- 1USDA Forest Service, Pacific Northwest Research Station, Wenatchee, WA, United States
- 2Department of Ecosystem Science and Management, University of Wyoming, Laramie, WY, United States
- 3USDA Forest Service, Pacific Southwest Research Station, Placerville, CA, United States
- 4School of Environmental and Forest Sciences, University of Washington, Seattle, WA, United States
- 5Pacific Northwest National Laboratory, Richland, WA, United States
- 6School of Civil and Environmental Engineering, University of Washington, Seattle, WA, United States
Introduction: Forest landscapes offer resources and ecosystem services that are vital to the social, economic, and cultural well-being of human communities, but managing for these provisions can require socially and ecologically relevant trade-offs. We designed a spatial decision support model to reveal trade-offs and synergies between ecosystem services in a large eastern Cascade Mountain landscape in Washington State, USA.
Methods: We used process-based forest landscape (LANDIS-II) and hydrology (DHSVM) models to compare outcomes associated with 100 years of simulated forest and wildfire dynamics for two management scenarios, Wildfire only and Wildfire + Treatments. We then examined the strength and spatial distribution of potential treatment effects and trends in a set of resources and ecosystem services over the simulation period.
Results: We found that wildfire area burned increased over time, but some impacts could be mitigated by adaptation treatments. Treatment benefits were not limited to treated areas. Interestingly, we observed neighborhood benefits where fire spread and severity were reduced not only in treated patches but in adjacent patches and landscapes as well, creating potential synergies among some resource benefits and services. Ordinations provided further evidence for two main kinds of outcomes. Positive ecological effects of treatments were greatest in upper elevation moist and cold forests, while positive benefits to human communities were aligned with drier, low- and mid-elevation forests closer to the wildland urban interface.
Discussion: Our results contribute to improved understanding of synergies and tradeoffs linked to adaptation and restoration efforts in fire-prone forests and can be used to inform management aimed at rebuilding resilient, climate-adapted landscapes.
Introduction
Forested landscapes provide ecosystem services and resources that are vital to the social, economic, and cultural well-being of human communities (Dale et al., 2001). Adapting to climate change while maintaining ecosystem functioning requires the analysis of complex tradeoffs (Bennett et al., 2009), often in the face of considerable uncertainty (Millar et al., 2007). The purpose of this study was to advance methods to evaluate such tradeoffs, mitigate future uncertainty through simulation modeling, and to assess the potential for adaptation actions to improve ecosystem functioning among seven broad categories of social-ecological health.
Ecosystems confer many benefits directly, including trails and access to open space for recreation, fish and wildlife resources, cultural resources for Indigenous tribes, sustainable fiber production and wood supply, firewood, and edible mushrooms. Indirect benefits are often more diffuse, yet they too can be of profound importance to human communities both near and far. These more indirect benefits include carbon sinks and stocks, plant and animal biotic and genetic diversity, water quality and quantity, self-stabilizing ecosystem processes, and maintaining healthy riparian geomorphology (Bisson et al., 2003).
An ecosystem service of particular importance in the Intermountain West is the preservation of winter snowpacks and the regulation of stream flows. Patchy forest mosaics can reduce snow interception while still providing shade, which can reduce snowpack attenuation and increase late season stream flows, providing critical habitat for anadromous and coldwater fish populations (Lundquist et al., 2013). At regional and global scales, forests provide crucial regulation of atmospheric carbon by storing it in long-term carbon sinks and stocks (Magnani et al., 2007). The carbon storage potential of forests is essential to global efforts to curb anthropogenic global warming, and there has been a surge in interest from governments and industries to enhance the carbon storage in live forests to offset carbon emissions (Badgley et al., 2022).
The viability and integrity of these vital ecosystem functions is jeopardized by the rapid and interactive stresses of climate change, long-term drought, large-scale insect outbreaks, and increasing wildfire activity (Barnosky et al., 2012; Seidl et al., 2016). There is mounting concern that positive feedbacks between climate change and wildfire (Loehman et al., 2014; Calkin et al., 2015) will significantly compromise the capacity of forests to offset climate change (Walker et al., 2019; Coop et al., 2020; Clarke et al., 2022; Wu et al., 2023), and this is driving a paradigm shift in land and resource management. Land managers and policy makers are increasingly concerned with the social-ecological values provided by intact forest landscapes, and management of public forest lands is turning toward managing for multiple benefits, maintaining ecosystem functions, and bolstering resilience to climate warming and novel disturbance regimes (Millar et al., 2007; Franklin and Johnson, 2012; Hessburg et al., 2015, 2021).
This has created an expanding need for both quantitative and qualitative techniques that can aide in strategic landscape management planning and tradeoff analyses at fine, meso-, and broad-scales. Managing for these multiple benefits is a wicked problem (Davies et al., 2015). How does one integrate a diverse, dynamic, and often conflicting set of stakeholder values? How are community needs balanced with ecological priorities? How are adaptation plans reconciled with economic constraints? The planning process can be aided by decision support models that can aggregate spatial data layers and knowledge bases that can inform strategic management planning processes across large landscapes (Vogler et al., 2015; Abelson et al., 2021; Povak et al., 2022; Reynolds et al., 2023).
Decision support systems facilitate the quantitative assessment of potential synergies (i.e., treatments may benefit multiple ecosystem services) and tradeoffs (i.e., benefits to one service necessitates reduction in another) between different ecosystem services (Maron and Cockfield, 2008; Bennett et al., 2009; Povak et al., 2022). These tools are used to translate social-ecological values into quantitative evaluations of resource conditions (i.e., departure from target conditions) and their potential for adaptation and maintenance, enabling managers to develop strategic plans with explicit consideration of the ecosystem services that are of the greatest value locally and regionally (Eriksson et al., 2022). By identifying areas where adaptation treatments can produce synergistic benefits, managers are able to allocate resources to improve the impact of each dollar spent (e.g., Ager et al., 2016).
A common example of a treatment synergy involves wildfire risk reduction, economic recovery, and tree density reduction in fire-prone forests (e.g., Vogler et al., 2015; Ager et al., 2016). Adaptation treatments can produce co-benefits among all three topics, and this forms the basis for many restoration prioritization efforts throughout the west (Prichard et al., 2021). Predicting potential treatment synergies and tradeoffs becomes increasingly complicated as we consider additional ecosystem services. For example, adding in surface fuel reduction can negatively impact carbon sequestration and some wildlife habitats in the short term (Underwood et al., 2010), but can have positive long-term effects by mediating future fire severity and probability of stand-replacing fire (Tempel et al., 2015; Krofcheck et al., 2018).
Evidence also suggests that forest thinning can enhance streamflow directly by reducing evapotranspiration and increasing snowpack retention (Dickerson-Lange et al., 2021), but the relationship between forest cover and snow retention is complex – particularly across large environmental gradients (Dickerson-Lange et al., 2021). Some evidence suggests that forest cover tends to decrease streamflow in warmer climates by increasing evapotranspiration and snow sublimation losses while accelerating snow attenuation through downwelling radiation. However, in cold climates, forest cover can also increase snowpack retention by reducing spring melt out rates (Lundquist et al., 2013). Thus, treatment outcomes relevant to the provision of key ecosystem services and resources can vary by virtue of the intrinsic relationships among the biophysical setting and vegetation characteristics.
The potential to find synergistic effects of forest treatments is further complicated by the uneven distribution of ecosystem functions in large landscapes. From a human community perspective, areas that are a high priority for wildfire risk reduction exist primarily in dry and moist mixed coniferous forests adjacent to human communities in the wildland-urban interface (WUI, Ager et al., 2021), while areas that are most important for carbon storage, habitat, and snowpack regulation occur primarily in mid- and upper-montane forests away from human communities. Consequently, while there may be potential alignment between several priority topics, this potential can be unattainable in forest landscapes where high priority ecosystem services reside in different parts of the landscape (Povak et al., 2022).
Here, we built a spatial decision support model to quantitatively examine these complex tradeoffs in space and time. We evaluated how management actions may enhance ecosystem functioning, and where forest adaptation treatments can produce synergistic effects across ecosystem conditions and functions. We applied this decision support tool to a large, fire-prone landscape in north-central Washington State in the rain shadow on the eastern slopes of the Cascade Mountain Range. We simulated 100 years of forest succession and disturbance dynamics in this landscape using a process-based forest landscape model (LANDIS-II, Scheller et al., 2007). We aimed to advance a generalizable understanding of synergies and tradeoffs deriving from potential adaptation treatments in fire-excluded forests (Hessburg et al., 2005, 2019), and to evaluate where management may be most effective for building resilient, climate-adapted social-ecological landscapes. A key advancement made by the current study was the coupling of the LANDIS-II model with a distributed hydrology-soil-vegetation-model (DHSVM; Wigmosta et al., 1994) to better identify treatment effects on snowpack and streamflow within large landscapes.
Methods
Study area
We performed this study in a large landscape (4,524 km2) in north-central Washington State. The study area is defined by the hydrologic domains of the Wenatchee and Entiat River sub-basins, an area characterized by steep elevational gradients (187–2,870 m), diverse forest types, and an historically active fire regime. The climate in this large landscape is humid continental with warm-dry summers, cold-wet winters, and most precipitation occurring as snow. To capture the ingress of wildfires from adjacent watersheds when running the landscape simulations, we included a 5-km buffer around the study domain (an additional 1,554 km2), but this area was excluded during analysis. The landscape was represented as a 90-m raster grid, and all input layers were resampled to 90-m resolution using nearest neighbor for categorical data and bilinear interpolation for continuous data.
The study landscape is composed of a diverse mix of forest and nonforest types, with grasslands, shrub steppe lands, and dry ponderosa pine (Pinus ponderosa Dougl. ex Laws.) woodlands in the lowest elevations. With increasing elevation, open woodlands give way to open and closed canopy dry mixed-conifer forests of ponderosa pine and Douglas-fir (Pseudotsuga menziesii Mirb. Franco) in the low and middle elevations, especially on dry aspects and ridgetops. In middle and upper elevations dry mixed-conifer forests yield to moist mixed-conifer forests comprised of Douglas-fir, western larch (Larix occidentalis Nutt.), and lodgepole pine (Pinus contorta Dougl.) on moist aspects and in valley bottoms. Above the moist mixed-conifer forests are subalpine forests comprising lodgepole pine, subalpine fir (Abies lasiocarpa (Hook.) Nutt.) and Engelmann spruce (Picea engelmannii Parry ex Engelm.) in pure and mixed conditions. As precipitation increases closer to the Cascade crest, composition transitions to Douglas-fir-western hemlock (Tsuga heterophylla (Raf.) Sarg.) and Douglas-fir-western redcedar (Thuja plicata Donn ex D.Don) forests, and in upper elevations with greater winter snowpack, Pacific silver fir (A. amabilis Douglas ex J.Forbes) and noble fir (A. procera Rehder) occur. Mountain hemlock (Tsuga mertensiana (Bong.) Carr.), subalpine larch (Larix lyallii Parl.), and whitebark pine (Pinus albicaulis Engelm.) cap the highest mountain peaks below upper treeline.
Urban and exurban human community patterns also vary across these broad elevational gradients, which range from 200 to 3,700-m. The highest population densities occur in the lower elevations in semiarid steppe lands, dry forests, and woodlands, which then grade into public and private forest lands, and wilderness or roadless areas in the higher elevations to the west, running all of the way to the Cascade crest. Owing to the large elevation and climatic gradients, deeply dissected topography, and a broad range of forest types (Hessburg et al., 2000), historical wildfire activity included a mix of low, moderate, and high severity regimes, and mixed severity fire was present among all forest types (Hessburg et al., 2007, 2019). Accelerated timber harvest, primarily between 1940 and 1990, and wildfire exclusion from around 1850 to the present day (Hagmann et al., 2021), led to the densification and homogenization of forests throughout the study area (Hessburg and Agee, 2003; Hessburg et al., 2005). Together, along with anthropogenic climatic changes (Dahl et al., 2023), these influences have created an urgent need for forest adaptation treatments throughout the landscape (USDA Forest Service, 2022).
Simulation modeling
We used LANDIS-II (Scheller et al., 2007) to simulate forest successional dynamics, tree growth, regeneration, and wildfire dynamics. LANDIS-II is an open-source modeling framework that allows users to choose from a variety of sub-models (“extensions”) to account for different disturbance processes, treatment scenarios, and methods of modeling successional dynamics. Competition and succession are modeled as aspatial processes that occur within each cell, while seed dispersal, fire, and treatments are simulated as spatial processes that can spread contagiously across the landscape. A summary of our modeling framework is provided below; comprehensive details about initialization, calibration, and validation of our LANDIS-II model can be found in Furniss et al. (2022a).
Modeling framework
In LANDIS-II, vegetation within each raster cell (here, 90-m resolution) is represented by one or more “cohorts” (i.e., unique species–age combinations), and the relative abundance (i.e., density) of each cohort is tracked as cohort biomass. The map of existing vegetation (circa 2020) was generated using TreeMap (Riley et al., 2021), a random forest imputed map and database of forest inventory plots that provides wall-to-wall coverage for the contiguous United States (US).
We used the Net Ecosystem Carbon and Nitrogen (NECN; Scheller et al., 2011) extension to simulate tree growth, succession, and carbon dynamics. The NECN extension models tree growth and mortality based on a combination of species-specific parameters that determine how each species responds to temperature, water, and light conditions at each site. Light availability is a dynamic, cell-level attribute that changes as the model runs and influences the growth and recruitment rates within each cell.
We modeled wildfire, prescribed (hereafter, Rx) fire, and suppression effort with the Social-Climate Related Pyrogenic Processes and their Landscape Effects (SCRPPLE; Scheller et al., 2019) extension. This fire extension is calibrated by parameterizing a model that determines fire spread probability as a function of available fuels, fire weather index (FWI), wind speed and direction, and incoming fireline intensity. Fire effects are determined by a separate severity algorithm that models mortality as a function of fire intensity, water availability, and the fire tolerance of each cohort present at each site.
Vegetation pathway groups (PWGs)
The LANDFIRE biophysical settings (BpS)1 raster was used to allocate PWGs across the study area and to assign broad vegetation types. These data correspond with vegetation types that were likely present prior to Euro-American colonization given the biogeoclimatic conditions and characteristic disturbance regime (Rollins, 2009). We used the BpS group level attribute to assign each 90-m cell to one of water, snow/ice, rock, barren, grassland, shrubland, hardwood/riparian, alpine meadow, cold forests, or mixed conifer forest. The cold forest and mixed conifer forest BpS classes were further differentiated into dry-mixed (DMC), moist-mixed (MMC), cold-dry (CDC), or cold moist (CMC) conifer forest conditions based on topographic position, aspect, and elevation.
Mixed-conifer cells were assigned to DMC below where annual precipitation was below 300 mm, and MMC where mean temperatures in July–August were below 14°C or where annual precipitation was ≥600 mm and July–September precipitation was ≥75 mm. Remaining mixed conifer forests were assigned to DMC if they were on ridges, flat areas, or south facing aspects, and MMC if they were in valley bottoms or north aspects. Cold forests were partitioned into CDC and CMC based on topographic position alone. CMC was assigned to stands in valleys, toe slopes, and north aspects. CDC was assigned to stands on ridges, south aspects, and flat areas.
Cell membership within a PWG was used in only two ways. First, climate data were downscaled from the native 4-km raster (see Climate data) using an area-weighted mean for 177 unique “ecoregion” polygons representing each PWG within each Hydrologic Unit Codes (HUC) 10 (∼20–80,000 ha; Seaber et al., 1987) watershed. Second, PWG association was used as a grouping variable when analyzing results. Otherwise, the PWG association of each cell had no bearing on the model behavior within that cell (i.e., recruitment and dispersal were determined by species in the surrounding cells, not the PWG identity of a given cell). As such, PWG identity was only loosely tied to model behavior via climate inputs.
Management patches
We developed topographically entrained management patches using a 30-m digital elevation model (DEM) from the USGS National Elevation Data repository. Patches were primarily defined using a landscape topographic template as described by Hessburg et al. (2015) to spatially allocate forest restoration treatments across the study area. This template was further subdivided by hydrologic divides, land ownership, and land use allocation. Hydrologic divides were derived from nested HUC10 (∼20–80,000 ha) and HUC12 (∼10–40,000 ha, Seaber et al., 1987). A minimum mapping unit (MMU) of 4-ha (five contiguous cells) was applied to create management treatment patches. The 10th, 50th, and 90th quantiles of patch size were 4.9 ha, 11.3 ha, and 43.7 ha, respectively. All raw and derived input spatial layers were clipped to the study area, and the spatial patches and attributes were then processed through a series of spatial intersections where the MMU was enforced at each step to best maintain the integrity of the input data.
Management scenarios
To evaluate the potential efficacy of land management actions, we developed two management scenarios: a baseline scenario (hereafter, Wildfire Only), and a landscape restoration scenario designed to simulate ongoing and planned management actions in the study domain (hereafter, Wildfire + Treatment). The logic behind the Wildfire Only scenario was that under “business-as-usual” wildfire suppression actions, where the worst 1–2% of wildfires that burn under extreme fire weather conditions evade suppression and account for the vast majority of area burned (Calkin et al., 2005; Cova et al., 2023). The remainder are typically suppressed (Calkin et al., 2015; North et al., 2015). For the Wildfire + Treatment scenario, management actions were assigned to restore climate adapted forest conditions and bolster resilience to wildfires.
For the Wildfire + Treatment scenario, we simulated a landscape-scale forest adaptation strategy designed to improve heterogeneity, reduce density, and enhance overall resilience. We implemented treatments with the Biomass Harvest extension (Gustafson et al., 2000). To do so, we partitioned the landscape into four management zones: wildlands, dry forests, moist forests, and industrial timber lands (Supplementary Figure S1; Supplementary Table S1), within which we applied different treatment prescriptions. Other land uses including water and urban/rural development were not treated.
Wildlands were primarily comprised of cold-moist and cold-dry forests on steep slopes and in the upper elevations of the study domain. The wildland management zone was delineated based on land designated as wilderness and roadless areas. Although we did not explicitly consider road accessibility when delineating the treatable areas, their designation as non-roadless and actively managed forests implies that road access is not a barrier to treatment implementation.
Treatments occurred at the patch-level, where patches were selected at random and evaluated for their eligibility for treatment (see Management Patches section above). If eligible, treatments were applied (see Simulated Treatments section below), and additional patches were selected until the target treatment rate (% area per year) was reached. Eligible patches included those outside of wildland areas, while patches located in federal wildlands (Supplementary Figure S1) were always considered ineligible. Eligible patches were not always available for treatment, as we applied a post-treatment buffer so that the same patch would not be treated again too soon following initial treatment (subsequent treatments had to be ≥10 years in dry forests, ≥30 years in moist forests). Because of this, target treatment area was not attainable in all years, but treatment rates eventually converged on roughly 4,500 ha per year (Supplementary Table S1). Patches were developed by grouping pixels that shared similar ownership, topographic setting, and potential vegetation type (here called Vegetation Pathway Groups, see section above).
Simulated treatments
Treatment types were consistent within each management zone. The following prescriptions were developed to simulate current and anticipated forest management activities for the study domain, with the necessary simplifications for implementation with LANDIS-II. We developed these management scenarios based on expert opinion and input from State and Federal partners.
In dry forests, treatments were designed to reduce surface fuels, retain large trees, and to shift composition toward climate- and fire-adapted species. This was achieved by thinning from below, removing 90% of young stems (<50 years old) of all species and 80% of mature stems (50–120 years old) of shade-tolerant species (all species except Pinus ponderosa, P. monticola, and Larix occidentalis). Trees older than 120 years old were not thinned. Slash and non-live surface fuels were reduced by 90% to simulate post-harvest broadcast burning. Treatments were applied to adjacent patches until treatment areas were between 20 and 100 ha in size to reduce wildfire contagion across multiple patches. Target treatment area was 3% ∙ yr.−1 to achieve fuel reduction efforts as quickly as possible.
In moist forest, treatments were designed to increase heterogeneity of forest successional conditions and diversify habitat by creating small gaps and openings. This involved moderate to high intensity thinning (up to 75% canopy cover reduction) among intermediate aged stems of all species (<120 years old) in small spatially disjunct openings within patches (<3 ha). Maximum size of any opening was set at 30% of any treated patch. Surface fuels were reduced by 50% to simulate slash piling and burning. The target treatment area was set at 1% ∙ yr.−1 of available patches to maintain a sustainable level of biomass extraction throughout the 100-yr simulation.
Industrial forest lands were treated by means of clearcutting on a 35–50-year rotation. The treatment rate was set at 4% ∙ yr.−1, and surface fuels were reduced by 50% to represent slash piling and burning.
21st century climate data
We used the MACAv2-METDATA CCSM4 dataset, a gridded dataset containing daily climate projections at a 4-km resolution for the contiguous US (Abatzoglou and Brown, 2012). We elected to use this single climate forecast to isolate possible variability due to uncertainty in future climate. This climate dataset contains projections through 2099; for the years 2,100–2,120, we resampled the years 2080–2099 using a temporally autocorrelated resampling procedure to extend the climate data through the end of our simulation period.
We used the RCP 8.5 emission scenario, as recent evidence suggests that it is appropriate scenario for representing likely climate warming in this domain through the end of the 21st century (Schwalm et al., 2020). In a companion study, we evaluate climate change effects against a baseline “no change” climate scenario (Furniss et al., 2024) to assess the impacts of climate change, but those results were omitted from the present study so that we could focus on spatial variability in treatment effects.
Hydrology modeling
To model the effects of wildfire and forest adaptation treatments on snowpack retention and streamflow, we used the Distributed Hydrology Soil Vegetation Model (DHSVM; Wigmosta et al., 1994). DHSVM is one of a select few hydrology models that can simulate the multitude of vegetation-soil-hydrology dynamics at fine (90-m) spatial scales. The model simulates snow interception by forest canopies, canopy shading and snowmelt, evapotranspiration, soil water storage, and runoff. We note that DHSVM is a spatial hydrology model rather than a coupled climate-vegetation model, so it therefore cannot simulate the effects of altered evapotranspiration (e.g., forest treatments) on future precipitation. This does not pose a significant limitation for our purposes the vast majority of precipitation that falls on the Cascade Range originates in the Pacific Ocean.
We introduced temporal variability in the DHSVM vegetation layer by updating four key vegetation parameters annually: leaf area index (LAI), canopy height (HT), fractional cover (FC), and vegetation class. These parameters output from LANDIS-II at decadal time steps (determined based on the “succession” timestep), so we temporally interpolated maps to an annual resolution by means of linear ramp functions. LANDIS-II does not directly calculate HT and FC. To satisfy these DHSVM inputs, we built a generalized linear model using Forest Inventory and Analysis (FIA) data to predict HT and FC as a function of LANDIS-II-derived species composition, age, biomass, and biophysical setting. We used generalized linear mixed effects models, implemented with the lme4 package in R (Bates et al., 2015). We modeled fractional coverage as a linear function of stand biomass, and we modeled canopy height as a linear function of stand age and stand biomass. Both models contained random slopes and intercepts for vegetation pathway group.
The DHSVM model was driven by meteorology data derived from the 1/16 degree Livneh dataset (Livneh et al., 2015). The initial snow parameters were calibrated using snow water equivalent (SWE) observations from nearby SNOTEL stations. The model was then further calibrated using streamflow records (USGS gauges 12,456,500, 1,245,800) for the Wenatchee and Entiat sub-basins from the water years 1997–2003 and 1966–1971, respectively. Future climate forecasts were derived from the MACAv2-LIVNEH climate dataset (Abatzoglou and Brown, 2012). This dataset was selected because the projections were based on the same Livneh dataset used to calibrate DHSVM (Livneh et al., 2015), and the future trends tracks (but does not precisely match) the MACAv2-METDATA dataset used with our LANDIS-II model.
Decision support model development
To characterize and compare the effects of each management scenario on ecosystem services and resources, we defined the following seven Primary Topic areas: Sustainable Biomass, Economics, Carbon, Water, Wildfire, Forest Health, and Landscape Integrity. These topic areas were chosen to represent a diversity and breadth of ecosystem services that are of key concern for managers and stakeholders in the western US (Staudinger et al., 2012; Nelson et al., 2013; Weiskopf et al., 2020). We then developed a set of indicator metrics which were used to quantify current conditions, future trends, and treatment effects on ecosystem condition for each Primary Topic area (Table 1). These seven Primary Topics were also grouped into three broad, Resilience Topics (Ecological, Social, and Economic) to represent the potential of each management scenario to produce benefits to social-ecological resilience.
We generated a spatial decision support system (DSS) using maps of each metric at the beginning and end of the simulation. We used ramp functions to transform the native units of each metric into a “strength-of-evidence” (SOE) score. These SOE scores represented the degree to which a value-based premise (e.g., more carbon storage is desirable) was supported. SOE scores ranged from −1 to +1, where +1 indicated strong support for more favorable conditions (e.g., high carbon storage), while −1 indicated no support for the premise (e.g., low carbon storage). The cutoff values (thresholds for −1 and 1) for the ramp functions used to score SOE for each metric were set at the 10th and 90th quantile for current condition data (i.e., at simulation year 0). This range was established to represent a common range of conditions (the median 80% range) when considering the full range of the data for each metric. Values less than the 10th percentile reflected no support for the premise, while values greater than the 90th percentile reflected full support for the premise. Values between the 10th and 90th percentile formed the ramp function.
We chose the simulation midpoint, year 2070, to assess treatment effects on simulated future landscapes. We chose this year because it provided a long enough period to treat the entirety of the treatable landscape at a realistic rate (1–4% ∙ yr.−1), and to potentially reveal any delayed ecological impact of treatments.
We calculated SOE scores separately for current conditions, future trend in the absence of treatment (difference between year 50 and year 0 under the Wildfire Only scenario), and for treatment effects (difference between year 50 under each treatment scenario and year 50 under the Wildfire Only scenario). For the future trend, we centered the SOE scores so that an SOE = 0 indicated no difference, positive values indicate that conditions improved by year 50, and negative values indicated that conditions worsened over time. For the treatment efficacy scores, positive values indicated the treatment scenario produced greater benefits compared to the baseline scenario at the same point in time (2070, simulation midpoint). These spatial SOE scores were averaged across all metrics in each topic area to generate maps of potential treatment efficacy among the seven Primary Topics of a scenario.
Ordinations
We assessed the degree of alignment between the seven Primary Topics using Nonmetric Multidimensional Scaling (NMDS) ordinations. We used the metaMDS() function from the vegan R package (Oksanen et al., 2022), with Bray-Curtis distance and 99 maximum random starts. In each patch, we used the SOE scores for current conditions, future trend, and treatment efficacy among each of the Primary Topic areas to generate the dissimilarity matrix used in ordination. We used patch-level covariates including vegetation type, land use allocation, and topographic position to plot centroids and ellipses around patches with similar biophysical characteristics. In the resulting ordinations, axes represent the two primary dimensions of dissimilarity, according to the Primary Topic SOE scores for each stand. Stands with similar scores will be close together, while stands with disparate scores will be further apart.
Treatment efficacy by biophysical setting
We evaluated the effects of watershed- and patch-scale biophysical attributes on Overall treatment efficacy using simple linear models and pair-wise boxplot comparisons using Wilcoxon rank-sum tests. Watershed-level variables included HUC12 area, total area treated, total area burned, proportion area treated, and proportion area burned. Patch-scale variables included vegetation pathway group, topographic position, and land ownership.
Software and model calibration
Landscape simulations were generated with LANDIS-II v7.0 (Scheller et al., 2007) using the NECN v6.8 (Scheller et al., 2011), SCRPPLE v3.2.2 (Scheller et al., 2019), and Biomass Harvest v4.4 (Gustafson et al., 2000) extensions. All data pre-processing, post-processing, and subsequent analyses were performed in R v4.1.3 (R Core Team, 2020) using the terra, whitebox, vegan, and tidyr packages. We performed extensive calibration and validation of our application of the LANDIS-II model before performing this study. Full details regarding model development and testing may be found in Furniss et al. (2022a).
Results
Maps of current conditions (simulation year 0) revealed intuitive patterns prior to any trend analysis. For example, many ecosystem services, including those subtopics bundled under the Carbon, Water, and Forest Health primary topics (Table 1), were spatially concentrated in the mid- to upper-elevations, and in wildlands unaffected by past logging but significantly affected by a fire exclusion history (Figure 1). This lack of fire over the past century allowed forests to densify and invade former wet and dry meadows and shrublands producing an uncharacteristic (Hagmann et al., 2021) abundance of forest cover, above normal NPP and net ecosystem exchange values, and related carbon accumulation (Supplementary Figures S2–S4). Water conditions were also best in the upper elevations where fire frequency tended to be lower and snow accumulation was greatest leading to greater snow water relative to lower elevations. Higher quality forest health conditions were also greatest at these elevations owing to a relative lack of past timber harvest, greater abundance of old growth forest patches and those with large old trees, reduced drought, better conifer seed rain after disturbances, and broader heterogeneity of forest successional conditions (Table 1).
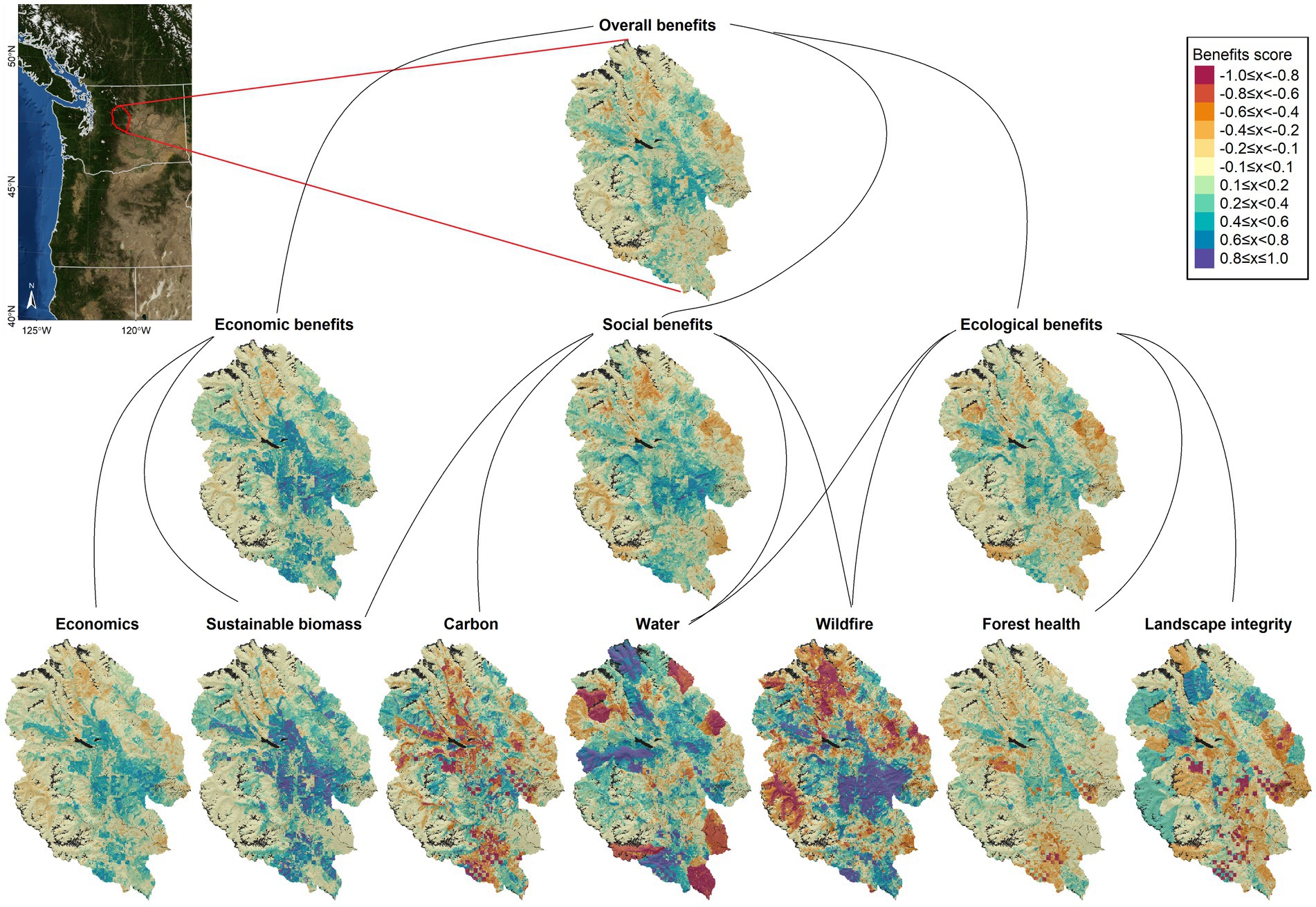
Figure 1. Spatial distribution of potential treatment efficacy (i.e., difference in the benefits score between the Wildfire + Treatment scenario and the Wildfire Only scenario after 50 years of treatment). Cool colors indicate positive benefits and high treatment efficacy (i.e., treatment scenario was better), neutrals indicate no difference, and warm colors indicate that treatments made conditions worse (i.e., Wildfire Only scenario was better). Figure 4 depicts where treatments were applied.
Future trends relative to current conditions were also intuitive, with most forests increasing in carbon storage, especially in younger patches. These gains were particularly notable in young to mature forests at mid-elevations where biomass was projected to accumulate rapidly in the absence of continued harvest (Supplementary Figure S2). Snowpack and stream flows were projected to decrease throughout the century, with the most significant declines occurring in upper elevation watersheds (Supplementary Figure S3). Forest health was projected to increase over time in both upper and lower elevations, while mid-elevations showed a decline in forest health in the absence of treatment (Supplementary Figure S4).
Maps of treatment efficacy showed the highest potential efficacy in areas with intermediate current and future conditions (Figure 1; Supplementary Figures S2–S4). Areas that were projected to improve significantly over time in the absence of treatment had reduced potential for treatments to be effective, while areas that were projected to decline also had reduced capacity for treatments to help. Potential treatment efficacy among Economics, Sustainable biomass, and Wildfire topics was concentrated in the WUI and in actively managed parts of the landscape where most mechanical treatments could be focused (Figure 1). Conversely, treatment efficacy for Carbon, Water, and Landscape Integrity, was highest in middle and upper elevation wilderness and roadless areas (north and west parts of the landscape), where wildfire as a treatment was only possible. Forest health was best optimized at mid-elevations where the landscape was more successionally heterogeneous, heavily forested, and distance to seed source after disturbances tended to be shortest.
When taken together, Overall benefits were well-distributed throughout the landscape (Figure 1). They were not easily characterized by ownership or biophysical setting (Figures 2, 3; Supplementary Figures S5, S6). Instead, they were determined by a combination of direct treatment effects and indirect neighborhood benefits associated with reductions in severe wildfire behavior (Figures 4, 5). Economic benefits were concentrated in actively managed forests, especially in private industrial forests managed exclusively for timber production. These private industrial timber lands were most abundant in the central and southern portions of the landscape, in areas dominated by mixed-conifer forests (both moist and dry) at mid to low elevations (Supplementary Figure S1). Potential treatment benefits Overall and among Social and Ecological topics were also greatest in mid-elevation mixed conifer forests (Figures 6, 3), where closer examination revealed differences in the land ownerships (hence, treatment types) that produced the greatest benefits for these topics (Figure 6; Supplementary Figure S6). The Social topic category included secondary topics that required tradeoffs such as Carbon storage and Wildfire risk reduction, where high SOE scores associated with aggressive wildfire risk reduction were offset by the reduced capacity for carbon storage. These tradeoffs created a somewhat muddied map where high SOE scores were found across all four management zones (including untreated areas; Figures 1, 6). The Ecological topic area also included Forest health and Landscape integrity categories, whose SOE scores were greatest in patches that experienced non-stand replacing wildfire or were treated with adaptation treatments.
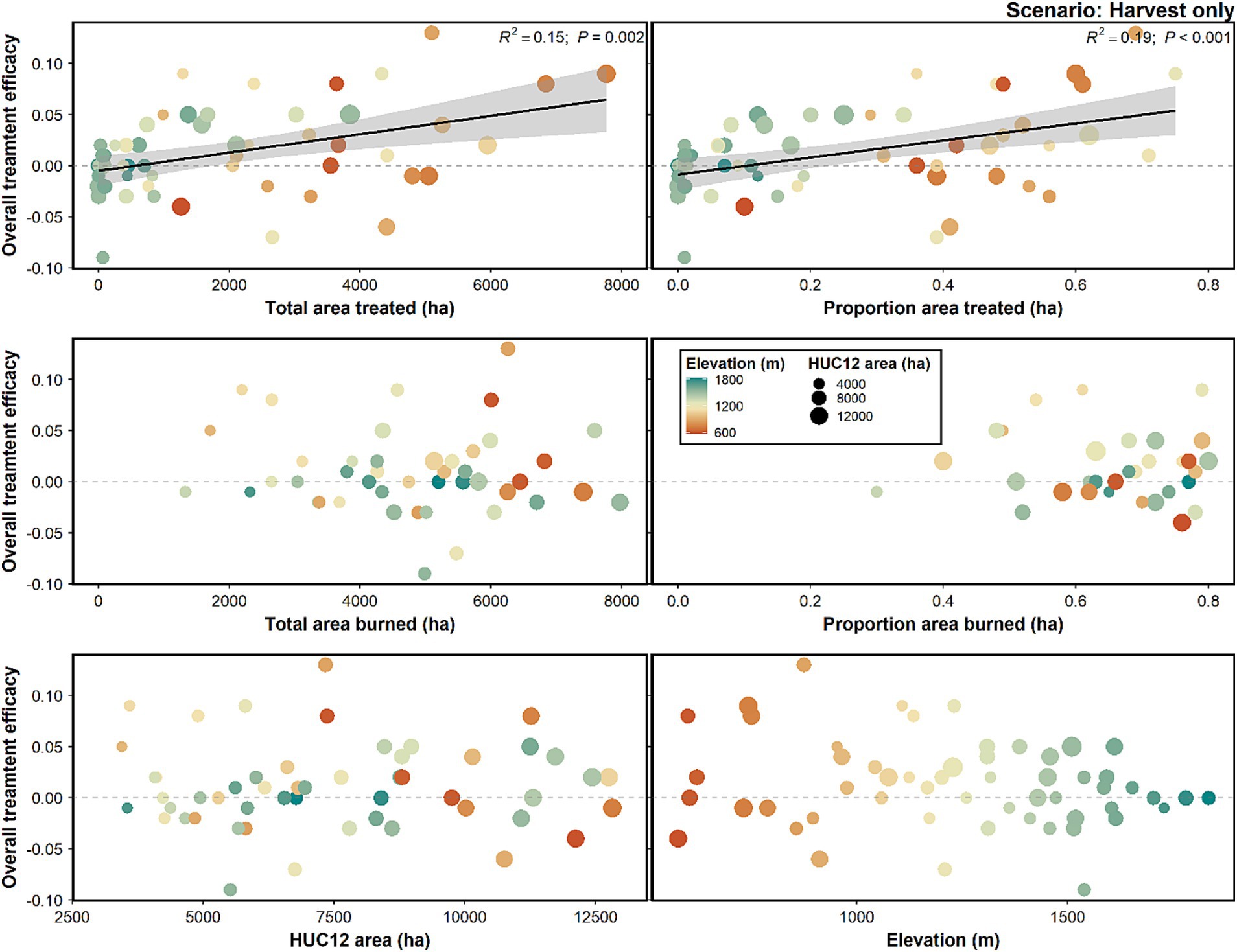
Figure 2. Strength of evidence scores for overall treatment efficacy (top panel in Figure 1). Points represent individual HUC12s, within which overall treatment efficacy was averaged. These scores were plotted against area treated, area burned, HUC12 area, and elevation.
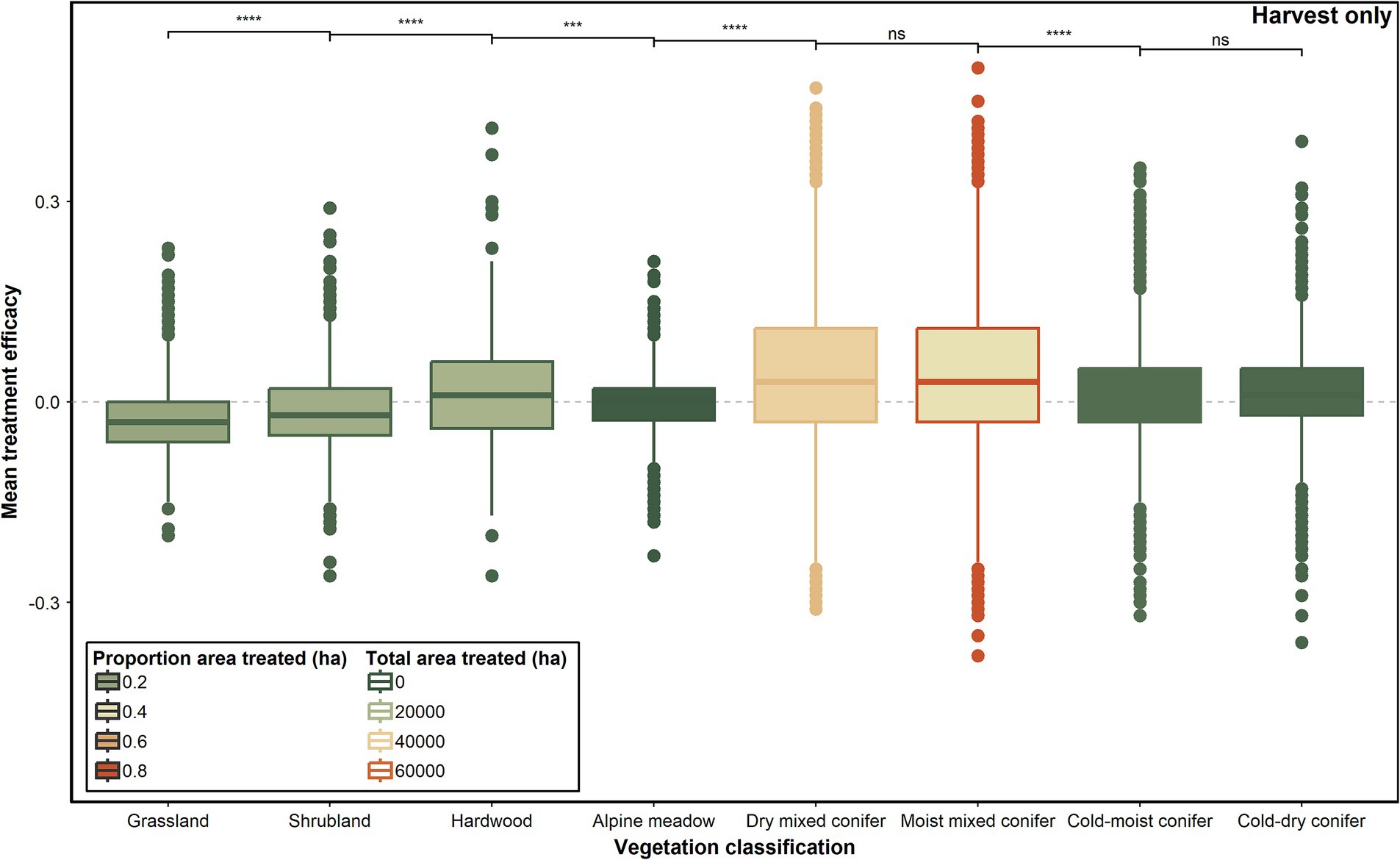
Figure 3. Overall treatment efficacy by vegetation pathway group. Points represent individual patches. Positive treatment efficacy indicates that the Wildfire + Treatment scenario performed better than the Wildfire Only scenario, while negative values indicate that the treatment scenario made conditions worse.
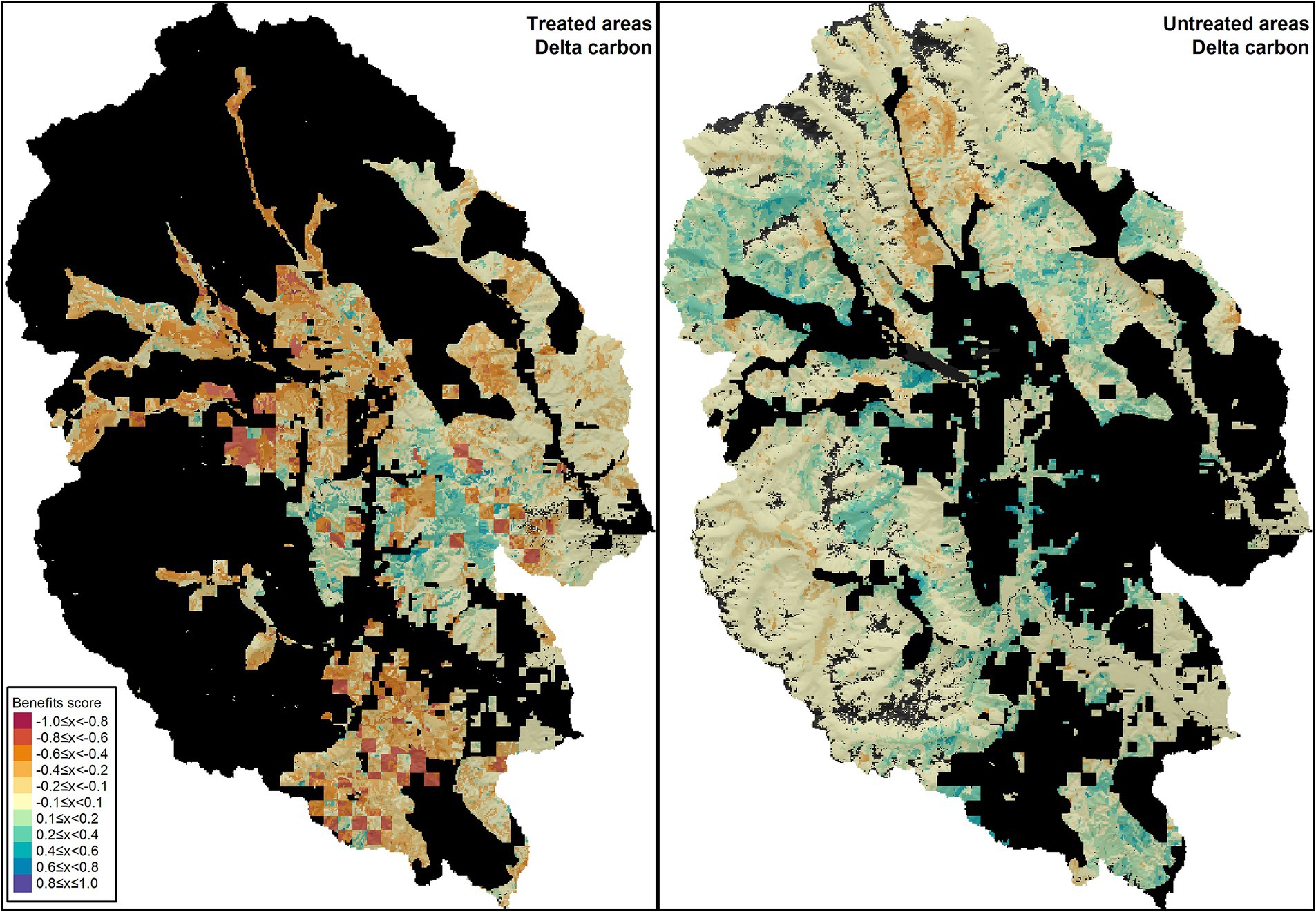
Figure 4. Neighborhood effects on carbon storage comparing managed (left) and wildlands (right). Red indicates less carbon, blue indicates more carbon.
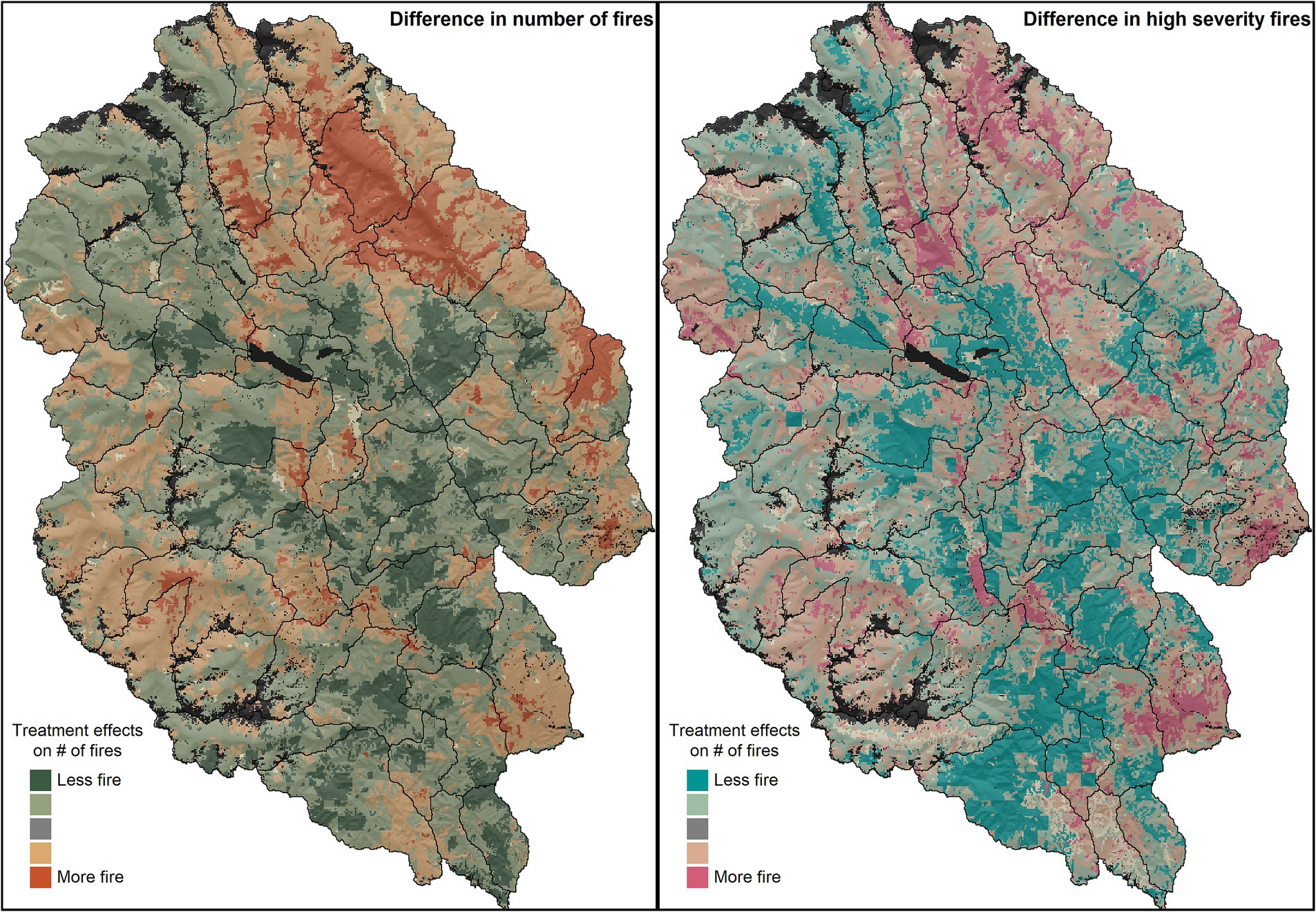
Figure 5. Treatment effects on fire frequency (left) and high severity fire frequency (right). The maps depict delta fire activity, defined here as the difference in number of fires between the baseline Wildfire Only scenario and the Wildfire + Treatment scenario.
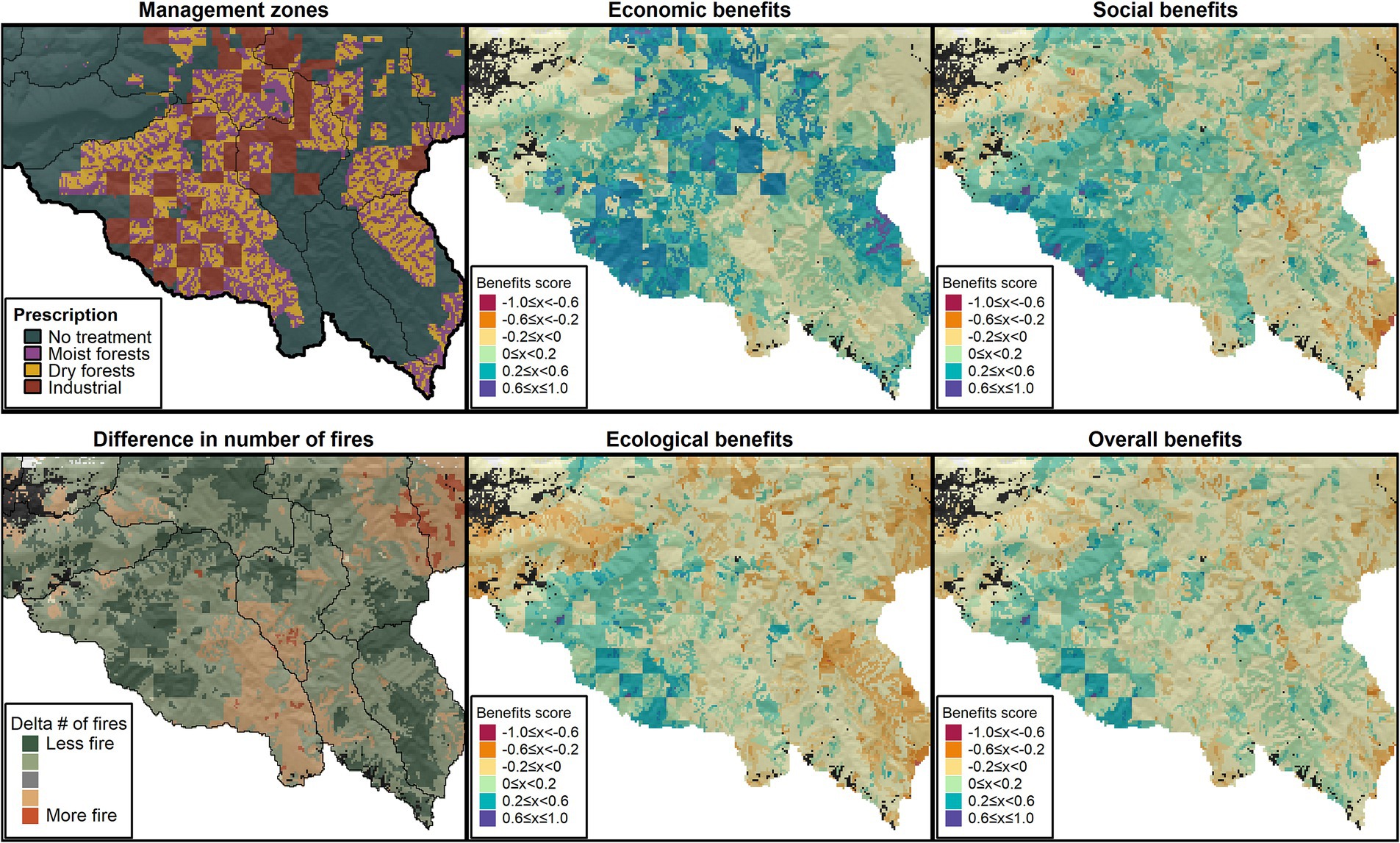
Figure 6. Zoom to southern portion of the study domain showing treatment types applied based on land ownership allocation and forest type (top left), difference in number of times burned (bottom left), and treatment effects (“Benefits score”) among Primary Topics (center and right panels).
Much of the ecological functioning in Social and Ecological topic areas (e.g., carbon storage, snowpack retention) resided in federal wildlands where mechanical treatments were not applied; wildfire therefore played the primary role in rendering benefits among these topics. As such, treatments not only delivered important proximal effects within treated patches, but also had significant distal impacts on forest, fuels, and wildfire contagion (neighborhood benefits) in untreated areas.
Neighborhood benefits
A major advantage of spatial simulation models is that neighborhood effects of treatments may be observed in nearby untreated patches. For the Wildfire + Treatment scenario, no active management was applied in public wildlands (i.e., no harvesting, and identical levels of fire suppression as the Wildfire Only scenario), yet treatment benefits were evident in these areas as well due to both long- and short-range neighborhood effects (Figure 4). We detected both positive and negative effects of treatments in untreated areas, with positive effects primarily occurring adjacent to treated areas, and negative effects occurring in more distant areas. Potential carbon storage was reduced by treatments within the treated patches (mechanical thinning reduced aboveground biomass), but these losses were more than offset by increases in carbon storage in adjacent untreated areas (Figure 4, left column). Adaptation-oriented treatments on public lands were designed to reduce potential fire severity, fire spread rates, and risk transmission to adjacent lands, and neighborhood effects were likely facilitated by these treatments. In other words, treatments reduced the connectivity and potential contagion of fire of the landscape, and this reduced fire risk even among untreated stands. A review of maps of wildfire burned area supported this observation, showing that mechanical treatments in only 40% of the landscape influenced patterns of wildfire frequency and severity across the entire landscape (Figure 5).
Ordinations
NMDS ordinations further contextualized these results (Figure 7). Relative to current conditions, wildfire-driven improvements to the Water topic occurred at the highest elevations, comprising mainly cold-dry and cold-moist forests in (untreated) federal wildlands (Figure 7). Wildfire risk was greatest in low elevation forests with high canopy bulk density. Other topics were more clustered in the middle, with watershed size, ownership, and topographic position contributing to their different positions in ordination space.
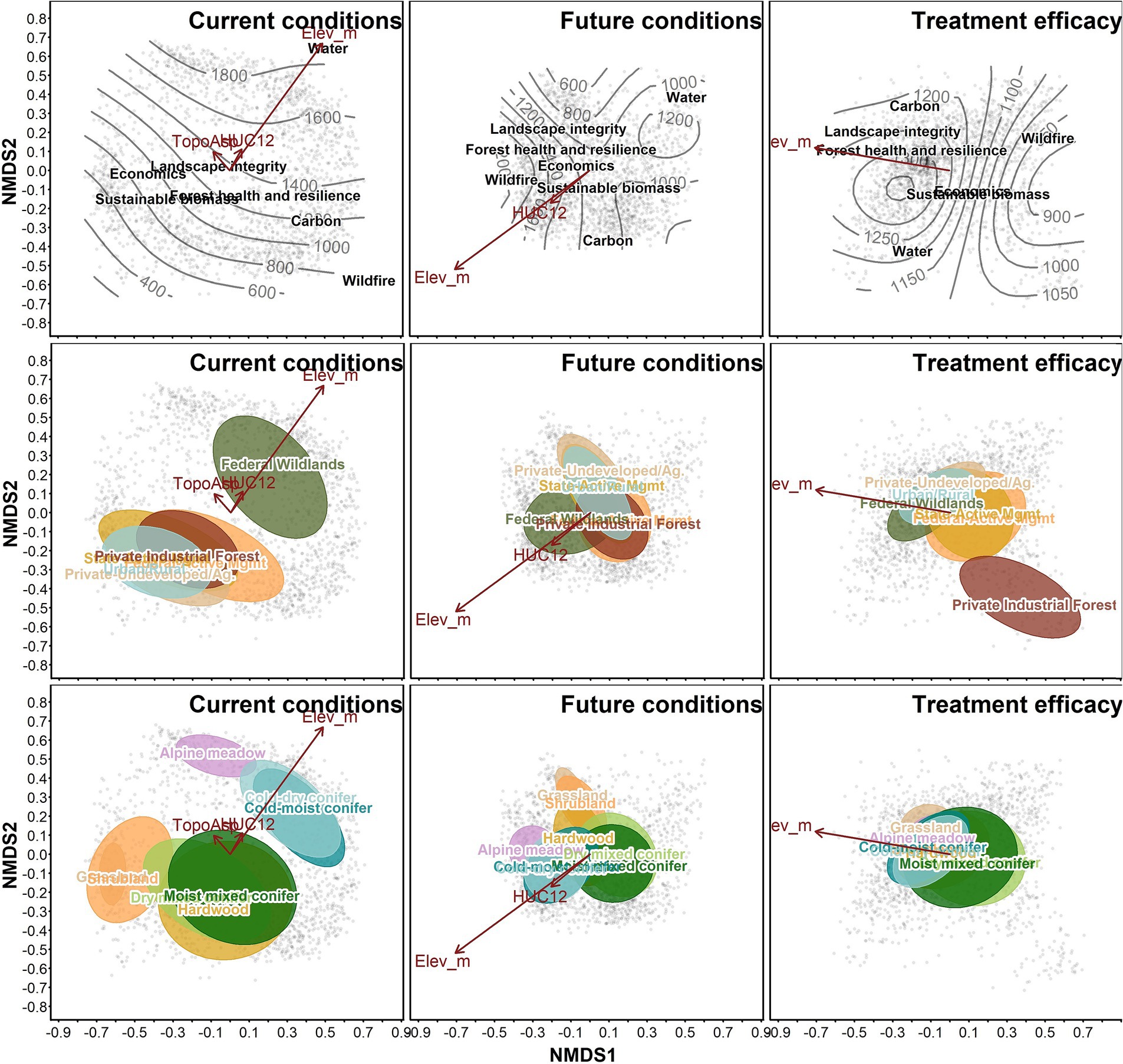
Figure 7. Ordinations of treatment efficacy by Primary Topic. Each point represents a patch, with treatment efficacy among the seven primary topics used as “species” for the NMDS ordination. Columns represent current conditions, future conditions, and potential treatment efficacy, respectively. Contours in the top row indicate elevation. Vector lines represent correlations with elevation (“Elev_m”), topographic position (“TopoAsp”), and HUC12 area (“HUC12”). Polygons in the middle and bottom row represent the centroid and standard deviation of categorical environmental co-variates including land ownership (middle row) and vegetation pathway group (bottom row).
These associations of Primary Topics changed when we analyzed future conditions under climate change. Sustainable biomass, and Economic gains were aligned with mid-elevation conditions, in actively managed forests. Wildfire risk was lowest in upper elevations in federal wildlands, while Carbon was greatest in moist mixed-conifer and private industrial forests, where forests had the greatest potential to accumulate biomass in the Wildfire Only scenario. Trends under the Water topic in the context of climate change were neither aligned with other topics, nor with any vegetation types, elevation belts, or ownership categories. This underscores climate change predictions for later in the 21st century that future snowpack and stream flows will decrease across the entire landscape (Mote et al., 2018; Supplementary Figure S3).
Ordination of treatment effects revealed potential synergy and tradeoffs between benefits among the Primary Topics. Although the spatial distribution of high treatment efficacy was somewhat nuanced and unanticipated (Figure 5), those findings were consistent for multiple topics which yielded intuitive groupings of Primary Topics in ordination space. Sustainable Biomass, and Economics were closely aligned, as were Landscape integrity and Forest health. Water, Wildfire risk, and Carbon pinned the edges of the ordination space, indicating that tradeoffs will likely be necessitated to improve conditions among those three topics (Figure 7). Treatment efficacy showed a high degree of overlap among ownership categories, except for private industrial forests, which was isolated from other land ownership classes and Primary Topic centroids.
Projected future conditions with and without management
With respect to future conditions, benefit scores show high treatment efficacy, which was calculated as the potential for simulated treatments to produce benefits in comparison to the baseline Wildfire Only scenario. That is, benefits were not necessarily aligned with the degree of current or future ecosystem functioning. For example, carbon storage was highest in wilderness and roadless areas at upper elevations, and carbon remained high in those areas in the future scenarios (including Wildfire Only). Treatment efficacy for improved carbon storage, in contrast, was higher in the more fire-prone forests at low and mid-elevations, where treatments reduced severe fire risk (Figure 5), thereby increasing potential carbon storage compared to the Wildfire Only scenario (Supplementary Figure S2). This finding highlighted that treatments led to short-term losses in carbon storage, which were later compensated for by long-term gains due to reduced fire impacts and other stand dynamics related causes of mortality.
Treatment efficacy increased with area treated
Overall treatment efficacy was positively related to both total and proportional area treated (Figure 2). High area treated was more common in low to mid-elevation watersheds (HUC12 units), largely due to ownership patterns on the landscape (Supplementary Figure S1). Proportion area treated was also closely related to elevation, as high elevation watersheds tend to be smaller due to steep and highly dissected terrain and less treatable due to access and land ownership allocation (wilderness or roadless). Neither area burned, watershed area, nor elevation were related to overall treatment efficacy. Land ownership, topographic position, and vegetation pathway group had detectable effects on treatment efficacy, although the effect sizes were small (Figure 3; Supplementary Figures S5, S6). Treatment efficacy was highest in dry mixed conifer and moist mixed conifer zones (Figure 3), and on actively managed federal lands (which were primarily composed of dry and mixed conifer forests; Supplementary Figure S6).
Discussion
Tradeoffs and synergies were evident among all topics. SOE scores were strongest at the Primary Topic level, with scores averaging out to result in more intermediate values when we examined Economic, Social, Ecological, and Overall priority areas (Figure 1). The reduction in SOE indicates the presence of tradeoffs, which are inevitable with such diverse topic areas. Areas of alignment did exist, however, primarily in mid-elevation areas where treatments were concentrated and most patches were treatable (Figure 1; Supplementary Figure S1). This is where treatment efficacy was highest because forests were most responsive to forest adaptation treatments (Figure 1), and where neighborhood effects were strongest (Figure 4).
Clearcutting in private industrial timber lands produced the strongest benefits for Economic topic areas, but as would be expected, these treatments were less effective at producing improved benefit scores for Social and Ecological topics. All treatments reduced wildfire risk, but we did not observe that this effect was dependent on treatment intensity (clearcutting versus restorative thinning). Private industrial lands delivered similar levels of wildfire risk reduction as did nearby public lands treated with fuel-reduction treatments, but the clearcut treatments applied on private lands were less effective at producing benefits among other topic categories (Figure 6). Industrial plantations have a place on the forested landscape where fiber production and economic returns are the primary management objective, but these results revealed that adaptation-oriented treatments were better at accomplishing wildfire risk reduction in conjunction with diverse ecosystem benefits.
Forest adaptation treatments aimed at wildfire risk reduction, increasing successional heterogeneity, and restoring landscape resilience produced the intended effects, generally reducing wildfire risk and increasing benefits for the surrounding patches. Because of this, the greatest levels of treatment synergy across topic areas were often observed not in treated patches, but in patches that existed within proximity to those that were treated. These untreated patches benefited from the reduction in fire spread and severity offered by adjacent treated areas while retaining higher levels of carbon, resulting in higher Social, Ecological, and Overall benefits scores (Figure 6).
The existence of neighborhood benefits was a key finding, demonstrating the need to take a holistic and multi-scale approach to landscape restoration and adaptation. This provides evidence that the strategic placement of treatments can effectively impede fire flow (e.g., Stevens et al., 2016), but further work will be necessary to determine how to optimize the spatial allocation of treatments to maximize these potential neighborhood benefits among multiple topic areas. Treating individual patches resulted in relatively intuitive treatment effects within patches, but the more widespread effects were the ways that treatments moderated wildfire activity on over half of the landscape that was not treatable by mechanical methods (Figures 4, 5). This point is especially salient in large, wildland-dominated landscapes that are commonplace throughout the intermountain west. Landscape resilience is not simply the sum of treatment effects in treated areas. True ecological resilience will require more holistic thinking about dynamic disturbance regimes, restabilizing patterns at large to small scales, and re-establishing the ecological feedback mechanisms that operate at fine, meso-, and broad-scales (sensu Hessburg et al., 2019). Such information is crucial as efforts to increase the pace and scale of management across the western US continue.
Our results further suggest that monitoring of treatment impacts needs to consider not only proximal patch-level impacts of treatments but also broader neighborhood effects as treated areas begin to mount over space and time. Direct evidence of landscape-level benefits of treatments are difficult to assess in situ, but simulation models are particularly adept at capturing these dynamics and showing how treatments across a fraction of the landscape can influence broader landscape-level patterns and processes (Stevens et al., 2016).
A feature of our modeling approach is that treatment in one patch will influence dynamics in neighboring patches. As such, the benefit scores that we generated do not correlate perfectly with “priority for restoration,” as many of the areas with high benefit scores are themselves untreatable. And in treatable stands, the benefit score cannot be solely attributed to the treatment applied in that stand, as it was not possible to differentiate direct treatment effects and neighborhood benefits in treated stands. Instead, these benefit scores should be considered as meso-scale information about where on the landscape the greatest potential for improving resource benefits exists. More tactical prioritization and field evaluation methods can be used to determine the sequence of treated patches and to inform optimal treatment staging.
Our simulation methods revealed several tradeoffs and synergies among ecosystem functions. Wildfire and Carbon were hyperdispersed in ordination space, reinforcing the idea that managing for wildfire risk reduction and carbon storage requires tradeoffs (Figure 7). Surprisingly, Water and Wildfire were misaligned as well. One may have expected more synergy between Water and Wildfire as both should have been benefited by the density reduction associated with forest adaptation treatments (Lundquist et al., 2013; Vogler et al., 2015; Furniss et al., 2022b), but this misalignment reflects a spatial segregation of these two topics on the landscape. High treatment efficacy for wildfire risk reduction occurred in low and middle elevations, yet these areas had low treatment efficacy for Water because these low elevation areas are where snowpack is projected to experience significant declines (Mote et al., 2018; Supplementary Figure S3).
Carbon storage was aligned with Landscape Integrity and Forest Health, as all three of these topics valued old trees and high patch biomass. Economics and Sustainable Biomass were also well aligned, landing near the Wildfire topic but at slightly higher elevations. This grouping in the NMDS ordinations confirms the known potential for synergy between wildfire risk reduction and economically viable thinning treatments (Ager et al., 2016).
Neither watershed area nor elevation were related to overall treatment efficacy (Figure 2). The most likely explanation for this is that there were spatial tradeoffs within the treated landscape that balanced overall treatment gains related to the untreated landscape (Figure 1). Some areas were enhanced while other areas were hampered, and these divergent SOE scores averaged out when we examined overall benefits as a function of watershed size and elevation. Treated area (both total and proportion) did increase overall treatment efficacy, and our results suggest that treating over 2,000 ha and at least 20% of the area within an individual HUC12-level watershed was necessary to produce even minimal positive treatment benefits (Figure 2). This is consistent with other studies that have found treatment rates of 20–40% are needed to mediate wildfire spread and severity on large landscapes (Finney, 2007; Povak et al., 2023). Area burned was not related to treatment efficacy, likely because area burned included low-, moderate-, and high-severity fire, which would yield divergent impacts conferred by fire.
One source of uncertainty that we were not able to examine in this study is the relative influence of different management and future climate scenarios on future ecosystem function. Comparing several scenarios with a spatial DST yielded an overwhelming amount of information. To present the results of multiple management scenarios, we prepared a companion study (Furniss et al., 2024) that distilled results for each pathway group, enabling us to evaluate differences between management scenarios over time. In another study, Maxwell et al. (2022) explored the relative influence of different climate scenarios on future trajectories using a similar LANDIS-II model application. In this study, we isolated a single climate projection and a single management scenario to make our results digestible, enabling us to focus on the spatial variability in treatment efficacy and neighborhood benefits associated with treatments.
Integrating potential climate change impacts into strategic landscape planning will be key to help managers make climate informed solutions and determine where investments can be made on the landscape with the highest likelihood of sustained success. While many climate-informed management frameworks have been developed (e.g., Schuurman et al., 2022), few offer the ability to analyze tradeoffs associated with treating forest patches versus allowing natural disturbances to drive forest development and wildfire dynamics. Here, we parsed the problem of identifying treatment needs into three facets: where are resources currently deficient (i.e., current conditions), what is the trajectory of a given resource benefit over time under climate change and natural disturbances (i.e., future trajectory), and where is there evidence that treatments can improve conditions compared to a no-treatment future (i.e., treatment efficacy). This information can be used to partition the landscape into landscape priorities. For example, areas that show improvement over time under natural disturbances can be assigned a low priority for treatment. Conversely, areas that show a steady decline in ecosystem service provisioning over time can be assigned higher treatment priority, with the highest priority assigned to areas where management investments are projected to be most effective. Povak et al. (2024) provide a quantitative framework for evaluating current and potential future conditions using a similar simulation modeling platform. Our future work will explore how integrating treatment efficacy into this framework can further assist management in targeting treatment areas that can sustain ecosystem functions, where they are currently operable but declining, and where treatment has the highest potential to improve conditions over time.
Conclusion
Landscape simulation models offer a way to mechanistically represent a multitude of social-ecological processes shaping forest ecosystems over large spaces and long timespans. By coupling such a model with a distributed hydrology model, we developed novel methodology for simulating the effects of vegetation dynamics, management actions, and climate effects on future snowpack and streamflow dynamics. We synthesized the myriad of data layers resulting from these complex models using a spatial decision support tool to assess future trajectories and potential treatment benefits among 40 different metrics comprising seven Primary Topics areas representing concepts of significant social-ecological value and concern.
Wildfire area burned was projected to increase over time, and this trend was only partially offset by forest adaptation treatments. Overall treatment efficacy was highest in dry and moist mixed conifer forests on actively managed federal lands, an unsurprising result that lends credibility to the modeling framework. Treatments had strong neighborhood benefits, where fire spread and severity were reduced not only in treated patches but in adjacent patches as well. Tradeoffs and synergies were evident in maps of treatment efficacy, with the greatest potential treatment efficacy being found in mid-elevation areas where future conditions were projected to be somewhat worse, but where these trends were mild enough that treatments were able still confer significant benefits. Ordinations provided further evidence for potential treatment synergy, and they revealed surprising tradeoffs as well, primarily due to the high degree of spatial heterogeneity in ecosystem functioning within our large study landscape. These results contribute to improved understanding of synergies and tradeoffs linked to adaptation and restoration efforts in fire-prone forests, and may be used in strategic planning to guide where management may be most effective for building resilient, climate-adapted social-ecological landscapes.
Data availability statement
Publicly available datasets were analyzed in this study. This data can be found here: all datasets used in this manuscript are publicly available via the citations provided throughout the manuscript.
Author contributions
TF: Conceptualization, Data curation, Formal analysis, Investigation, Methodology, Visualization, Writing – original draft, Writing – review & editing. NP: Conceptualization, Writing – review & editing, Methodology, Supervision. PH: Conceptualization, Funding acquisition, Supervision, Writing – review & editing, Methodology. RS: Conceptualization, Methodology, Writing – review & editing. ZD: Formal analysis, Methodology, Writing – review & editing. MW: Conceptualization, Methodology, Supervision, Writing – review & editing, Funding acquisition.
Funding
The author(s) declare financial support was received for the research, authorship, and/or publication of this article. This work was partially funded the US Department of Energy (USDOE) Bioenergy Technologies Office (DE-SC0017519/0005) and supported through an appointment of the senior author to the Research Participation Program at the USDA-FS, Pacific Northwest Research Station (PNWRS), administered by the Oak Ridge Institute for Science and Education (ORISE) through an interagency agreement between the USDOE and the PNWRS.
Acknowledgments
We thank two reviewers for comments on a previous draft of this manuscript. Any use of trade, firm, or product names is for descriptive purposes only and does not imply endorsement by the U.S. Government.
Conflict of interest
The authors declare that the research was conducted in the absence of any commercial or financial relationships that could be construed as a potential conflict of interest.
Publisher’s note
All claims expressed in this article are solely those of the authors and do not necessarily represent those of their affiliated organizations, or those of the publisher, the editors and the reviewers. Any product that may be evaluated in this article, or claim that may be made by its manufacturer, is not guaranteed or endorsed by the publisher.
Author disclaimer
The views of the authors expressed herein do not represent the official views of the USDOE, ORISE, or the USDA-FS.
Supplementary material
The Supplementary material for this article can be found online at: https://www.frontiersin.org/articles/10.3389/ffgc.2023.1269081/full#supplementary-material
Footnotes
References
Abatzoglou, J. T., and Brown, T. J. (2012). A comparison of statistical downscaling methods suited for wildfire applications. Int. J. Climatol. 32, 772–780. doi: 10.1002/joc.2312
Abelson, E. S., Reynolds, K. M., Manley, P., and Paplanus, S. (2021). Strategic decision support for long-term conservation management planning. For. Ecol. Manag. 497:119533. doi: 10.1016/j.foreco.2021.119533
Ager, A. A., Day, M. A., Ringo, C., Evers, C. R., Alcasena, F. J., Houtman, R. M., et al. (2021). Development and application of the fireshed registry. Washington, DC: United States Department of Agriculture, Forest Service.
Ager, A. A., Day, M. A., and Vogler, K. (2016). Production possibility frontiers and socioecological tradeoffs for restoration of fire adapted forests. J. Environ. Manag. 176, 157–168. doi: 10.1016/j.jenvman.2016.01.033
Badgley, G., Freeman, J., Hamman, J. J., Haya, B., Trugman, A. T., Anderegg, W. R. L., et al. (2022). Systematic over-crediting in California’s forest carbon offsets program. Glob. Chang. Biol. 28, 1433–1445. doi: 10.1111/gcb.15943
Barnosky, A. D., Hadly, E. A., Bascompte, J., Berlow, E. L., Brown, J. H., Fortelius, M., et al. (2012). Approaching a state shift in Earth’s biosphere. Nature 486, 52–58. doi: 10.1038/nature11018
Bates, D., Mächler, M., Bolker, B., and Walker, S. (2015). Fiting linear mixed-effects models using lme4. J. Stat. Softw. 67. doi: 10.18637/jss.v067.i01
Bennett, E. M., Peterson, G. D., and Gordon, L. J. (2009). Understanding relationships among multiple ecosystem services: relationships among multiple ecosystem services. Ecol. Lett. 12, 1394–1404. doi: 10.1111/j.1461-0248.2009.01387.x
Bisson, P. A., Rieman, B. E., Luce, C., Hessburg, P. F., Lee, D. C., Kershner, J. L., et al. (2003). Fire and aquatic ecosystems of the western USA: current knowledge and key questions. For. Ecol. Manag. 178, 213–229. doi: 10.1016/S0378-1127(03)00063-X
Calkin, D. E., Gebert, K. M., Jones, J. G., and Neilson, R. P. (2005). Forest service large fire area burned and suppression expenditure trends, 1970–2002. J. For. 103, 179–183. doi: 10.1093/jof/103.4.179
Calkin, D. E., Thompson, M. P., and Finney, M. A. (2015). Negative consequences of positive feedbacks in US wildfire management. Forest Ecosyst. 2:9. doi: 10.1186/s40663-015-0033-8
Clarke, H., Nolan, R. H., De Dios, V. R., Bradstock, R., Griebel, A., Khanal, S., et al. (2022). Forest fire threatens global carbon sinks and population centres under rising atmospheric water demand. Nat. Commun. 13:7161. doi: 10.1038/s41467-022-34966-3
Coop, J. D., Parks, S. A., Stevens-Rumann, C. S., Crausbay, S. D., Higuera, P. E., Hurteau, M. D., et al. (2020). Wildfire-driven forest conversion in Western North American landscapes. Bioscience 70, 659–673. doi: 10.1093/biosci/biaa061
Cova, G., Kane, V. R., Prichard, S., North, M., and Cansler, C. A. (2023). The outsized role of California’s largest wildfires in changing forest burn patterns and coarsening ecosystem scale. For. Ecol. Manag. 528:120620. doi: 10.1016/j.foreco.2022.120620
Dahl, K. A., Abatzoglou, J. T., Phillips, C. A., Ortiz-Partida, J. P., Licker, R., Merner, L. D., et al. (2023). Quantifying the contribution of major carbon producers to increases in vapor pressure deficit and burned area in western US and southwestern Canadian forests. Environ. Res. Lett. 18:064011. doi: 10.1088/1748-9326/acbce8
Dale, V. H., Joyce, L. A., Mcnulty, S., Neilson, R. P., Ayres, M. P., Flannigan, M. D., et al. (2001). Climate change and Forest disturbances. Bioscience 51:723. doi: 10.1641/0006-3568(2001)051[0723:CCAFD]2.0.CO;2
Davies, K. K., Fisher, K. T., Dickson, M. E., Thrush, S. F., and Le Heron, R. (2015). Improving ecosystem service frameworks to address wicked problems. Ecol. Soc. 20. doi: 10.5751/ES-07581-200237
Dickerson-Lange, S. E., Vano, J. A., Gersonde, R., and Lundquist, J. D. (2021). Ranking forest effects on snow storage: a decision tool for forest management. Water Resour. Res. 57. doi: 10.1029/2020WR027926
Eriksson, M., Safeeq, M., Pathak, T., Egoh, B. N., and Bales, R. (2022). Using stakeholder-based fuzzy cognitive mapping to assess benefits of restoration in wildfire-vulnerable forests. Restorat. Ecol. 31:e13766. doi: 10.1111/rec.13766
Finney, M. A. (2007). A computational method for optimising fuel treatment locations. Int. J. Wildland Fire 16:702. doi: 10.1071/WF06063
Franklin, J. F., and Johnson, K. N. (2012). A restoration framework for federal forests in the Pacific northwest. J. For. 110, 429–439. doi: 10.5849/jof.10-006
Furniss, T. J., Das, A. J., Mantgem, P. J., Stephenson, N. L., and Lutz, J. A. (2022b). Crowding, climate, and the case for social distancing among trees. Ecol. Appl. 32:e2507. doi: 10.1002/eap.2507
Furniss, T. J., Hessburg, P. F., Povak, N. A., Salter, R. B., and Wigmosta, M. S. (2022a). Predicting future patterns, processes, and their interactions: benchmark calibration and validation procedures for forest landscape models. Ecol. Model. 473:110099. doi: 10.1016/j.ecolmodel.2022.110099
Furniss, T. J., Povak, N. A., Hessburg, P. F., Salter, R. B., Duan, Z., and Wigmosta, M. S. (2024). Simulated wildland fire use builds resilient landscapes. Front. For. Glob. Climate Change In review
Gustafson, E. J., Shifley, S. R., Mladenoff, D. J., Nimerfro, K. K., and He, H. S. (2000). Spatial simulation of forest succession and timber harvesting using LANDIS. Can. J. For. Res. 30, 32–43. doi: 10.1139/x99-188
Hagmann, R. K., Hessburg, P. F., Prichard, S. J., Povak, N. A., Brown, P. M., Fulé, P. Z., et al. (2021). Evidence for widespread changes in the structure, composition, and fire regimes of western North American forests. Ecol. Appl. 31:e02431. doi: 10.1002/eap.2431
Hessburg, P. F., and Agee, J. K. (2003). An environmental narrative of inland Northwest United States forests, 1800–2000. For. Ecol. Manag. 178, 23–59. doi: 10.1016/S0378-1127(03)00052-5
Hessburg, P. F., Agee, J. K., and Franklin, J. F. (2005). Dry forests and wildland fires of the inland Northwest USA: contrasting the landscape ecology of the pre-settlement and modern eras. For. Ecol. Manag. 211, 117–139. doi: 10.1016/j.foreco.2005.02.016
Hessburg, P. F., Charnley, S., Gray, A. N., Spies, T. A., Peterson, D. W., Flitcroft, R. L., et al. (2021). Climate and wildfire adaptation of inland northwest US forests. Front. Ecol. Environ. 20, 40–48. doi: 10.1002/fee.2408
Hessburg, P. F., Churchill, D. J., Larson, A. J., Haugo, R. D., Miller, C., Spies, T. A., et al. (2015). Restoring fire-prone inland Pacific landscapes: seven core principles. Landsc. Ecol. 30, 1805–1835. doi: 10.1007/s10980-015-0218-0
Hessburg, P. F., Miller, C. L., Parks, S. A., Povak, N. A., Taylor, A. H., Higuera, P. E., et al. (2019). Climate, environment, and disturbance history govern resilience of western North American forests. Front. Ecol. Evol. 7:239. doi: 10.3389/fevo.2019.00239
Hessburg, P. F., Salter, R. B., and James, K. M. (2007). Re-examining fire severity relations in pre-management era mixed conifer forests: inferences from landscape patterns of forest structure. Landsc. Ecol. 22, 5–24. doi: 10.1007/s10980-007-9098-2
Hessburg, P. F., Salter, R. B., Richmond, M. B., and Smith, B. G. (2000). Ecological subregions of the interior Columbia Basin, USA. Appl. Veg. Sci. 3, 163–180. doi: 10.2307/1478995
Krofcheck, D. J., Hurteau, M. D., Scheller, R. M., and Loudermilk, E. L. (2018). Prioritizing forest fuels treatments based on the probability of high-severity fire restores adaptive capacity in Sierran forests. Glob. Chang. Biol. 24, 729–737. doi: 10.1111/gcb.13913
Livneh, B., Bohn, T. J., Pierce, D. W., Munoz-Arriola, F., Nijssen, B., Vose, R., et al. (2015). A spatially comprehensive, hydrometeorological data set for Mexico, the US, and southern Canada 1950–2013. Scientific data 2, 1–12. doi: 10.1038/sdata.2015.42
Loehman, R. A., Reinhardt, E., and Riley, K. L. (2014). Wildland fire emissions, carbon, and climate: seeing the forest and the trees – a cross-scale assessment of wildfire and carbon dynamics in fire-prone, forested ecosystems. For. Ecol. Manag. 317, 9–19. doi: 10.1016/j.foreco.2013.04.014
Lundquist, J. D., Dickerson-Lange, S. E., Lutz, J. A., and Cristea, N. C. (2013). Lower forest density enhances snow retention in regions with warmer winters: a global framework developed from plot-scale observations and modeling. Water Resour. Res. 49, 6356–6370. doi: 10.1002/wrcr.20504
Magnani, F., Mencuccini, M., Borghetti, M., Berbigier, P., Berninger, F., Delzon, S., et al. (2007). The human footprint in the carbon cycle of temperate and boreal forests. Nature 447, 849–851. doi: 10.1038/nature05847
Maron, M., and Cockfield, G. (2008). Managing trade-offs in landscape restoration and revegetation projects. Ecol. Appl. 18, 2041–2049. doi: 10.1890/07-1328.1
Maxwell, C., Scheller, R. M., Long, J. W., and Manley, P. (2022). Forest management under uncertainty: the influence of management versus climate change and wildfire in the Lake Tahoe Basin, USA. Ecol. Soc. 34 doi: 10.5751/ES-13278-270215
Millar, C. I., Stephenson, N. L., and Stephens, S. L. (2007). Climate change and forests of the future: managing in the face of uncertainty. Ecol. Appl. 17, 2145–2151. doi: 10.1890/06-1715.1
Mote, P. W., Li, S., Lettenmaier, D. P., Xiao, M., and Engel, R. (2018). Dramatic declines in snowpack in the western US. NPJ Climate Atmos. Sci. 1:2 doi: 10.1038/s41612-018-0012-1
Nelson, E. J., Kareiva, P., Ruckelshaus, M., Arkema, K., Geller, G., Girvetz, E., et al. (2013). Climate change’s impact on key ecosystem services and the human well-being they support in the US. Front. Ecol. Environ. 11, 483–893. doi: 10.1890/120312
North, M. P., Stephens, S. L., Collins, B. M., Agee, J. K., Aplet, G., Franklin, J. F., et al. (2015). Reform forest fire management. Science 349, 1280–1281. doi: 10.1126/science.aab2356
Oksanen, J., Simpson, G. L., Blanchet, F. G., Kindt, R., Legendre, P., and Minchin, P. R., et al. (2022). vegan: Community Ecology Package
Povak, N. A., Furniss, T. J., Hessburg, P. F., Salter, R. B., Wigmosta, M., Duan, Z., et al. (2022). Evaluating basin-scale forest adaptation scenarios: wildfire, streamflow, biomass, and economic recovery synergies and trade-offs. Front. For. Glob. Change 5:805179. doi: 10.3389/ffgc.2022.805179
Povak, N. A., Hessburg, P. F., Salter, R. B., Prichard, S. J., and Gray, R. W. (2023). System-level feedbacks of active fire regimes in large landscapes. Fire Ecol. 19. doi: 10.1186/s42408-023-00197-0
Povak, N. A., Manley, P. N., and Wilson, K. N. (2024). Integrating climate adaptation strategies in spatial decision support systems. Front. For. Glob. Climate Change In review
Prichard, S. J., Hessburg, P. F., Hagmann, R. K., Povak, N. A., Dobrowski, S. Z., Hurteau, M. D., et al. (2021). Adapting western North American forests to climate change and wildfires: 10 common questions. Ecol. Appl. 31:e02433. doi: 10.1002/eap.2433
R Core Team (2020). R: A language and environment for statistical computing. R Foundation for Statistical Computing: Vienna, Austria
Reynolds, K. M., Hessburg, P. F., Lakicevic, M., Povak, N. A., Salter, R. B., Sullivan, T. J., et al. (2023). Assessing impacts of sulfur deposition on aquatic ecosystems: a decision support system for the southern Appalachians. Ecosphere 14:e4507. doi: 10.1002/ecs2.4507
Riley, K. L., Grenfell, I. C., Finney, M. A., and Wiener, J. M. (2021). TreeMap, a tree-level model of conterminous US forests circa 2014 produced by imputation of FIA plot data. Sci. Data 8:11. doi: 10.1038/s41597-020-00782-x
Rollins, M. G. (2009). LANDFIRE: a nationally consistent vegetation, wildland fire, and fuel assessment. Int. J. Wildland Fire 18:235. doi: 10.1071/WF08088
Scheller, R. M., Domingo, J. B., Sturtevant, B. R., Williams, J. S., Rudy, A., Gustafson, E. J., et al. (2007). Design, development, and application of LANDIS-II, a spatial landscape simulation model with flexible temporal and spatial resolution. Ecol. Model. 201, 409–419. doi: 10.1016/j.ecolmodel.2006.10.009
Scheller, R. M., Hua, D., Bolstad, P. V., Birdsey, R. A., and Mladenoff, D. J. (2011). The effects of forest harvest intensity in combination with wind disturbance on carbon dynamics in Lake states Mesic forests. Ecol. Model. 222, 144–153. doi: 10.1016/j.ecolmodel.2010.09.009
Scheller, R., Kretchun, A., Hawbaker, T. J., and Henne, P. D. (2019). A landscape model of variable social-ecological fire regimes. Ecol. Model. 401, 85–93. doi: 10.1016/j.ecolmodel.2019.03.022
Schuurman, G. W., Cole, D. N., Cravens, A. E., Covington, S., Crausbay, S. D., Hoffman, C. H., et al. (2022). Navigating ecological transformation: resist–accept–direct as a path to a new resource management paradigm. Bioscience 72, 16–29. doi: 10.1093/biosci/biab067
Schwalm, C. R., Glendon, S., and Duffy, P. B. (2020). RCP8. 5 tracks cumulative CO2 emissions. Proc. Natl. Acad. Sci. 117, 19656–19657. doi: 10.1073/pnas.2007117117
Seaber, P. R., Kapinos, F. P., and Knapp, G. L.. (1987). Hydrologic unit maps. US Government Printing Office: Washington, DC
Seidl, R., Spies, T. A., Peterson, D. L., Stephens, S. L., and Hicke, J. A. (2016). Searching for resilience: addressing the impacts of changing disturbance regimes on forest ecosystem services. J. Appl. Ecol. 53, 120–129. doi: 10.1111/1365-2664.12511
Staudinger, M. D., Grimm, N. B., Staudt, A., Carter, S. L., and Chapin, F. S.. (2012). Impacts of climate change on biodiversity, ecosystems, and ecosystem services. United States Global Change Research Program: Washington, DC 139
Stevens, J. T., Collins, B. M., Long, J. W., North, M. P., Prichard, S. J., Tarnay, L. W., et al. (2016). Evaluating potential trade-offs among fuel treatment strategies in mixed-conifer forests of the Sierra Nevada. Ecosphere 7:e01445. doi: 10.1002/ecs2.1445
Tempel, D. J., Gutiérrez, R., Battles, J. J., Fry, D. L., Su, Y., Guo, Q., et al. (2015). Evaluating short-and long-term impacts of fuels treatments and simulated wildfire on an old-forest species. Ecosphere 6, 1–18. doi: 10.1890/ES15-00234.1
Underwood, E. C., Viers, J. H., Quinn, J. F., and North, M. (2010). Using topography to meet wildlife and fuels treatment objectives in fire-suppressed landscapes. Environ. Manag. 46, 809–819. doi: 10.1007/s00267-010-9556-5
USDA Forest Service (2022). Confronting the wildfire crisis: a strategy for protecting communities and improving resilience in America’s forests : Washington, DC: USDA Forest Service. 25.
Vogler, K. C., Ager, A. A., Day, M. A., Jennings, M., and Bailey, J. D. (2015). Prioritization of forest restoration projects: tradeoffs between wildfire protection, ecological restoration and economic objectives. Forests 6, 4403–4420. doi: 10.3390/f6124375
Walker, X. J., Baltzer, J. L., Cumming, S. G., Day, N. J., Ebert, C., Goetz, S., et al. (2019). Increasing wildfires threaten historic carbon sink of boreal forest soils. Nature 572, 520–523. doi: 10.1038/s41586-019-1474-y
Weiskopf, S. R., Rubenstein, M. A., Crozier, L. G., Gaichas, S., Griffis, R., Halofsky, J. E., et al. (2020). Climate change effects on biodiversity, ecosystems, ecosystem services, and natural resource management in the United States. Sci. Total Environ. 733:137782. doi: 10.1016/j.scitotenv.2020.137782
Wigmosta, M. S., Vail, L. W., and Lettenmaier, D. P. (1994). A distributed hydrology-vegetation model for complex terrain. Water Resour. Res. 30, 1665–1679. doi: 10.1029/94WR00436
Keywords: carbon, climate change, decision support systems, forest landscape models, ecological modeling, LANDIS-II, landscape ecology, temperate forests
Citation: Furniss TJ, Povak NA, Hessburg PF, Salter RB, Duan Z and Wigmosta M (2023) Informing climate adaptation strategies using ecological simulation models and spatial decision support tools. Front. For. Glob. Change. 6:1269081. doi: 10.3389/ffgc.2023.1269081
Edited by:
Arshad Ali, Hebei University, ChinaReviewed by:
Christian Temperli, Swiss Federal Institute for Forest, Snow and Landscape Research (WSL), SwitzerlandDavid Ellison, University of Bern, Switzerland
Copyright © 2023 Furniss, Povak, Hessburg, Salter, Duan and Wigmosta. This is an open-access article distributed under the terms of the Creative Commons Attribution License (CC BY). The use, distribution or reproduction in other forums is permitted, provided the original author(s) and the copyright owner(s) are credited and that the original publication in this journal is cited, in accordance with accepted academic practice. No use, distribution or reproduction is permitted which does not comply with these terms.
*Correspondence: Tucker J. Furniss, dHVja2VyLmZ1cm5pc3NAdXd5by5lZHU=