- 1College of Forestry, Fujian Agriculture and Forestry University, Fuzhou, China
- 2Southern Swedish Forest Research Center, Swedish University of Agricultural Sciences, Lomma, Sweden
Aims: The aim of this study was to analyze changes in emission of water-soluble ions in fine particulate matter over time and in different southwest forest areas in China based on China’s Forestry Statistical Yearbook and MODIS satellite fire point data.
Methods: We took 6 dominant tree species samples in the southwestern forest region of China and simulated combustion using controllable biomass combustion devices. Based on the spatial analysis method of ArcGIS, combining satellite fire point data and official statistical yearbooks, we analyzed the spatial and temporal dynamics of emissions of water-soluble ions in PM2.5 released by forest fires in southwestern forest areas from 2004 to 2021.
Results: The total amount of forest biomass combusted in southwest forest areas was 64.43 kt. Among the different forest types, the proportion of burnt subtropical evergreen broad-leaved forest was the largest (60.49%) followed by subtropical mixed coniferous and broad-leaved forest (22.78%) and subtropical evergreen coniferous forest (16.72%). During the study period, 61.19 t of water-soluble ions were released in PM2.5 from forest fires, and the emissions of Li+, Na+, NH4+, K+, Mg2+, Ca2+, F−, Cl−, Br−, NO3−, PO43− and SO42− were 0.48 t, 11.54 t, 2.51 t, 19.44 t, 2.12 t, 2.92 t, 1.94 t, 12.70 t, 1.12 t, 1.18 t, 1.17 t and 4.07 t, respectively. Yunnan was the province with the highest emissions of water-soluble ions in PM2.5 in the southwest forest areas, and the concentration K+ was the highest. Emission of water-soluble ions in Yunnan and Sichuan all showed a significant downward trend, while the overall decrease in Tibet, Chongqing and Guizhou was not significant. The peak emission of water-soluble ions in PM2.5 during forest fires appeared in spring and winter, which accounted for 87.66% of the total emission.
Discussion: This study reveals the spatiotemporal changes in water-soluble ion emissions from forest fires, by studying the spatiotemporal dynamics of water-soluble ions in PM2.5, we can better understand the sources, distribution, and change patterns of these ions, as well as their impact on the atmospheric environment, ecosystems, and climate change. This information is crucial for predicting and managing air pollution, as well as developing effective forest management and environmental protection policies to respond to fires; and hence concerted fire prevention efforts should be made in each province, taking into account the season with higher probability of fire occurrence to reduce the potential impact of fire-related pollutions.
Highlights
• The largest release of water-soluble ions from burning is broad-leaved forest.
• Spatio-temporal distribution of water-soluble ions in forest fires was analyzed.
• The total emission of water-soluble ions in PM2.5 was the highest in spring.
1. Introduction
With the intensification of global climate change, the frequency of forest fires and the burnt areas have shown an increasing trend in the past decades (Seidl et al., 2017; Xu et al., 2020; Kharuk et al., 2021; Prichard et al., 2021). In the context of climate change, many studies have shown a significant increase in forest fires (Jain et al., 2021), which are also a factor exacerbating air pollution and climate change (Sonwani et al., 2022). The occurrence, frequency, and intensity of forest fires are also related to constantly changing weather and climate conditions, such as rising temperatures, insufficient precipitation, increased drought days, and El Niño-Southern Oscillation (ENSO) events (Bytnerowicz et al., 2007; Jain et al., 2021). These factors may lead to an increase in fire incidence, burned areas, and increased pollutant emissions from fire activities (Larkin, 2005). Long periods of dry weather can carry away moisture from the atmosphere and soil, significantly increasing the likelihood of drought and forest fires (Prasad et al., 2008; Whitman et al., 2019). Globally, the annual forest fire area reaches 3.36 ~ 3.5 × 106 ha (Fang et al., 2018), accounting for 0.86% of the total forest area, and about 2,814 Tg of forest resources have been burnt down (Yan et al., 2006; Grillakis et al., 2022). Forest fires can break the carbon balance of forests, releasing a large amount of greenhouse gases and pollutants (Hazra and Gallagher, 2022; Iraci et al., 2022). Every year, forest fires release 38 Tg of particulate pollutants into the atmospheric environment (Hoelzemann, 2004; Giglio et al., 2006; Yan et al., 2006; Jin et al., 2017), which is harmful to the atmospheric environment and forest ecosystems (Aguilera et al., 2021), and cause mechanical damage and long-term chemical poisoning to animals and plants (Reisen et al., 2015; Val Martin et al., 2018; Wan et al., 2019).
Currently, a large number of studies have shown that the particulate matter emitted from forest fires mainly consists of organic compounds, inorganic salts, and metallic elements, among others. There are many factors that influence the composition of particulate matter, including the type and combustible content of the burning material, combustion temperature, oxygen concentration, humidity, and combustion status. Inorganic salts mainly come from the chemical reactions of aerosols and particulate matter during the combustion process, such as sulfates, nitrates, and chlorides. These inorganic salts are emitted into the atmosphere and form water-soluble ion aerosols, which pollute the atmospheric environment through interaction with water vapor and clouds (Alves et al., 2011; Hu et al., 2018). These water-soluble ions then undergo atmospheric transport and diffusion, eventually settling into forest ecosystems and adversely affecting vegetation, soil, and water bodies (Bergeron et al., 2004; Swami, 2017). Therefore, forest fires significantly affect forest structure, ecological processes, and hydrological and biogeochemical cycles (Bond and Keeley, 2005). In this situation, frequent forest fire activities may worsen air quality and seriously affect human health (Takahashi et al., 2020).
Large amounts of water-soluble ions can be carried in the particulate matter released by forest fires (Guo et al., 2020). Although the proportion of water-soluble ions in particulate matter is less than 10%, it has an important impact on the mechanism of formation, surface properties, and acidity and alkalinity of particulate matter (Wang H. et al., 2021; Yang W. et al., 2021). Water-soluble ions can be directly dissolved in water and cause direct harm to the ecological environment (Wang et al., 2002). Water-soluble ions (NO3−, SO42−, NH4+, and Cl−) have high water absorption, which affect the degree of light transmission of particulate matter in humid environments, resulting in low visibility weather and even haze weather (Chen et al., 2014). Water-soluble ions (Na+, Ca2+, and Mg2+) affect plant growth, and these salt ions cause damage to plants through ionic stress and indirect dehydration (Parvin et al., 2019; Wang X. et al., 2021). With the development of remote sensing and satellite communication technologies, the use of satellite data to study the smoke emissions and distribution of forest fires in specific areas has gradually increased (Wardoyo et al., 2011; Sahu and Sheel, 2013). However, there are few reports currently available on the emission of water-soluble ions from forest fires. Therefore, studying the pollutant emission characteristics of forest biomass burning and analyzing their temporal and spatial distribution are of great significance for forest fire prevention, air quality management, and atmospheric model simulation (He et al., 2011).
In this study, we analyzed the spatial and temporal dynamics of emissions of water-soluble ions in PM2.5 released by forest fires in southwest forest areas from 2004 to 2021. The southwest forest area is one of the “three major forest areas” in China, with high forest coverage and frequent forest fires, emitting a large amount of pollutants every year. In view of this, based on the spatial analysis method of ArcGIS, combining satellite fire point data and official statistical yearbooks, a fire dataset based on satellite observations is a convenient, easily accessible, and reliable tool for continuous monitoring of forest fires around the world (Bar et al., 2020; Yang X. et al., 2021), this paper estimated the emission of water-soluble ions in PM2.5 released by forest biomass burning in southwest forest areas, and revealed the temporal and spatial distribution of water-soluble ions. Such a study is of great significance for evaluating the impact of forest fires on the atmosphere and ecological environment.
2. Materials and methods
2.1. Overview of the study area
The study was conducted in southwest forests, which are the second largest natural forests in China that are distributed in five provinces, Chongqing, Sichuan, Yunnan, Guizhou, and Tibet (Figure 1). The area of the Southwest Forest Region is about 39 million ha, accounting for 22.6% of the country’s total forest area. The forest coverage rate is 20.2%, the forest stock is about 3.37 billion cubic meters, accounting for 1/4 of the country. The southwest forest areas are located in the high mountains and valleys, and the tree species are mainly pine and fir. The vegetation types include evergreen broad-leaved forest, evergreen coniferous forest, and mixed coniferous and broad-leaved forest. The types of tree species are complex, and there are various types of fire sources, which are frequent and hard-hit area by forest fire in China. According to the data of “China Forestry Statistical Yearbook,” from 2004 to 2021, a total of 25,092 forest fires occurred in all provinces in the southwestern forest areas, with an average annual burned area of about 1.94 × 104 hm2.
2.2. Experiment method
2.2.1. Sample collection
The vegetation types in the southwest forest areas are mainly coniferous forest and broad-leaved forest. The dominant tree species are Pnus yunnanensis, Pinus armandii, Keteleeria evelyniana, Quercus variabilis, Alnus nepalensis, and Cyclobalanopsis glaucoides. According to the ninth national forest resources inventory, their distribution and volume account for 93.44 and 92.08% of the total forest resources in the southwest forest areas (Table 1).
The test samples were collected in April 2021 from the Bijiashan Catalpa Garden Farm in Anning City, Yunnan Province, Huaguo Family Forest Farm in Chengdu City, Sichuan Province, Yongchuan State-owned Forest Farm in Chongqing City, Changpoling State-owned Forest Farm in Guiyang City, Guizhou Province, and Linzhi County Forest Farm in Tibet. Three dominant coniferous tree species were collected in coniferous forest, three dominant broad-leaved tree species were collected in the broad-leaved forest, and the above six dominant tree species were collected in coniferous broad-leaved forest.
In the forest away from the edge of the stand, five sample plots of 10 m × 10 m were randomly set, six trees of the same species were selected in each sample plot, and 20 g of branches, leaves and bark samples were collected in the upper, middle and lower parts of the tree. Collect three samples from each part, and collect 54 samples from each province. Mix all samples of the same species in the same sample plot evenly and divide them into three equal parts as combustion samples, each province has 18 combustion samples. The collected samples were dried in a 105°C drying oven to avoid the influence of moisture content on the emission factors, and the samples were packed with well-ventilated kraft envelope paper, and labeled and stored in a cool place. The samples were cut to about 4 cm length for full combustion, and the analytical balance (PY-E627, China Puyun, with an accuracy of 0.001 g) was used for accurately weigh 40 g per part for combustion testing.
Through indoor fire simulation device, the emission factors of water-soluble ions in PM2.5 released by combustion of six dominant tree species in the southwest forest areas were measured. The coniferous forest types in the southwest forest areas were represented by Pnus yunnanensis, Pnus armandii, and Keteleeria evelyniana, while the broad-leaved forest types were represented by Quercus variabilis, Alnus nepalensis and Cyclobalanopsis glaucoides. Based on the experimental measurement of various pollutant emission factors, combined with the spatial analysis of forest fire density in southwest forest areas, the emission and spatial distribution prediction of water-soluble ions in PM2.5 released by forest fires in southwest forest areas from 2004 to 2021 were obtained.
2.2.2. Determination of PM2.5 compositions
After the collected samples were dried, dust removed and weighed, a simulated combustion test was carried out in a self-designed simulated combustion device (Figure 2). The particle sampler (US, SKC-DPS) started sampling immediately after each combustion until the sample film (PTFE membrane 46.2 mm with support ring, Whatman) was full. The fully harvested sample film was wrapped with thin foil and weighed after equilibrating at room temperature for 24 h. In addition, the PM2.5 under the condition of unburned samples was collected as a blank control, and each litter was subjected to five times parallel simulated combustions under the burning state, and two filters were used to collect PM2.5 each time.
We used TSI-8533 particle analyzer (United States, accuracy 0.001 mg·m−3) to monitor the fine particles (PM2.5) emitted by fuel combustion in real time. This instrument is based on the principle of spectroscopic infrared and can monitor and record the concentration of particulate matter emitted during biomass combustion online. Before each test, the instrument uses a standard to calibrate to zero. During the test, data were debugged, recorded, saved and exported, and the recording interval was 5 s.
The concentrations of six anions (F−, Cl−, NO3−, Br−, SO42−, and PO43−) and six cations (Na+, NH4+, K+, Mg2+, Ca2+, and Li+) were determined in the water extract of the sample filter. In order to extract the water-soluble ions from the filters, the filters for gravimetric analysis were individually placed in 12 mL vials containing 10 mL of distilled deionized water (resistivity 18.2 MΩ). The vials were placed in an ultrasonic water bath and shaken with a mechanical shaker for 1 h. The extract was filtered through a microporous membrane with a pore size of 0.45 μm, and the filtrate was stored in a clean test tube at 4°C before analysis. The extract was centrifuged for 5 min and the contents of 12 water-soluble ions were determined by ICS1100 ion chromatograph (Dionex Inc., Sunnyvale, CA, United States). For the cation analyses, the instrument was equipped with an IonPacCS12A column (20 mmol/L methanesulfonic acid as the eluent), while an ASRS-4num column (25 mmol/L KOH as the eluent) was used for anions. The measurements were taken under the following conditions: column temperature: 30°C; flow rate: 1.0 mL/min; injection volume: 20 μL; flow precision <±0.1%; and flow rate maximum error 0.1%. Detection limits were 4.5 mg·L−1 for Na+, 4.0 mg·L−1 for NH4+, 10.0 mg·L−1 for K+, Li+, Mg2+, and Ca2+, 0.5 mg·L−1 for F− and Cl−, 15 mg·L−1 for PO43− and NO3−, and 20 mg·L−1 for SO42−. Standard samples are added after every 50 samples measured, and the standard concentration working range is between 1 and 1,000 ppm. Standard reference materials produced by the National Research Center for Certified Reference Materials (Beijing, China) were analyzed for quality control and assurance purposes. Data from blank samples were subtracted from the corresponding sample data after analysis (Wang et al., 2015; Guo et al., 2018).
In this paper, the carbon balance method was used to calculate the emission factor (Zhang et al., 2000). The basic assumption of this method is that the combustion of carbon in combustibles is mainly converted into the forms of CO, CO2, total hydrocarbon (THC), particulate matter, and ash carbon.
2.3. Data sources for forest fires
In this study, the official statistical data and satellite fire point data were used to calculate forest fires emissions in the southwest forest areas and analyze their temporal and spatial distribution. The area of forest fires and the area of different forest types in each province in the study area are derived from the “China Forestry Statistical Yearbook” (2004–2021) and the results of forest resource inventory in each province (China Forestry Network). Based on the results of the inventory of forest resources in each province in the study area, the proportion of the area of different forest types is used as the burning ratio, and the burning area of different forest types in different provinces is determined.
The satellite fire point data were derived from MODIS forest fire data with a high resolution (1 km), fire points with a reliability greater than 80% are selected and suitable for Chinese region (Amraoui et al., 2015). Using ArcGIS 10.7 software, combined with the Chinese vegetation functional map (Institute of Environment and Engineering in Cold and Arid Regions, Chinese Academy of Sciences, spatial resolution 1 km), the fire points in non-forest land areas were eliminated and the forest fire point data in the study area from 2004 to 2021 were extracted, including the fire start time and geographic coordinates of each fire point. In this paper, the forest biomass density refers to the research results of Ni et al. (2001) and Michel (2005) on forest resource density in China, the combustion efficiency of forest resources refers to Michel’s conclusion in East Asia, and the combustion efficiency of trees is selected by 25% in turn (Table 2).
2.4. Calculation of forest biomass burning and pollutant emissions
Forest biomass burning is calculated using the formula below (1) (Lu et al., 2011):
where Mk,i is the burning amount of the i-th forest type in k province, t; Sk is the total forest fire area in k province, km2 (“China Forestry Statistical Yearbook”); Pk,i is the burning ratio (the results of forest resource inventory in each province) of the i-th forest type in k province; Ni is the biomass density of the i-th forest, t/km2; ηi is the burning efficiency of the i-th forest type.
According to the basic data and emission factors obtained by consulting relevant statistical data and literature, the total amount of pollutants discharged is calculated by the formula below (2):
where En is the amount of polluting gas emissions, t; Mk,i is the combustion amount of the i-th forest type in k province, t; EFi is the emission factor of the i-th kind of biomass burning polluting gas, g/kg.
2.5. Temporal and spatial characteristics of pollutant emissions in the study area
In recent years, satellite data have been widely used to reveal the spatial and temporal distribution of pollutants due to their strong timeliness, high resolution, and wide coverage (Jin et al., 2022). Based on MODIS forest fire data, the grid weight method is used to calculate the grid emission intensity and spatial distribution of different pollutants in the study area (Jin et al., 2018). The specific methods were as follows:
1. We took the province as the unit, and counted the total number of fire points within the scope of each province.
2. Use ArcGIS to grid the entire study area (10 km × 10 km), we extracted the number of forest fire points in each grid, and obtained the spatial weight of each grid according to the formula below (3).
Where: Ej is the distribution weight of j grid; FCj is the number of green fire points in j grid; FCk is the total number of forest fire points in k province.
1. According to the amount of pollutants released by forest fires in each region, the grid emissions of different pollutants in the southwest forest area were obtained by combining the grid weights.
In addition, the Mann-Kandell trend test method in Python library was used to analyze the temporal trends and significance of different pollutant discharges in the southwest forest regions from 2004 to 2021.
2.6. Uncertainty analysis
The IPCC error propagation formula is an important method to evaluate the accuracy of air pollutant emission inventories, and it is widely used in the uncertainty analysis of emission inventories under different activity levels (IPCC, 1997). In this paper, the uncertainty of emission inventory is quantitatively analyzed according to the IPCC uncertainty assessment formula.
When the uncertain quantities are combined as a sum, the total uncertainty is calculated by equation (4):
Where Utotal is the overall uncertainty, Xi and Ui are the uncertain quantity and the related percentage uncertainty, respectively;
When the uncertain quantities are combined by multiplication, the total uncertainty is calculated by equation (5):
Where Ui is the percentage uncertainty associated with each quantity.
3. Results and discussion
3.1. Spatial and temporal distribution of forest fires in the southwest forest area
According to the “China Forestry Statistical Yearbook,” the temporal and spatial distribution of forest fires in the southwestern forest regions from 2004 to 2021 was obtained. The results show that the spatial distribution of forest fires in the southwestern forest area was relatively scattered (Figure 3). Except for Chongqing, which had a low density of forest fires, there were medium to high-density forest fires in other areas. Among them, southern Sichuan, southwestern Guizhou, southeastern Tibet, and the whole province of Yunnan were fire-prone areas. The results also showed that there were strong spatial differences in forest fire emissions in the forest areas of Southwest China, indicating that the spatial distribution of forest fires in the forest areas of Southwest China is uneven. This is in line with the research conclusions of Su et al. (2015) and Tian et al. (2013).
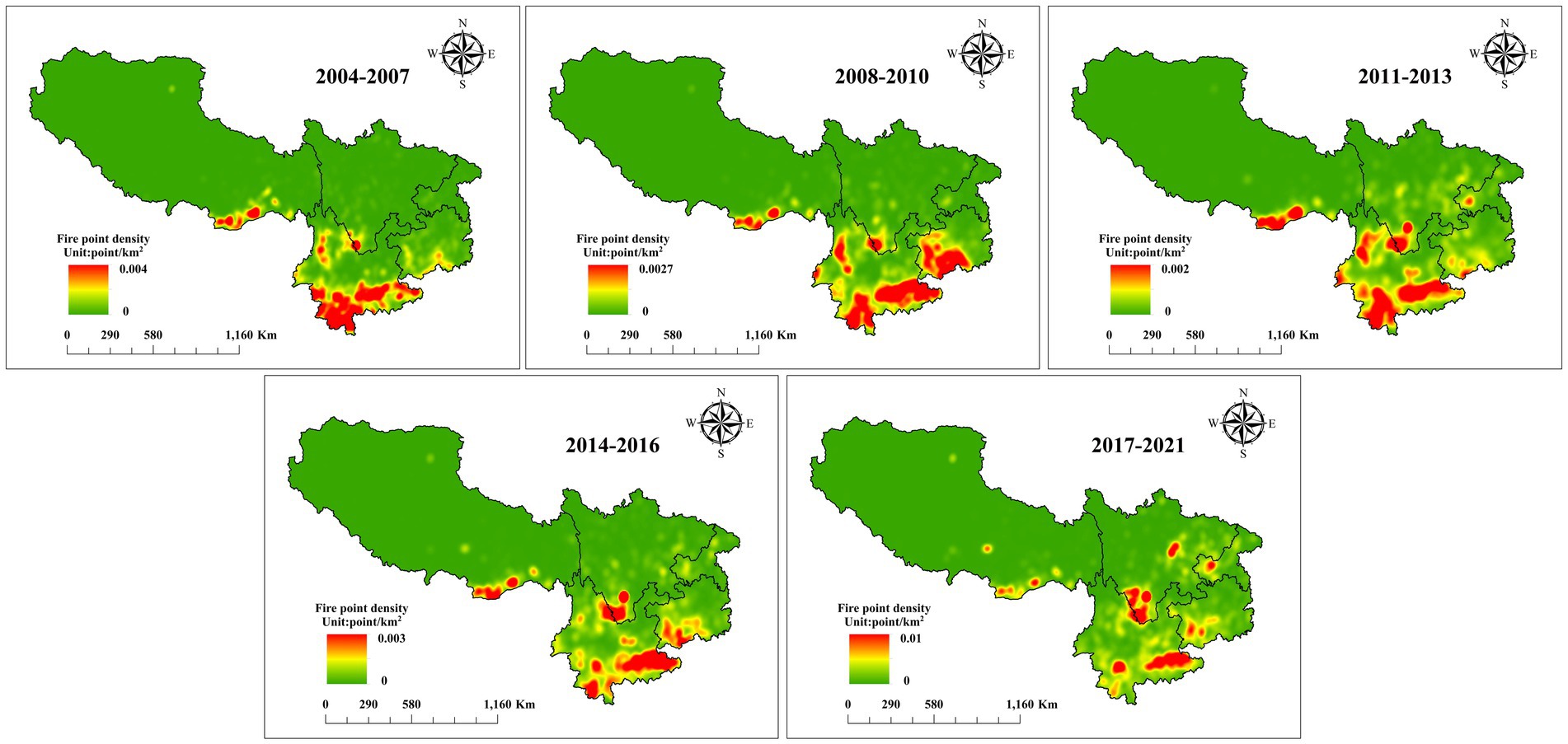
Figure 3. Schematic diagram of forest fire density in southwest forest area from 2004 to 2021. In order to reveal the dynamic changes in forest fire density, the entire time scale was divided into five times spans (2004–2007, 2008–2010, 2011–2013, 2014–2016, and 2017–2021).
The spatiotemporal distributions of the number and area of forest fires in each province in the southwestern forest region are shown in Figure 4. The number and area of forest fires differed greatly in different regions. A total of 25,092 forest fires occurred in the southwest forest area in 18 years, with a total area of 3.49 × 105 hm2. Although there were fluctuations in the number and area of forest fires in each province, the overall trend was decreasing. Among them, the number and area of forest fires in Chongqing and Guizhou decreased significantly, while only the number of forest fires in Yunnan decreased significantly. In addition, there were significant differences in forest fire intensity in different provinces. The province with the highest number of forest fires was Guizhou, accounting for 53.04%. The province with the highest forest fire area was Yunnan, accounting for 42.44%. The forest fire intensity in Tibet was the lowest, and the proportion of forest fire frequency and area was less than 1%.
The comprehensive results show that the southwest forest area is a high-incidence area of forest fires in China, and the distribution is scattered, and there are high-density forest fire areas in all provinces. Wang et al. (2019) studied the characteristics and driving factors of forest fires in the forest areas of Southwest China and concluded that the forest fire in the southwest forest area is strongly affected by climate and topographic factors. The terrain of the southwest forest area is complex, and fires are mainly distributed in mountainous and forest ecosystems, especially those in alpine forests, the humus layer is relatively thick, there are more combustibles under the forest, and the increase in biomass accumulation and frequent human activities are the main reasons for the frequent occurrence and dispersion of forest fires in the area (Sun et al., 2022; Babu et al., 2023). Understanding the geographical distribution of fires helps predict and warn of high incidence periods and high-risk areas of fires, and protecting and managing mountain and forest ecosystems is crucial for reducing fire risk (Aragão et al., 2023). This enables local governments and emergency services to take necessary measures, such as vegetation management measures, clearing of combustibles, establishing fire prevention lines, etc., to maintain the health of these ecosystems and reduce the occurrence and spread of fires (Terrier et al., 2013; Molina et al., 2019).
3.2. Emission of water-soluble ions in PM2.5 released by combustion of different forest types
The emission factors of water-soluble ions in PM2.5 emitted by combustion of different forest types in the southwestern forest area are shown in Table 3. The concentrations of Na+ and K+ were the dominant water-soluble ions in PM2.5, followed by Cl− emission, which accounted for 33.66, 19.36, and 19.07% of the total water-soluble ions, respectively. These ions in forest fire particulate matter mainly come from natural plants, with high levels of Na+, K+, and Cl− in trees and plant tissues (Huang et al., 2023). When a fire breaks out, these ions are released by plant combustion. In addition, the soil also contains Na+, K+, and Cl−. When a fire burns down vegetation and comes into contact with the soil, these ions in the soil will also be released into the smoke. The high-temperature flame of forest fires can promote the evaporation and release of Na+, K+, and Cl− from plants. At high temperatures, these ionic compounds can decompose and enter the flue gas in a gaseous form (Ma et al., 2021), which explains why the concentration of these ions is relatively high during fires. In addition, the emissions of total water-soluble ion of different forest types were 0.8725 g/kg in subtropical evergreen coniferous forests, 0.9808 g/kg in subtropical evergreen broad-leaved forests, and 0.9259 g/kg in subtropical evergreen broad-leaved mixed forests. The subtropical evergreen broad-leaved forest were the forest types with the highest emission factors of water-soluble ions in PM2.5, followed by the subtropical coniferous and broad-leaved mixed forest, indicating that the pollution from burning of broadleaf trees was greater than that of coniferous tree species. Water-soluble ions are important chemical components of particulate matter, which can reflect the formation mechanism of particulate matter and affect the surface quality (Wang et al., 2003) and acidity and alkalinity of particulate matter (Ye et al., 2003).
3.3. Forest biomass burning and emissions of water-soluble ion in PM2.5
The amount of forest biomass burning caused by forest fires in the southwest forest area from 2004 to 2021 is shown in Table 4. A total of 64.43 kt of forest resources were burned in the southwest forest area, among which Yunnan had the highest degree of burning of forest resources, accounting for 52.94%, followed by Guizhou (43.12%), Sichuan (1.69%), Chongqing (1.67%), and Tibet (0.57%). In addition, different forest types have different degrees of burning in different regions. The burning proportions of evergreen coniferous forest, evergreen broad-leaved forest, and coniferous and broad-leaved mixed forest were 16.72, 60.49, and 22.78%, respectively. Among them, the evergreen broad-leaved forest was the most severely burned forest type in the provinces in the study area, and the burning ratio ranged from 0.45% (Tibet) to 34.42% (Yunnan).
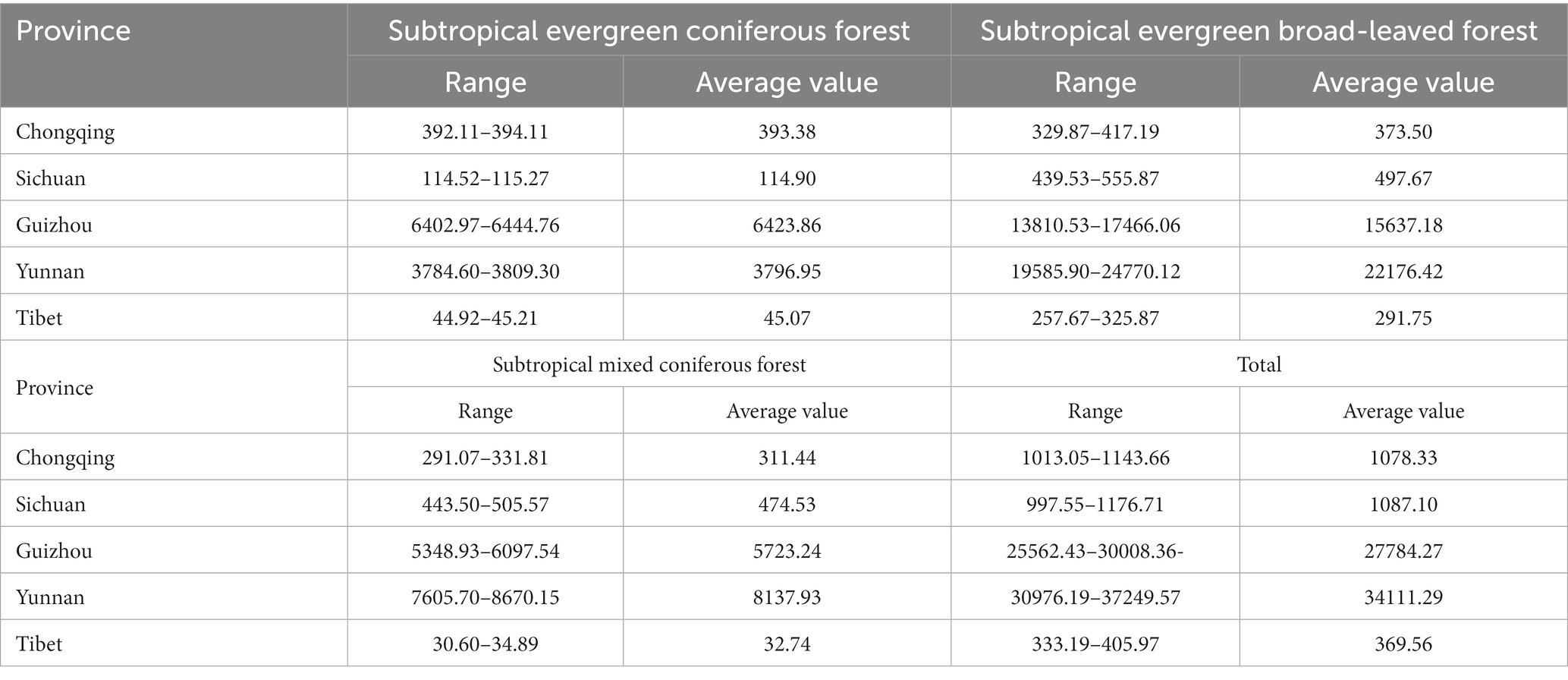
Table 4. The burning amount of various forest materials in the southwest forest area from 2004 to 2021 (t).
According to the amount of forest biomass burned and combined with emission factors, the emission of water-soluble ions in PM2.5 from 2004 to 2021 in southwest forest provinces was obtained (Table 5). The results show that Guizhou and Yunnan were the provinces with the highest emission intensity, accounting for 42.88 and 53.23% of the total in the region; Tibet had the lowest emission intensity, which is 0.58%. The main water-soluble ions in PM2.5 released by forest fires in the study area were Na+, K+, and Cl−, having the highest release ratio; Li+ emission was the lowest, less than 1% emission ratio. The reason for the above difference is that the emission factors of different water-soluble ions of PM2.5 are different.
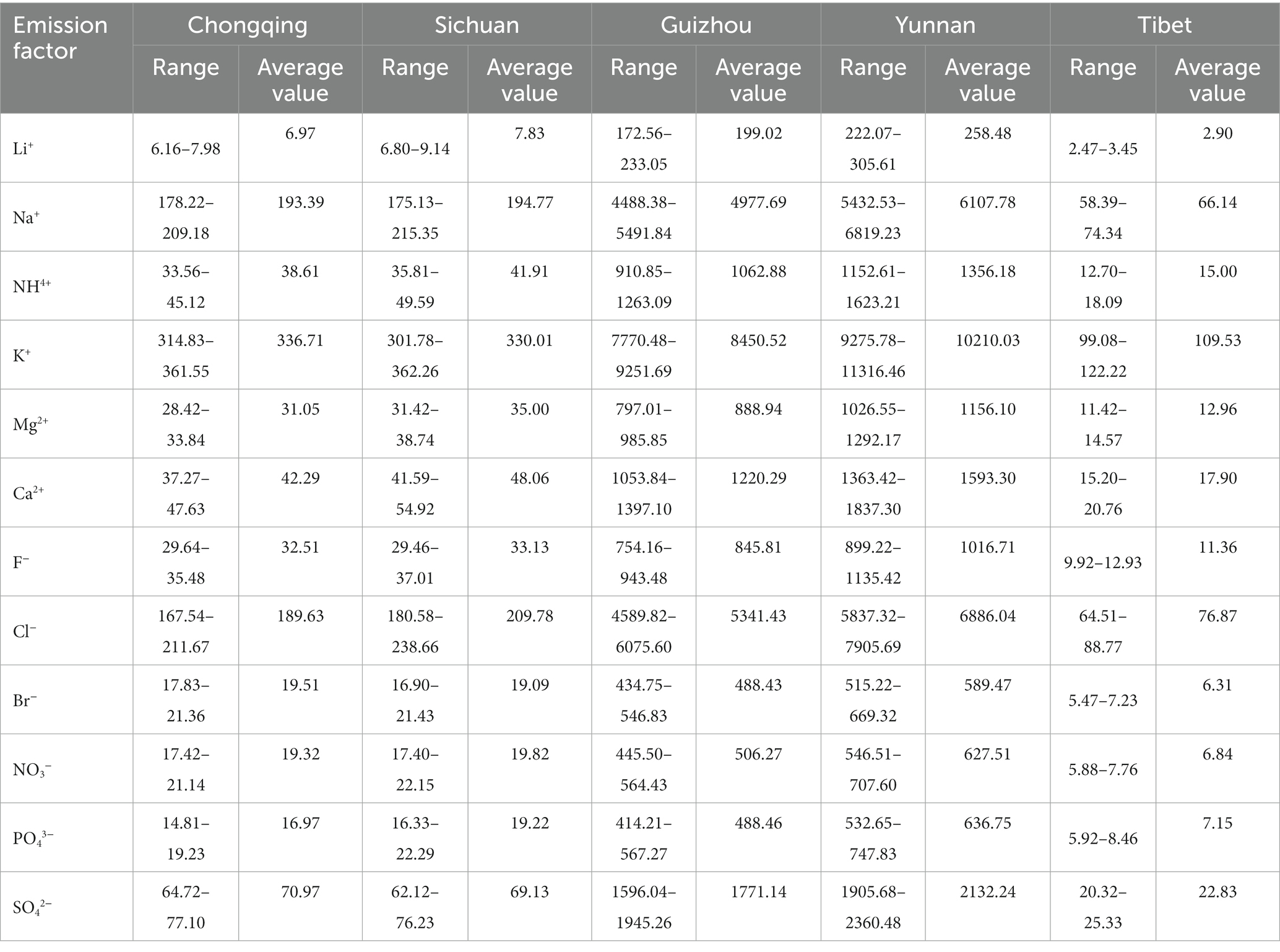
Table 5. Inventory of water-soluble ion emissions in PM2.5 in southwest forest areas from 2004 to 2021 (Kg).
From 2004 to 2021, more than half of the average annual emissions of pollutants came from Yunnan. This may not just because Yunnan province has a larger forest cover. Although the forest coverage rate of Sichuan is second only to that of Yunnan, the emission of forest fires is far lower than that of Yunnan. The climate conditions in Yunnan Province have played a driving role in the frequency of fires. The climate characteristics of Yunnan include distinct dry and wet seasons and uneven rainfall factors, which make forest fires prone to occurrence and significantly increase the risk of fires, leading to a large amount of forest fire pollutant emissions. Human activities have also to some extent exacerbated the problem of forest fire pollution in Yunnan Province. Illegal wildfire sources, farmland waste incineration, forest land development, and logging activities can all cause fires. Some remote areas in Yunnan Province lack effective supervision and control, which also increases the probability of fire occurrence. Therefore, further research is needed on more factors affecting forest fire emissions. The emission from biomass combustion in Tibet is less than 1% of that in the southwest forest area, which is consistent with the findings of Yin et al. (2019). The relatively high emissions in the provinces are mainly related to the large area of forest coverage, meteorological drought and frequent human activities (Cochrane and Barber, 2009). The southwest forest area is dominated by pines, especially Pinus yunnanensis and Pinus armandii with higher proportion. The bark and branches and leaves of these species are rich in flammable oils. The accumulation of combustibles on the ground is more prone to surface fires, while coniferous forests are prone to crown fires and have favorable conditions for fires, so the probability of fires increases. The huge uncertainty of official statistics cannot be ignored. The southwest forest area contains many remote and sparsely populated areas (Li et al., 2015). Therefore, more attention should be paid to the monitoring and management of forest fire emissions in the southwest forest area.
3.4. Temporal trend in emissions of water-soluble ions in PM2.5
The Mann-Kandell trend test method in Python library was used to analyze the annual trends of emissions of water-soluble ions in PM2.5 in each province in the southwestern forest region (Figure 5). The results show that the temporal characteristics of pollutant emissions from forest fires were consistent with the temporal characteristics of forest fires. The water-soluble ions in PM2.5 released by forest fires in the southwestern forest area from 2004 to 2021 all showed a downward trend. According to the China Forestry Statistical Yearbook, although the forest fire area in each province in the study area fluctuated from 2004 to 2021, the overall trend was declining. In addition, the changes of different pollutants in different regions are different. All water-soluble ions in Tibet, Chongqing, and Guizhou tended to decrease, however they decreased significantly in Yunnan and Sichuan. The main reason for the downward trend was the area change of forest fires in each province. The improvement of forest management and fire monitoring technology may be one of the reasons for this trend (Hantson et al., 2013), with many places adopting stricter fire monitoring measures to improve early warning capabilities for fire outbreaks. This allows forest fires to be detected and extinguished earlier, thereby reducing smoke and PM2.5 emissions caused by fires (Xiang et al., 2023). In recent years, environmental protection issues have attracted widespread attention, and people’s concern about atmospheric quality has significantly increased. This has led to more resources being used to take measures to reduce the impact of fires on atmospheric quality (Andela et al., 2017).
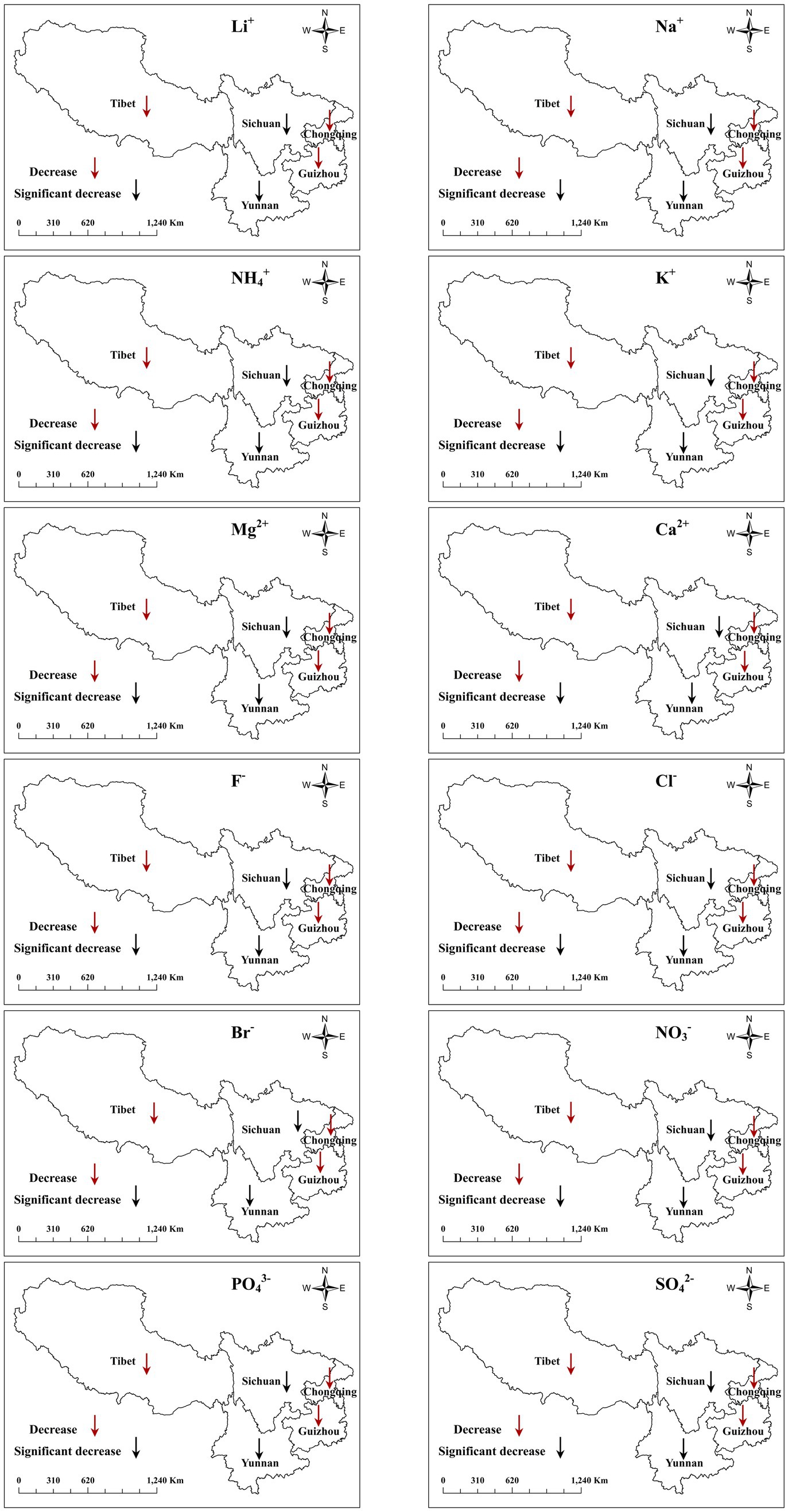
Figure 5. Temporal trend test of water-soluble ions in PM2.5 emitted by forest fires in southwest forest areas from 2004 to 2021.
The monthly distribution of emissions of total water-soluble ions in PM2.5 from 2004 to 2021 in the southwest forest area is shown in Figure 6. The concentrations of water-soluble ions in PM2.5 in the southwest forest area were from February to April, reaching the peak in March. The emissions of the total water-soluble ions accounted for 22, 25.83, and 17.18%, in February, March, and April, respectively. Among the water-soluble ions, K+, Na+, and Cl− had the highest emission in PM2.5. From February to April, it is a high incidence of forest fire, and in March, the pollutant emission reached the peak. During this period, the fire sources of spring plowing increased (Li et al., 2020), and the Qingming Festival sacrificial activities were held frequently (Li et al., 2015), which is consistent with the time of forest fire discharge in the southwest forest area. The spring season in the southwest forest area is the season with the highest emissions of water-soluble ions in PM2.5, accounting for 51.32%, followed by winter (36.34%), summer (7.61%), and autumn (4.74%). Southwest China has a subtropical monsoon climate with high temperature and rain in summer and autumn, which may result in the absence of forest fires for several months (Ai-feng, 2011; Li et al., 2016). In contrast, the emissions from June to November are always small, accounting for 12.35%, and the proportion of water-soluble ions in PM2.5 from December to May is 87.66%. Forest fires in the southwestern region mainly occur from January to May, which may be related to the climate characteristics and vegetation types of the region. At this time, the climate is dry, the wind is strong, and there are more combustibles on the trees and surface. Relatively low precipitation and higher temperature create meteorological conditions that are more conducive to the occurrence of fires (van der Werf et al., 2008). Secondly, agricultural activities have also increased the risk of fires during this period, such as farmland clearing and land preparation work, which can sometimes inadvertently trigger fires. Human activities have also increased the frequency of fires during this season. In spring, people often engage in outdoor activities, such as camping and barbecues, which increase the risk of fires. Therefore, strengthening and effectively controlling forest fires in Southwest China from January to May is of great significance for improving regional air quality.
The monthly distribution of emissions of total water-soluble ion in PM2.5 among different provinces in the southwest forest area is shown in Figure 7. In Chongqing, the highest emission of water-soluble ions in PM2.5 was in July (26.6%) and August (26.4%), and the highest emission of water-soluble ions in PM2.5 in Guizhou and Yunnan was in February (36.9, 23.8%) and March (26.2, 29.5%), Sichuan’s highest emissions of PM2.5 water-soluble ions were in January, February and March (14.4, 12.3, and 12.3%), while the highest emissions of water-soluble ion in PM2.5 in Tibet were in March (33.7%) and April (19.4%). In Chongqing, emissions of water-soluble ions in PM2.5 mainly occurred in summer, and the emission of water-soluble ions in PM2.5 in Guizhou, Yunnan, Sichuan, and Tibet mainly occurred in spring and winter; due to the differences in climatic conditions and forest combustion. In terms of total emissions, spring contributed the most emissions due to the influence of dry weather, the lowest emissions occurred in the rainy season, summer and autumn (Yin et al., 2019). This pattern favorably influences fire conditions, such as the moisture content of vegetation and the monsoon (Song et al., 2009). It is dry and rainless in spring and winter, leaving a large number of dead leaves on the ground, and the water content is extremely low, which provides the fuel stock for forest fires in spring and winter. In summer and autumn, the rainfall is abundant, the moisture content of combustibles is high, the air humidity is increased, and it is not easy for forest fires to occur. Therefore, the southwestern provinces should take corresponding measures to control the occurrence of forest fires based on historical data and conditions. The results of this study are similar to those of Wang et al. (2019), Song et al. (2022), and Yin et al. (2019).
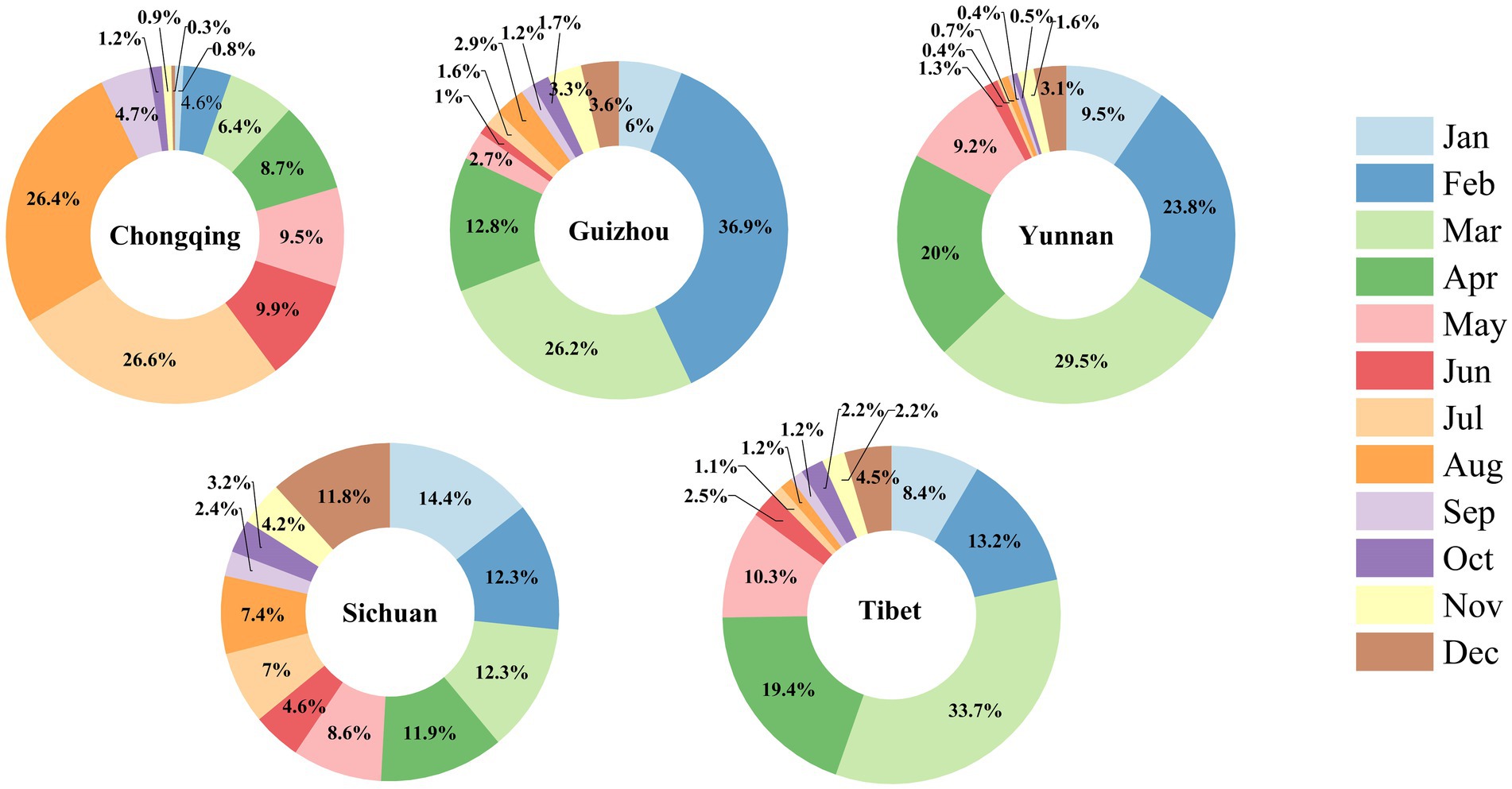
Figure 7. Monthly proportion of total water-soluble ion emissions in PM2.5 in each province in southwest forest area from 2004 to 2021.
3.5. Spatial distribution of emissions of water-soluble ions in PM2.5
The spatial distribution of different water-soluble ions in PM2.5 in 10 km × 10 km grid in the southwest forest area is shown in Figure 8. The results show that the spatial distribution of water-soluble ions in PM2.5 released by forest fires in the southwestern forest area displayed clear spatial heterogeneity, and the discharge of various water-soluble ions was spatially unbalanced, with more in the south and less in the north. The southern part of the study area is seriously affected by water-soluble ions in PM2.5. Among them, Yunnan province and southern Guizhou had the highest areas of various water-soluble ion grid emissions, while other regions also had grids with large water-soluble ion emissions. These areas are characterized by dense population, abundant forest resources, and large cultivated land (Song et al., 2022). In general, the spatial distribution of water-soluble ions in PM2.5 is closely related to the distribution of forest fires in these areas: the higher the density of forest fires and the greater the area of forest, the higher the unit emission intensity of water-soluble ions. The emission profiles of all water-soluble ions exhibited similar spatial distributions and showed a pattern consistent with the combustion zone. Therefore, the emission intensity of water-soluble ions in PM2.5 was positively correlated with the area burned by forest fires (Song et al., 2022). As a whole, the emissions of water-soluble ions in PM2.5 released during forest fires in the southwest forest area show strong spatial differences. In addition, the changes in emissions of water-soluble ion in PM2.5 in different provinces are significantly different, which also illustrates the huge spatial diversity emissions of water-soluble ion in PM2.5 from forest fires in southwestern China.
Forest fires will be accompanied by the production of a large amount of particulate matter, and water-soluble ions are an important part of particulate matter (Ma et al., 2021). Water soluble ions can affect and alter the chemical composition of rainfall, affect air quality, and have climate impacts when encountering rainfall, water-soluble ions have the role of surface activators (Liu et al., 2022), SO42−,NO3−, and NH4+ in water-soluble ions have strong hygroscopic effect (Tutsak and Kocak, 2019), which reduces atmospheric visibility and acidifies atmospheric precipitation, resulting in acid rain (Kong et al., 2014). Water-soluble ions migrate and transform with atmospheric particulate matter and enter the water body and surface soil under the action of dry and wet deposition, thereby destroying the soil pH balance and microbial community, and affecting the ecological environment (Stone et al., 2010). A study on forest fires in California, United States found that the pollutants of forest fires affect the absorption of N by vegetation, causing the ecosystem to form different patches (Grogan et al., 2000). Therefore, after the fire, human intervention should be carried out in time to forest-affected areas to accelerate the ecological restoration process. Plant species with poor flammability and strong fire resistance form biological fireproof forest belts, thus they are suitable for afforestation of mountainous terrain. In addition measures, such as reduction in human activities; trimming of polluted soil, rational irrigation and fertilization, should be carried out to improve soil physical and chemical properties and to ensure the growth and recovery of vegetation; proper fire monitoring through build an “air-ground-underground” multiple monitoring system and timely and accurately providing fire information to relevant departments are also essential to control forest fire. Through this series of measures, the source of fire will be effectively curbed and the post-disaster ecological recovery will be accelerated. The southwest forest area is rich in plant resources, scattered forest resources, forest fire prevention is of great significance, so it is of great practical significance to study the distribution characteristics of forest fires in time and space.
3.6. Uncertainty of pollutant emissions
The establishment of pollutant emission inventories is influenced by multiple factors such as combustion area, emission factors, forest biomass density, and combustion efficiency. According to the principle of IPCC uncertainty analysis (IPCC, 1997), in this study, the uncertainty of the emission results of each pollutant was calculated based on the quantitative calculation of the emission results of each pollutant using formulas (4) and (5) of the errors of each factor error (Table 6). Since the forest burning area comes from the public data of the government statistics department, the accuracy is high, and the error range is not more than 5%. Emission factors can be affected by vegetation types, combustion patterns, and environmental characteristics. In this study, the mean value of multiple measurements of the main vegetation types in each region were used as the final emission factor to increase reliability, with the estimation error ranging from 9 to 17.9%. In terms of biomass density uncertainty, we combined data from the 6, 7, 8, and 9th forest resource inventory and the model results of Piao et al. (2007), and the error range is 20%. Combustion efficiency is another crucial factor for biomass combustion emission estimation. To enhance the reliability of this study, the mean value of multiple measured combustion efficiency of the same vegetation type was used as the final combustion efficiency, with the error controlled within 50% (Jin et al., 2018).
Due to the inherent characteristics of forest fires, accurately monitoring and quantifying the burned areas of different forest types after a fire disaster poses significant challenges, accurate data regarding the extent of forest fire damage are notably scarce (Giglio et al., 2009). Therefore, this study is based on official statistical data and determines the burning area according to the area ratio of different forest types to reduce the uncertainty of emission inventory. Nevertheless, there is still a certain bias between the burning area values of different forest types used in this paper and the real forest fire situation, which is also an important research direction to improve the accuracy of emission inventories in the future. At present, there is a lack of measured data on the combustion emission factors and combustion efficiency of forest tree species in China (Hao et al., 2016), which leads to the lack of consideration in the spatial heterogeneity of forest particulate matter emissions in the southwest forest area. Thus, it is necessary to strengthen the actual measurement of forest fire particulate matter emissions in the future, by conducting field sampling and analysis in different types of forests and regions, more empirical data on the emission factors and combustion efficiency of forest tree species can be obtained. Using more advanced models and algorithms, predictions and estimates can be made for areas lacking empirical data. Establishing a long-term monitoring network can continuously collect and update data, thereby reducing uncertainty, fully consider the particulate matter emissions of different tree species in the southwest forest area and further improve the accuracy of estimating forest fire pollutant emission.
MODIS is an important remote sensing tool widely used in fire monitoring. Although satellite data is widely used, they also have limitations, including data quality, spatial resolution, and algorithms used in data extraction (Bilgiç et al., 2023). For example, due to various factors such as canopy vegetation or cloud cover (Liu et al., 2020), MODIS combustion area data may be underestimated, the quality and accuracy of the data may be affected, and small-scale fires may be difficult to plot (Roy and Boschetti, 2009). This may lead to the omission of fire pixels, thereby underestimating the total emissions in the study area. In addition, if forest fires are located at the edge of the scan, the MODIS pixel size increases several times, and many small fires will be missed (Liu et al., 2015). When using MODIS fire point data, meteorological data, ground observations, and satellite images are combined to improve the reliability and accuracy of fire monitoring and reduce the impact of uncertainty.
4. Conclusion
Based on the findings of the present study, the following conclusions can be drawn. The forest fire area in the southwest forest area from 2004 to 2021 was 3.49 × 105hm2, and 64.43 kt of forest resources were burned. The average total biomass burned was the highest for evergreen broad-leaved forest (38.98 kt) followed by coniferous and broad-leaved mixed forest (14.68 kt), and finally evergreen coniferous forest (10.77 kt). The province with the largest number of forest fires in the southwest forest area was Guizhou (13,310 times), followed by Yunnan (5,238 times), Sichuan (5,132 times), Chongqing (1,288 times), and Tibet (124 times). The province with the largest forest fire area in the southwest was Yunnan (1.48 × 105 hm2), followed by Guizhou (1.33 × 105 hm2), Sichuan (5.93 × 104 hm2), Chongqing (5.22 × 103 hm2), and Tibet (3.35 × 103 hm2). From 2004 to 2021, forest fires in the southwest forest area released a total of 61.19 t of water-soluble ions in PM2.5. Yunnan is the province with the highest water-soluble ion emission, and K+ is the ion with the highest emission in PM2.5. The emission of water-soluble ions in Yunnan and Sichuan all showed a significant downward trend, while the overall decrease in Tibet, Chongqing, and Guizhou was not significant. Yunnan and Guizhou are the concentrated areas for the emission of various water-soluble ions. The total emission of water-soluble ions in PM2.5 in the southwest forest area was the highest in spring (51.32%), followed by winter (36.34%), and March was the peak month (25.83%). The water-soluble ions in PM2.5 can settle on the surface of plants and soil through rainfall, leading to soil acidification. Soil acidification can lead to the inability of plant roots to effectively absorb key nutrients, which may affect plant growth and health, thereby affecting the stability of the entire ecosystem. The sedimentation of water-soluble ions can also lead to the accumulation of harmful substances in the soil, which can affect the ecological function of the soil and even cause damage to the microbial community in the soil, thereby affecting the health and quality of the soil. In addition, the sedimentation of water-soluble ions may also have an impact on the quality of water bodies. When these ions settle into lakes, rivers, and groundwater, they may cause water pollution problems, harm aquatic organisms and ecosystems, and thus disrupt the balance of aquatic ecosystems. Hence concerted fire prevention efforts should be made in each province, taking into account the season with higher probability of fire occurrence to reduce the potential impact of fire-related pollutions.
Data availability statement
The original contributions presented in the study are included in the article/supplementary material, further inquiries can be directed to the corresponding author.
Author contributions
XZ: conceptualization, methodology, field sampling, test determination, software, data curation, and writing-original draft preparation. YM: field sampling, test determination, software, data curation, visualization, and investigation. ZH: field sampling, test determination, visualization, and investigation. CZ: field sampling, supervision, software, and validation. HL: methodology, software, and data curation. MT: writing—reviewing and editing. FG: conceptualization and writing—reviewing and editing. All authors contributed to the article and approved the submitted version.
Funding
The study was financially supported by the grant from the National Natural Science Foundation of China (Grant No. 32171807) to FG.
Conflict of interest
The authors declare that the research was conducted in the absence of any commercial or financial relationships that could be construed as a potential conflict of interest.
Publisher’s note
All claims expressed in this article are solely those of the authors and do not necessarily represent those of their affiliated organizations, or those of the publisher, the editors and the reviewers. Any product that may be evaluated in this article, or claim that may be made by its manufacturer, is not guaranteed or endorsed by the publisher.
References
Aguilera, R., Corringham, T., Gershunov, A., and Benmarhnia, T. (2021). Wildfire smoke impacts respiratory health more than fine particles from other sources: observational evidence from Southern California. Nat. Commun. 12:1493. doi: 10.1038/s41467-021-21708-0
Ai-feng, L. (2011). Study on the relationship among forest fire, temperature and precipitation and its spatial–temporal variability in China. Agric. Sci. Technol. Hunan 12, 1396–1400.
Alves, C., Gonçalves, C., Fernandes, A. P., Tarelho, L., and Pio, C. (2011). Fireplace and woodstove fine particle emissions from combustion of western Mediterranean wood types. Atmos. Res. 101, 692–700. doi: 10.1016/j.atmosres.2011.04.015
Amraoui, M., Pereira, M. G., DaCamara, C. C., and Calado, T. J. (2015). Atmospheric conditions associated with extreme fire activity in the Western Mediterranean region. Sci. Total Environ. 524-525, 32–39. doi: 10.1016/j.scitotenv.2015.04.032
Andela, N., Morton, D. C., Giglio, L., Chen, Y., van der Werf, G. R., Kasibhatla, P. S., et al. (2017). A human-driven decline in global burned area. Science 356, 1356–1362. doi: 10.1126/science.aal4108
Aragão, M. D. A., Fiedler, N. C., Ramalho, A. H. C., Menezes, R. A. S., Silva, E. C. G. D., Juvanhol, R. S., et al. (2023). Risk of forest fires occurrence on a transition island Amazon-Cerrado: where to act? For. Ecol. Manag. 536:120858. doi: 10.1016/j.foreco.2023.120858
Babu, K. N., Gour, R., Ayushi, K., Ayyappan, N., and Parthasarathy, N. (2023). Environmental drivers and spatial prediction of forest fires in the Western Ghats biodiversity hotspot, India: An ensemble machine learning approach. For. Ecol. Manag. 540:121057. doi: 10.1016/j.foreco.2023.121057
Bar, S., Parida, B. R., and Pandey, A. C. (2020). Landsat-8 and Sentinel-2 based Forest fire burn area mapping using machine learning algorithms on GEE cloud platform over Uttarakhand, Western Himalaya. Remote Sens. Appl. Soc. Environ. 18:100324. doi: 10.1016/j.rsase.2020.100324
Bergeron, Y., Gauthier, S., Flannigan, M., and Kafka, V. (2004). Fire regimes at the transition between mixedwood and coniferous boreal forest in northwestern Quebec. Ecology 85, 1916–1932. doi: 10.1890/02-0716
Bilgiç, E., Tuna Tuygun, G., and Gündüz, O. (2023). Development of an emission estimation method with satellite observations for significant forest fires and comparison with global fire emission inventories: application to catastrophic fires of summer 2021 over the eastern Mediterranean. Atmos. Environ. 308:119871. doi: 10.1016/j.atmosenv.2023.119871
Bond, W., and Keeley, J. (2005). Fire as a global ‘herbivore’: the ecology and evolution of flammable ecosystems. Trends Ecol. Evol. 20, 387–394. doi: 10.1016/j.tree.2005.04.025
Bytnerowicz, A., Omasa, K., and Paoletti, E. (2007). Integrated effects of air pollution and climate change on forests: a northern hemisphere perspective. Environ. Pollut. 147, 438–445. doi: 10.1016/j.envpol.2006.08.028
Chen, J., Qiu, S., Shang, J., Wilfrid, O. M. F., Liu, X., Tian, H., et al. (2014). Impact of relative humidity and water soluble constituents of PM2.5 on visibility impairment in Beijing. Aerosol Air Quality Res. 14, 260–268. doi: 10.4209/aaqr.2012.12.0360
Cochrane, M. A., and Barber, C. P. (2009). Climate change, human land use and future fires in the Amazon. Glob. Chang. Biol. 15, 601–612. doi: 10.1111/j.1365-2486.2008.01786.x
Fang, J., Yu, G., Liu, L., Hu, S., and Chapin, F. S. (2018). Climate change, human impacts, and carbon sequestration in China. Proc. Natl. Acad. Sci. U. S. A. 115, 4015–4020. doi: 10.1073/pnas.1700304115
Giglio, L., Loboda, T., Roy, D. P., Quayle, B., and Justice, C. O. (2009). An active-fire based burned area mapping algorithm for the MODIS sensor. Remote Sens. Environ. 113, 408–420. doi: 10.1016/j.rse.2008.10.006
Giglio, L., van der Werf, G. R., Randerson, J. T., Collatz, G. J., and Kasibhatla, P. (2006). Global estimation of burned area using MODIS active fire observations. Atmos. Chem. Phys. 6, 957–974. doi: 10.5194/acp-6-957-2006
Grillakis, M., Voulgarakis, A., Rovithakis, A., Seiradakis, K. D., Koutroulis, A., Field, R. D., et al. (2022). Climate drivers of global wildfire burned area. Environ. Res. Lett. 17:045021. doi: 10.1088/1748-9326/ac5fa1
Grogan, P., Burns, T. D., and Chapin Iii, F. S. (2000). Fire effects on ecosystem nitrogen cycling in a Californian bishop pine forest. Oecologia 122, 537–544. doi: 10.1007/s004420050977
Guo, F., Ju, Y., Wang, G., Alvarado, E. C., Yang, X., Ma, Y., et al. (2018). Inorganic chemical composition of PM2.5 emissions from the combustion of six main tree species in subtropical China. Atmos. Environ. 189, 107–115. doi: 10.1016/j.atmosenv.2018.06.044
Guo, L., Ma, Y., Tigabu, M., Guo, X., Zheng, W., and Guo, F. (2020). Emission of atmospheric pollutants during forest fire in boreal region of China. Environ. Pollut. 264:114709. doi: 10.1016/j.envpol.2020.114709
Hantson, S., Padilla, M., Corti, D., and Chuvieco, E. (2013). Strengths and weaknesses of MODIS hotspots to characterize global fire occurrence. Remote Sens. Environ. 131, 152–159. doi: 10.1016/j.rse.2012.12.004
Hao, W. M., Petkov, A., Nordgren, B. L., Corley, R. E., Silverstein, R. P., Urbanski, S. P., et al. (2016). Daily black carbon emissions from fires in northern Eurasia for 2002–2015. Geosci. Model Dev. 9, 4461–4474. doi: 10.5194/gmd-9-4461-2016
Hazra, D., and Gallagher, P. (2022). Role of insurance in wildfire risk mitigation. Econ. Model. 108:105768. doi: 10.1016/j.econmod.2022.105768
He, M., Zheng, J., Yin, S., and Zhang, Y. (2011). Trends, temporal and spatial characteristics, and uncertainties in biomass burning emissions in the Pearl River Delta, China. Atmos. Environ. 45, 4051–4059. doi: 10.1016/j.atmosenv.2011.04.016
Hoelzemann, J. J. (2004). Global wildland fire emission model (GWEM): evaluating the use of global area burnt satellite data. J. Geophys. Res. 109:D14S04. doi: 10.1029/2003jd003666
Hu, Y., Fernandez-Anez, N., Smith, T. E. L., and Rein, G. (2018). Review of emissions from smouldering peat fires and their contribution to regional haze episodes. Int. J. Wildland Fire 27:293. doi: 10.1071/wf17084
Huang, Z., Ma, Y., Zhan, X., Lin, H., Zheng, C., Tigabu, M., et al. (2023). Composition of inorganic elements in fine particulate matter emitted during surface fire in relation to moisture content of forest floor combustibles. Chemosphere 312:137259. doi: 10.1016/j.chemosphere.2022.137259
IPCC (1997). “Quantifying uncertainties in practice, chapter 6” in Good Practice Guidance and Uncertainty.
Iraci, L. T., Parworth, C. L., Yates, E. L., Marrero, J. E., and Ryoo, J. M. (2022). A collection of airborne measurements and analyses of Trace gases emitted from multiple fires in California. Earth Space Sci. 9:e2021EA002116. doi: 10.1029/2021ea002116
Jain, M., Saxena, P., Sharma, S., and Sonwani, S. (2021). Investigation of Forest fire activity changes over the Central India domain using satellite observations during 2001–2020. GeoHealth 5:e2021GH000528. doi: 10.1029/2021gh000528
Jin, Q., Ma, X., Wang, G., Yang, X., and Guo, F. (2018). Dynamics of major air pollutants from crop residue burning in mainland China, 2000-2014. J. Environ. Sci. (China) 70, 190–205. doi: 10.1016/j.jes.2017.11.024
Jin, Q.-F., Wang, W.-H., Ma, X.-Q., Yang, S.-Y., and Guo, F.-T. (2017). Temporal and spatial dynamics of pollutants emission from forest fires in Fujian during 2000-2010. Chin. Environ. Sci. 37, 476–485.
Jin, Q., Wang, W., Zheng, W., Innes, J. L., Wang, G., and Guo, F. (2022). Dynamics of pollutant emissions from wildfires in mainland China. J. Environ. Manag. 318:115499. doi: 10.1016/j.jenvman.2022.115499
Kharuk, V. I., Ponomarev, E. I., Ivanova, G. A., Dvinskaya, M. L., Coogan, S. C. P., and Flannigan, M. D. (2021). Wildfires in the Siberian taiga. Ambio 50, 1953–1974. doi: 10.1007/s13280-020-01490-x
Kong, L., Yang, Y., Zhang, S., Zhao, X., Du, H., Fu, H., et al. (2014). Observations of linear dependence between sulfate and nitrate in atmospheric particles. J. Geophys. Res. Atmos. 119, 341–361. doi: 10.1002/2013jd020222
Larkin, N. K. (2005). Global seasonal temperature and precipitation anomalies during El Niño autumn and winter. Geophys. Res. Lett. 32:L16705. doi: 10.1029/2005gl022860
Li, J., Li, Y., Bo, Y., and Xie, S. (2016). High-resolution historical emission inventories of crop residue burning in fields in China for the period 1990–2013. Atmos. Environ. 138, 152–161. doi: 10.1016/j.atmosenv.2016.05.002
Li, J., Song, Y., Huang, X., and Li, M. (2015). Comparison of forest burned areas in mainland China derived from MCD45A1 and data recorded in yearbooks from 2001 to 2011. Int. J. Wildland Fire 24:103. doi: 10.1071/wf14031
Li, L., Wang, K., Chen, W., Zhao, Q., Liu, L., Liu, W., et al. (2020). Atmospheric pollution of agriculture-oriented cities in Northeast China: a case in Suihua. J. Environ. Sci. (China) 97, 85–95. doi: 10.1016/j.jes.2020.04.038
Liu, X., Li, Y., Ma, K., Yang, L., Li, M., Li, C., et al. (2022). Spatial distribution and potential sources of arsenic and water-soluble ions in the snow at Ili River valley. Chemosphere 295:133845. doi: 10.1016/j.chemosphere.2022.133845
Liu, T., Mickley, L. J., Marlier, M. E., DeFries, R. S., Khan, M. F., Latif, M. T., et al. (2020). Diagnosing spatial biases and uncertainties in global fire emissions inventories: Indonesia as regional case study. Remote Sens. Environ. 237:111557. doi: 10.1016/j.rse.2019.111557
Liu, M., Song, Y., Yao, H., Kang, Y., Li, M., Huang, X., et al. (2015). Estimating emissions from agricultural fires in the North China plain based on MODIS fire radiative power. Atmos. Environ. 112, 326–334. doi: 10.1016/j.atmosenv.2015.04.058
Lu, Z., Zhang, Q., and Streets, D. G. (2011). Sulfur dioxide and primary carbonaceous aerosol emissions in China and India, 1996–2010. Atmos. Chem. Phys. 11, 9839–9864. doi: 10.5194/acp-11-9839-2011
Ma, Y., Zheng, W., Guo, X., Tigabu, M., and Guo, F. (2021). Effect of forest floor fuel moisture content on chemical components of PM2.5 emitted during combustion. Chemosphere 279:130547. doi: 10.1016/j.chemosphere.2021.130547
Michel, C. (2005). Biomass burning emission inventory from burnt area data given by the SPOT-VEGETATION system in the frame of TRACE-P and ACE-Asia campaigns. J. Geophys. Res. 110:D09304. doi: 10.1029/2004jd005461
Molina, J. R., Lora, A., Prades, C., and Rodríguez y Silva, F. (2019). Roadside vegetation planning and conservation: new approach to prevent and mitigate wildfires based on fire ignition potential. For. Ecol. Manag. 444, 163–173. doi: 10.1016/j.foreco.2019.04.034
Ni, J., Zhang, X.-S., and Scurlock, J. M. O. (2001). Synthesis and analysis of biomass and net primary productivity in Chinese forests. Ann. For. Sci. 58, 351–384. doi: 10.1051/forest:2001131
Parvin, K., Hasanuzzaman, M., Bhuyan, M., Mohsin, S. M., and Fujita, A. M. (2019). Quercetin mediated salt tolerance in tomato through the enhancement of plant antioxidant defense and glyoxalase systems. Plants 8:247. doi: 10.3390/plants8080247
Piao, S., Fang, J., Zhou, L., Tan, K., and Tao, S. (2007). Changes in biomass carbon stocks in China's grasslands between 1982 and 1999. Glob. Biogeochem. Cycles 21:GB2004. doi: 10.1029/2005gb002634
Prasad, V. K., Badarinath, K. V. S., and Eaturu, A. (2008). Biophysical and anthropogenic controls of forest fires in the Deccan plateau, India. J. Environ. Manag. 86, 1–13. doi: 10.1016/j.jenvman.2006.11.017
Prichard, S. J., Hessburg, P. F., Hagmann, R. K., Povak, N. A., Dobrowski, S. Z., Hurteau, M. D., et al. (2021). Adapting western North American forests to climate change and wildfires: 10 common questions. Ecol. Appl. 31:e02433. doi: 10.1002/eap.2433
Reisen, F., Duran, S. M., Flannigan, M., Elliott, C., and Rideout, K. (2015). Wildfire smoke and public health risk. Int. J. Wildland Fire 24:1029. doi: 10.1071/wf15034
Roy, D. P., and Boschetti, L. (2009). Southern Africa validation of the MODIS, L3JRC, and GlobCarbon burned-area products. IEEE Trans. Geosci. Remote Sens. 47, 1032–1044. doi: 10.1109/TGRS.2008.2009000
Sahu, L. K., and Sheel, V. (2013). Spatio-temporal variation of biomass burning sources over south and Southeast Asia. J. Atmos. Chem. 71, 1–19. doi: 10.1007/s10874-013-9275-4
Seidl, R., Thom, D., Kautz, M., Martin-Benito, D., Peltoniemi, M., Vacchiano, G., et al. (2017). Forest disturbances under climate change. Nat. Clim. Chang. 7, 395–402. doi: 10.1038/nclimate3303
Song, Y., Liu, B., Miao, W., Chang, D., and Zhang, Y. (2009). Spatiotemporal variation in nonagricultural open fire emissions in China from 2000 to 2007. Glob. Biogeochem. Cycles 23:GB2008. doi: 10.1029/2008gb003344
Song, R., Wang, T., Han, J., Xu, B., Ma, D., Zhang, M., et al. (2022). Spatial and temporal variation of air pollutant emissions from forest fires in China. Atmos. Environ. 281:119156. doi: 10.1016/j.atmosenv.2022.119156
Sonwani, S., Hussain, S., and Saxena, P. (2022). Air pollution and climate change impact on forest ecosystems in Asian region—a review. Ecosyst. Health Sustain. 8:2090448. doi: 10.1080/20964129.2022.2090448
Stone, E., Schauer, J., Quraishi, T. A., and Mahmood, A. (2010). Chemical characterization and source apportionment of fine and coarse particulate matter in Lahore, Pakistan. Atmos. Environ. 44, 1062–1070. doi: 10.1016/j.atmosenv.2009.12.015
Su, L.-J., He, Y.-J., and Chen, S.-Z. (2015). Temporal and spatial characteristics and risk analysis of Forest fires in China from 1950 to 2010. For. Sci. 51, 88–96.
Sun, Y., Zhang, Q., Li, K., Huo, Y., and Zhang, Y. (2022). Trace gas emissions from laboratory combustion of leaves typically consumed in forest fires in Southwest China. Sci. Total Environ. 846:157282. doi: 10.1016/j.scitotenv.2022.157282
Takahashi, M., Feng, Z., Mikhailova, T. A., Kalugina, O. V., Shergina, O. V., Afanasieva, L. V., et al. (2020). Air pollution monitoring and tree and forest decline in East Asia: a review. Sci. Total Environ. 742:140288. doi: 10.1016/j.scitotenv.2020.140288
Terrier, A., Girardin, M. P., Périé, C., Legendre, P., and Bergeron, Y. (2013). Potential changes in forest composition could reduce impacts of climate change on boreal wildfires. Ecol. Appl. 23, 21–35. doi: 10.1890/12-0425.1
Tian, X., Zhao, F., Shu, L., and Wang, M. (2013). Distribution characteristics and the influence factors of forest fires in China. For. Ecol. Manag. 310, 460–467. doi: 10.1016/j.foreco.2013.08.025
Tutsak, E., and Kocak, M. (2019). High time-resolved measurements of water-soluble sulfate, nitrate and ammonium in PM2.5 and their precursor gases over the eastern Mediterranean. Sci. Total Environ. 672, 212–226. doi: 10.1016/j.scitotenv.2019.03.451
Val Martin, M., Kahn, R., and Tosca, M. (2018). A global analysis of wildfire smoke Injection Heights derived from space-based multi-angle imaging. Remote Sens. 10:1609. doi: 10.3390/rs10101609
van der Werf, G. R., Randerson, J. T., Giglio, L., Gobron, N., and Dolman, A. J. (2008). Climate controls on the variability of fires in the tropics and subtropics. Glob. Biogeochem. Cycles 22:3122. doi: 10.1029/2007gb003122
Wan, X., Kawamura, K., Ram, K., Kang, S., Loewen, M., Gao, S., et al. (2019). Aromatic acids as biomass-burning tracers in atmospheric aerosols and ice cores: a review. Environ. Pollut. 247, 216–228. doi: 10.1016/j.envpol.2019.01.028
Wang, G. H., Huang, L. M., Gao, S. X., Gao, S. T., and Wang, L. S. (2002). Characterization of water-soluble species of PM10 and PM2.5 aerosols in urban area in Nanjing, China. Atmos. Environ. 36, 1299–1307. doi: 10.1016/S1352-2310(01)00550-7
Wang, X., Qin, Y., Qin, J., Long, X., Qi, T., Chen, R., et al. (2021). Spectroscopic insight into the pH-dependent interactions between atmospheric heavy metals (cu and Zn) and water-soluble organic compounds in PM2.5. Sci. Total Environ. 767:145261. doi: 10.1016/j.scitotenv.2021.145261
Wang, G., Wang, H., Yu, Y., Gao, S., Feng, J., Gao, S., et al. (2003). Chemical characterization of water-soluble components of PM10 and PM2.5 atmospheric aerosols in five locations of Nanjing. Atmospher. Environ. 37, 2893–2902. doi: 10.1016/s1352-2310(03)00271-1
Wang, H., Wang, X., Zhou, H., Ma, H., Xie, F., Zhou, X., et al. (2021). Stoichiometric characteristics and economic implications of water-soluble ions in PM2.5 from a resource-dependent city. Environ. Res. 193:110522. doi: 10.1016/j.envres.2020.110522
Wang, W., Zhang, Q., Luo, J., Zhao, R., and Zhang, Y. (2019). Estimation of Forest fire emissions in Southwest China from 2013 to 2017. Atmosfera 11:15. doi: 10.3390/atmos11010015
Wang, H., Zhu, B., Shen, L., Xu, H., An, J., Xue, G., et al. (2015). Water-soluble ions in atmospheric aerosols measured in five sites in the Yangtze River Delta, China: size-fractionated, seasonal variations and sources. Atmos. Environ. 123, 370–379. doi: 10.1016/j.atmosenv.2015.05.070
Wardoyo, A., Morawska, L., and Ristovski, Z. (2011). CO2 emissions from the combustion of native Australian trees. Int. J. Basic Appl. Sci. 11, 70–77.
Whitman, E., Parisien, M.-A., Thompson, D. K., and Flannigan, M. D. (2019). Short-interval wildfire and drought overwhelm boreal forest resilience. Sci. Rep. 9:18796. doi: 10.1038/s41598-019-55036-7
Xiang, M., Xiao, C., Feng, Z., and Ma, Q. (2023). Global distribution, trends and types of active fire occurrences. Sci. Total Environ. 902:166456. doi: 10.1016/j.scitotenv.2023.166456
Xu, R., Yu, P., Abramson, M. J., Johnston, F. H., Samet, J. M., Bell, M. L., et al. (2020). Wildfires, global climate change, and human health. N. Engl. J. Med. 383, 2173–2181. doi: 10.1056/NEJMsr2028985
Yan, X., Ohara, T., and Akimoto, H. (2006). Bottom-up estimate of biomass burning in mainland China. Atmos. Environ. 40, 5262–5273. doi: 10.1016/j.atmosenv.2006.04.040
Yang, W., Pudasainee, D., Gupta, R., Li, W., Wang, B., and Sun, L. (2021). An overview of inorganic particulate matter emission from coal/biomass/MSW combustion: sampling and measurement, formation, distribution, inorganic composition and influencing factors. Fuel Process. Technol. 213:106657. doi: 10.1016/j.fuproc.2020.106657
Yang, X., Zhao, C., Yang, Y., Yan, X., and Fan, H. (2021). Statistical aerosol properties associated with fire events from 2002 to 2019 and a case analysis in 2019 over Australia. Atmos. Chem. Phys. 21, 3833–3853. doi: 10.5194/acp-21-3833-2021
Ye, B., Ji, X., Yang, H., Yao, X., Chan, C. K., Cadle, S. H., et al. (2003). Concentration and chemical composition of PM2.5 in Shanghai for a 1-year period. Atmos. Environ. 37, 499–510. doi: 10.1016/S1352-2310(02)00918-4
Yin, L., Du, P., Zhang, M., Liu, M., Xu, T., and Song, Y. (2019). Estimation of emissions from biomass burning in China (2003–2017) based on MODIS fire radiative energy data. Biogeosciences 16, 1629–1640. doi: 10.5194/bg-16-1629-2019
Keywords: emission of pollutants, forest fire, PM2.5, spatio-temporal distribution, water-soluble inorganic ions
Citation: Zhan X, Ma Y, Huang Z, Zheng C, Lin H, Tigabu M and Guo F (2023) Temporal and spatial dynamics in emission of water-soluble ions in fine particulate matter during forest fires in Southwest China. Front. For. Glob. Change. 6:1250038. doi: 10.3389/ffgc.2023.1250038
Edited by:
Zhenyu Xing, University of Calgary, CanadaReviewed by:
Ying Xiong, University of Michigan, United StatesPallavi Saxena, University of Delhi, India
Copyright © 2023 Zhan, Ma, Huang, Zheng, Lin, Tigabu and Guo. This is an open-access article distributed under the terms of the Creative Commons Attribution License (CC BY). The use, distribution or reproduction in other forums is permitted, provided the original author(s) and the copyright owner(s) are credited and that the original publication in this journal is cited, in accordance with accepted academic practice. No use, distribution or reproduction is permitted which does not comply with these terms.
*Correspondence: Futao Guo, guofutao@126.com
†These authors share first authorship