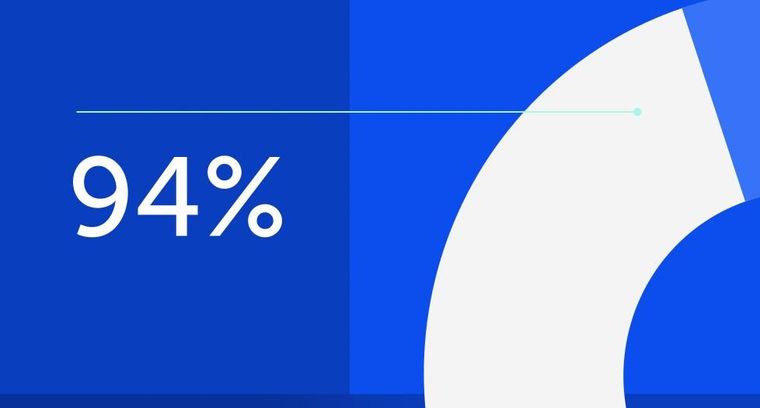
94% of researchers rate our articles as excellent or good
Learn more about the work of our research integrity team to safeguard the quality of each article we publish.
Find out more
REVIEW article
Front. For. Glob. Change, 06 July 2023
Sec. Temperate and Boreal Forests
Volume 6 - 2023 | https://doi.org/10.3389/ffgc.2023.1198186
This article is part of the Research TopicThe Varying Responses of Vegetation Activity to Climate Change in Northern Hemisphere ForestsView all 8 articles
Climate change significantly impacts global agricultural production, giving rise to considerable uncertainties. To explore these climate impacts, three independent methods have been employed: manipulated experiments, process-based crop models, and empirical statistical models. However, the uncertainty stemming from the use of different methods has received insufficient attention, and its implications remain unclear, necessitating a systematic review. In this study, we conducted a comprehensive review of numerous previous studies to summarize the historic development and current status of each method. Through a method comparison, we identified their respective strengths, limitations, and ideal areas of application. Additionally, we outlined potential prospects and suggested directions for future improvements, including clarifying the response mechanisms, updating simulation technologies, and developing multi-method ensembles. By addressing the knowledge gap regarding method differences, this review could contribute to a more accurate assessment of climate impacts on agriculture.
The impact of global climate change on agricultural production in the 21st century has been significant, many countries and regions worldwide have observed reduced yields in crops such as wheat, maize, rice, and oilseed rape (Luo et al., 2005; Arora, 2019; Ray et al., 2019; Sultan et al., 2019; Ortiz-Bobea et al., 2021; Lachaud et al., 2022; Chandio et al., 2023). It is expected that temperatures will continue to rise, leading to an increase in extreme weather events. This trend adds to the agricultural production uncertainty, particularly for major crops such as maize, rice, and soybeans (Vogel et al., 2019; Pörtner et al., 2022). Without adaptation measures, global yields of important food crops could decline by 12–20% by the end of this century (Lobell and Gourdji, 2012; Wheeler and Von Braun, 2013; Challinor et al., 2014; Aggarwal et al., 2019). Therefore, accurately assessing the impact of climate change on crop yields is crucial for ensuring global food security.
There are several methods are used for climate change’s effects on agriculture research, such as manipulated experiments, process-based crop models, and empirical statistical models. Field experiments was the earliest commonly used to expose crops to different climatic conditions, either through natural variations or controlled climate factors, to study their impact on crop growth and yield. With technological advancements, process-based crop models and empirical statistical models have become more prominent. Process-based crop models utilize computer simulations to quantitatively analyze the physiological mechanisms and dynamic processes of crop growth and yield. Empirical statistical models establish mathematical relationships between climate change and crop yield. Over time, significant progress have been made in developing these methodologies. For example, Shi et al. (2013) identified uncertainties in statistical models related to research scale, collinearity of variables, and detrending. Similarly, White et al. (2011) and Rötter et al. (2018) evaluated existing process based crop models, assessing their simulation effects and research standards while highlighting sources of error and limitations. However, many current studies tend to focus on specific research methods, which may introduce biases and uncertainties into climate change impact studies. This limitation reduces the comprehensiveness and reliability of individual approaches. For example, empirical statistical models, based on limited historical observations, face challenges in accurately predicting future yield-climate relationships (Lobell et al., 2006). Conversely, process-based crop models rely on empirical formulas to approximate internal crop growth processes (Wang et al., 2022). Therefore, it is crucial to understand the advantages and disadvantages of each method and explore avenues for future improvement.
This paper presents a comprehensive review of recent advancements in research methods used to study the impacts of climate change on agriculture and adaptation strategies. Its primary aim is to provide researchers with a deeper understanding of existing methods and serve as a reference for future methodological innovations and interdisciplinary collaborations. To achieve this goal, the paper systematically examines three major methods: manipulated experiments, process-based crop models, and empirical statistical models. It critically evaluates the advantages, disadvantages, and directions for future improvement for each method. Additionally, the paper explores the interconnectedness of multiple methodological approaches and their relevance to current research. It also discusses the challenges associated with current methods and highlights potential future research prospects.
Manipulated experiments involve setting different environmental conditions during crop growth to simulate the impact of climate change on crop yield. As an early research method, manipulated experiments has evolved from utilizing natural climate variations to artificial control. This method is simple and straightforward, with a high level of operability. Initially, artificial manipulated experiments were conducted in growth chambers or greenhouse, where temperature, light, water, and gas control experiments were carried out in adjustable but fully enclosed environments. Modern large-scale artificial greenhouse relies on facilities such as strip lights, removable platforms, and exhaust systems to achieve uniform distribution of meteorological factors such as light, temperature, and water. Real-time monitoring and precise control are achieved with the support of computers. Hatfield and Prueger (2015) set up warmer conditions in an artificial climate chamber and found maize yield was significantly reduced. Besides, temperature effects are increased by water deficits and excess.
However, artificial greenhouse block the exchange of water and gases between crops and the external environment, lacking the comprehensive effects of the natural environment. To avoid abnormal increases in humidity and temperature caused by fully enclosed environments, open-top chambers (OTCs) and open-air CO2 enrichment systems (Free-Air CO2 Enrichment, FACE) have been used. OTCs is a gas-enriched greenhouse without a cover on the top, this design ensures sufficient exchange of water and gases between crops and the external environment, significantly enhancing the ability to simulate realistic growth conditions. OTCs have been widely used in experiments investigating the effects of gases such as CO2 and O2 on crop growth (Rogers et al., 1994; Ziska et al., 1997; Ewert et al., 2002; Kakani et al., 2003; Ainsworth et al., 2012). However, there are still some differences in conditions such as wind speed and light within the OTCs compared to natural conditions. Over time, the outer film of the OTCs may undergo oxidation, yellowing, and dirt accumulation, leading to shading of solar radiation and affecting the experimental outcomes (Leadley and Drake, 1993).
To further enhance simulation realism, researchers have increasingly turned to FACE systems. FACE systems release CO2 or CO2-rich air from above the ground onto plant canopies and adjust CO2 flow rates through feedback mechanisms (Long et al., 2004), enabling studies on the effects of elevated CO2 and O3 concentrations on crops’ productivity (Long et al., 2006; Myers et al., 2014). Kimball et al. (2002) conducted experiments in different countries using the FACE system and found elevated CO2 increased crop yield substantially in C3 species, but little in C4. Combining an infrared heater with the FACE system to conduct experiments, known as T-FACE (Temperature-FACE), allows for the research of the combined effects of temperature and CO2 concentration on crops (Ruiz-Vera et al., 2013). Compared to OTC, FACE systems effectively reduce edge effects and cause minimal disturbance to farmland microclimates (Ainsworth et al., 2008). Nonetheless, the vertical gradient of CO2 concentration gradually decreases, which is also influenced by wind speed (Long et al., 2004). Currently, FACE systems are evolving toward genetic variation and transgenic technology research aimed at adapting agricultural planting systems to future climates (Ainsworth et al., 2020).
Process-based crop models are a type of models based on the internal physiological mechanisms of crops, which can comprehensively consider the relationships among the soil-crop-atmosphere system. They describe various physiological processes of crop growth as equations and incorporate various environmental factors (meteorological modules such as temperature, water, and light, as well as soil parameters, cultivars, and agronomic management). The earliest crop models can be traced back to the model on corn canopy photosynthetic rate developed by de Wit (1965). Subsequently, scientists from different countries have developed series of models, such as the DSSAT series (Jones et al., 2003), and the APSIM series (Keating et al., 2003), WOFOST (Van Diepen et al., 1989), CropSyst (Stöckle et al., 2003), SIMPLE (Zhao et al., 2019), etc. In recent years, through setting different climate inputs, crop models have been widely applied in studies on the impacts of climate change on crop yields, whether in long-term changes (Leng and Hall, 2019; Shahid et al., 2021) or in quantifying extreme weather events (Xiao et al., 2022a; Júnior et al., 2023). Researchers have integrated and compared multiple crop models, consistently reached the conclusion that global warming has a significant negative impact on crop yields (Asseng et al., 2013; Bassu et al., 2014; Sultan et al., 2019; Zhao et al., 2022).
Process-based crop models based on site scale consider only the small-scale climate of individual locations. To incorporate large-scale regions, countries, or global scales, it is necessary to utilize crop models at a larger scale. One commonly approach is the grid-scale crop model. It involves dividing the geographic region into grids of specific resolutions and inputs gridded meteorological data, soil data, management data. For example, Deryng et al. (2011) used the PEGASUS model to simulate the response of major cereal crops to future climate change under different agronomic management. Rosenzweig et al. (2014) combined multiple global crop models to simulate the yield impact under historical climate conditions and found that the results were in good agreement with observed values, confirming the applicability of global grid-scale crop models. In recent years, the application of machine learning methods has made remarkable progress in regional or global-scale research, complementing traditional modeling methods through data-driven approaches (Reichstein et al., 2019). Studies have shown that machine learning-based crop modeling systems can accurately and rapidly predict crop yields in large regions at different spatial resolutions (Xiao et al., 2022b).
Empirical statistical models is an approach used by establishing mathematical models that describe the relationship between climate factors and crop production. These models rely on both crop yield data and climate data to establish this correlation. Prior to developing empirical statistical models, it is necessary to separate the climate yield from trend yield and error terms. This is crucial because variations in crop yield are influenced not only by climate change but also by factors like technological advancements. Common methods employed to detrend the original yield data include differencing, multi-year moving averages, linear regression, and filtering analysis (Meza and Silva, 2009; Osborne and Wheeler, 2013; Troy et al., 2015; Kukal and Irmak, 2018). Additionally, considering the nonlinear relationship between economic factors and natural factors in grain production (Xu et al., 2021), econometric models have been introduced. These models include production functions (Just and Pope, 1978; Isik and Devadoss, 2006), economic-climate models (C-D-C models) (Chou and Ye, 2006), and the Ricardo model (Deressa and Hassan, 2009).
Empirical statistical methods have evolved from simple univariate linear regression models to more complex multivariate regression models, incorporating multiple influencing factors. The development has further advanced with the integration of machine learning and deep learning techniques, enabling the transition from univariate to multivariate and from linear to nonlinear modelling. The univariate linear regression model establishes a straightforward linear relationship between crop yield and a single climate factor (Parry and Martens, 1999). For example, Peng et al. (2004) constructed a simple univariate linear model using rice yield data and seasonal temperature data from observation stations in the Philippines. They found a significant negative correlation between rice yield and minimum temperature, with approximately a 10% yield reduction for each 1°C increases in minimum temperature. Univariate regression models can only consider the influence of a specific climate condition, such as temperature alone, and cannot account for all factors affecting yield (Carter et al., 1992). However, climate change is complex and often involves multiple simultaneous climate conditions impacting crop yield. Hence, the application of multivariate regression models has emerged. Multivariate regression models establish climate-yield correlation models with multiple climate conditions as independent variables and yield as the dependent variable. These models can be categorized based on temporal variations (time series models), spatial variations (cross-sectional models), or both (panel models) (Lobell and Burke, 2010). They have demonstrated good performance in simulating the impacts of climate change on crops like maize, wheat, and soybeans (Malikov et al., 2020; Ranjan et al., 2020). For instance, Lobell and Field (2007) established a multivariate linear regression model between temperature, precipitation, and yield, revealing a clear negative response of global wheat, maize, and barley yields to temperature increases. Schlenker and Lobell (2010) developed panel models and projected varying degrees of decline in crop yields such as maize, sorghum, and millet in Sub-Saharan Africa by the mid-century as climate change progresses.
While regression models excel at capturing linear relationships, they face limitations when it comes to simulating nonlinear relationships. To overcome this limitation, machine learning techniques have gained widespread use in climate change impact assessment (Cao et al., 2021; Guo et al., 2021; Lischeid et al., 2022). Machine learning approaches employ semi-parametric variables based on deep neural networks (Crane-Droesch, 2018) or decision systems like support vector machines and fuzzy logic for yield modelling (Palanivel and Surianarayanan, 2019). They leverage algorithms such as decision trees and random forests for prediction (Jeong et al., 2016). Machine learning demonstrates significant potential in yield assessment, surpassing traditional regression models. Unlike traditional empirical statistical models that rely on specific-shaped response functions, machine learning compensates for their limitations by effectively capturing complex nonlinear relationships in high-dimensional datasets. For example, Leng and Hall (2020) compared the performance of machine learning and multivariate regression models and found that machine learning explained 93% of the yield variability, significantly higher than the 51% explained by multivariate regression. Moreover, under a global warming scenario of 2°C, the maize yield in the United States is projected to decrease by 13.5% (Leng and Hall, 2020).
To date, these methods have been widely used. However, due to differences in their underlying principles, these methods have both advantages and disadvantages in practical applications (Table 1).
Using manipulated experiments to precisely regulate the thresholds of climate factors can simulate the actual environmental conditions of crop growth and development, resulting in high reliability of the obtained results. Therefore, the results obtained from controlled experiments are often used to calibrate crop models (Asseng et al., 2004). However, the experimental period of manipulated experiments is dependent on the crop’s growth cycle, and it involves complex operations, a long experimental cycle, and substantial human and material resources. Consequently, it is challenging to conduct long-term studies on future climate changes spanning several decades to centuries. Furthermore, due to the heterogeneity of the climate terrain and soil in the experimental area, the site-based experimental results have poor representativeness to larger areas. To upscale to the regional scale, a large amount of experimental data might be needed. A plausible solution is to establish a unified research framework. For example, Coordinated Distributed Experiments (CDE) offers the advantage of addressing global problems while ensuring the inherent accuracy of control experiments (Fraser et al., 2013).
Process-based crop models can effectively simulate the physical mechanisms of crop growth and strictly control the impact of single variables, avoiding the need for long-term field experiments. However, operating such models requires a significant amount of parameter calibration work, especially for large scale and long term simulations, and the process is complex. Within the model, crop growth processes and growing environments are approximately described using empirical or descriptive formulas, which results in some deviations in the response of crop physiological processes. Moreover, this uncertainty varies among different models (Wang et al., 2022).
Empirical statistical models evaluate and predict crop yields by establishing a correlation between climate factors and historical yields, avoiding the complex tuning required by crop models and the need for inputting soil properties and management practices measured in the field. These models are relatively easy to apply and suitable for regional and global-scale studies. However, the climate factors used as input for these models are often monthly or seasonal averages, smoothing the impact of climate variability during the growing season and neglecting the effects of extreme weather events (Chen et al., 2004). Additionally, the accuracy of detrending methods used in empirical statistical models is difficult to evaluate. Moreover, empirical statistical models are based on limited historical observations, which introduce sampling uncertainty and simulation bias (Lobell et al., 2006). Because these models lack a reliable physical mechanism for extrapolation, they exhibit uncertainty in predicting yields beyond the historical range, and are more suitable for historical or near future studies.
The majority of existing yield prediction models operate as “one-way” mechanisms, failing to account for the fact that crops can alter their morphology and physiological functions to adapt to climate change. Moreover, current models are inadequate in assessing the chain reaction of crops to a series of weather events, their ability to adapt to the combined effects of multiple factors, and their response to the precursors of impact factors (Suzuki et al., 2014). In the future, more attention should be paid to the comprehensive influence of soil conditions, hydrological cycle, pest problems, and other factors in crop models (Newbery et al., 2016; Basso et al., 2018; Deutsch et al., 2018; Tomaz et al., 2020; Wei et al., 2021; Denissen et al., 2022). Additionally, the potential impact of climate change on crop production is not limited to the growth period, as environmental changes during non-growth periods can also indirectly affect crop production. Therefore, it is necessary to establish a model that comprehensively considers the basic knowledge of plant physiology and atmospheric science, including feedback mechanisms (Tonnang et al., 2022), in order to achieve a balance between the authenticity and controllability of the simulation.
The incorporation of emerging technologies can significantly enhance the research capabilities of traditional methods during the process of methodological development. For instance, the integration of remote sensing, big data, and artificial intelligence into existing approaches (Jiang et al., 2020) can address more complex data acquisition and processing requirements, enabling large-scale simulation and regulation. The utilization of remote sensing technology allows for weather and crop data with multiple spatial and temporal resolutions, enabling the assimilation of dynamic crop phenotype data provided by satellites to achieve closer real-time monitoring. This, in turn, enhances the ability of crop models to monitor and predict large-scale crop yields (Huang et al., 2019). However, the current stage of development of data assimilation technology and its application in this field is still in its early stages. As such, machine learning technologies may be key to enhancing the maturity of this approach (Cai et al., 2019).
Many researchers have assembled multiple crop models to optimize simulation effectiveness and avoid systematic errors associated with a single model (Bassu et al., 2014; Martre et al., 2015), which have been shown to provide more reliable results (Asseng et al., 2013). However, integrating models does not fundamentally improve the underlying mechanisms, combining physiological principles and basic science can be essential (Yin et al., 2021). What is more remarkable is that combining multiple methods can effectively reduce uncertainty (Zhao et al., 2017). For instance, the integration of manipulated experiments with process-based crop models can supplement missing modules within existing models or establish more targeted new models by observing various physiological processes throughout the entire growth period of crops under particular conditions. In addition, developing a joint model that combines process-based crop models with statistical models (Roberts et al., 2017) can be beneficial. Such models use simple statistical models to summarize and statistically analyze simulation results generated by process-based crop models. By using polynomials and limited weather variables, these models can accurately replicate process-based crop model results in global grid cells, avoiding the complex parameter adjustment process associated with process-based crop models while also predicting long-term trends (Blanc and Sultan, 2015).
CZ motivated the conception and review design. XF and HT wrote the draft of the manuscript. CZ and JC contributed to the manuscript revision. All authors contributed to the article and approved the submitted version.
This work was supported by the National Natural Science Youth Foundation of China (42201032), the National Natural Science Fund for Excellent Young Scholars (Overseas), and the Fundamental Research Funds for the Central Universities (15053348).
The authors declare that the research was conducted in the absence of any commercial or financial relationships that could be construed as a potential conflict of interest.
All claims expressed in this article are solely those of the authors and do not necessarily represent those of their affiliated organizations, or those of the publisher, the editors and the reviewers. Any product that may be evaluated in this article, or claim that may be made by its manufacturer, is not guaranteed or endorsed by the publisher.
Aggarwal, P., Vyas, S., Thornton, P., Campbell, B. M., and Kropff, M. (2019). Importance of considering technology growth in impact assessments of climate change on agriculture. Glob Food Sec. 23, 41–48. doi: 10.1016/j.gfs.2019.04.002
Ainsworth, E. A., Leakey, A. D., Ort, D. R., and Long, S. P. (2008). FACE-ing the facts: Inconsistencies and interdependence among field, chamber and modeling studies of elevated [CO2] impacts on crop yield and food supply. New Phytol. 179, 5–9. doi: 10.1111/j.1469-8137.2008.02500.x
Ainsworth, E. A., Lemonnier, P., and Wedow, J. (2020). The influence of rising tropospheric carbon dioxide and ozone on plant productivity. Plant Biol. 22, 5–11. doi: 10.1111/plb.12973
Ainsworth, E. A., Yendrek, C. R., Sitch, S., Collins, W. J., and Emberson, L. D. (2012). The effects of tropospheric ozone on net primary productivity and implications for climate change. Annu. Rev. Plant Biol. 63, 637–661. doi: 10.1146/annurev-arplant-042110-103829
Arora, N. K. (2019). Impact of climate change on agriculture production and its sustainable solutions. Environ. Sustain. 2, 95–96. doi: 10.1007/s42398-019-00078-w
Asseng, S., Ewert, F., Rosenzweig, C., Jones, J. W., Hatfield, J. L., Ruane, A. C., et al. (2013). Uncertainty in simulating wheat yields under climate change. Nat. Clim. Change 3, 827–832. doi: 10.1038/nclimate1916
Asseng, S., Jamieson, P., Kimball, B., Pinter, P., Sayre, K., Bowden, J., et al. (2004). Simulated wheat growth affected by rising temperature, increased water deficit and elevated atmospheric CO2. Field Crops Res. 85, 85–102.
Basso, B., Dumont, B., Maestrini, B., Shcherbak, I., Robertson, G. P., Porter, J. R., et al. (2018). Soil organic carbon and nitrogen feedbacks on crop yields under climate change. Agric. Environ. Lett. 3:180026. doi: 10.2134/ael2018.05.0026
Bassu, S., Brisson, N., Durand, J. L., Boote, K., Lizaso, J., Jones, J. W., et al. (2014). How do various maize crop models vary in their responses to climate change factors? Glob. Change Biol. 20, 2301–2320. doi: 10.1111/gcb.12520
Blanc, E., and Sultan, B. (2015). Emulating maize yields from global gridded crop models using statistical estimates. Agric. For. Meteorol. 214, 134–147. doi: 10.1016/j.agrformet.2015.08.256
Cai, Y., Guan, K., Lobell, D., Potgieter, A. B., Wang, S., Peng, J., et al. (2019). Integrating satellite and climate data to predict wheat yield in Australia using machine learning approaches. Agric. For. Meteorol. 274, 144–159. doi: 10.1016/j.agrformet.2019.03.010
Cao, J., Zhang, Z., Tao, F., Zhang, L., Luo, Y., Zhang, J., et al. (2021). Integrating multi-source data for rice yield prediction across China using machine learning and deep learning approaches. Agric. For. Meteorol. 297:108275. doi: 10.1016/j.agrformet.2020.108275
Carter, T. R., Parry, M., Nishioka, S., and Harasawa, H. (1992). Preliminary guidelines for assessing impacts of climate change. Geneva: IPCC.
Challinor, A. J., Watson, J., Lobell, D. B., Howden, S., Smith, D., and Chhetri, N. (2014). A meta-analysis of crop yield under climate change and adaptation. Nat. Clim. Change 4, 287–291. doi: 10.1038/nclimate2153
Chandio, A. A., Jiang, Y., Amin, A., Ahmad, M., Akram, W., and Ahmad, F. (2023). Climate change and food security of South Asia: Fresh evidence from a policy perspective using novel empirical analysis. J. Environ. Plan. Manag. 66, 169–190. doi: 10.1080/09640568.2021.1980378
Chen, C. C., McCarl, B. A., and Schimmelpfennig, D. E. (2004). Yield variability as influenced by climate: A statistical investigation. Clim. Change 66, 239–261. doi: 10.1023/B:CLIM.0000043159.33816.e5
Chou, J. M., and Ye, D. Z. (2006). Assessing the effect of climate changes on grains yields with a new economy-climate model. Clim. Environ. Res. 11, 347–353.
Crane-Droesch, A. (2018). Machine learning methods for crop yield prediction and climate change impact assessment in agriculture. Environ. Res. Lett. 13:114003.
Denissen, J. M., Teuling, A. J., Pitman, A. J., Koirala, S., Migliavacca, M., Li, W., et al. (2022). Widespread shift from ecosystem energy to water limitation with climate change. Nat. Clim. Change 12, 677–684. doi: 10.1038/s41558-022-01403-8
Deressa, T. T., and Hassan, R. M. (2009). Economic impact of climate change on crop production in Ethiopia: Evidence from cross-section measures. J. Afr. Econ. 18, 529–554. doi: 10.1093/jae/ejp002
Deryng, D., Sacks, W., Barford, C., and Ramankutty, N. (2011). Simulating the effects of climate and agricultural management practices on global crop yield. Glob. Biogeochem. Cycles 25.
Deutsch, C. A., Tewksbury, J. J., Tigchelaar, M., Battisti, D. S., Merrill, S. C., Huey, R. B., et al. (2018). Increase in crop losses to insect pests in a warming climate. Science 361, 916–919. doi: 10.1126/science.aat3466
Ewert, F., Rodriguez, D., Jamieson, P., Semenov, M., Mitchell, R., Goudriaan, J., et al. (2002). Effects of elevated CO2 and drought on wheat: Testing crop simulation models for different experimental and climatic conditions. Agric. Ecosyst. Environ. 93, 249–266. doi: 10.1016/S0167-8809(01)00352-8
Fraser, L. H., Henry, H. A., Carlyle, C. N., White, S. R., Beierkuhnlein, C., Cahill, J. F., et al. (2013). Coordinated distributed experiments: An emerging tool for testing global hypotheses in ecology and environmental science. Front. Ecol. Environ. 11:147–155. doi: 10.1890/110279
Guo, Y., Fu, Y., Hao, F., Zhang, X., Wu, W., Jin, X., et al. (2021). Integrated phenology and climate in rice yields prediction using machine learning methods. Ecol. Indicat. 120:106935. doi: 10.1016/j.ecolind.2020.106935
Hatfield, J. L., and Prueger, J. H. (2015). Temperature extremes: Effect on plant growth and development. Weather Clim. Extremes 10, 4–10.
Huang, J., Gómez-Dans, J. L., Huang, H., Ma, H., Wu, Q., Lewis, P. E., et al. (2019). Assimilation of remote sensing into crop growth models: Current status and perspectives. Agric. For. Meteorol. 276:107609. doi: 10.1016/j.agrformet.2019.06.008
Isik, M., and Devadoss, S. (2006). An analysis of the impact of climate change on crop yields and yield variability. Appl. Econ. 38, 835–844. doi: 10.1080/00036840500193682
Jeong, J. H., Resop, J. P., Mueller, N. D., Fleisher, D. H., Yun, K., Butler, E. E., et al. (2016). Random forests for global and regional crop yield predictions. PLoS One 11:e0156571. doi: 10.1371/journal.pone.0156571
Jiang, H., Hu, H., Zhong, R., Xu, J., Xu, J., Huang, J., et al. (2020). A deep learning approach to conflating heterogeneous geospatial data for corn yield estimation: A case study of the US Corn Belt at the county level. Glob. Change Biol. 26, 1754–1766. doi: 10.1111/gcb.14885
Jones, J. W., Hoogenboom, G., Porter, C. H., Boote, K. J., Batchelor, W. D., Hunt, L., et al. (2003). The DSSAT cropping system model. Eur. J. Agron. 18, 235–265. doi: 10.1016/S1161-0301(02)00107-7
Júnior, R. D. S. N., Deswarte, J. C., Cohan, J. P., Martre, P., van der Velde, M., Lecerf, R., et al. (2023). The extreme 2016 wheat yield failure in France. Glob. Change Biol. 29, 3130–3146. doi: 10.1111/gcb.16662
Just, R. E., and Pope, R. D. (1978). Stochastic specification of production functions and economic implications. J. Econ. 7, 67–86. doi: 10.1016/0304-4076(78)90006-4
Kakani, V., Reddy, K., Zhao, D., and Sailaja, K. (2003). Field crop responses to ultraviolet-B radiation: A review. Agric. For. Meteorol. 120, 191–218. doi: 10.1016/j.agrformet.2003.08.015
Keating, B. A., Carberry, P. S., Hammer, G. L., Probert, M. E., Robertson, M. J., Holzworth, D., et al. (2003). An overview of APSIM, a model designed for farming systems simulation. Eur. J. Agron. 18, 267–288. doi: 10.1016/S1161-0301(02)00108-9
Kimball, B., Kobayashi, K., and Bindi, M. (2002). Responses of agricultural crops to free-air CO2 enrichment. Adv. Agron. 77, 293–368.
Kukal, M. S., and Irmak, S. (2018). Climate-driven crop yield and yield variability and climate change impacts on the US Great Plains agricultural production. Sci. Rep. 8:3450. doi: 10.1038/s41598-018-21848-2
Lachaud, M. A., Bravo-Ureta, B. E., and Ludena, C. E. (2022). Economic effects of climate change on agricultural production and productivity in Latin America and the Caribbean (LAC). Agric. Econ. 53, 321–332. doi: 10.1111/agec.12682
Leadley, P. W., and Drake, B. G. (1993). Open top chambers for exposing plant canopies to elevated CO 2 concentration and for measuring net gas exchange. Vegetatio 104, 3–15. doi: 10.1007/BF00048141
Leng, G., and Hall, J. (2019). Crop yield sensitivity of global major agricultural countries to droughts and the projected changes in the future. Sci. Total Environ. 654, 811–821.
Leng, G., and Hall, J. W. (2020). Predicting spatial and temporal variability in crop yields: An inter-comparison of machine learning, regression and process-based models. Environ. Res. Lett. 15:044027. doi: 10.1088/1748-9326/ab7b24
Lischeid, G., Webber, H., Sommer, M., Nendel, C., and Ewert, F. (2022). Machine learning in crop yield modelling: A powerful tool, but no surrogate for science. Agric. For. Meteorol. 312:108698. doi: 10.1016/j.agrformet.2021.108698
Lobell, D. B., and Burke, M. B. (2010). On the use of statistical models to predict crop yield responses to climate change. Agric. For. Meteorol. 150, 1443–1452. doi: 10.1016/j.agrformet.2010.07.008
Lobell, D. B., and Field, C. B. (2007). Global scale climate–crop yield relationships and the impacts of recent warming. Environ. Res. Lett. 2:014002.
Lobell, D. B., and Gourdji, S. M. (2012). The influence of climate change on global crop productivity. Plant Physiol. 160, 1686–1697. doi: 10.1104/pp.112.208298
Lobell, D. B., Field, C. B., Cahill, K. N., and Bonfils, C. (2006). Impacts of future climate change on California perennial crop yields: Model projections with climate and crop uncertainties. Agric. For. Meteorol. 141, 208–218. doi: 10.1016/j.agrformet.2006.10.006
Long, S. P., Ainsworth, E. A., Leakey, A. D., Nosberger, J., and Ort, D. R. (2006). Food for thought: Lower-than-expected crop yield stimulation with rising CO2 concentrations. Science 312, 1918–1921. doi: 10.1126/science.1114722
Long, S. P., Ainsworth, E. A., Rogers, A., and Ort, D. R. (2004). Rising atmospheric carbon dioxide: Plants FACE the future. Annu. Rev. Plant Biol. 55, 591–628. doi: 10.1146/annurev.arplant.55.031903.141610
Luo, Q., Bellotti, W., Williams, M., and Bryan, B. (2005). Potential impact of climate change on wheat yield in South Australia. Agric. For. Meteorol. 132, 273–285. doi: 10.1016/j.agrformet.2005.08.003
Malikov, E., Miao, R., and Zhang, J. (2020). Distributional and temporal heterogeneity in the climate change effects on US agriculture. J. Environ. Econ. Manag. 104:102386. doi: 10.1016/j.jeem.2020.102386
Martre, P., Wallach, D., Asseng, S., Ewert, F., Jones, J. W., Rötter, R. P., et al. (2015). Multimodel ensembles of wheat growth: Many models are better than one. Glob. Change Biol. 21, 911–925. doi: 10.1111/gcb.12768
Meza, F. J., and Silva, D. (2009). Dynamic adaptation of maize and wheat production to climate change. Clim. Change 94, 143–156. doi: 10.1007/s10584-009-9544-z
Myers, S. S., Zanobetti, A., Kloog, I., Huybers, P., Leakey, A. D., Bloom, A. J., et al. (2014). Increasing CO2 threatens human nutrition. Nature 510, 139–142. doi: 10.1038/nature13179
Newbery, F., Qi, A., and Fitt, B. D. (2016). Modelling impacts of climate change on arable crop diseases: Progress, challenges and applications. Curr. Opin. Plant Biol. 32, 101–109. doi: 10.1016/j.pbi.2016.07.002
Ortiz-Bobea, A., Ault, T. R., Carrillo, C. M., Chambers, R. G., and Lobell, D. B. (2021). Anthropogenic climate change has slowed global agricultural productivity growth. Nat. Clim. Change 11, 306–312. doi: 10.1038/s41558-021-01000-1
Osborne, T. M., and Wheeler, T. R. (2013). Evidence for a climate signal in trends of global crop yield variability over the past 50 years. Environ. Res. Lett. 8:024001. doi: 10.1088/1748-9326/8/2/024001
Palanivel, K., and Surianarayanan, C. (2019). An approach for prediction of crop yield using machine learning and big data techniques. Int. J. Comput. Eng. Technol. 10, 110–118. doi: 10.34218/IJCET.10.3.2019.013
Parry, M., and Martens, P. (1999). “Impact Assessment of Climate Change,” in Climate change: An integrated perspective. advances in global change research, eds P. Martens, J. Rotmans, D. Jansen, and K. Vrieze (Dordrecht: Springer), 201–238. doi: 10.1007/0-306-47982-6_6
Peng, S., Huang, J., Sheehy, J. E., Laza, R. C., Visperas, R. M., Zhong, X., et al. (2004). Rice yields decline with higher night temperature from global warming. Proc. Natl. Acad. Sci. U.S.A. 101, 9971–9975.
Pörtner, H.-O., Roberts, D. C., Adams, H., Adler, C., Aldunce, P., Ali, E., et al. (2022). Climate change 2022: Impacts, adaptation and vulnerability. Geneva: IPCC.
Ranjan, R. K., Kumari, M., Rahman, M., Panda, C., and Homa, F. (2020). Effect of climate variables on yield of major crop in Samastipur district of Bihar: A time series analysis. Econ. Affairs 65, 637–644. doi: 10.46852/0424-2513.4.2020.21
Ray, D. K., West, P. C., Clark, M., Gerber, J. S., Prishchepov, A. V., and Chatterjee, S. (2019). Climate change has likely already affected global food production. PLoS One 14:e0217148. doi: 10.1371/journal.pone.0217148
Reichstein, M., Camps-Valls, G., Stevens, B., Jung, M., Denzler, J., and Carvalhais, N. (2019). Deep learning and process understanding for data-driven Earth system science. Nature 566, 195–204. doi: 10.1038/s41586-019-0912-1
Roberts, M. J., Braun, N. O., Sinclair, T. R., Lobell, D. B., and Schlenker, W. (2017). Comparing and combining process-based crop models and statistical models with some implications for climate change. Environ. Res. Lett. 12:095010. doi: 10.1088/1748-9326/aa7f33
Rogers, H. H., Runion, G. B., and Krupa, S. V. (1994). Plant responses to atmospheric CO2 enrichment with emphasis on roots and the rhizosphere. Environ. Pollut. 83, 155–189. doi: 10.1016/0269-7491(94)90034-5
Rosenzweig, C., Elliott, J., Deryng, D., Ruane, A. C., Müller, C., Arneth, A., et al. (2014). Assessing agricultural risks of climate change in the 21st century in a global gridded crop model intercomparison. Proc. Natl. Acad. Sci. U. S. A. 111, 3268–3273. doi: 10.1073/pnas.1222463110
Rötter, R. P., Hoffmann, M., Koch, M., and Müller, C. (2018). Progress in modelling agricultural impacts of and adaptations to climate change. Curr. Opin. Plant Biol. 45, 255–261. doi: 10.1016/j.pbi.2018.05.009
Ruiz-Vera, U. M., Siebers, M., Gray, S. B., Drag, D. W., Rosenthal, D. M., Kimball, B. A., et al. (2013). Global warming can negate the expected CO2 stimulation in photosynthesis and productivity for soybean grown in the Midwestern United States. Plant Physiol. 162, 410–423. doi: 10.1104/pp.112.211938
Shahid, M. R., Wakeel, A., Ishaque, W., Ali, S., Soomro, K. B., and Awais, M. (2021). Optimizing different adaptive strategies by using crop growth modeling under IPCC climate change scenarios for sustainable wheat production. Environ. Dev. Sustain. 23, 11310–11334.
Schlenker, W., and Lobell, D. B. (2010). Robust negative impacts of climate change on African agriculture. Environ. Res. Lett. 5:014010.
Shi, W., Tao, F., and Zhang, Z. (2013). A review on statistical models for identifying climate contributions to crop yields. J. Geogr. Sci. 23, 567–576. doi: 10.1007/s11442-013-1029-3
Stöckle, C. O., Donatelli, M., and Nelson, R. (2003). CropSyst, a cropping systems simulation model. Eur. J. Agron. 18, 289–307.
Sultan, B., Defrance, D., and Iizumi, T. (2019). Evidence of crop production losses in West Africa due to historical global warming in two crop models. Sci. Rep. 9:12834. doi: 10.1038/s41598-019-49167-0
Suzuki, N., Rivero, R. M., Shulaev, V., Blumwald, E., and Mittler, R. (2014). Abiotic and biotic stress combinations. New Phytol. 203, 32–43. doi: 10.1111/nph.12797
Tomaz, A., Palma, P., Alvarenga, P., and Gonçalves, M. C. (2020). “Soil salinity risk in a climate change scenario and its effect on crop yield,” in Climate change and soil interactions, eds M. N. Prasad and M. Pietrzykowski (Amsterdam: Elsevier), 351–396. doi: 10.1016/B978-0-12-818032-7.00013-8
Tonnang, H. E., Sokame, B. M., Abdel-Rahman, E. M., and Dubois, T. (2022). Measuring and modelling crop yield losses due to invasive insect pests under climate change. Curr. Opin. Insect Sci. 50:100873. doi: 10.1016/j.cois.2022.100873
Troy, T. J., Kipgen, C., and Pal, I. (2015). The impact of climate extremes and irrigation on US crop yields. Environ. Res. Lett. 10:054013. doi: 10.1088/1748-9326/10/5/054013
Van Diepen, C. V., Wolf, J. V., Van Keulen, H., and Rappoldt, C. (1989). WOFOST: A simulation model of crop production. Soil Use Manag. 5, 16–24. doi: 10.1111/j.1475-2743.1989.tb00755.x
Vogel, E., Donat, M. G., Alexander, L. V., Meinshausen, M., Ray, D. K., Karoly, D., et al. (2019). The effects of climate extremes on global agricultural yields. Environ. Res. Lett. 14:054010. doi: 10.1088/1748-9326/ab154b
Wang, E., He, D., Wang, J., Lilley, J. M., Christy, B., Hoffmann, M. P., et al. (2022). How reliable are current crop models for simulating growth and seed yield of canola across global sites and under future climate change? Clim. Change 172:20. doi: 10.1007/s10584-022-03375-2
Wei, S., Peng, A., Huang, X., Deng, A., Chen, C., and Zhang, W. (2021). Contributions of climate and soil properties to wheat and maize yield based on long-term fertilization experiments. Plants 10:2002. doi: 10.3390/plants10102002
Wheeler, T., and Von Braun, J. (2013). Climate change impacts on global food security. Science 341, 508–513. doi: 10.1126/science.1239402
White, J. W., Hoogenboom, G., Kimball, B. A., and Wall, G. W. (2011). Methodologies for simulating impacts of climate change on crop production. Field Crops Res. 124, 357–368. doi: 10.1016/j.fcr.2011.07.001
Xiao, L., Asseng, S., Wang, X., Xia, J., Zhang, P., Liu, L., et al. (2022a). Simulating the effects of low-temperature stress on wheat biomass growth and yield. Agric. For. Meteorol. 326:109191. doi: 10.1016/j.agrformet.2022.109191
Xiao, L., Wang, G., Zhou, H., Jin, X., and Luo, Z. (2022b). Coupling agricultural system models with machine learning to facilitate regional predictions of management practices and crop production. Environ. Res. Lett. 17:114027. doi: 10.1088/1748-9326/ac9c71
Xu, Y., Chou, J., Yang, F., Sun, M., Zhao, W., and Li, J. (2021). Assessing the sensitivity of main crop yields to climate change impacts in China. Atmosphere 12:172. doi: 10.3390/atmos12020172
Yin, X., Struik, P. C., and Goudriaan, J. (2021). On the needs for combining physiological principles and mathematics to improve crop models. Field Crops Res. 271:108254. doi: 10.1016/j.fcr.2021.108254
Zhao, C., Liu, B., Piao, S., Wang, X., Lobell, D. B., Huang, Y., et al. (2017). Temperature increase reduces global yields of major crops in four independent estimates. Proc. Natl. Acad. Sci. U.S.A. 114, 9326–9331.
Zhao, C., Liu, B., Xiao, L., Hoogenboom, G., Boote, K. J., Kassie, B. T., et al. (2019). A SIMPLE crop model. Eur. J. Agron. 104, 97–106. doi: 10.1016/j.eja.2019.01.009
Zhao, C., Stockle, C. O., Karimi, T., Nelson, R. L., van Evert, F. K., Pronk, A. A., et al. (2022). Potential benefits of climate change for potatoes in the United States. Environ. Res. Lett. 17:104034.
Keywords: crop yield, climate change, research methods, manipulated experiment, process-based crop model, empirical statistical model
Citation: Feng X, Tian H, Cong J and Zhao C (2023) A method review of the climate change impact on crop yield. Front. For. Glob. Change 6:1198186. doi: 10.3389/ffgc.2023.1198186
Received: 31 March 2023; Accepted: 01 June 2023;
Published: 06 July 2023.
Edited by:
Hongfang Zhao, East China Normal University, ChinaReviewed by:
Liujun Xiao, Nanjing Agricultural University, ChinaCopyright © 2023 Feng, Tian, Cong and Zhao. This is an open-access article distributed under the terms of the Creative Commons Attribution License (CC BY). The use, distribution or reproduction in other forums is permitted, provided the original author(s) and the copyright owner(s) are credited and that the original publication in this journal is cited, in accordance with accepted academic practice. No use, distribution or reproduction is permitted which does not comply with these terms.
*Correspondence: Chuang Zhao, emhhb2NodWFuZ0BjYXUuZWR1LmNu
†These authors have contributed equally to this work and share first authorship
Disclaimer: All claims expressed in this article are solely those of the authors and do not necessarily represent those of their affiliated organizations, or those of the publisher, the editors and the reviewers. Any product that may be evaluated in this article or claim that may be made by its manufacturer is not guaranteed or endorsed by the publisher.
Research integrity at Frontiers
Learn more about the work of our research integrity team to safeguard the quality of each article we publish.