depending on the frequency of brain waves, two characteristic parameters assessed by specific stimuli are widely used, one is the alpha wave that occurs during relaxed consciousness (8–12 Hz), and the other is the beta wave that occurs during active and focused thinking and thinking (12–30 Hz) (Zangeneh Soroush et al., 2017; Sanei and Chambers, 2013). These EEG indicators, together with certain channels, can be used as biomarkers to analyze relaxation arousal responses in real space, such as the RAB: α/β ratio indicator and the brain wave channels F4 and F3 (Kim et al., 2021; Ramirez et al., 2015). In this study, α wave was used as the stress index. The higher the α wave level was, the deeper relaxation and satisfaction were represented. The β/α index could also reflect the nerve pressure, and the higher the index was, the greater the pressure of the subjects (Lin et al., 2023).
Using β wave, θ/β for attention indicators reference. The higher the beta wave level, the higher the positive thinking ability; θ/β also reflects neural attention, with lower θ/β values indicating greater concentration (Lin et al., 2023; Wu et al., 2023).
The eeglab2021 toolkit based on matlab was used to pre-process the EEG data, which was manually interpolated and eliminated by 1–40 Hz band-pass filtering, and the whole brain average was used as the data re-reference. Welch method was used to perform power spectrum analysis on the de-noised data. The data was divided into small segments according to 2 s and the average power spectrum was calculated after power spectrum analysis for each segment. The calculated power spectrum was divided into alpha (8–12 Hz) and beta (12–30 Hz) frequency bands. The topographic maps of power spectrum under different conditions are obtained by calculating the mean value in each frequency band. Data analysis using SPSS 2022. On the premise of normal distribution and homogeneity of variance, the paired sample t-test was used to compare whether there were significant differences between the pre and post-tests of viewing different groups of pictures. If not, use non-parametric test.
According to the pairwise sample T-test of α wave test values before and after comparison, it can be seen from Figure 6a that α wave levels of all groups decreased to different degrees after observation, among which G1 group and G3 group significantly decreased (p < 0.05), and the power spectrum topographic maps of the two groups were shown in Figure 6b. G1 group was 2.41 ± 3.26 before viewing, 19.21 ± 1.18 after viewing, G3 group was 16.90 ± 2.32 before viewing, 13.22 ± 3.09 after viewing. G2 group (Pre-test: 23.94 ± 3.50; Post-test: 16.90 ± 2.32), G4 group (Pre-test: 10.06 ± 3.65; Post-test: 9.83 ± 3.73), G5 group (Pre-test: 16.63 ± 3.97; Post-test: 15.52 ± 4.41) There was no significant change in α wave test values before and after viewing, and their p-values were 0.208, 0.068, 0.062, respectively. As can be seen from Table 1, there were significant differences in β/α indexes in G3 group (Pre-test: −0.49 ± 1.24; Post-test: 0.59 ± 0.26, p = 0.042), G1, G2, G4, G5 were not significantly different (p > 0.05).
Figure 6. (a) Five groups of α wave (8–12 Hz) pre-test and post-test paired sample T-test. (b) Pre-measured and post-measured alpha wave (8–12 Hz) EEG topographic maps of G1 and G2 groups [Spatial distribution of power along the scalp in the alpha (8–12 Hz) band].
According to the paired T-test of 16 EEG channels measured before and after α waves (Figure 7), there were no significant differences before and after tests in group G4, while significant or extremely significant differences existed in groups G1, G2, G3 and G5 in channels Fpz, F3, F4, C3, Cz, P4, P8, O1 and O2 before and after tests. There were significant differences in the three channels: Cz (Pre-test: 23.78 ± 16.03; Post-test: 16.03 ± 2.57; p = 0.048), O1 (Pre-test: 23.28 ± 3.16; Post-test: 17.12 ± 1.60; p = 0.049), O2 (Pre-test: 25.05 ± 3.17; Post-test: 19.13 ± 0.66; p = 0.039). G2 group showed significant difference on channel O1, with the pre-test value of 23.57 ± 3.73 and the post-test value of 16.38 ± 1.43.G3 group showed significant differences in Fpz (p = 0.044), F3 (p = 0.030), F4 (p = 0.046), P4 (p = 0.033), O2 (p = 0.040) channels, and extremely significant differences in C3 channels (Pre-test: 17.90 ± 1.80; Post-test: 12.66 ± 3.57; p = 0.004). Group G5 was measured in Cz (Pre-test: 18.60 ± 5.14; Post-test: 15.20 ± 5.30; p = 0.047), P8 (Pre-test: 17.93 ± 4.25; Post-test: 15.92 ± 4.27; p = 0.047) showed significant difference.
Figure 7. Sixteen EEG channels in Bitbrain were paired with a α-wave (8–12 Hz) pre-test and a post-test sample t-test.
According to Figure 8a, it can be seen that the β waves of G1, G2 and G3 groups before and after image stimulation showed extremely significant (p < 0.01) and significant (p < 0.05) changes, while there was no significant difference between G4 and G5 groups (p > 0.05). In G1 group, the values were 18.33 ± 1.98 before viewing and 24.31 ± 2.89 after viewing, p = 0.005, and the difference was extremely significant. In G2 group, the values were 19.34 ± 0.37 before viewing and 23.93 ± 3.91 after viewing, p = 0.047; in G3 group, the values were 11.80 ± 3.91 before viewing and 15.37 ± 3.09 after viewing, p = 0.046, with significant differences. The power spectrum topographic map of group G1, G2 and G3 is shown in Figure 9. As can be seen from Figure 8b group G1 (Pre-test: 1.64 ± 0.61; Post-test: 1.04 ± 0.009; p = 0.015) and G3 group (Pre-test: 0.80 ± 0.14; Post-test: 0.65 ± 0.14; p = 0.046) showed significant difference in t test of θ/β index (p < 0.05), G2 group, G4 group, G5 group had no significant difference (p > 0.05).
Figure 8. (a) Five groups of β wave (12-30 Hz) pre-test and post-test paired sample T-test (b) θ/β pre-test and post-test paired sample T-test.
Figure 9. Pre-measured and post-measured β-wave (12–30 Hz) EEG topographic maps of G1, G2 and G3 groups [The spatial distribution of power in the beta (12–30 Hz) band along the scalp].
According to the paired sample T-test of the 16 EEG channels shown in Figure 10, there were no significant differences in the 16 channels of group G5, while there were significant or extremely significant differences in the 8 channels of Fpz, F3, F4, F8, C3, P4, O1 and O2 in groups G1, G2, G3 and G4.Among them, G1 group showed a significant difference on Fpz channel, 19.09 ± 1.53 before viewing, 23.64 ± 2.31 after viewing, p = 0.008, F8 (Pre-test: 18.72 ± 1.97; Post-test: 24.48 ± 2.90), P4 (Pre-test: 18.44 ± 1.86; Post-test: 24.49 ± 2.87), O1 (Pre-test: 16.16 ± 2.17; Post-test: 23.22 ± 2.85), O2 (Pre-test: 18.11 ± 1.55; Post-test: 25.16 ± 2.86) showed significant difference on the channel, p-values were 0.048, 0.046, 0.037, 0.047, respectively. G2 group showed significant difference in O1 channel before and after observation, which was 16.59 ± 1.49 before observation and 23.65 ± 2.85 after observation, p = 0.047. G3 group showed significant difference in F3, F4 and C3 channels, with p-values of 0.035, 0.039 and 0.043, respectively. G4 group showed significant difference in F8, 8.60 ± 4.58 before viewing and 9.26 ± 4.43 after viewing, p = 0.046.
Figure 10. Sixteen EEG channels in Bitbrain were paired with a pre-test and a post-test sample t at β waves (12–30 Hz).
In this study, four color-quantified urban landscape forests and one set of urban environments were used to further confirm the more quantitative feedback of green space on EEG indicators of stress relief. After the short duration test in this study, α waves were decreased to different degrees, and the G1 and G3 groups were significantly decreased (p < 0.05). Color plays a major role in stimulating alpha waves (Wang et al., 2021), and G1 group has different gradient groups of all green (G:100%, R:0%, Y:0%), which is different from previous studies on the correlation between relaxation therapy and alpha waves in natural landscapes (Wang et al., 2021). Combined with the performance of other indicators in G1 group in this study, absolute alpha waves decreased significantly. Absolute beta waves increased significantly, which means that as alpha waves representing relaxed consciousness decreased, beta waves representing high arousal, emotional anxiety, anger, tension, and excitement (Lin et al., 2023) increased. Most of the previous studies used a relatively simple dichotomy to compare urban environment with green environment. In such experimental environment, exposure to nature increases positive emotion, which mediates the increase of natural connections in similar states (Korpela et al., 2017), thus triggering the driving force of the restoration effect of natural environment and the potential interaction and cognitive benefits. The G3 group (G:66.66%, R:0%, Y:33.33%) also showed a significant decrease in alpha waves and a significant increase in the β/α ratio, with higher β/α power indicating higher stress levels (Lin et al., 2023), indicating that the G3 experimental setup effectively reduced the stress level. Similar to the G1 group, our study showed a significant increase in absolute beta waves, golden-yellow plants can induce a feeling of warmth and brightness in space (Guo et al., 2020), and some may contain non-green leaves (Kexiu et al., 2021) presented seasonal characteristics such as ginkgo biloba and sophobia may have a neural relaxation feedback with “green.” This suggests that the yellow and green color changes used in the experiment caused a certain level of arousal. This suggests that the yellow and green color changes used in the experiment caused a certain level of arousal.
In terms of the 16 channels of absolute alpha wave, the green used by group G1 in the experiment was graded and matched according to the three elements of color, and the green characteristics were more obvious and diverse. These different shades of green may affect the reduction of pressure, and show a very significant increase in high arousal. We noticed that G1 group had significant differences in occipital channel 2 where O1 and O2 were located, which was similar to Matlovic et al. (2016) who found that alpha waves were most visible in parietal and occipital lobes. Previous studies have found that the activation of the frontal and parietal lobes of the brain when measuring the brain’s response to space (Kim et al., 2021) can explain the effectiveness of G3 group stimulation in the experiment and the activation of the frontal cortex during highly concentrated mental activities. In different groups of urban landscape forests quantified with different colors, the decrease in α wave and β/α ratio indicated a decrease in relaxation and physiological arousal (Luo et al., 2023). Despite overwhelming evidence that plants are good for stress recovery, almost all of these studies put participants in favorable recovery comparison experiments. The color limitation of urban landscape forests caused by resource imbalance should also be taken into account in the experimental setting. The charm of the forest lies in the unpredictability of the experience, especially the different landscape elements presented in the changing seasons (Wu et al., 2023), and the restoration benefits of the different visual effects presented by these plant landscapes should be widely explored.
Exposure to green environments can improve and enhance people’s ability to concentrate (Jiang et al., 2015). In G1 group, β wave increased significantly after image stimulation (Pre-test: 18.33 ± 1.98; Post-test: 24.31 ± 2.89; p = 0.005). There were significant differences in the G2 group (Pre-test: 19.34 ± 0.37, Post-test: 23.93 ± 3.91) and the G3 group (Pre-test: 0.80 ± 0.14; Post-test: 0.65 ± 0.14; p = 0.046). This indicates that the urban landscape forest with a single green color and two color combinations has better feedback in the recovery and improvement of attention. Studies have shown that the greater the visual stimulation of a landscape, the absolute beta wave increases significantly, causing anxiety or alertness (Wang and Xu, 2021). The ability to concentrate is positively correlated with the degree of preference for natural environment (Jiang et al., 2015), and the degree of preference for red and yellow leaf color is higher (Wang et al., 2020). Subjects induced stress and distraction at a set time, affected visual perception through color matching in four groups of visual stimuli, and the landscape forest composed of one color (green) and two colors (green and yellow, green and red) showed a significant increase in beta waves. This is consistent with the conclusion of this study. The three-color G4 group did not show an increase in absolute beta waves, and the attention recovery theory explains the restorative effect of natural environments through involuntary or effortless attention participation (Poulsen et al., 2022), and colorful landscapes better stimulate visual function and attract the attention of observers (Elsadek and Fujii, 2014). As the color increases, the complexity of the image also increases, which undoubtedly weakens the ease of visual features in the natural environment, thus reducing the recovery benefit. θ/β power was negatively correlated with attention control. The higher the θ/β power, the lower the attention control (Lin et al., 2023). Increasing plant area or plant layer richness can improve attention recovery (Zhu et al., 2023), and the power of G1 and G3 groups decreased significantly after viewing images, indicating that attention control was significantly increased, which was consistent with the conclusion of absolute β value.
In the study of the differences of 16 EEG channels, except group G5, the other groups had significant differences in different degrees of channels. According to the channels corresponding to brain regions, G1 group had the most significant differences in the number of channels. Besides the midfrontal line, the corresponding F8 channel in the prefrontal lobe, P4 channel in the parietal lobe, O1 and O2 channel in the occipital lobe were significantly different. Activity in the parietal lobe, which is associated with goal orientation, varied with behavioral priorities during viewing natural scenery, and the left parietal lobe and the right frontal lobe were activated when measuring the brain’s response to space (Kim et al., 2021; Baciu et al., 1999). The channel differences in the occipital lobe of the diachronic test in the study indicated that the information specificity of the color features in the experimental stimulus induced the differences in the value of the pre and post measurement indicators, and a single color change may provide a more consistent and predictable arousal response, including the significant difference in the O2 channel in the G2 group. The color characteristics used by G2 group are green and red. Previous studies have shown that red plants can induce high arousal (Elliot, 2019). Bright colors can cause strong visual stimulation, which may not be conducive to the brain’s recovery from anxiety and relaxation, but is conducive to excitement or arousal (Hao et al., 2024). In this study, the brain waves extracted from the experimental filtering and processing signals can represent certain emotional states, but it does not mean that emotions and brain waves are completely consistent (Kim et al., 2021). For example, in the G3 group with high arousal and attention recovery, no significant differences were found in the occitalo-related channels (O1, O2 channels) responsible for visual processing, and only in the frontal lobe F3, O2 channels. There was significant difference between F4 channel and central C3 channel. The frontal lobe is usually associated with higher cognitive functions, including learning, decision making, and control of autonomous movement (Wu et al., 2023), suggesting that the color features of the C3 group are related to the frontal cortex to some extent, and are consistent with previous studies showing that beta waves are most prominent in the frontal cortex during high mental or focused activities (Matlovic et al., 2016).
The color optimization of urban landscape forest should be regarded as one of the positive health resources. Detailed landscape color research provides the basis for this study. Because plant materials are more accessible and seasonal than other landscapes, potential targets for intervention in improving health or wellbeing require further investigation. The results showed that the feedback stress reduction potential of landscape forest with different color characteristics was inconsistent with previous studies, and had high recovery in the EEG feedback of attention and arousal. The monochrome group G1 had the most significant effect on attention and arousal recovery, the two-color group G2 and G3 were significant, and the three colors G4 group had no significant difference. A large number of studies have shown the high recoverability of green plants. Due to the richness of colors, the same hue may show different colors due to different brightness and saturation. Although only green is used in the monochrome in this study, the shade and hue are different. In the initial stage of landscape forest planning, different plant materials can be used to trigger the awakening effect, and the sense of wildness and friendliness can be evoked through the change of the same color system (Zhang et al., 2013). Adjustments including some stand planting plans (such as tree mix) produce periodic color changes of the same tree species. Secondly, we suggest controlling the species of trees in urban landscape forests to control the number of colors. The results showed that the experimental group composed of three colors had no significant changes in each EEG index. When the combination and layout of different elements formed different landscape environments, the plant diversity represented by color richness may affect perceptual recovery. When the space area, space coverage area, space closure rate and aspect ratio (Zhao et al., 2023) are consistent, the use of one or two plant color combinations can achieve better restorative health benefits.
Our study included the following limitations. First, this study used electroencephalography (EEG) to quantify the relevant indicators after viewing different urban landscape forests. Healthy brains show high variability, and the sensitivity of dynamic changes in neural activity may be ignored when the variability of brain signals is measured by EEG at the global level (Gibson et al., 2022). Although relevant preprocessing was performed in the study to reduce data redundancy, a comprehensive assessment combined with other physiological or psychological measures can provide more accurate conclusions. Second, this study only discussed the variable of color characteristics of urban landscape forest. The composition of urban forest environment under artificial influence may be more complex, and more detailed location, density, arrangement and combination, and openness should be taken into consideration in future comprehensive experiments. Third, field response collection allows real-time assessment of “stimulus-emotional response,” which reflects the natural participation of daily realization, rather than the use of hypotheses (Tomasso et al., 2023), which is obviously missing in this experiment, the study results are also limited by the participant group and the number of experimenters. In the future, sample diversity should be compared with field experiments to expand the applicability of the results of this study.
This study is helpful to understand the EEG feedback of urban landscape forest to stress—attention under different color characteristics. Our study of the potential benefits of image stimulation based on different color quantizations for EEG outcomes. Our results show that different color groups, especially landscape forests with a single green change, exhibit higher EEG arousal and attention recovery responses, followed by yellow and green, and the color combination of red and green has a high response to α waves, β/α ratio. The health benefits of urban landscape forests should not be a single. This study provides a conclusion on the diversity of wellbeing based on EEG feedback, and the combination of diverse physiological feedback is needed to draw further reliable conclusions for color quantification of urban green space, so as to provide a basic and scientific basis for multifunctional urban forest management and planning.
The raw data supporting the conclusions of this article will be made available by the authors, without undue reservation.
Written informed consent was obtained from the individual(s) for the publication of any potentially identifiable images or data included in this article.
LW: Conceptualization, Data curation, Formal analysis, Project administration, Software, Writing – original draft, Writing – review & editing. YZ: Data curation, Methodology, Software, Writing – original draft. MM: Data curation, Methodology, Software, Supervision, Writing – original draft. CL: Formal analysis, Methodology, Supervision, Writing – original draft. QZ: Conceptualization, Data curation, Software, Writing – original draft. WZ: Conceptualization, Software, Writing – review & editing. XS: Conceptualization, Software, Writing – review & editing. JL: Conceptualization, Software, Writing – review & editing. JM: Investigation, Software, Writing – review & editing. YL: Conceptualization, Funding acquisition, Resources, Visualization, Writing – review & editing. QD: Conceptualization, Software, Validation, Writing – review & editing.
The author(s) declare that financial support was received for the research, authorship, and/or publication of this article. This research received funding Sichuan Tourism University University-level scientific research project: research on health benefits and mechanism under the coupling effect of different spatial characteristics of urban landscape forests (2023SCTUBSZK14), Sichuan Tourism University University-level scientific research project: research on the impact and mechanism of urban landscape forests on the physical and mental health of sub-healthy people (18/794002) and Sichuan Landscape and Recreation Research Center: Research on the Development of Regional Characteristic Leisure Agriculture Landscapes in the Context of Industrial Integration: A Case Study of Chengdu City (JGYQ2024015).
The authors declare that the research was conducted in the absence of any commercial or financial relationships that could be construed as a potential conflict of interest.
All claims expressed in this article are solely those of the authors and do not necessarily represent those of their affiliated organizations, or those of the publisher, the editors and the reviewers. Any product that may be evaluated in this article, or claim that may be made by its manufacturer, is not guaranteed or endorsed by the publisher.
Baciu, M., Koenig, O., Vernier, M. P., Bedoin, N., Rubin, C., and Segebarth, C. (1999). Categorical and coordinate spatial relations: fMRI evidence for hemispheric specialization. Neuro Rep. Commun. Neurosci. Res. 10, 1373–1378.
Butt, S., Smith, S. M., Moola, F., and Conway, T. M. (2021). The relationship between knowledge and community engagement in local urban forest governance: a case study examining the role of resident association members in Mississauga, Canada. Urban Forestr. Urban Greening 60:127054. doi: 10.1016/j.ufug.2021.127054
Deng, L., Li, X., Luo, H., Fu, E. K., Ma, J., Sun, L. X., et al. (2020). Empirical study of landscape types, landscape elements and landscape components of the urban park promoting physiological and psychological restoration. Urban For. Urban Green 48:126488. doi: 10.1016/j.ufug.2019.126488
Elliot, A. J. (2019). A historically based review of empirical work on color and psychological functioning: content, methods, and recommendations for future research. Rev. Gen. Psychol. 23, 177–200. doi: 10.1037/gpr0000170
Elsadek, M., and Fujii, E. (2014). People’s psycho-physiological responses to plant scape colors stimuli: a pilot study. Int. J. Psychol. Behav. Sci 4, 70–78. doi: 10.5923/j.ijpbs.20140402.02
Gibson, E., Lobaugh, N. J., Joordens, S., and McIntosh, A. R. (2022). EEG variability: task-driven or subject-driven signal of interest? NeuroImage 252:119034. doi: 10.1016/j.neuroimage.2022.119034
Guo, L. N., Zhao, R. L., Ren, A. H., Niu, L. X., and Zhang, Y. L. (2020). Stress recovery of campus street trees as visual stimuli on graduate students in autumn. Int. J. Environ. Res. Public Health 17:148. doi: 10.3390/ijerph17010148
Hao, N., Li, X., Han, D., and Nie, W. (2024). Quantifying the impact of street greening during full-leaf seasons on emotional perception: guidelines for resident well-being. Forests 15:119. doi: 10.3390/f15010119
Jang, H. S., Kim, J., Kim, K. S., and Pak, C. H. (2014). Human brain activity and emotional responses to plant color stimuli. Color Res. Appl. 39, 307–316. doi: 10.1002/col.21788
Jiang, B., Larsen, L., Deal, B., and Sullivan, W. C. (2015). A dose–response curve describing the relationship between tree cover density and landscape preference. Landsc. Urban Plan. 139, 16–25. doi: 10.1016/j.landurbplan.2015.02.018
Kexiu, L., Elsadek, M., Liu, B., and Fujii, E. (2021). Foliage colors improve relaxation and emotional status of university students from different countries. Heliyon 7:e06131. doi: 10.1016/j.heliyon.2021.e06131
Kim, S., Park, H., and Choo, S. (2021). Effects of changes to architectural elements on human relaxation-arousal responses: based on VR and EEG. Int. J. Environ. Res. Public Health 18:4305. doi: 10.3390/ijerph18084305
Korpela, K., Savonen, E. M., Anttila, S., Pasanen, T., and Ratcliffe, E. (2017). Enhancing wellbeing with psychological tasks along forest trails. Urban For. Urban Green. 26, 25–30. doi: 10.1016/j.ufug.2017.06.004
Lin, W., Chen, Q., Jiang, M., Tao, J., Liu, Z., Zhang, X., et al. (2020). Sitting or walking? Analyzing the neural emotional indicators of urban green space behavior with mobile EEG. J. Urban Health 97, 191–203. doi: 10.1007/s11524-019-00407-8
Lin, W., Zeng, C., and Bao, Z. (2023). The therapeutic look up: stress reduction and attention restoration vary according to the sky-leaf-trunk (SLT) ratio in canopy landscapes. Landsc. Urban Plan. 234:104730. doi: 10.1016/j.landurbplan.2023.104730
Luo, L., Yu, P., and Jiang, B. (2023). Differentiating mental health promotion effects of various bluespaces: an electroencephalography study. J. Environ. Psychol. 88:102010. doi: 10.1016/j.jenvp.2023.102010
Martens, D., Gutscher, H., and Bauer, N. (2011). Walking in “wild” and “tended” urban forests: the impact on psychological well-being. J. Environ. Psychol. 31, 36–44. doi: 10.1016/j.jenvp.2010.11.001
Matlovic, T., Gaspar, P., Moro, R., Simko, J., and Bielikova, M. (2016). “Emotions detection using facial expressions recognition and EEG,” in 2016 11th International Workshop on Semantic and Social Media Adaptation and Personalization (SMAP). Piscataway, NJ: IEEE, 18–23.
Neale, C., Aspinall, P., Roe, J., Tilley, S., Mavros, P., Cinderby, S., et al. (2017). The aging urban brain: analyzing outdoor physical activity using the emotiv affectiv suite in older people. J. Urban Health 94, 869–880. doi: 10.1007/s11524-017-0191-9
Neale, C., Griffiths, A., Chalmin-Pui, L. S., Mendu, S., Boukhechba, M., and Roe, J. (2021). Color aesthetics: a transatlantic comparison of psychological and physiological impacts of warm and cool colors in garden landscapes. Wellbeing Space Soc. 2:100038. doi: 10.1016/j.wss.2021.100038
Norwood, M. F., Lakhani, A., Fullagar, S., Maujean, A., Downes, M., Byrne, J., et al. (2019). A narrative and systematic review of the behavioural, cognitive and emotional effects of passive nature exposure on young people: evidence for prescribing change. Landsc. Urban Plan. 189, 71–79. doi: 10.1016/j.landurbplan.2019.04.007
Paraskevopoulou, A. T., Kamperi, E., Demiris, N., Economou, M., Theleritis, C., Kitsonas, M., et al. (2018). The impact of seasonal colour change in planting on patients with psychotic disorders using biosensors. Urban For. Urban Green. 36, 50–56. doi: 10.1016/j.ufug.2018.09.006
Poulsen, M. N., Nordberg, C. M., Fiedler, A., DeWalle, J., Mercer, D., and Schwartz, B. S. (2022). Factors associated with visiting freshwater blue space: the role of restoration and relations with mental health and well-being. Landsc. Urban Plan. 217:104282. doi: 10.1016/j.landurbplan.2021.104282
Ramirez, R., Palencia-Lefler, M., Giraldo, S., and Vamvakousis, Z. (2015). Musical neurofeedback for treating depression in elderly people. Front. Neurosci. 9:150420. doi: 10.3389/fnins.2015.00354
Shoari, N., Ezzati, M., Doyle, Y. G., Wolfe, I., Brauer, M., Bennett, J., et al. (2021). Nowhere to play: available open and green space in greater London schools. J. Urban Health 98, 375–384. doi: 10.1007/s11524-021-00527-0
Song, C., Ikei, H., and Miyazaki, Y. (2021). Effects of forest-derived visual, auditory, and combined stimuli. Urban For. Urban Green. 64:127253. doi: 10.1016/j.ufug.2021.127253
Tomasso, L. P., Spengler, J. D., Catalano, P. J., Chen, J. T., and Laurent, J. G. C. (2023). In situ psycho-cognitive assessments support self-determined urban green exercise time. Urban For. Urban Green. 86:128005. doi: 10.1016/j.ufug.2023.128005
Tyrväinen, L., Ojala, A., Korpela, K., Lanki, T., Tsunetsugu, Y., and Kagawa, T. (2014). The influence of urban green environments on stress relief measures: a field experiment. J. Environ. Psychol. 38, 1–9. doi: 10.1016/j.jenvp.2013.12.005
Wang, Z., Li, M., Zhang, X., and Song, L. (2020). Modeling the scenic beauty of autumnal tree color at the landscape scale: a case study of Purple Mountain, Nanjing, China. Urban Forestr. Urban Green. 47:126526. doi: 10.1016/j.ufug.2019.126526
Wang, Y., Wang, S., and Xu, M. (2021). The function of color and structure based on EEG features in landscape recognition. Int. J. Environ. Res. Public Health 18:4866. doi: 10.3390/ijerph18094866
Wang, Y., and Xu, M. (2021). Electroencephalogram application for the analysis of stress relief in the seasonal landscape. Int. J. Environ. Res. Public Health 18:8522. doi: 10.3390/ijerph18168522
Wang, R., Zhao, J., Meitner, M. J., Hu, Y., and Xu, X. (2019). Characteristics of urban green spaces in relation to aesthetic preference and stress recovery. Urban For. Urban Green. 41, 6–13. doi: 10.1016/j.ufug.2019.03.005
Wolch, J. R., Byrne, J., and Newell, J. P. (2014). Urban green space, public health, and environmental justice: the challenge of making cities ‘just green enough’. Landsc. Urban Plan. 125, 234–244. doi: 10.1016/j.landurbplan.2014.01.017
Wu, L., Luo, S., Li, D., Chen, Q., Li, J., and Wen, J. (2023). Effects of deciduous forests on adolescent emotional health in urban areas: an example from the autumn Ginkgo Forest in Chengdu. Forests 14:1099. doi: 10.3390/f14061099
Zangeneh Soroush, M., Maghooli, K., Kamaledin Setarehdan, S., and Nasrabadi, A. M. (2017). A review on EEG signals based emotion recognition. Int. Clin. Neurosci. J. 4, 118–129. doi: 10.15171/icnj.2017.01
Zhang, H., Chen, B., Sun, Z., and Bao, Z. (2013). Landscape perception and recreation needs in urban green space in Fuyang, Hangzhou, China. Urban Forestr. Urban Green. 12, 44–52. doi: 10.1016/j.ufug.2012.11.001
Zhao, W., Li, X., Zhu, X., Ye, H., and Xu, H. (2023). Restorative properties of green sheltered spaces and their morphological characteristics in urban parks. Urban For. Urban Green. 86:127986. doi: 10.1016/j.ufug.2023.127986
Zhu, H., Yang, F., Bao, Z., and Nan, X. (2021). A study on the impact of visible green index and vegetation structures on brain wave change in residential landscape. Urban For. Urban Green. 64:127299. doi: 10.1016/j.ufug.2021.127299
Keywords: urban landscape forest, brain electricity, color quantization, stress, attention
Citation: Wu L, Zhang Y, Mao M, Li C, Zhang Q, Zhao W, Sui X, Li J, Ma J, Li Y and Dong Q (2024) Evergreen or seasonal? Quantitative research on the color of urban scenic forests based on stress—attention electroencephalogram feedback. Front. For. Glob. Change. 7:1495806. doi: 10.3389/ffgc.2024.1495806
Received: 16 September 2024; Accepted: 05 November 2024;
Published: 20 November 2024.
Edited by:
Mohammad Imam Hasan Reza, Centre for Environment and Sustainability, Presidency Education, BangladeshReviewed by:
Naseem Akhtar Qureshi, Al-Falah University, IndiaCopyright © 2024 Wu, Zhang, Mao, Li, Zhang, Zhao, Sui, Li, Ma, Li and Dong. This is an open-access article distributed under the terms of the Creative Commons Attribution License (CC BY). The use, distribution or reproduction in other forums is permitted, provided the original author(s) and the copyright owner(s) are credited and that the original publication in this journal is cited, in accordance with accepted academic practice. No use, distribution or reproduction is permitted which does not comply with these terms.
*Correspondence: Meiqin Mao, MDAwMTU5N0BzY3R1LmVkdS5jbg==
Disclaimer: All claims expressed in this article are solely those of the authors and do not necessarily represent those of their affiliated organizations, or those of the publisher, the editors and the reviewers. Any product that may be evaluated in this article or claim that may be made by its manufacturer is not guaranteed or endorsed by the publisher.
Research integrity at Frontiers
Learn more about the work of our research integrity team to safeguard the quality of each article we publish.