- Center for Ecological Research, Key Laboratory of Sustainable Forest, Ecosystem Management-Ministry of Education, Northeast Asia Biodiversity Research Center, Northeast Forestry University, Harbin, China
The multidimensionality of leaf traits allows plants to have diverse survival strategies to adapt to complex living environments. Whether the anatomical traits of leaves are associated with leaf economic traits and which group of traits are more strongly correlated with soil fertility factors remains unclear. We measured four leaf economic traits, four anatomical traits, and five soil fertility factors of eight coexisting broadleaf species distributed in mixed broadleaved-Korean pine (Pinus koraiensis) forests located in Northeast China. Results show a strong interdependence between economic and anatomical traits (p < 0.05). The range of variation between economic and anatomical traits were almost equal, but the causes of variation were different. Specific leaf area was positively correlated with the abaxial epidermis, negatively correlated with the ratio of spongy tissue to leaf thickness (ST/LT), and not correlated with adaxial epidermis. Leaf dry matter content was negatively correlated with the abaxial epidermis and adaxial epidermis, positively correlated with ST/LT. Specific leaf area, palisade tissue, and ST/LT showed stronger correlation with soil fertility factors than other traits. Soil fertility factors dominating trait variation were dependent upon the trait. Our results suggest anatomical traits can be considered in economic trait dimension. The coupled relationship between anatomical and economic traits is potentially a cost-effective adaptation strategy for species to improve efficiency in resource utilization. Our results provide evidence for the complex soil-trait relationship and suggest that future studies should emphasize the role of anatomic traits in predicting soil fertility changes.
1. Introduction
Plant leaf functional traits, specifically traits related to leaf persistence such as light capture and gas exchange, are important indicators in identifying plant life history strategies and predicting community assembly (Valladares and Niinemets, 2008; Terashima et al., 2011; Liu C. C. et al., 2020; Ni et al., 2022). Many studies have shown that traits vary along certain dimensions (Wright et al., 2007; Li et al., 2015; Liu C. C. et al., 2019; Yang Y. Z. et al., 2019). For example, the leaf economics spectrum dimension, represented by the negative correlation between specific leaf area (SLA), leaf nitrogen content (LN) and leaf lifespan, is the balance between plant leaf resource acquisition (higher SLA, LN but shorter leaf lifespan) and conservation (lower SLA, LN but longer leaf lifespan) (Wright et al., 2004); In recent years, anatomical traits [e.g., adaxial and abaxial epidermis (AD; AB) and palisade tissue (PT)] attracted more attention from ecologists (Chen and Wang, 2009; Santos et al., 2015), because of the critical role in light absorption and gas exchange capacity of a leaf (Evans et al., 2009; Kröber et al., 2014; Ni et al., 2022). In this dimension, thinner epidermal thickness and thicker PT tend to allow deeper light penetration and faster gas diffusion rates (Verboven et al., 2015). However, the relationship between these two trait dimensions and their response to soil fertility remains unclear.
Relationships between leaf economic and anatomical traits have been examined at the species and community level. Coble and Cavaleri (2017) reported a positive correlation between PT and leaf mass per area (the reciprocal of the SLA) in sugar maple. Liu C. C. et al. (2019) found AB was negatively correlated with leaf dry matter content (LDMC) and reported a weak correlation between anatomical and economic traits in temperate and subtropical forests. Several of the relevant studies are limited when exploring the correlation between individual traits. The correlation between anatomical and economic traits has rarely been verified, especially for co-existing species in the same forest type at a regional scale.
The correlation between soil fertility factors and leaf traits reflects the trade-off between plant growth and nutrient conservation (Liu Z. L. et al., 2020). However, which groups of functional traits are strongly associated with soil fertility gradients remains unclear (Wright et al., 2005; Maire et al., 2015; Stark et al., 2017; Peng et al., 2018). Leaf economic traits, such as SLA, are considered good predictors of soil fertility. Hodgson et al. (2011) studied the correlation between economic traits and soil fertility and found SLA had a better response to soil fertility than other traits. Widely accepted is Westoby’s (1998) conclusion that SLA is good indicator of soil fertility (Laughlin et al., 2010; De Frenne et al., 2011). Therefore, economic traits may have a stronger correlation with soil fertility factors. Anatomical traits are often reported as being closely related to the acquisition and utilization of light. PT can affect photosynthetic efficiency by regulating chloroplast content (Terashima et al., 2011; He et al., 2017) and sponge tissue can affect light density in leaves by regulating intercellular spaces (Kröber et al., 2014). Thus, anatomical traits may be more correlated with environmental factors significantly affecting the composition and structure of leaf cells and intercellular air space.
Soil fertility clearly influences the variation of leaf traits, but which soil fertility factors dominates the trait variation is unclear (Chartzoulakis et al., 2002; Ordoñez et al., 2009; Maire et al., 2015). Soil pH is closely related to soil mineralogical composition and can significantly affect the distribution of nutrients in soil (Uehara and Gilman, 1981; Quesada et al., 2010), and ultimately lead to changes in relative above-ground biomass (Zheng and Ma, 2018). Nutrient elements in the soil, which are important source of organic compounds for plants, significantly affect the variation in plant traits (Fan et al., 2015; Yang D. X. et al., 2019). Plants adapted to low soil fertility environments usually build leaves adapted to lower respiratory carbon and water loss, e.g., higher LDMC but lower LN (Ordoñe et al., 2010; Jager et al., 2015; Lin et al., 2020). In addition, soil water content (SWC) affects the efficiency of plant nutrient and water uptake and utilization by affecting soil microbial viability and root development (Liu et al., 2010; Liu C. et al., 2019). The response of plants to soil gradient is related to the cause and extent of trait variation (Funk, 2008; Siefert and Ritchie, 2016; Zhou et al., 2018). A wider range of intraspecific traits variation is thought to provide plants with greater access to limiting resources (Lin et al., 2020). da Silveira Pontes et al. (2010) found trait plasticity regulates plant responses to nitrogen enrichment in grasslands. Ishii et al. (2018) found plants with higher intra-individual plasticity had greater acclimation potential to environmental perturbation. Therefore, intraspecific traits variation should be considered when predicting the response of plant to soil fertility gradients.
In this study, we selected eight coexisting broadleaf species as target species, as they are dominant and commonly associated with the distribution of mixed broadleaved-Korean pine (Pinus koraiensis) forests in Northeast China (Wang, 1994). Eight leaf traits (including four leaf economic traits and four anatomical traits) and five soil fertility factors were measured. The variation in soil fertility factors in the study area provide an ideal setting for testing soil fertility-trait relationships. We sought to answer the following questions: (1) what is the relationship between economic and anatomical traits? We hypothesize that there should be coupling relationship between economic traits and vein traits. (2) Which group of functional traits are more strongly associated with soil fertility gradients? We hypothesize that economic traits would show a stronger correlation with soil fertility than vein traits. (3) Which soil fertility factor dominates leaf trait variation? We hypothesize that soil nutrient may dominate traits variation.
2. Materials and methods
2.1. Sample design
Our study sites are distributed in the mixed broadleaved-Korean pine forests in Northeast China (Wang, 1994; Supplementary Table 1). Within each site, we selected eight coexisting broadleaf species in mid-July to August (Supplementary Table 2). Leaf sample collection for each species took place in south facing slopes with similar slope degrees. Three trees with similar tree height (measured by tree altimeter) and diameter at breast height were selected. Information for the sampled trees is shown in Supplementary Table 2. We cut 6 branches beginning at the first living branch to the top of the tree: upper south and north, middle south and north, lower south and north. In each sampling unit, 5 healthy and fully expanded leaves were selected to measure SLA and LDMC, and five leaves were selected to be fixed in a buffered formalin-acetic acid-alcohol fixation solution (70% ethanol: formalin: glacial acetic acid = 90: 5: 5) to measure anatomical traits, e.g., AB, AD, PT, and spongy tissue (ST). Totally, we collected 180 leaves to measure SLA, LDMC and anatomical traits for each tree species. Additionally, 10–20 leaves were used to measure LN and leaf phosphorus content (LP) (Table 1). Soil samples were collected for each sample tree. We removed the leaf litter and then collected soil samples at 0–10 cm depth, with three replicates. The three subsamples were then mixed together, stored in vacuum plastic bags and transported to the lab.
2.2. Leaf trait measurements
For each sampled leaf, we used a micrometer to measure the non-main vein three times and then took the average value of the leaf thickness (precision: 0.01). We then measured fresh leaf mass (precision: 0.0001 g) and scanned leaf area (precision: 0.01 cm2; BenQ Corporation, Suzhou, China, 300 dpi resolution). After, we oven-dried the leaves to a constant weight (65°C, at least 72 h) and measured the mass (precision: 0.0001 g). SLA (cm2 g –1, SLA = leaf area/leaf dry mass) and LDMC (g g–1, LDMC = leaf dry mass/fresh mass) were then calculated. We used a pulverizer to grind all the leaves into powder and oven-dried them. We then measured LN [mg g–1, Hanon K9840 auto-Kjeldahl analyzer (Jinan Hanon Instruments Co. Ltd., Jinan, China)] and LP [mg g–1, molybdenum blue colorimetric method, AQ2 automatic discontinuous chemical analyzer (SEAL Analytical, Inc., Mequon, WI, USA)] after digested with H2SO4-H2O2.
To measure anatomical traits, we first progressively dehydrated the leaves with ethanol series (70, 85, 95, and 100%) and then infiltrated them with warm paraffin. We obtained the leaf sections (7 μm) using a rotary microtome (KD-2258, Zhejiang, China). We then stained (Safranin and Fast Green), mounted and sealed (neutral glue) the leaves to take photographs. We used a light microscope (Olympus Electronics, Inc., Tsukuba, Japan) to measure AB, AD, PT, and ST [by using electronic image analysis equipment (cellSens Standard 1.11 software, Olympus Electronics Inc., Tsukuba, Japan)]. The ratio of spongy tissue to leaf thickness (ST/LT) was calculated by dividing the ST by the LT.
2.3. Soil factors measurements
After measuring soil samples for SWC [g g–1, oven-drying method (Liu Z. L. et al., 2020)], we dried the soils and measured soil pH (HANNAPH2l1 pH meter), soil total carbon [mg g–1, multiN/C3000 (Analytic Jena AG, Jena, Germany)], soil total nitrogen [mg g–1, Hanon K9840 auto Kjeldahl analyzer (Jinan Hanon Instruments Co. Ltd., Jinan, China)] and soil total phosphorus (mg g–1, molybdenum blue colorimetric method).
2.4. Statistical analysis
We used principal component analysis (PCAs) to determine the multivariate associations of leaf traits (PCAs) in R 3.4.2 (R Core Team, 2017). Eight leaf traits of eight species from three sites were input in the PCA. The first principal axis values of economic and anatomical traits were used to analyze the correlation between the two groups of traits (Li et al., 2015). For eight leaf traits, we used “lme” function in the “nlme” package to fit a linear mixed model (nested levels: leaf, direction, canopy, tree, and site). The “varcomp” function in the “ape” package was used to calculate the variance components associated with the nested level. The correlation between economic and anatomical traits, the correlation between the two sets of traits, and the correlation of soil fertility factors were analyzed using Pearson correlation analysis. General linear model was used to estimate responses of the eight leaf traits to soil fertility factors. We calculated the variance inflation factor (VIF) between every two bivariate independent variables in the model to prevent collinearity from affecting model accuracy (Supplementary Table 3). VIF was all less than 10, indicating no issues with collinearity (Dormann et al., 2013).
3. Results
The first two axis described 55.9% of the total variance (Figure 1A and Supplementary Figure 1). The first PCA axis (PC1) explained 33.5% of the variance and showed strong loadings from SLA and LDMC. The second PCA axis (PC2) explained an additional 22.4% of the variance and showed strong loadings from AD, AB, and ST/LT (Figure 1A and Table 2). Covariation occurred between the PC1 scores of leaf economics traits and anatomical traits (p < 0.05). The covariation further confirmed anatomical traits could be assigned to economic traits (Figure 1B).
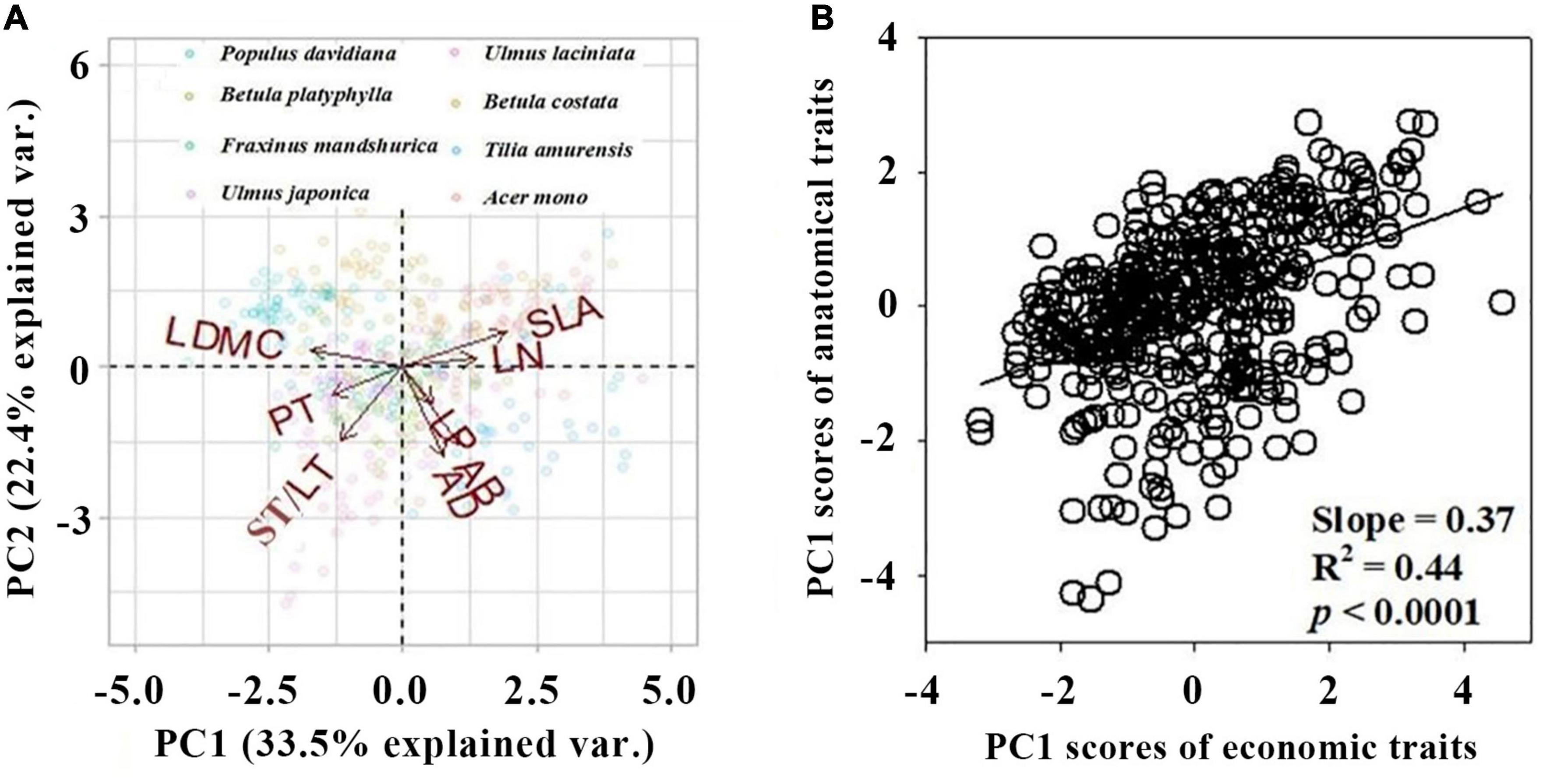
Figure 1. Principal component analysis (PCA) of eight leaf traits of eight species. (A) Biplot of the first two principal components loaded by trait variables. Arrows represent the principal component loadings associated with the leaf traits. The proportion of the total variation explained by the first two components is shown in parentheses next to the axis label. (B) The bivariate relationships between PC1 scores of economic trait and anatomical traits. All traits were log-transformed before analysis. Leaf trait abbreviations are provided in Table 1.
The variation range of economic traits (17–30%) and anatomical traits (18–29%) was similar but the causes of trait variation were different. Intraspecific factors (tree + canopy + direction) explained most of the variation in economic traits (34–65%); while interspecific factors (species + site) explained most for most of the variation in anatomical traits (55–71%) (Figure 2). For economic traits, among the three intraspecific factors, direction explained the most trait changes in SLA, LN, and LP, followed by canopy. For LDMC, canopy explained the most trait variation followed by direction. For anatomical traits, tree species explained the most trait variation (Figure 2). We observed a significant correlation between economic traits and anatomical traits except for SLA vs. AD (p < 0.05; Figure 3). SLA was negatively correlated with AD and positively correlated with ST/LT. LDMC was positively correlated with AD and AB and negatively correlated with ST/LT (Figure 3).
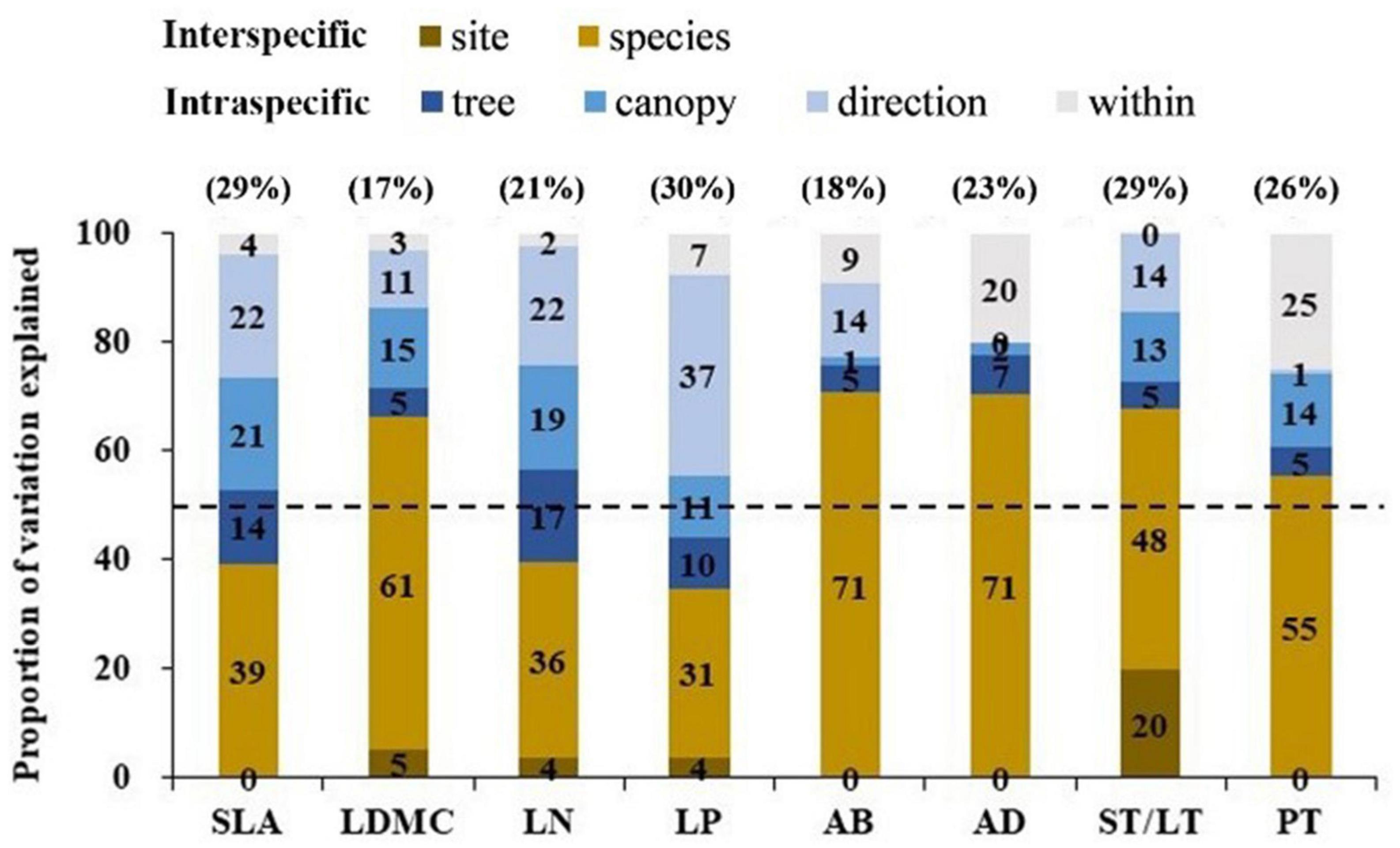
Figure 2. Variance partitioning of the full nested linear models on four economic traits and four anatomical traits across five nested ecological scales. The number in brackets above the graph is the overall variation coefficient (%) of this trait. The 50% thresholds are given by a dashed line. Leaf trait abbreviations are provided in Table 1.
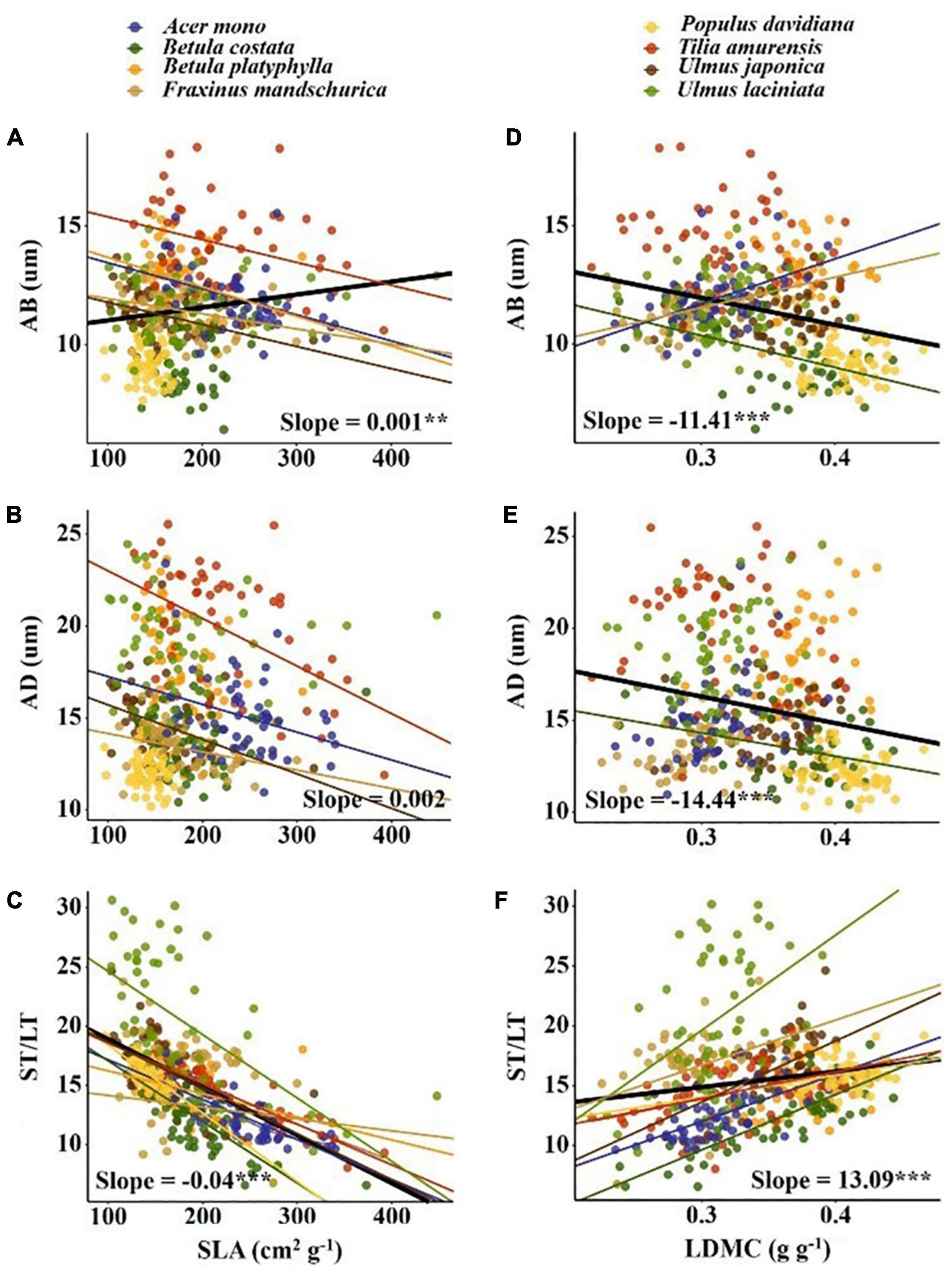
Figure 3. Pearson’s correlations between economic traits and anatomical traits. The solid line indicates that the correlation between leaf traits and soil fertility factor is significant at the 0.05 level. Slope and correlation significance in each figure were calculated at the interspecific level. (A–C) The relationship between SLA and AB, AD, ST/LT; (D–F) the relationship between LDMC and AB, AD, ST/LT.
Significant correlations occurred between most economic and anatomical traits and soil fertility factors (p < 0.05; Figures 4, 5). For economic traits, SLA was significantly correlated with five soil fertility factors. Other economic traits were not correlated with soil fertility factors, with the exception of LDMC vs. pH and STN, LN vs. pH and SWC, LP vs. STP and SWC (Figure 4). For anatomical traits, AB was significantly correlated with STN and pH, and AD was significantly correlated with STN and SWC, PT and ST/LT were significantly correlated with soil fertility factors except PT vs. pH and ST/LT vs. SWC (Figure 5).
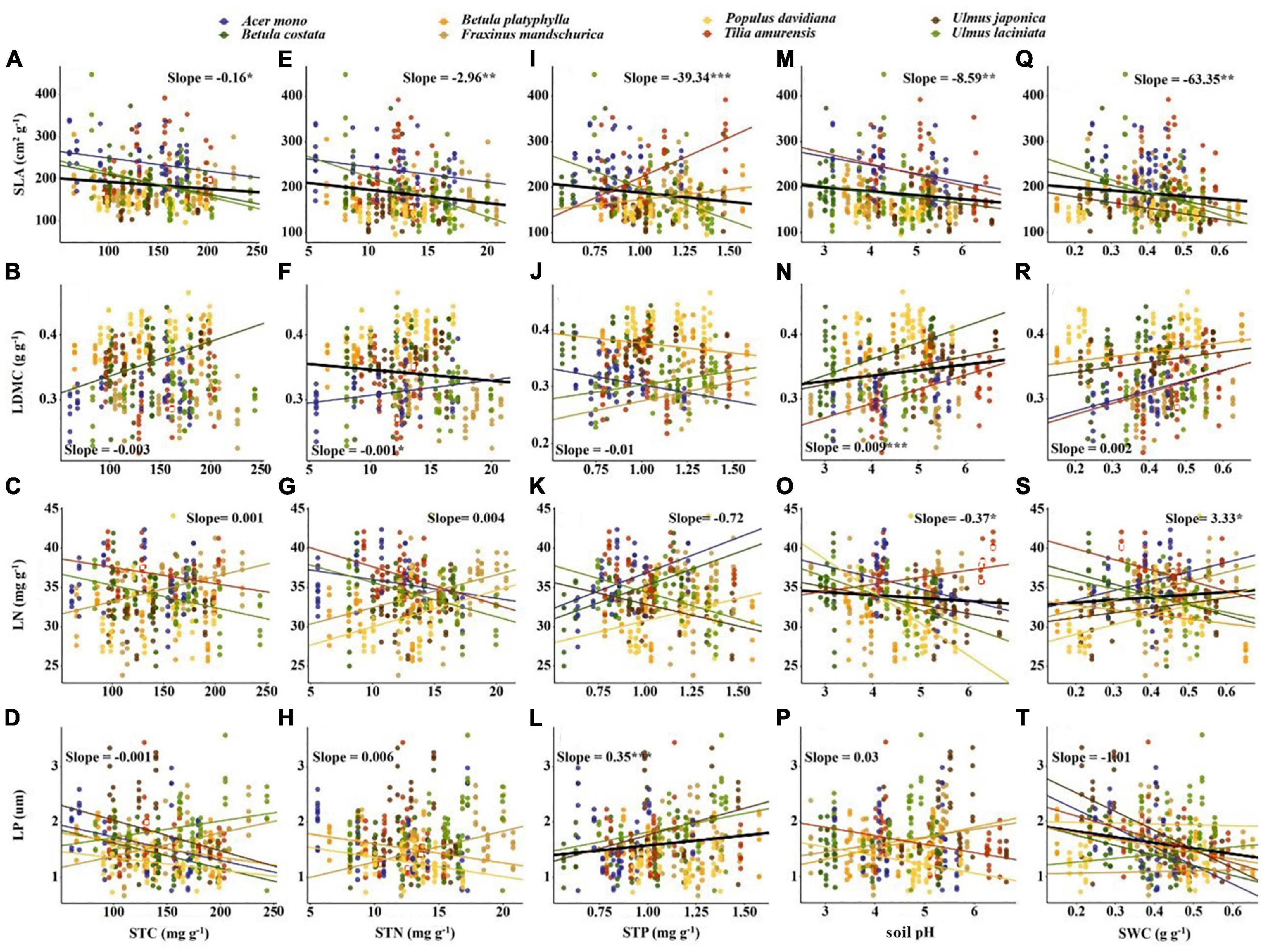
Figure 4. Correlation between soil fertility factors and economic traits. The solid line indicates that the correlation between leaf traits and soil fertility factor is significant at the 0.05 level. Slope and correlation significance in each figure were calculated at the interspecific level. (A–D) The relationship between STC and economic traits; (E–H) the relationship between STN and economic traits; (I–L) the relationship between STP and economic traits; (M–P) the relationship between soil pH and economic traits; (Q–T) the relationship between SWC and economic traits.
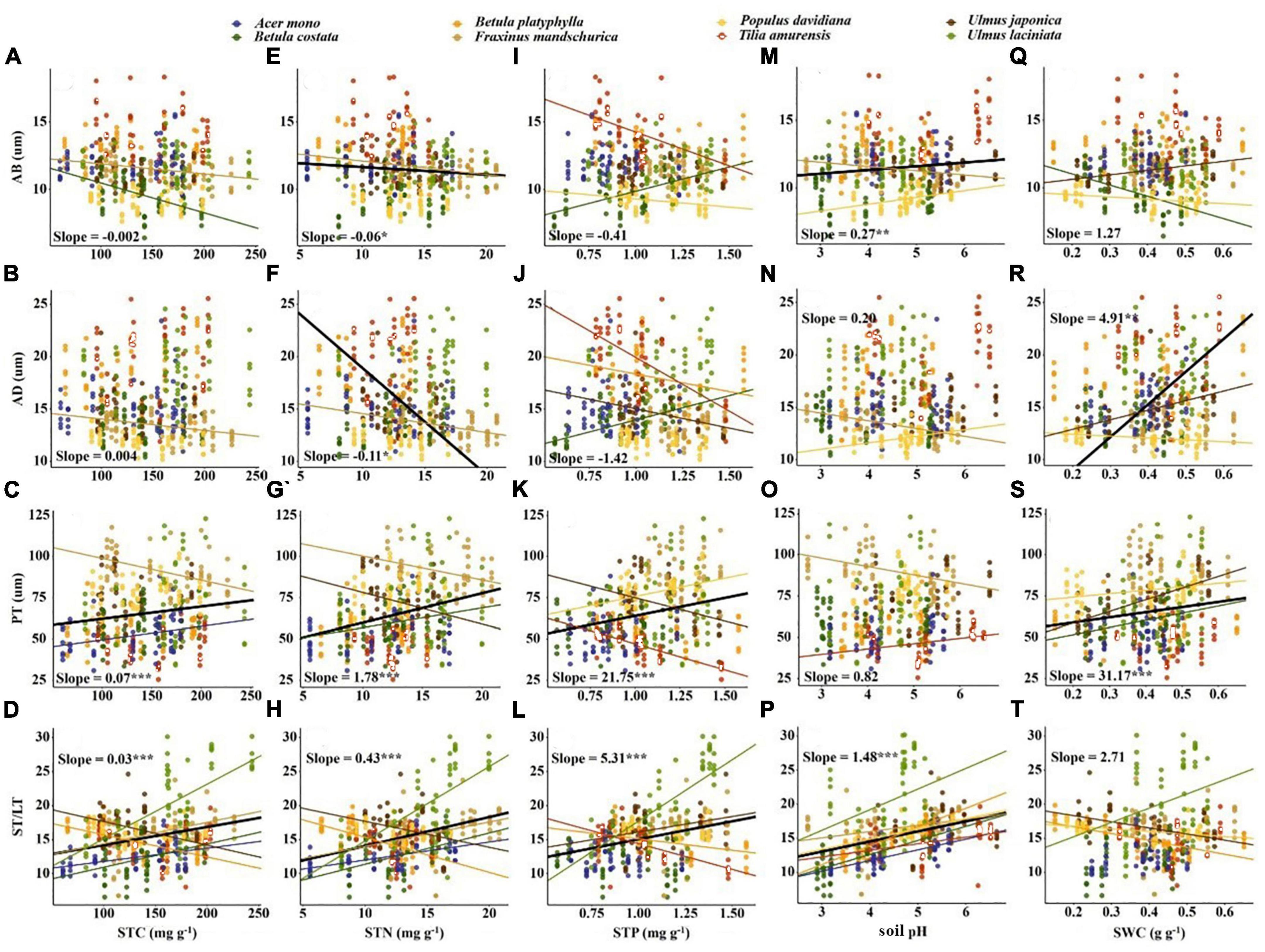
Figure 5. Correlation between soil fertility factors and anatomical traits. The solid line indicates that the correlation between leaf traits and soil fertility factor is significant at the 0.05 level. Slope and correlation significance in each figure were calculated at the interspecific level. (A–D) The relationship between STC and anatomical traits; (E–H) the relationship between STN and anatomical traits; (I–L) the relationship between STP and anatomical traits; (M–P) the relationship between soil pH and anatomical traits; (Q–T) the relationship between SWC and anatomical traits.
Soil fertility factors had stronger effects on anatomical traits compared to economic traits (Table 3). For economic traits, STN significantly affected SLA and LDMC. STP and SWC significantly affected SLA and LP. pH significantly affected SLA, LDMC, and LN (Table 3). For anatomical traits, STC significantly affected PT. STN affected all anatomical traits except AB. STP affected all anatomical traits except AD. pH significantly affected AB and PT, and SWC affected all anatomical traits (Table 3).
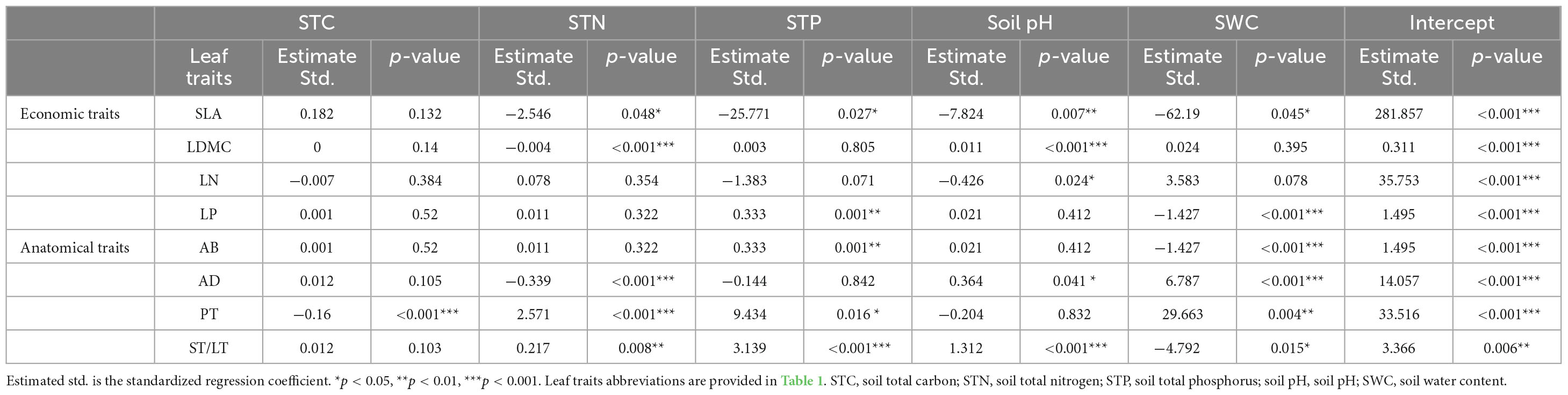
Table 3. The influence of five soil fertility factors on economic and anatomical traits by using a General linear model (GLM).
4. Discussion
4.1. Relationships between economic traits and anatomical traits
We found a strong interdependence between economic traits and anatomical traits in mixed broadleaved-Korean pine (P. koraiensis) forests (Figure 1). Our results support the first hypothesis. The coupled correlations are potentially a more cost-effective adaptation strategy for species to improve resource utilization efficiency (Yin et al., 2017; Liu C. C. et al., 2020). Our study is consistent with the findings of Liu C. C. et al. (2019). The leaf economic trait axis showed strong loadings from SLA and LDMC. This describes a shift from higher leaf dry-mass investment efficiency and lower moisture diffusion resistance to lower leaf dry-mass investment efficiency and higher moisture diffusion resistance (Wilson et al., 1999; Wright et al., 2004). Leaf anatomical trait axis showed strong loadings from AB, AD, and ST/LT. This describes the co-variation of leaf mechanical stability and gas exchange rate. Anatomical traits are often considered to be important indicators of photosynthesis (Terashima et al., 2011; Coble and Cavaleri, 2017). Leaf photosynthesis is constrained by economic traits because high photosynthetic rates need efficient water supply and thus higher dry-mass investment (for example, to build a vein structure) (Sack and Scoffoni, 2013). Therefore, coupling between leaf construction costs and leaf photosynthesis might drive the coordination of plant economic and anatomical traits.
The range of intraspecific variation of economic traits and anatomical traits was almost equal, but the causes of intraspecific variation were different (Figure 2). For economic traits (except LDMC), intraspecific factors explain most of the trait variation. Among intraspecific factors, direction explained the most trait variation (22–37%, Figure 2), indicating these traits were highly responsive to light variation (Coble et al., 2017). For anatomical traits, interspecific factors explain most of the trait variation. Among interspecific factors, tree species explained most of the variation (48–71%, Figure 2), suggesting anatomical traits were less influenced by microclimate factors and more influenced by leaf construction traits or large-scale environmental factor variation. The target tree species selected for our study have a wide range of shade tolerance. The significant effect of shade tolerance on anatomical traits supports the results of Zhang et al. (2019). In addition, mycorrhizal differences between tree species may also be the cause of trait variation, because different mycorrhizal tree species may adapt to different soil nutrients (e.g., arbuscular mycorrhizal species tend to be adapted to areas with fertile soil; while ectomycorrhizal species tend to be adapted to areas with infertile soil) (Phillips et al., 2013; Mao et al., 2019). Our results suggest when examining the effects of intraspecific variation on traits, the causes of intraspecific variation should be considered.
Significant correlations between economic and anatomical traits reflect trade-offs between plant leaf construction cost and photosynthetic capacity (Supplementary Figure 2). SLA was positively correlated with AB (Figure 3A). SLA reflects the light-capturing ability of the leaf (Wright et al., 2004) and AB reflects the ability to reduce the reflection of scattered light (Liu C. C. et al., 2019). Therefore, higher AB corresponds to a higher SLA and may signify the adaptation of plants to low light environment. SLA was negatively correlated with ST/LT (Figure 3C). This trade-off was also found at the intraspecific level in large-scale environments (Liu C. C. et al., 2019). For leaves, a higher ST/LT represents a higher proportion of ST and a higher ST is beneficial for leaves to better utilize scattered light (Terashima et al., 2011; Kröber et al., 2014). This may be the reason for the negative correlation between ST/LT and SLA. With the increase of LDMC, AB and AD decrease but ST/LT increases (Figures 3D–F). LDMC is an indicator of leaf moisture content. The increase in LDMC prompted a decrease in intercellular space and water content but increase in dry matter mass per volume (Wilson et al., 1999; Shipley and Vu, 2002). The thicker epidermis helps increase radiation intensity inside the leaf and reduce mechanical damage from herbivores and wind (Thomas and Winner, 2002). The negative correlation between LDMC and the epidermis may be a trade-off between nutrient accumulation and protection. Higher LDMC corresponds to higher ST/LT, which may be an adaptive strategy for plants to maintain minimum water content and maximize light absorption.
4.2. Economic and anatomical traits show specific responses to soil fertility
The results showed that the response of economic and anatomical traits to soil fertility was different, and the main driving factors of trait variation depended on the trait, which may be related to the potential tradeoff between leaf light capture and water conduction. Our results do not support the second and third hypotheses.
For economic traits, SLA demonstrated a stronger correlation with soil fertility factors than other economic traits (e.g., LDMC, Table 3). This supports the findings from Laughlin et al. (2010) and De Frenne et al. (2011), who suggested SLA can better predict soil fertility than other traits. The correlation between SLA and soil nutrients in our study (Figures 4A, E, I) were inconsistent with previous research results (Ordoñez et al., 2009; Maire et al., 2015). This may be attributed to the sampling site location in northeast China. The northeastern Chinese climate may affect the correlation between SLA and soil nutrients factors (Simpson et al., 2016). We also found a negative correlation between LDMC and STN, suggesting the preservation of more organic matter in leaves from infertile soil is of great significance for plant survival (Jager et al., 2015). In addition, past studies report soil nutrients usually correspond to leaf nutrient content, such as low LP was often found in low soil P environment (Ordoñez et al., 2009; Fan et al., 2015). The correlation between LP and STP in our study also fit into this framework (Figure 4L), however, no such relationship was found between other leaf nutrients and soil nutrient factors. The negative correlation between LP and SWC supports the results of Ordoñez et al. (2009) and Maire et al. (2015). The area with high soil moisture is also relatively high in soil P due to leaching, thus corresponding higher LP. We also found a positive correlation between LN and SWC (Wright et al., 2003), which may be because in arid areas, lower LN was beneficial to reduce photosynthetic water consumption and provide a conservative survival strategy for plants (Wright et al., 2005). Similar correlations were not found between SLA and SWC (Figure 4Q), which may be due to the large difference in shade tolerance of the selected tree species in our study. In addition, higher pH often implies higher nutrient utilization efficiency and usually corresponds to higher SLA, LN and lower LDMC. However, our results were contrary to this and may be due to the lower precipitation levels of our sampling area. Additionally, soil pH was more related to non-climatic factors, such as topography (Jenny, 1941), thus showing contrary results.
For anatomical traits, ST/LT and PT were positively correlated with STC, STN, and STP, while AB and AD were negatively correlated with STN. Soil nutrients, aboveground biomass, and canopy cover increase while the light density decreases (Hautier et al., 2009; Lin et al., 2020). Thicker PT and ST can contain more chloroplasts and have larger intercellular spaces (Mendes et al., 2001; Terashima et al., 2011; He et al., 2017). Thinner epidermis allows for facilitated CO2 diffusion within a leaf (Verboven et al., 2015), thereby optimizing photosynthesis to adapt to low light environment. Plants tend to choose the quick-return strategy in lower pH soils. A thicker AB helps to reduce reflected light (Liu C. C. et al., 2019) and a larger ST/LT can improve the gas transport efficiency, both of which support rapid resource acquisition by plants (Verboven et al., 2015). Lower AD and PT correspond to soil with lower water content (Figures 5R, S). Leaves building smaller cells to maintain turgor may be a survival strategy against drought (Li et al., 2011), such results are also found in herbs and shrubs (He et al., 2017). Furthermore, we emphasize the importance of PT and ST/LT in predicting soil fertility due to the strong correlation between these two anatomical traits and soil fertility factors (Figure 5). This result may be related to the close relationship between these two traits and SLA, as SLA can be explained by the inverse of leaf thickness and leaf density (Poorter et al., 2009). Intercellular space size determines leaf thickness, while PT thickness determines leaf density (Sack et al., 2013; Onoda et al., 2017). Therefore, PT and ST/LT show a stronger response to soil fertility than epidermal thickness.
5. Conclusion
Our results clearly show a coupling relationship between economic and anatomical traits. We found difference in the causes of trait variation. Relative to economic traits, SLA had a stronger correlation with soil fertility than other traits. Relative to anatomical traits, PT and ST/LT had a stronger correlation with soil fertility than other traits and the main soil fertility factors driving the variation was dependent on traits. Our results provide a new perspective for understanding the correlation between leaf traits and soil fertility factors.
Data availability statement
The raw data supporting the conclusions of this article will be made available by the authors, without undue reservation.
Author contributions
ZL developed the idea. ZL and MJ carried out the experiment. MJ analyzed the dataset. GJ contributed to interpretation of data. MJ wrote the manuscript with substantial contributions from ZL, GJ, and QG. All authors contributed to the article and approved the submitted version.
Funding
This work was supported by the National Key R&D Program of China (2022YFD2201100), the National Natural Science Foundation of China (31971636), and the Fundamental Research Funds for the Central Universities (2572022DS13).
Acknowledgments
We would like to thank Dr. Joseph Elliot at the University of Kansas for his assistance with English language and grammatical editing of the manuscript.
Conflict of interest
The authors declare that the research was conducted in the absence of any commercial or financial relationships that could be construed as a potential conflict of interest.
Publisher’s note
All claims expressed in this article are solely those of the authors and do not necessarily represent those of their affiliated organizations, or those of the publisher, the editors and the reviewers. Any product that may be evaluated in this article, or claim that may be made by its manufacturer, is not guaranteed or endorsed by the publisher.
Supplementary material
The Supplementary Material for this article can be found online at: https://www.frontiersin.org/articles/10.3389/ffgc.2023.1232333/full#supplementary-material
References
Chartzoulakis, K., Patskas, A., Kofidis, G., Bosabalidis, A. M., and Nastou, A. (2002). Water stress affects leaf anatomy, gas exchange, water relations and growth of two avocado cultivers. Sci. Hortic. 95, 39–50.
Chen, L., and Wang, R. Z. (2009). Anatomical and physiologica divergences and compensatory effects in two Leymus chinensis (Poaceae) ecotypes in Northeast China. Agr. Ecosyst. Environ. 134, 46–52.
Coble, A. P., and Cavaleri, M. A. (2017). Vertical leaf mass per area gradient of mature sugar maple reflects both height-driven increases in vascular tissue and light-driven increases in palisade layer thickness. Tree Physiol. 37, 1337–1351. doi: 10.1093/treephys/tpx016
Coble, A. P., Fogel, M. L., and Parker, G. G. (2017). Canopy gradients in leaf functional traits for species that differ in growth strategies and shade tolerance. Tree Physiol. 37, 1415–1425. doi: 10.1093/treephys/tpx048
da Silveira Pontes, L., Louault, F., Carrere, P., Maire, V., Andueza, D., and Soussana, J. F. (2010). The role of plant traits and their plasticity in the response of pasture grasses to nutrients and cutting frequency. Ann. Bot. 105, 957–965. doi: 10.1093/aob/mcq066
De Frenne, P., Graae, B. J., Kolb, A., Shevtsova, A., Baeten, L., Brunet, J., et al. (2011). An intraspecific application of the leaf-height-seed ecology strategy scheme to forest herbs along a latitudinal gradient. Ecography 34, 132–140.
Dormann, C. F., Elith, J., Bacher, S., Buchmann, C., Carl, G., Carré, G., et al. (2013). Collinearity: A review of methods to deal with it and a simulation study evaluating their performance. Ecography 36, 27–46. doi: 10.1111/j.1600-0587.2012.07348.x
Evans, J. R., Kaldenhoff, R., Genty, B., and Terashima, I. (2009). Resistances along the CO2 diffusion pathway inside leaves. J. Exp. Bot. 60, 2235–2248. doi: 10.1093/jxb/erp117
Fan, H., Wu, J., Liu, W., Yuan, Y., Hu, L., and Cai, Q. (2015). Linkages of plant and soil C:N:P stoichiometry and their relationships to forest growth in subtropical plantations. Plant Soil 392, 127–138. doi: 10.7717/peerj.9274
Funk, J. L. (2008). Differences in plasticity between invasive and native plants from a low resource environment. J. Ecol. 96, 1162–1173.
Hautier, Y., Pascal, A. N., and Andy, H. (2009). Competition for light causes plant biodiversity loss after eutrophication. Science 324, 636–638. doi: 10.1126/science.1169640
He, N. P., Liu, C. C., Tian, M., Li, M. L., Yang, H., Yu, G. R., et al. (2017). Variation in leaf anatomical traits from tropical to cold-temperate forests and linkage to ecosystem functions. Funct. Ecol. 32, 10–19.
Hodgson, J. G., Montserrat-Marti, G., Charles, M., Jones, G., Wilson, P., Shipley, B., et al. (2011). Is leaf dry matter content a better predictor of soil fertility than specific leaf area? Ann. Bot. 108, 1337–1345. doi: 10.1093/aob/mcr225
Ishii, H. R., Horikawa, S., Noguchi, Y., and Azuma, W. (2018). Variation of intra-crown leaf plasticity of Fagus crenata across its geographical range in Japan. For. Ecol. Manag. 429, 437–448.
Jager, M. M., Richardson, S. J., Bellingham, P. J., Clearwater, M. J., Laughlin, D. C., and De Deyn, G. (2015). Soil fertility induces coordinated responses of multiple independent functional traits. J. Ecol. 103, 374–385.
Jenny, H. (1941). Factors of soil formation: A system of quantitative pedology. Dover, NY: McGraw-Hill Book Company, Inc.
Kröber, W., Heklau, H., and Bruelheide, H. (2014). Leaf morphology of 40 evergreen and deciduous broadleaved subtropical tree species and relationships to functional ecophysiological traits. Plant Biol. 17, 373–383. doi: 10.1111/plb.12250
Laughlin, D. C., Leppert, J. J., Moore, M. M., and Sieg, C. H. (2010). A multi-trait test of the leaf-height-seed plant strategy scheme with 133 species from a pine forest flora. Funct. Ecol. 24, 493–501.
Li, F. L., Bao, W. K., and Wu, N. (2011). Morphological, anatomical and physiological responses of Campylotropis polyantha (Franch.) Schindl. seedlings to progressive water stress. Sci. Hortic. 127, 436–443.
Li, L., McCormack, M. L., Ma, C. G., Kong, D. L., Zhang, Q., Chen, X. Y., et al. (2015). Leaf economics and hydraulic traits are decoupled in five species-rich tropical-subtropical forests. Ecol. Lett. 18, 899–906. doi: 10.1111/ele.12466
Lin, G. G., Zeng, D. H., and Mao, R. (2020). Traits and their plasticity determine responses of plant performance and community functional property to nitrogen enrichment in a boreal peatland. Plant Soil 1, 151–167.
Liu, C. C., Li, Y., Xu, L., Chen, Z., and He, N. P. (2019). Variation in leaf morphological, stomatal, and anatomical traits and their relationships in temperate and subtropical forests. Sci. Rep. 9:5803. doi: 10.1038/s41598-019-42335-2
Liu, C. C., Li, Y., Zhang, J., Baird, A. S., and He, N. P. (2020). Optimal community assembly related to leaf economic- hydraulic-anatomical traits. Front. Plant Sci. 11:341. doi: 10.3389/fpls.2020.00341
Liu, C., Xiang, W. H., Zou, L. M., Lei, P. F., Zeng, Y. L., Ouyang, S., et al. (2019). Variation in the functional traits of fine roots is linked to phylogenetics in the common tree species of Chinese subtropical forests. Plant Soil 436, 347–364.
Liu, G., Freschet, G. T., Pan, X., Cornelissen, J. H., Li, Y., and Dong, M. (2010). Coordinated variation in leaf and root traits across multiple spatial scales in Chinese semi-arid and arid ecosystems. New Phytol. 188, 543–553. doi: 10.1111/j.1469-8137.2010.03388.x
Liu, Z. L., Hikosaka, K., Li, F. R., and Jin, G. Z. (2020). Variations in leaf economics spectrum traits for an evergreen coniferous species: Tree size dominates over environment factors. Funct. Ecol. 34, 458–467.
Maire, V., Wright, I. J., Prentice, I. C., Batjes, N. H., Bhaskar, R., van Bodegom, P. M., et al. (2015). Global effects of soil and climate on leaf photosynthetic traits and rates. Global Ecol. Biogeogr. 24, 706–717.
Mao, Z. K., Corrales, A., Zhu, K., Yuan, Z. Q., Lin, F., Ye, J., et al. (2019). Tree mycorrhizal associations mediate soil fertility effects on forest community structure in a temperate forest. New Phytol. 223, 475–486. doi: 10.1111/nph.15742
Mendes, M. M., Gazarini, L. C., and Rodrigues, M. L. (2001). Acclimation of Myrtus communis to contrasting Mediterranean light environments-effects on structure and chemical composition of foliage and plant water relations. Environ. Exp. Bot. 45, 165–178. doi: 10.1016/s0098-8472(01)00073-9
Ni, X. F., Sun, L. J., Cai, Q., Ma, S. H., Feng, Y. H., Sun, Y. F., et al. (2022). Variation and determinants of leaf anatomical traits from boreal to tropical forests in eastern China. Ecol. Indic. 140:108992.
Onoda, Y., Wright, I. J., Evans, J. R., Hikosaka, K., Kitajima, K., Niinemets, Ü, et al. (2017). Physiological and structural tradeoffs underlying the leaf economics spectrum. New Phytol. 214, 1447–1463.
Ordoñe, J. C., Bodegom, P. M., Witte, J. P. M., Bartholomeus, R. P., Hal, J. R. V., and Aerts, R. (2010). Plant strategies in relation to resource supply in mesic to wet environments: Does theory mirror nature? Am. Nat. 175, 225–239. doi: 10.1086/649582
Ordoñez, J. C., Bodegom, P. M., Witte, J. P. M., Wright, I. J., Reich, P. B., and Aerts, R. (2009). A global study of relationships between leaf traits, climate and soil measures of nutrient fertility. Global Ecol. Biogeogr. 18, 137–149. doi: 10.1002/ecy.4053
Peng, C. Z., Chang, L. L., Yang, Q., Tong, Z., Wang, D., Tan, Y. H., et al. (2018). Comparative physiological and proteomic analyses of the chloroplasts in halophyte Sesuvium portulacastrum under differential salt conditions. J. Plant Physiol. 232, 141–150. doi: 10.1016/j.jplph.2018.10.028
Phillips, R. P., Brzostek, E., and Midgley, M. G. (2013). The mycorrhizal-associated nutrient economy: A new framework for predicting carbon-nutrient couplings in temperate forests. New Phytol. 199, 41–51. doi: 10.1111/nph.12221
Poorter, H., Niinemets, Ü, Poorter, L., Wright, I. J., and Villar, R. (2009). Causes and consequences of variation in leaf mass per area (LMA): A meta-analysis. New Phytol. 182, 565–588. doi: 10.1111/j.1469-8137.2009.02830.x
Quesada, C. A., Lloyd, J., Schwarz, M., Patiño, S., Baker, T. R., Czimczik, C., et al. (2010). Variations in chemical and physical properties of Amazon forest soils in relation to their genesis. Biogeosciences 7, 1515–1541.
R Core Team (2017). R: A language and environment for statistical computing. Vienna: R Foundation for Statistical Computing.
Sack, L., and Scoffoni, C. (2013). Leaf venation: Structure, function, development, evolution, ecology and applications in the past, present and future. New Phytol. 198, 983–1000. doi: 10.1111/nph.12253
Sack, L., Scoffoni, C., John, G. P., Poorter, H., Mason, C. M., Mendez-Alonzo, R., et al. (2013). How do leaf veins influence the worldwide leaf economic spectrum? Review and synthesis. J. Exp. Bot. 64, 4053–4080. doi: 10.1093/jxb/ert316
Santos, K. R., Pereira, M. P., Ferreira, A. C. G., Rodrigues, L. C. D. A., Castro, E. M. D., Corrêa, F. F., et al. (2015). Typha domingensis Pers. growth responses to leaf anatomy and photosynthesis as influenced by phosphorus. Aquat. Bot. 122, 47–53.
Shipley, B., and Vu, T. T. (2002). Dry matter content as a measure of dry matter concentration in plants and their parts. New Phytol. 153, 359–364.
Siefert, A., and Ritchie, M. E. (2016). Intraspecific trait variation drives functional responses of old-field plant communities to nutrient enrichment. Oecologia 181, 245–255. doi: 10.1007/s00442-016-3563-z
Simpson, A. H., Richardson, S. J., and Laughlin, D. C. (2016). Soil-climate interactions explain variation in foliar, stem, root and reproductive traits across temperate forests. Glob. Ecol. Biogeogr. 25, 964–978.
Stark, J., Lehman, R., Crawford, L., Enquist, B. J., and Blonder, B. (2017). Does environmental heterogeneity drive functional trait variation? A test in montane and alpine meadows. Oikos 126, 1650–1659.
Terashima, I., Hanba, Y. T., Tholen, D., and Niinemets, Ü (2011). Leaf functional anatomy in relation to photosynthesis. Plant Physiol. 155, 108–116.
Thomas, S., and Winner, W. (2002). Photosynthetic differences between saplings and adult trees: An integration of field results by meta-analysis. Tree Physiol. 22, 117–127. doi: 10.1093/treephys/22.2-3.117
Uehara, G., and Gilman, G. (1981). The mineralogy, chemistry, and physics of tropical soils with variable charge clays. Boulder, CO: Westview Press.
Valladares, F., and Niinemets, Ü (2008). Shade tolerance, a key plant feature of complex nature and consequences. Annu. Rev. Ecol. Evol. S. 39, 237–257.
Verboven, P., Herremans, E., Helfen, L., Ho, Q. T., Abera, M., Baumbach, T., et al. (2015). Synchrotron X-ray computed laminography of the three-dimensional anatomy of tomato leaves. Plant J. 81, 169–182. doi: 10.1111/tpj.12701
Westoby, M. (1998). A leaf-height-seed (LHS) plant ecology strategy scheme. Plant Soil 199, 213–227.
Wilson, P. J., Thompson, K., and Hodgson, J. G. (1999). Specific leaf area and leaf dry matter content as alternative predictors of plant strategies. New Phytol. 143, 155–162. doi: 10.1002/ecy.3986
Wright, I. J., Ackerly, D. D., Frans, B., Harms, K. E., Guillermo, I. M., Miguel, M. R., et al. (2007). Relationships among ecologically important dimensions of plant trait variation in seven neotropical forests. Ann. Bot. 99, 1003–1015. doi: 10.1093/aob/mcl066
Wright, I. J., Reich, P. B., and Westoby, M. (2003). Least-cost input mixtures of water and nitrogen for photosynthesis. Am. Nat. 161, 98–111. doi: 10.1086/344920
Wright, I. J., Reich, P. B., Cornelissen, J. H., Falster, D. S., Garnier, E., Hikosaka, K., et al. (2005). Assessing the generality of global leaf trait relationships. New Phytol. 166, 485–496.
Wright, I. J., Reich, P. B., Westoby, M., Ackerly, D. D., Baruch, Z., Bongers, F., et al. (2004). The worldwide leaf economics spectrum. Nature 428, 821–827.
Yang, D. X., Song, L., and Jin, G. Z. (2019). The soil C:N:P stoichiometry is more sensitive than the leaf C:N:P stoichiometry to nitrogen addition: A four-year nitrogen addition experiment in a Pinus koraiensis plantation. Plant Soil 442, 183–198.
Yang, Y. Z., Wang, H., Harrison, S. P., Prentice, I. C., Wright, I. J., Peng, C., et al. (2019). Quantifying leaf trait covariation and its controls across climates and biomes. New Phytol. 221, 155–168. doi: 10.1111/nph.15422
Yin, Q., Wang, L., Lei, M. L., Dang, H., Quan, J. X., Tian, T. T., et al. (2017). The relationships between leaf economics and hydraulic traits of woody plants depend on water availability. Sci. Total Environ. 621, 245–252. doi: 10.1016/j.scitotenv.2017.11.171
Zhang, X. S., Jin, G. Z., and Liu, Z. L. (2019). Contribution of leaf anatomical traits to leaf mass per area among canopy layers for five coexisting broadleaf species across shade tolerances at a regional scale. Forest Ecol. Manag. 452:117569.
Zheng, Z., and Ma, P. F. (2018). Changes in above and belowground traits of a rhizome clonal plant explain its predominance under nitrogen addition. Plant Soil 432, 415–424.
Keywords: leaf trait multidimensionality, economic traits, anatomical traits, soil fertility factors, plant adaptive strategy
Citation: Jin MY, Jin GZ, Guo QX and Liu ZL (2023) Responses of economic and anatomical leaf traits to soil fertility factors in eight coexisting broadleaf species in temperate forests. Front. For. Glob. Change 6:1232333. doi: 10.3389/ffgc.2023.1232333
Received: 31 May 2023; Accepted: 04 July 2023;
Published: 18 July 2023.
Edited by:
Ling Zhang, Jiangxi Agricultural University, ChinaReviewed by:
Shuai Ouyang, Central South University of Forestry and Technology, ChinaQing-Wei Wang, Chinese Academy of Sciences (CAS), China
Copyright © 2023 Jin, Jin, Guo and Liu. This is an open-access article distributed under the terms of the Creative Commons Attribution License (CC BY). The use, distribution or reproduction in other forums is permitted, provided the original author(s) and the copyright owner(s) are credited and that the original publication in this journal is cited, in accordance with accepted academic practice. No use, distribution or reproduction is permitted which does not comply with these terms.
*Correspondence: Zhili Liu, bGl1emwyMDkzQDEyNi5jb20=