- 1Chongqing Jinfo Mountain Karst Ecosystem National Observation and Research Station, School of Geographical Sciences, Southwest University, Chongqing, China
- 2Institute of Geographic Sciences and Natural Resources Research, Chinese Academy of Sciences, Beijing, China
Introduction: Plantation forest is an important component of global forest resources. The accurate estimation of tree aboveground biomass (AGB) in plantation forest is of great significance for evaluating the carbon sequestration capacity. In recent years, UAV-borne LiDAR has been increasingly applied to forest survey, but the traditional allometric model for AGB estimation cannot be directly used without the diameter at breast height (DBH) of individual trees. Therefore, it is practicable to construct a novel allometric model incorporating the crown structure parameters, which can be precisely extracted from UAV LiDAR data. Additionally, the reduction effect of adjacent trees on crown area (Ac) should be taken into account.
Methods: In this study, we proposed an allometric model depending on the predictor variables of Ac and trunk height (H). The UAV-borne LiDAR was utilized to scan the sample plot of dawn redwood (DR) trees in the test site. The raw point cloud was first normalized and segmented into individual trees, whose Acs and Hs were sequentially extracted. To mitigate the effects of adjacent trees, the initial Acs were corrected to refer to the potential maximum Acs under undisturbed growth conditions. Finally, the corrected Acs (Acc) and Hs were input into the constructed allometric model to achieve the AGBs of DR trees.
Results and discussion: According to accuracy assessment, coefficient of determination (R2) and root mean square error (RMSE) of extracted Hs were 0.9688 and 0.51 m; R2 and RMSE of calculated AGBs were 0.9432 and 10.91 kg. The unrestricted growth parts of the tree crowns at the edge of a plantation forest could be used to derive the potential maximum Ac. Compared with the allometric models for AGB estimation relying only on trunk H or on initial Ac and H, the novel allometric model demonstrated superior performance in estimating the AGBs of trees in a plantation forest.
1. Introduction
Plantation forestry is gradually becoming a significant component of global forest resources (FAO, 2020) and is playing an increasingly crucial role in the global carbon cycle (Fang et al., 2001; Lal, 2005; Chen et al., 2011; Lun et al., 2018; Diao et al., 2022). More importantly, the contribution of plantation forest is expected to surpass that of natural forest in carbon neutralization (Justine et al., 2015; Chen et al., 2022). As the key indicator, the aboveground biomass (AGB) of a forest is vital for quantitatively estimating its carbon sequestration (Dubayah et al., 2010; Chen et al., 2015; Li et al., 2015; Dube and Mutanga, 2016; Lu et al., 2017; Su et al., 2020). Furthermore, the tree AGB is the primary component of the total biomass of a plantation forest (Ming et al., 2012; Yu et al., 2013; Zheng et al., 2014; Li, 2019; Guo et al., 2022). Therefore, the accurate AGB estimation of trees in plantation forest is of great significance for evaluating its carbon sequestration capacity and ecological service functions.
As an active remote sensing technology, light detection and ranging (LiDAR) has stronger penetrability and can overcome the problem of signal saturation in synthetic aperture radar (SAR) and optical remote sensing (Lu et al., 2014; Hu et al., 2016; Nie et al., 2017; Jiang et al., 2020; Su et al., 2020). It can obtain detailed information on the three-dimensional (3D) spatial structure of a forest and the underlying terrain (Popescu et al., 2002; Hilker et al., 2010; Li et al., 2016). Hence, it is a more effective tool to study the extraction of structure parameters of individual trees and the indirect estimation of forest AGB in various forest environments (Hu et al., 2016; Nie et al., 2017; Jiang et al., 2020; Lu et al., 2020; Xu et al., 2021). According to the carrying platform, LiDAR deployments can be classified into four types: space-borne laser scanner (SLS), airborne laser scanner (ALS), vehicle-borne laser scanner (VLS), and terrestrial laser scanner (TLS) (Wu et al., 2013; Chen et al., 2021). Due to the reference beam divergence, the footprint of individual pulses from SLS is on a scale of several meters in diameter (Gong et al., 2011; Sun et al., 2011), making it nearly impossible to identify individual trees in a forest. The platform movement of VLS can be blocked by floor obstacles (Beland et al., 2019), resulting in limited spatial coverage for the data collection of a forest. The poor mobility and complex field operations of TLS restrict repeated surveys for a large forest (Wu et al., 2013; Jiang et al., 2022). In contrast, ALS can provide accurate and dense measurements of multiple forest types with a large spatial coverage (Wu et al., 2013; White et al., 2016). Particularly, unmanned aerial vehicle (UAV)-borne LiDAR has the advantages of higher precision data, lower cost, and stronger maneuverability, and is optimal for forest AGB estimation at the single tree scale (Liu et al., 2018; Wu et al., 2019; Wang et al., 2020; Chen et al., 2021).
The tree biomass equation is the most common and efficient method for non-destructively estimating forest AGB at the individual tree scale (Alvarez et al., 2012; Chave et al., 2014; Paul et al., 2016; Luo et al., 2020) and is generally realized by establishing the allometric relationship between the structure parameters of individual trees and the AGBs. Theoretically, both trunk height (H) and diameter at breast height (DBH) affect the tree AGB (Allouis et al., 2011; Paul et al., 2016; Shi and Liu, 2017). DBH is the most pivotal and commonly used predictor in the allometric equations for tree AGB estimation (Alvarez et al., 2012; Paul et al., 2016; Fu et al., 2018), but it cannot be directly obtained using UAV-borne LiDAR (Allouis et al., 2011; Wu et al., 2013; Lu et al., 2020; Xu et al., 2021). H can be directly and accurately extracted from the UAV LiDAR data (Wallace et al., 2014; Liu et al., 2018; Lu et al., 2020; Ma et al., 2022) and can be utilized as the only predictor to construct the allometric equation to estimate the tree AGB (Blujdea et al., 2012; Goodman et al., 2013; Lin et al., 2018). However, the single parameter H will unavoidably introduce uncertainties into the resulting AGB. The structure parameters reflecting the size and shape of the tree crown (e.g., crown height, crown width, crown diameter, crown projection area, crown surface area, and crown volume) have also exhibited certain allometric relationship with the H/DBH of a single tree (Li, 2019). Therefore, several researchers have tried incorporating crown parameters into the AGB estimation (Popescu, 2007; Wan-Mohd-Jaafar et al., 2017; Liu et al., 2021; Lin et al., 2022) and achieved improved accuracies. However, unlike the DBH, the crown parameters were seriously affected by neighboring trees, especially in a natural forest with multiple tree species and uneven ages. Hence, it will inevitably introduce uncertainties into the estimated AGB using crown parameters. This problem has been greatly weakened in plantation forests, which are intensively managed, comprise only one or two tree species, are even-aged, and are planted at regular spacing (FAO, 2020).
Nevertheless, not all crown parameters are suitable to be combined with trunk height to estimate the tree AGB of a plantation forest. As the point cloud is scanned by UAV-borne LiDAR from the air, it is difficult to obtain those structure parameters involving the crown base, including crown height, crown surface area, and crown volume (Wu et al., 2013; Beland et al., 2019; Xu et al., 2021). As for crown width and diameter, they are more suitable for trees with relatively symmetrical crowns. In contrast, the crown area (Ac) can not only be accurately extracted from the UAV LiDAR data through individual tree segmentation (Kuyah et al., 2012; White et al., 2016; Xu et al., 2021) but can also well reflect the size and shape of tree crowns. Although relatively uniformly distributed in the plantation forest, the individual trees at different positions (in the interior or at the edge) are shaded or squeezed to different extents by adjacent tree crowns. Hence, the Acs of trees with similar trunk heights are not always approximate. Under undisturbed growth conditions, the Ac and H/DBH of an individual tree also have a certain allometric relationship (Allouis et al., 2011; Fu et al., 2018; Li, 2019). Therefore, it is expected to further enhance the precision of AGB estimation based on Ac and H by correcting the initial Acs of trees in a plantation forest according to a unified rule.
In this article, we aim to apply the UAV LiDAR data to extract and correct Ac and construct a novel allometric model based on corrected Ac (Acc) and H to precisely calculate the AGB of plantation trees. The specific objectives comprise (1) establishing the allometric model based on H to retrieve the potential maximum Ac of an individual tree under undisturbed growth conditions, and then combining the initial Ac extracted from the LiDAR point cloud to derive the Acc with the correction equation; (2) constructing the allometric model with predictors of Acc and H to more precisely estimate the AGB of plantation trees with UAV-borne LiDAR.
2. Test site and materials
2.1. Test site
Located in the Beibei District of Chongqing municipality in southwest China and close to Jinyun Mountain and Jialing River (Figure 1a), the test site has elevations varying from 164.52 m to 174.06 m (Figure 1b). This region has the characteristics of a moist monsoon climate in the subtropics, with an average yearly temperature of 18°C and an average yearly precipitation of 1156 mm. The research target is one plot of plantation trees planted along Ma'anxi Creek, and the dawn redwood (DR for short, Metasequoia glyptostroboides Hu and Cheng) is the only tree species. Some shrubs and weeds were sporadically distributed underneath the trees (Figure 1c). Due to its characteristics of fast growth, high yield, forest formation, and strong adaptability, the DR tree has been widely cultivated around the world and categorized as one of the principal afforestation tree species in China (Ma, 2007). The DR trees in the test site grow in their natural state and are nearly planted with regular spacing. Their crowns have a steeple-like shape from the horizontal perspective and a nearly circular shape from the vertical perspective. Hence, it is an appropriate site to study the allometric model with Acc and H as predictors for precisely estimating the AGBs of plantation trees.
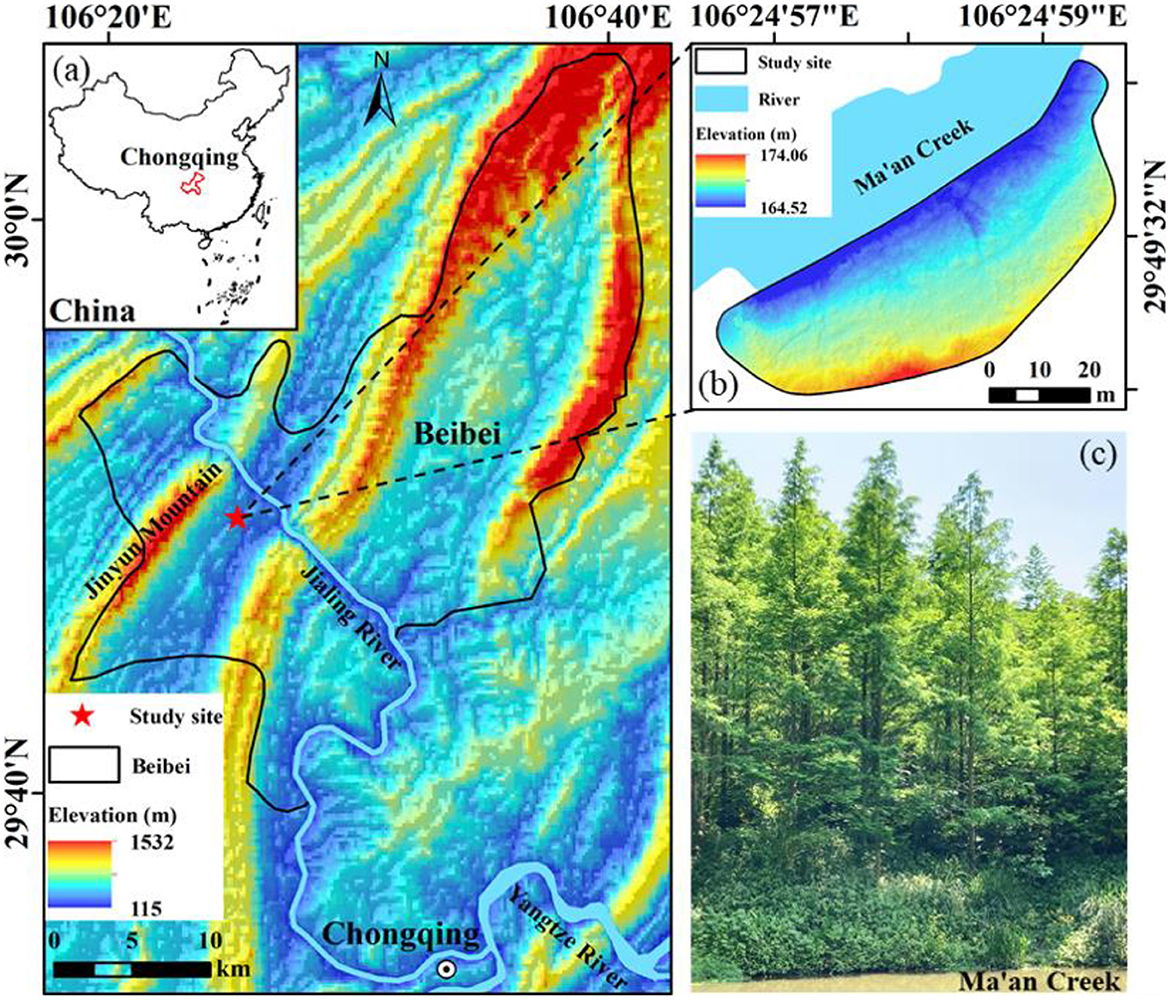
Figure 1. (a) Spatial location of the test site; (b) terrain of the test site rendered by elevation; (c) sample DR trees from the horizontal perspective.
2.2. UAV LiDAR data
In this study, the FEIMA D2000 equipped with D-LiDAR2000 (Figure 2A) was used to scan the test site. The quad-rotor drone has a maximum take-off weight of 3.35 kg and a maximum load of 750 g. The LiDAR system is a light-weight and high-precision airborne laser sensor with a weight of 680 g, a measurement accuracy of up to 3 cm (50 m flight height), three returns of each pulse, and a point frequency of 240 ktps/s. Prior to UAV flight, the technical parameters were preset as follows: the flight trajectory was a cross one (Figure 2B), the flight height was 80 m above the ground, both the longitudinal and lateral overlap were 80%, the flight speed was 5 m/s, and the scanning mode was area scanning. The raw data acquired by the laser scanner were processed using the included software to produce a LAS-formatted point cloud with the coordinate system of WGS84/UTM Zone 48N and a point density of about 2,800 points/m2 (Figure 2C).
2.3. Field-measured data
The data measured in the field were crucial to establishing an allometric model and assessing the accuracy of the estimated AGBs. As shown in Figure 3C, 60 DR trees were selected as samples in this study. Among them, 15 trees marked with cyan points (ID: 1–15) were used for crown area correction, 30 with black points (ID: 16–45) were for fitting biomass models, and 15 trees with white points (ID: 46–60) were for accuracy evaluation. The DBHs and Hs of all sample trees were measured using a total station (Figure 3A) and a DBH tape (Figure 3B) separately and are shown in Table 1.
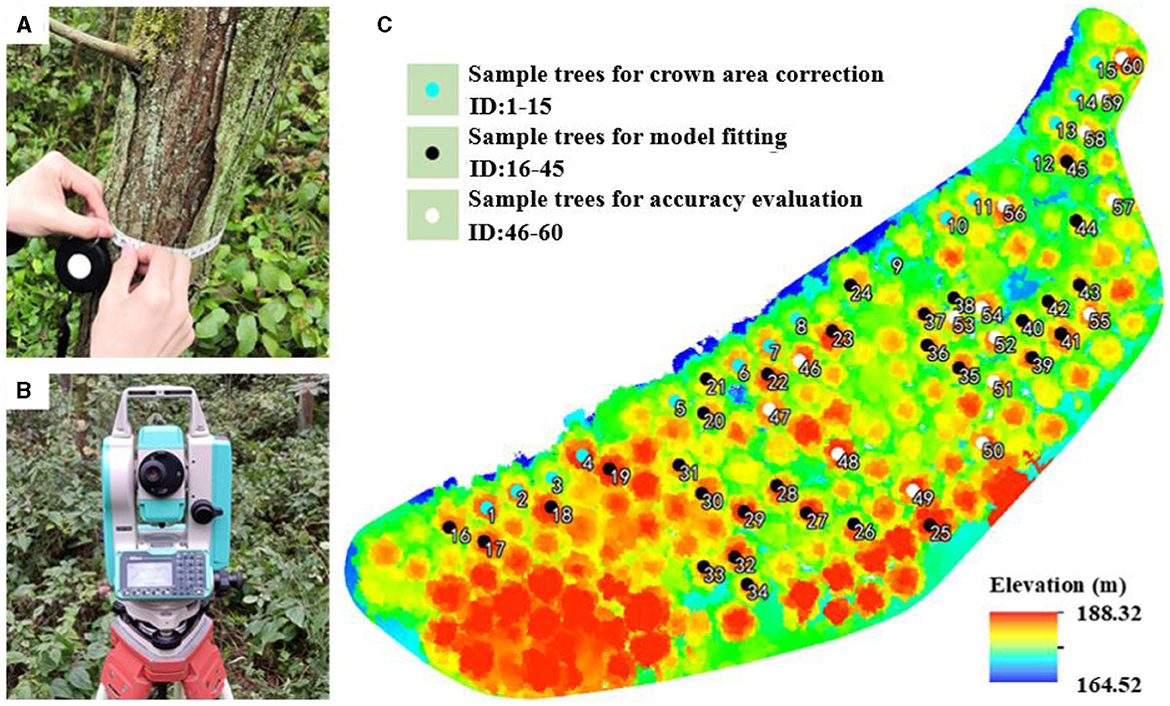
Figure 3. Field measurement: (A) DBH tape for measuring DBH; (B) total station for measuring H; (C) spatial distribution of sample DR trees in the test site.
3. Methods
To combine Ac and H to accurately estimate AGBs of DR trees in the test site, the accurate Ac and an applicable allometric model with predictors of corrected Ac and H are required. The point cloud was first normalized using a digital elevation model (DEM), which was produced from the filtered ground points. Second, the seed points were generated from the canopy height model (CHM), which was obtained from classified vegetation points. Third, individual trees were segmented from the normalized point cloud using seed points, and their Hs and initial Acs were extracted. Fourth, the equation for retrieving the potential maximum Ac of an individual tree under undisturbed growth conditions was established using the derived maximum Ac and measured H of those sample trees on the riverside. Fifth, the retrieved maximum Acs and the initial Acs of all other trees were combined to acquire the corrected Acs using the correction equation. Finally, the AGBs of sample DR trees for model fitting were calculated using field-measured DBHs and Hs and integrated with the corresponding corrected Acs and measured Hs to construct the novel allometric model. Thus, the AGBs of DR trees in the test site could be achieved by importing the corrected Acs and obtained Hs into the newly constructed allometric model. The entire flow chart is illustrated in Figure 4.
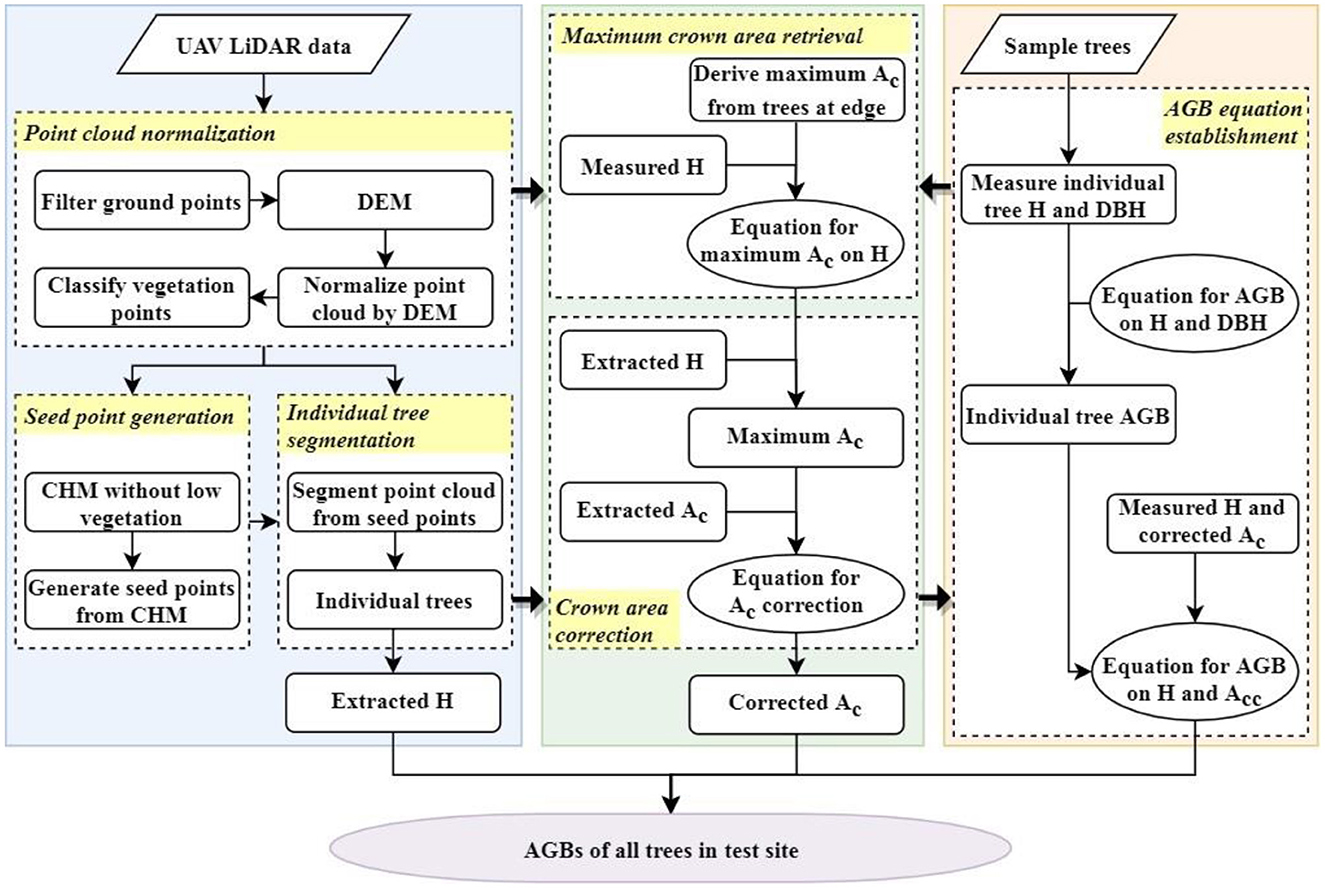
Figure 4. Flow chart of extracting and correcting crown area to precisely estimate AGBs of trees in plantation forest with UAV LiDAR data.
3.1. Point cloud normalization
To eliminate the influence of topographic relief, the values in the Z-axis of non-ground points would be normalized from altitudes relative to sea level to heights relative to the ground. Before normalization, the LiDAR point cloud (Figure 2C) was tailored to the test site and removed noise points. The ground points were separated using the enhanced progressive TIN (triangulated irregular network) densification algorithm (Zhao et al., 2016). The point cloud was first gridded, and the lowest points in grid cells were used as seed points to establish the initial TIN. Then it was densified on a layer-wise basis via iterated processing until each ground point meeting the thresholds of iterative distance and angle (1.4 m and 20°) was found. Subsequently, the resulting ground points were utilized to produce a raster DEM with the IDW (inverse distance weighting) interpolation method. Finally, the normalization was accomplished by subtracting the elevations of the corresponding DEM pixels from those of non-ground points. Then, the normalized points were separated into low, medium, and high vegetation in accordance with the height separation points of 2 m and 5 m (ASPRS, 2005).
3.2. Seed point generation
It will improve the accuracy of segmenting individual trees from the point cloud by using tree tops as seed points. The local maxima filter (Wulder et al., 2000) is the most typical and widely applied method for tree top detection. The idea of this method is to utilize a moving window to search for the points with the local maximum heights as tree tops. In this study, a raster CHM was first produced from the classified vegetation points. In light of field survey and measurement, the heights to crown bases (HCB) of DR trees in the test site were no <2.5 m. Hence, the CHM pixels, which had values lower than 2.5 m, would be deleted to eradicate the low vegetation (shrubs and weeds). Afterward, the seed points were generated by detecting the local maximum heights in the rest of the CHM pixels through a suitable moving window, whose size was determined by the crown size and stand structure characteristics.
3.3. Individual tree segmentation
Individual tree segmentation is the prerequisite for extracting tree-scale parameters, including trunk height and crown area. In this study, the segmentation algorithm developed by Li et al. (2012) was adopted to segment individual trees out of the point cloud acquired by the UAV-borne LiDAR. The points of two neighboring trees were sequentially separated and assigned from top to bottom according to the specified spacing threshold, minimal spacing rule, and planar outlines of tree crowns. It is very critical to appropriately set the spacing threshold. When it is too large or too small, the phenomenon of under-segmentation or over-segmentation will appear. The common practice is to set the maximum crown radius in the test site as the spacing threshold. The seed points generated in the previous subsection were used as individual tree tops. The normalized elevations of these tree tops were actually their Hs. The individual DR trees were eventually segmented, and the point cloud of each tree was separately projected onto a 2D plane to generate the minimum convex hull. The resulting polygon was considered the crown outline, and thus its area was the Ac of the tree.
3.4. Crown area correction
According to the field investigation, the branches of adjacent DR trees in the test site overlapped each other in various degrees at different positions (in the interior or at the edge). As a response, the sizes and shapes of crowns were usually reduced and changed on the parts shaded and squeezed by adjacent crowns. As indicated in Figure 5A, tree T1 was at the edge of the forest (on the riverside), and tree T2 was in the interior. The branches of T1 on the riverside grew unrestricted, but those on the other side seriously overlapped with T2. Although T2 was slightly taller than T1, the Ac of T2 was smaller than that of T1 (Figure 5B). Hence, it was a good practice to correct the initial Acs of trees with reference to the maximum Acs of trees growing under undisturbed conditions. The allometric relationship between the potential maximum Ac and the height of an individual tree needed to be constructed.
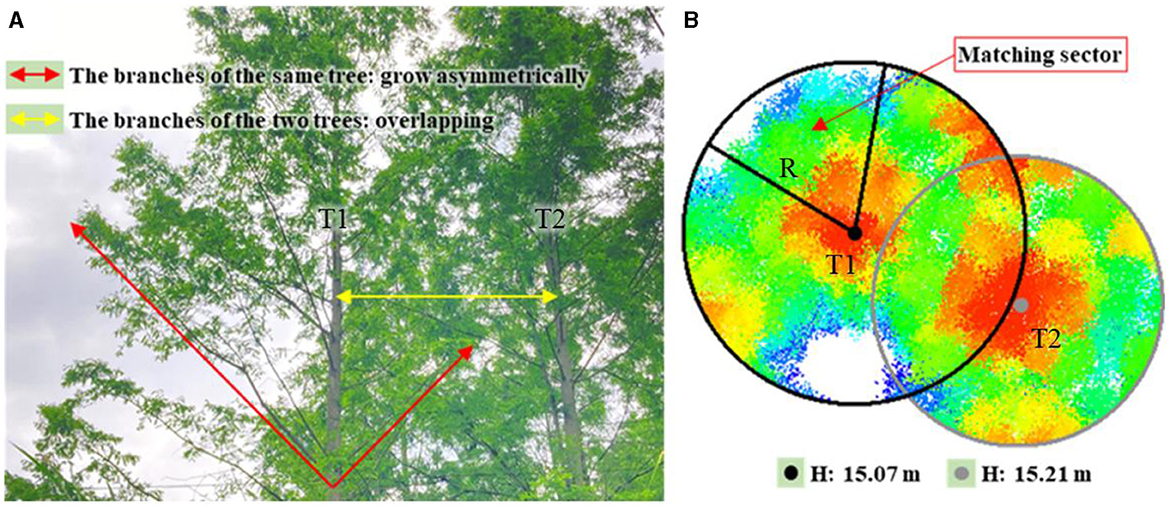
Figure 5. (A) Overlapping crowns of tree T1 at the edge and T2 in the interior of the plantation forest; (B) unrestricted growth part of T1 crown was extracted using a matching sector.
Since there were no DR trees completely undisturbed by neighboring trees in the test site, the maximum Acs could not be directly obtained. As the growth of a tree crown at the forest edge was frequently unrestricted in the outward direction, the outward part of the crown could proportionally derive an entire Ac that would be taken as the potential maximum Ac of the tree. As shown in Figure 5B, the tree top of T1 was used as the center to create a circumcircle covering the entire tree crown, and then the unrestricted growth part of the crown was extracted using a matching sector. Afterward, the potential maximum Ac of T1 could be calculated using Eq. (1).
where Acmax is the potential maximum Ac of a tree (m2), Acs is the area of the unrestricted part of the crown extracted by the matching sector (m2), R represents the radius of the circumcircle (m), and S represents the area of the matching sector (m2).
In this study, the power function (Eq. 2) was determined as the allometric model for retrieving the maximum Ac under undisturbed growth conditions (see section 5.1 for comparisons with other allometric models). By fitting the Hs and calculating the maximum Acs of sample trees (ID: 1–15) on the riverside with Eq. (2), the coefficient values would be determined. Thus, the maximum Acs of all DR trees in the test site could be obtained by entering the extracted Hs into Eq. (2).
where Acmax is the maximum Ac of an individual tree (m2), a and b are model coefficients, and H is the extracted trunk height from the point cloud (m).
Affected by adjacent crowns, the actual crown size of a DR tree would shrink to a certain degree, and the corresponding AGB would be slightly reduced. Therefore, the final Ac that was used to estimate the tree AGB should be a value between the maximum Ac and the initial Ac. Through repeated experiments, Eq. (3) was proposed as the empirical equation for crown area correction and demonstrated sound performance in estimating the AGBs of single DR trees in the test site.
where Ac is the crown area of an individual tree initially extracted from a point cloud (m2), Acc is the corrected Ac of the tree (m2), and Acmax is the potential maximum Ac of the tree (m2).
3.5. Allometric model for tree AGB estimation
According to the biomass models for China's tree species (Luo et al., 2020), the allometric model on DBH and H suitable for calculating the AGB of individual DR trees in the test site is as follows:
where W is single tree AGB (kg), D is trunk DBH (cm), and H is trunk height (m).
Accordingly, the AGB of DR trees (ID: 16–45) for model fitting could be obtained by putting their measured Hs and DBHs into Eq. (4).
As the H could be conveniently obtained from UAV LiDAR data and the Acc could be calculated using Eqs. (2, 3) the quantitative relation between Acc, H, and AGB was required to be established. The power function (Eq. 5) was determined to be the novel allometric model for AGB estimation in the study (see section 5.2 for comparison with other allometric models).
where W is the individual tree AGB (kg), α and β are model coefficients, H is trunk height (m), and Acc is the corrected Ac of the tree (m2).
By fitting the extracted Hs, corrected Acs, and estimated AGBs of sample trees (ID: 16–45) with Eq. (5), model coefficients α and β could be ascertained, and the allometric model with predictors of Acc and H for AGB estimation would be constructed. Hence, the AGB of each DR tree in the test site could be achieved by putting the corrected Ac and extracted H into the new allometric model.
4. Results
4.1. Generated seed points
The noise points were first cleaned from the point cloud of the test site using the software LiDAR360 (https://www.lidar360.com/). Then, the cleaned point cloud was normalized as Figure 6A, which represented the surface characteristics of tree crowns. The two key factors affecting seed point generation were the resolution of CHM and the size of the moving window for filtering the local maxima. Through repeated experiments, the CHM with a resolution of 0.3 m was appropriate for this purpose. With consideration of approximately round crown outlines and the maximum radius of DR tree crowns in the test site, a circle with a radius of 3 m was utilized to detect the local maxima of the CHM and generate seed points. According to the field survey, there were 154 DR trees in the test site. However, in order to avoid over-segmentation (e.g., crown overlap) and under-segmentation (e.g., no gap between trees due to lower vegetation occlusion), 192 local maximum pixels were finally marked as seed points (Figure 6B) to improve the segmentation accuracy.
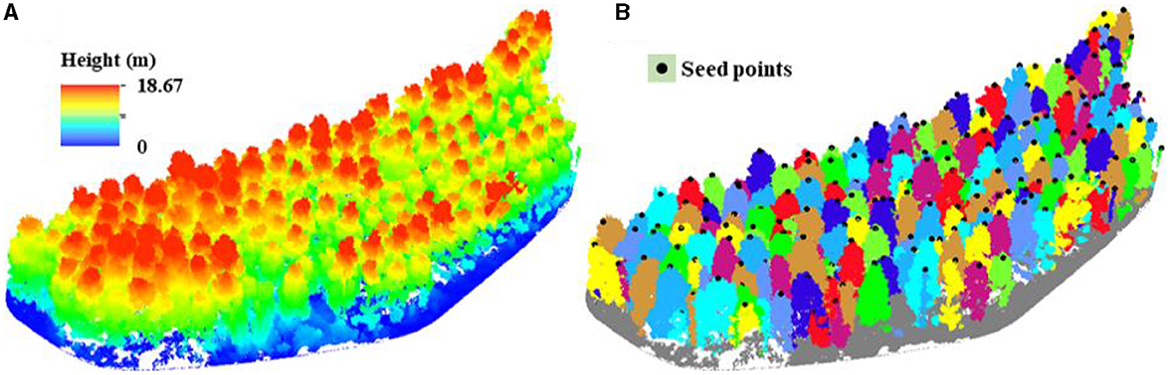
Figure 6. (A) Normalized UAV LiDAR point cloud of the test site; (B) identified individual DR trees with seed points.
4.2. Segmented individual trees
According to field observation and measurement, the Hs, HCBs, and crown radiuses of DR trees in the test site were no <5 m, no <2.5 m, and no more than 3 m, respectively. Therefore, the normalized point cloud was used to segment individual trees based on seed points, with a minimum trunk height of 5 m, an aboveground height of 2.5 m, and a spacing threshold of 3 m. As a result, 154 DR trees in the test site were correctly identified (Figure 6B), and their Hs and initial Acs were also obtained. The extracted Hs ranged from 8.14 m to 16.58 m (Figure 7A), with an average value of 12.74 m. The extracted initial Acs and Hs of DR trees in the test site are listed in Table 2.
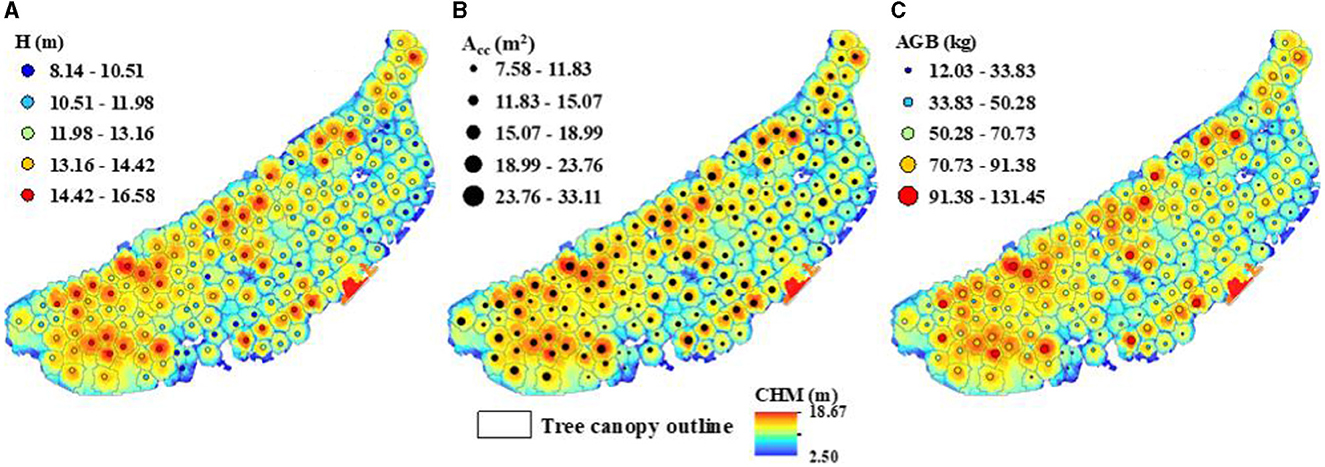
Figure 7. Segmented individual DR trees in the test site: (A) Hs extracted from UAV LiDAR data; (B) Accs calculated on maximum Ac and initial Ac; (C) the AGBs estimated on H and Acc.
4.3. Corrected crown areas
To obtain the potential maximum Acs of sample DR trees (ID: 1–15) on the riverside for crown area correction, the radiuses of their crown circumcircles, the areas of the matching sectors, and the parts of crown areas contained in the matching sectors were input into Eq. (1). The three parameters and the resulting maximum Acs were listed in columns R, S, Acs, and Acmax of Table 3. Then, the calculated maximum Acs and the measured Hs in Table 1 were utilized to fit Eq. (2). The model coefficients a and b were ascertained as 0.2962 and 1.6863, respectively. Thus, the allometric model for retrieving the potential maximum Ac of a DR tree under undisturbed growth conditions based on H was as follows:
where Acmax is the potential maximum Ac of a single tree (m2), and H is the trunk height (m).
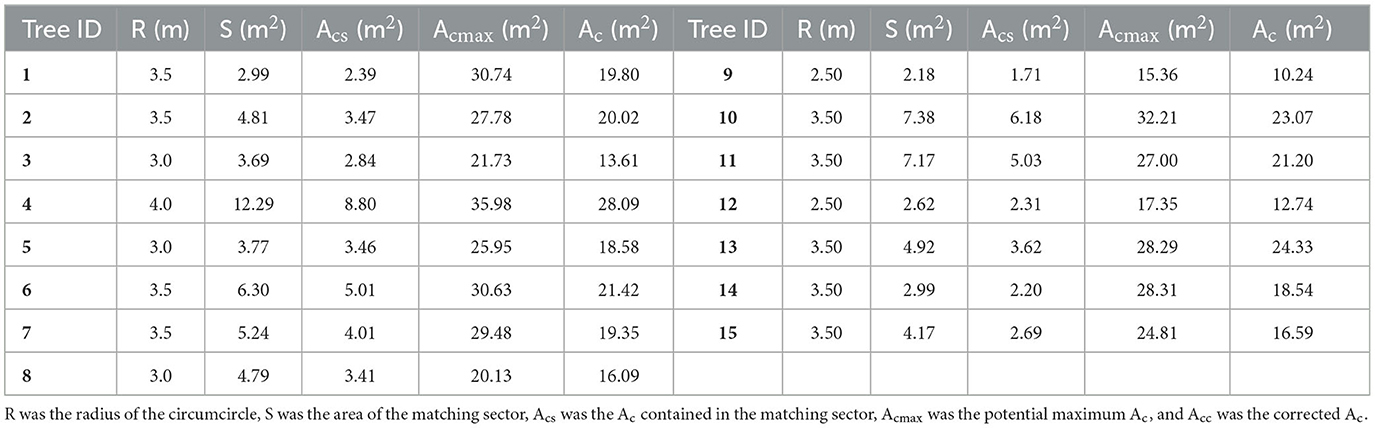
Table 3. R, S, Acs, Acmax, and Ac of sample DR trees (ID: 1–15) on the riverside for crown area correction.
As indicated in Figure 8A, the coefficient of determination (R2) of individual tree Acmaxs derived by Eq. (6) was 0.9572, and root mean square error (RMSE) was 1.22 m2. Since R2 was so close to 1.0 and RMSE was far below the mean Ac of 26.37 m2, the potential maximum Ac and the measured H were soundly fitted.
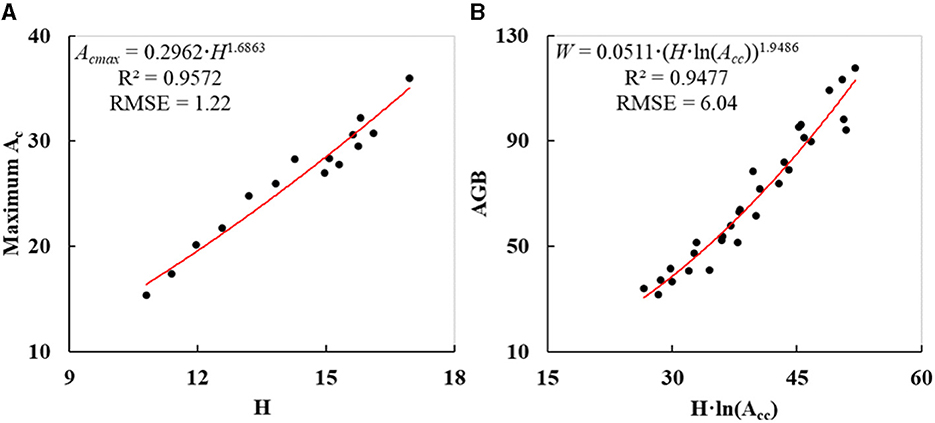
Figure 8. Fitted curves of individual DR trees: (A) potential maximum Ac derived on H; (B) AGB estimated on Acc and H.
The extracted Hs of other DR trees in the test site were input into Eq. (6) to obtain the corresponding maximum Acs. Then the calculated maximum Acs and the initial Acs extracted by individual tree segmentation were input into Eq. (3) to obtain the finally corrected Acs of all DR trees in the test site (Figure 7B). The corrected Acs varied from 7.58 m2 to 33.11 m2, with a mean value of about 17.32 m2. The maximum Acs and corrected Acs of sample trees (ID: 16–60) were listed in columns Acmax and Acc of Table 4, respectively. Compared with the initially extracted Ac, the corrected Ac increased by 1.29 m2 on average.
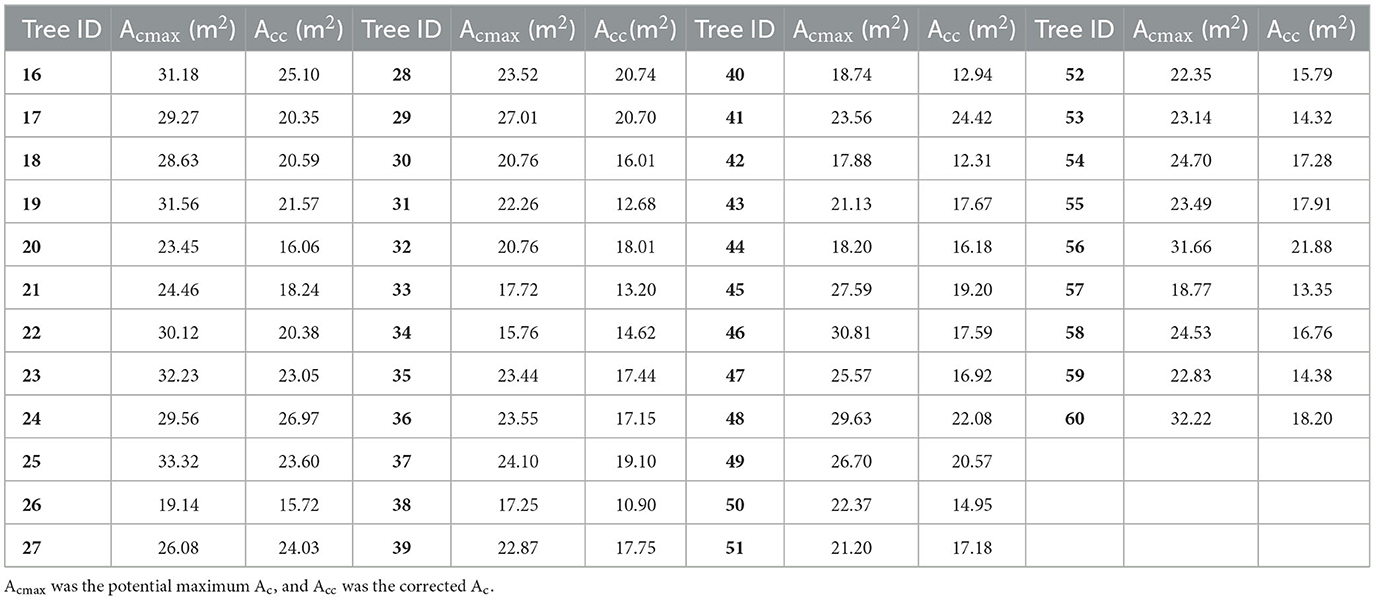
Table 4. Acmax and Acc of sample DR trees for model fitting (ID: 16–45) and accuracy evaluation (ID: 46–60).
4.4. Estimated AGBs
The AGBs of DR trees in Table 5 for model fitting were first calculated with Eq. (4) based on the measured DBHs and Hs in Table 1. Then, the estimated AGBs and their corresponding measured Hs in Table 1 and the corrected Ac in Table 4 were utilized to fit Eq. (5). The model coefficients α and β were ascertained as 0.0511 and 1.9486, respectively. Thus, the allometric model for estimating the DR tree AGB according to corrected Ac and H was as follows:
where W is the tree AGB (kg), H is the trunk height (m), and Acc is the corrected Ac of the tree (m2).
As indicated in Figure 8B, R2 and RMSE of individual tree AGBs calculated by Eq. (7) were 0.9477 and 6.04 kg, respectively. Since R2 was so close to 1.0 and RMSE was far below the mean AGB of 68.36 kg, the calculated AGB and the product of H and logarithmic Acc were soundly fitted. Then, the extracted Hs and corrected Acs of other DR trees were input into Eq. (7) to obtain their AGBs. The resulting AGBs varied from 12.03 kg to 131.45 kg (Figure 7C) with a mean value of 58.06 kg, and the AGB of DR trees in the test site was nearly 8.9 t in total.
4.5. Accuracy evaluation
In this study, the accuracy of trunk Hs extracted from the point cloud scanned by UAV-borne LiDAR was first evaluated. The Hs of sample DR trees (Figure 3C) are shown in Table 2, and the corresponding measured Hs are shown in Table 1. As indicated in Figure 9A, R2 and RMSE of the resulting Hs were 0.9688 and 0.51 m, implying that the Hs extracted from UAV LiDAR data had sound accuracy. The Acs of sample DR trees that were initially extracted and subsequently corrected are listed in Tables 2, 4, respectively.
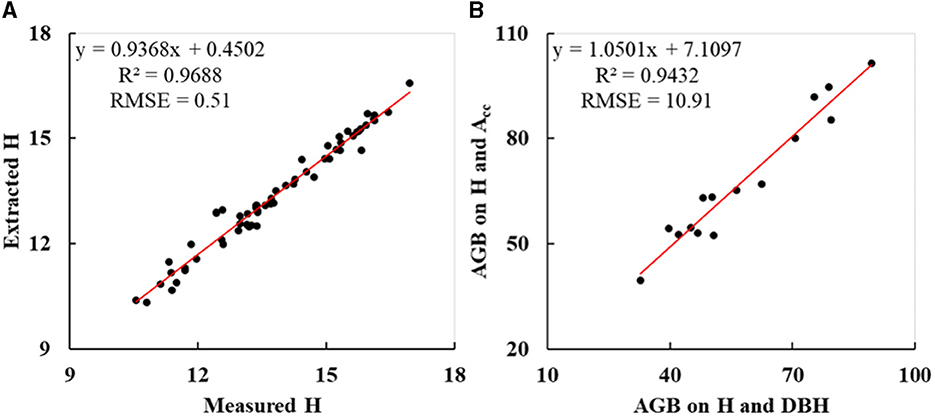
Figure 9. Accuracy evaluation of sample DR trees: (A) Hs extracted from UAV LiDAR data; (B) AGBs estimated on H and Acc.
For the purpose of evaluating the accuracy of calculated AGBs by the newly constructed allometric model on corrected Ac and H, the AGBs of sample DR trees (ID: 46–60) were calculated using Eqs. (4) and (7), respectively (Table 6). As indicated in Figure 9B, R2 and RMSE were 0.9432 and 10.91 kg, implying a high correlation between the AGB calculated on Acc and H and that on DBH and H.
5. Discussion
5.1. Relationship between trunk height and crown area
In the study, the maximum Ac of a tree referred to the crown area of the tree that grew under undisturbed conditions and was used to correct the initial Ac to mitigate the impacts from adjacent trees. As the potential maximum Acs of most trees could not be directly obtained, the power function with trunk height (H) as the base (Eq. 6) was suggested to retrieve them in section 3.4. It was based on the fact that the crown area of a tree exhibited a certain allometric relationship with H under undisturbed growth conditions (Enquist and Niklas, 2001; Hulshof et al., 2015; Shi and Liu, 2017). The fitting data for the maximum Ac retrieval model were frequently collected from the trees at the edge or in sparsely distributed parts of the plantation forest. Actually, the other three possible retrieval models for the maximum Ac with the variable of “H” had also been tested, comprising exponential function, linear function, and logarithmic function. The measured Hs in Table 1 and the derived maximum Acs in Table 3 of sample trees (ID: 1–15) were utilized to fit them separately. The fitting degree has a positive correlation with the value of R2. The fitted curves are indicated in Figures 10A–C, and the resulting R2 were 0.9433, 0.954, and 0.9545. R2 of all models demonstrated a good positive relationship between potential maximum Ac and H, which was in accordance with other studies (Liu et al., 2019; Zhao et al., 2021). With the highest R2, the power function model adopted in this study (Eq. 6) was optimal for expressing the allometric growth relationship between the potential maximum Ac and H.
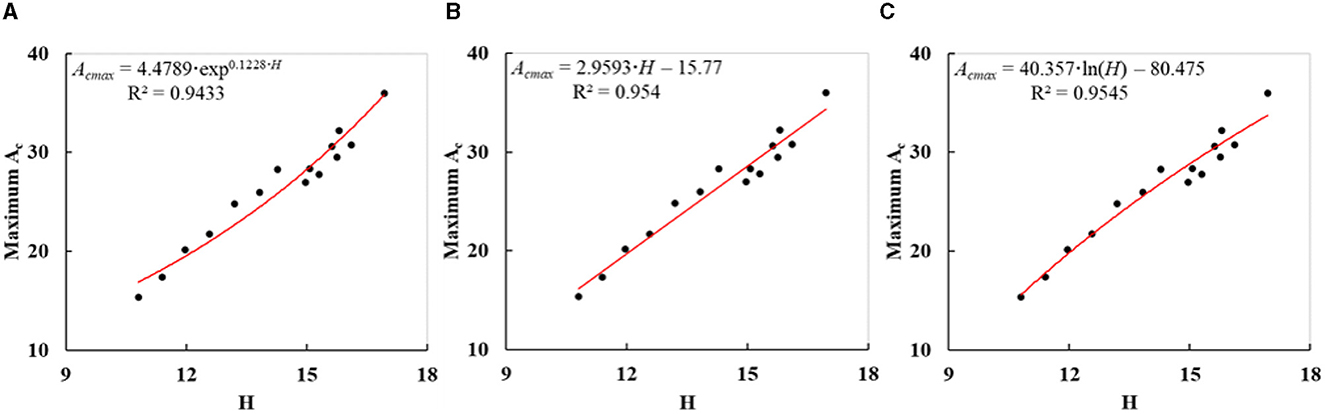
Figure 10. Fitted curves (A–C) of three other allometric models for retrieving the potential maximum Ac (Acmax) based on H.
5.2. Comparison of various allometric models for tree AGB estimation
To achieve a precise AGB calculation of plantation trees, it is significant to construct a suitable allometric model with predictors that could be obtained by UAV-borne LiDAR. According to section 3.5, the power function with “H · ln(Acc)” as the base was suggested as the allometric model. In fact, nine other potential allometric models were also experimented. The field-measured Hs in Table 1, initial Acs in Table 2 or corrected Acs in Table 4, and calculated AGBs in Table 5 of sample DR trees (ID: 16–45) (Figure 3C) were utilized to fit them separately. The allometric equation with the single predictor of H was suggested by Lin et al. (2018). The fitted curve is illustrated in Figure 11A with the R2 of 0.928. As shown in Figure 11B, the initial Ac was replaced by the corrected Ac to fit Eq. (5), and the R2 of the fitted curve was 0.9184. With regard to the power functions that depended on Acc and H, a few more compositions were tried as the bases comprising “H·Acc”, “H+Acc”, “H+ln(Acc)”, and “H·(ln(Acc))2”. The fitted curves are indicated in Figures 11C–F, and the resulting R2 were 0.9063, 0.8903, 0.9451, and 0.922. In addition, we fitted several other classical function models with the same base of “H·ln(Acc)” comprising exponential function, linear function, and logarithmic function. The fitted curves are indicated in Figures 11G–I, and the resulting R2 were 0.9449, 0.9364, and 0.9133. Since the R2 of the fitted curve in Figure 8B was 0.9477, the applied allometric model (Eq. 7) had the highest degree of model fitting. Compared with Figure 11B, the corrected Ac exhibited better performance in estimating AGBs of plantation forest trees than the uncorrected Ac. Additionally, three other allometric models in Figures 11E, G, H had a larger R2 than the allometric model in Figure 11A. They demonstrated again that the AGB calculation of plantation trees on Acc and H outperformed that depending solely on H.
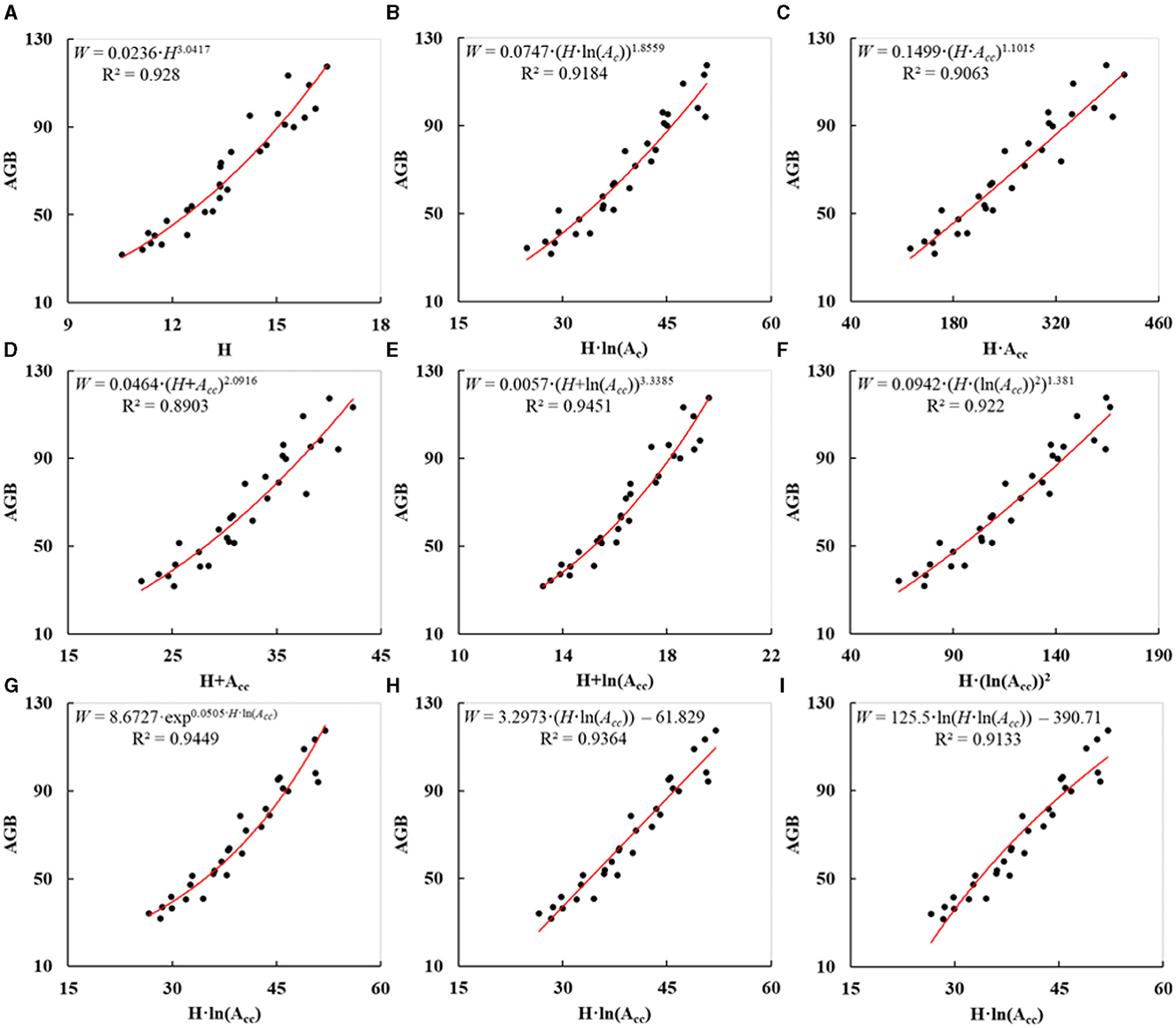
Figure 11. Fitted curves (A–I) of nine other allometric models for estimating AGBs of plantation trees.
5.3. Error analysis and possible enhancements
Though UAV-borne LiDAR was employed to obtain precise AGB estimation of DR trees in a plantation forest, certain factors may have affected the accuracy. For example, due to the fear of hitting high buildings near the test site (see Figure 2C), the aboveground flight altitude of the UAV was preset to 80 m. It would inevitably reduce the elevation accuracy of the point cloud scanned by LiDAR, ultimately affecting the accuracy of tree segmentation and height extraction. The measured DBHs and Hs of sample DR trees, which were taken as the truths for model fitting and verification, possibly had some systematic errors owing to the instrument quality and usage procedure. According to section 3.4, the crowns of those trees on the riverside were used to derive the potential maximum Acs under undisturbed growth conditions. However, the unrestricted part of the crowns accounted for only a small part of the entire crowns, inevitably bringing certain errors to the resulting data. The AGBs of the sample DR trees were possibly not precisely achieved since we directly applied the allometric model and the corresponding coefficient values from the reference.
Despite all this, certain steps can be adopted to enhance the AGB estimation precision of plantation trees in specific application scenarios. For instance, the UAV flight altitude can be lowered to 40 m through fine operations on the premise of safety, thus improving the elevation precision of the resulting point cloud. The errors of field-measured data can be minimized by using standardized procedures and using the average value of multiple measurements. If possible, the sample trees whose entire crowns or the majority of crowns grew unrestricted should be selected to calculate the potential maximum Acs. The coefficient values of the adopted allometric model on DBH and H can be ascertained via practical tests, rather than straightforward empirical ones for the DR species.
6. Conclusion
In this article, the UAV LiDAR data were utilized to extract and correct crown area for the precise AGB estimation of trees in a plantation forest. The following were the principal conclusions we drew:
• The approach of extracting and correcting Ac extracted from the UAV LiDAR data to precisely estimate the AGBs of plantation forest trees proved effective. The R2 and RMSE of resulting AGBs of DR trees in the test site were 0.9432 and 10.91 kg.
• The unrestricted growth parts of the tree crowns at the edge of a plantation forest could be used to derive the potential maximum Ac. The power function model demonstrated the highest fitting degree between the potential maximum Ac and H, and the R2 and RMSE were 0.9572 and 1.22 m2, respectively.
• Compared with the allometric models for AGB estimation depending only on trunk H or on initial Ac and H, the novel allometric model proposed in this study was based on the predictor variables of corrected Ac and H and demonstrated superior performance in estimating the AGBs of trees in a plantation forest.
Though this study offers the applicable methodology to calculate the AGBs of individual DR trees in a plantation forest using UAV-borne LiDAR, certain reasons still impacted the precision, including excessive UAV flight altitude, systematic measurement errors, a lack of undisturbed crowns, and non-localized coefficients of the allometric model. Hence, it is always a real challenge to precisely achieve the AGBs of plantation trees with UAV LiDAR data.
Additionally, the crown area of a single tree in a plantation forest is mainly determined by tree spacing, species, and age. Therefore, the suitable spacing between trees should be kept while planting trees, and the crown overlap will be reduced and the trees will grow lusher. It also prevents the underneath branches and leaves from withering due to the lack of sunlight. Thus, the total AGBs of plantation forest trees will increase, and more carbon will be stored. Hence, it is very meaningful to study how to plan suitable spacing for the plantation forest trees to maximize the AGB and carbon sequestration. Meanwhile, the allometric relationship between DBH and the potential maximum Ac and the allometric models for AGB calculation of different tree species at various ages also need to be studied in future.
Data availability statement
The original contributions presented in the study are included in the article/supplementary material, further inquiries can be directed to the corresponding author.
Author contributions
JL: conceptualization, formal analysis, funding acquisition, investigation, methodology, writing—original draft, and writing—review and editing. DC: data curation, investigation, methodology, and writing—original draft. SY and XL: data curation and investigation. All authors read and approved the final version of the manuscript.
Funding
This study was partially sponsored by the National Natural Science Foundation of China (nos. 32071678 and 41830648) and the Key Research and Development Program of the Sichuan Province (no. 2022YFQ0035).
Conflict of interest
The authors declare that the research was conducted in the absence of any commercial or financial relationships that could be construed as a potential conflict of interest.
Publisher's note
All claims expressed in this article are solely those of the authors and do not necessarily represent those of their affiliated organizations, or those of the publisher, the editors and the reviewers. Any product that may be evaluated in this article, or claim that may be made by its manufacturer, is not guaranteed or endorsed by the publisher.
References
Allouis, T., Durrieu, S., Vega, C., and Couteron, P. (2011). “Exploiting fullwavefrom lidar signals to estimate timber volume and above-ground biomass of individual trees,” in 2011 IEEE International Geoscience and Remote Sensing Symposium. Vancouver, BC: IEEE, 1251–1254.
Alvarez, E., Duque, A., Saldarriaga, J., Cabrera, K., and Salas, G. D. (2012). Tree above-ground biomass allometries for carbon stocks estimation in the natural forests of Colombia. For. Ecol. Manage. 267, 297–308. doi: 10.1016/j.foreco.2011.12.013
ASPRS (2005). LAS 1, 4. Specification Approved by ASPRS Board. Available online at: https://www.asprs.org/divisions-committees/lidar-division/laser-las-file-format-exchange-activities (accessed June 26, 2022).
Beland, M., Parker, G., Sparrow, B., Harding, D., Chasmer, L., Phinn, S., et al. (2019). On promoting the use of lidar systems in forest ecosystem research. For. Ecol. Manage. 450, 117484. doi: 10.1016/j.foreco.2019.117484
Blujdea, V. N. B., Pilli, R., Dutca, I., Ciuvat, L., and Abrudan, I. V. (2012). Allometric biomass equations for young broadleaved trees in plantations in Romania. For. Ecol. Manage. 264, 172–184. doi: 10.1016/j.foreco.2011.09.042
Chave, J., Réjou-Méchain, M., Búrquez, A., Chidumayo, E., Colgan, M. S., Delitti, W. B. C., et al. (2014). Improved allometric models to estimate the aboveground biomass of tropical trees. Glob. Change Biol. 20, 3177–3190. doi: 10.1111/gcb.12629
Chen, D. M., Zhang, C. L., Wu, J. P., Zhou, L. X., Lin, Y. B., Fu, S. L., et al. (2011). Subtropical plantations are large carbon sinks: evidence from two monoculture plantations in South China. Agric. For. Meteorol. 151, 1214–1225. doi: 10.1016/j.agrformet.2011.04.011
Chen, Q., Laurin, G. V., and Valentini, R. (2015). Uncertainty of remotely sensed aboveground biomass over an African tropical forest: propagating errors from trees to plots to pixels. Remote Sens. Environ. 160, 134–143. doi: 10.1016/j.rse.2015.01.009
Chen, X. X., Jiang, K., Zhu, Y. S., Wang, X. J., and Yun, T. (2021). Individual tree crown segmentation directly from UAV-borne LiDAR data using the PointNet of deep learning. Forests 12, 131. doi: 10.3390/f12020131
Chen, Z., Dayananda, B., Fu, B., Li, Z. W., Jia, Z. Y., Hu, Y., et al. (2022). Research on the potential of forestry's carbon-neutral contribution in China from 2021 to 2060. Sustainability 14, 5444. doi: 10.3390/su14095444
Diao, J. J., Liu, J. X., Zhu, Z. L., Wei, X. Y., and Li, M. S. (2022). Active forest management accelerates carbon storage in plantation forests in Lishui, southern China. For. Ecosyst. 9, 100004. doi: 10.1016/j.fecs.2022.100004
Dubayah, R. O., Sheldon, S. L., Clark, D. B., Hofton, M. A., Blair, J. B., Hurtt, G. C., et al. (2010). Estimation of tropical forest height and biomass dynamics using lidar remote sensing at La Selva, Costa Rica. J. Geophys. Res.-Biogeosci. 115, G00E09. doi: 10.1029/2009JG000933
Dube, T., and Mutanga, O. (2016). The impact of integrating WorldView-2 sensor and environmental variables in estimating plantation forest species aboveground biomass and carbon stocks in uMgeni Catchment, South Africa. ISPRS-J. Photogramm. Remote Sens. 119, 415–425. doi: 10.1016/j.isprsjprs.2016.06.017
Enquist, B. J., and Niklas, K. J. (2001). Invariant scaling relations across tree-dominated communities. Nature 410, 655–660. doi: 10.1038/35070500
Fang, J. Y., Chen, A. P., Peng, C. H., Zhao, S. Q., and Ci, L. (2001). Changes in forest biomass carbon storage in China between 1949 and 1998. Science 292, 2320–2322. doi: 10.1126/science.1058629
FAO (2020). Global Forest Resources Assessment 2020. Available online at: https://www.fao.org/forest-resources-assessment/2020/en/ (accessed June 26, 2022).
Fu, L. Y., Liu, Q. W., Sun, H., Wang, Q. Y., Li, Z. Y., Chen, E. X., et al. (2018). Development of a system of compatible individual tree diameter and aboveground biomass prediction models using error-in-variable regression and airborne LiDAR data. Remote Sens. 10, 325. doi: 10.3390/rs10020325
Gong, P., Li, Z., Huang, H. B., Sun, G. Q., and Wang, L. (2011). ICESat GLAS data for urban environment monitoring. IEEE Trans. Geosci. Remote Sensing 49, 1158–1172. doi: 10.1109/TGRS.2010.2070514
Goodman, R. C., Phillips, O. L., Torres, D. D., Freitas, L., Cortese, S. T., Monteagudo, A., et al. (2013). Amazon palm biomass and allometry. For. Ecol. Manage. 310, 994–1004. doi: 10.1016/j.foreco.2013.09.045
Guo, S., Zhao, H. B., Zhou, G. Y., Long, W. G., Gan, G. L., Wu, S. Y., et al. (2022). Biomass allocation of different species plantations in subtropical area of China. For. Res. 35, 182–189.
Hilker, T., van Leeuwen, M., Coops, N. C., Wulder, M. A., Newnham, G. J., Juup, D. L. B., et al. (2010). Comparing crown metrics derived from terrestrial and airborne laser scanning in a Douglas-fir dominated forest stand. Trees 24, 819–832. doi: 10.1007/s00468-010-0452-7
Hu, T. Y., Su, Y. J., Xue, B. L., Liu, J., Zhao, X. Q., Fang, J. Y., et al. (2016). Mapping global forest aboveground biomass with spaceborne LiDAR, optical imagery, and forest inventory data. Remote Sens. 8, 565. doi: 10.3390/rs8070565
Hulshof, C. M., Swenson, N. G., and Weiser, M. D. (2015). Tree height-diameter allometry across the United States. Ecol. E. 5, 1193–1204. doi: 10.1002/ece3.1328
Jiang, R., Lin, J. Y., and Li, T. X. (2022). Refined aboveground biomass estimation of moso bamboo forest using culm lengths extracted from TLS POINT CLOUD. Remote Sens. 14, 5537. doi: 10.3390/rs14215537
Jiang, X. D., Li, G. Y., Lu, D. S., Chen, E. X., and Wei, X. L. (2020). Stratification-based forest aboveground biomass estimation in a subtropical region using airborne lidar data. Remote Sens. 12, 1101. doi: 10.3390/rs12071101
Justine, M. F., Yang, W. Q., Wu, F. Z., Tan, B., Khan, M. N., Zhao, Y. Y., et al. (2015). Biomass stock and carbon sequestration in a chronosequence of Pinus massoniana plantations in the upper reaches of the Yangtze River. Forests 6, 3665–3682. doi: 10.3390/f6103665
Kuyah, S., Muthuri, C., Jamnadass, R., Mwangi, P., Neufeldt, H., Dietz, J., et al. (2012). Crown area allometries for estimation of aboveground tree biomass in agricultural landscapes of western Kenya. Agrofor. Syst. 86, 267–277. doi: 10.1007/s10457-012-9529-1
Lal, R. (2005). Forest soils and carbon sequestration. For. Ecol. Manage. 220, 242–258. doi: 10.1016/j.foreco.2005.08.015
Li, L., Guo, Q. H., and Xu, G. C. (2015). Lidar with multi-temporal MODIS provide a means to upscale predictions of forest biomass. ISPRS-J. Photogramm. Remote Sens. 102, 198–208. doi: 10.1016/j.isprsjprs.2015.02.007
Li, W. K., Guo, Q. H., Jakubowski, M. K., and Kelly, M. (2012). A new method for segmenting individual trees from the lidar point cloud. Photogramm. Eng. Remote Sens. 78, 75–84. doi: 10.14358/PERS.78.1.75
Li, Z. Y., Liu, Q. W., and Pang, Y. (2016). Review on forest parameters inversion using LiDAR. J. Remote Sens. 20, 1138–1150. doi: 10.11834/jrs.20165130
Lin, J. Y., Chen, D. C., Wu, W. J., and Liao, X. H. (2022). Estimating aboveground biomass of urban forest trees with dual-source UAV acquired point clouds. Urban Gree. 69, 127521. doi: 10.1016/j.ufug.2022.127521
Lin, J. Y., Wang, M. M., Ma, M. G., and Lin, Y. (2018). Aboveground tree biomass estimation of sparse subalpine coniferous forest with UAV oblique photography. Remote Sens. 10, 1849. doi: 10.3390/rs10111849
Liu, H. R., Fan, W. W., Xu, Y. S., and Lin, W. S. (2021). Single tree biomass estimation based on UAV LiDAR point cloud. J. Cent. South Univ. For. Technol. 41, 92–99.
Liu, K., Shen, X., Cao, L., Wang, G. B., and Cao, F. L. (2018). Estimating forest structural attributes using UAV-LiDAR data in Ginkgo plantations. ISPRS-J. Photogramm. Remote Sens. 146, 465–482. doi: 10.1016/j.isprsjprs.2018.11.001
Liu, Z. J., Guan, Y. Y., Xu, Y. L., and Xu, Y. (2019). Analysis on structure feature of Metasequoia glyptostroboides and Ginkgo biloba standing tree in urban area of Qingdao City Guangxi. For. Sci. 48, 127–132.
Lu, D. S., Chen, Q., Wang, G. X., Liu, L. J., Li, G. Y., Moran, E., et al. (2014). A survey of remote sensing-based aboveground biomass estimation methods in forest ecosystems. Int. J. Digit. Earth 9, 63–105. doi: 10.1080/17538947.2014.990526
Lu, J. B., Wang, H., Qin, S. H., Cao, L., Pu, R. L., Li, G. L., et al. (2020). Estimation of aboveground biomass of Robinia pseudoacacia forest in the Yellow River Delta based on UAV and Backpack LiDAR point clouds. Int. J. Appl. Earth Obs. Geoinf. 86, 102014. doi: 10.1016/j.jag.2019.102014
Lu, X. M., Zheng, G., Miller, C., and Alvarado, E. (2017). Combining multi-source remotely sensed data and a process-based model for forest aboveground biomass updating. Sensors 17, 2062. doi: 10.3390/s17092062
Lun, F., Liu, Y., He, L., Yang, L., Liu, M. C., Li, W. H., et al. (2018). Life cycle research on the carbon budget of the Larix principis-rupprechtii plantation forest ecosystem in North China. J. Clean Prod. 177, 178–186. doi: 10.1016/j.jclepro.2017.12.126
Luo, Y. J., Wang, X. K., Ouyang, Z. Y., Lu, F., Feng, L. G., Tao, J., et al. (2020). A review of biomass equations for China's tree species. Earth Syst. Sci. Data 12, 21–40. doi: 10.5194/essd-12-21-2020
Ma, J. S. (2007). A worldwide survey of cultivated Metasequoia glyptostroboides Hu and Cheng (Taxodiaceae: Cupressaceae) from 1947 to 2007. Bull. Peabody Mus. Natl. Hist. 48, 235–253. doi: 10.3374/0079-032X(2007)48(235:AWSOCM)2.0.CO;2
Ma, K. S., Chen, Z. X., Fu, L. Y., Tian, W. L., Jiang, F. G., Yi, J., et al. (2022). Performance and sensitivity of individual tree segmentation methods for UAV-LiDAR in multiple forest types. Remote Sens. 14, 298. doi: 10.3390/rs14020298
Ming, A. G., Jia, H. Y., Tao, Y., Lu, L. H., Su, J. M., Shi, Z. M., et al. (2012). Biomass and its allocation in a 28-year-old Mytilaria laosensis plantation in southwest Guangxi. Chin. J. Ecol. 31, 1050–1056.
Nie, S., Wang, C., Zeng, H. C., Xi, X. H., and Li, G. C. (2017). Above-ground biomass estimation using airborne discrete-return and full-waveform LiDAR data in a coniferous forest. Ecol. Indic. 78, 221–228. doi: 10.1016/j.ecolind.2017.02.045
Paul, K. I., Roxburgh, S. H., Chave, J., England, J. R., Zerihun, A., Specht, A., et al. (2016). Testing the generality of above-ground biomass allometry across plant functional types at the continent scale. Glob. Change Biol. 22, 2106–2124. doi: 10.1111/gcb.13201
Popescu, S. C. (2007). Estimating biomass of individual pine trees using airborne lidar. Biomass Bioenerg. 31, 646–655. doi: 10.1016/j.biombioe.2007.06.022
Popescu, S. C., Wynne, R. H., and Nelson, R. F. (2002). Estimating plot-level tree heights with lidar: local filtering with a crown-height based variable window size. Comput. Electron. Agric. 37, 71–95. doi: 10.1016/S0168-1699(02)00121-7
Shi, L., and Liu, S. R. (2017). Methods of estimating forest biomass: a review. Biomass Vol. Estim. Valoriz. Energ. 12, 23–46. doi: 10.5772/65733
Su, H. Y., Shen, W. J., Wang, J. R., Ali, A., and Li, M. S. (2020). Machine learning and geostatistical approaches for estimating aboveground biomass in Chinese subtropical forests. For. Ecosyst. 7, 64. doi: 10.1186/s40663-020-00276-7
Sun, G., Ranson, K. J., Guo, Z., Zhang, Z., Montesano, P., Kimesb, D., et al. (2011). Forest biomass mapping from lidar and radar synergies. Remote Sens. Environ. 115, 2906–2916. doi: 10.1016/j.rse.2011.03.021
Wallace, L., Musk, R., and Lucieer, A. (2014). An assessment of the repeatability of automatic forest inventory metrics derived from UAV-borne laser scanning data. IEEE Trans. Geosci. Remote Sensing 52, 7160–7169. doi: 10.1109/TGRS.2014.2308208
Wang, D. Z., Wan, B., Liu, J., Su, Y. J., Guo, Q. H., Qiu, P. H., et al. (2020). Estimating aboveground biomass of the mangrove forests on northeast Hainan Island in China using an upscaling method from field plots, UAV-LiDAR data and Sentinel-2 imagery. Int. J. Appl. Earth Obs. Geoinf. 85, 101986. doi: 10.1016/j.jag.2019.101986
Wan-Mohd-Jaafar, W. S., Woodhouse, I. H., Silva, C. A., Omar, H., and Hudak, A. T. (2017). Modelling individual tree aboveground biomass using discrete return lidar in lowland dipterocarp forest of Malaysia. J. Trop. For. Sci. 29, 465–484. doi: 10.26525/jtfs2017.29.4.465484
White, J. C., Coops, N. C., Wulder, M. A., Vastaranta, M., Hilker, T., Tompalski, P., et al. (2016). Remote Sens. technologies for enhancing forest inventories: a review. Can. J. Remote Sens. 42, 619–641. doi: 10.1080/07038992.2016.1207484
Wu, B., Yu, B. L., Yue, W. H., Shu, S., Tan, W. Q., Hu, C. L., et al. (2013). A voxel-based method for automated identification and morphological parameters estimation of individual street trees from mobile laser scanning data. Remote Sens. 5, 584–611. doi: 10.3390/rs5020584
Wu, X., Shen, X., Cao, L., Wang, G., and Cao, F. (2019). Assessment of individual tree detection and crown cover estimation using unmanned aerial vehicle based light detection and ranging (UAV-LiDAR) data in planted forests. Remote Sens. 11, 908. doi: 10.3390/rs11080908
Wulder, M., Niemann, K. O., and Goodenough, D. G. (2000). Local maximum filtering for the extraction of tree locations and basal area from high spatial resolution imagery. Remote Sens. Environ. 73, 103–114. doi: 10.1016/S0034-4257(00)00101-2
Xu, D. D., Wang, H. B., Xu, W. X., Luan, Z. Q., and Xu, X. (2021). LiDAR applications to estimate forest biomass at individual tree scale: opportunities, challenges and future perspectives. Forests 12, 550. doi: 10.3390/f12050550
Yu, Y. F., Song, T. Q., Zeng, F. P., Peng, W. X., Wen, Y. G., Huang, C. B., et al. (2013). Dynamic changes of biomass and its allocation in Cunninghamia lanceolata plantations of different stand ages. Chin. J. Ecol. 32, 1660–1666.
Zhao, B. G., Zhu, J., Ai, X. R., Yao, L., Guo, Q. J., Hong, J. F., et al. (2021). Path analysis of DBH and crown width of native Metasequoia glyptostroboides population. J. Northeast For. Univ. 49, 16–20.
Zhao, X. Q., Guo, Q. H., Su, Y. J., and Xue, B. L. (2016). Improved progressive TIN densification filtering algorithm for airborne LiDAR data in forested areas. ISPRS-J. Photogr. Remote Sens. 117, 79–91. doi: 10.1016/j.isprsjprs.2016.03.016
Keywords: plantation forest, aboveground biomass (AGB), UAV-borne LiDAR, allometric model, trunk height, crown area
Citation: Lin J, Chen D, Yang S and Liao X (2023) Precise aboveground biomass estimation of plantation forest trees using the novel allometric model and UAV-borne LiDAR. Front. For. Glob. Change 6:1166349. doi: 10.3389/ffgc.2023.1166349
Received: 25 May 2023; Accepted: 04 September 2023;
Published: 06 October 2023.
Edited by:
Thomas J. Dean, Louisiana State University, United StatesReviewed by:
Pinliang Dong, University of North Texas, United StatesCory Garms, Spectral Sciences, United States
Copyright © 2023 Lin, Chen, Yang and Liao. This is an open-access article distributed under the terms of the Creative Commons Attribution License (CC BY). The use, distribution or reproduction in other forums is permitted, provided the original author(s) and the copyright owner(s) are credited and that the original publication in this journal is cited, in accordance with accepted academic practice. No use, distribution or reproduction is permitted which does not comply with these terms.
*Correspondence: Jiayuan Lin, am9leWxpbkBzd3UuZWR1LmNu