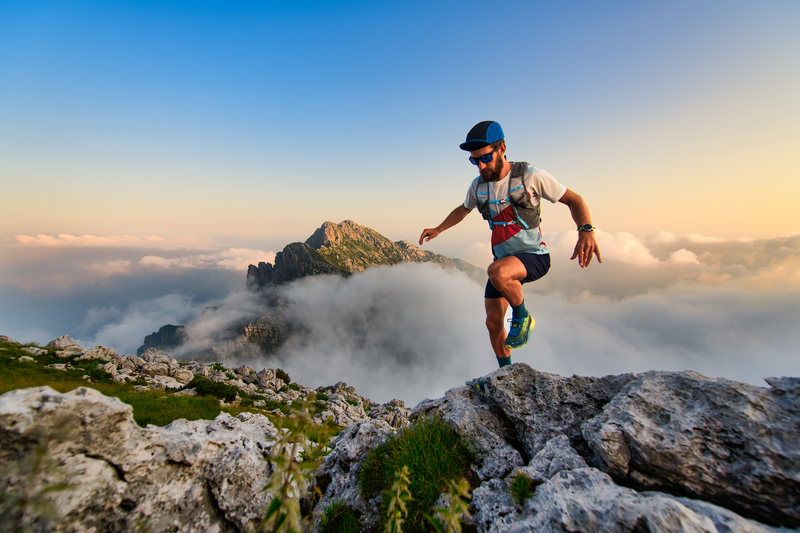
94% of researchers rate our articles as excellent or good
Learn more about the work of our research integrity team to safeguard the quality of each article we publish.
Find out more
ORIGINAL RESEARCH article
Front. For. Glob. Change , 28 August 2023
Sec. Forest Hydrology
Volume 6 - 2023 | https://doi.org/10.3389/ffgc.2023.1232410
Evapotranspiration (ET) from tropical forests plays a significant role in regulating the climate system. Forests are diverse ecosystems, encompass heterogeneous site conditions and experience seasonal fluctuations of rainfall. Our objectives were to quantify ET from a tropical rainforest using high-resolution thermal images and a simple modeling framework. In lowland Sumatra, thermal infrared (TIR) images were taken from an uncrewed aerial vehicle (UAV) of upland and riparian sites during both dry and wet seasons. We predicted ET from land surface temperature data retrieved from the TIR images by applying the DATTUTDUT energy balance model. We further compared the ET estimates to ground-based sap flux measurements for selected trees and assessed the plot-level spatial and temporal variability of ET across sites and seasons. Average ET across sites and seasons was 0.48 mm h–1, which is comparable to ET from a nearby commercial oil palm plantation where this method has been validated against eddy covariance measurements. For given trees, a positive correlation was found between UAV-based ET and tree transpiration derived from ground-based sap flux measurements, thereby corroborating the observed spatial patterns. Evapotranspiration at upland sites was 11% higher than at riparian sites across all seasons. The heterogeneity of ET was lower at upland sites than at riparian sites, and increased from the dry season to the wet season. This seasonally enhanced ET variability can be an effect of local site conditions including partial flooding and diverse responses of tree species to moisture conditions. These results improve our understanding of forest-water interactions in tropical forests and can aid the further development of vegetation-atmosphere models. Further, we found that UAV-based thermography using a simple, energy balance modeling scheme is a promising method for ET assessments of natural (forest) ecosystems, notably in data scarce regions of the world.
Evapotranspiration (ET) is a major component of the hydrological cycle through which water is returned to the atmosphere by simultaneous transpiration and evaporation from land surfaces. Evapotranspiration (ET) from forests, particularly from tropical forests plays a key role in the climate system because it regulates hydrological fluxes and land surface temperatures (LST) (Groombridge and Jenkins, 2002). Globally, forests have the highest contribution to total terrestrial ET (≈ 45%) (Oki and Kanae, 2006). The magnitude and variability of forest ET varies strongly across space and time and is driven by key climatic variables such as solar radiation, water vapor deficit and precipitation (Shuttleworth, 1988; von Randow et al., 2004). In wet forests, where water-availability is not a limiting factor, water vapor deficit and net radiation are strong seasonal controls of ET (Souza-Filho et al., 2005; Negrón-Juárez et al., 2007; Da Rocha et al., 2009; Costa et al., 2010). Locally, ET is also strongly influenced by site conditions. Studies have shown that tree transpiration across forest types from boreal to tropical stands is influenced by differences in topography and flooding conditions (Kang et al., 2004; Chen et al., 2005; Metzen et al., 2019; Gutierrez Lopez et al., 2021). A previous study in a boreal forest showed considerable spatial variation in ET along a moisture gradient from forested wetland to upland forest (Loranty et al., 2008). In lowland Sumatra, rainforest transpiration rates were higher at upland sites compared to valley sites which were subject to varying flooding conditions (Ahongshangbam et al., 2020). Likewise, topography and flooding also influenced water use of rubber trees and oil palms in the same region, with higher transpiration rates at upland sites than at valley sites (Hardanto et al., 2017).
To capture the complex spatio-temporal variability of ET in ecosystems requires an approach with appropriate resolution in both space and time. Commonly used techniques to quantify tree water use include eddy covariance and sap flux methods which have been useful for studying temporal dynamics; they are however stationary and have limited spatial coverage which leads to large uncertainties when scaling-up these point measurements to larger areas (Baldocchi, 2003; Flo et al., 2019). At larger spatial scales, remotely-sensed satellite and aerial imagery have been widely used to predict ET from different land-cover types using energy-balance models (Allen et al., 2011). However, the image resolution is often too coarse for studying stand and tree level variability in ET (0.3–5 km for satellite; 2–5 m for aerial) and satellite images over the tropics are often obscured by cloud cover (Kustas et al., 2003; Allen et al., 2011; Acharya et al., 2021) while overpass frequency at best allows for a seasonal analysis of ET variability. Uncrewed aerial vehicles (UAVs) as remote sensing platforms equipped with thermal infrared (TIR) sensors offer a flexible option for assessing land surface temperature (LST) at high resolution which can capture details of land covers such as dense vegetation and multi-storied tree canopies. LST is a key variable used for predicting surface energy fluxes and hence ET (Taheri et al., 2022). LST data has been found to be a reliable proxy for estimating surface energy fluxes of vegetation cover including from heterogeneous vegetation such as natural forests (Lapidot et al., 2019). Recording LST in close proximity to the surface using UAVs has several advantages over TIR methods from satellites, most notably the substantially increased spatial resolution, the high flexibility regarding time of day and the ability to acquire data under cloudy conditions. Additionally, measurement errors from atmospheric scattering and absorption are reduced compared to satellite methods due to the shorter distance the signal travels between sensor and surface (Hill et al., 2020). Taking into account some of the limitations of UAVs with regard to spatial extent (limited by battery capacity), temporal resolution (restricted by logistics) and unfavorable weather conditions such as strong winds or rainfall (Acharya et al., 2021), UAV-based LST assessments seem particularly suited for assessing differences across sites at different temporal scales from (sub)daily to seasonal, including from heterogeneous vegetation cover such as natural forests.
Surface energy balance (SEB) models are used to estimate actual ET as the residual term of the energy balance equation (Allen et al., 2007). A key driving force of available surface energy is LST which along with ancillary information such as wind speed, air temperature, vapor pressure and solar radiation are used to determine ET (Taheri et al., 2022). Surface energy balance models for deriving ET were originally developed for satellite-based data which have a very coarse spatial resolution compared to UAV-recorded data. Therefore, it is necessary to test the effect of pixel resolution on SEB models using high-resolution, UAV-based LST data. The DATTUTDUT model performed well over grassland and vineyard land covers when validated against eddy covariance measurements using high-resolution LST data from UAVs (Xia et al., 2016; Brenner et al., 2018). The DATTUTDUT model utilizes the difference in temperature extremes within a thermal image and is characterized by a low complexity and simple parametrisation scheme to simulate surface energy fluxes (Timmermans et al., 2015). In a minimal setup, this model requires only LSTs as input. Using relative LSTs has the advantage of minimizing errors and uncertainties associated with absolute accuracy of LST data, in situ climatic inputs and empirical parametrization of aerodynamic terms over other more complex energy balance models (Allen et al., 2007). Further, the model has been tested in a recent field experiment in a mature, tropical oil palm plantation which showed that ET predictions from the DATTUTDUT model were in high agreement with eddy covariance observations when used with additional inputs indicating statistical interchangeability of methods (Ellsäßer et al., 2021). In a tropical oil palm agroforest, UAV-based thermography was also successful in predicting transpiration of trees and palms (Ellsäßer et al., 2020a). Inclusion of in-situ measured short-wave radiation (Rs) in the model accounts for cloudy-sky conditions and relative humidity thereby broadening the applicability of the model and improving its prediction accuracy (Ellsäßer et al., 2020b). The model however, does not perform well in dry and low canopy cover conditions limiting its utility (Timmermans et al., 2015). While previous studies have tested this approach over crops and grassland, we wanted to test this simple modeling approach on tropical forests.
Our study was conducted in the Harapan rainforest, which is situated in an undulating, lowland area of Sumatra, Indonesia. The region is characterized by high deforestation rates over the last decades and today is dominated by rubber (Hevea brasiliensis) and oil palm (Elaeis guineensis) plantations (Clough et al., 2016; Melati, 2017). A study focusing on sap-flux measurements across different land-use types in the region indicated that intensively-managed oil palm plantations can have transpiration rates exceeding that of rainforests (Röll et al., 2019). Transpiration by non-native, monocultural rubber and oil palm plantations was significantly influenced by site and season (Hardanto et al., 2017). However, such influences have not yet been assessed for ET in the remaining lowland rainforests with high tree species richness and potentially site-adapted species compositions. Therefore, our objectives were (1) to quantify ET of a tropical rainforest via UAV-based thermography and (subsequent) energy balance modeling; (2) to compare UAV-data-based ET estimates to transpiration rates derived from independent ground-based sap flux measurements; and (3) to assess the spatial and temporal variability of ET across sites and seasons.
The study area is located in the Harapan rainforest (98,455 ha, −2.23333°, 103.31667°) which is a moist evergreen forest in Jambi province, Sumatra (Indonesia) (Laumonier and Seameo-Biotrop, 1997). The climate is tropical humid with relatively constant average daily air temperature (Drescher et al., 2016). The study area has a mean annual temperature of 26.7 ± 0.4°C, and a mean annual rainfall of 2075.4 ± 94 mm (data from 2014 to 2019; Darras et al., 2019) and mean air humidity of 88 ± 2.2% (mean ± SD; data from 2014 to 2019; unpublished data). Rainfall is seasonal, with a relatively drier period occurring between June and September (average monthly precipitation <120 mm), henceforth referred to as the dry season, and the remaining months referred to as the wet season (Drescher et al., 2016). The terrain is low-lying (30–120 m a.s.l.) and gently undulating (Harrison and Swinfield, 2015). The forest is partly degraded due to a history of selective logging and has been classified as ‘primary degraded forest’ (Margono et al., 2014; Drescher et al., 2016), but since 2008 is a designated protected area called PT. Restorasi Ekosistem Indonesia (PT. REKI). In areas that have remained relatively intact, eight plots of 50 m×50 m size were established, with four plots located at upland sites (Drescher et al., 2016) and four at riparian sites (Hennings et al., 2021; Supplementary Figure 1). The upland and riparian sites have a mean elevation of 61 m and 42 m a.s.l., respectively (Camarretta et al., 2021). The riparian sites have small streams running through or along them and are subject to intermittent flooding during the wet season (Paoletti et al., 2018). A tree inventory of the four upland sites found a total of 380 tree species (data from 2017; tree diameter at breast height ≥10 cm) with a tree density of 652 trees ha–1 and sum of basal area of 30 m2 ha–1 (Rembold et al., 2017). At the four riparian sites, a total of 308 species were found (tree diameter at breast height ≥10 cm) with 553 trees ha–1and a basal area of 21 m2 ha–1 (data from 2017; Brambach and Kreft, unpublished data). Further study site and forest inventory information in Supplementary Table 1. The trees at the riparian sites include more trees of the Macaranga genus an indicator of forest disturbance, were of smaller stature and had a lower biomass compared to the upland sites (Ahongshangbam et al., 2020; Kotowska and Waite, unpublished data; Remboldt et al., unpublished data). Soils in the area have been classified as sandy-loam Acrisols and clay-loam Stagnosols at the upland and riparian sites, respectively (Guillaume et al., 2015; Koks, 2019).
The UAV flights were conducted in three time periods: November 2016 (wet season; n = 12; 5 days), July 2017 (early dry season; n = 11; 7 days) and September 2017 (late dry season; n = 21; 8 days). Upscaling methods using evaporative fraction to convert instantaneous ET to daily ET performed best for forests at solar noon (Jiang et al., 2021). However, constantly varying weather conditions especially during the rainy season imposed a challenge to conduct the flight mission exactly at noon. Therefore, we planned one flight every hour within a time window of 10:00 to 14:00 h for each plot and season. An octocopter UAV (MikroKopter EASY Okto V3, HiSystems GmbH, Germany) was fitted with a radiometric thermal sensor (FLIR Tau 2 640, FLIR Systems, USA) and a ThermalCapture module add-on which enables uniform thermal measurements and increases the accuracy of absolute LST measurements (TeAx Technology, Germany). The sensor measures radiation in the TIR spectral band (7.5–13.5 μm) with a relative thermal sensitivity of 0.05 K. The thermal camera has an optical resolution of 640 × 512 pixels and a field of view of 45°× 37° (f = 13 mm). The camera was mounted on a gimbal to ensure nadir view. An onboard GPS (MKBNSS V3 GPS/GLONASS, HiSystems, Germany) was used for recording location and time. Flight planning was conducted using the MikroKopter-Tool V2.14b software (HiSystems GmbH, 2016). Flight paths were designed as superimposed circular and grid patterns to ensure high overlap (minimum 80%) between images and a flight altitude of 80 m above ground and on average approximately 40 m above the canopy.
For each flight, individual TIR frames were matched with the according geolocation via their time stamps. This resulted in an average of 199, 135, and 396 TIR frames from each flight for the time periods November 2016, July 2017, and September 2017, respectively. The images were then exported to Agisoft Metashape Professional v1.7.2 software (Agisoft LLC, 2021, Russia; RRID:SCR_018119)] and blurry images were visually identified and removed before further processing. No processing of temperature data was necessary as the images are radiometric wherein each pixel contains an absolute temperature value. The built-in workflow in Agisoft Metashape Professional v1.7.2 software was used to create LST orthomosaic maps by stitching together the selected individual TIR frames from each flight. The orthomosaics have a resolution of 10 cm px–1. The LST orthomosaics were then exported to QGIS3 and clipped to the exact plot boundaries (QGIS Association, 2021; RRID:SCR_018507). Next, a quality filter was applied to all orthomosaics based on percentage of pixels containing temperature data within the respective (50×50 m) plot boundaries (total pixels = data pixels+no data pixels for a given plot), and those with ≥66.6% data coverage were selected for energy balance modeling, yielding a final sample of n = 44 flight missions.
To estimate ET maps of the rainforest from the LST orthomosaics, we used the DATTUTDUT energy-balance model (Timmermans et al., 2015). The model works by parameterizing each variable in the energy-balance equation in terms of the absolute temperature at each pixel and the temperature extremes where the minimum temperature corresponds to the 0.5% lowest temperature and the maximum temperature to hottest pixel in the image, within the respective plot boundaries. We applied the QGIS3 plug-in QWaterModel v1.4 (Ellsäßer et al., 2020b), which implements the DATTUTDUT model with a graphical user interface. Energy-balance modeling is sensitive to the pixel resolution, so all LST maps were set to the native resolution (10 cm px–1) as recommended in previous studies applying the DATTUTDUT model (Xia et al., 2016; Brenner et al., 2018). Previous studies also reported that the accuracy of ET predictions with the DATTUTDUT model compared to reference eddy covariance measurements increased when measured solar radiation (Rs, W m–2) was included, rather than modeling radiation from location and time of day (Brenner et al., 2018; Ellsäßer et al., 2020b). We thus used ground-based Rs measurements from an onsite meteorological station as model input. The Rs data were measured using a global radiation sensor (CMP3 Pyranometer, Kipp & Zonen, Delf, the Netherlands) installed at a height of 3 m above ground (Meijide et al., 2018). The input parameters atmospheric transmissivity, atmospheric emissivity and surface emissivity remained at their default values (Timmermans et al., 2015), and the time period was set to hourly (3600 s). For each LST orthomosaic input, QWaterModel provides a six-band raster containing net radiation (Rn, W m–2), sensible heat flux (H, W m–2), latent heat flux (LE, W m–2), ground heat flux (G, W m–2), evaporative fraction (EF) and evapotranspiration (ET, mm h–1). Example ET maps of all eight study plots from the late dry season are provided in Figure 1.
Figure 1. Evapotranspiration (ET) maps (resolution: 10 cm px– 1) from the Harapan rainforest at four upland (HF) and four riparian (HFr) plots in the late dry season (September, 2017; solar radiation ≥700 W m– 2).
An independent study estimated transpiration rates for 36 trees in the riparian plots using ground-based sap flux measurements (Ahongshangbam et al., 2020). Sap flux was measured using the thermal dissipation method which estimates the sap flux density (Js) (Granier, 1985). The measurements were taken between September and November 2016 and overlap with UAV missions of this study from the wet season. A relationship between water conductive area (Ac, cm2) and tree diameter at breast height derived for forest trees in the study region was used to estimate Ac for each tree (Röll et al., 2019). The Js values were averaged to obtain mean daily Js [g cm–2 d–1] and multiplied with the Ac [cm2] of each tree to derive mean daily water use of the whole tree [kg d–1] (henceforth WU). For the comparison of sap flux-derived transpiration to the UAV-derived ET estimates, WU data of three sunny days were averaged for each tree i.e., the values represent the maximum daily tree water use.
For estimating hourly ET [mm h–1] of the 36 sap flux trees, shapefiles delineating the crowns as available from a previous study (Ahongshangbam et al., 2020) were clipped to the DATTUTDUT-derived ET maps, and all pixels within a respective crown were averaged. To align the ET estimates of tree crowns with the daily time step of the WU data, the evaporative fraction (EF) method was applied (Jackson et al., 1983; Jiang et al., 2021) to obtain daily evapotranspiration, ET24 [kg m–2 d–1]. This method is applicable for cloud-free days and performs best for forest ecosystems with values obtained close to noon. To account for variability in cloud cover we used measured solar radiation (Rs) data.
where, LE is the latent heat flux [W m–2], Rs,24 is the daily solar radiation [MJ m–2 d–1], Rs,h is the mean hourly solar radiation [W m–2] and λ is the latent heat of vaporization [MJ kg–1]. The subscripts h and 24 define hourly and 24-h time period, respectively. Rs was averaged over a 24-h time period to calculate the daily average solar radiation [W m–2] and converted to MJ m–2 d–1 using a conversion factor of 0.0864 (Allen et al., 1998). The latent heat of vaporization (λ) is estimated following Timmermans et al. (2015):
Tmin [K] is the minimum temperature within the image, taken as the 0.5% quantile of the pixel values. To convert the daily ET values per unit ground area [kg m–2 d–1] to daily ET per tree [kg d–1], they were multiplied by the respective crown projection areas [m2] of these trees as available from a previous study (Ahongshangbam et al., 2020).
Using a Passing-Bablok (PB) regression model, we compared ET predictions from the UAV-based method to independent sap flux measurements as both methods have associated and unknown measurement uncertainties (Passing and Bablok, 1983). The residuals did not follow a normal distribution based on visual inspection of residuals vs. fitted plot (Legendre and Legendre, 2012). However, the PB regression does not require any assumptions about the sample distribution or measurement errors and is not sensitive to outliers (Passing and Bablok, 1983; Bilić-Zulle, 2011). This method fits the intercept and slope of the linear equation. If the confidence intervals of the intercept and slope do not include 1 and 0, it indicates statistically significant bias between the methods (Passing and Bablok, 1983; Legendre and Legendre, 2012). Further, we used a Bland-Altman (BA) plot to visualize differences between the methods (Bland and Altman, 1999). The BA plot is a graphical approach to compare two measurement methods of the same variable by plotting the difference between each paired measurement against its mean (Bland and Altman, 1999). For the BA plot, we used log transformed values of original data as the assumption of normal distribution for the difference of values between the two methods was not met (Shapiro-Wilk: p > 0.05) (Bland and Altman, 1999; Giavarina, 2015). Using log-transformed data, we confirmed that the difference values are normally distributed (Shapiro-Wilk: p < 0.05) and >95% of data points fall within the ±1.96 SD of mean difference also called as the agreement interval (the range within which most differences between paired measurements will lie) (Bland and Altman, 1999). A linear regression was fitted between the differences between paired values and their means to determine the trend of proportional bias.
We used multiple linear regression analysis to test whether site and season were predictors of plot-level mean ET (ETmean model) and standard deviation of ET (ETSD model). We included solar short-wave radiation (Rs) as a covariate term in the models as we wanted to interpret the predicted ET values at a given Rs, and focused on mean ET at mean Rs. The normality of residuals was confirmed using the Shapiro-Wilk test at a 5% significance level. We used the Breusch-Pagan test to assess homoscedasticity and confirmed the residuals have equal variance at a 5% significance level. An F-test for joint significance was used to test overall effects of predictors of both models at a 5% significance level. Since the models have two categorical predictors, a generalized variance inflation factor (GVIF) was computed to test for collinearity of model predictors where the square of GVIF[1/(2 × Df)] is considered to be equivalent to variance inflation factor (VIF) values (Fox and Monette, 1992). A GVIF value < 3 indicates low collinearity for regression models and all predictors in both models were below this threshold (Zuur et al., 2010; Harrison et al., 2018; see Supplementary Table 2). Further, a Pearson’s chi-square test showed no significant correlation between the two categorical variables season and site (χ2(2, N = 44) = 2.20, p = 0.33). In order to compare our categorical predictors categorical predictors (site levels: upland, riparian and season levels: early dry, late dry, wet) we performed a contrasts analysis based on the predicted means. We estimated predicted means derived from the models using the emmeans R package to account for unbalanced data (not all plots are covered in all seasons) (Lenth, 2022; RRID:SCR_018734; Searle et al., 1980). All statistical analyses and plotting were done using R Statistical software version 4.1.2 (R Core Team, 2021; RRID:SCR_001905).
During the UAV flights, solar radiation ranged between 229 and 1095 W m–2 (mean: 729 ± 214 W m–2) representing fully cloud-covered to full-sunlight conditions. Stand-level hourly ET predicted by the DATTUTDUT model ranged from 0.07 to 0.80 mm h–1 with a mean of 0.48 ± 0.18 mm h–1 for all plots across sites and seasons (n = 44). Extrapolated to daily ET the values ranged from 1.62 to 4.42 mm d–1 (mean: 3.26 ± 0.73 mm d–1).
We compared daily ET predictions of 36 individual trees from the UAV-based approach to daily transpiration rates from independent sap flux measurements at the four riparian plots using a PB regression. It showed a high level of agreement between ET and the independent transpiration estimates (R2 = 0.67, p < 0.001, n = 36; Figure 2A). However, the 95% CIs for intercept (–270.7 and –47.1) and slope (9.8 and 19.1) of the PB regression do not include the values 0 and 1, respectively, which indicates that the two methods cannot be used interchangeably. From the BA plot, the mean difference between the two methods was on average 7.03 ± 1.8 kg d–1 (mean ± SD) after back-transforming the log values (Figure 2B).
Figure 2. (A) Comparison between thermography derived evapotranspiration (ET) and sap fluxed derived transpiration (WU). Passing-Bablok regression analysis between ET predictions and TR estimates (n = 36). Regression line equation: y = –103.56+12.06x. Here, ET refers to daily water evaporated and transpired per tree and WU to daily water transpired per tree for the same sample of trees. (B) Bland-Altman plot comparing log-transformed values of modeled ET from thermography and tree transpiration from sap flux measurements. The x- and y-axes represent the mean and the difference between values from the two methods for each tree, respectively. The black solid and dashed horizontal lines indicate the mean difference of values between the two methods and the ±1.96 SD of the difference between values from both methods, respectively. The green dashed line indicates the regression line for the mean and difference of the paired values.
Multiple linear regression analyses were performed to test the effects of site and season on plot-level mean ET (ETmean model) and within-plot heterogeneity of ET (ETSD model). The ETmean model was statistically significant (p < 0.001; Table 1). Using a F-test for joint significance testing, we found significant effects of site (p < 0.01) in this model. Predicted mean ET at the upland sites was significantly higher (11%) than at riparian sites across all seasons (p = 0.003) (Figure 3A), while the differences in predicted ET between seasons were not significant (Figure 3B). The ETSD model was also statistically significant (p < 0.001; Table 1). The F-test indicated significant effects of season (p < 0.01) and site (p < 0.05) in this model. The within-plot heterogeneity of ET was significantly lower at upland sites (7%) than at riparian sites (p = 0.045) (Figure 3C). Effects of season on variability of ET were found between early dry and rainy seasons (p = 0.053) as well as the late dry and rainy seasons (p = 0.002; Figure 3D). Therein, ETSD was enhanced by 14% from the late dry season to the wet season.
Table 1. Linear model summaries with mean evapotranspiration (ETmean) and its standard deviation (ETSD) as response variables and site, season and solar radiation as predictors.
Figure 3. Evapotranspiration (ET) from the Harapan rainforest across sites and season. Predicted means of site and season from the ETmean model (A,B) and ETsd model (C,D) are shown. The predicted means were adjusted for mean covariate values (solar radiation of 730 W m– 2). Means not sharing any letter are significantly different by the Tukey-test at the 5% level of significance.
The mean daily ET in our rainforest study (3.26 ± 0.73 mm d–1) was similar to values reported from other tropical rainforests e.g., in the Amazon (3.51 ± 0.75 mm d–1, Da Rocha et al., 2004; 3.58 mm d–1, 3.49 mm d–1, 3.57 mm d–1, 3.11 mm d–1, Costa et al., 2010) and in a lowland dipterocarp forest in Peninsular Malaysia (3.24 ± 0.86 mm d–1, Lion et al., 2017). Our hourly ET values for lowland rainforests are comparable to ET rates derived from a nearby commercial, mature oil palm plantation where the DATTUTDUT approach had been validated with eddy covariance measurements (Meijide et al., 2017; Ellsäßer et al., 2021). This finding is in line with previous work reporting that stand-scale (evapo)transpiration of commercial oil palm plantations can match or even surpass rates observed in previously logged lowland rainforests (Röll et al., 2019).
We compared UAV-based ET predictions to transpiration rates estimated from in-situ sap flux measurements for 36 individual trees and found a strong positive correlation between the two methods, thereby corroborating the observed spatial patterns of forest ET. This UAV-based method has been previously tested against eddy covariance measurements in a nearby, mature, oil palm plantation where the results indicated a high congruence of ET estimates between the two methods for variable weather conditions and times of day (Ellsäßer et al., 2021). However, we also observed statistically significant bias between the methods with substantially lower values derived from sap flux measurements. This may be attributed to several factors. First of all, each method measures a different flux. The UAV-based method captures ET which is the sum of all transpiration and evaporation from the land surface while the sap flux method estimates tree transpiration only. A tropical rainforest also comprises of other plants such as epiphytes and lianas, and in addition, there is the understorey layer both of which can contribute significantly to net ecosystem ET (Jiménez-Rodríguez et al., 2020; Miller et al., 2021). Further, evaporation of rainfall intercepted by vegetation, in particular during the wet season, as well as from creeks running through the riparian sites may have contributed substantially to total ET (Zhong et al., 2022). Additionally, previous studies reported that the thermal dissipation probe method can substantially underestimates sap flux density of forest trees when species-specific calibration is not carried out (Fuchs et al., 2017; Flo et al., 2019). In addition to the measurement uncertainties associated with both methods, these factors may contribute to the high observed differences between UAV-derived ET and ground measurement-based transpiration.
In our study, ET from upland sites was 11% higher than from riparian sites. Similar findings i.e., higher transpiration at upland than at riparian rainforest sites, were reported in a previous study encompassing the same study sites, with an approach of upscaling sap flux measurements to stand transpiration via UAV-derived photogrammetry (Ahongshangbam et al., 2020). Likewise, a sap flux study in the same region reported higher transpiration of oil palm and rubber plantations at upland sites compared to adjacent valley sites (Hardanto et al., 2017). Further, our results are in line with previous studies reporting that ET rates from forests are influenced landscape position (Mackay et al., 2010; Burenina et al., 2022). Other factor such as species composition, stand structure and topographic characteristics (e.g., slope, orientation, drainage position) were linked to transpiration patterns in forests in previous studies (Daws et al., 2002; Mitchell et al., 2012; Metzen et al., 2019). Indeed, forest structure varied notably between our upland and riparian study sites. The trees at the riparian sites were of smaller stature, had on average 43% lower biomass compared to the upland sites and showed signs of forest disturbance (Ahongshangbam et al., 2020; Kotowska and Waite, unpublished results; Rembold et al., unpublished results). There is evidence from studies correlating ET rate to forest structure variables such as leaf area index (LAI), sapwood area, height and tree biomass with ET in general increasing with these variables (Álvarez-Dávila et al., 2017; Jaramillo et al., 2018; Metzen et al., 2019; Valdés-Uribe et al., 2023). The history of disturbance and the generally smaller trees at the riparian sites suggests regenerating forest stands, which may also influence transpiration rates (Ghimire et al., 2022). We also found that within-plot heterogeneity of ET was significantly higher at riparian sites compared to upland sites across seasons (7%), which may stem from more heterogeneous vegetation cover at riparian sites. Overall, our findings highlight the importance of topographic position and local site conditions in explaining spatial variability of ET in a tropical rainforest.
We found no significant differences in mean ET across seasons at a given level of radiation. The sustained ET rates during the dry season may indicate that our study sites in the Harapan rainforest are not strongly water-limited. Our findings are in line with studies reporting that ET rates from tropical forests were similar or even higher during the dry season as compared to the wet season (Da Rocha et al., 2004; Hutyra et al., 2007; Negrón-Juárez et al., 2007; Costa et al., 2010). We also observed a tendency toward reduced ET in the wet season, which may be due to atmospheric characteristics such as lower vapor pressure deficit but may also be influenced by soil moisture and partial flooding conditions. In our study, season was a significant factor in explaining the within-plot heterogeneity of ET. During the wet season an increased heterogeneity of ET was observed which was significantly higher than in the late dry season (14%). This seasonally enhanced variability can be an effect of local site conditions including partial flooding and diverse responses of different tree species to moisture conditions.
In a broader context, UAV-based assessments of ET based on a simple approach requiring minimal data can be useful in several contexts. In numerous tropical regions, forest conversion to agriculture and forest degradation continues at high rates with severe impacts on local to global climate systems (Bala et al., 2007; Malhi et al., 2014; Sabajo et al., 2017). Thereby, development of near real-time ET assessment methods can be valuable to better understand consequences of rainforest conversion on ET, especially in regions with limited availability of meteorological variables required by more complex energy-balance ET models. Further, by expanding the applicability of the DATTUTDUT model to a more complex ecosystem, it may facilitate landscape-scale assessments of ET including various land covers. Given the large potential and need for ecosystem restoration in rainforest regions, our study can provide reference information from near-natural vegetation regarding ET from the Harapan forest which is a large-scale (≈ 100,000 ha) ecosystem restoration area (Harrison and Swinfield, 2015; Brancalion et al., 2019; van Noordwijk et al., 2022). In this context, assessments of ET and its variability in tropical rainforests are of particular importance to narrow persisting observation and knowledge gaps in thus far underrepresented ecosystems. UAV-based ET assessments provide high-resolution reference data that are rapidly available and less cumbersome than manual ground truthing methods and might therefore be a valuable asset to reference large scale satellite data-based studies (Suir et al., 2021) useful for large-scale monitoring of ET. Lastly, tropical forests exert a net biophysical cooling effect at the global level which is important for climate change mitigation; concurrently changes to forest cover, structure, composition affect water and energy balances (Lawrence et al., 2022). The presented methodology is able to capture the complex spatio-temporal variability of ET and highlights the importance of including local conditions in order to reduce uncertainties arising from the simplification of hydrological processes. This can improve our understanding of the response of tropical forests to climate change with respect to the hydrological cycle and can inform local adaptation strategies.
In a lowland tropical rainforest, with undulating terrain and seasonal fluctuations of rainfall, we estimated ET using UAV-acquired TIR imaging and a simple modeling scheme using the DATTUTDUT model and assessed its variability across sites and seasons. While previous studies have tested this approach over crops and grassland, we applied this method to tropical forests. Testing this method against independent sap flux measurements corroborated the observed spatial patterns of ET. Applying the UAV-based method, we found that site and season significantly contributed to the variability of ET. While mean ET was higher at upland sites, riparian sites exhibited a higher spatial variability of ET. Our results expand the applicability of the fast-developing UAV-based methods in ecohydrological research to tropical forests. Our approach using a simple-to-use and implement modeling scheme might be useful for ET assessments in data-scarce regions. Further, the results can support the development of vegetation-atmosphere models by including site-specific and seasonal differences of ET in their calibration. Forest ecosystems significantly influence the hydrological balance within a watershed and these results can improve our understanding of the response of forests to a warming climate with regard to water use and inform local adaptation efforts to climate change.
The raw data supporting the conclusions of this article will be made available by the authors, without undue reservation.
DH conceptualized the study in cooperation with HH. MB led the writing of the manuscript. DH supervised the work. FE designed the field campaign, collected the UAV data, and supported the processing of the data. JA provided the sap flux data. CS provided the meteorological data. MB conducted the data processing, model application, statistical analysis, and production of plots in cooperation mainly with DH. IM provided statistical advice. AR supported the data processing, analysis, and supervision. MB and DH created a first version of the manuscript, which was further improved in cooperation with all co-authors. All authors contributed to the article and approved the submitted version.
This study was funded by the Deutsche Forschungsgemeinschaft (DFG, German Research Foundation)—project number 192626868—in the framework of the collaborative German-Indonesian research project CRC990 (subprojects A02, A03, and Z02).
We thank the Ministry of Research, Technology and Higher Education (Ristekdikti) for providing the research permit for the field work (nos. 322/SIP/FRP/E5 /Dit.KI/IX/2016, 329/SIP/FRP/E5/Dit.KI/IX/2016, and 28/EXT/ SIP/FRP/E5/Dit.KI/VII/2017).
The authors declare that the research was conducted in the absence of any commercial or financial relationships that could be construed as a potential conflict of interest.
All claims expressed in this article are solely those of the authors and do not necessarily represent those of their affiliated organizations, or those of the publisher, the editors and the reviewers. Any product that may be evaluated in this article, or claim that may be made by its manufacturer, is not guaranteed or endorsed by the publisher.
The Supplementary Material for this article can be found online at: https://www.frontiersin.org/articles/10.3389/ffgc.2023.1232410/full#supplementary-material
Abtew, W., and Melesse, A. (2013). “Climate change and evapotranspiration,” in Evaporation and evapotranspiration, eds W. Abtew and A. Melesse (Dordrecht: Springer), 197–202.
Acharya, B. S., Bhandari, M., Bandini, F., Pizarro, A., Perks, M., Joshi, D. R., et al. (2021). Unmanned aerial vehicles in hydrology and water management: Applications, challenges, and perspectives. Water Resour. Res. 57, 1–33. doi: 10.1029/2021WR029925
Agisoft LLC (2021). Agisoft metashape professional edition (Version 1.7.2) [Photogrammetric Software]. Available online at: https://www.agisoft.com/downloads/installer (accessed February 3, 2021).
Ahongshangbam, J., Röll, A., Ellsäßer, F., Hendrayanto, H., and Hölscher, D. (2020). Airborne tree crown detection for predicting spatial heterogeneity of canopy transpiration in a tropical rainforest. Remote Sens. 12:651. doi: 10.3390/rs12040651
Allen, R. G., Pereira, L. S., Howell, T. A., and Jensen, M. E. (2011). Evapotranspiration information reporting: I. Factors governing measurement accuracy. Agric. Water Manag. 98, 899–920. doi: 10.1016/j.agwat.2010.12.015
Allen, R. G., Pereira, L. S., Raes, D., and Smith, M. (1998). Crop evapotranspiration: Guidelines for computing crop water requirements. Rome: FAO.
Allen, R. G., Tasumi, M., and Trezza, R. (2007). Satellite-based energy balance for Mapping Evapotranspiration with Internalized Calibration (METRIC)—Model. J. Irrig. Drain Eng. 133, 380–394. doi: 10.1061/(ASCE)0733-94372007133:4(380)
Álvarez-Dávila, E., Cayuela, L., González-Caro, S., Aldana, A. M., Stevenson, P. R., Phillips, O., et al. (2017). Forest biomass density across large climate gradients in northern South America is related to water availability but not with temperature. PLoS One 12:e0171072. doi: 10.1371/journal.pone.0171072
Bala, G., Caldeira, K., Wickett, M., Phillips, T. J., Lobell, D. B., Delire, C., et al. (2007). Combined climate and carbon-cycle effects of large-scale deforestation. Proc. Natl. Acad. Sci. U.S.A. 104, 6550–6555. doi: 10.1073/pnas.0608998104
Baldocchi, D. D. (2003). Assessing the eddy covariance technique for evaluating carbon dioxide exchange rates of ecosystems: Past, present and future. Glob. Change Biol. 9, 479–492. doi: 10.1046/j.1365-2486.2003.00629.x
Bilić-Zulle, L. (2011). Comparison of methods: Passing and Bablok regression. Biochem. Med. 21, 49–52. doi: 10.11613/bm.2011.010
Bland, J. M., and Altman, D. G. (1999). Measuring agreement in method comparison studies. Stat. Methods Med. Res. 8, 135–160. doi: 10.1177/096228029900800204
Brancalion, P. H. S., Niamir, A., Broadbent, E., Crouzeilles, R., Barros, F. S. M., Almeyda Zambrano, A. M., et al. (2019). Global restoration opportunities in tropical rainforest landscapes. Sci. Adv. 5:eaav3223. doi: 10.1126/sciadv.aav3223
Brenner, C., Zeeman, M., Bernhardt, M., and Schulz, K. (2018). Estimation of evapotranspiration of temperate grassland based on high-resolution thermal and visible range imagery from unmanned aerial systems. Int. J. Remote Sens. 39, 5141–5174. doi: 10.1080/01431161.2018.1471550
Burenina, T. A., Danilova, I. V., and Mikheeva, N. A. (2022). Spatial-temporal dynamics of evapotranspiration in the podkamennaya Tunguska River Basin. Contemp. Probl. Ecol. 15, 449–458. doi: 10.1134/S1995425522050043
Camarretta, N., Knohl, A., Erasmi, S., Schlund, M., Seidel, D., and Ehbrecht, M. (2021). Data from: ALS metrics for core plots. GRO data. Available online at: https://doi.org/10.25625/AIKOI9 (accessed March 15, 2022).
Chen, J. M., Chen, X., Ju, W., and Geng, X. (2005). Distributed hydrological model for mapping evapotranspiration using remote sensing inputs. J. Hydrol. 305, 15–39. doi: 10.1016/j.jhydrol.2004.08.029
Clough, Y., Krishna, V. V., Corre, M. D., Darras, K., Denmead, L. H., Meijide, A., et al. (2016). Land-use choices follow profitability at the expense of ecological functions in Indonesian smallholder landscapes. Nat. Commun. 7:13137. doi: 10.1038/ncomms13137
Costa, M. H., Biajoli, M. C., Sanches, L., Malhado, A. C. M., Hutyra, L. R., Da Rocha, H. R., et al. (2010). Atmospheric versus vegetation controls of Amazonian tropical rain forest evapotranspiration: Are the wet and seasonally dry rain forests any different? J. Geophys. Res. 115, 1–9. doi: 10.1029/2009JG001179
Da Rocha, H. R., Goulden, M. L., Miller, S. D., Menton, M. C., Pinto, L. D. V. O., de Freitas, H. C., et al. (2004). Seasonality of water and heat fluxes over a tropical forest in Eastern Amazonia. Ecol. Appl. 14, 22–32. doi: 10.1890/02-6001
Da Rocha, H. R., Manzi, A. O., Cabral, O. M., Miller, S. D., Goulden, M. L., Saleska, S. R., et al. (2009). Patterns of water and heat flux across a biome gradient from tropical forest to savanna in Brazil. J. Geophys. Res. 114:G00B12. doi: 10.1029/2007JG000640
Darras, K. F. A., Corre, M. D., Formaglio, G., Tjoa, A., Potapov, A., Brambach, F., et al. (2019). Reducing fertilizer and avoiding herbicides in oil palm plantations—ecological and economic valuations. Front. For. Glob. Change 2:65. doi: 10.3389/ffgc.2019.00065
Daws, M. I., Mullins, C. E., Burslem, D. F., Paton, S. R., and Dalling, J. W. (2002). Topographic position affects the water regime in a semideciduous tropical forest in Panamá. Plant Soil 238, 79–90. doi: 10.1023/A:1014289930621
Drescher, J., Rembold, K., Allen, K., Beckschäfer, P., Buchori, D., Clough, Y., et al. (2016). Ecological and socio-economic functions across tropical land use systems after rainforest conversion. Philos. Trans. R. Soc. B 371:20150275. doi: 10.1098/rstb.2015.0275
Ellsäßer, F., Röll, A., Ahongshangbam, J., Waite, P.-A., Hendrayanto, Schuldt, B., et al. (2020a). Predicting tree sap flux and stomatal conductance from drone-recorded surface temperatures in a mixed agroforestry system—A machine learning approach. Remote Sens. 12:4070. doi: 10.3390/rs12244070
Ellsäßer, F., Röll, A., Stiegler, C., Hendrayanto, and Hölscher, D. (2020b). Introducing QWaterModel, a QGIS plugin for predicting evapotranspiration from land surface temperatures. Environ. Modell. Softw. 130:104739. doi: 10.1016/j.envsoft.2020.104739
Ellsäßer, F., Stiegler, C., Röll, A., June, T., Knohl, A., and Hölscher, D. (2021). Predicting evapotranspiration from drone-based thermography – a method comparison in a tropical oil palm plantation. Biogeosciences 18, 861–872. doi: 10.5194/bg-18-861-2021
ESRI (2022). World imagery. Available online at: https://www.arcgis.com/home/item.html?id=10df2279f9684e4a9f6a7f08febac2a9 (accessed April 19, 2022).
Flo, V., Martinez-Vilalta, J., Steppe, K., Schuldt, B., and Poyatos, R. (2019). A synthesis of bias and uncertainty in sap flow methods. Agric. For. Meteorol. 271, 362–374. doi: 10.1016/j.agrformet.2019.03.012
Fox, J., and Monette, G. (1992). Generalized collinearity diagnostics. J. Am. Stat. Assoc. 87, 178–183. doi: 10.2307/2290467
Fuchs, S., Leuschner, C., Link, R., Coners, H., and Schuldt, B. (2017). Calibration and comparison of thermal dissipation, heat ratio and heat field deformation sap flow probes for diffuse-porous trees. Agric. For. Meteorol. 24, 151–161. doi: 10.1016/j.agrformet.2017.04.003
Ghimire, C. P., van Meerveld, H. J., Zwartendijk, B. W., Bruijnzeel, L. A., Ravelona, M., Lahitiana, J., et al. (2022). Vapour pressure deficit and solar radiation are the major drivers of transpiration in montane tropical secondary forests in eastern Madagascar. Agric. For. Meteorol. 326:109159. doi: 10.1016/j.agrformet.2022.109159
Giavarina, D. (2015). Understanding bland altman analysis. Biochem. Med. 25, 141–151. doi: 10.11613/BM.2015.015
Granier, A. (1985). Une nouvelle méthode pour la mesure du flux de sève brute dans le tronc des arbres. Ann. For. Sci. 42, 193–200.
Groombridge, B., and Jenkins, M. (2002). World atlas of biodiversity: Earth’s living resources in the 21st century. Berkeley: UNEP World Conservation Monitoring Centre.
Guillaume, T., Damris, M., and Kuzyakov, Y. (2015). Losses of soil carbon by converting tropical forest to plantations: Erosion and decomposition estimated by δ(13) C. Glob. Change Biol. 21, 3548–3560. doi: 10.1111/gcb.12907
Gutierrez Lopez, J., Tor-Ngern, P., Oren, R., Kozii, N., Laudon, H., and Hasselquist, N. J. (2021). How tree species, tree size, and topographical location influenced tree transpiration in northern boreal forests during the historic 2018 drought. Glob. Change Biol. 27, 3066–3078. doi: 10.1111/gcb.15601
Hardanto, A., Röll, A., Niu, F., Meijide, A., Hendrayanto, and Hölscher, D. (2017). Oil palm and rubber tree water use patterns: Effects of topography and flooding. Front. Plant Sci. 8:452. doi: 10.3389/fpls.2017.00452
Harrison, R. D., and Swinfield, T. (2015). Restoration of logged humid tropical forests: An experimental programme at Harapan Rainforest, Indonesia. Trop. Conserv. Sci. 8, 4–16. doi: 10.1177/194008291500800103
Harrison, X. A., Donaldson, L., Correa-Cano, M. E., Evans, J., Fisher, D. N., Goodwin, C. E. D., et al. (2018). A brief introduction to mixed effects modelling and multi-model inference in ecology. PeerJ 6:e4794. doi: 10.7717/peerj.4794
Hennings, N., Becker, J. N., Guillaume, T., Damris, M., Dippold, M. A., and Kuzyakov, Y. (2021). Riparian wetland properties counter the effect of land-use change on soil carbon stocks after rainforest conversion to plantations. Catena 196:104941. doi: 10.1016/j.catena.2020.104941
Hill, D. J., Pypker, T. G., and Church, J. (2020). “Applications of Unpiloted Aerial Vehicles (UAVs) in forest hydrology,” in Forest-water interactions, eds D. F. Levia, D. E. Carlyle-Moses, S. Iida, B. Michalzik, K. Nanko, and A. Tischer (Cham: Springer), 55–85.
Hutyra, L. R., Munger, J. W., Saleska, S. R., Gottlieb, E., Daube, B. C., Dunn, A. L., et al. (2007). Seasonal controls on the exchange of carbon and water in an Amazonian rain forest. J. Geophys. Res. 112, 1–16. doi: 10.1029/2006JG000365
Jackson, R. D., Hatfield, J. L., Reginato, R. J., Idso, S. B., and Pinter, P. J. (1983). Estimation of daily evapotranspiration from one time-of-day measurements. Agric. Water Manage. 7, 351–362. doi: 10.1016/0378-3774(83)90095-1
Jaramillo, F., Cory, N., Arheimer, B., Laudon, H., van der Velde, Y., Hasper, T. B., et al. (2018). Dominant effect of increasing forest biomass on evapotranspiration: Interpretations of movement in Budyko space. Hydrol. Earth Syst. Sci. 22, 567–580. doi: 10.5194/hess-22-567-2018
Jiang, L., Zhang, B., Han, S., Chen, H., and Wei, Z. (2021). Upscaling evapotranspiration from the instantaneous to the daily time scale: Assessing six methods including an optimized coefficient based on worldwide eddy covariance flux network. J. Hydrol. 596:126135. doi: 10.1016/j.jhydrol.2021.126135
Jiménez-Rodríguez, C. D., Coenders-Gerrits, M., Wenninger, J., Gonzalez-Angarita, A., and Savenije, H. (2020). Contribution of understory evaporation in a tropical wet forest during the dry season. Hydrol. Earth Syst. Sci. 24, 2179–2206. doi: 10.5194/hess-24-2179-2020
Kang, S., Lee, D., and Kimball, J. S. (2004). The effects of spatial aggregation of complex topography on hydroecological process simulations within a rugged forest landscape: Development and application of a satellite-based topoclimatic model. Can. J. For. Res. 34, 519–530. doi: 10.1139/x03-213
Koks, J. A. (2019). Tropical forest conversion to rubber and oil palm plantations: Landscape-scale and inter-annual variability of soil Greenhouse Gas (GHG) fluxes and the contribution of tree-stem emissions to the soil GHG budget in Jambi province, Sumatra, Indonesia. [Doctoral Thesis]. Göttingen: Georg-August-Universität Göttingen, Faculty of Forest Sciences and Forest Ecology.
Kustas, W. P., Norman, J. M., Anderson, M. C., and French, A. N. (2003). Estimating subpixel surface temperatures and energy fluxes from the vegetation index–radiometric temperature relationship. Remote Sens. Environ. 85, 429–440. doi: 10.1016/S0034-4257(03)00036-1
Lapidot, O., Ignat, T., Rud, R., Rog, I., Alchanatis, V., and Klein, T. (2019). Use of thermal imaging to detect evaporative cooling in coniferous and broadleaved tree species of the Mediterranean maquis. Agric. For. Meteorol. 271, 285–294. doi: 10.1016/j.agrformet.2019.02.014
Laumonier, Y., and Seameo-Biotrop (1997). The vegetation and physiography of sumatra. Dordrecht: Springer.
Lawrence, D., Coe, M., Walker, W., Verchot, L., and Vandecar, K. (2022). The unseen effects of deforestation: Biophysical effects on climate. Front. For. Glob. Change 5:756115. doi: 10.3389/ffgc.2022.756115
Lenth, R. V. (2022). emmeans: Estimated marginal means, aka least-squares means (version 1.7.2). Available online at: https://cran.r-project.org/package=emmeans (accessed November 16, 2022).
Lion, M., Kosugi, Y., Takanashi, S., Noguchi, S., Itoh, M., Katsuyama, M., et al. (2017). Evapotranspiration and water source of a tropical rainforest in peninsular Malaysia. Hydrol. Process. 31, 4338–4353. doi: 10.1002/hyp.11360
Loranty, M. M., Mackay, D. S., Ewers, B. E., Adelman, J. D., and Kruger, E. L. (2008). Environmental drivers of spatial variation in whole-tree transpiration in an aspen-dominated upland-to-wetland forest gradient. Water Resour. Res. 44, 1–15. doi: 10.1029/2007WR006272
Mackay, D. S., Ewers, B. E., Loranty, M. M., and Kruger, E. L. (2010). On the representativeness of plot size and location for scaling transpiration from trees to a stand. J. Geophys. Res. 115:G02016. doi: 10.1029/2009JG001092
Malhi, Y., Gardner, T. A., Goldsmith, G. R., Silman, M. R., and Zelazowski, P. (2014). Tropical Forests in the Anthropocene. Annu. Rev. Environ. Resour. 39, 125–159. doi: 10.1146/annurev-environ-030713-155141
Margono, B. A., Potapov, P. V., Turubanova, S., Stolle, F., and Hansen, M. C. (2014). Primary forest cover loss in Indonesia over 2000–2012. Nat. Clim. Change 4, 730–735. doi: 10.1038/nclimate2277
Meijide, A., Badu, C. S., Moyano, F., Tiralla, N., Gunawan, D., and Knohl, A. (2018). Impact of forest conversion to oil palm and rubber plantations on microclimate and the role of the 2015 ENSO event. Agric. For. Meteorol. 252, 208–219. doi: 10.1016/j.agrformet.2018.01.013
Meijide, A., Röll, A., Fan, Y., Herbst, M., Niu, F., Tiedemann, F., et al. (2017). Controls of water and energy fluxes in oil palm plantations: Environmental variables and oil palm age. Agric. For. Meteorol. 239, 71–85. doi: 10.1016/j.agrformet.2017.02.034
Melati, D. N. (2017). The use of remote sensing data to monitor land use systems and forest variables of the tropical rainforest landscape under transformation in Jambi Province, Sumatra, Indonesia. [Ph D Dissertation]. Göttingen: Georg-August-Universität Göttingen, Faculty of Forest Sciences and Forest Ecology.
Metzen, D., Sheridan, G. J., Benyon, R. G., Bolstad, P. V., Griebel, A., and Lane, P. N. J. (2019). Spatio-temporal transpiration patterns reflect vegetation structure in complex upland terrain. Sci. Total Environ. 694:133551. doi: 10.1016/j.scitotenv.2019.07.357
Miller, G., Hartzell, S., and Porporato, A. (2021). Ecohydrology of epiphytes: Modelling water balance, CAM photosynthesis, and their climate impacts. Ecohydrology 14:e2275. doi: 10.1002/eco.2275
Mitchell, P. J., Benyon, R. G., and Lane, P. N. (2012). Responses of evapotranspiration at different topographic positions and catchment water balance following a pronounced drought in a mixed species eucalypt forest, Australia. J. Hydrol. 440-441, 62–74. doi: 10.1016/j.jhydrol.2012.03.026
Negrón-Juárez, R. I., Hodnett, M. G., Fu, R., Goulden, M. L., and von Randow, C. (2007). Control of dry season evapotranspiration over the Amazonian forest as inferred from observations at a Southern Amazon forest site. J. Clim. 20, 2827–2839. doi: 10.1175/JCLI4184.1
Oki, T., and Kanae, S. (2006). Global hydrological cycles and world water resources. Science 313, 1068–1072. doi: 10.1126/science.1128845
Paoletti, A., Darras, K., Jayanto, H., Grass, I., Kusrini, M., and Tscharntke, T. (2018). Amphibian and reptile communities of upland and riparian sites across Indonesian oil palm, rubber and forest. Glob. Ecol. Conserv. 16:e00492. doi: 10.1016/j.gecco.2018.e00492
Passing, H., and Bablok, W. (1983). A new biometrical procedure for testing the equality of measurements from two different analytical methods: Application of linear regression procedures for method comparison studies in clinical chemistry, part I. J. Clin. Chem. Clin. Biochem. 21, 709–720. doi: 10.1515/cclm.1983.21.11.709
QGIS Association (2021). QGIS Geographic Information System (version 3.18). Available online at: http://www.qgis.org (accessed November 16, 2022).
R Core Team (2021). R: A language and environment for statistical computing. Vienna: R Foundation for Statistical Computing.
Rembold, K., Mangopo, H., Tjitrosoedirdjo, S. S., and Kreft, H. (2017). Plant diversity, forest dependency, and alien plant invasions in tropical agricultural landscapes. Biol. Conserv. 213, 234–242. doi: 10.1016/j.biocon.2017.07.020
Röll, A., Niu, F., Meijide, A., Ahongshangbam, J., Ehbrecht, M., Guillaume, T., et al. (2019). Transpiration on the rebound in lowland Sumatra. Agric. For. Meteorol. 274, 160–171. doi: 10.1016/j.agrformet.2019.04.017
Sabajo, C. R., Le Maire, G., June, T., Meijide, A., Roupsard, O., and Knohl, A. (2017). Expansion of oil palm and other cash crops causes an increase of the land surface temperature in the Jambi province in Indonesia. Biogeosciences 14, 4619–4635. doi: 10.5194/bg-14-4619-2017
Searle, S. R., Speed, F. M., and Milliken, G. A. (1980). Population marginal means in the linear model: An alternative to least squares means. Am. Stat. 34, 216–221. doi: 10.2307/2684063
Shuttleworth, W. J. (1988). Evaporation from Amazonian rainforest. Proc. R. Soc. Lond. B. 233, 321–346. doi: 10.1098/rspb.1988.0024
Souza-Filho, J. D. C., Ribeiro, A., Costa, M. H., and Cohen, J. C. P. (2005). Control mechanisms of the seasonal variation of transpiration in a northeast Amazonian tropical rainforest. Acta Amazonica 35, 223–229. doi: 10.1590/S0044-59672005000200012
Suir, G. M., Saltus, C. L., Sasser, C. E., Harris, J. M., Reif, M. K., Diaz, R., et al. (2021). Evaluating drone truthing as an alternative to ground truthing: An example with wetland plant identification. Vicksburg, MS: Engineer Research and Development Center.
Taheri, M., Mohammadian, A., Ganji, F., Bigdeli, M., and Nasseri, M. (2022). Energy-based approaches in estimating actual evapotranspiration focusing on land surface temperature: A review of methods, concepts, and challenges. Energies 15:1264. doi: 10.3390/en15041264
Timmermans, W. J., Kustas, W. P., and Andreu, A. (2015). Utility of an automated thermal-based approach for monitoring evapotranspiration. Acta Geophys. 63, 1571–1608. doi: 10.1515/acgeo-2015-0016
Valdés-Uribe, A., Hölscher, D., and Röll, A. (2023). ECOSTRESS reveals the importance of topography and forest structure for evapotranspiration from a tropical forest region of the andes. Remote Sens. 15:2985. doi: 10.3390/rs15122985
van Noordwijk, M., van Oel, P., Muthuri, C., Satnarain, U., Sari, R. R., Rosero, P., et al. (2022). Mimicking nature to reduce agricultural impact on water cycles: A set of mimetrics. Outlook Agric. 51, 114–128. doi: 10.1177/00307270211073813
von Randow, C., Manzi, A. O., Kruijt, B., de Oliveira, P. J., Zanchi, F. B., Silva, R. L., et al. (2004). Comparative measurements and seasonal variations in energy and carbon exchange over forest and pasture in South West Amazonia. Theor. Appl. Climatol. 78, 5–26. doi: 10.1007/s00704-004-0041-z
Xia, T., Kustas, W. P., Anderson, M. C., Alfieri, J. G., Gao, F., McKee, L., et al. (2016). Mapping evapotranspiration with high-resolution aircraft imagery over vineyards using one- and two-source modeling schemes. Hydrol. Earth Syst. Sci. 20, 1523–1545. doi: 10.5194/hess-20-1523-2016
Zhong, F., Jiang, S., van Dijk, A. I. J. M., Ren, L., Schellekens, J., and Miralles, D. G. (2022). Revisiting large-scale interception patterns constrained by a synthesis of global experimental data. Hydrol. Earth Syst. Sci. 26, 5647–5667. doi: 10.5194/hess-26-5647-2022
Keywords: close-range sensing, DATTUTDUT, heterogeneity, land surface temperature, seasonality, site conditions
Citation: Bulusu M, Ellsäßer F, Stiegler C, Ahongshangbam J, Marques I, Hendrayanto H, Röll A and Hölscher D (2023) UAV-based thermography reveals spatial and temporal variability of evapotranspiration from a tropical rainforest. Front. For. Glob. Change 6:1232410. doi: 10.3389/ffgc.2023.1232410
Received: 31 May 2023; Accepted: 11 August 2023;
Published: 28 August 2023.
Edited by:
John T. Van Stan, Cleveland State University, United StatesReviewed by:
Kelly Cristina Tonello, Federal University of São Carlos, BrazilCopyright © 2023 Bulusu, Ellsäßer, Stiegler, Ahongshangbam, Marques, Hendrayanto, Röll and Hölscher. This is an open-access article distributed under the terms of the Creative Commons Attribution License (CC BY). The use, distribution or reproduction in other forums is permitted, provided the original author(s) and the copyright owner(s) are credited and that the original publication in this journal is cited, in accordance with accepted academic practice. No use, distribution or reproduction is permitted which does not comply with these terms.
*Correspondence: Medha Bulusu, bWVkaGEuYnVsdXN1QHVuaS1nb2V0dGluZ2VuLmRl
Disclaimer: All claims expressed in this article are solely those of the authors and do not necessarily represent those of their affiliated organizations, or those of the publisher, the editors and the reviewers. Any product that may be evaluated in this article or claim that may be made by its manufacturer is not guaranteed or endorsed by the publisher.
Research integrity at Frontiers
Learn more about the work of our research integrity team to safeguard the quality of each article we publish.