- Heilongjiang Province Key Laboratory of Geographical Environment Monitoring and Spatial Information Service in Cold Regions, Harbin Normal University, Harbin, China
Investigating the long-term dynamics in the canopy proves to be difficult due to the short observational records of the normalized difference vegetation index (NDVI). To explore the linkage between tree growth, NDVI dynamics and large-scale atmospheric circulation in the Greater Khingan Mountain, Northeast China, we established a chronology of Larix gmelinii tree ring width at three elevations (870–920 m, 1,100–1,150 m and 1,270–1,320 m) in the northern foothills of the mountain range. We then calculated the correlations between the tree ring chronologies and NDVI and climate factors, and reconstructed the NDVI time series from June to September 1759–2021 in the region based on the middle-elevation tree ring chronology. The results identify the positive effect of temperature (r = 0.56, p < 0.01) and the negative effect of precipitation (r = −0.44, p < 0.01) in the growing season as the main influencing factors of NDVI for the study period (1981–2019). The 11-year moving average of the reconstructed NDVI series reveals two periods of high canopy vigor (1898–1926 and 2009–2013) and three periods of low canopy vigor (1860–1962, 1882–1888 and 1968–1977) in the last 263 years. These periods correspond to drought events recorded in the historical literature. Wavelet analysis shows that the reconstructed sequences exhibited 11–13, 23–25, and 39–42 years period variations. Integrating this with spatial correlation analysis reveals that tree growth in the Mangui region was impacted by the combined effect of the North Pacific Decadal Oscillation and the North Atlantic Multidecadal Oscillation. The results of this paper provide a reference for the study of vegetation change patterns in the northern foothills of the Greater Khingan Mountains.
1 Introduction
The United Nations Intergovernmental Panel on Climate Change (IPCC) Sixth Climate Assessment Report states that carbon emissions are still on the rise and the global warming trend continues (Piao and Wang, 2023). As a climate change-sensitive area, China’s climate warming has led to an increase in the level of climate risk in China, and the increase in extreme weather and climate events (National Climate Center of China Meteorological Administration, 2022) has seriously harmed the ecosystem. Therefore, in order to mitigate the adverse effects of climate change, the Chinese government has made a major decision to strive to achieve “carbon neutrality” by 2060 (Piao et al., 2022). The carbon sink function in the ecosystem plays an important role in the realization of carbon neutrality. As the main body of carbon sequestration in the terrestrial ecosystem, the forest ecosystem plays a key role in mitigating rising atmospheric CO2 concentrations and global warming (Pan et al., 2011). Moreover, climate change has also changed the structure of the forest, and the response of the forest to the climate is not only affected by internal factors in the forest (e.g., stand structure and species abundance), but is also closely related to the external environment (e.g., site characteristics and soil type) (Seidl et al., 2017). Therefore, the response of forest ecosystems to climate change is a focal concern for the scientific community. Remote sensing technology is widely used in forest-related research. For example, based the on normalized difference vegetation index (NDVI) and robust satellite techniques (RST), Filizzola et al. (2022) found that drought events caused by climate change in the 2000s led to the decline and death of oak forests in southern Italy. Correa-Díaz et al. (2020) combined tree ring isotope sequences with remote sensing data to investigate the spatio-temporal variability in the response of forests to the rise in CO2. The combination of remote sensing and dendrochronology connects space and time, which is of great significance for revealing the changing trend of forest ecosystem productivity on a large spatio-temporal scale (Correa-Díaz et al., 2020). As one of the most commonly used indirect measurements of canopy photosynthesis and remote sensing indicators of forest productivity, NDVI has been proven to be related to the radial growth of trees. For example, Vicente-Serrano et al. (2016) determined a positive correlation between interannual NDVI variability and annual tree growth in most forests by comparing available tree annual rings with NDVI at the global scale. Moreover, NDVI can accurately reflect the growth status and vegetation cover of surface vegetation. Thus, it is widely used to study the response of vegetation change to climate and water environments, as well as to monitor the growth status of plants and to investigate the spatial and temporal changes of land cover (Sellers et al., 1995; Fang et al., 2004; Mao et al., 2022; Verhoeven and Dedoussi, 2022; Wei and Wan, 2022). However, the collection of NDVI observational data began in 1972 to monitoring vegetation systems in the Great Plains (Rouse et al., 1973). Such a short time period is unable to meet the needs of long-timescale studies and as a consequence, other proxy data need to be introduced.
The radial growth process of trees is influenced not only by their genetic characteristics but also by the growing environment and climate change (Fritts, 1976). Thus, a large amount of climate information in historical periods is accurately recorded in the tree rings. Compared with other natural archives, tree rings have the advantages of high resolution, accurate dating, wide distribution, easy access, and sensitivity to environmental changes. As a consequence, dendrochronology has become an important approach for the study of paleoclimatic environmental changes (Wu, 1990). Previous studies have determined a strong correlation between summer NDVI and tree ring width (Wang et al., 2017; Zhang T. W. et al., 2018; Qin et al., 2022). Therefore, the annual tree ring is currently the most common proxy adopted for the study of long-timescale NDVI trends (Castillo et al., 2015; Thomte et al., 2022). For example, in a study on Larix chinensis Beissn. in the Taibai Mountains of the Qinling Mountains, Qin et al. (2022) determined NDVI in July to have a highly significant positive correlation with the tree ring width and subsequently reconstructed the NDVI from this month using a linear model.
However, the relationship between tree rings and NDVI is very different due to the differences in local conditions. For example, Pompa-García et al. (2022) found that the relationship between the tree ring chronology of Mexican coniferous species and NDVI did not vary with altitude, however, differences were observed for latitudes changing from south to north, and the relationship gradually weakened toward the north. But Wen et al. (2022) found that the correlation between the tree ring width of Picea schrenkiana and NDVI in the Tianshan Mountains decreased with increasing altitude, with gradually decreasing trend from the west to east in longitude. Tree age that also affects the relationship between tree radial growth and canopy conditions. The photosynthetic capacity, cambium activity and sensitivity to CO2 of young trees are higher than those of old trees, while the actual vegetation greenness and NDVI of young trees tend to be higher than the observed values, and the opposite is true for old forests. This may affect the link between tree rings and NDVI (Berra et al., 2017; Correa-Díaz et al., 2019; Mašek et al., 2023).
Related studies in China began relatively late, and are mainly concentrated in the Tianshan and Qinling areas. Scholars have determined the vegetation growth dynamics in the study area to be driven and regulated by large-scale circulation. Wang et al. (2017) investigated the tree ring width and NDVI of Pinus tabuliformis in the Huanglong Mountain of the Qinling Mountains and found that the changes in NDVI from June to July were related to the sea temperature of the western Pacific and Indian Ocean. Zhang T. W. et al. (2018) reconstructed the July–October NDVI in the region using a tree ring chronology of the Alatau Mountains and determined the NDVI to be influenced by drought events and large-scale circulation over Eurasia. Large-scale circulation affects the climate in climate-sensitive areas by redistributing the energy and matter on the Earth, which subsequently changes the precipitation and temperature in the area, and ultimately has an impact on the ecosystem. However, research on the effects of large-scale circulation on the radial growth of trees and vegetation coverage in the Greater Khingan Mountains, as well as the changes in the relationship between tree ring width and NDVI across different altitudes, is still limited. There is a strong linkage between climate change, forest cover change and the hydrological processes in the region (Mao et al., 2021). In particular, hydrological processes such as precipitation, snow melt, permafrost melt, and run off have important impacts on forest recovery and ecological security in the Greater Khingan Mountains. Therefore, it is of great practical value and scientific significance to investigate the relationship between the vegetation evolution process and climate change in the region and to clarify the water vapor transport mechanism in the region (Duan et al., 2017).
In this paper, we studied the dominant tree species Larix gmelinii at three elevations in the Mangui region of the Greater Khingan Mountains. The aims of the paper were to: (1) analysis the link between larch radial growth and NDVI, as well as the effect of elevation on the correlation between them; (2) identify the climate variables [temperature, precipitation, vapor pressure deficit (VPD), and drought severity index (scPDSI)] affecting vegetation cover; and (3) reconstruct NDVI trends in the region during the historical period based on the larch tree ring width index. This work acts as a reference to understand the historical trends and patterns of forest change in the region of the Greater Khingan Mountains. The results provide a scientific basis for forest management and vegetation resource restoration.
2 Materials and methods
2.1 Overview of study area
The study area is located in Inner Mongolia at the northwest slope of the northern foot of the Greater Khingan Mountains (51°51′02– 52°30′52″N, 121°05′44″–122°47′05″E). It is a medium-low mountainous landscape with a gentle slope. The highest elevation is 1,409 m and the lowest is 509 m. Local government reported the study area belongs to the cool temperate zone and has a continental monsoon climate, with low temperatures and humidity throughout the year, a snow period of up to 160 days, an average annual temperature of −5.8°C, an extreme minimum temperature of −49.6°C, and annual precipitation of 470–850 mm that is mainly concentrated in July and August. The total forest area is approximately 3,903 km2 and the forest coverage rate is 94%. The dominant tree species in the sample plots is Larix gmelinii, with other key tree species including Betula platyphylla Suk, Pinus sylvestris var. mongolica and Picea asperata Mast. The Greater Khingan Mountains are one of the most sensitive areas in China in terms of climate vulnerability and climate change response (Zhu et al., 2021). Their forest ecosystem plays an irreplaceable role in water conservation, soil conservation and biodiversity protection, and is considered as “an important ecological barrier in the north of the motherland” (Guo and Zhang, 2013) (Figure 1).
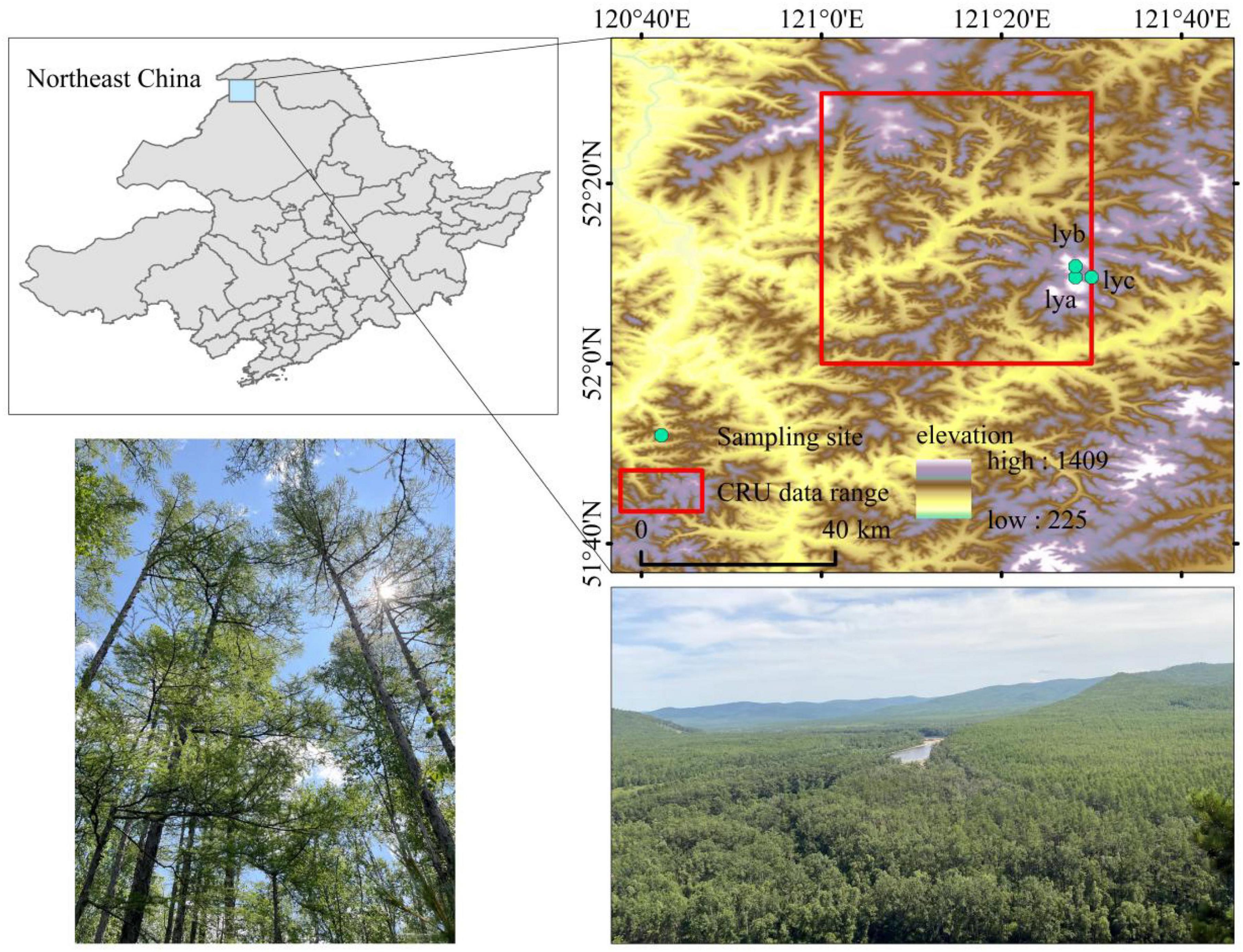
Figure 1. Map of the sampling site. lya, high elevation sampling sites. lyb, middle elevation sampling sites. lyc, low elevation sampling sites.
2.2 Data sources
The mean temperature (T), precipitation (P) and scPDSI were obtained from the Climate Research Unit (CRU TS 4.04) at the University of East Anglia. The data are from a month-by-month grid point dataset with a 0.5° × 0.5° resolution, provided by the Royal Netherlands Meteorological Institute. VPD was derived from ERA-5 reanalysis products (0.25° × 0.25°) using the Equations 1–3.
Where SVP is saturated vapors, AVP is actual vapors, Ta is air temperature, hmean is monthly average relative humidity.
The NDVI was obtained from the 0.5° × 0.5° NOAA/NCEI CDR NDVI analysis month-by-month grid point dataset provided by the National Center for Environmental Information (NCEI) for regions between 52.00°–52.50°N and 121.00°–121.50°E during the years from 1981 to 2020. Table 1 reports the details of the climate data. The study area clearly exhibits the phenomenon of simultaneous rain and heat, with wet and cool summers, and cold and dry winters. In 1981–2020, the average precipitation from May to September was 388.12 mm, accounting for 86.42% of the multi-year average annual precipitation. The multi-year average temperature and precipitation reached a maximum of 17°C and 115.98 mm in July, respectively, with a slight decrease in precipitation and a slight increase in average temperature during the growing season (June–September).
2.3 Sample collection and determination of chronology
Following the basic principles of dendrochronology (Stokes and Smiley, 1996), tree ring samples were collected at three elevations in July 2022 on the northwest slope of the Greater Khingan Mountains in the Beian Forest, Mangui Forestry Bureau. We selected trees with the following criteria: (i) low level of human intervention; (ii) visually older; and (iii) with good growth conditions for themselves and the surrounding trees. A total of 23, 24, and 22 trees were selected at high, medium, and low elevations, respectively. A total of two core samples (10 mm increment borer diameter) were sampled at chest height (1.3 m above ground) along parallel and vertical slopes. To increase the reliability of the sample, a fine core sample (5.15 mm increment borer diameter) was drilled in a random direction at the chest height of the tree. Habitat conditions were recorded for each sampling site, and a total of 191 complete sample cores were retained. Table 2 reports the sampling point information.
According to the basic procedure of tree annual ring analysis (Speer, 2010), the cores were air-dried, fixed, and sanded before being pre-treated. The annual ring width of the sample cores was measured using a LINTAB 6.0 (Rinntech, Heidelberg, Germany) annual ring width meter with an accuracy of 0.001 mm. The measured tree ring width series were cross-dated using PAST5 (Version 1.2, Knibbe, SCIEM) in order to determine whether the measurement sequence lacks annual rings or includes false rings. COFECHA (Holmes, 1983) was employed to perform quality inspection on the dating results, repeat for cross-dating and quality checks, and eliminate sample sequences with poor correlation with the main sequence. Each annual series was detrended using the “ModNegExp” method from the dplR package in R (Bunn, 2010). The detrended series were then synthesized into a standard chronology of tree ring using a double-weighted average method (Fritts, 2001).
2.4 Data processing
SPSS 25 (IBM, SPSS Inc) was employed to investigate the correlation between larch tree ring width, NDVI, and climatic factors in the Mangui region using the Pearson correlation (Mašek et al., 2023). The historical changes of NDVI in the region were reconstructed using a power function regression model, and the stability and reliability of the reconstructed equations were tested using the segmental test method (Qin et al., 2022). In order to investigate how well the reconstructed NDVI for the Mangui region represents the NDVI variation in a larger area, we employed AVHRR NDVI 0.1° × 0.1° data of the same period (1981–2020) in KNMI Climate Explorer (Trouet and Van Oldenborgh, 2013) for the spatial correlation analysis of the reconstructed series. In order to analyze the impact of large-scale atmospheric circulation on vegetation in the area, spatial correlation analysis between the reconstructed NDVI sequence and sea surface temperature (SST) and sea ice cover (SIC) was also performed in KNMI. The periodic characteristics of the reconstructed NDVI series were analyzed via wavelet analysis (Qin et al., 2022). An 11-year moving average was used to present the low-frequency information in the reconstructed series, and periods with a sliding average higher than the mean of the reconstructed NDVI series plus one standard deviation were classified as “extremely high” periods, while those with a sliding average lower than the mean of the reconstructed NDVI series minus one standard deviation were classified as “extremely low” periods.
3 Results
3.1 Larix chronological characteristics
A standard chronology of larch tree ring widths at three elevations was established, The main characteristic parameters are shown in Table 3. The standard chronology of tree ring width is shown in Figure 2. It can be seen from Table 2 that the mean sensitivity, correlation coefficients between trees, signal-to-noise ratio, and expressed population signal of high-elevation larch are the largest. The first-order serial autocorrelation value of larch at mid-altitude is the largest, and the years with sub-sample signal strength > 0.85 are also the longest. The values of mean ring width and standard deviation of larch at low elevation are the largest.
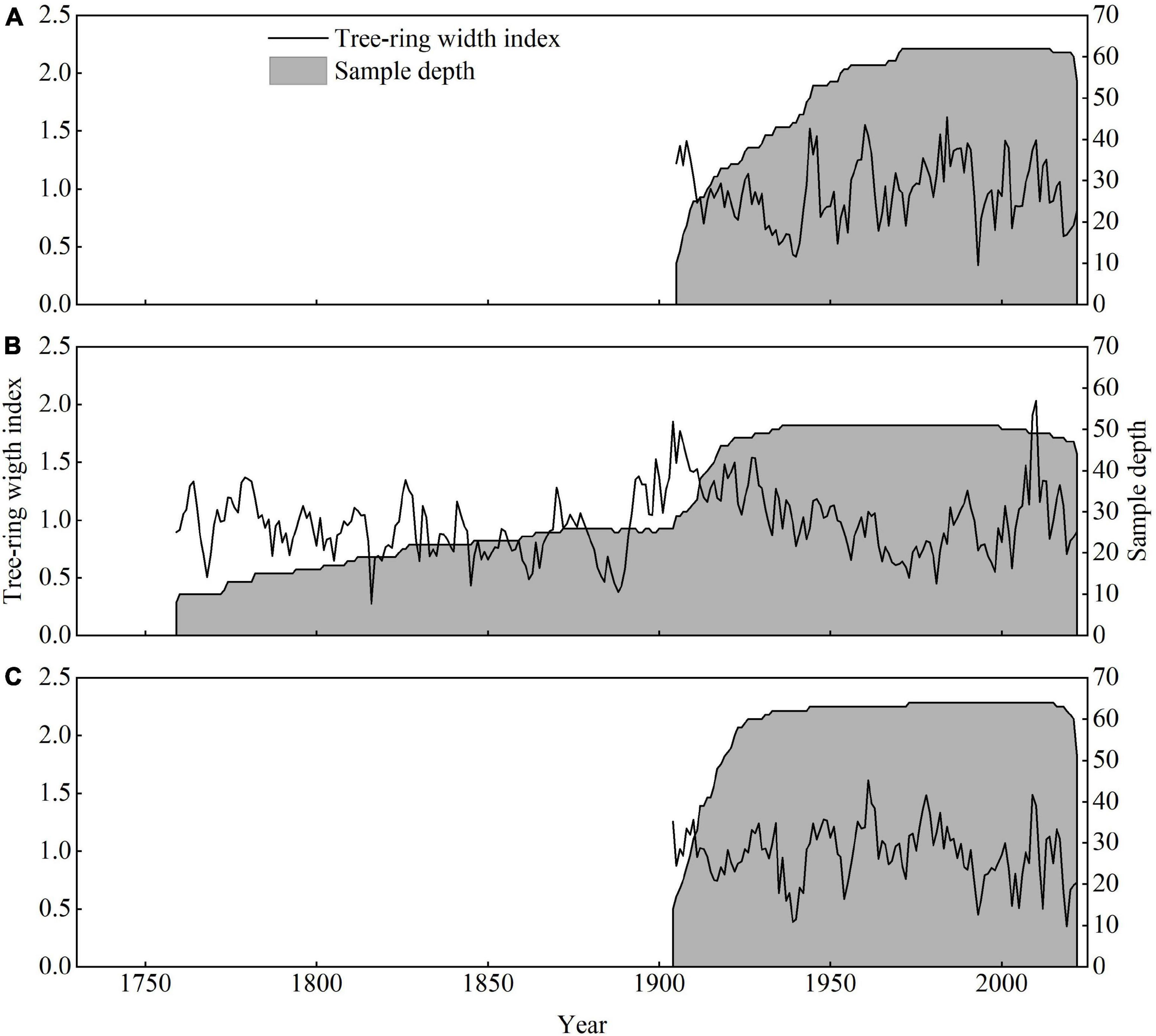
Figure 2. Standard chronology of larch at different altitudes. (A) High elevation chronology. (B) Middle elevation chronology. (C) Low elevation chronology. First year of SSS > 0.85.
3.2 Relationship between radial growth and NDVI at different elevations
In order to better understand the relationship between larch radial growth and NDVI, we performed correlation analysis between NDVI and tree ring width in each month. Moreover, the single-month NDVI was sequentially combined to analyze the correlation between the three elevations tree ring width indices and the NDVI of the combined months. The tree ring width of larch at the three altitudes was positively correlated with summer NDVI. The larch tree ring width index at high and low elevations showed a highly significant positive correlation with NDVI in August of the current year (p < 0.05). The larch tree ring width index at mid-elevation exhibited a significant positive correlation (p < 0.05) with NDVI in May of the current year and a highly significant positive correlation with the average value of NDVI in June to September of the current year (p < 0.01), exhibiting the highest correlation coefficient compared to the other months at three altitudes (r = 0.664, p < 0.01) (Figure 3).
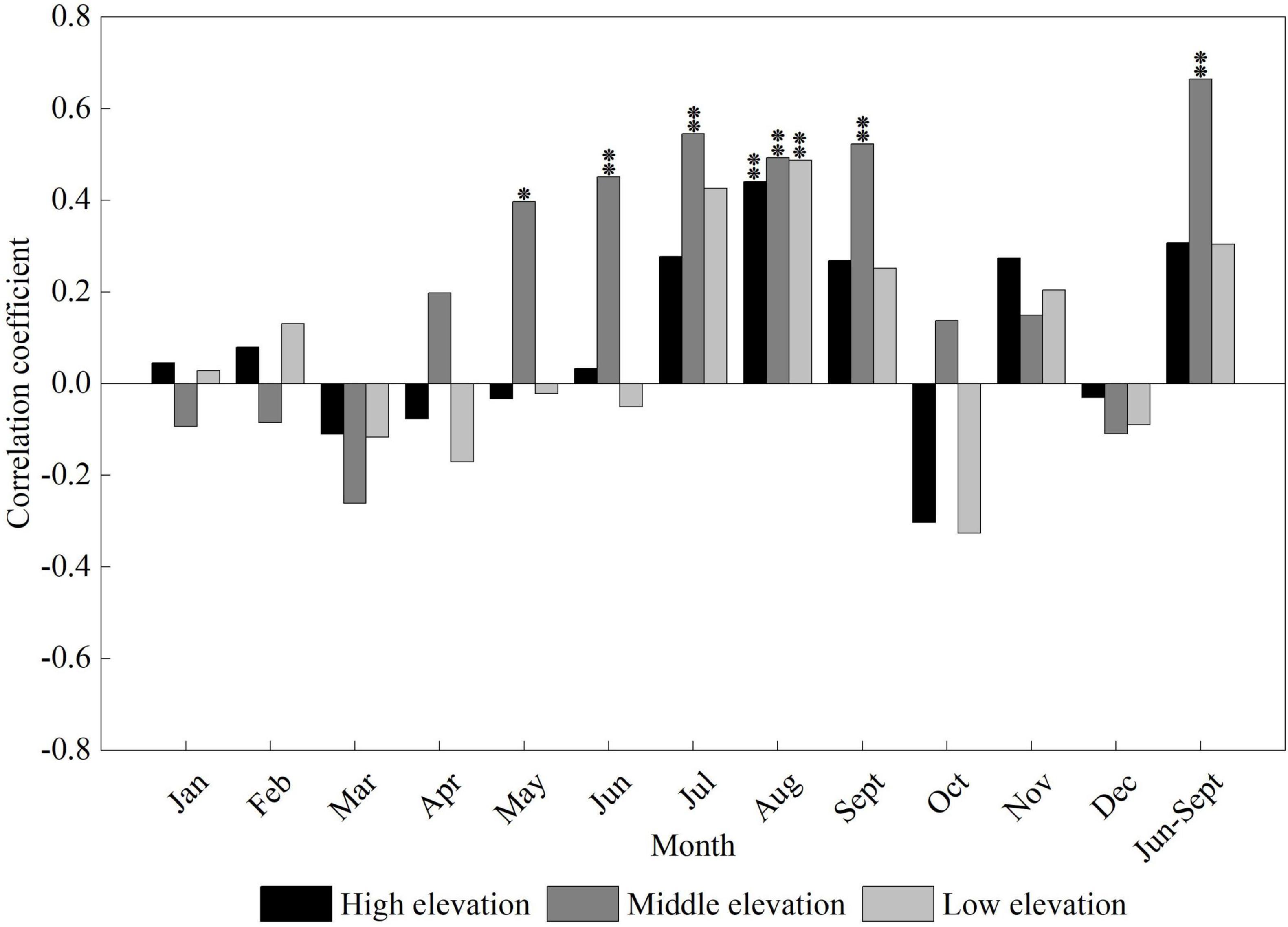
Figure 3. Correlation analysis of annual tree ring width index and NDVI of larch at different elevations. * and ** represent the significance at the 95 and 99% confidence levels, respectively.
3.3 Correlation between NDVI and climate
Based on the above correlation analysis results between tree rings and NDVI, this article selects the mid-elevation larch chronology for further analysis. Figure 4 presents the correlation between the tree ring width and the climate of each month. The tree ring width was highly significantly positive correlated with temperature in May, August, and June–September of the current year (p < 0.01), and significantly positively correlated with temperature in June of the current year (p < 0.05). It was also significantly positively correlated with precipitation in November of the previous year until March of the current year (p < 0.05), and highly significantly positive correlated with precipitation in December of the current year (p < 0.01).
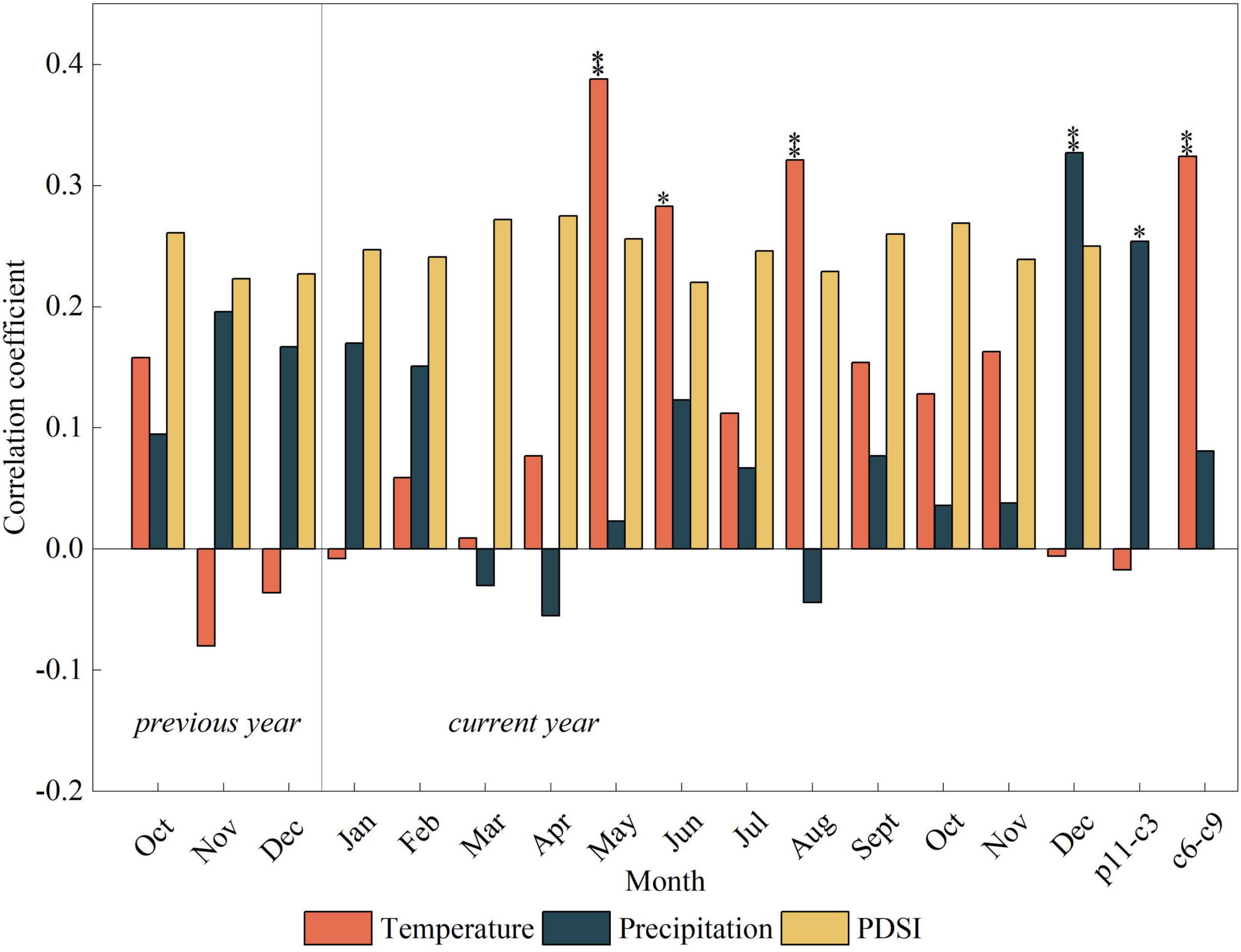
Figure 4. Correlation analysis of tree ring width index and climate factors. *, **, same as in Figure 3. p11-c3, November of the previous year to March of the current year. c6-c9, June to September of current year.
Figure 5 presents the correlation between the instrumental NDVI from June to September and the climate of each month. The NDVI during this period was highly significantly positive correlated with temperature in June, August, and June–September of the current year (p < 0.01). It was also significantly positively correlated with precipitation in November of the previous year until March of the current year (p < 0.05), and highly significantly negatively correlated with precipitation in June–September of the current year (p < 0.01). A highly significant negatively correlation (p < 0.01) with the scPDSI in August and September of the current year. Furthermore there was a highly significantly positive correlated (p < 0.01) with the VPD in June–September of the current year.
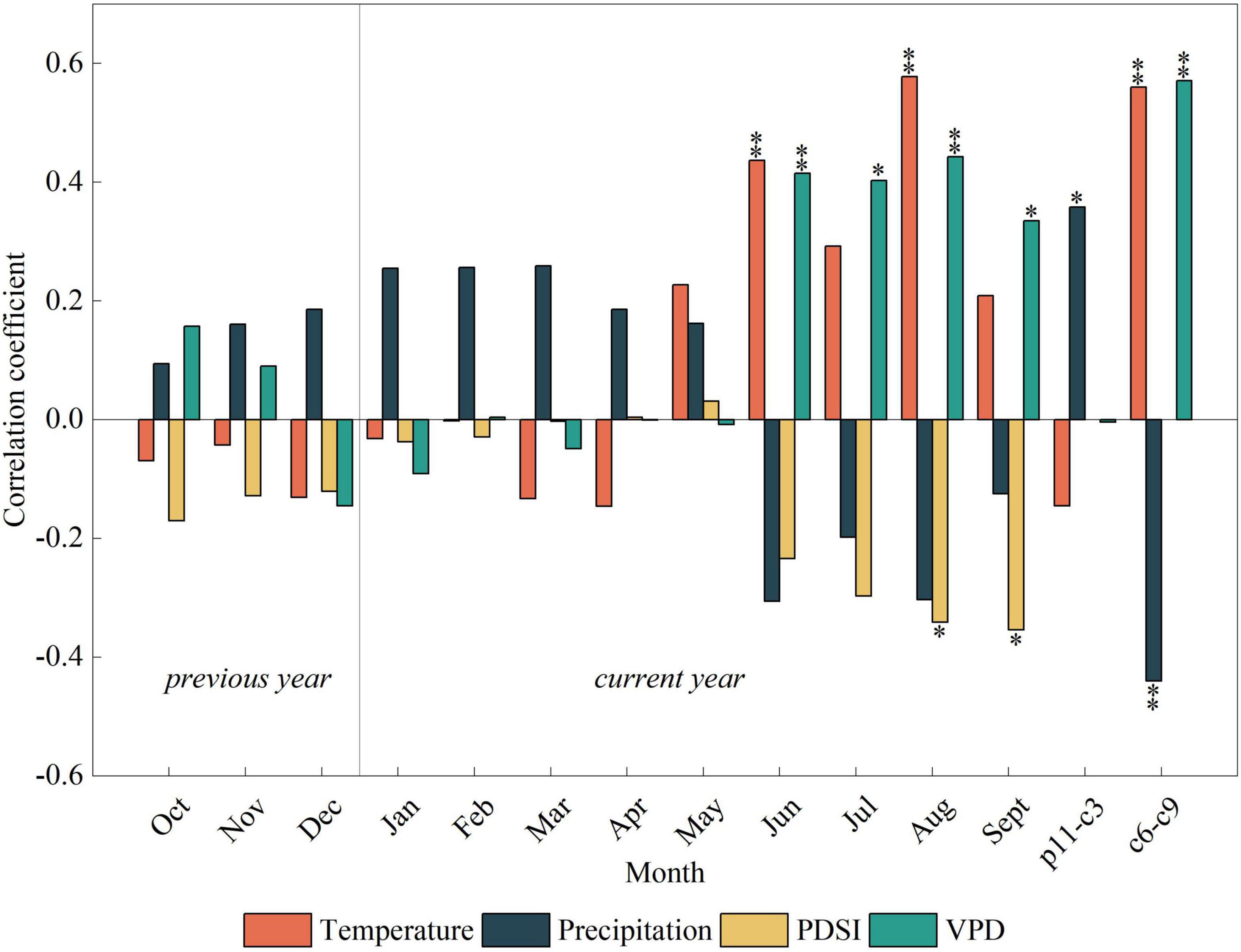
Figure 5. Correlation analysis between June–September NDVI and climate factors. The symbols in the figure are the same as those in Figure 4.
3.4 Reconstruction and features of NDVI
Based on the correlation between the standardized chronology of larch and NDVI, the months from June to September with the highest correlation coefficients were selected. Following this, the NDVI of June to September was reconstructed for the study region using the chronology of tree rings in the mid-altitude region. The reconstruction equation is described as Equation 4:
where STDlyb is the standardized chronology for mid-elevation regions. The explained variance of the reconstructed equation was 47.9%, and 46.4% after factoring out degrees of freedom (F = 33.954). The stability and reliability of the reconstructed equations were tested using the segmental test method, and the reconstruction results were examined using 1981–2001 and 2002–2019 as the calibration periods, respectively (Table 4). Both the reduction of error and coefficient of efficiency exceeded zero, indicating the reliability and stability of the reconstruction equations.
The reconstruction results reveal over the past 263 years reveal a minimum NDVI value in the study area of 0.541 in 1816 and maximum of 0.678 in 2010, with a mean of 0.621 (Figure 6). We then performed a 11-year moving average of the reconstructed NDVI. The results reveal two extremely high periods of canopy vigor (1898–1926 and 2009–2013) during the 263 years and three extremely low periods of canopy vigor (1860–1862, 1882–1888, and 1968–1977). This paper uses wavelet analysis to calculate the period of the reconstructed sequence (Figure 7). We identified 11–13, 23–25, and 39–42 years cycle variations in the June–September NDVI series reconstructed over the past 263 years.
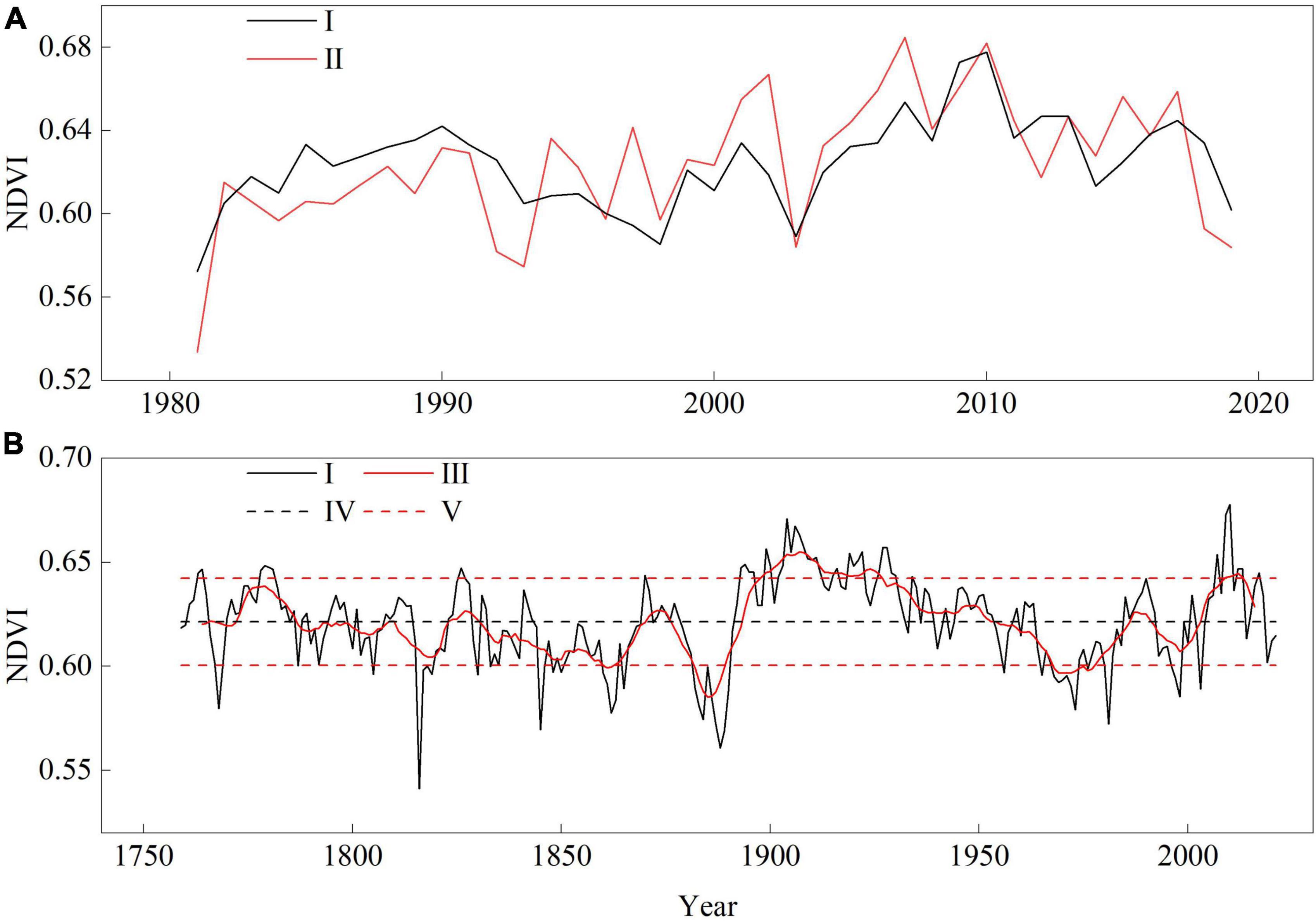
Figure 6. (A) Comparison of measured and reconstructed NDVI values. (B) NDVI reconstructed series and 11-year moving average. I, NDVI reconstructed values; II, NDVI observed values; III, NDVI 11-year moving average; IV, NDVI mean; V, NDVI mean ± standard deviation.
3.5 Spatial correlation analysis of reconstructed NDVI
The results of spatial correlation analysis of the reconstructed NDVI series reveal that the reconstruction results are highly correlated with the mountainous areas of the Greater Khingan Mountains region, the Lesser Khingan Mountains region, and the Changbai Mountains (Figure 8). This indicates that the reconstruction results determined in this paper exhibit a high spatial representativeness of the mountainous areas within Northeastern China and nearby countries. The spatial correlation results of the reconstructed NDVI series with sea surface temperature (Figure 9) and show that the reconstructed sequences generally exhibited significant negative correlations (p < 0.05) with the SST in the northern Pacific Ocean, the Atlantic Ocean, and the sea around China and Antarctica. Spatial correlation analysis of the reconstructed sequences with the Arctic SIC (Figure 10) revealed a significant negative correlation (p < 0.05) between the reconstructed sequences and Arctic SIC.
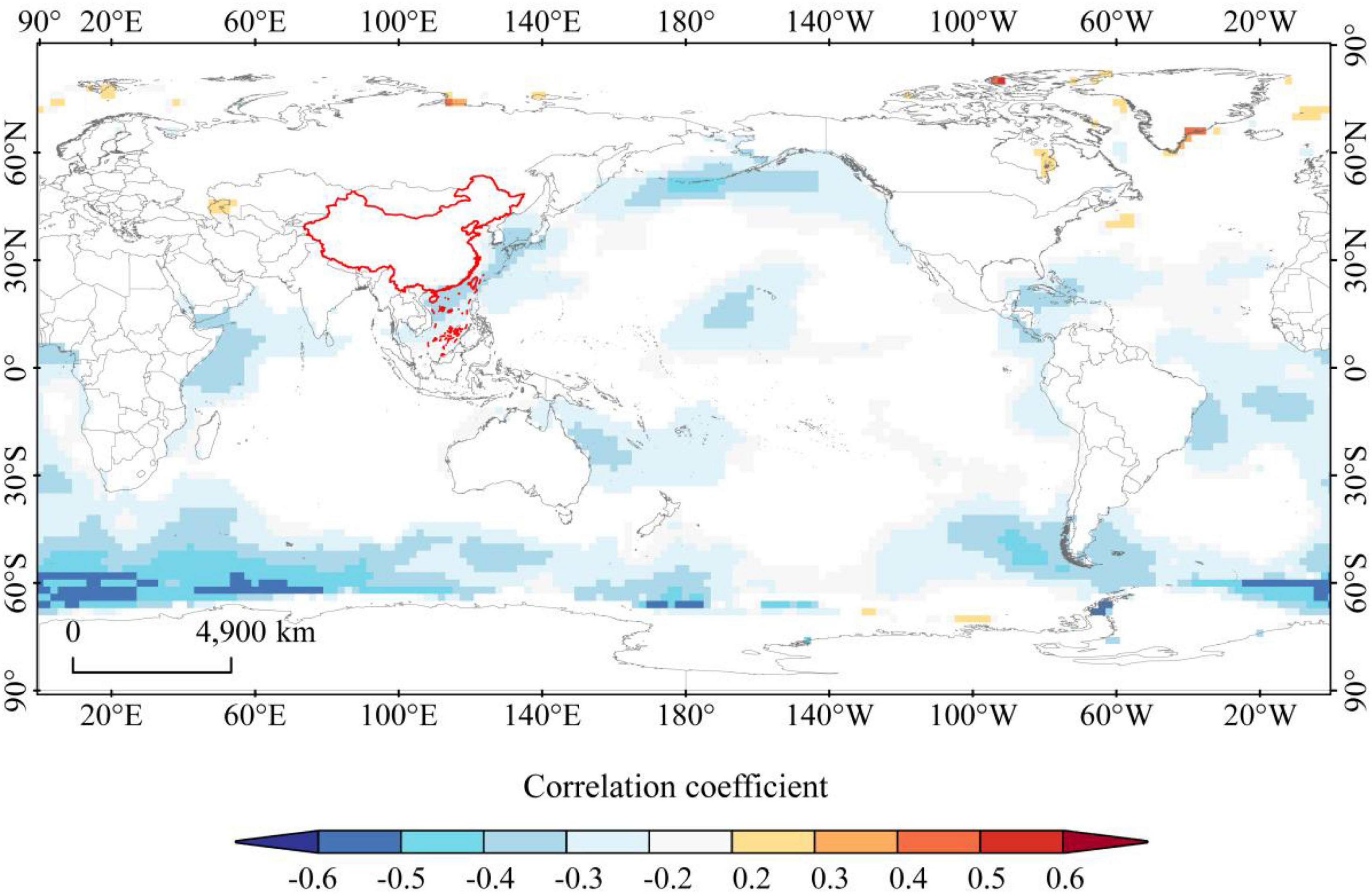
Figure 9. Spatial correlation analysis between reconstrued NDVI series and SST during the same period.
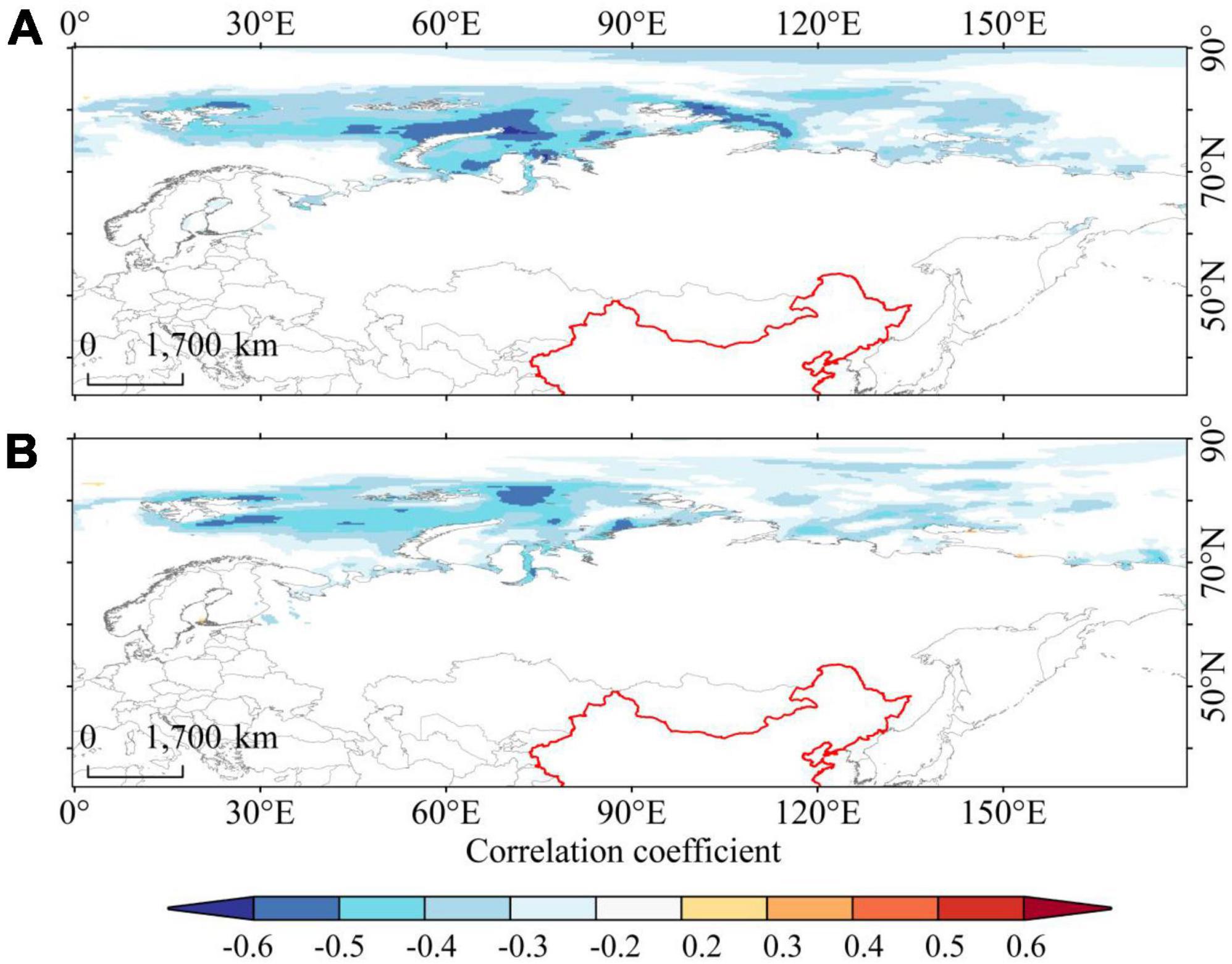
Figure 10. Spatial correlation analysis between reconstrued NDVI series and Arctic SIC. (A) Jan–Mar SIC. (B) May–Sept SIC.
4 Discussion
4.1 Tree growth
The statistical analysis of the chronological parameters of larch tree rings at the three elevation (Table 3) reveals the mean sensitivity of the three chronologies range from 0.250 to 0.263, with greater values at higher elevation. This indicates that the sensitivity of larch to climate change information increases with elevation, and thus the limiting effect of climate factors also increases (Qin et al., 2022). The standard deviations ranged from 0.271 to 0.297 (mm) and decreased with the increasing altitude. This reveals that all three chronologies contained more climate change information and decreased with increasing elevation (Correa-Díaz et al., 2020). The first-order autocorrelation coefficients were determined as 0.618, 0.772, and 0.769 for the three elevations of high, medium and low, respectively. The largest first-order autocorrelation coefficient was observed for the middle elevation, indicating that changes in climatic factors from the previous year and using of stored carbohydrates had the greatest effect on larch radial growth in the middle elevation (Zhang X. et al., 2018). The signal-to-noise ratios of all three elevation chronologies were high, and were enhanced for elevations between 14.664 and 33.645. This demonstrates that the availability of climate information contained in the larch tree ring variation increased with the elevation, as did the quality (Pompa-García et al., 2022). The overall representativeness of the three samples from high to low elevation were 0.971, 0.950, and 0.936, all of which are greater than 0.85. This reveals that the annual surface quality was maximized at higher elevations (Jiang et al., 2017).
4.2 NDVI reconstruction and historical events
In the process of conducting regression analysis, we compared the reconstruction results of different regression models and observed that the power function regression model yielded superior results compared to the linear regression model. This finding leads us to believe that the correlation between tree ring width and NDVI may exhibit nonlinear variations. The radial growth of trees can be influenced by the local environment, resulting in a bimodal or unimodal xylogenesis pattern (Vicente-Serrano et al., 2016; Mašek et al., 2024). Additionally, the temperature required for xylem formation is higher than that needed for photosynthesis. These factors may contribute to nonlinear changes in the relationship between tree rings and NDVI (Rossi et al., 2011).
This study focused on reconstructing the NDVI from June to September in the Mangui area spanning from 1759 to 2021. To the best of our knowledge, this represents the longest reconstructed NDVI sequence in the region. However, it is important to acknowledge the limitations of the current research. The data resolution for both climate and NDVI is relatively coarse, making it difficult to discern differences across the three elevations. The highest correlation with NDVI was observed at mid-elevation, possibly due to the forest populations at this elevation serving as a more representative sample of the average NDVI within the grid. Additionally, the verification period was limited to only 18 years due to the availability of NDVI data. This constraint may have implications for the calibration and validation analyses. Nevertheless, we firmly believe that this study holds potential implications for understanding changes in the forest canopy within the region.
Previous studies have shown that climate change not only alters the dormancy period of trees, but also affects the end-of-growth period, and together with altitude, latitude, and vegetation type, it induces changes in the greenness of tree canopies (Ge et al., 2016; Cai et al., 2021). However, Guo and Zhang (2013) pointed out that the autumn phenology of coniferous forests in the northern Greater Khingan Mountains is mainly inhibited by autumn precipitation and promoted by temperature in the same period. This is similar to the NDVI results in the growing season presented in this paper. As an indicator of vegetation growth, NDVI is closely related to the photosynthesis and energy absorption of the canopy. The NDVI measured during the growth season is helpful to understand the development status of vegetation, the change of vitality and the predictions of productivity. For example, Phan et al. (2021) predicted tea leaf yield using the NDVI measured during the growing season. Based on our results, the tree ring width chronology at middle altitudes can represent the NDVI changes of larch during the growing season in Mangui to a certain extent. The reconstruction results help to determine the impact of climate change on forest dynamics in the study area.
Since the 1960s, extreme weather events have been a frequent occurrence in eastern Inner Mongolia. Studies in this region have shown that extreme precipitation events are significantly associated with changes in large-scale circulation patterns. Moreover, extreme precipitation is prone to catastrophic weather (e.g., high winds and low temperatures), leading to the freezing of trees, crops, and other vegetation (Zhang B. et al., 2018). The Chinese Meteorological Disasters Encyclopedia (Inner Mongolia Volume) (Shen, 2008) reports the disaster events caused by meteorological factors that have caused a great impact on Inner Mongolia from ancient times to the present. Years such as 1960–1963, 1970–1975, 1980–1982, and 1986–1989 all correspond to the low periods of the reconstructed NDVI (less than the 1759–2021 mean). Moreover, the reconstructed data reveals 14 extremely low periods (1956, 1965, 1968–1973, 1976, 1980–1981, and 1996–1998) between 1949 and 2000. These extremely low value years were clearly recorded in the Chinese Meteorological Disasters Encyclopedia. A comparison of the extreme years with the historical disaster events reveals that there were 13 years in which both drought and flood occurred. The only exception is 1976, where no floods occurred in the study area, but cold damage were recorded during the growing season (May–September), with mean temperatures 1–2°C lower than average in previous years. This may have caused the extremely low NDVI of that year, which is consistent with the findings of Zhang X. et al. (2018) NDVI within Northeast China is mainly controlled by temperature and precipitation. The accuracy of the reconstructed series is further verified by comparing hazard events and revealing the ability of the reconstructed series to respond to extreme climate events over long time scales.
4.3 Radial growth of larch in relation to NDVI and climate factors
The tree ring widths at all three elevations exhibited a significant positive correlation with NDVI in August of the current year, indicating that NDVI can well reflect the growth status of plants in the region (Jie et al., 2019). In particular, the response of the standardized chronology of larch to NDVI was stronger in the middle elevations than in the high and low elevation areas. This may be because human activity is more prominent in areas with lower altitudes. Note that the impact of human activity on vegetation is two-fold. On the one hand, the implementation of environmental management projects improves the ecological environment and increases the vegetation cover and vegetation productivity in forests, which induces an increasing NDVI trend. On the other hand, the greater number of forest fires and artificial surfaces have an inhibiting effect on the growth of vegetation, consequently reducing NDVI (Mao et al., 2021). Moreover, environmental conditions such as soil and climate will also change with the altitude. These changes affect the nutrient content and physiological activities of plants, making green forests at different altitudes show distinct greening and browning patterns (Correa-Díaz et al., 2019). In high-altitude areas, both the vegetation and forest community types change. For example, birch gradually decreases and subsequently disappear, while Pinus massoniana gradually increases. The vegetation coverage density also exhibits a gradually decreasing trend, which may partially explain the lower correlation between tree rings and NDVI at high elevations (Estrella et al., 2021).
Correlation analysis between NDVI observations and climate for the period of June–September revealed (Figure 5) that the NDVI in the fast-growing season was promoted by precipitation in the winter and spring of the previous year. This may be because the study area is located in a high-latitude alpine region with cold and long winters. The main form of winter precipitation is snowfall, which stores water in the form of snow. When the temperature rises in spring, the snow melts into soil water, providing sufficient water for the early growing season (Wang et al., 2017). The thicker snow layer is conducive to soil insulation, alleviates the damage caused by low temperature to trees and enhances the activity of soil microorganisms, thereby improving soil nutrients and promoting the activity of plant canopy and cambium (Gao et al., 2022). This study found that NDVI in the growing season (from June to September) is significantly correlated with VPD in the same period. Studies have confirmed that changing VPD and soil drying are one of the main reasons in affecting vegetation dynamics (Wen et al., 2023). Plants regulate photosynthesis by controlling stomatal activity and xylem conductance to cope with excessive water shortage caused by excessive VPD (Yuan et al., 2019). And in some arid regions, vegetation is more sensitive to VPD than precipitation. However, in the Mangui region where the regional climate is relatively humid and soil water contents is high, the higher VPD will adjust soil water by affecting soil evaporation so as to change the soil water conditions in this region and make larch in a suitable growing environment (Wen et al., 2023).
Drought and precipitation in the growing season both inhibited canopy vigor in the same period. However, we found that drought had less impact on the radial growth of larch in the region, while precipitation will promote the radial growth of larch. The growth of larch requires high water conditions, and drought or high humidity environments will lead to poor growth (Chinese Academy of Sciences Editorial Board of The Flora of China, 1978). This is consistent with previous research results on larch in the nearby area unanimous (e.g., eastern Siberia) (Tei et al., 2019). Furthermore, the growth of plant leaves is mainly controlled by water, and mild water stress will cause trees to adjust physiological activities (e.g., stomatal conductance and transpiration rate) to improve the photosynthetic capacity of mature leaves. When the drought degree exceeds a certain threshold, plants will reduce the leaf area growth and change the carbon allocation pattern in order to reduce transpiration and water loss (Khan et al., 2022). However, extreme precipitation in summer may cause damage to forests in middle and high altitudes, resulting in leaf frostbite and inhibiting tree growth. This reduces canopy activity and causes needle leaf browning (Tei et al., 2019). Moreover, the soil water content is inversely proportional to the soil air. When the soil humidity is high, the soil air will inevitably decrease, which will affect the photosynthesis, growth and development of plants (Liu et al., 2015). Studies have shown that summer precipitation can impact the growth of trees by affecting the number and size of tracheid cells, and the leaf vein density of some species decreases with the increase of precipitation, thus limiting the production capacity of leaves (McKown and Dengler, 2010; Liu et al., 2015; Wang et al., 2021).
The impact of temperature on canopy vigor in this area is generally opposite to that of precipitation, particularly in the growing season, with a highly significant positive correlation between NDVI and precipitation in the same period. The larch tree ring width index exhibited a significant positive correlation with temperature, and the correlation was greater in winter than in summer. The impact of temperature on plant growth is complex. For example, it not only affects plant respiration, photosynthesis and other metabolic processes, but also the absorption, transportation and synthesis of organic matter of nutrients (Khan et al., 2022). However, the effect of temperature on trees is not linear. As the temperature rises within a certain range, the maximum photosynthetic rate and optimum photosynthetic temperature of trees will also rise. This enhances the growth of plant organs and xylem, thereby promoting the growth of the tree canopy and radial direction (Jiang et al., 2017; Peng et al., 2021). Temperatures exceeding this range will easily lead to forest decline or even death.
4.4 Reconstructing the relationship between NDVI and large-scale regional climate environments
Figure 6B depicts an obvious fluctuation change in the reconstructed sequence. In order to explore the specific change characteristics of this fluctuation, we employ wavelet analysis to calculate the period of the reconstructed sequence. In particular, the 11–13 cycle may be related to the sunspot activity cycle (MacDonald and Case, 2005). Solar radiation variations directly affect plant growth (Kasatkina et al., 2019; Šimůnek et al., 2021), have a moderating effect on sea and land temperature fluctuations, and can indirectly influence wind anomalies and precipitation. According to Hao et al. (2021) the Mangui area is rich in solar resources and the annual radiation declined significantly between 1977 and 1989. This is consistent with the low-value period observed for the reconstructed NDVI series in this paper. The 23–25 chronostratigraphic cycle may be associated with the Pacific decadal oscillation (PDO) (Han et al., 2017). The reconstructed sequence exhibited a significant positive correlation with the PDO index of the current year (r = 0.228, p < 0.05). Zhang et al. (2020) reported that the effect of PDO on tree growth is not directly related to NDVI, but some underlying mechanism links the two. For example, variations in precipitation due to evapotranspiration anomalies in the North Pacific caused by PDO may have an influence on the changes in NDVI across the study area (Yang et al., 2021). The 39–42 cycle is present throughout the reconstructed sequence and exhibits a 30–60 year cycle variation during 1845–1935, which may be associated with the North Atlantic multidecadal oscillation (AMO). The reconstructed sequence exhibited a significant positive correlation with the AMO index of the current year (r = 0.394, p < 0.01). AMO and PDO act together to influence the hydrothermal conditions in the study area. Zhu et al. (2021) identified the large-scale circulation caused by AMO an important driver of drought in the Greater Khingan Mountains. Wang et al. (2011) found that the AMO positive phase leads to weaker winter winds and stronger summer winds in East Asia, resulting in winters that are warmer and wetter than average and a longer growing season in Northeast China. To verify the above conclusions, the reconstructed sequences were spatially correlated with the global sea surface temperature (SST) (Figure 9). It can be seen from Figure 9 that the reconstructed NDVI sequence shows a significant negative correlation with the SST of multiple sea areas. The temperature in the study area is simultaneously influenced by a combination of sea surface temperatures from several sea areas, and the dominant sea areas vary with the growth stages. This further reveals the simultaneous influence of the solar cycle activity, PDO, and AMO on NDVI cycle variations in the Greater Khingan Mountains region. Other large-scale circulations may also have an impact, however, further studies are required to clarify this.
The water cycle in the middle and high latitudes is influenced by both the SST and Arctic sea ice (Sun et al., 2020). The impact of the sea ice signal on the yearly climate can be long-term. The Indian Ocean SST anomaly and the Arctic sea ice concentration (SIC) anomaly in the first four months of the year act synergistically to induce the emergence of cold eddies in Northeast China. This results in extreme weather, such cold damage and precipitation anomalies (Lin et al., 2023). It can be seen from Figure 10 that both winter and summer Arctic SIC has an impact on tree growth in the study area. This may be attributed to the great changes in atmospheric circulation caused by the melting Arctic sea ice, the enhancement of the Siberian high pressure system, and the response of the Okhotsk offshore air cyclone. These factors affect surface temperature in mid-latitudes during autumn and winter, leading to the weakening of latitudinal westerly winds. This consequently increases the frequency and magnitude of extremely low temperatures and snowstorm events in the north of China, as well as the precipitation in mid-latitudes due to the melting of sea ice in summer (Blackport et al., 2019; Chripko et al., 2021).
5 Conclusion
In this paper, we analyzed the correlation between the tree ring width and NDVI of larch at three elevations. We found that the link between the tree ring width of Larix gmelinii at middle altitudes and the NDVI sequence (r = 0.664, p < 0.01) in the growing season was stronger than that at high and low altitudes. Based on this, a regression model was established to reconstruct the canopy vigor changes at the northern foot of the Greater Khingan Mountains from June to September 1759–2021. Canopy vigor changes in the study area has two extremely high value periods (1898–1926 and 2009–2013) and three extremely low value periods (1860–1962, 1882–1888, and 1968–1977). The vegetation dynamics in the growing season (Jun to Sept) are closely related to the hydrothermal conditions in the same period, and are affected by precipitation throughout the year. The impact of temperature on the forest during the growing season is observed to be stronger than that of precipitation.
The reconstructed NDVI sequence has 11–13 year, 23–25 year and 39–42 year cycle changes. These cycles indicate the presence of a teleconnection between forest dynamics in this region and large-scale climate circulation (such as the sunspot cycle, PDO and AMO). NDVI is related to the Arctic SIC. By analyzing the impact of climate on NDVI, we found that both drought and precipitation were negatively correlated with NDVI, which may be caused by species habits, nonlinear changes of tree growth in response to climate, and the interaction of multiple climatic factors. Therefore, further research is needed to improve our understanding of the links between tree radial growth, NDVI, and climate change. This experiment still has some limitations. The three sampling sites are close to each other, and the coarser resolution of AVHRR NDVI cannot distinguish differences in altitude. The next step will be to extract higher-precision NDVI for follow-up work.
Data availability statement
The raw data supporting the conclusions of this article will be made available by the authors, without undue reservation.
Author contributions
XL: Conceptualization, Data curation, Investigation, Methodology, Project administration, Software, Writing – original draft, Writing – review & editing. ZW: Formal analysis, Methodology, Writing – review & editing. TL: Investigation, Writing – review & editing. XW: Data curation, Writing – review & editing. AW: Validation, Writing – review & editing. DZ: Conceptualization, Project administration, Resources, Validation, Writing – review & editing.
Funding
The authors declare financial support was received for the research, authorship, and/or publication of this article. This research was funded by the National Natural Science Foundation of China (No. 41671064) and Natural Science Foundation of Heilongjiang Province (No. LH2021D012).
Acknowledgments
Sun Rui and Huang Jingwen provided assistance during data collection.
Conflict of interest
The authors declare that the research was conducted in the absence of any commercial or financial relationships that could be construed as a potential conflict of interest.
Publisher’s note
All claims expressed in this article are solely those of the authors and do not necessarily represent those of their affiliated organizations, or those of the publisher, the editors and the reviewers. Any product that may be evaluated in this article, or claim that may be made by its manufacturer, is not guaranteed or endorsed by the publisher.
References
Berra, F. E., Fontana, C. D., and Kuplich, M. T. (2017). TREE AGE AS ADJUSTMENT FACTOR TO NDVI. Rev. Árvore. 41:7. doi: 10.1590/1806-90882017000300007
Blackport, R., Screen, J. A., van der Wiel, K., and Bintanja, R. (2019). Minimal influence of reduced Arctic sea ice on coincident cold winters in mid-latitudes. Nat. Clim. Change 9, 697–704. doi: 10.1038/s41558-019-0551-4
Bunn, A. G. (2010). Statistical and visual crossdating in R using the dplR library. Dendrochronologia 28, 251–258. doi: 10.1016/j.dendro.2009.12.001
Cai, S. H., Song, X. N., Hu, R. H., and Guo, D. (2021). Ecosystem-dependent responses of vegetation coverage on the tibetan plateau to climate factors and their lag periods. Isprs Int. J. Geo Inf. 10:394. doi: 10.3390/ijgi10060394
Castillo, J. D., Voltas, J., and Ferrio, J. P. (2015). Carbon isotope discrimination, radial growth, and NDVI share spatiotemporal responses to precipitation in Aleppo pine. Trees 29, 223–233. doi: 10.1007/s00468-014-1106-y
Chripko, S., Msadek, R., Sanchez-Gomez, E., Terray, L., Bessières, L., and Moine, M. (2021). Impact of reduced arctic sea ice on northern hemisphere climate and weather in autumn and winter. J. Clim. 34, 5847–5867. doi: 10.1175/JCLI-D-20-0515.1
Correa-Díaz, A., Silva, L. C. R., Horwath, W. R., Gómez-Guerrero, A., Vargas-Hernández, J., Villanueva-Díaz, J., et al. (2020). From trees to ecosystems: Spatiotemporal scaling of climatic impacts on Montane landscapes using dendrochronological, isotopic, and remotely sensed data. Glob. Biogeochem. Cy. 34:e2019GB006325. doi: 10.1029/2019GB006325
Correa-Díaz, A., Silva, L. C. R., Horwath, W. R., Gómez-Guerrero, A., Vargas-Hernández, J., Villanueva-Díaz, J., et al. (2019). Linking remote sensing and dendrochronology to quantify climate-induced shifts in high-elevation forests over space and time. J. Geophys. Res. 124, 166–183. doi: 10.1029/2018JG004687
DiGirolamo, N., Parkinson, C. L., Cavalieri, D. J., Gloersen, P., and Zwally, H. J. (2022). Sea ice concentrations from nimbus-7 SMMR and DMSP SSM/I-SSMIS Passive Microwave Data, Version 2 [Data Set]. Boulder, CO: NASA National Snow and Ice Data Center Distributed Active Archive Center, doi: 10.5067/MPYG15WAA4WX
Duan, L. L., Man, X. L., Kurylyk, B. L., Cai, T. J., and Li, Q. (2017). Distinguishing streamflow trends caused by changes in climate, forest cover, and permafrost in a large watershed in northeastern China. Hydrol. Process. 31, 1938–1951. doi: 10.1002/hyp.11160
Estrella, E. H., Stoeth, A., Krakauer, N. Y., and Devineni, N. (2021). Quantifying vegetation response to environmental changes on the galapagos islands, ecuador using the normalized difference vegetation index (NDVI). Environ. Res. Commun. 3, 1–23. doi: 10.1088/2515-7620/ac0bd1
Fang, J. Y., Piao, S. L., He, J. S., and Ma, W. H. (2004). Increasing terrestrial vegetation activity in China, 1982–1999. Sci. China Ser. C 47, 229–240. doi: 10.1007/BF03182768
Filizzola, C., Carlucci, M. A., Genzano, N., Ciancia, E., Lisi, M., Pergola, N., et al. (2022). Robust satellite-based identification and monitoring of forests having undergone climate-change-related stress. Land Basel. 11:825. doi: 10.3390/land11060825
Fritts, H. C. (1976). Tree ring and climate. London: Academic Press, doi: 10.1016/B978-0-12-268450-0.X5001-0
Gao, S., Liang, E. Y., Liu, R. S., Babst, F., Camarero, J. J., Fu, Y. S. H., et al. (2022). An earlier start of the thermal growing season enhances tree growth in cold humid areas but not in dry areas. Nat. Ecol. Evol. 6, 397–404. doi: 10.1038/s41559-022-01668-4
Ge, Q. S., Dai, J. H., Cui, H. J., and Wang, H. J. (2016). Spatiotemporal variability in start and end of growing season in China related to climate variability. Remote Sens. Basel. 8:433. doi: 10.3390/rs8050433
Guo, X. Y., and Zhang, H. Y. (2013). The vegetation dynamic research under of eco-geographical region framework on greater Khingan Mountains. Sci. Geogr. Sin. 33, 181–188. doi: 10.13249/j.cnki.sgs.2013.02.011
Han, Z. X., Su, T., Zhi, R., and Feng, G. L. (2017). Effects of moisture budget changes on pacific evaporation associated with pacific decadal oscillation and ENSO in boreal winter. Chin. J. Atmos. Sci. 41, 1316–1331. doi: 10.3878/j.issn.1006-9895.1702.16257
Hao, Y. Z., Li, X. H., Hu, Y. N., and Zhao, J. Q. (2021). Variation law and influencing factors of solar energy resources in Inner Mongolia in 57 years. Acta Energ. Sol. Sin. 42, 145–151. doi: 10.19912/j.0254-0096.tynxb.2019-0868
Holmes, R. L. (1983). Computer-assisted quality control in tree-ring dating and measurement. Tree Ring Bull. 43, 69–78.
Jiang, P., Liu, H. Y., Wu, X. C., and Wang, H. Y. (2017). Tree-ring-based SPEI reconstruction in central Tianshan Mountains of China since A.D. 1820 and links to westerly circulation. Int. J. Climatol. 37, 2863–2872. doi: 10.1002/joc.4884
Jie, Y., Wang, Z. Q., Suld, B., Zhang, D., Yan, Y. L., Chen, T. L., et al. (2019). Changing trends of NDVI and their responses to climatic variation in different types of grassland in Inner Mongolia from 1982 to 2011. Sustain. Basel. 11, 3256–3256. doi: 10.3390/su11123256
Kasatkina, E. A., Shumilov, O. I., and Timonen, M. (2019). Solar activity imprints in tree ring-data from northwestern Russia. J. Atmos. Sol Terr. Phys. 193:105075. doi: 10.1016/j.jastp.2019.105075
Khan, A., Shen, F., Yang, L., Xing, W., and Clothier, B. (2022). Limited acclimation in leaf morphology and anatomy to experimental drought in temperate forest species. Biology 11:1186. doi: 10.3390/biology11081186
Lin, Y. T., Fang, Y. H., Zhao, C. Y., Gong, Z. Q., Yang, S. Q., and Yu, Y. Q. (2023). the coordinated influence of Indian Ocean sea surface temperature and arctic sea ice on anomalous northeast China cold vortex activities with different paths during late summer. Adv. Atmos. Sci. 40, 62–77. doi: 10.1007/s00376-022-1415-9
Liu, Y. J., Zhu, L. J., Su, J. J., and Wang, X. C. (2015). Impact of decreasing precipitation on Larix gmelinii radial growth in Maoershan, Xiaoxing’an Mountain, China. Acta Ecol. Sin. 35, 4527–4537. doi: 10.5846/stxb201406291339
MacDonald, G. M., and Case, R. A. (2005). Variations in the pacific decadal oscillation over the past millennium. Geophys. Res. Lett. 32:L08703. doi: 10.1029/2005GL022478
Mao, X. P., Xi, J. J., Fan, J. H., Lv, Y. Y., Xu, W. G., Wang, Z., et al. (2021). Dynamic analysis and prediction of landscape pattern in Daxinganling forest-grass ecotone in the Inner Mongonia. Acta Ecol. Sin. 41, 8623–8634. doi: 10.5846/stxb202006191604
Mao, X., Ren, H. L., and Liu, G. (2022). Primary interannual variability patterns of the growing-season NDVI over the tibetan plateau and main climatic factors. Remote Sens. Basel 14:5183. doi: 10.3390/rs14205183
Mašek, J., Tumajer, J., Lange, J., Kaczka, R., Fišer, P., and Treml, V. (2023). Variability in tree-ring width and NDVI responses to climate at a landscape level. Ecosystems 26, 1144–1157. doi: 10.1007/s10021-023-00822-8
Mašek, J., Tumajer, J., Lange, J., Vejpustková, M., Kašpar, J., Šamonil, P., et al. (2024). Shifting climatic responses of tree rings and NDVI along environmental gradients. Sci. Total Environ. 908:168275. doi: 10.1016/j.scitotenv.2023.168275
McKown, A. D., and Dengler, N. G. (2010). Vein patterning and evolution in C4 plants. Botany 88, 775–786. doi: 10.1139/B10-055
National Climate Center of China Meteorological Administration (2022). Blue book on climate change in China 2022. PeKing: Science Press.
Pan, Y., Birdsey, R. A., Fand, J., Houghton, R., Kauppi, P. E., Kurz, W. A., et al. (2011). A large and persistent carbon sink in the world’s forests. Science 333, 988–993. doi: 10.1126/science.1201609
Peng, Z. T., Guo, M. M., Zhang, Y. D., Gu, F. X., Shao, H., and Liu, S. R. (2021). Effects of abrupt warming on Picea likiangensis var. balfouriana and Abies squamata growth at tree line in Dafu, Sichuan, China. Acta Ecol. Sin. 41, 8202–8211. doi: 10.5846/stxb202012113161
Phan, P., Chen, N., Xu, L., Dao, D. M., and Dang, D. (2021). NDVI variation and yield prediction in growing season: A case study with tea in Tanuyen Vietnam. Atmosphere 12:962. doi: 10.3390/atmos1208096
Piao, S. L., and Wang, X. H. (2023). Biological systems under climate change: What do we learn from the IPCC AR6. Glob. Change Biol. 29, 5120–5121. doi: 10.1111/gcb.16857
Piao, S. L., Yue, C., Ding, J. Z., and Guo, Z. T. (2022). Perspectives on the role of terrestrial ecosystems in the ‘carbon neutrality’ strategy. Sci. China Earth Sci. 52, 1419–1426. doi: 10.1360/SSTe-2022-0011
Pompa-García, M., Vivar-Vivar, E. D., Sigala-Rodríguez, J. A., and Padilla-Martínez, J. R. (2022). What are contemporary mexican conifers telling us? A perspective offered from tree rings linked to climate and the NDVI along a spatial gradient. Remote Sens. Basel 14:4506. doi: 10.3390/rs14184506
Qin, J., Bai, H., Zhao, P., Fang, S., Xiang, Y., and Huang, X. (2022). Dendrochronology-based normalized difference vegetation index reconstruction in the Qinling Mountains, North-Central China. Forests 13:443. doi: 10.3390/f13030443
Rossi, S., Morin, H., Deslauriers, A., and Plourde, P.-Y. (2011). Predicting xylem phenology in black spruce under climate warming. Glob. Change Biol. 17, 614–625. doi: 10.1111/j.1365-2486.2010.02191.x
Rouse, J. W., Haas, R. H., Schell, J. A., and Deering, D. W. (1973). “Monitoring vegetation systems in the great plains with ERTS,” in Proceedings of the 3rd ERTS symposium, NASA SP-351, (Washington DC).
Seidl, R., Thom, D., Kautz, M., Martin-Benito, D., Peltoniemi, M., Vacchiano, G., et al. (2017). Forest disturbances under climate change. Nat. Clim. Change 7, 395–402. doi: 10.1038/nclimate3303
Sellers, P. J., Meeson, B. W., Hall, F. G., Asrar, G., Murphy, R. E., Schiffer, R. A., et al. (1995). Remote sensing of the land surface for studies of global change: Models-algorithms-experiments. Remote Sens. Environ. 51, 3–26. doi: 10.1016/0034-4257(94)00061-Q
Shen, J. G. (2008). Grand dictionary of china meteorological disasters: Inner Mongolia volume. PeKing: Meteorological Press.
Šimůnek, V., Vacek, Z., Vacek, S., Ripullone, F., Hájek, V., and D’Andrea, G. (2021). Tree rings of European beech (Fagus sylvatica L.) indicate the relationship with solar cycles during climate change in central and Southern Europe. Forests 12:259. doi: 10.3390/f12030259
Stokes, M. A., and Smiley, T. L. (1996). An introduction to tree-ring dating. Tucson, AZ: University of Arizona Press.
Sun, C., Liu, Y., and Zhang, J. (2020). Roles of sea surface temperature warming and loss of Arctic Sea ice in the enhanced summer wetting trend over northeastern Siberia during recent decades. J. Geophys. Res. Atmos. 125:e2020JD032557. doi: 10.1029/2020JD032557
Tei, S., Sugimoto, A., Kotani, A., and Ohta, T. (2019). Strong and stable relationships between tree-ring parameters and forest-level carbon fluxes in a Siberian larch forest. Polar Sci. 21, 146–157. doi: 10.1016/j.polar.2019.02.001
The Flora of China (1978). Chinese academy of sciences editorial board of “The Flora of China”, Vol. 7. PeKing: Science Press, 187.
Thomte, L., Bhagabati, A. K., and Shah, S. K. (2022). Soil moisture-based winter–spring drought variability over West Karbi Anglong region, Assam, Northeast India using tree-rings of Pinus kesiya. Environ. Challeng. 7:100512. doi: 10.1016/j.envc.2022.100512
Trouet, V., and Van Oldenborgh, G. J. (2013). KNMI climate explorer: A web-based research tool for high-resolution paleoclimatology. Tree Ring Res. 69, 3–13. doi: 10.3959/1536-1098-69.1.3
van der Schrier, G., Barichivich, J., Briffa, K. R., and Jones, P. D. (2013). A scPDSI-based global data set of dry and wet spells for 1901–2009. J. Geophys. Res. Atmos. 118, 4025–4048. doi: 10.1002/jgrd.50355
Verhoeven, V. B., and Dedoussi, I. C. (2022). Annual satellite-based NDVI-derived land cover of Europe for 2001–2019. J. Environ. Manag. 302:113917. doi: 10.1016/j.jenvman.2021.113917
Vermote and Eric; NOAA CDR Program (2019). NOAA climate data record (CDR) of AVHRR normalized difference vegetation index (NDVI), version 5. Asheville, NC: NOAA National Centers for Environmental Information, doi: 10.7289/V5ZG6QH9
Vicente-Serrano, S. M., Camarero, J. J., Olano, J. M., Martín-Hernández, N., Peña-Gallardo, M., Tomás-Burguera, M., et al. (2016). Diverse relationships between forest growth and the normalized difference vegetation index at a global scale. Remote Sens. Environ. 187, 14–29. doi: 10.1016/j.rse.2016.10.001
Wang, C. S., Lv, W. W., Sun, J. P., Zhou, Y., Jiang, L. L., Li, B. W., et al. (2021). Responses of plant leaf traits to simulated rainfall changes in alpine region. Acta Ecol. Sin. 41, 9760–9772. doi: 10.5846/stxb202012253269
Wang, H. Q., Chen, F., Zhang, R. B., and Qin, L. (2017). Seasonal dynamics of vegetation of the central Loess Plateau (China) based on tree rings and their relationship to climatic warming. Environ. Dev. Sustain. 19, 2535–2546. doi: 10.1007/s10668-016-9870-z
Wang, X., Brown, P. M., Zhang, Y., and Song, L. (2011). Imprint of the Atlantic multidecadal oscillation on tree-ring widths in Northeastern Asia since 1568. PLoS One 6:e22740. doi: 10.1371/journal.pone.0022740
Wei, Z., and Wan, X. (2022). Spatial and temporal characteristics of NDVI in the Weihe river basin and its correlation with terrestrial water storage. Remote Sens. Basel 14:5532. doi: 10.3390/rs14215532
Wen, R. H., Jiang, P., Qin, M. O., Jia, Q. Y., Cong, N., Wang, X. Y., et al. (2023). Regulation of NDVI and ET negative responses to increased atmospheric vapor pressure deficit by water availability in global drylands. Front. For. Glob. Change 6:1164347. doi: 10.3389/ffgc.2023.1164347
Wen, Y., Jiang, Y., Jiao, L., Hou, C. X., and Xu, H. (2022). Inconsistent relationships between tree ring width and normalized difference vegetation index in Montane evergreen coniferous forests in arid regions. Trees 36, 379–391. doi: 10.1007/s00468-021-02211-x
Wigley, T. M. L., Briffa, K. R., and Jones, P. D. (1984). On the average value of correlated time series, with applications in dendroclimatology and hydrometeorology. J. Appl. Meteor. Climatol. 23, 201–213. doi: 10.1175/1520-0450(1984)023<0201:OTAVOC>2.0.CO;2
Yang, L., Li, J. R., Peng, J. F., Huo, J. X., and Chen, L. (2021). Temperature variation and influence mechanism of Pinus tabulaeformis ring width recorded since 1801 at Yao Mountain, He’nan Province. Acta Ecol. Sin. 41, 79–91. doi: 10.5846/stxb201908101675
Yuan, W. P., Zheng, Y., Piao, S. L., Ciais, P., Lombardozzi, D., Wang, Y., et al. (2019). Increased atmospheric vapor pressure deficit reduces global vegetation growth. Sci. Adv. 5:eaax1396. doi: 10.1126/sciadv.aax1396
Zhang, L. J., Li, Y. S., Zhang, F., Chen, L., Pan, T., Wang, B., et al. (2020). Changes of winter extreme precipitation in Heilongjiang province and the diagnostic analysis of its circulation features. Atmos. Res. 245:105094. doi: 10.1016/j.atmosres.2020.105094
Zhang, B., Liu, X. F., Zheng, G. F., Wang, F., and Wang, S. Y. (2018). Variation of the days of extreme precipitation in Ningxia in summer and its causes. Trans. Atmos. Sci. 41, 176–185. doi: 10.13878/j.cnki.dqkxxb.20171102001
Zhang, T. W., Zhang, R. B., Lu, B., Mambetov, B. T., Kelgenbayev, N., Dosmanbetov, N., et al. (2018). Picea schrenkiana tree-ring chronologies development and vegetation index reconstruction for the Alatau Mountains, Central Asia. Geochronometria 45, 107–118. doi: 10.1515/geochr-2015-0091
Zhang, X., Song, W. Q., Zhao, H. Y., Zhu, L. J., and Wang, X. C. (2018). Variation of July NDVI recorded by tree-ring index of Pinus koraiensis and Abies nephrolepis forests in the southern Xiaoxing’an Mountains of northeastern China. J. Beijing For. Univ. 40, 9–17. doi: 10.13332/j.1000-1522.20180295
Keywords: tree ring, Greater Khingan Mountain, NDVI, reconstruction, Larix gmelinii
Citation: Li X, Wang Z, Luo T, Wang X, Wang A and Zhang D, (2024) Reconstruction of NDVI based on Larix gmelinii tree-rings during June–September 1759–2021. Front. For. Glob. Change 7:1283956. doi: 10.3389/ffgc.2024.1283956
Received: 27 August 2023; Accepted: 04 June 2024;
Published: 19 June 2024.
Edited by:
Ned Fetcher, Wilkes University, United StatesReviewed by:
Xiaomin Zeng, Shaanxi Normal University, ChinaEnrico Tonelli, Marche Polytechnic University, Italy
Arian Correa-Diaz, Instituto Nacional de Investigaciones Forestales, Agrícolas y Pecuarias, Mexico
Copyright © 2024 Li, Wang, Luo, Wang, Wang and Zhang. This is an open-access article distributed under the terms of the Creative Commons Attribution License (CC BY). The use, distribution or reproduction in other forums is permitted, provided the original author(s) and the copyright owner(s) are credited and that the original publication in this journal is cited, in accordance with accepted academic practice. No use, distribution or reproduction is permitted which does not comply with these terms.
*Correspondence: Dongyou Zhang, zhangdy@hrbnu.edu.cn