- 1The State Key Laboratory of Information Engineering in Surveying, Mapping and Remote Sensing, Wuhan University, Wuhan, China
- 2Department of Geosciences, University of Arkansas, Fayetteville, AR, United States
Vegetation phenology is a key indicator of vegetation-climate interactions and carbon sink changes in ecosystems. Therefore, it is very important to understand the temporal and spatial variability of vegetation phenology and the driving climatic determinants [e.g., temperature (Ts) and soil moisture (SM)]. Vegetation greenness and photosynthetic phenology were derived using the double logistic (DL) method to enhance vegetation index (EVI) and solar-induced chlorophyll fluorescence (SIF) spring and autumn phenology, respectively. The growing season length (GSL) of greenness phenology (about 100 days) derived EVI was longer than GSL of photosynthetic phenology (about 80 days) derived SIF. Although their overall spatiotemporal pattern trends were consistent, photosynthetic phenology varied 1.4 to 3.1 times more than greenness phenology over time. In addition, SIF-based photosynthetic phenology and EVI-based greenness phenology showed consistent factors of drivers but differed to some extent in spatial patterns and the most relevant preseason dates. Spring photosynthetic phenology was mainly influenced by pre-season mean cumulative Ts (about 90 days). However, greenness phenology was controlled by both pre-seasons mean cumulative Ts [(about 55 days) and mean cumulative SM (about 40 days)]. Autumn photosynthetic phenology was controlled by both periods’ mean cumulative Ts [(about 20 days) and SM (about 20 days)], but autumn greenness phenology was mainly influenced by pre-season mean cumulative Ts (85 days). The comparison analysis of SIF and EVI phenology helps to understand the difference between photosynthetic phenology and greenness phenology at a regional scale.
1. Introduction
Vegetation phenology changes are mainly characterized by key phenology parameters such as the start of the season (SOS) and end of the season (EOS) (Melaas et al., 2016; Hufkens et al., 2019; Salas, 2020). Vegetation phenology is an important variable in studies on food security (Lobell et al., 2008; dela Torre et al., 2021a), drought (Brown and de Beurs, 2008), forest fire risk (Westerling, 2006), and ecosystem carbon balance and water and energy exchange (Piao et al., 2008; Richardson et al., 2013; Keenan et al., 2014; Jin et al., 2017; Wang et al., 2021). Meanwhile, vegetation phenology can be used to understand the effects of global or regional climate change on vegetation processes and vegetation-environment interactions (Buermann et al., 2018; Richardson et al., 2018). Consequently, the study of vegetation phenology changes in ecosystems is of great importance to gain insight into climate change and the carbon cycle.
Traditionally, vegetation phenology research has been based on field observations, which are costly, discontinuous of spatial scale, and subjective to the observer’s judgment. With the development of remote sensing technology, it is able to quantify vegetation phenology at the pixel scale, i.e., “Land Surface Phenology (LSP)” (Henebry and de Beurs, 2013), making it potential for regional and even global-scale studies of relationship between vegetation phenology and climate change (White et al., 1997). Traditional vegetation indices (e.g., normalized difference vegetation index, NDVI and enhanced vegetation index, EVI) mainly respond to seasonal changes in vegetation greenness without reflecting the actual vegetation photosynthetic (Wang et al., 2019; Zhang et al., 2020). Therefore, the timing and duration of vegetation greenness activity are called greenness phenology. Recently, satellite remote sensing of solar-induced chlorophyll fluorescence (SIF) has become available (Meroni et al., 2009; Frankenberg et al., 2011). Several studies have shown that SIF is correlated with ecosystem gross primary productivity (GPP) (Frankenberg et al., 2011; Zarco-Tejada et al., 2013; Li et al., 2018). SIF monitors the intrinsic photosynthetic processes of vegetation (Tang et al., 2016). Therefore, SIF is available for vegetation photosynthetic phenology studies (Jeong et al., 2017; Chang et al., 2019; Wang et al., 2019; Zhang et al., 2020). The timing and duration of vegetation photosynthetic activity are called photosynthetic phenology. Nowadays, studies have shown differences between greenness and photosynthetic phenology (Zhang et al., 2020; Xie et al., 2022), which overestimates the length of vegetation photosynthetic phenology (Jeong et al., 2017), indicating a systematic bias in seasonality between vegetation structure and function (Yin et al., 2020). Therefore, it is necessary to quantitatively assess the differences between greenness phenology and photosynthetic phenology, to understand the potential of greenness phenology to characterize photosynthetic phenology and the reasons for the differences.
In the context of climate change, it is widely accepted that SOS is advanced, while EOS is delayed so that it extends the length of the growing season (Buermann et al., 2018). Previous studies have suggested that pre-season precipitation may indirectly affect changes in SOS by increasing its water demand (Yang et al., 2017). And it has also been suggested that early SOS is caused by global warming (Shen et al., 2015; Li et al., 2022; Wang et al., 2022). Moreover, studies have shown that pre-season temperature and precipitation play a key role in regulating greenness phenology (Ge et al., 2015; Cao et al., 2018; Ren et al., 2019). In general, the results of the above studies were derived from greenness vegetation indices for phenology. At present, the SIF-based phenology study is in its start-up phase (Meng et al., 2021). In addition, studies have shown that SIF is more sensitive to water and heat stress than greenness vegetation indices (Yoshida et al., 2015; Song et al., 2018; Liu et al., 2021). Vegetation photosynthesis is subject to stress from environmental factors in the light use efficiency (LUE) model (Monteith, 1972; Porcar-Castell et al., 2014; dela Torre et al., 2021b). Wang et al. (2019) showed that SIF-derived phenology was two to four times more sensitive to climate than EVI-derived phenology. However, the pre-season time difference between photosynthetic and greenness phenology in response to pre-season water and heat is unclear.
In summary, while EVI indicates vegetation structural information, SIF is a probe of vegetation photosynthesis. The differences between the photosynthetic and greenness phenology derived from EVI and SIF and its response to pre-season water and heat are unclear. We used the double logistic (DL) method to derive SOS and EOS for SIF and EVI, respectively, and investigated their spatial and temporal variation. The objectives of this study are to explore two issues: (1) to explore the spatial and temporal patterns of photosynthetic and greenness phenology, and (2) to explore the pre-season length of water and heat of photosynthesis and greenness phenology.
2. Materials and method
2.1. Study area
This paper takes Heilongjiang Province, Jilin Province, and the eastern part of the Inner Mongolia Autonomous Region (Hulun Beier, Hinggan League, Tongliao, and Chifeng) as the study area (40.08–53.55°N, 115.52°-135.08°E) (Figure 1). Because vegetation phenology in the northern hemisphere at mid to high latitudes is sensitive to climate change (Schwartz et al., 2006; Jeong et al., 2011). The eastern part belongs to the temperate monsoon climate with annual precipitation ranging from 400 to 700 mm, while the western part belongs to the temperate continental climate with annual precipitation between 90 and 400 mm (Mao et al., 2012). The study area has four distinct seasons, with both hot and rainy seasons and long, cold winters. It is in the middle latitudes, and vegetation is sensitive to changes in the climatic environment. Also, the study area is abundant in vegetation types, such as grasslands, deciduous broadleaf forests, mixed forests, woody savannas, and croplands.
2.2. Datasets
In this study, various data products were used to explore the differences between vegetation greenness phenology and vegetation photosynthetic phenology. For consistency, the spatial resolution of these datasets was unified by mean method to 0.25° and time span from 2003 to 2018 in the analysis of the time lag between vegetation phenology and climatic factors.
2.2.1. Solar-induced chlorophyll fluorescence (SIF)
Contiguous SIF (CSIF) and global OCO-2 SIF (GOSIF) datasets were used to derive photosynthetic phenology. The CSIF dataset was generated using MODIS surface reflectance and a neural network approach (Zhang et al., 2018a).1 CSIF dataset captures the seasonal and spatial changes of the original OCO-2 SIF in the far-infrared band (767 nm), which is shown to be closely related to the spatial and temporal variations of GPP (Zhang et al., 2016, 2018a; Sun et al., 2017). In this study, we used CSIF clear-daily from 2003 to 2019 with a temporal resolution of 4 days and spatial resolution of 0.05°. The CSIF clear sky product was chosen because of its strong correlation with the satellite retrieval SIF and also with GPP from the vorticity flux towers (Zhang et al., 2018a,b).
The GOSIF dataset with the continuous high spatial and temporal resolution was developed from discrete OCO-2 SIF, MODIS products, meteorological reanalysis data, and multiple regressions (Li and Xiao, 2019).2 In this study, we used GOSIF from 2003 to 2019 with a temporal resolution of 8-day and spatial resolution of 0.05°. GOSIF had strong consistency (R2 = 0.73, p < 0.001) with GPP from 91 FLUXNET2015 dataset (Li and Xiao, 2019).
2.2.2. MODIS products
Vegetation cover types were obtained from the MODIS MCD12C1 land cover type product of the International Geosphere-Biosphere Programme (IGBP) classification scheme. MODIS IGBP land cover type data are annual synthetic products with a spatial resolution of 0.05°. In addition, the 8-day synthetic surface spectral reflectance from MODIS MOD09A1 was used to calculate the EVI from 2003 to 2019. They are all available for free download at https://search.earthdata.nasa.gov/.
2.2.3. Climate data
Temperatures (Ts) were downloaded from the China Meteorological Forcing Dataset (CMFD, Yang and He, 2019; He et al., 2020)3 with a temporal resolution of days and a spatial resolution of 0.1°. In addition, soil moisture (SM) data were downloaded from the Global Land Evaporation Amsterdam Model (GLEAM) v3.5a (Miralles et al., 2011; Martens et al., 2017),4 with a temporal resolution of days and a spatial resolution of 0.25°.
2.3. Double logistic phenology method
We used a logistic function to fit the SIF and EVI observations (Zhang et al., 2003; Ganguly et al., 2010). To further eliminate the effect of outliers, the data were smoothed using Savitzky–Golay (SG) filtering (Chen et al., 2004). The logistic functional fitting method has advantages for estimating phenology with noisy data (Hird and Mcdermid, 2009). The fitting equation is (Zhang et al., 2003; Ganguly et al., 2010):
For consistency, hereinafter referred to as SOS, EOS, and GSL based on CSIF as SOSCSIF, EOSCSIF, and GSLCSIF, respectively; and SOS, EOS, and GSL based on GOSIF as SOSGOSIF, EOSGOSIF, and GSLGOSIF respectively; and SOS, EOS, and GSL based on EVI as SOSEVI, EOSEVI, and GSLEVI, respectively.
2.4. Analysis
We analyzed the temporal and spatial patterns of phenology parameters for each dataset in the study area. We used multi-year means to represent the spatial patterns of SOS, EOS, and GSL and analyzed the rate of change of SOS, EOS, and GSL over time using the linear trend with the equation.
Both SOS and EOS were strongly controlled by preseason climatic factors (Fu et al., 2015; Güsewell et al., 2017). To further understand the potential climate drivers of spatial and temporal patterns of phenology, we explored the correlations between phenological parameters and preseason climate factors using correlation and partial correlation analyses, extending forward in 5-day intervals from the SOS and EOS dates to the 200th day.
The date when climatic factors had the maximum absolute value of the correlation coefficient was defined as the preseason date when the phenological parameters were most correlated with climatic factors. The pixels that reached 95% significance were found according to correlation coefficient significance reference table. The partial correlation formula is:
3. Results
3.1. Temporal variation of photosynthetic and greenness phenology
From Figure 2, SOSCSIF and SOSEVI derived by DL from 2003 to 2019 showed advancement at the rate of-0.469 days/year (p < 0.01) and-0.324 days/year (p < 0.05), respectively, indicating that the rate of change of SOSCSIF was greater than that of SOSEVI over time. Then, EOSCSIF and EOSEVI derived using DL were delayed at the rates of 0.218 days/year (p < 0.05) and 0.023 days/year (p > 0.05), respectively over time, where the delay rate of EOSCSIF was clearly higher than that of EOSEVI. Finally, GSLCSIF (0.675 days/year, p < 0.01) still lengthened at a greater rate than GSLEVI (0.216 days/year, p > 0.05) over time. The change rates of SOSCSIF, EOSCSIF, and GSLCSIF were more than the change rates of SOSEVI, EOSEVI, and GSLEVI over time. Moreover, the rate of change of SOSGOSIF, EOSGOSIF, and GSLGOSIF compared with SOSEVI, EOSEVI, and GSLEVI was consistent with the findings of the rate of change of SOSCSIF, EOSCSIF, and GSLCSIF compared with SOSEVI, EOSEVI, and GSLEVI (Supplementary Figure S1). In summary, the rate of change in photosynthetic phenology (SOS, EOS, and GSL) derived from SIF over time was greater than the rate of change in greenness phenology (SOS, EOS, and GSL) derived from EVI over time.
3.2. Spatial variation of photosynthetic and greenness phenology
SOSCSIF (or SOSGOSIF) were dated from mid-April to May (Figure 3A and Supplementary Figure S2A), but SOSEVI was dated from late March to mid-April (Figure 3D), with a clear spatial pattern in which the croplands were apparently later than the natural vegetation (Figures 1, 3A,D and Supplementary Figure S2A). Meanwhile, From Figure 3G and Supplementary Figure S2J, SOSEVI was earlier than SOSCSIF (or SOSGOSIF), with mean values of 99.69, 122.68, and 130.31 days, respectively. EOSCSIF (or EOSGOSIF) and EOSEVI showed an increasing trend from north to south (Figures 4B,E and Supplementary Figure S2B), but EOSEVI (mean 283.26 days) was later than EOSCSIF (mean 281.76 days) and EOSGOSIF (281.48 days) (Figure 3H and Supplementary Figure S2H). The croplands’ SOS was later than natural vegetation and GSLCSIF (or GSLGOSIF) was also markedly shorter than natural vegetation for croplands (Figure 3C and Supplementary Figure S2C), but GSLEVI did not perform clearly (Figure 3F). In terms of the length of GSL, from longest to shortest growing period were GSLEVI (185.62 days), GSLGOSIF (158.81 days), and GSLCSIF (151.65 days) (Figure 3I and Supplementary Figure S2I). Overall, SOSEVI spanned about 2 months. EOSCSIF and EOSGOSIF were shorter (about 1 month), but EOSEVI spanned 2.5 months. GSLCSIF and GSLGOSIF had an 80-day spanned. GSLEVI spanned 100 days. The results showed that the length of the greenness phenology growth period represented by EVI was greater than that characterized by SIF for photosynthetic phenology.
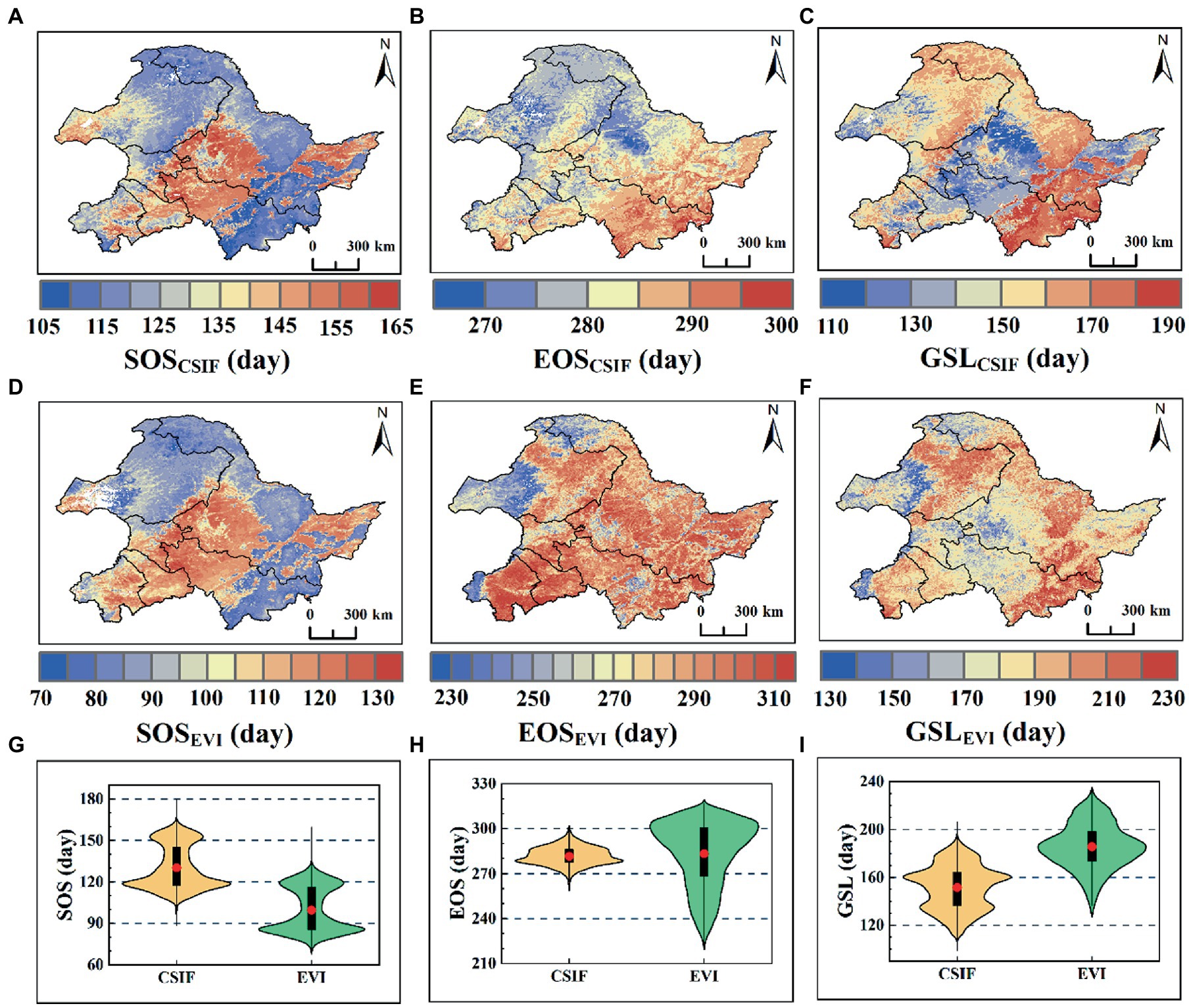
Figure 3. Spatial patterns of SOS, EOS and GSL means based on DL extracted CSIF and EVI datasets. (A–C) Spatial patterns of SOS, EOS, and GSL derived CSIF; (D–F) Spatial patterns of SOS, EOS, and GSL derived EVI; (G–I) Violin plots, where red dots indicate means, black rectangular boxes cover the interquartile range, and thin black lines reach the 5th and 95th percentiles.
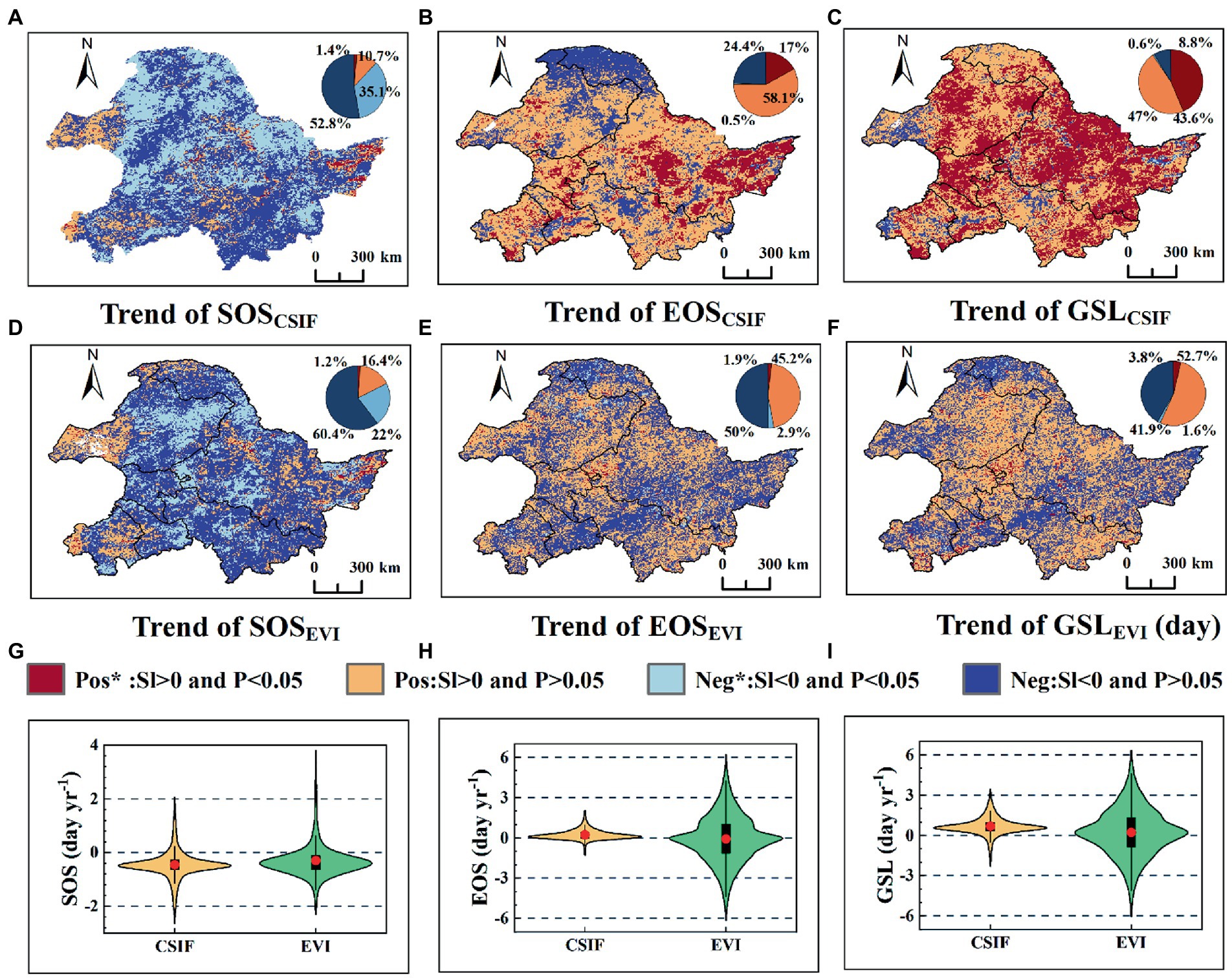
Figure 4. Spatial patterns of correlations between SOS and EOS extracted from CSIF and EVI and pre-season Ts, SM. (A–D) Spatial patterns of correlation coefficients between SOS, EOS derived CSIF and preseason Ts, SM; (E–H) Spatial patterns of correlation coefficients between SOS, EOS derived EVI and preseason Ts, SM; (I–L) Violin plots, where red dots indicate the mean, black rectangular boxes cover the interquartile range, and thin black lines reach the 5th and 95th percentiles. The black dot markers indicate that the 95% significance level was reached.
Next, we further analyzed the trends of SOS, EOS, and GSL pixels level derived from CSIF, GOSIF, and EVI, respectively, from 2003 to 2019. From Figure 5G and Supplementary Figure S3G, most areas of SOSCSIF, SOSGOSIF, and SOSEVI showed an early trend, with 87.9, 89.2, and 82.4% of the areas advanced, respectively, of which 35.1, 44.4, and 22.0% of the pixels reached 95% significant level, respectively (Figures 5A,D and Supplementary Figure S3A). From Figure 5K and Supplementary Figure S3K, the areas of EOSCSIF, EOSGOSIF, and EOSEVI delays varied widely, with 75.1, 67.8, and 47.0% of the pixels showing a delayed trend, respectively, and the percentage of those reaching the 95% significance level was also smaller, 17.0, 13.7, and 1.9%, respectively (Figures 5B,E and Supplementary Figure S3B). Comparison between SOS and EOS revealed that the area of SOS advancement and the percentage of reaching 95% significance were both obviously larger than the area of EOS delay and the percentage of reaching 95% significance. From Figure 5I and Supplementary Figure S3I, GSLCSIF, GSLGOSIF, and GSLEVI showed a lengthened trend, with 90.6, 90.5, and 56.5% of the area showing a lengthened trend, respectively, of which 43.6, 43.7, and 3.8% of the pixels reached the 95% significance level (Figures 5C,F and Supplementary Figure S3C). The spatial variations of CSIF and GOSIF-derived phenological parameters were highly consistent, and the spatial trends between CSIF (or GOSIF) and EVI-extracted SOS were universally consistent. The results showed that the spatial patterns of photosynthetic phenology and greenness phenology differed to some extent, especially EOS and GSL.
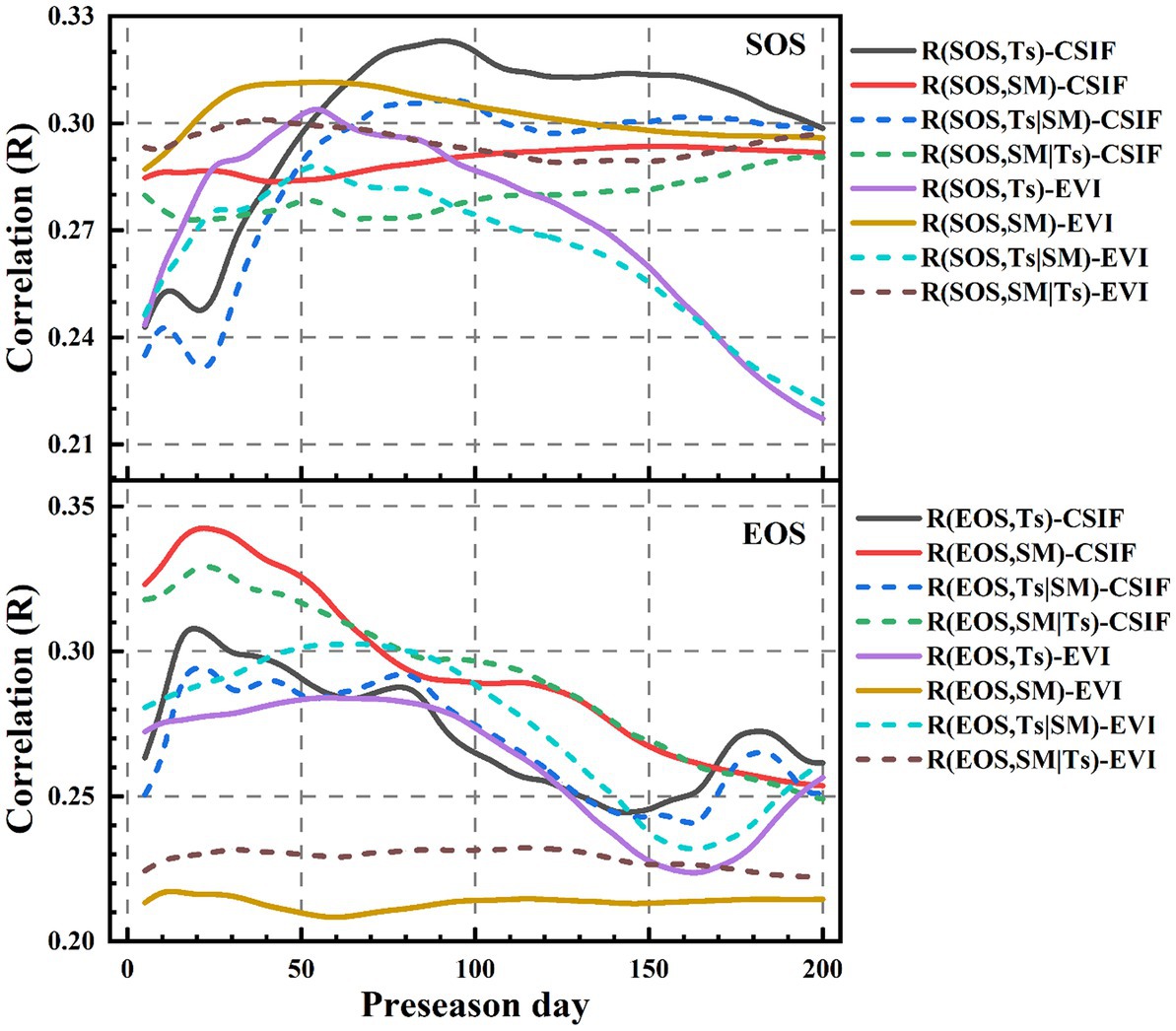
Figure 5. Spatial patterns of SOS, EOS, and GSL change rates and significance based on DL extracted CSIF and EVI datasets. (A–C) Spatial patterns of SOS, EOS, and GSL derived CSIF temporal rates of change and significance; (D–F), Spatial patterns of SOS, EOS, and GSL derived EVI temporal rates of change and significance; (G–I) Violin plots, where red dots indicate means, black rectangular boxes cover the interquartile range, and thin black lines reach the 5th and 95th percentiles.
3.3. Climatic determinants of photosynthetic and greenness phenology
Ts and SM are necessary for vegetation growth. Ts and SM affect vegetation photosynthesis under climate change (Zhang et al., 2010; Chong et al., 2017; Dang et al., 2022; Haerani et al., 2023; Zhao et al., 2023). Thus, the correlation and partial correlation analysis were used to explore the correlation between phenological parameters and preseason Ts and SM. From Figure 6, the correlation coefficients between SOS, EOS derived from CSIF, EVI, respectively, and preseason Ts, SM showed increasing then decreasing trends, respectively. Studies have shown that Ts and precipitation before the occurrence of phenology events play a key role in regulating phenology (Ge et al., 2015; Cao et al., 2018; Ren et al., 2019). This paper showed that the maximum correlation coefficients between SOSEVI and preseason Ts were reached at the cumulative mean Ts of 2–3 months using correlation and partial correlation coefficients analysis (Figure 6), which was consistent with the existing study (Wu and Liu, 2013). But SOSCSIF reached the maximum correlation about 1 month earlier than SOSEVI (Figure 6). Compared to Ts, the correlation coefficients between SOSCSIF and preseason SM (months 5–6) took longer to reach maximum correlation (Figure 6), which was consistent with previous finding (Piao et al., 2006). However, SOSEVI was consistent with preseason SM and Ts. The maximum correlation coefficient was reached between EOS and preseason Ts, SM ranging from 20 to 60 days. And the timing of the maximum correlation coefficients between EOSCSIF, EOSEVI and preseason Ts, SM was generally consistent (Figure 6). In addition, SOSGOSIF and EOSGOSIF were consistent with the responses of SOSCSIF and EOSCSIF to preseason Ts and SM (Figure 6 and Supplementary Figure S4).
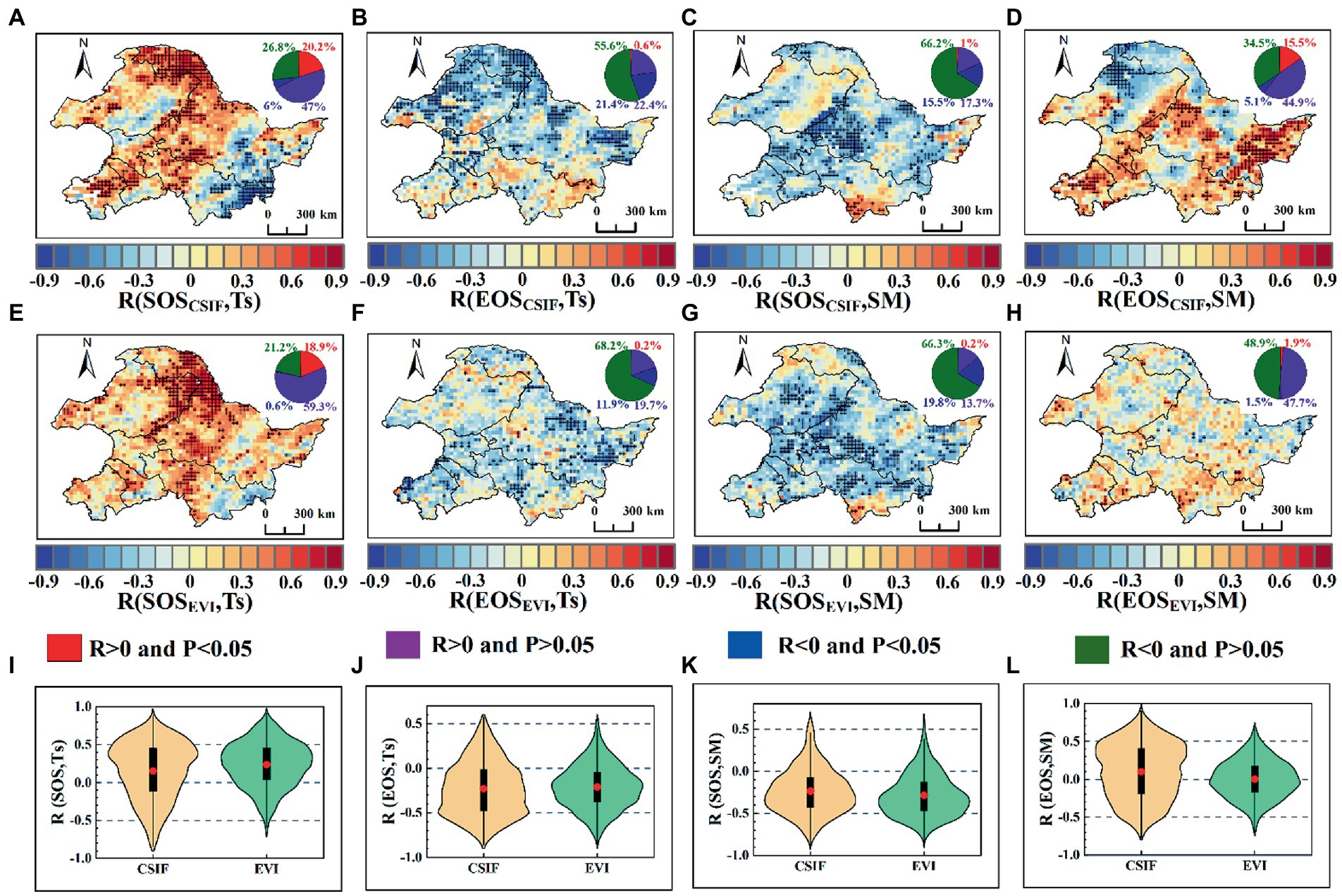
Figure 6. Correlation coefficients and partial correlation coefficients between CSIF, EVI derived SOS, EOS, respectively and pre-season Ts, SM over time.
We further analyzed the spatial distribution of correlation coefficients between SOS, EOS and preseason Ts, SM from the pixel level. From Figure 4I, SOSCSIF and SOSEVI were mostly positively correlated with Ts, with mean values of 0.153 and 0.237, respectively. The positive correlation coefficients between SOSCSIF, SOSEVI and Ts were 67.2 and 78.1% of the area, respectively, with positive correlation coefficients reaching 95% significance level accounting for 20.2 and 18.8% of the total number of pixels, respectively. The negative correlation coefficients between Ts and SOSCSIF, SOSEVI were mainly concentrated in the southeast of the pixels (Figures 4A,E). However, most areas of correlation coefficients between Ts and EOSCSIF, EOSEVI were negative with mean values of-0.229 and-0.209, respectively (Figure 4J). The area of negative correlation coefficients between EOSCSIF, EOSEVI and Ts were 77 and 80.1%, respectively, where the negative correlation coefficients reached 95% significance levels of 21.4 and 11.9% of the total number of pixels (Figures 4B,F). Compared to Ts, the correlations of SM with SOS and EOS were generally opposite, i.e., while SM was negatively correlated with SOS, SM was positively correlated with EOS (Figures 4K,L). The mean values of the correlation coefficients between SOSCSIF, SOSEVI and SM were −0.237 and −0.287, respectively, with the areas of negative correlation accounting for 81.7 and 86.1%, respectively, of which the areas reaching 95% significance level were 15.6 and 19.8%, respectively, and the areas reaching significance were mainly distributed in the middle (Figures 4C,G). The mean values of SM correlation coefficients with EOSCSIF, and EOSEVI were 0.1 and 0.0003, respectively (Figure 4L), with positive correlations of 60.4% (15.5% reaching 95% significance level) and 49.6% (1.9% reaching 95% significance level), respectively, concentrated in the southwest, upper center, and southeast. In addition, SOSGOSIF, EOSGOSIF and SOSCSIF, EOSCSIF responded consistently with pre-season Ts and SM at the pixel scale (Figure 4 and Supplementary Figure S5). The analysis at the pixel scale showed that the SM affected a larger area for SOS, but Ts affect a much larger area of EOS.
Next, we analyzed the spatial distribution between SOS, EOS and preseason Ts, SM using partial correlation (Figure 7). The overall partial correlation coefficients between preseason Ts and SOSCSIF [59.9% (12.75% reaching 95% significance level)], SOSEVI [74% (14.3% reaching 95% significance level)] were positive, where the mean values of the partial correlation coefficients were 0.081 and 0.197, respectively. The area of negative partial correlation between preseason Ts and SOSCSIF, SOSEVI were 40.1% (6.6% reaching 95% significance level) and 26% (0.6% reaching 95% significance level), respectively, concentrated in the southeast (Figures 7A,E,I). Compared to SOS, the partial correlations between preseason Ts and EOSCSIF [75.1% (18.5% reaching 95% significance level)] and EOSEVI [83% (12.3% reaching 95% significance level)] were overall negative, where the mean values of the partial correlation coefficients were −0.212 and −0.235 (Figures 7B,F,J). In addition, the partial correlations between preseason SM and SOSCSIF, SOSEVI were mostly negative. The mean values of the partial correlation coefficients between preseason SM and SOSCSIF, SOSEVI were −0.228 and −0.252, respectively, with the proportion of negative correlations for the pixels being 81.8% (13.2% reaching 95% significance level) and 82.2% (14.5% reaching 95% significance level), respectively (Figures 7C,G,K). However, the area of preseason SM positively correlated with EOSCSIF and EOSEVI were 58.5 and 47.6%, respectively, with 12.8 and 2.1% reaching the 95% significance level. Preseason SM was negatively correlated with EOSCSIF and EOSEVI with an area of 41.5% (5.8% reaching 95% significance level) and 52.4% (2% reaching 95% significance level), respectively. The area of positive and negative effects of preseason SM on EOS was approximately equal. The negative effects of preseason SM on EOSCSIF were mainly concentrated in the north, but the negative effects on EOSEVI were dispersed over the whole study area. Moreover, the partial correlation coefficients between preseason SM and EOSCSIF (0.079) and EOSEVI (−0.007) were around 0 (Figures 7D,H,L). Moreover, the partial correlation between SOSGOSIF, EOSGOSIF and SOSEVI, EOSEVI with pre-season Ts, SM compared with CSIF of phenology vs. EVI of phenology was highly consistent (Figure 7 and Supplementary Figure S6). Thus, the results indicated that the preseason SM affected SOS over a larger area, but EOS was more influenced by Ts.
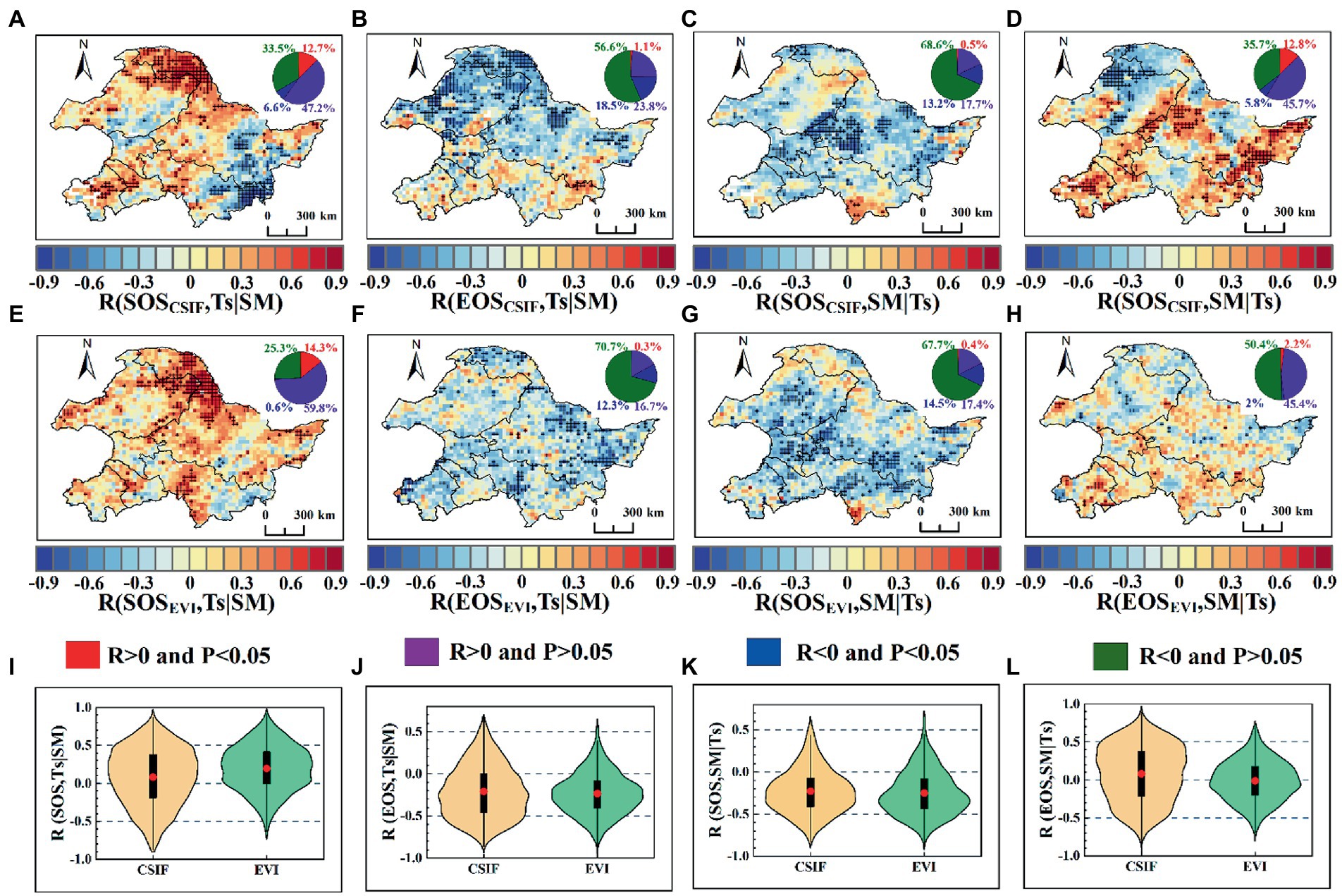
Figure 7. Spatial patterns of partial correlations between SOS and EOS extracted from CSIF and EVI and preseason Ts, SM. (A–D) Spatial patterns of partial correlation coefficients between SOS, EOS derived CSIF and preseason Ts, SM; (E–H) Spatial patterns of partial correlation coefficients between SOS, EOS derived EVI and preseason Ts, SM; (I–L) Violin plots, where red dots indicate the mean, black rectangular boxes cover the interquartile range, and thin black lines reach the 5th and 95th percentiles. The black dot markers indicate that the 95% significance level was reached.
4. Discussion
4.1. Comparison of photosynthesis and greenness phenology
Studies have found that there were systematic differences between photosynthetic and greenness phenology (Figures 2, 3). SOSEVI was earlier than SOSCSIF (or SOSGOSIF), but EOSEVI was later than EOSCSIF (or EOSGOSIF), resulting in greenness phenology growing approximately 20 days longer than photosynthetic phenology (Figure 3 and Supplementary Figure S2), which was consistent with the results of existing studies (Jeong et al., 2017; Meng et al., 2021). Moreover, the rate of change of photosynthetic phenology over time was 1.4–3.1 times greater than that of greenness phenology (Figure 2 and Supplementary Figure S1), which agreed with the results of existing work (Wang et al., 2019). The LUE model is available to explain the different performances in terms of EVI and SIF phenology (Walther et al., 2016; Yang et al., 2022). EVI is a robust proxy for fraction of absorbed photosynthetically active radiation (FAPAR) (Myneni and Williams, 1994) where not all photosynthetically active radiation (PAR) absorbed by the vegetation canopy is used for photosynthesis (Zhang et al., 2018c) but only light absorbed by chlorophyll is used for photosynthesis. The timing of photosynthesis in spring is later than the timing of growing leaves, which varies with leaf structure and longevity (Kikuzawa, 2003). Photosynthesis stops between chlorophyll reduction and leaf abscission due to light limitation (Daumard et al., 2010; Medvigy et al., 2013; Zhang et al., 2020). Also, there are differences in the phenology curves of SIF and EVI; both SIF and EVI are single-peaked curves, with the single peak of the SIF curve being steeper than that of EVI, and EVI decline in autumn slightly later than SIF resulting in a later autumn phenology extraction (Walther et al., 2016; Liu et al., 2018). In addition, SOSCSIF (or SOSGOSIF) was highly consistent with SOSEVI in terms of spatial trends (Figures 3, 5 and Supplementary Figures S2, S3), however, EOSCSIF (or EOSGOSIF) was somewhat different from EOSEVI in terms of spatial trends (Figures 3, 5 and Supplementary Figures S2, S3), which was consistent with the available findings (Meng et al., 2021). The FAPAR by chlorophyll is the dominant factor in spring photosynthetic phenology, while the total amount of photosynthetically active radiation absorbed by chlorophyll is the dominant factor in autumn when radiation determines photosynthetic phenology (Yang et al., 2022). Besides, autumn photosynthetic phenology is controlled by photoperiod, even if the leaves remain green (Bauerle et al., 2012). Therefore, there were some differences between the photosynthetic phenology of SIF and the greenness phenology of EVI using the same method of extraction.
4.2. Comparison of photosynthetic and greenness phenology in response to pre-season climatic determinants
We explored the spatial distribution of preseason Ts or SM with phenological parameters (SOS and EOS) using both correlation analysis and partial correlation analysis, and the results showed that the results of correlation analysis and partial correlation analysis were in high consistency (Figures 4, 7 and Supplementary Figures S5, S6). The spatial patterns of SOSCSIF (or SOSGOSIF), SOSEVI with preseason Ts or SM showed high consistency, but the spatial patterns of EOSCSIF (or EOSGOSIF) with preseason Ts or SM were somewhat different from those of EOSEVI with preseason Ts or SM. It may be related to the fact that SIF curves are more similar to NDVI in spring than in autumn (Jeong et al., 2017). In contrast to the greenness phenology information identified by EVI, SIF is mechanistically related to photosynthesis so it can rapidly respond to almost any factor that regulates photosynthetic activity (Porcar-Castell et al., 2014). This is because the photosynthetic phenology derived from SIF can also be constrained by environmental stress, as indicated by environmental factors in the LUE model (Monteith, 1972).
4.3. Limitations and outlook
This paper only considered the effects of Ts and SM on vegetation phenology. For vegetation phenology, radiation (Cong et al., 2017; Zhang et al., 2020), photoperiod (Basler and Korner, 2014), and accumulated temperature (Fu et al., 2013; Vitasse et al., 2017) may play more important roles. For example, the effect of radiation on photosynthetic phenology and greenness phenology varied considerably, especially at high latitudes in the Northern Hemisphere where photosynthetic phenology was affected by radiation limitation (Zhang et al., 2020). In addition, the study area included a larger crop area and anthropogenic influences on phenology were not considered. It is difficult to know how much each of the multiple influencing factors contributes, especially considering the coupling of solar radiation, human activities, and climatic environmental factors on vegetation phenology is difficult to distinguish their respective contributions. Third, the low spatial resolution of SIF used in this study is subject to scale effects (Liu et al., 2019; Shao et al., 2021), making it necessary to use high-resolution remote sensing data to derive vegetation phenology for the study. Finally, the impact of urban expansion (Zhuang et al., 2022a) and salinization (Zhuang et al., 2021, 2022b), which affect the photosynthesis of vegetation and cause changes in phenology, may also be considered. Therefore, future work will investigate the mechanisms and future predictions of climatic conditions on phenology evolution, the effects of urbanization and land use change on phenology and the combined effects of lag and scale effects on photosynthetic and greenness phenology.
5. Conclusion
In the study, DL was used to derive vegetation greenness and photosynthetic phenology to explore their spatial and temporal patterns and the relationship between pre-season climate determinants. The spatial and temporal variation of photosynthetic phenology extracted based on SIF and greenness phenology extracted by EVI were analyzed, and the results showed that there were systematic differences between photosynthetic and greenness phenology. The greenness phenology averages about 20 days longer than the photosynthetic phenology averages. Moreover, the rate of change of photosynthetic phenology over time was 1.4–3.1 times greater than that of greenness phenology. In addition, the results showed that photosynthetic phenology and greenness phenology were influenced by the same main factors. For spring greenness and photosynthetic phenology were highly consistent with pre-season Ts and SM, but for autumn greenness and photosynthetic phenology varied somewhat to pre-season Ts and SM. This study will help to better understand the differences between changes in vegetation greenness and photosynthetic phenology and their response to climatic factors, as well as to better employ vegetation phenology in the estimation of gross vegetation productivity.
Data availability statement
The original contributions presented in the study are included in the article/Supplementary material, further inquiries can be directed to the corresponding author.
Author contributions
CD conceived the idea and wrote the draft. ZS provided funding acquisition and supervision. XH revised the manuscript. QZ, GC, and JQ analyzed the data and validation. All authors contributed to the article and approved the submitted version.
Funding
This work was supported in part by the National Natural Science Foundation of China (Grant No. 42090012), the Guangxi Science and Technology Program (Grant No. 2021AB30019), 03 Special Research and 5G Project of Jiangxi Province in China (Grant No. 20212ABC03A09), Zhuhai Industry University Research Cooperation Project of China (Grant No. ZH22017001210098PWC), Sichuan Science and Technology Program (Grant Nos. 2022YFN0031, 2023YFS0381, and 2023YFN0022), Hubei Key R&D Plan (Grant No. 2022BAA048), and Zhizhuo Research Fund on Spatial–Temporal Artificial Intelligence (Grant No. ZZJJ202202).
Acknowledgments
The authors thank the reviewers for improving the manuscript with substantive and thoughtful comments and thank editor for feedback and assistance throughout.
Conflict of interest
The authors declare that the research was conducted in the absence of any commercial or financial relationships that could be construed as a potential conflict of interest.
Publisher’s note
All claims expressed in this article are solely those of the authors and do not necessarily represent those of their affiliated organizations, or those of the publisher, the editors and the reviewers. Any product that may be evaluated in this article, or claim that may be made by its manufacturer, is not guaranteed or endorsed by the publisher.
Supplementary material
The Supplementary material for this article can be found online at: https://www.frontiersin.org/articles/10.3389/ffgc.2023.1172220/full#supplementary-material
Footnotes
2. ^http://data.globalecology.unh.edu/data/GOSIF_v2/
3. ^http://data.tpdc.ac.cn/en/data/8028b944-daaa-4511-8769-965612652c49/
References
Basler, D., and Korner, C. (2014). Photoperiod and temperature responses of bud swelling and bud burst in four temperate forest tree species. Tree Physiol. 34, 377–388. doi: 10.1093/treephys/tpu021
Bauerle, W. L., Oren, R., Way, D. A., Qian, S. S., Stoy, P. C., Thornton, P. E., et al. (2012). Photoperiodic regulation of the seasonal pattern of photosynthetic capacity and the implications for carbon cycling. Proc. Natl. Acad. Sci. U. S. A. 109, 8612–8617. doi: 10.1073/pnas.1119131109
Brown, M. E., and de Beurs, K. M. (2008). Evaluation of multi-sensor semi-arid crop season parameters based on NDVI and rainfall. Remote Sens. Environ. 112, 2261–2271. doi: 10.1016/j.rse.2007.10.008
Buermann, W., Forkel, M., O’Sullivan, M., Sitch, S., Friedlingstein, P., Haverd, V., et al. (2018). Widespread seasonal compensation effects of spring warming on northern plant productivity. Nature 562, 110–114. doi: 10.1038/s41586-018-0555-7
Cao, R. Y., Shen, M. G., Zhou, J., and Chen, J. (2018). Modeling vegetation green-up dates across the Tibetan plateau by including both seasonal and daily temperature and precipitation. Agric. For. Meteorol. 249, 176–186. doi: 10.1016/j.agrformet.2017.11.032
Chang, Q., Xiao, X., Jiao, W., Wu, X., Doughty, R., Wang, J., et al. (2019). Assessing consistency of spring phenology of snow-covered forests as estimated by vegetation indices, gross primary production, and solar-induced chlorophyll fluorescence. Agric. For. Meteorol. 275, 305–316. doi: 10.1016/j.agrformet.2019.06.002
Chen, J., Jönsson, P., Tamura, M., Gu, Z. H., Matsushita, B., and Eklundh, L. (2004). A simple method for reconstructing a high-quality NDVI time-series data set based on the Savitzky–Golay filter. Remote Sens. Environ. 91, 332–344. doi: 10.1016/j.rse.2004.03.014
Chong, K. L., Kanniah, K. D., Pohl, C., and Tan, K. P. (2017). A review of remote sensing applications for oil palm studies. Geo. Spat. Inf. Sci 20, 184–200. doi: 10.1080/10095020.2017.1337317
Cong, N., Shen, M. G., and Piao, S. L. (2017). Spatial variations in responses of vegetation autumn phenology to climate change on the Tibetan plateau. J. Plant Ecol. 10, 744–752. doi: 10.1093/jpe/rtw084
Dang, C., Shao, Z., Huang, X., Qian, J., Cheng, G., Ding, Q., et al. (2022). Assessment of the importance of increasing temperature and decreasing soil moisture on global ecosystem productivity using solar-induced chlorophyll fluorescence. Glob. Chang. Biol. 28, 2066–2080. doi: 10.1111/gcb.16043
Daumard, F., Champagne, S., Fournier, A., Goulas, Y., Ounis, A., Hanocq, J.-F., et al. (2010). A field platform for continuous measurement of canopy fluorescence. IEEE Trans. Geosci. Remote 48, 3358–3368. doi: 10.1109/tgrs.2010.2046420
dela Torre, D. M. G., Gao, J., and Macinnis-Ng, C. (2021b). Remote sensing-based estimation of rice yields using various models: A critical review. Geo. Spat. Inf. Sci 24, 580–603. doi: 10.1080/10095020.2021.1936656
dela Torre, D. M. G., Gao, J., Macinnis-Ng, C., and Shi, Y. (2021a). Phenology-based delineation of irrigated and rain-fed paddy fields with Sentinel-2 imagery in Google earth engine. Geo. Spat. Inf. Sci 24, 695–710. doi: 10.1080/10095020.2021.1984183
Frankenberg, C., Fisher, J. B., Worden, J., Badgley, G., Saatchi, S. S., Lee, J., et al. (2011). New global observations of the terrestrial carbon cycle from GOSAT: Patterns of plant fluorescence with gross primary productivity. Geophys. Res. Lett. 38:L17706. doi: 10.1029/2011gl048738
Fu, Y. H., Campioli, M., Deckmyn, G., and Janssens, I. A. (2013). Sensitivity of leaf unfolding to experimental warming in three temperate tree species. Agric. For. Meteorol. 181, 125–132. doi: 10.1016/j.agrformet.2013.07.016
Fu, Y. H., Zhao, H. F., Piao, S. L., Peaucelle, M., Peng, S. S., Zhou, G. Y., et al. (2015). Declining global warming effects on the phenology of spring leaf unfolding. Nature 526, 104–107. doi: 10.1038/nature15402
Ganguly, S., Friedl, M. A., Tan, B., Zhang, X. Y., and Verma, M. (2010). Land surface phenology from MODIS: Characterization of the collection 5 global land cover dynamics product. Remote Sens. Environ. 114, 1805–1816. doi: 10.1016/j.rse.2010.04.005
Ge, Q. S., Wang, H. J., Rutishauser, T., and Dai, J. H. (2015). Phenological response to climate change in China: A meta-analysis. Glob. Change Biol. 21, 265–274. doi: 10.1111/gcb.12648
Gonsamo, A., Chen, J. M., and Ooi, Y. W. (2017). Peak season plant activity shif towards spring is refected by increasing carbon uptake by extratropical ecosystems. Glob. Change Biol. 24, 2117–2128. doi: 10.1111/gcb.14001
Güsewell, S., Furrer, R., Gehrig, R., and Pietragalla, B. (2017). Changes in temperature sensitivity of spring phenology with recent climate warming in Switzerland are related to shifts of the preseason. Glob. Change Biol. 23, 5189–5202. doi: 10.1111/gcb.13781
Haerani, H., Apan, A., Nguyen-Huy, T., and Basnet, B. (2023). Modelling future spatial distribution of peanut crops in Australia under climate change scenarios. Geo. Spat. Inf. Sci. 1–20. doi: 10.1080/10095020.2022.2155255
He, J., Yang, K., Tang, W. J., Lu, H., Qin, J., Chen, Y. Y., et al. (2020). The first high-resolution meteorological forcing dataset for land process studies over China. Sci. Data 7:25. doi: 10.1038/s41597-020-0369-y
Henebry, G. M., and de Beurs, K. M. (2013). “Remote sensing of land surface phenology: A prospectus” in Phenology: An Integrative Environmental Science. ed. M. D. Schwartz (Dordrecht: Springer Netherlands), 385–411.
Hird, J. N., and Mcdermid, G. J. (2009). Noise reduction of ndvi time series: An empirical comparison of selected techniques. Remote Sens. Environ. 113, 248–258. doi: 10.1016/j.rse.2008.09.003
Hufkens, K., Melaas, E. K., Mann, M. L., Foster, T., Ceballos, F., Robles, M., et al. (2019). Monitoring crop phenology using a smartphone based near-surface remote sensing approach. Agric. For. Meteorol. 265, 327–337. doi: 10.1016/j.agrformet.2018.11.002
Jeong, S. J., Ho, C. H., Gim, H. J., and Brown, M. E. (2011). Phenology shifts at start vs. end of growing season in temperate vegetation over the northern hemisphere for the period 1982–2008. Glob. Change Biol. 17, 2385–2399. doi: 10.1111/j.1365-2486.2011.02397.x
Jeong, S.-J., Schimel, D., Frankenberg, C., Drewry, D. T., Fisher, J. B., Verma, M., et al. (2017). Application of satellite solar-induced chlorophyll fluorescence to understanding large-scale variations in vegetation phenology and function over northern high latitude forests. Remote Sens. Environ. 190, 178–187. doi: 10.1016/j.rse.2016.11.021
Jin, J. X., Wang, Y., Zhang, Z., Magliulo, V., Jiang, H., and Cheng, M. (2017). Phenology plays an important role in the regulation of terrestrial ecosystem water-use efficiency in the northern hemisphere. Remote Sens. 9:664. doi: 10.3390/rs9070664
Keenan, T. F., Gray, J., Friedl, M. A., Toomey, M., Bohrer, G., Hollinger, D. Y., et al. (2014). Net carbon uptake has increased through warming-induced changes in temperate forest phenology. Nat. Clim. Chang. 4, 598–604. doi: 10.1038/nclimate2253
Kikuzawa, K. (2003). Phenological and morphological adaptations to the light environment in two woody and two herbaceous plant species. Funct. Ecol. 17, 29–38. doi: 10.1046/j.1365-2435.2003.00707.x
Li, M., Wang, X., and Chen, J. (2022). Assessment of grassland ecosystem services and analysis on its driving factors: A case study in Hulunbuir grassland. Front. Ecol. Evol. 10:25. doi: 10.3389/fevo.2022.841943
Li, X., and Xiao, J. F. (2019). A global, 0.05-degree product of solar-induced chlorophyll fluorescence derived from OCO-2, MODIS, and reanalysis data. Remote Sens. 11:517. doi: 10.3390/rs11050517
Li, X., Xiao, J. F., He, B. B., Arain, M. A., Beringer, J., Desai, A. R., et al. (2018). Solar-induced chlorophyll fluorescence is strongly correlated with terrestrial photosynthesis for a wide variety of biomes: First global analysis based on OCO-2 and flux tower observations. Glob. Change Biol. 24, 3990–4008. doi: 10.1111/gcb.14297
Liu, Y., Dang, C., Yue, H., Lyu, C., and Dang, X. (2021). Enhanced drought detection and monitoring using sun-induced chlorophyll fluorescence over Hulun Buir grassland, China. Sci. Total Environ. 770:145271. doi: 10.1016/j.scitotenv.2021.145271
Liu, L. C., Shen, M., Chen, J., Wang, J., and Zhang, X. (2019). How does scale effect influence spring vegetation phenology estimated from satellite-derived vegetation indexes? Remote Sens. 11:2137. doi: 10.3390/rs11182137
Liu, X. T., Zhou, L., Shi, H., Wang, S. Q., and Chi, Y. G. (2018). Phenological characteristics of temperate coniferous and broad-leaved mixed forests based on multiple remote sensing vegetation indices, chlorophyll fluorescence and CO2 flux data. Acta Ecol. Sin. 38, 3482–3494. doi: 10.5846/stxb20170821150
Lobell, D. B., Burke, M. B., Tebaldi, C., Mastrandrea, M. D., Falcon, W. P., and Naylor, R. L. (2008). Prioritizing climate change adaptation needs for food security in 2030. Science 319, 607–610. doi: 10.1126/science.1152339
Mao, D. H., Wang, Z. M., Han, J. X., and Ren, C. Y. (2012). Spatio-temporal pattern of net primary productivity and its driven factors in Northeast China in 1982-2010. Sci. Geogr. Sin. 32, 1106–1111. doi: 10.13249/j.cnki.sgs.2012.09.1106
Martens, B., Miralles, D. G., Lievens, H., van der Schalie, R., de Jeu, R. A. M., Fernández-Prieto, D., et al. (2017). GLEAM v3: Satellite-based land evaporation and root-zone soil moisture. Geosci. Model Dev. 10, 1903–1925. doi: 10.5194/gmd-10-1903-2017
Medvigy, D., Jeong, S.-J., Clark, K. L., Skowronski, N. S., and Schäfer, K. V. R. (2013). Effects of seasonal variation of photosynthetic capacity on the carbon fluxes of a temperate deciduous forest. J. Geophys. Res. 118, 1703–1714. doi: 10.1002/2013jg002421
Melaas, E. K., Friedl, M. A., and Richardson, A. D. (2016). Multiscale modeling of spring phenology across deciduous forests in the eastern United States. Glob. Change Biol. 22, 792–805. doi: 10.1111/gcb.13122
Meng, F. D., Huang, L., Chen, A. P., Zhang, Y., and Piao, S. L. (2021). Spring and autumn phenology across the Tibetan plateau inferred from normalized difference vegetation index and solar-induced chlorophyll fluorescence. Big Earth Data 5, 182–200. doi: 10.1080/20964471.2021.1920661
Meroni, M., Rossini, M., Guanter, L., Alonso, L., Rascher, U., Colombo, R., et al. (2009). Remote sensing of solar-induced chlorophyll fluorescence: Review of methods and applications. Remote Sens. Environ. 113, 2037–2051. doi: 10.1016/j.rse.2009.05.003
Miralles, D. G., Holmes, T. R. H., De Jeu, R. A. M., Gash, J. H., Meesters, A. G. C. A., and Dolman, A. J. (2011). Global land-surface evaporation estimated from satellite-based observations. Hydrol. Earth Syst. Sci. 15, 453–469. doi: 10.5194/hess-15-453-2011
Monteith, J. L. (1972). Solar radiation and productivity in tropical ecosystems. J. Appl. Ecol. 9:747. doi: 10.2307/2401901
Myneni, R. B., and Williams, D. L. (1994). On the relationship between FAPAR and NDVI. Remote Sens. Environ. 49, 200–211. doi: 10.1016/0034-4257(94)90016-7
Piao, S. L., Ciais, P., Friedlingstein, P., Peylin, P., Reichstein, M., Luyssaert, S., et al. (2008). Net carbon dioxide losses of northern ecosystems in response to autumn warming. Nature 451, 49–52. doi: 10.1038/nature06444
Piao, S. L., Fang, J. Y., Zhou, L. M., Ciais, P., and Zhu, B. (2006). Variations in satellite-derived phenology in China’s temperate vegetation. Glob. Change Biol. 12, 672–685. doi: 10.1111/j.1365-2486.2006.01123.x
Porcar-Castell, A., Tyystjärvi, E., Atherton, J., van der Tol, C., Flexas, J., Pfündel, E. E., et al. (2014). Linking chlorophyll a fluorescence to photosynthesis for remote sensing applications: Mechanisms and challenges. J. Exp. Bot. 65, 4065–4095. doi: 10.1093/jxb/eru191
Ren, S. L., Qin, Q. M., Ren, H. Z., Sui, J., and Zhang, Y. (2019). New model for simulating autumn phenology of herbaceous plants in the inner Mongolian grassland. Agric. For. Meteorol. 275, 136–145. doi: 10.1016/j.agrformet.2019.05.011
Richardson, A. D., Hufkens, K., Milliman, T., Aubrecht, D. M., Furze, M. E., Seyednasrollah, B., et al. (2018). Ecosystem warming extends vegetation activity but heightens vulnerability to cold temperatures. Nature 560, 368–371. doi: 10.1038/s41586-018-0399-1
Richardson, A. D., Keenan, T. F., Migliavacca, M., Ryu, Y., Sonnentag, O., and Toomey, M. (2013). Climate change, phenology, and phenological control of vegetation feedbacks to the climate system. Agric. For. Meteorol. 169, 156–173. doi: 10.1016/j.agrformet.2012.09.012
Salas, E. A. L. (2020). Waveform LiDAR concepts and applications for potential vegetation phenology monitoring and modeling: A comprehensive review. Geo. Spat. Inf. Sci 24, 179–200. doi: 10.1080/10095020.2020.1761763
Schwartz, M. D., Ahas, R., and Aasa, A. (2006). Onset of spring starting earlier across the northern hemisphere. Glob. Change Biol. 12, 343–351. doi: 10.1111/j.1365-2486.2005.01097.x
Shao, Z., Wu, W., and Li, D. (2021). Spatio-temporal-spectral observation model for urban remote sensing. Geo. Spat. Inf. Sci. 24, 372–386. doi: 10.1080/10095020.2020.1864232
Shen, M., Piao, S., Cong, N., Zhang, G., and Jassens, I. A. (2015). Precipitation impacts on vegetation spring phenology on the Tibetan plateau. Glob. Change Biol. 21, 3647–3656. doi: 10.1111/gcb.12961
Song, L., Guanter, L., Guan, K., You, L., Huete, A., Ju, W., et al. (2018). Satellite sun-induced chlorophyll fluorescence detects early response of winter wheat to heat stress in the Indian Indo-Gangetic Plains. Glob. Change Biol. 24, 4023–4037. doi: 10.1111/gcb.14302
Sun, Y., Frankenberg, C., Wood, J. D., Schimel, D. S., Jung, M., Guanter, L., et al. (2017). OCO-2 advances photosynthesis observation from space via solar-induced chlorophyll fluorescence. Science 358:5747. doi: 10.1126/science.aam5747
Tang, J., Körner, C., Muraoka, H., Piao, S., Shen, M., Thackeray, S. J., et al. (2016). Emerging opportunities and challenges in phenology: A review. Ecosphere 7:e01436. doi: 10.1002/ecs2.1436
Vitasse, Y., Signarbieux, C., and Fu, Y. H. (2017). Global warming leads to more uniform spring phenology across elevations. Proc. Natl. Acad. Sci. U. S. A. 115, 1004–1008. doi: 10.1073/pnas.1717342115
Walther, S., Voigt, M., Thum, T., Gonsamo, A., Zhang, Y., Köhler, P., et al. (2016). Satellite chlorophyll fluorescence measurements reveal large-scale decoupling of photosynthesis and greenness dynamics in boreal evergreen forests. Glob. Change Biol. 22, 2979–2996. doi: 10.1111/gcb.13200
Wang, L., De Boeck, H. J., Chen, L., Song, C., Chen, Z., McNulty, S., et al. (2022). Urban warming increases the temperature sensitivity of spring vegetation phenology at 292 cities across China. Sci. Total Environ. 834:155154. doi: 10.1016/j.scitotenv.2022.155154
Wang, S. H., Ju, W. M., Peuelas, J., Cescatti, A., Zhou, Y., Fu, Y. Y., et al. (2019). Urban-rural gradients reveal joint control of elevated CO2 and temperature on extended photosynthetic seasons. Nat. Ecol. Evol. 3, 1076–1085. doi: 10.1038/s41559-019-0931-1
Wang, Y., Xu, W., Yuan, W., Chen, X., Zhang, B., Fan, L., et al. (2021). Higher plant photosynthetic capability in autumn responding to low atmospheric vapor pressure deficit. Innovation 2:100163. doi: 10.1016/j.xinn.2021.100163
Westerling, A. L. (2006). Warming and earlier spring increase Western U.S. Forest wildfire activity. Science 313, 940–943. doi: 10.1126/science.1128834
White, M. A., Thornton, P. E., and Running, S. W. (1997). A continental phenology model for monitoring vegetation responses to interannual climatic variability. Global Biogeochem. Cy. 11, 217–234. doi: 10.1029/97gb00330
Wu, X. C., and Liu, H. Y. (2013). Consistent shifts in spring vegetation green-up date across temperate biomes in China, 1982-2006. Glob. Change Biol. 19, 870–880. doi: 10.1111/gcb.12086
Xie, Q., Cleverly, J., Moore, C. E., Ding, Y., Hall, C. C., Ma, X., et al. (2022). Land surface phenology retrievals for arid and semi-arid ecosystems. ISPRS J. Photogramm. 185, 129–145. doi: 10.1016/j.isprsjprs.2022.01.017
Yang, Y., Chen, R., Yin, G., Wang, C., Liu, G., Verger, A., et al. (2022). Divergent performances of vegetation indices in extracting photosynthetic phenology for northern deciduous broadleaf forests. IEEE Geosci. Remote Sens. Lett. 19, 1–5. doi: 10.1109/LGRS.2022.3182405
Yang, K., and He, J. (2019). China meteorological forcing dataset (1979-2018). Beijing: National Tibetan Plateau Data Center.
Yang, J., Zhang, X. C., Luo, Z. H., and Yu, X. J. (2017). Nonlinear variations of net primary productivity and its relationship with climate and vegetation phenology, China. Forests 8:361. doi: 10.3390/f8100361
Yin, G., Verger, A., Filella, I., Descals, A., and Peñuelas, J. (2020). Divergent estimates of Forest photosynthetic phenology using structural and physiological vegetation indices. Geophys. Res. Lett. 47:e2020GL089167. doi: 10.1029/2020gl089167
Yoshida, Y., Joiner, J., Tucker, C., Berry, J., Lee, J.-E., Walker, G., et al. (2015). The 2010 Russian drought impact on satellite measurements of solar-induced chlorophyll fluorescence: Insights from modeling and comparisons with parameters derived from satellite reflectances. Remote Sens. Environ. 166, 163–177. doi: 10.1016/j.rse.2015.06.008
Zarco-Tejada, P. J., Morales, A., Testi, L., and Villalobos, F. J. (2013). Spatio-temporal patterns of chlorophyll fluorescence and physiological and structural indices acquired from hyperspectral imagery as compared with carbon fluxes measured with eddy covariance. Remote Sens. Environ. 133, 102–115. doi: 10.1016/j.rse.2013.02.003
Zhang, L., Chen, X., Cai, X., and Salim, H. (2010). Spatial-temporal changes of NDVI and their relations with precipitation and temperature in Yangtze River basin from 1981 to 2001. Geo. Spat. Inf. Sci. 13, 186–190. doi: 10.1007/s11806-010-0339-1
Zhang, Y., Commane, R., Zhou, S., Williams, A. P., and Gentine, P. (2020). Light limitation regulates the response of autumn terrestrial carbon uptake to warming. Nat. Clim. Chang. 10, 739–743. doi: 10.1038/s41558-020-0806-0
Zhang, X. Y., Friedl, M. A., Schaaf, C. B., Strahler, A. H., Hodges, J. C. F., Gao, F., et al. (2003). Monitoring vegetation phenology using MODIS. Remote Sens. Environ. 84, 471–475. doi: 10.1016/s0034-4257(02)00135-9
Zhang, Y., Joiner, J., Alemohammad, S. H., Zhou, S., and Gentine, P. (2018a). A global spatially contiguous solar-induced fluorescence (CSIF) dataset using neural networks. Biogeosciences 15, 5779–5800. doi: 10.5194/bg-15-5779-2018
Zhang, Y., Xiao, X. M., Jin, C., Dong, J. W., Zhou, S., Wagle, P., et al. (2016). Consistency between sun-induced chlorophyll fluorescence and gross primary production of vegetation in North America. Remote Sens. Environ. 183, 154–169. doi: 10.1016/j.rse.2016.05.015
Zhang, Y., Xiao, X., Wolf, S., Wu, J., Wu, X., Gioli, B., et al. (2018c). Spatio-temporal convergence of maximum daily light-use efficiency based on radiation absorption by canopy chlorophyll. Geophys. Res. Lett. 45, 3508–3519. doi: 10.1029/2017gl076354
Zhang, Y., Xiao, X. M., Zhang, Y. G., Wolf, S., Zhou, S., Joiner, J., et al. (2018b). On the relationship between sub-daily instantaneous and daily total gross primary production: Implications for interpreting satellite-based SIF retrievals. Remote Sens. Environ. 205, 276–289. doi: 10.1016/j.rse.2017.12.009
Zhao, F., Peng, Z., Qian, J., Chu, C., Zhao, Z., Chao, J., et al. (2023). Detection of geothermal potential based on land surface temperature derived from remotely sensed and in-situ data. Geo. Spat. Inf. Sci. 1–17. doi: 10.1080/10095020.2023.2178335
Zhuang, Q., Shao, Z., Huang, X., Zhang, Y., Wu, W., Feng, X., et al. (2021). Evolution of soil salinization under the background of landscape patterns in the irrigated northern slopes of Tianshan Mountains, Xinjiang, China. Catena 206:105561. doi: 10.1016/j.catena.2021.105561
Zhuang, Q., Shao, Z., Li, D., Huang, X., Altan, O., Wu, S., et al. (2022a). Isolating the direct and indirect impacts of urbanization on vegetation carbon sequestration capacity in a large oasis city: Evidence from Urumqi, China. Geo. Spat. Inf. Sci., 1–13. doi: 10.1080/10095020.2022.2118624
Keywords: solar-induced chlorophyll fluorescence, EVI, greenness phenology, photosynthetic phenology, climate change
Citation: Dang C, Shao Z, Huang X, Zhuang Q, Cheng G and Qian J (2023) Vegetation greenness and photosynthetic phenology in response to climatic determinants. Front. For. Glob. Change. 6:1172220. doi: 10.3389/ffgc.2023.1172220
Edited by:
Yan Li, Nanjing Forestry University, ChinaReviewed by:
Ning Li, Nanjing Forestry University, ChinaHesong Wang, Beijing Forestry University, China
Ying Guo, Nanjing Forestry University, China
Copyright © 2023 Dang, Shao, Huang, Zhuang, Cheng and Qian. This is an open-access article distributed under the terms of the Creative Commons Attribution License (CC BY). The use, distribution or reproduction in other forums is permitted, provided the original author(s) and the copyright owner(s) are credited and that the original publication in this journal is cited, in accordance with accepted academic practice. No use, distribution or reproduction is permitted which does not comply with these terms.
*Correspondence: Zhenfeng Shao, c2hhb3poZW5mZW5nQHdodS5lZHUuY24=