- 1Department of Ecology, School of Life Sciences/State Key Laboratory of Biocontrol, Sun Yat-sen University, Guangzhou, China
- 2Research Institute of Sun Yat-sen University in Shenzhen, Shenzhen, China
- 3Guangdong Neilingding Futian National Nature Reserve, Shenzhen, China
Litterfall may facilitate the outperformance of exotic species by inducing better resource acquisition and reproductive performance. However, the drivers that determine litterfall patterns in exotic and native species have remained insufficiently investigated due to the lack of long-term observations. Here, we employed empirical dynamic modeling on a two-decade-long litterfall observation (1999–2019) in a subtropical mangrove forest in Shenzhen, China, to evaluate the relative importance of environmental drivers on different species. We found that mangrove leaf litterfall in both exotic and native species was strongly altered by temperature, and the causal relationship was stronger in the dominant exotic species Sonneratia apetala compared to native species. However, the main driver of reproductive output differed largely between exotic and native species; temperature was the main cause of native reproduction, whereas coastal nutrients drove the reproductive output of exotic species. Our study highlighted that high nutrient availability in Shenzhen Bay in the past decade allowed the exotic species S. apetala to gain better reproductive output than native species. We imply that enriched nutrients in coastal water likely contributed to exotic dominance in China’s coastal mangrove forest.
1. Introduction
Mangroves are highly productive ecosystems, but they are prone to natural disturbances and human stressors such as land-use change and coastal eutrophication (Friess et al., 2019). Litterfall is one of major pathways of soil modification and nutrient cycling (Wardle et al., 2004) and may induce plant–soil feedback on species growth and competitiveness, which in turn facilitate the invasion process (Eppinga et al., 2011). Previous studies have produced knowledge on the role of litterfall in species interaction and the functional stability of mangrove ecosystems (e.g., Feller et al., 2015), but how the environment shapes litterfall patterns in different species remains insufficiently investigated, particularly for coastal mangroves that suffer from eutrophication and ongoing climate change (Branoff, 2018). This knowledge gap limits our understanding of the environment contributions to the exotic dominance in China’s coastal mangrove forests and hinders the prediction of ecosystem responses in the context of climate change.
The ongoing climate change is altering various components of the environment at different rates and has been exerting influence on natural ecosystems with additional uncertainties due to human-induced stressors (IPCC, 2022). Climatic signals (i.e., temperature and rainfall), local resource availability, and other environmental stressors (e.g., mean sea level and salinity) may differentially drive the long-term adaptation across different mangrove species (Bernhardt et al., 2020). Theoretically, plants optimize growth and reproduction with adaptive strategies, thereby matching their life-cycle events with optimal climatic conditions (Iler et al., 2021), and longer-term local adaptation may confer on native species a better ability to anticipate regular environmental changes. However, the complexity of environment interactions in the real world can hinder organisms from exploiting environmental cues; the capacity to utilize reliable information varies substantially among species (Bernhardt et al., 2020). Invasive exotic species often exhibit high environmental sensitivity and phenological flexibility (i.e., they are more capable of exploiting environmental cues) and are likely preferred in a changeable environment (Richards et al., 2006).
Resource availability is changeable through tidal currents in intertidal areas occupied by mangrove species, and litterfall plays an important role in nutrient cycling and soil modification and may be especially helpful in niche construction and invader success (Eppinga et al., 2011). The influx and outflux of nutrients and other materials in a mangrove forest are determined by hydrogeological conditions and connectivity to nearby ecosystems (Lee, 1995; Meijer et al., 2021). For example, mangrove forests near urban areas can receive enriched nutrients from sewage disposal (Branoff, 2018), while those growing in habitats with strong weathering of highly leached soils may encounter nutrient deficiency (Reef et al., 2010). There are growing demands for a comparison between species on their sensitivity to environmental change after the great insights gained from previous studies into the functional mechanisms of mangrove adaptability to different conditions. It has long been suggested that evergreen mangrove trees alter the rates of resource allocation and leaf shedding to preserve the budget balance in canopy according to different environmental contexts (Hikosaka, 2004). Therefore, temporal variations of litterfall that are associated with environmental changes may reflect species preference and tolerance of different environmental contexts.
Previous research has linked litterfall to plant–soil feedback and functional stability in plant communities (Wimmler et al., 2021). Mangrove litter inputs of leaves and propagules not only modify soil properties but are important food sources for benthic fauna, which determine the limiting nutrients for plants and may induce feedback on plant growth and survival (Cannicci et al., 2008; Cherif and Loreau, 2008). For example, litter inputs in mangroves in Belize and Florida, United States, contributed to soil surface elevation and in turn stabilized the mangrove ecosystems (McKee, 2011). Moreover, in a previous study of the Shenzhen mangroves in China, the litterfall of the exotic species Sonneratia apetala supplied nutrients and organic matter to the soil and associated fauna, leading to a positive feedback on growth and dispersal of the species (Liu et al., 2014). Despite numerous field observations that have documented the temporal synchrony of mangrove litterfall and meteorological variations (e.g., Duke, 1990; Liu et al., 2014), most were restricted by relatively short periods to identify interannual patterns and offered limited insight into how the environment shapes species response in terms of litter biomass. Moreover, between-species contrasts in litterfall variations at seasonal versus inter-annual scales can reflect species sensitivity to environmental changes. The analysis of litterfall response to environmental change on a monthly and annual basis, and especially the contrast between native and exotic species, can inform us of the environmental cues utilized by different species and will benefit our management strategy to adapt to the ongoing climate change.
Mangroves are vulnerable to climatic variations, but disturbances may create opportunities for exotic species when they exhibit higher plasticity and adaptability than native species (Funk, 2008). Successful exotic species are often characterized as resource-exploitative species (Daehler, 2003; Knauf et al., 2021). They grow with native species and utilize resources at an efficiency comparable to the natives when resources are limited (Funk and Vitousek, 2007), but they can capitalize more on additional resources at a fast response (Daehler, 2003; Knauf et al., 2021), and may in turn suppress the canopy growth of native species and take over areas after perturbations (Biswas et al., 2012). The resource-exploitative feature is important for exotic species entering urban mangroves, where the fast response and high plasticity allow the species to seize opportunity and gain more advantage in growth and reproduction over others in the context of additional resources, such as nutrient enrichment from urban sewage disposal (Branoff, 2018). As a result, successful exotic species may respond more tightly and positively than native species to environmental variations (Liu et al., 2017) and exhibit more litterfall to alter soil properties and neighboring organisms (Smith et al., 2021).
The exotic S. apetala in China’s coastal mangrove forests offers a good object for the examination of environmental contributions on mangrove litterfall between exotic and native species. S. apetala has been introduced from Bangladesh (the Sundarbans mangrove) to multiple coastal mangrove forests in China for afforestation since the 1990s (Zan et al., 2001; Ren et al., 2009). The range of latitude of the main planting area in China was consistent with the forests from where the species originated (Tian et al., 2010), and it currently thrives and covers a vast area without human intervention (Biswas et al., 2018; Zhang et al., 2022). The dominance of this new “immigrant” has triggered a considerable debate on its invasiveness and has been attracting numerous research teams to evaluate the risks posed to native species (Zan et al., 2003; Ren et al., 2009). Meanwhile, the past four decades have witnessed an economic boost in China, and coastal ecosystems have encountered multiple stresses associated with economic development such as coastal eutrophication (Wang et al., 2018). The soil and coastal water have experienced eutrophic status in recent decades (Gao et al., 2018), and coastal nitrogen and phosphorus availabilities exhibit a large variation in the Shenzhen mangrove (Zhou et al., 2020; Supplementary Figure 4). It remains unclear whether eutrophic status in mangrove waters contributes to the exotic dominance of S. apetala and what environmental drivers contribute most to growth and reproduction in exotic species compared to native species.
To address this information gap, we collected litterfall in a subtropical mangrove forest in China monthly for 21 years (1999–2019), and we used the long-term litter dynamic as a potential surrogate for the final phase responses of different mangrove species to environmental perturbations. The present study had two major objectives: (1) to examine the relative importance of environmental drivers on the litterfall of exotic and native species in terms of leaves and reproductive parts; (2) to examine whether local nutrient enrichment (partly due to coastal eutrophication) drove the dissimilarity in litter biomass between exotic and native species. We hypothesized that leaf litterfall biomass in exotic species would covary with local nutrient availability, and the correlation would be stronger than that in native species (H1). In addition, we hypothesized that nutrient availability positively drove exotic species to produce more reproductive litterfall and increased the difference in the reproductive output between exotic and native species (H2).
2. Materials and methods
2.1. Study site
Our study site sits in the Guangdong Neilingding Futian National Nature Reserve (FNNR) in Shenzhen, southern China (22°31′ N, 114°05′ E). The FNNR is a Ramsar site located to the northeast of Shenzhen Bay (also named Deep Bay in Hong Kong), close to downtown Shenzhen and sharing water with Mai Po Nature Reserve, a Ramsar site in Hong Kong that hosts similar mangrove communities (Supplementary Figures 1A, B). Shenzhen Bay is a semi-enclosed bay with semi-diurnal tides. The climate in the FNNR is characterized as a subtropical monsoonal climate, with a mean annual temperature of 23.07°C and a total annual precipitation of 1,508 mm during the period of our study. The wet season in the FNNR begins in May and ends in September, in which period over 80% of the rain falls (Supplementary Figure 2).
The mangrove forest in the FNNR distributes along a 9-km coastline from east to west, occupying an area of approximately 100 ha. There are four tree species dominating the forest, namely, Kandelia obovata, Avicennia marina, S. apetala, and S. caseolaris. K. obovata and A. marina were naturally distributed in the FNNR (Li et al., 1992). S. apetala was first introduced to the Dongzhaigang mangrove of Hainan, at approximately 500-km south of the Shenzhen mangrove, in the 1980s from the Sundarbans mangrove (Zan et al., 2003), while S. caseolaris was a native species in China but naturally distributed only on Hainan Island. Two Sonneratia species were introduced to the FNNR from the Dongzhaigang mangrove in 1993 and 1995, respectively. These species grow well and form dense populations in the FNNR (Wang et al., 2002). Despite the fact that S. caseolaris is naturally distributed in China, we have defined the two Sonneratia species as exotic species to the FNNR in our study and K. obovata and A. marina as native species.
2.2. Litter collection
In 1999, we set up 12 traps in five stands that were dominated by single species with no vertical stratification (hereinafter referred to as “assemblages,” Supplementary Figure 1C) for litter collection. The assemblages of exotic S. apetala and S. caseolaris were planted in 1994 and 1996, respectively, referred as 28a Sa and 26a Sc. The forest ages of the native A. marina and K. obovata assemblages were assigned from documentations of the FNNR, namely, 83-aged A. marina (83a Am), and 28- and 83-aged K. obovata (28a/83a Ko). All traps were formed of 1.5 mm fiberglass mesh, measuring 1×1 m, and were tied to branches below the canopy of the tree at a height above the highest tidal level to prevent litter loss from tidal fluctuation.
Litter samples were harvested monthly from January 1999 to December 2019. During the sampling period, all traps were maintained in the same location, and in case of damage, the traps were replaced by a new trap at the same location in the immediate subsequent collection. The harvested mangrove litter for each trap was carefully sorted into leaves, twigs, and reproductive parts (including inflorescence, propagules, and fruits) and weighed separately after oven-drying at 80°C for 72 h. We used the biomass of leaves and reproductive organs from the harvested samples. Missing values in the biomass time series were filled with linear interpolation based on the trap and type of litter by using the “zoo” package (Zeileis and Grothendieck, 2005) in R (version 4.0.4) before further analysis.
2.3. Local environment
The time series of the environmental variables were obtained from the nearest available stations and downloaded from online databases provided by the Hong Kong Observatory (HKO) and Environmental Protection Department (HKEPD). Monthly mean air temperature and total rainfall were measured by the HKO at Lau Fau Shan (LFS weather station, 22°28′N, 113°59′E), and monthly mean sea level was calculated by the HKO from every 4-min measurement at Tsim Bei Tsui (TBT tide gauge, 22°29′N, 114°00′E). Both stations are managed by the HKO (Supplementary Figure 1B). Water salinity and nutrients were sampled at a fixed location near the center of Shenzhen Bay (DM2 site, at 2-metre depth, 22°30′N, 113°60′E) by the HKEPD monthly with a marine monitoring vessel. All environmental data were cleaned by filtering outliers and scaled to zero mean and unit variance for further analysis.
2.4. Statistical analysis
We examined the environmental contributions on the mangrove litterfall at monthly and annual scales. The relationships between the monthly litterfall and environmental variations can be non-linear and seasonal dependent, and we employed two algorithms of empirical dynamic modeling (EDM) to clarify whether the environmental driver was a potential cause of litterfall variation and to estimate its effect size on each mangrove assemblage across seasons. In addition to seasonal impacts, we also estimated environmental contributions on an annual basis using structural equation modeling (SEM) and redundancy analysis (RDA). The code used to perform these analyses and create figures is available at https://github.com/tkwongspace/FrontForGlobChange_2023.
2.4.1. Estimation of environmental effect size at monthly scale
We employed EDM to explore the contributions of each environmental driver to the mangrove litterfall of different assemblages. EDM was developed to handle the ubiquity of non-linearity and the diversity of environmental responses in natural ecosystems. It is free from fixed equations and statistical assumptions but relies on the reconstruction of state space by time-delay embedding based on Takens’ theorem (Takens, 1981). It interprets rules and associations of time series from their own history and can efficiently reduce the challenges in recognizing relationships and forecast dynamics in natural ecosystems (Chang et al., 2017). We first investigated which environmental drivers determined litterfall in exotic and native species using convergent cross mapping (CCM, Sugihara et al., 2012), and we subsequently created different scenarios based on optimal models using an S-map (sequential locally weighted global linear map, Sugihara, 1994) to estimate the effect size of the target driver on the mangrove litterfall.
Temporal contributions of the environment were demonstrated by empirical associations between the driver and litter biomass, i.e., the cross-prediction skill in CCM. The algorithm has been efficiently used for distinguishing associations in the natural world (e.g., van Nes et al., 2015). CCM identifies causal relationships by searching for cross-prediction skills in different sampling sizes, and a causal association between the litterfall and environmental driver was demonstrated by a monotonic increase with the sampling size (sensu convergence, Sugihara et al., 2012). The time series of the trap-specific litter biomass was converted via first-order difference to ensure stationarity before model construction. Meanwhile, we standardized the time series of each environmental driver by removing the mean and converting to unit variance. We constructed CCM for the first-order differenced time series of the litterfall in each assemblage against each driver with the “rEDM” package (version 1.9.2, Park et al., 2021) in R and used the Kendall’s τ to test the significance of a monotonic increase in CCM cross-mapping skills. To avoid false detection of causal links due to common temporal confounders and to address CCM sensitivity to periodic fluctuations, we compared cross-mapping skills with two null model performances generated with the surrogate time series: the seasonal null model preserves the seasonal signal while randomizing the interannual anomalies (Deyle et al., 2016), while the stricter Ebisuzaki null model conserves any periodicity (beyond seasonality) and randomizes the phases of Fourier-transformed time series (Ebisuzaki, 1997).
To estimate the effect size of different drivers on litter variations across assemblages, we employed the S-map algorithm for scenario exploration. The S-map calculates linear regression on a set of weighted points in the state space. The weights of each point were determined by Euclidean distance and a localization parameter to distinguish non-linearity in empirical relationships and to improve model forecast ability (Chang et al., 2017). We built a univariate S-map model for each assemblage with a different driver using the “rEDM” package, with the first 179 months (i.e., time range between February 1999 and December 2013) as the training set and the remainder as the testing set.
Subsequent to the model construction, we created a modified environmental driver scenario to estimate the effect size following the method of Nova et al. (2020). First, we modified the values of the single-target driver in the testing set by adding or subtracting a small value to its observation while maintaining the other drivers unchanged. The modification of the target driver X at time t is described as:
where and Xt denote the modified and observed value of X at time t, respectively, and ΔX is equal to 10% of the standardized deviation of the full-time series of X, i.e., ΔX=0.1σX. Second, the value of the litter time series at time t was estimated with the optimal S-map model. The effect estimate (EE) of the driver X at time t is defined as:
where denotes the predicted biomass difference between month t and t-1 (due to first-order difference on litter time series) under conditions of modified or original Xt together with values of other drivers Et. The positive or negative sign of ΔX was the same as that in the X modification (Equation 1). We summarized the mean and 95% confidence interval of EE across all time locations for the effect size of the target driver.
2.4.2. Estimation of environmental effect size at annual scale
We employed SEM for path analysis to quantify the direct and indirect effects among the different environmental drivers on the mangrove litterfall on an annual basis. SEM is a popular method that has been adapted to various ecological disciplines such as ecological communities (Chapin et al., 2016) and life history strategies (Vile et al., 2006). SEM allows us to efficiently clarify and interpret complex causal connections among multiple predictors and responses (Grace et al., 2010). We built an a priori hypothetical model based on ecological knowledge and our understanding of the relationships among the environmental drivers and their potential influence on the mangrove litterfall (Supplementary Figure 3). We assumed that the output of the annual litterfall in the mangrove assemblages can be directly influenced by all the environmental drivers (i.e., mean air temperature, annual precipitation, mean sea level, mean salinity, mean coastal nitrogen, and phosphorus availability) and that local predictors (coastal salinity and nutrients) can be influenced by climatic variables (temperature, rainfall, and sea level).
Prior to fitting the SEM, we examined the relationships between the environmental proxies (Supplementary Figure 10) and removed bivariate correlations higher than 0.85 (Kline, 2005). We subsequently linearized the relationships by taking the natural logarithm of the annual litterfall biomass and then standardizing and centering the data of both the biomass and environment. SEM models were fit using the “lavaan” package in R (Rosseel, 2012). We first simplified the initial model by removing predictor variables that had no significant effect (i.e., variables with p > 0.05) on litterfall biomass and then added missing paths one at a time back to the model. The importance of the missing path was estimated with the modification indices and based on their ecological sense (Grace et al., 2012). The optimal SEM model was selected based on a chi-square test, the root mean square error of approximation (RMSEA), the comparative fit index (CFI), and the standardized root mean square residual (Grace et al., 2012; Weston and Gore, 2016). We additionally built RDA models for the annual output of leaf and reproductive litterfall across all assemblages with extra environmental proxies in addition to the annual mean for a comprehensive illustration. The RDA models were established using the “vegan” package (version 2.6-4, Oksanen et al., 2022) in R, and the optimal structure of RDA model was selected with forward selection procedures based on the adjusted R-squared (Supplementary Tables 4, 5).
3. Results
3.1. Temporal variations of litter biomass and environment
We found strong seasonality in the mangrove litterfall (Figure 2 and Supplementary Figure 5) and environment (Supplementary Figure 2) with different trends across the 20 years (Figure 1 and Supplementary Figure 4). Phenophases in terms of litterfall were distinct among the species. The biomass of the leaf and reproductive litter in native 83a A. marina changed synchronously, while phase shifts were apparent in the exotic species and native K. obovata of both age classes (Supplementary Figure 6). Exotic 28a S. apetala and 26a S. caseolaris increased the leaf litter biomass before the rise of the reproductive litter, while both age classes of native K. obovata exhibited a reversed order (Figure 2).
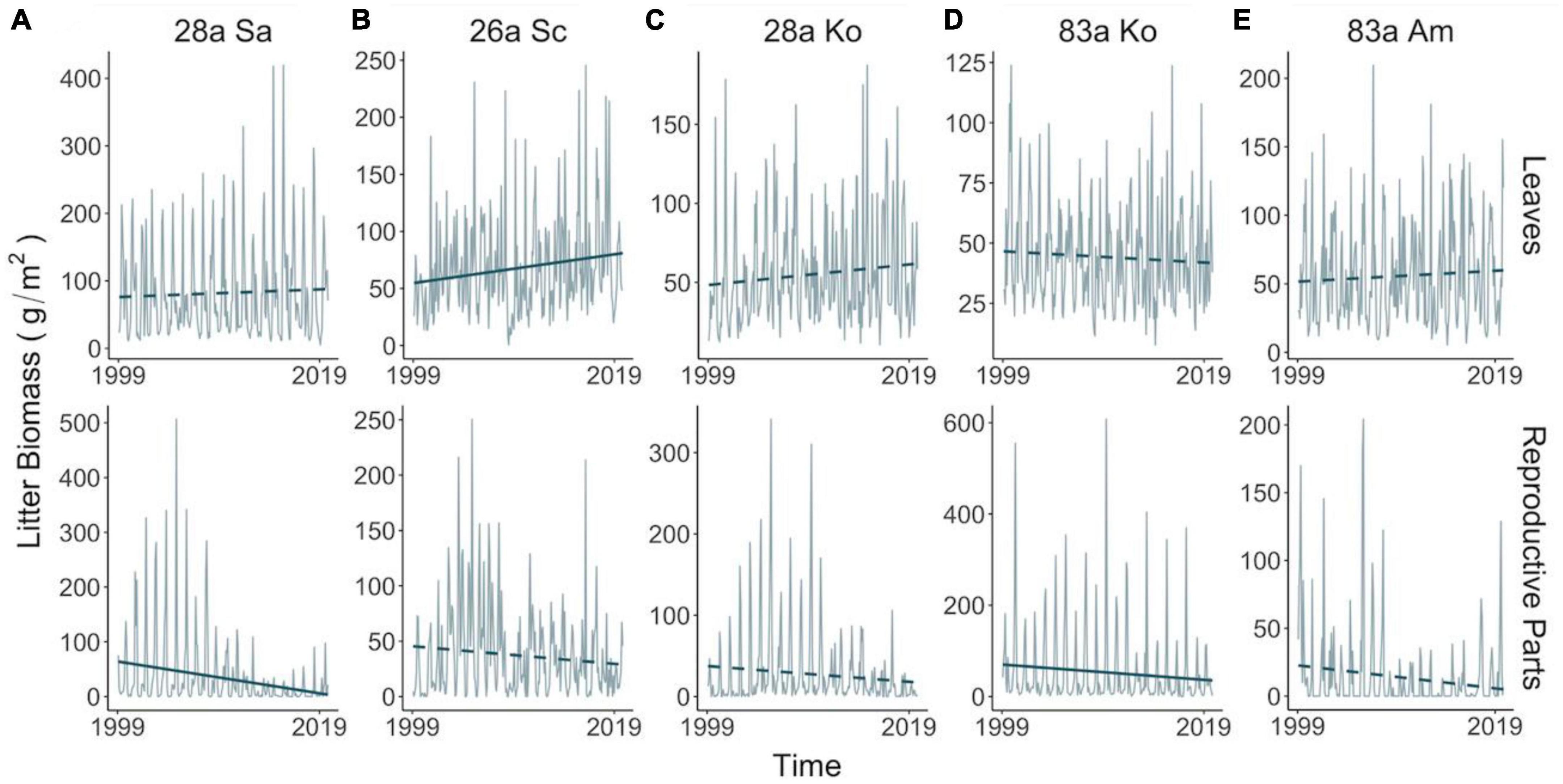
Figure 1. Temporal variations in litter biomass of leaves (upper panel) and reproductive parts (lower panel) in exotic (A,B) and native (C–E) assemblages in 1999–2019. Lines and bars in gray illustrate monthly mean and standardized error, respectively, of litter mass harvested from all trap replicates. Blue lines demonstrate temporal trend of litter mass in the two decades, with solid or dashed type denoting significance (at 0.05 level). Temporal trend and associated significance were calculated based on a 36-month-long moving mean and a linear mixed-effect model with a within-group autoregressive process of order 1 (i.e., AR1) correction structure for each assemblage and litter type. Species: Sa, S. apetala; Sc, S. caseolaris; Ko, K. obovata; Am, A. marina.
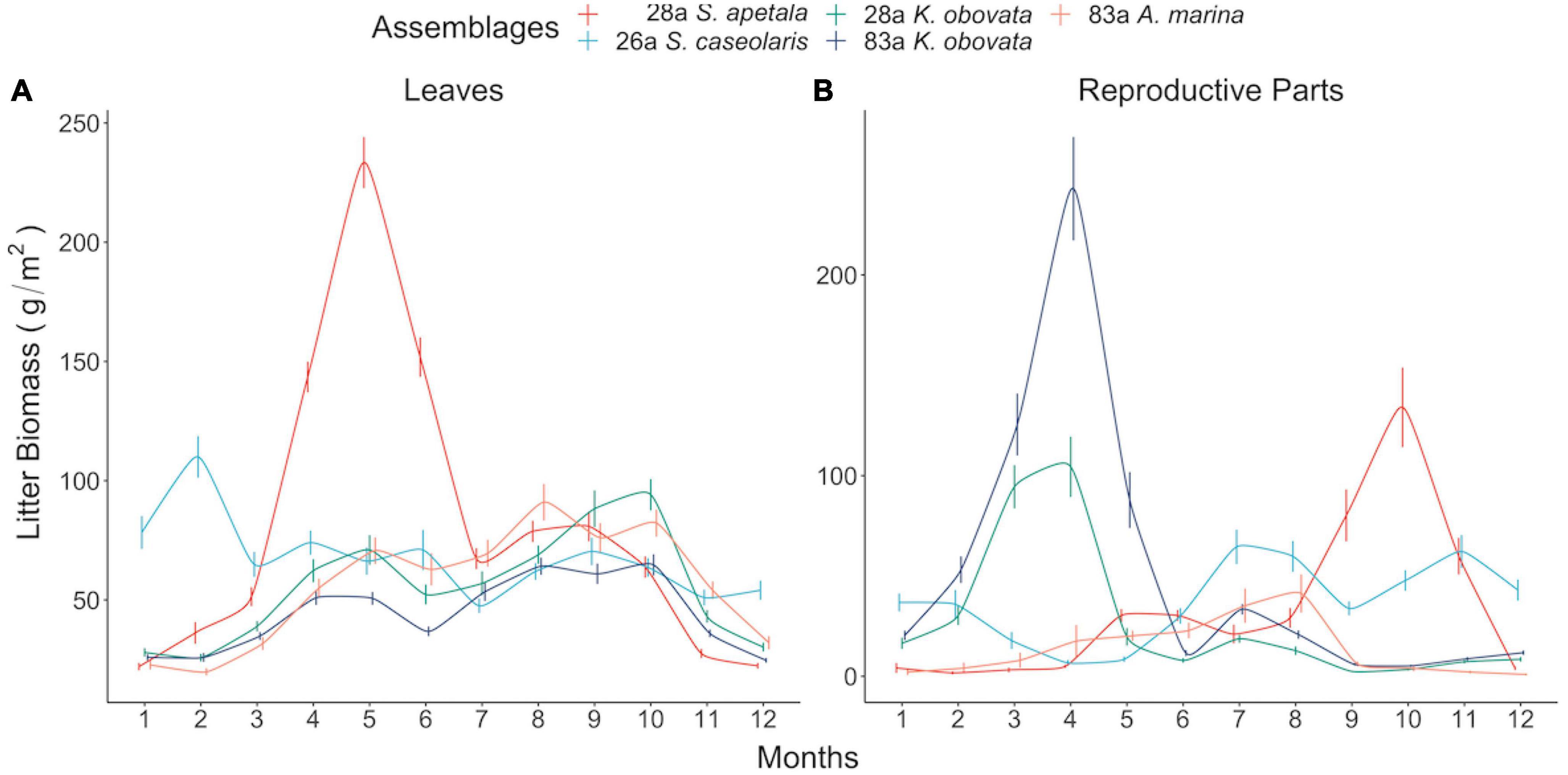
Figure 2. Seasonal pattern of leaf (A) and reproductive parts (B) litterfall biomass of different assemblages. Each point and associated bar denote two-decade mean and standard error in corresponding month.
The leaf litter exhibited no temporal trend in the native species nor in exotic 28a S. apetala, while the leaf litter biomass of exotic 26a S. caseolaris was rising (Figures 1A, B). From a seasonality perspective, native 83a A. marina and both age classes of K. obovata displayed a comparable pattern of leaf litter variations, with increments in spring and summer leading to a peak around August (Figure 2A and Supplementary Figures 6C–E). However, exotic 28a S. apetala exhibited a dramatic gain in leaf litter in spring (March to May) and exotic 26a S. caseolaris in winter (December to February, Figure 2A). The substantial spring increments in the leaf litter of the exotic species may explain the larger amount of annual leaf litter biomass in the exotic species compared to the native species (Figure 3A).
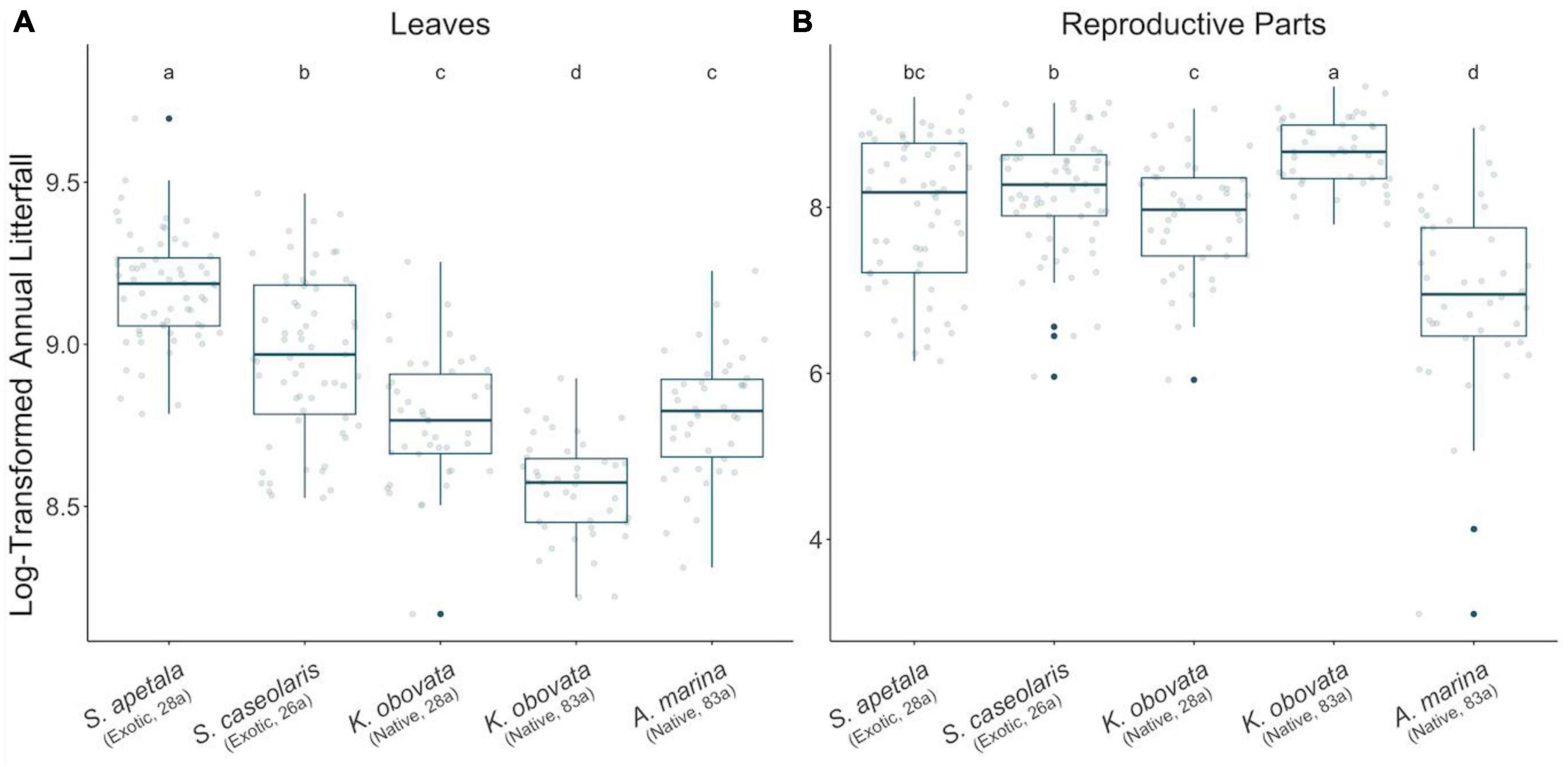
Figure 3. Annual litter biomass of leaves (A) and reproductive parts (B) in the two decades. All values were measured in unit of g/m2 and log transformed. Points in gray illustrate annual mass of litter for each year while blue solid points demonstrate outliers. A thickened line within the box marks the mean, and the lower and upper hinges of each box correspond to the first and third quantiles (i.e., the 25th and 75th percentiles). Whiskers illustrate 1.5 times inter-quartile range (IQR, the distance between the first and third quartiles). Letters above each box indicate the significance of between-group differences by Welch’s ANOVA (at 0.05 level).
As for the reproductive litter, exotic 28a S. apetala and native 83a K. obovata both presented a decreasing trend in the litter biomass of reproductive parts along the two decades (Figure 1). Nevertheless, the reproductive litter biomass was the highest annually in native 83a K. obovata (Supplementary Figure 4B) due to a substantial rise in the reproductive biomass during the reproductive phase (Figure 4B and Supplementary Figures 5, 6). The reproductive litter of exotic 28a S. apetala mostly occurred in September (Figure 2A and Supplementary Figures 5, 6), while exotic 26a S. caseolaris exhibited a bimodal pattern in the reproductive litter biomass that climbed upward in both summer and late autumn each year (Figure 2A and Supplementary Figures 5, 6). Native 83a A. marina presented a relatively small variation of the reproductive litter across months, and its biomass always peaked in the summer (Figure 2B and Supplementary Figures 5, 6).
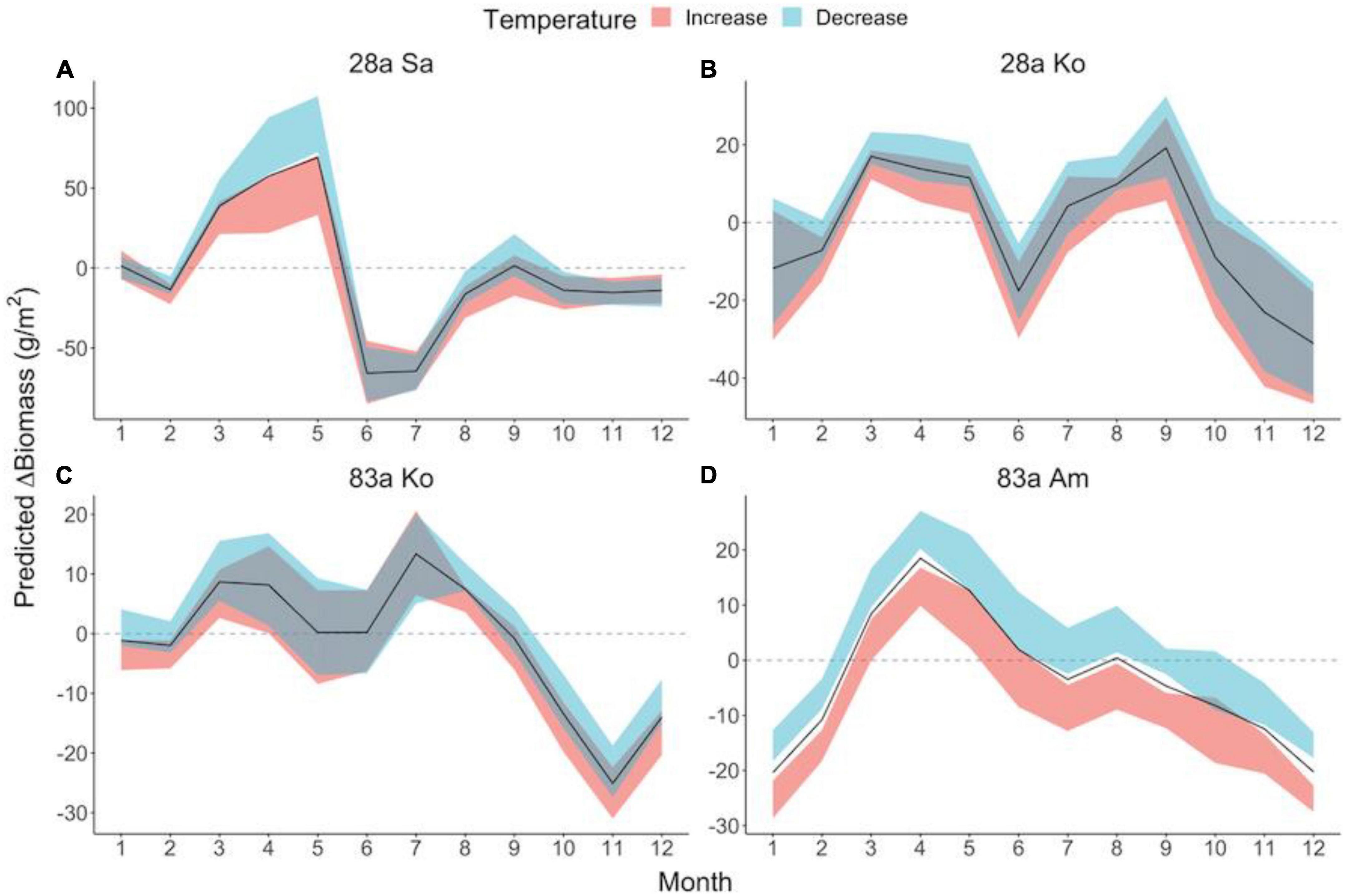
Figure 4. Seasonal patterns of temperature effect sizes on mangrove leaf litter biomass in exotic (A) and native (B–D) assemblages. Predicted ΔBiomass demonstrates the biomass increment from the previous month under given thermal conditions, measuring the effect size of modified temperature. Solid lines denote predicted ΔBiomass of leaf litter under observed temperature. Ribbons filled in red and blue denote 95% confidence interval of predicted ΔBiomass in the context of increased and decreased temperature, respectively. The dashed line in gray illustrates zero ΔBiomass compared to the previous month. Mangrove species: Sa, S. apetala; Ko, K. obovata; Am, A. marina.
The seasonality of rainfall shapes the dry and wet seasons in the FNNR (Supplementary Figure 2). The temperature varied in the same pace with rainfall, while coastal water salinity dropped in the wet seasons and climbed up in the dry seasons. However, the monthly mean sea level and nutrient availability did not follow the alternation of dry–wet seasons. The mean sea level peaked in October after a climb from its lowest point in April but dropped quickly when winter came, while coastal nitrogen and phosphorus presented a bimodal pattern with two peaks in mid-summer (June to July) and mid-winter (December to February).
3.2. Environmental drivers on monthly variations in litter biomass
Temperature, rainfall, sea level, and coastal salinity exerted significant impacts on leaf litter change in both the exotic and native species (Table 1), but the effect sizes on each assemblage were season dependent (Supplementary Table 1). The leaf litter biomass of all the mangrove assemblages, except 26a S. caseolaris, exhibited a high dependence on temperature, rainfall, and coastal salinity, with exotic 28a S. apetala presenting the highest correlation with these environmental variations (Table 1). Temperature generally exerted a negative influence on the monthly ΔBiomass of the leaf litter in exotic 28a S. apetala and the native assemblages (Figure 4A and Supplementary Figure 7A). Sea level rise generally facilitated a monthly increment in the leaf litter biomass of exotic 26a S. caseolaris but also promoted spring leaf litterfall in exotic 28a S. apetala, whereas it inhibited the leaf litter in native 83a K. obovata (Supplementary Figure 7A and Supplementary Table 1). The leaf litter ΔBiomass of exotic 28a S. apetala positively responded to rising salinity, while native 83a A. marina and 83a K. obovata were negatively influenced (Supplementary Figure 7A and Supplementary Table 1). Coastal nitrogen availability was a key driver promoting monthly ΔBiomass in the leaf litter of native 28a K. obovata, but we found no significant correlations between the mangrove leaf litter variations and coastal phosphorus availability (Table 1 and Supplementary Figure 7A).
The result of CCM highlighted that the exotic and native species used different environmental information as cues for reproductive litterfall production (Table 1). The monthly ΔBiomass of the native species was tightly linked to climatic drivers (i.e., temperature and rainfall) and coastal salinity. Of the three stands dominated by the native species (i.e., 83a A. marina and 28a and 83a K. obovata), temperature exhibited the strongest but most complex impacts on the litter ΔBiomass compared to other drivers (Table 1 and Supplementary Table 2). However, the reproductive output of exotic 28a S. apetala exhibited resilience to temperature but was more attuned to coastal nutrient availability. Coastal nitrogen and phosphorus determined the monthly ΔBiomass of the reproductive litter in exotic 28a S. apetala but also in both native 28a and 83a K. obovata (Table 1), the impacts of which were complex (Supplementary Figure 8). Enrichment of nitrogen appeared to exhibit a negative influence on native 83a K. obovata (Supplementary Figure 7B and Supplementary Table 2). A rise in coastal salinity may negatively impact the reproductive litter variations in exotic 26a S. caseolaris and in native 28a and 83a K. obovata, but it appeared to facilitate monthly ΔBiomass of reproduction in 83a A. marina across months (Supplementary Figure 5B and Supplementary Table 2).
3.3. Annual-scale environmental contributions
On an annual basis, the mangrove litterfall presented a balance across years, while reproductive output appeared to decrease in the two decades (Supplementary Figure 9). Climatic variables (i.e., temperature, rainfall, and sea level) exhibited strong influence on local drivers (i.e., coastal salinity and nitrogen) and exerted direct and indirect impacts on the mangrove litterfall (Figure 5).
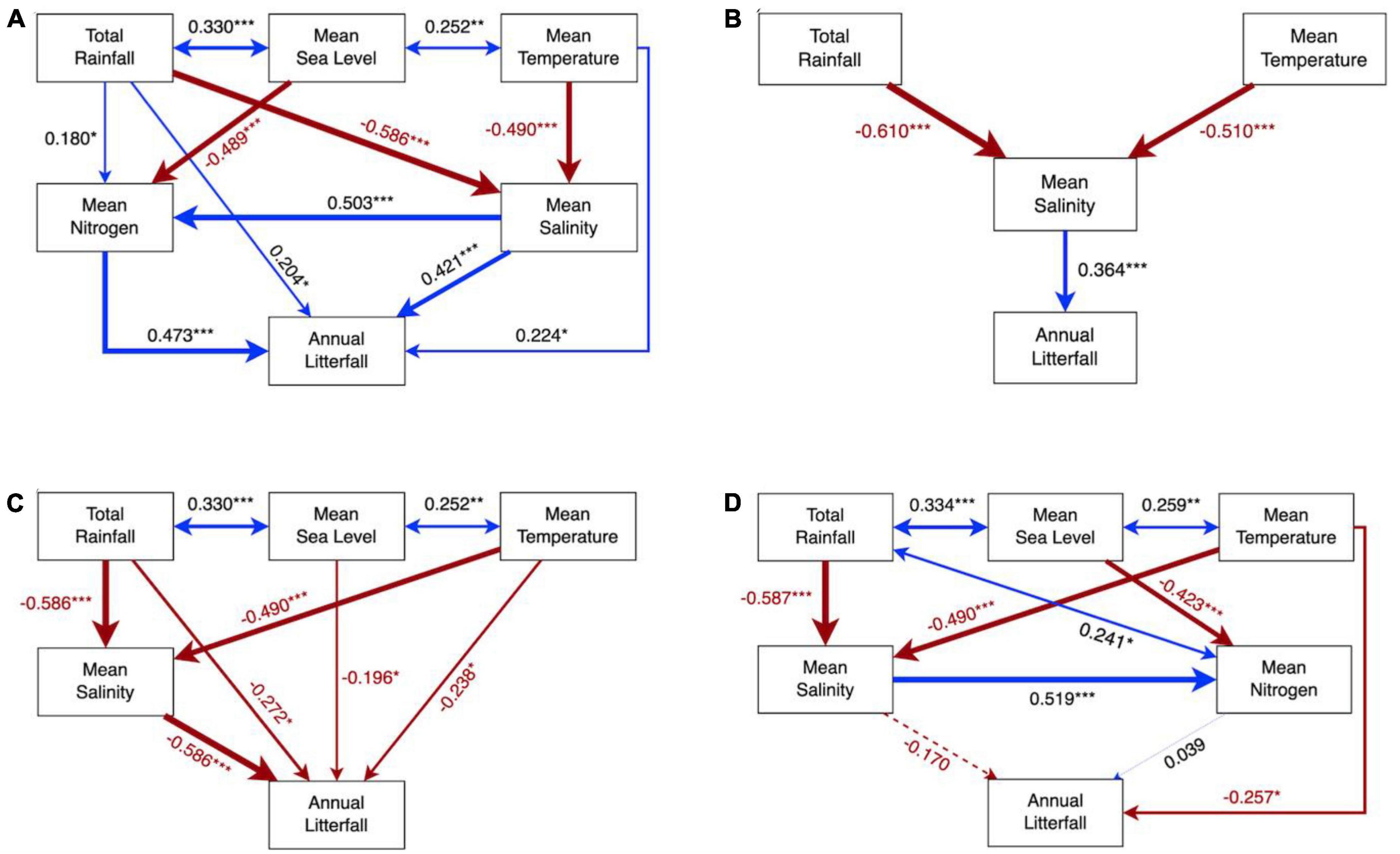
Figure 5. Structural equation model of environmental contributions on mangrove litterfall of reproductive parts (A,B) and leaves (C,D) in exotic (left panel) and native assemblages (right panel). Numbers adjacent to arrows are standardized path coefficients, analogous to relative regression weights, and indicative of the effect size of the relationship. Continuous and dashed arrows indicate significant and non-significant relationships, respectively. Positive correlations were demonstrated by arrows in blue, while negative correlations are in red. Arrow width is proportional to the strength of the relationship. *P < 0.05, ** P < 0.01, and *** P < 0.001.
The SEM models of reproductive output supported our hypothesis by showing that the reproductive output of exotic species was positively linked with multiple drivers, among which mean coastal nitrogen availability exhibited the strongest influence, while native species only directly correlated with coastal salinity (Figures 5A, B). More specifically, salinity and nitrogen explained more than half of the variance in the reproductive output of the exotic species compared to temperature and rainfall (Figure 5A and Table 2), while the reproductive litterfall in the native species was mainly altered by coastal salinity together with indirect impacts from temperature and rainfall (Figure 5B and Table 2). In addition, temperature and rainfall exerted positive direct impacts but negative indirect impacts on the exotic species, resulting in non-significant total impacts on the reproductive litterfall of the exotic assemblages (Table 2). The optimal RDA model of the reproductive litterfall also suggested that local drivers (i.e., coastal salinity and nutrients) exerted significant impacts compared to climatic factors on inter-annual variations in the mangrove reproductive biomass (Supplementary Figure 11A and Supplementary Table 3). Mean coastal salinity and nitrogen availability contributed highly to the annual biomass of the reproductive litter in the exotic assemblages, particularly 28a S. apetala (Supplementary Figure 11A). Our RDA model also suggested that two 83a native assemblages were less vulnerable to environmental variations in terms of the reproductive litterfall compared to 28a K. obovata and the exotic species (Supplementary Figure 11A).
Our SEM model highlighted that the leaf litterfall of exotic 28a S. apetala and 26a S. caseolaris was linked to multiple drivers including temperature, rainfall, sea level, and salinity, all of which exerted direct and negative impacts (Figure 5C). However, the indirect impacts of temperature and rainfall were positive on the leaf litterfall of the exotic assemblages, leading to non-significant total impacts (Table 2). Contrary to the exotic species, which linked the leaf litterfall to multiple drivers, the leaf litterfall of the native assemblages was only significantly correlated with the impacts of temperature (Figure 5D and Table 2). The optimal RDA model of the leaf litterfall highlighted that minimum sea level, maximum salinity, and mean nitrogen availability in coastal water significantly determined the annual leaf litterfall biomass in mangrove assemblages (Supplementary Table 3). Exotic 26a S. caseolaris and native 28a K. obovata appeared to respond comparably to salinity and sea level changes, while the leaf litter biomass of exotic 28a S. apetala was likely more sensitive to mean coastal nitrogen availability (Supplementary Figure 11B).
4. Discussion
Understanding environmental contributions to mangrove litterfall can enhance our ability to predict ecosystem response and to anticipate potential risks in the context of climate change. Our study highlighted that temperature was the most important driver of monthly leaf litterfall and exerted an overall negative impact on mangrove species. The leaf litter in the exotic species, particularly 28a S. apetala, linked more tightly with climatic variations than the native species in the FNNR. As for the reproductive output, coastal nitrogen significantly drove the reproductive litterfall in exotic 28a S. apetala and native K. obovata of both age 28 and 83, while coastal nitrogen positively correlated with the reproductive output in the exotic species but not in the native species, which can further increase the quantity difference between the exotic and native species in the reproductive litter biomass. We implied that the enrichment of coastal nutrients in recent decades appeared to be an important contributor to the current exotic dominance in the mangrove forests of China.
The results of the CCM and S-map models in our study suggested a strong impact of temperature on the leaf litterfall in both the exotic and native species (Table 1). This is not surprising because many physiological activities such as photosynthesis and leaf senescence in mangrove trees are highly determined by temperature (Aspinwall et al., 2021). The timing of leaf senescence has long been considered as an adaptive behavior to optimize carbon gain (Wright et al., 2005), and it varies in importance according to environmental cues such as temperature (Körner and Basler, 2010). In our study site of Shenzhen Bay, temperature always lay within a range suitable for mangrove growth and reproduction, and a warmer month offered these trees a more preferable condition to budget more leaves in the canopy, which may lead to a decreased leaf litterfall biomass. The magnitude of change in the monthly ΔBiomass of the leaf litterfall against temperature likely reflected the canopy plasticity of the species. Furthermore, the effect size of the temperature varied across seasons, and we noted that temperature exhibited little influence on the S. apetala leaf litterfall in the summer (Figure 4), corresponding to the cyclone season of the Pacific. We supposed that wind, particularly in storms and cyclones, is also a strong factor in shaping mangrove canopy, and its influence may exceed that of temperature on mangrove litterfall; however, this requires further study. Nevertheless, our results highlighted that the higher responsive S. apetala may be more capable of exploiting environmental cues and gaining increased fitness in the context of climate warming (Cleland et al., 2012).
The difference between exotic and native species in determinants of the reproductive litter demonstrated that species are influenced and may respond differently in future climate change (Figure 5 and Tables 1, 2). Mangrove species have developed a tight coupling of tree physiology with nutrient availability due to the volatile environment in the intertidal area, and the response to nutrient availability can reveal intrinsic differences among species (Reef et al., 2010). The complex consequence of additional nutrients on the monthly ΔBiomass of the reproductive litter (Supplementary Figure 8) reflects an undetectable effect of nutrient enrichment at a short time scale (Wong et al., 1995), but at the annual scale, we suggested a stronger coupling between coastal nitrogen and reproductive output in the exotic species compared to the native species (Figures 5A, B and Table 2), particularly for 28a S. apetala (Supplementary Figure 11A). One of the reasons may be the high demand for nutrients in S. apetala reproduction, as it appeared to allocate more nutrients to reproductive organs compared to the native species in the FNNR (Zan et al., 2002), and it also exhibited a high rate of fruit abortion (Aluri, 2022). A quick response to capitalize additional nutrients for reproductive output, similar to the example of A. germinans (black mangrove) in Florida mangroves (Dangremond et al., 2020), may allow exotic S. apetala to compete in established mangrove forests for seedling establishment (Gross, 1984) and range expansion (Hong et al., 2021). However, we found large differences between the two exotic species S. apetala and S. caseolaris in their trend and seasonality of litterfall production, which corresponded to previous studies that demonstrated a contrast between the two species in environmental sensitivity (Nguyen et al., 2021). Our environmental proxies explained the limited variations in the S. caseolaris leaf or reproductive litterfall at both monthly and annual scales, suggesting they could be determined by other unmeasured variables, e.g., local evaporation (Chen et al., 2009) and biological interactions with benthic fauna (Cannicci et al., 2008). We expect more empirical studies that can improve our understanding of the physiological mechanisms of litterfall responses in mangrove species.
Our SEM models indicated that the exotic species appeared to have more reliable environmental cues for leaf and reproductive litter output compared to the native species (Figure 5). The existence of multiple cues is a core prerequisite for adaptive predictive plasticity of a species to anticipate future selection by modifying the expression of specific traits (Bonamour et al., 2019). As we demonstrated hereinabove, exotic S. apetala exhibited a high climatic sensitivity in the monthly leaf litterfall (Table 1), while the reproductive output of this species was resilient to climatic variations but rather positively correlated with coastal nutrients (Supplementary Figure 11 and Tables 1, 2). As such, exotic S. apetala appeared to have a stronger capability than the native species to exploit environmental cues and gain fitness when confronted with coastal eutrophication or global warming. In the case of the coastal mangroves that lie adjacent to urban areas, which are often seen in Asia (Branoff, 2017), these forests likely experience the coupled challenges of climate change and coastal pollution. In this context, S. apetala was likely preferred and allowed to thrive in these mangrove forests, as has occurred along China’s coastline in recent decades. It is noteworthy that, due to actions of pollution control by governments to clean up and maintain the assimilative capacity of the coastal water in Shenzhen Bay (HKEPD, 2007), nitrogen and phosphorus loads in this area have been decreasing in recent decades (Supplementary Figure 4) and may contribute to the substantial decrease of the S. apetala reproductive litterfall (Figure 1). We recommend more empirical research to further understand how exotic S. apetala may respond to the novel condition and whether the nutrient-induced growth and reproduction in exotic species can benefit native species.
Mangrove rehabilitation is a strategy that is often employed to combat the substantial loss of mangrove forests, and mangrove planting is the dominant strategy among projects around the world (Gatt et al., 2022). Great efforts have been taken around the world to restore mangrove forests, but most have been plagued by the poor matching of sites and species (Gatt et al., 2022). This is mainly due to a limited number of species candidates for restoration projects when constrained to the native species pool and the strict criteria in the selection of planting species that require careful evaluation to avoid potential loss in biodiversity and ecosystem services. In our case, the high environmental sensitivity of S. apetala can help with the success of efficient afforestation and soil properties modification for the recolonization of faunal and native tree species (Ren et al., 2010), but the benefits versus the costs of the transfer of exotic species require longer-term evaluation of their influence on local biodiversity and native species (Davidson et al., 2018). Our study emphasized that empirical investigations on the litterfall response to different environmental drivers can hint at context preference and tolerance in different species, which may benefit forest management and species selection in ecological restoration projects.
In summary, we revealed the contrasts in the key drivers on leaf and reproductive litterfall between exotic and native mangrove species and highlighted that exotic S. apetala exhibited a high climatic sensitivity in leaf litter production but a climate-resilient reproductive output, which positively linked with coastal nutrients. On the contrary, the litterfall of leaves and reproductive parts in the native species were more responsive to climatic variations and were mainly determined by temperature. The enrichment of coastal nitrogen and phosphorus appeared to promote reproductive output in exotic S. apetala and further increase the quantity difference in the reproductive litterfall biomass between the exotic and native species. We imply that nutrient enrichment in recent decades may be an important contributor to the exotic dominance in China’s coastal mangrove forests.
Data availability statement
The raw data supporting the conclusions of this article will be made available by the authors, without undue reservation.
Author contributions
ZH and SY designed the study. ZH, ZL, and DZ collected the data in the field. ZH performed the statistical analyses and wrote the first draft. MH and SY contributed to the revisions. All authors contributed to the article and approved the submitted version.
Funding
This research was financially supported by the Shenzhen Science and Technology Innovation Program (Project no. JCYJ20180504170040910) and National Natural Science Foundation of China (Grant 31770513).
Acknowledgments
We are grateful to Zhengjiao Liu and other colleagues for their assistance in the field survey data collection.
Conflict of interest
The authors declare that the research was conducted in the absence of any commercial or financial relationships that could be construed as a potential conflict of interest.
Publisher’s note
All claims expressed in this article are solely those of the authors and do not necessarily represent those of their affiliated organizations, or those of the publisher, the editors and the reviewers. Any product that may be evaluated in this article, or claim that may be made by its manufacturer, is not guaranteed or endorsed by the publisher.
Supplementary material
The Supplementary Material for this article can be found online at: https://www.frontiersin.org/articles/10.3389/ffgc.2023.1183432/full#supplementary-material
References
Aluri, J. S. R. (2022). “A review of the reproductive ecology of mangrove plant species,” in Mangroves: Biodiversity, livelihoods and conservation, eds S. C. Das Pullaiah and E. C. Ashton (Singapore: Springer), 33–70.
Aspinwall, M. J., Faciane, M., Harris, K., O’Toole, M., Neece, A., Jerome, V., et al. (2021). Salinity has little effect on photosynthetic and respiratory responses to seasonal temperature changes in black mangrove (Avicennia germinans) seedlings. Tree Physiol. 41, 103–118. doi: 10.1093/treephys/tpaa107
Bernhardt, J. R., O’Connor, M. I., Sunday, J. M., and Gonzalez, A. (2020). Life in fluctuating environments. Philos. Trans. R. Soc. B Biol. Sci. 375:20190454. doi: 10.1098/rstb.2019.0454
Biswas, S. R., Biswas, P. L., Limon, S. H., Yan, E.-R., Xu, M.-S., and Khan, M. S. I. (2018). Plant invasion in mangrove forests worldwide. For. Ecol. Manag. 429, 480–492. doi: 10.1016/j.foreco.2018.07.046
Biswas, S. R., Khan, M. S. I., and Mallik, A. U. (2012). Invaders’ control on post-disturbance succession in coastal mangroves. J. Plant Ecol. 5, 157–166. doi: 10.1093/jpe/rtr050
Bonamour, S., Chevin, L.-M., Charmantier, A., and Teplitsky, C. (2019). Phenotypic plasticity in response to climate change: The importance of cue variation. Philos. Trans. R. Soc. B Biol. Sci. 374:20180178. doi: 10.1098/rstb.2018.0178
Branoff, B. (2018). “Urban mangrove biology and ecology: Emergent patterns and management implications,” in Threats to Mangrove Forests, 1 Edn, eds C. Makowski and C. W. Finkl (New York, NY: Springer), 521–537.
Branoff, B. L. (2017). Quantifying the influence of urban land use on mangrove biology and ecology: A meta-analysis. Glob. Ecol. Biogeogr. 26, 1339–1356. doi: 10.1111/geb.12638
Cannicci, S., Burrows, D., Fratini, S., Smith, T. J., Offenberg, J., and Dahdouh-Guebas, F. (2008). Faunal impact on vegetation structure and ecosystem function in mangrove forests: A review. Aquat. Bot. 89, 186–200. doi: 10.1016/j.aquabot.2008.01.009
Chang, C.-W., Ushio, M., and Hsieh, C. (2017). Empirical dynamic modeling for beginners. Ecol. Res. 32, 785–796. doi: 10.1007/s11284-017-1469-9
Chapin, F. S., Conway, A. J., Johnstone, J. F., Hollingsworth, T. N., and Hollingsworth, J. (2016). Absence of net long-term successional facilitation by alder in a boreal Alaska floodplain. Ecology 97, 2986–2997. doi: 10.1002/ecy.1529
Chen, L., Zan, Q., Li, M., Shen, J., and Liao, W. (2009). Litter dynamics and forest structure of the introduced Sonneratia caseolaris mangrove forest in Shenzhen China. Estuar. Coast. Shelf Sci. 85, 241–246. doi: 10.1016/j.ecss.2009.08.007
Cherif, M., and Loreau, M. (2008). When microbes and consumers determine the limiting nutrient of autotrophs: A theoretical analysis. Proc. R. Soc. B Biol. Sci. 276, 487–497. doi: 10.1098/rspb.2008.0560
Cleland, E. E., Allen, J. M., Crimmins, T. M., Dunne, J. A., Pau, S., Travers, S. E., et al. (2012). Phenological tracking enables positive species responses to climate change. Ecology 93, 1765–1771. doi: 10.1890/11-1912.1
Daehler, C. C. (2003). Performance comparisons of co-occurring native and alien invasive plants: Implications for conservation and restoration. Annu. Rev. Ecol. Evol. Syst. 34, 183–211. doi: 10.1146/annurev.ecolsys.34.011802.132403
Dangremond, E. M., Simpson, L. T., Osborne, T. Z., and Feller, I. C. (2020). Nitrogen enrichment accelerates mangrove range expansion in the temperate–tropical ecotone. Ecosystems 23, 703–714. doi: 10.1007/s10021-019-00441-2
Davidson, I. C., Cott, G. M., Devaney, J. L., and Simkanin, C. (2018). Differential effects of biological invasions on coastal blue carbon: A global review and meta-analysis. Global Change Biol. 24, 5218–5230. doi: 10.1111/gcb.14426
Deyle, E. R., Maher, M. C., Hernandez, R. D., Basu, S., and Sugihara, G. (2016). Global environmental drivers of influenza. Proc. Natl. Acad. Sci. U.S.A. 113, 13081–13086. doi: 10.1073/pnas.1607747113
Duke, N. C. (1990). Phenological trends with latitude in the mangrove tree Avicennia marina. J. Ecol. 78, 113–133. doi: 10.2307/2261040
Ebisuzaki, W. (1997). A method to estimate the statistical significance of a correlation when the data are serially correlated. J. Climate 10, 2147–2153.
Eppinga, M. B., Kaproth, M. A., Collins, A. R., and Molofsky, J. (2011). Litter feedbacks, evolutionary change and exotic plant invasion. J. Ecol. 99, 503–514. doi: 10.1111/j.1365-2745.2010.01781.x
Feller, I. C., Dangremond, E. M., Devlin, D. J., Lovelock, C. E., Proffitt, C. E., and Rodriguez, W. (2015). Nutrient enrichment intensifies hurricane impact in scrub mangrove ecosystems in the Indian River Lagoon, Florida, USA. Ecology 96, 2960–2972. doi: 10.1890/14-1853.1
Friess, D. A., Rogers, K., Lovelock, C. E., Krauss, K. W., Hamilton, S. E., Lee, S. Y., et al. (2019). The state of the World’s mangrove forests: Past, present, and future. Annu. Rev. Environ. Resour. 44, 89–115. doi: 10.1146/annurev-environ-101718-033302
Funk, J. L. (2008). Differences in plasticity between invasive and native plants from a low resource environment. J. Ecol. 96, 1162–1173. doi: 10.1111/j.1365-2745.2008.01435.x
Funk, J. L., and Vitousek, P. M. (2007). Resource-use efficiency and plant invasion in low-resource systems. Nature 446, 1079–1081. doi: 10.1038/nature05719
Gao, Y., Sun, L., Wu, C., Chen, Y., Xu, H., Chen, C., et al. (2018). Inter-annual and seasonal variations of phytoplankton community and its relation to water pollution in Futian Mangrove of Shenzhen China. Continent. Shelf Res. 166, 138–147. doi: 10.1016/j.csr.2018.07.010
Gatt, Y. M., Andradi-Brown, D. A., Ahmadia, G. N., Martin, P. A., Sutherland, W. J., Spalding, M. D., et al. (2022). Quantifying the reporting, coverage and consistency of key indicators in mangrove restoration projects. Front. For. Glob. Change 5:720394. doi: 10.3389/ffgc.2022.720394
Grace, J. B., Anderson, T. M., Olff, H., and Scheiner, S. M. (2010). On the specification of structural equation models for ecological systems. Ecol. Monogr. 80, 67–87. doi: 10.1890/09-0464.1
Grace, J. B., Schoolmaster, D. R., Guntenspergen, G. R., Little, A. M., Mitchell, B. R., Miller, K. M., et al. (2012). Guidelines for a graph-theoretic implementation of structural equation modeling. Ecosphere 3, 1–44. doi: 10.1890/es12-00048.1
Gross, K. L. (1984). Effects of seed size and growth form on seedling establishment of six monocarpic perennial plants. J. Ecol. 72, 369–387. doi: 10.2307/2260053
Hikosaka, K. (2004). Leaf canopy as a dynamic system: Ecophysiology and optimality in leaf turnover. Ann. Bot. 95, 521–533. doi: 10.1093/aob/mci050
HKEPD (2007). Western waters. Available online at: https://www.epd.gov.hk/epd/misc/marine_quality/1986-2005/eng/08_western_content.htm (accessed February 16, 2023).
Hong, P., Wen, Y., Xiong, Y., Diao, L., Gu, X., Feng, H., et al. (2021). Latitudinal gradients and climatic controls on reproduction and dispersal of the non-native mangrove Sonneratia apetala in China. Estuar. Coast. Shelf Sci. 248:106749. doi: 10.1016/j.ecss.2020.106749
Iler, A. M., CaraDonna, P. J., Forrest, J. R. K., and Post, E. (2021). Demographic consequences of phenological shifts in response to climate change. Annu. Rev. Ecol. Evol. Syst. 52, 221–245. doi: 10.1146/annurev-ecolsys-011921-032939
IPCC (2022). Climate change 2022: Impacts, adaptation, and vulnerability. Contribution of working group II to the sixth assessment report of the intergovernmental panel on climate Change. Cambridge: Cambridge University Press.
Kline, R. B. (2005). Principles and practice of structural equation modeling. New York, NY: Guilford.
Knauf, A. E., Litton, C. M., Cole, R. J., Sparks, J. P., Giardina, C. P., Gerow, K. G., et al. (2021). Nutrient-use strategy and not competition determines native and invasive species response to changes in soil nutrient availability. Restor. Ecol. 29:e13374. doi: 10.1111/rec.13374
Körner, C., and Basler, D. (2010). Phenology under global warming. Science 327, 1461–1462. doi: 10.1126/science.1186473
Lee, S. Y. (1995). Mangrove outwelling: A review. Hydrobiologia 295, 203–212. doi: 10.1007/bf00029127
Li, M., Lan, C., Chen, G., Li, S., Wong, Y. S., Tam, N. F. Y., et al. (1992). Mangrove community in Futian, Shenzhen (in Chinese). Ecol. Sci. 1, 40–44.
Liu, L., Li, F., Yang, Q., Tam, N. F. Y., Liao, W., and Zan, Q. (2014). Long-term differences in annual litter production between alien (Sonneratia apetala) and native (Kandelia obovata) mangrove species in Futian, Shenzhen, China. Mar. Pollut. Bull. 85, 747–753. doi: 10.1016/j.marpolbul.2014.04.047
Liu, Y., Oduor, A. M. O., Zhang, Z., Manea, A., Tooth, I. M., Leishman, M. R., et al. (2017). Do invasive alien plants benefit more from global environmental change than native plants? Global Change Biol. 23, 3363–3370. doi: 10.1111/gcb.13579
McKee, K. L. (2011). Biophysical controls on accretion and elevation change in Caribbean mangrove ecosystems. Estuar. Coast. Shelf Sci. 91, 475–483. doi: 10.1016/j.ecss.2010.05.001
Meijer, K. J., El-Hacen, E.-H. M., Govers, L. L., Lavaleye, M., Piersma, T., and Olff, H. (2021). Mangrove-mudflat connectivity shapes benthic communities in a tropical intertidal system. Ecol. Indic. 130:108030. doi: 10.1016/j.ecolind.2021.108030
Nguyen, H. T. T., Hardy, G. E. S. J., Le, T. V., Nguyen, H. Q., Le, D. H., Nguyen, T. V., et al. (2021). Mangrove dieback and leaf disease in Sonneratia apetala and Sonneratia caseolaris in Vietnam. Forests 12:1273. doi: 10.3390/f12091273
Nova, N., Deyle, E. R., Shocket, M. S., MacDonald, A. J., Childs, M. L., Rypdal, M., et al. (2020). Susceptible host availability modulates climate effects on dengue dynamics. Ecol. Lett. 24, 415–425. doi: 10.1111/ele.13652
Oksanen, J., Simpson, G., Blanchet, F., Kindt, R., Legendre, P., Minchin, P., et al. (2022). vegan: Community ecology package. Version 2.6-4. Available online at: https://CRAN.R-project.org/package=vegan (accessed october 11, 2023).
Park, J., Smith, C., Sugihara, G., and Deyle, E. (2021). rEDM: Empirical dynamic modeling (‘EDM’). Version 1.9.2. Available online at: https://CRAN.R-project.org/package=rEDM (accessed January 9, 2023).
Reef, R., Feller, I. C., and Lovelock, C. E. (2010). Nutrition of mangroves. Tree Physiol. 30, 1148–1160. doi: 10.1093/treephys/tpq048
Ren, H., Chen, H., Li, Z., and Han, W. (2010). Biomass accumulation and carbon storage of four different aged Sonneratia apetala plantations in Southern China. Plant Soil 327, 279–291. doi: 10.1007/s11104-009-0053-7
Ren, H., Lu, H., Shen, W., Huang, C., Guo, Q., Li, Z. A., et al. (2009). Sonneratia apetala Buch Ham in the mangrove ecosystems of China: An invasive species or restoration species? Ecol. Eng. 35, 1243–1248. doi: 10.1016/j.ecoleng.2009.05.008
Richards, C. L., Bossdorf, O., Muth, N. Z., Gurevitch, J., and Pigliucci, M. (2006). Jack of all trades, master of some? On the role of phenotypic plasticity in plant invasions. Ecol. Lett. 9, 981–993. doi: 10.1111/j.1461-0248.2006.00950.x
Rosseel, Y. (2012). lavaan: An R package for structural equation modeling. J. Stat. Softw. 48, 1–36. doi: 10.18637/jss.v048.i02
Smith, R. S., Blaze, J. A., Byers, J. E., and Friess, D. (2021). Dead litter of resident species first facilitates and then inhibits sequential life stages of range-expanding species. J. Ecol. 109, 1649–1664. doi: 10.1111/1365-2745.13586
Sugihara, G. (1994). Nonlinear forecasting for the classification of natural time series. Philos. Trans. R. Soc. Lond. Ser. A Phys. Eng. Sci. 348, 477–495. doi: 10.1098/rsta.1994.0106
Sugihara, G., May, R., Ye, H., Hsieh, C.-H., Deyle, E., Fogarty, M., et al. (2012). Detecting causality in complex ecosystems. Science 338, 496–500. doi: 10.1126/science.1227079
Takens, F. (1981). “Detecting strange attractors in turbulence,” in Dynamical systems and turbulence, eds D. Rand and L. S. Young (New York, NY: Springer), 366–381. doi: 10.1007/BF02368233
Tian, G., Chen, L., Peng, S., Yang, X., Chen, J., Chen, M., et al. (2010). Ecological traits of invasiveness of alien mangrove species Sonneratia apetala (in Chinese). Ecol. Environ. Sci. 19, 3014–3020. doi: 10.16258/j.cnki.1674-5906.2010.12.021
van Nes, E. H., Scheffer, M., Brovkin, V., Lenton, T. M., Ye, H., Deyle, E., et al. (2015). Causal feedbacks in climate change. Nat. Climate Change 5, 445–448. doi: 10.1038/nclimate2568
Vile, D., Shipley, B., and Garnier, E. (2006). A structural equation model to integrate changes in functional strategies during old-field succession. Ecology 87, 504–517. doi: 10.1890/05-0822
Wang, B. S., Liao, B. W., Wang, Y. J., and Zan, Q. J. (2002). Mangrove forest ecosystem and its sustainable development in Shenzhen Bay. Beijing: Science Press.
Wang, B., Xin, M., Wei, Q., and Xie, L. (2018). A historical overview of coastal eutrophication in the China Seas. Mar. Pollut. Bull. 136, 394–400. doi: 10.1016/j.marpolbul.2018.09.044
Wardle, D. A., Bardgett, R. D., Klironomos, J. N., Setälä, H., van der Putten, W. H., and Wall, D. H. (2004). Ecological linkages between aboveground and belowground biota. Science 304, 1629–1633. doi: 10.1126/science.1094875
Weston, R., and Gore, P. A. (2016). A brief guide to structural equation modeling. Couns. Psychol. 34, 719–751. doi: 10.1177/0011000006286345
Wimmler, M.-C., Bathmann, J., Peters, R., Jiang, J., Walther, M., Lovelock, C. E., et al. (2021). Plant–soil feedbacks in mangrove ecosystems: Establishing links between empirical and modelling studies. Trees 35, 1423–1438. doi: 10.1007/s00468-021-02182-z
Wong, Y. S., Lan, C. Y., Chen, G. Z., Li, S. H., Chen, X. R., Liu, Z. P., et al. (1995). Effect of wastewater discharge on nutrient contamination of mangrove soils and plants. Hydrobiologia 295, 243–254. doi: 10.1007/bf00029131
Wright, I. J., Reich, P. B., Cornelissen, J. H. C., Falster, D. S., Groom, P. K., Hikosaka, K., et al. (2005). Modulation of leaf economic traits and trait relationships by climate. Global Ecol. Biogeogr. 14, 411–421. doi: 10.1111/j.1466-822x.2005.00172.x
Zan, Q. J., Wang, B. S., Wang, Y. J., and Li, M. G. (2003). Ecological assessment on the introduced Sonneratia caseolaris and S. apetala at the Mangrove Forest of Shenzhen Bay, China. Acta Bot. Sin. 45, 544–551.
Zan, Q., Wang, Y., and Wang, B. (2002). Accumulation and cycle of N, P, K elements in Sonneratia apetala + S. caseolaris mangrove community at Futian of Shenzhen, China (in Chinese). Guihaia 22, 331–336.
Zan, Q., Wang, Y., Liao, B., and Zheng, D. (2001). Biomass and net productivity of Sonneratia apetala, S. caseolaris mangrove man-made forest (in Chinese). J. Wuhan Bot. Res. 19, 391–396.
Zeileis, A., and Grothendieck, G. (2005). zoo: S3 infrastructure for regular and irregular time series. J. Stat. Softw. 14, 1–27. doi: 10.18637/jss.v014.i06
Zhang, J., Lin, Q., Peng, Y., Pan, L., Chen, Y., Zhang, Y., et al. (2022). Distributions of the non-native Mangrove Sonneratia apetala in China: Based on google earth imagery and field survey. Wetlands 42:35. doi: 10.1007/s13157-022-01556-4
Keywords: coastal eutrophication, exotic dominance, litterfall, long-term monitoring, nutrients
Citation: Huang Z, Li Z, Wang M, Zhu D, Yang Q and Yu S (2023) Coastal nutrient enrichments facilitated reproductive output in exotic mangrove species over two decades. Front. For. Glob. Change 6:1183432. doi: 10.3389/ffgc.2023.1183432
Received: 20 March 2023; Accepted: 05 June 2023;
Published: 20 June 2023.
Edited by:
Nobuo Imai, Tokyo University of Agriculture, JapanReviewed by:
Masayuki Ushio, Hong Kong University of Science and Technology, Hong Kong SAR, ChinaShamim Ahmed, Technical University of Munich, Germany
Copyright © 2023 Huang, Li, Wang, Zhu, Yang and Yu. This is an open-access article distributed under the terms of the Creative Commons Attribution License (CC BY). The use, distribution or reproduction in other forums is permitted, provided the original author(s) and the copyright owner(s) are credited and that the original publication in this journal is cited, in accordance with accepted academic practice. No use, distribution or reproduction is permitted which does not comply with these terms.
*Correspondence: Shixiao Yu, lssysx@mail.sysu.edu.cn
†Present addresses: Zhen Li, Shenzhen Natural Resources and Real Estate Evaluation and Development Research Center, Shenzhen, China; Dehuang Zhu, College of Ecology and Resources Engineering, Wuyi University, Nanping, China