- 1Faculty of Environment, Science, and Economy, University of Exeter, Exeter, United Kingdom
- 2Helmholtz GFZ German Research Centre for Geoscience, Section 1.4 Remote Sensing and Geoinformatics, Potsdam, Germany
- 3School of Geographical Sciences, University of Bristol, Bristol, United Kingdom
- 4Instituto de Pesquisas Ambientais da Amazônia, Brasília, Brazil
- 5Programa de Pós-graduação em Biodiversidade e Conservação, Universidade Federal do Maranhão - UFMA, São Luís, Brazil
- 6Department of Geography, School of Environment Education and Development (SEED), University of Manchester, Manchester, United Kingdom
- 7Earth Observation and Geoinformatics Division, National Institute for Space Research (INPE), São José dos Campos, Brazil
1. Introduction
The use of Earth Observation data to monitor forests in space and time is crucial to our knowledge of forest dynamics at local, regional and global scales. Understanding carbon dynamics in forests is critical given the globally important carbon sink of tropical forests, which is declining due to climate change, deforestation and forest degradation (Hubau et al., 2020; Gatti et al., 2021; Fawcett et al., 2022). However, post-disturbance forest regrowth is also crucial in determining the extent of the carbon imbalance due to the magnitude of opposing processes of disturbance (carbon loss) and regrowth (carbon gain) (Fawcett et al., 2022). Perhaps nowhere is this more crucial to understand than in the Amazon, the largest tropical forested area on earth, storing approximately a quarter of the global aboveground biomass carbon (AGC) stocks (Fawcett et al., 2022). However, deforestation and degradation in the Amazon has been rising again in recent years, and the southeast of the Brazilian Amazon is now acting as a net carbon source (Gatti et al., 2021).
Secondary forests, forests naturally regrowing on areas of previously deforested, now abandoned lands, provide one way to help counterbalance this declining sink. Globally, passive and active forest restoration accounts for about 13% of the cost-effective land-based climate mitigation potential (Roe et al., 2021). Approximately half of secondary forests in Brazil are found in the Amazon, accounting for <2% of the country's area (~160,000 km2) (Almeida et al., 2016; TerraClass, 2023) but have a considerable climate mitigation potential within Brazil's Nationally Determined Contribution (up to 5% contribution by 2030) (Heinrich et al., 2021).
In this paper, we extend and combine the work of two previously published studies identifying secondary forest age (Silva et al., 2020) and their associated aboveground carbon recovery rates (Heinrich et al., 2021), publishing the full and updated temporal record of these datasets in a user-friendly toolkit called “RE:Growth” (https://ee-regrowth.projects.earthengine.app/view/regrowth). Based in Google Earth Engine (GEE), the RE:Growth toolkit can require little to no programming language knowledge and can be applied from local scale to the whole Brazilian Amazon biome. The aim of this toolkit is for it to be applicable to a range of users, importantly forest restoration project developers, as well as researchers, policymakers and other stakeholders. The toolkit can provide evidence-based information on secondary forests within a region of interest in the Brazilian Amazon, such as jurisdictional boundaries, which are often the most relevant for stakeholders and practitioners. For example, the toolkit can be used for providing spatial and quantitative data to inform spatial prioritization of secondary forest conservation and expansion.
2. Methods
2.1. Google Earth Engine as a user-friendly platform
Many Earth Observation-based datasets and analyses, stemming from peer-reviewed papers, are uploaded to open-access repositories such as, “CEDA,” “Zenodo,” and “FigShare.” While these repositories provide a means to store terabytes of data, the true “access” to the data is limited by the users' ability to download, view, and analyze the data. Analysis may require knowledge of programming languages, Geographic Information Systems (GIS) and general data manipulation. Larger, regional to global scale spatial forest data may be available on open data platforms such as “Global Forest Watch”; however, such platforms cannot include all forest-related datasets due to the sheer number that exist and the ability to analyze specific areas of interest is limited and can be slow to process.
More recently, cloud-based platforms for Earth Observation image processing and analysis, such as Google Earth Engine (GEE), are becoming increasingly common. GEE was developed to make high-performance computing accessible, to process large geospatial datasets, without the user relying on having large storage and offline processing power (Gorelick et al., 2017). However, processing and analyzing datasets in GEE still requires knowledge of either the programming language JavaScript or Python and requires users to create a GEE account, which relies on having a “Google mail” email address. In addition to the traditional programming-style interface, GEE functions exist to create interfaces that are more accessible to a wide audience. The user-interface can be published in the form of an “App” such that a user can visualize datasets and, in some instances, download the data without requiring a GEE account. The Re:Growth App introduced here takes this approach, providing easy, full open data access.
2.2. “RE:Growth” toolkit development
There have been both local and international efforts to protect and restore forests in the Amazon, with varying success. At international scales, the REDD+ framework is well-known, aimed at providing financial support to developing countries for “Reducing emissions from deforestation, degradation, and enhancing removals through conservation and sustainable management” (REDD+). Measuring, reporting, and verifying (MRV) the carbon removal (the “plus” in REDD+) component has been less well developed, partly because it is more challenging to track forest recovery and associated carbon fluxes.
The “RE:Growth” toolkit aims to address this information gap by bringing together two complementary, previously published studies (Silva et al., 2020; Heinrich et al., 2021). The first dataset identifies the extent and ages of secondary forests across Brazil using a Land-Use Land-Cover (LULC) dataset, MapBiomas from 1987 to 2018 (Silva et al., 2020). MapBiomas is based on Landsat data, offering annual information on LULC from 1985 and is updated annually with a new collection. MapBiomas Collection 8, the dataset used in this study, is available up to 2022 (Souza et al., 2020; MapBiomas Brasil., 2023a). The algorithm identifying the extent and ages of secondary forest has been updated from Silva et al. (2020) to improve processing time, replicability for future MapBiomas Collections and includes more recent years in the analysis. The algorithm tracks the temporal transition of a pixel classification from non-forested (anthropic) land to forested land in the MapBiomas dataset. Based on the consecutive number of years that a given pixel remains a forest, the age of that forest is estimated. If a conversion from secondary forest to non-forest occurs again, this is considered as “repeat deforestation.”
The second dataset used in the toolkit was a collection of 16 models that estimate the aboveground carbon accumulation with secondary forest age (Heinrich et al., 2021). Each model is unique according to climate regions in which the secondary forest grows and disturbances (fire and/or repeat deforestation) the area experiences (Heinrich et al., 2021). The four climate regions created by Heinrich et al. (2021) are based on similarities in shortwave radiation, annual precipitation, and water deficit based on the Maximum Cumulative Water Deficit index. Climate variables influence the rate of carbon accumulation in forests. The analysis overlayed the secondary forest extent and age dataset (Silva et al., 2020) with a spatial dataset of aboveground biomass for the year 2017 from the European Space Agency Climate Change Initiative (ESA-CCI). Using a space-for-time substitution approach, the aboveground carbon for a given secondary forest with a certain age in 2017 was estimated and aggregated within the four climate regions and according to disturbance history. A non-linear modeling approach was then used to model the aboveground carbon accumulation with secondary forest age. The final result was four models of aboveground carbon accumulation, each applicable to one of the four climate regions, and an additional three models for each climate region that consider if and when secondary forest experience burning, repeat deforestation or both disturbance types.
3. Functionalities of the “RE:Growth” toolkit
3.1. Selecting a region and year of interest (Steps 0 and 1)
Available in English and Portuguese, the toolkit has several key functionalities, enabling it to be used and adapted to user preferences (Figure 1). The user can select a region of interest (Step 0): the Brazilian Amazon biome, governmental units (states and municipalities), or they can draw their own region of interest (Steps 0.1 to 0.3). The subsequent information on the secondary forest analysis will then be clipped to the specified region (Figure 1). The user is then asked to select a year of interest (Step 1) to display the secondary forest ages within the specified region (Figure 1).
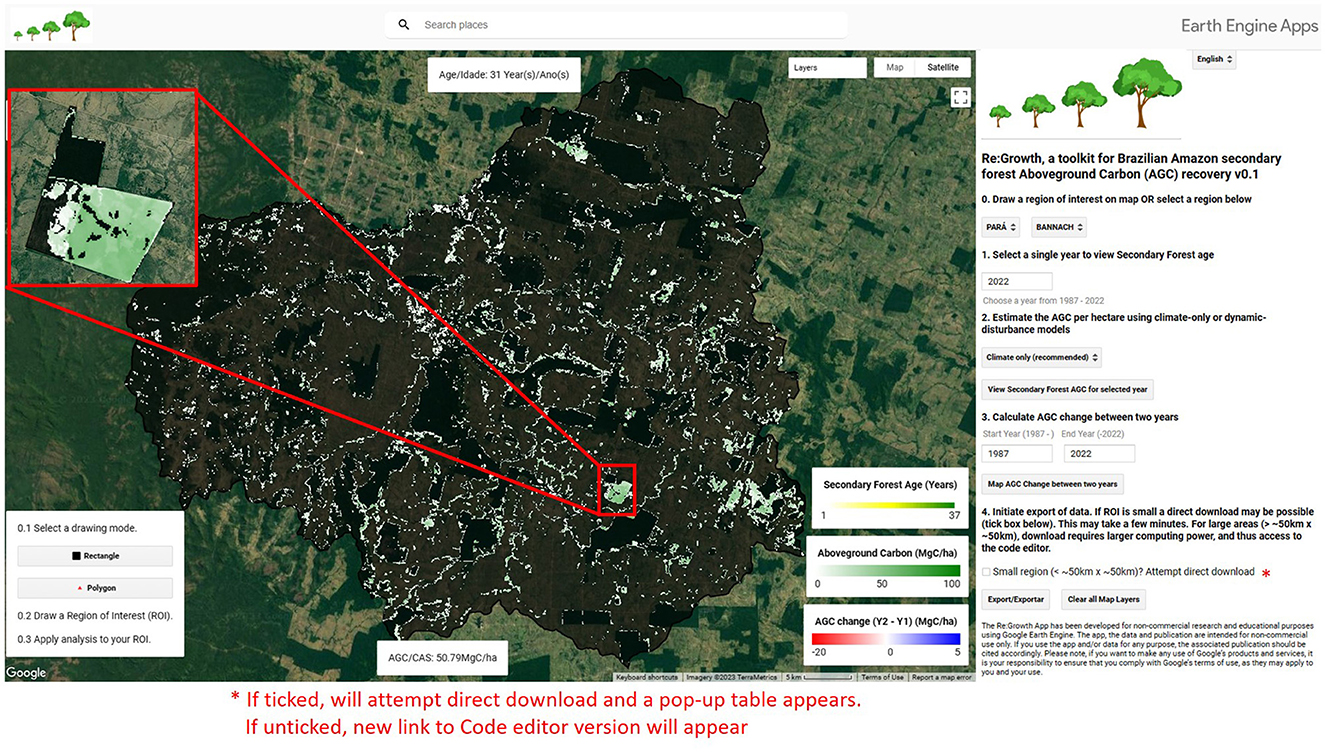
Figure 1. Screenshot example of the RE:Growth toolkit and its functionalities. The example region is the municipality of Bannach, Pará State in Eastern Amazon. Red inset box shows a zoomed in region of interest that was drawn using the drawing functionality available in the RE:Growth toolkit, seen in the bottom right of the image. Base map satellite image from Google.
3.2. Aboveground carbon accumulation and interannual changes (Steps 2 and 3)
In Step 2, the user needs to select which regrowth models to use from Heinrich et al. (2021). There are two options: (i) “Climate only” and (ii) “With disturbance.” The “Climate only” option applies the aboveground carbon regrowth models according to the four climate regions in which the secondary forests are regrowing. The second set of models (ii) take into account disturbance history by considering the number of times a pixel of secondary forest experienced deforestation and burning, based on the MODIS64 burnt area product available from 2001 to near present-day (Giglio et al., 2018). The “With Disturbance” models result in complex temporal regrowth rates due to the timing of the disturbance event and post-disturbance forest dynamics, and incorporate post-disturbance reductions in aboveground carbon and subsequent carbon accumulation with recovery. Based on the confidence interval for each regrowth model as presented in Heinrich et al. (2021), we estimate that the average confidence intervals for the “Climate only” and “With disturbance” regrowth models are ±18% and ±23%, respectively. These uncertainty estimates should be included when presenting estimates of aboveground carbon recovery for the user's region of interest.
The “With disturbance” regrowth models may more accurately estimate aboveground carbon fluxes, but with a greater uncertainty. It is recommended to apply the regrowth models “With disturbance” when there is explicit knowledge of the secondary forest plot disturbance history. Applying the “Climate only” regrowth models determines the maximum potential carbon accumulation in the absence of burning and deforestation, which secondary forest conservation should seek to achieve. As such, we recommend the user applies the “Climate only” regrowth models, especially when conducting analysis on the potential carbon stock of an area of secondary forest. Both the age and associated aboveground carbon for a given pixel can be viewed on the interactive map when that pixel is clicked.
Once the regrowth model has been selected, the user can determine the gains and losses in aboveground carbon between two specified time periods and add this calculation at the pixel level to view (Step 3). Carbon gains are due to ongoing regrowth in standing secondary forests and new recruits. Carbon losses are either due to the deforestation of secondary forests (“only” models) or due to a combination of deforestation and fire disturbance leading to a reduction but not complete removal of forest carbon (“With disturbance” models).
3.3. Downloading and exporting the data (Step 4)
Finally, the user can export the data for their given region of interest (Step 4). Step 4 is currently limited by GEEs restrictions on exporting and downloading data. Direct data downloading is limited to small regions. Specifically, downloading spatial data is limited to <32MB in size. Given these constraints to the GEE processing, there are two possible routes to exporting data using the published app: (i) if the region of interest is smaller than ~50 km2, the data can be directly processed, and a pop-up table will appear enabling the user to download the data. This is useful for project-scale developments of secondary forest plots and may even be possible at the municipality scale, depending on its size; (ii) if the region is larger than ~50 km2, the user is encouraged to not tick the box specifying the “small region” domain to avoid the toolkit from crashing. Instead, upon initiating the export, a new link will appear that gives access to the original GEE toolkit before publishing the App. This version of the toolkit includes the GEE “Code Editor,” containing all the code that was used to produce the toolkit, as well as the console panel that displays pixel-based information (“Inspector” tab) and is where the exporting of large data needs to be initiated (“Run” tab). The data can then be downloaded from the user's “GoogleDrive.” Tutorial videos giving examples of how to view, analyze, and download the dataset using both approaches are available (see “Data availability”).
Various, spatial, and aggregated data are available for download depending on the interface in which the export is initiated, i.e., using the toolkit App or Code Editor (Figure 2). In both cases, it is possible to download the spatial data of secondary forest age and aboveground carbon for the selected year of interest clipped to the specified region for analysis. The spatial forest aboveground carbon data can be downloaded as a “geoTiff” file for the selected year to analyze in any GIS software. The units were converted to KgC/pixel from MgC/ha by multiplying by the area of each pixel (~30 m pixel size) and then by 1000. This scaling was carried out so that the pixel data could be stored as integers to reduce the file size. When using the “App” version of the toolkit, the aboveground carbon for all secondary forests in the selected year and specified region are summed. The total aboveground carbon is available to download as a .csv file, with units of MgC (Figure 2B). Similarly, when using the “Code Editor” version, the aboveground carbon is also summed but data will be available for all years in the time-series (Figure 2A). Units are also in MgC.
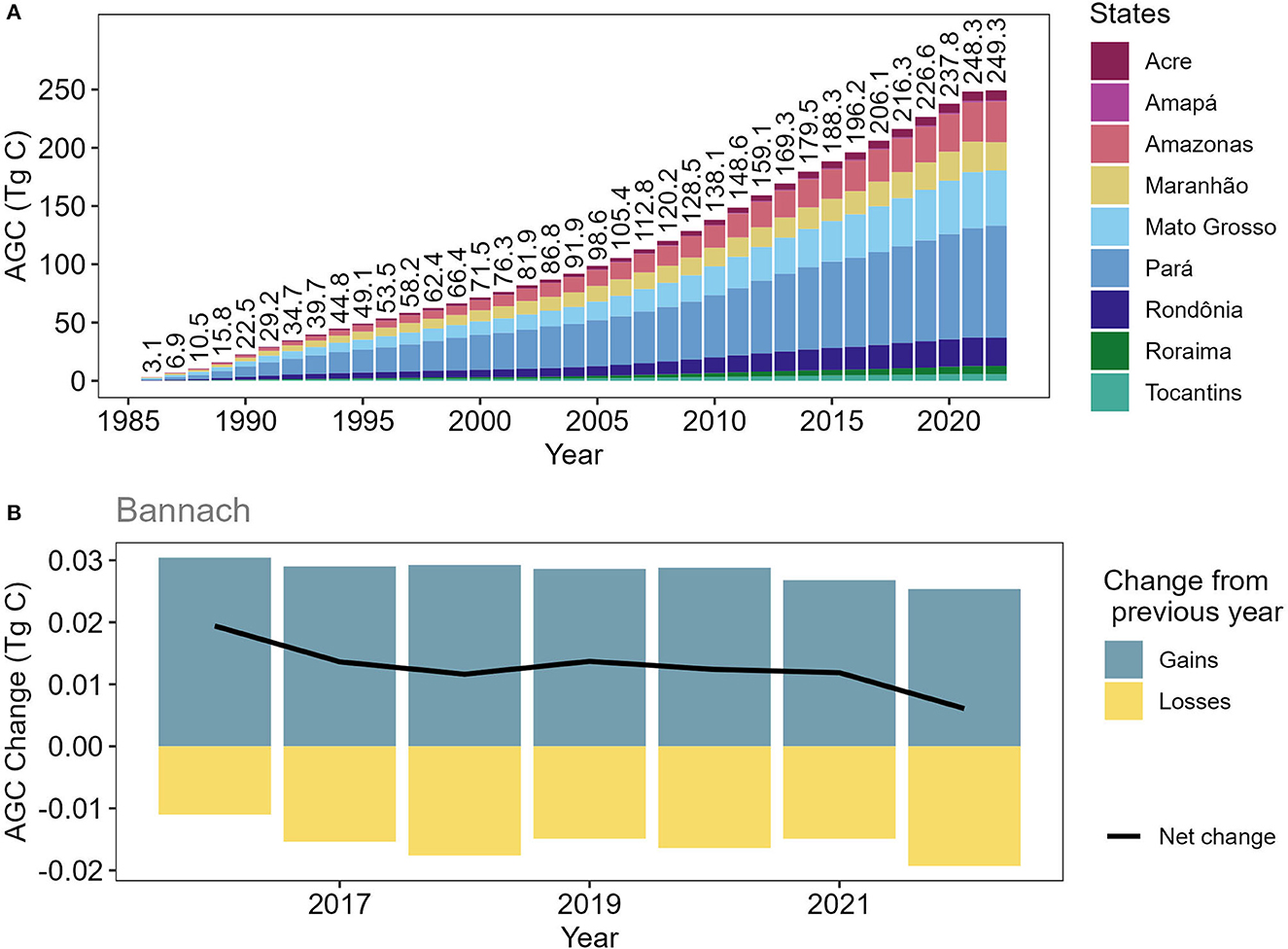
Figure 2. Example Aboveground Carbon (AGC) outputs from the RE:Growth toolkit. (A) Annual secondary forest AGC stock across the entire Amazon biome of Brazil split up according to the states within the biome for the entire period of analysis available (1986 to 2021). (B) AGC gains (from secondary forest regrowth) and losses (from secondary forest deforestation and disturbance) from previous year for the municipality of Bannach (seen in Figure 1) and interannual Net Change. In both (A, B) the “Climate only” regrowth models are applied.
4. Current limitations and future improvements
There are both practical and scientific limitations to the current approach and toolkit. A practical limitation of the current toolkit is that the direct download is only available for relatively small regions. As a result of this, a requirement when interested in larger regions, the user is required to have a Google mail and GEE account. Furthermore, viewing the toolkit and downloading the data requires a relatively stable and reliable internet connection to avoid time out issues, which is not always available for all users. Both these practical issues may limit the applicability of the toolkit for some users.
Scientifically, this toolkit relies primarily on two datasets (MapBiomas, and ESA-CCI AGB) and one modeling approach. Both the datasets have their assumptions, uncertainties, and drawbacks, as explored in the two studies this toolkit builds upon (Silva et al., 2020; Heinrich et al., 2021). For example, the proportion of secondary forest identified using the MapBiomas product explains about 63% of the secondary forest proportion found by TerraClass, a product from Brazil's National Institute for Space Research (INPE) (Silva et al., 2020). The ESA-CCI AGB product has been found to over-estimate in low biomass regions and under-estimate in high biomass regions (Araza et al., 2022). Additionally, there may be differences in land-use-land cover change between a given period when using different MapBiomas collections, leading to issues of consistency and comparability between the collections. For example, the total aboveground carbon stored in 2017 estimated using the MapBiomas collection 8 was 206.1 TgC (Figure 2A). This is 27% lower than was estimated using MapBiomas collection 3 by Heinrich et al. (2021) (281.8 TgC). It is always advisable to use the latest collection of MapBiomas and apply it consistently throughout the time period (MapBiomas Brasil., 2023a). The Re:Growth App is intended to be a “living model,” updated regularly with the latest, state-of-the-art input datasets to take such changes into account.
The MapBiomas Collections can misclassify areas of natural regrowing vegetation with other plantations, including tree crops such as oil palm (Nunes et al., 2020; Heinrich et al., 2021). While we have used other datasets to exclude regions of plantations (Harris et al., 2019; Descals et al., 2021) some, especially newer, plantations may still be identified as secondary forests. It is also important to consider the spatial scale of the toolkit, both the MapBiomas and ESA-CCI datasets are based on data with a moderate resolution of 30 m (MapBiomas) and 100 m (ESA-CCI AGB) spatial scale, making the toolkit's applicability to small-scale restoration projects potentially challenging. Furthermore, the toolkit currently only accounts for the largest carbon pool in the Brazilian Amazon, aboveground carbon (Berenguer et al., 2014). The toolkit has been designed as a framework that can be developed further in the future for a complete representation of the carbon fluxes that arise due to secondary forest loss and regrowth. A key consideration to make when designating areas for reforestation is the potential “leakage” of deforestation into other areas that may arise if reforestation or restoration projects are not sustainably implemented. This App currently does not currently have the functionalities to take leakage into account, however, it is an essential component to consider and potentially apply in future model developments.
We would like to acknowledge that five academic scientists designed the toolkit. There is potential to gain feedback and improve the toolkit functionalities with specific users in mind in future updates. Feedback can be made by emailing the corresponding author. Adaptations to the toolkit will be considered when updates are made in the future with newer Collections of MapBiomas. Such adaptations and improvements are likely to include: (i) expanding regions of interest available, such as selecting areas of conservation, protection or indigenous lands, or the option for the user to upload their own shapefile with a region of interest; (ii) including additional carbon pools, such as soil organic carbon (MapBiomas Brasil., 2023b); (iii) option to view future aboveground carbon gain potential if forests are allowed to regrow; (iv) the integration of other models estimating aboveground carbon accumulation that encompass other important environmental parameters (Cook-Patton et al., 2020; Chen et al., 2023); and (v) expanding the toolkit beyond the Brazilian Amazon as models of a similar nature become available for different ecoregions. Using GEE as the interface for the toolkit is advantageous as such future improvements can be readily incorporated, including annually updating it once new collections of MapBiomas become available.
5. Conclusion
In the United Nations decade of ecosystem restoration, there is increasing demand and potential to monitor the state and (carbon) fate of the world's forests. Applicable to the Brazilian Amazon, RE:Growth offers the first toolkit to analyze the aboveground carbon dynamics in secondary forests specifically. Allowing users to either draw their region of interest, select a municipality, state or indeed the whole region, this toolkit provides summary information and spatial data that the user can download and provide evidence-based information on the aboveground carbon accumulation in secondary forests. Such information can determine (measure) where secondary forests are growing or to verify other approaches to identify secondary forests, such as field data, upholding the importance of transparent, accurate, and credible MRV in REDD+ or similar projects. Given GEEs ability to process petabytes of data, and the existing and expanding availabilities of datasets on the GEE platform, an updated toolkit may be developed across the entire Amazon region and indeed, elsewhere where such datasets are available. Such large-scale, Earth Observation-based analysis has important implications for addressing the role of natural ecosystems to mitigating the climate emergency.
Author's note
Tutorial videos on how to use the Re:growth app are available here: https://www.youtube.com/watch?v=y7KhFwUYJyE&ab_channel=ThaisRosan (small-scale download), and here: https://www.youtube.com/watch?v=lFQbKw8bRSk&ab_channel=ThaisRosan (Code Editor-download option). The Re:Growth App has been developed for non-commercial research and educational purposes using Google Earth Engine. The app, the data and publication are intended for non-commercial use only. If you use the app and/or data for any purpose, the associated publication should be cited accordingly. Please note, if you want to make any use of Google's products and services, it is your responsibility to ensure that you comply with Google's terms of use, as they may apply to you and your use.
Data availability statement
The RE:Growth toolkit can be found at: https://ee-regrowth.projects.earthengine.app/view/regrowth, https://viola.users.earthengine.app/view/regrowth. The secondary forest age data is also available as a Google Earth Engine Asset (projects/ee-regrowth/assets/sforestAge_brazil_V8) for Brazil from 1986 to 2022. The GEE assets for associated aboveground carbon stocks are available for the Brazilian Amazon for each year available in the current version of the toolkit (1987 to 2022): (projects/ee-regrowth/assets/sforestAGC_climate_only_v1_1; projects/ee-regrowth/assets/sforestAGC_with_disturbance_v1_1).
Author contributions
VH, SS, and LA designed the concept of the toolkit and dataset. TR provided creative input on the functionalities of the toolkit. CS-J and VH were the original lead authors and developers of the code and dataset identifying secondary age and their regrowth rates. VH wrote the manuscript with input from all the authors and designed the RE:Growth toolkit with input from TR. All authors contributed to the article and approved the submitted version.
Funding
This research has been supported by the RECCAP2 project which is part of the ESA Climate Change Initiative (contract no. 22 4000123002/18/I-NB), and the H2020 European Institute of Innovation and Technology (4C; grant no. 821003). VH was in part supported by the CGIAR MITIGATE+ project, the WRI Land and Carbon Lab and the Open Earth Monitor Project funded by the European Union (grant agreement no.101059548). The University of Manchester funded CS-J through the Forest fragmentation mapping of Amazon and its vulnerable margin Amazon-Cerrado transition forests project. LA thanks partial support from CNPq (314416/2020-0), FAPESP (20/15230-5) and the Brazilian Space Agency (AEB).
Acknowledgments
We are grateful to the developers of the datasets used in this study and the original studies for providing free-open access to their data. Additional thanks to D. Fawcett for providing feedback during the toolkit development.
Conflict of interest
The authors declare that the research was conducted in the absence of any commercial or financial relationships that could be construed as a potential conflict of interest.
Publisher's note
All claims expressed in this article are solely those of the authors and do not necessarily represent those of their affiliated organizations, or those of the publisher, the editors and the reviewers. Any product that may be evaluated in this article, or claim that may be made by its manufacturer, is not guaranteed or endorsed by the publisher.
References
Almeida, C. A. D., Coutinho, A. C., Esquerdo, J. C. D. M., Adami, M., Venturieri, A., Diniz, C. G., et al. (2016). High spatial resolution land use and land cover mapping of the Brazilian Legal Amazon in 2008 using Landsat-5/TM and MODIS data. Acta. Amazonic. 46, 291–302. doi: 10.1590/1809-4392201505504
Araza, A., De Bruin, P., Herold, S., Quegan, M., Labriere, S., Rodriguez-Veiga, N., et al. (2022). A comprehensive framework for assessing the accuracy and uncertainty of global above-ground biomass maps. Remote Sens. Environ. 272, 112917. doi: 10.1016/j.rse.2022.112917
Berenguer, E., Ferreira, J., Gardner, T. A., Aragão, L. E. O. C., De Camargo, C. E., Cerri, P. B., et al. (2014). A large-scale field assessment of carbon stocks in human-modified tropical forests. Global Change Biol. 20, 3713–3726. doi: 10.1111/gcb.12627
Chen, N., Tsendbazar, N. E., Requena Suarez, D., Verbesselt, J., and Herold, M. (2023). Characterizing aboveground biomass and tree cover of regrowing forests in Brazil using multi-source remote sensing data. Remote Sens. Ecol. Conserv. 9, 553–567. doi: 10.1002/rse2.328
Cook-Patton, S. C., Leavitt, S. M., Gibbs, D., Harris, N. L., Lister, K., Anderson-Teixeira, K. J., et al. (2020). Mapping carbon accumulation potential from global natural forest regrowth. Nature 585, 545–550. doi: 10.1038/s41586-020-2686-x
Descals, A., Wich, S., Meijaard, E., Gaveau, D. L., Peedell, S., Szantoi, Z., et al. (2021). High-resolution global map of smallholder and industrial closed-canopy oil palm plantations. Earth Syst. Sci. Data 13, 1211–1231. doi: 10.5194/essd-13-1211-2021
Fawcett, D., Sitch, S., Ciais, P., Wigneron, J. P., Silva-Junior, C. H. L., Heinrich, V., et al. (2022). Declining Amazon biomass due to deforestation and subsequent degradation losses exceeding gains. Global Change Biol. 4, 1–13. doi: 10.1111/gcb.16513
Gatti, L. V., Basso, L. S., Miller, J. B., Gloor, M., Gatti Domingues, L., Cassol, H. L., et al. (2021). Amazonia as a carbon source linked to deforestation and climate change. Nature 595, 388–393. doi: 10.1038/s41586-021-03629-6
Giglio, L., Boschetti, L., Roy, D. P., Humber, M. L., and Justice, C. O. (2018). The Collection 6 MODIS burned area mapping algorithm and product. Remote Sens. Environ. 217, 72–85. doi: 10.1016/j.rse.2018.08.005
Gorelick, N., Hancher, M., Dixon, M., Ilyushchenko, S., Thau, D., Moore, R., et al. (2017). Google earth engine: planetary-scale geospatial analysis for everyone. Remote Sens. Environ. 202, 18–27. doi: 10.1016/j.rse.2017.06.031
Harris, N., Goldman, E. D., and Gibbes, S. (2019). Spatial Database of Planted Trees (SDPT Version 1, 0.). Washington, DC: World Resources Institute, 1–36.
Heinrich, V. H., Dalagnol, R., Cassol, H. L., Rosan, T. M., De Almeida, C. H., Silva Junior, C. T., et al. (2021). Large carbon sink potential of secondary forests in the Brazilian Amazon to mitigate climate change. Nat. Commun. 12, 1785. doi: 10.1038/s41467-021-22050-1
Hubau, W., Lewis, S. L., Phillips, O. L., Affum-Baffoe, K., Beeckman, H., Cuní-Sanchez, A., et al. (2020). Asynchronous carbon sink saturation in African and Amazonian tropical forests. Nature 579, 80–87. doi: 10.1038/s41586-020-2035-0
MapBiomas Brasil. (2023a). MapBiomas Project - Collection 8 (1985-2022) of Annual Series of Land Use and Land Cover Maps of Brazil. Available online at: https://brasil.mapbiomas.org/en/ (accessed October 9, 2023).
MapBiomas Brasil. (2023b). Annual Mapping of Soil Organic Carbon Stock in Brazil 1985–2021 (Beta Collection). Algorithm Theoretical Basis Document and Results. Available online at: http://data.mapbiomas.org/dataset.xhtml?persistentId=10.58053/MapBiomas/3KXXVV
Nunes, S., Oliveira, L., Siqueira, J., Morton, D. C., and Souza, C. M. (2020). Unmasking secondary vegetation dynamics in the Brazilian Amazon. Environ. Res. Lett. 15, 034057. doi: 10.1088/1748-9326/ab76db
Roe, S., Streck, C., Beach, R., Busch, J., Chapman, M., Daioglou, V., et al. (2021). Land-based measures to mitigate climate change: potential and feasibility by country. Global Change Biol. 27, 6025–6058. doi: 10.1111/gcb.15873
Silva Jr., C. H, Heinrich, V. H., Freire, A. T., Broggio, I. S., Rosan, T. M., Doblas, J., et al. (2020). Benchmark maps of 33 years of secondary forest age for Brazil. Sci. Data 7, 269. doi: 10.1038/s41597-020-00632-w
Souza, C. M., Shimbo, Z., Rosa, J., Parente, M. R. L. L. A., Alencar, A., Rudorff, B. F., and Azevedo, T. (2020). Reconstructing three decades of land use and land cover changes in brazilian biomes with landsat archive and earth engine. Remote Sens. 12, 2735. doi: 10.3390/rs12172735
TerraClass (2023). Mapa das Classes de uso e Cobertura da terra no Bioma Amazônia (Raster). Available online at: http://terrabrasilis.dpi.inpe.br/geonetwork/srv/eng/catalog.search#/metadata/f49a47f8-12ae-4052-8e0d-2e581458dc69 (accessed April 25, 2023).
Keywords: secondary forest, carbon sequestration, tropical forest, Amazon, toolkit, Google Earth Engine
Citation: Heinrich VHA, Sitch S, Rosan TM, Silva-Junior CHL and Aragão LEOC (2023) RE:Growth—A toolkit for analyzing secondary forest aboveground carbon dynamics in the Brazilian Amazon. Front. For. Glob. Change 6:1230734. doi: 10.3389/ffgc.2023.1230734
Received: 29 May 2023; Accepted: 03 October 2023;
Published: 27 October 2023.
Edited by:
Jürgen Homeier, University of Applied Sciences and Arts (HAWK), GermanyReviewed by:
Mateus Dantas De Paula, Senckenberg Biodiversity and Climate Research Centre, GermanyJames J. Gilroy, University of East Anglia, United Kingdom
Copyright © 2023 Heinrich, Sitch, Rosan, Silva-Junior and Aragão. This is an open-access article distributed under the terms of the Creative Commons Attribution License (CC BY). The use, distribution or reproduction in other forums is permitted, provided the original author(s) and the copyright owner(s) are credited and that the original publication in this journal is cited, in accordance with accepted academic practice. No use, distribution or reproduction is permitted which does not comply with these terms.
*Correspondence: Viola H. A. Heinrich, di5oZWlucmljaEBleGV0ZXIuYWMudWs=; dmlvbGEuaGVpbnJpY2hAZ2Z6LXBvdHNkYW0uZGU=