- 1Scientific Laboratory of Forest Health, Reshetnev Siberian State University of Science and Technology, Krasnoyarsk, Russia
- 2Faculty of Silviculture and Forest Engineering, Transilvania University of Brasov, Brasov, Romania
- 3Forest Research Institute (FRI), University of Sopron (UOS), Sárvár, Hungary
- 4Sukachev Institute of Forest SB RAS, Federal Research Center, Krasnoyarsk Science Center SB RAS, Krasnoyarsk, Russia
- 5Branch of the Russian Centre of Forest Health – Centre of Forest Health of Krasnoyarsk krai, Krasnoyarsk, Russia
Scots pine (Pinus sylvestris L.) is a widespread and economically important tree species in Eurasia. Southern Siberian, Scots pine mountain and foothills forests are especially valuable natural objects that help to stabilize environmental conditions. Due to the associated impact of natural and anthropogenic factors, the habitats of the species in southern Siberia are constantly shrinking. In this regard, the study of genetic diversity and structure of Scots pine forests in southern Siberia is very relevant. Here, we studied the genetic diversity and structure of eight Scots pine populations located in southern Middle Siberia (Russia). A high level of genetic diversity (HE = 0.518) was detected in the studied populations. No recent bottleneck effect, isolation by distance or isolation-by-environment were detected. Most genetic diversity was found within populations, while only 7% of genetic diversity occurred among populations. Both STRUCTURE analysis and UPGMA clustering showed two genetic groups. Two populations from the Minusink basin and a population from the Western Sayan Mts. formed the first group and the second group was composed of the other populations from Kuznetsk Alatau Mts., Central Tuva basin and Todzha basin. Our findings suggest that the studied Scots pine populations originate from different gene pools. The pattern of genetic diversity revealed by our study may be useful for the elaboration of conservation measures of genetic resources of Scots pine in southern Middle Siberia.
1. Introduction
Genetic diversity is recognized as one of three basic components of biodiversity, i.e., the genes, species and ecosystems (Hoban et al., 2020). It plays an important role in species adaption to changing climate, habitats, and biotic interactions (Spielman et al., 2004). Furthermore, it helps to maintain ecosystem functions, stability and services (Hoban et al., 2020). The characterization of genetic diversity pattern within species and among populations is a fundamental requirement for the establishment of programs aimed at biodiversity preservation (Belletti et al., 2017).
Scots pine (Pinus sylvestris L.) is one of the most widespread conifers in the world, which has great economic and ecological importance (Floran et al., 2011). Within its vast distribution, Scots pine grows in various soil and climatic conditions. It forms over 20 geographical races and about 100 forms and varieties (Ekart et al., 2014). A significant part of its range is located on the territory of Siberia where permafrost and forest fires determine the northern and southern boundaries of the species’ area of distribution. At the same time, the impact of fires has not only negative, but also positive consequences; it ensures the removal of ground cover that prevents the germination of pine seeds, as well as the mineralization of sandy and gravelly soils (Sannikov, 1992; Pimenov, 2015). In the south of Siberia, pine forests are classified as especially valuable natural objects that stabilize environmental conditions. The maximum spectrum of adaptive variations for Scots pine is observed in this part of the region. Relict morpho-and genotypes of Scots pine can still be preserved in the south of Siberia (Pimenov, 2015). In Asia, Scots pine growing in the south of 52°N was identified as a steppe Scots pine variety (Pinus sylvestris var. mongholica Litv.) (The Plant List, 2023; World Flora Online, 2023), which most often known as Syn.: P. s. ssp. Kulundensis (Pravdin, 1964). P. s. var. mongholica is exceptionally resistant to adverse conditions and, growing on the sandy shores of salt lakes, can tolerate slight salinization of the soil (Zvereva, 2017). In the southern border of the species` distribution range in Siberia Scots pine forms island-like and ribbon-like forests and due to the structure and location features, they can serve as important objects for studying such processes as isolation, selection, genetic drift, mutation and inbreeding (Novikova and Zhamyansuren, 2012).
In recent decades, due to the combined effect of natural and anthropogenic factors, the dieback of coniferous forests in the south of Siberia has become catastrophic (Bazhina, 2010; Pavlov et al., 2011). For instance, by the end of the last century, due to root rot damage, as well as wind damage, forest fire, sanitary cuttings, Minusinsk pine forests (Minusinsk basin) suffered on an area of 28 thousand hectares (Tatarintsev et al., 2015). Over the past decade, in Khamar-Daban mountain range (the south of Lake Baikal) 40% of Siberian stone pine (Pinus sibirica Du Tour) forests has died because of bacterial infection caused by Erwinia sp. and Pseudomonas sp. and damages caused by Dendrolimus sibiricus Tschetw (Stavnikov, 2013; Voronin et al., 2013). Furthermore, since 1970s, there has been an intensive dieback of Siberian fir (Abies sibirica Ledeb.) in the mountains of Southern Siberia (Khamar Daban Mts., Western and Eastern Sayan Mts.) (Tretyakova et al., 2008). In this regard, the study of genetic diversity and structure of coniferous forests in the south of Siberia is very relevant.
Population genetic studies of Scots pine based on isoenzyme, chloroplast DNA markers show the evidence of genetic heterogeneity of pine populations in the south of Siberia (Sannikov and Petrova, 2012; Ekart et al., 2014; Semerikov et al., 2014). This is mainly due to the island type of the species’ range in the steppes of Southern Transbaikalia, Northern Mongolia and its mountainous island type in the mountains of Southern Siberia and the Amur region (Sannikov and Petrova, 2012).
In this study, we used nuclear SSR markers to explore the genetic diversity and structure of Scots pine populations in southern Middle Siberia. Specifically, we aimed to: (a) assess the patterns of genetic diversity within and among populations, (b) test whether genetic differentiation is related to climatic variables.
2. Materials and methods
2.1. Plant material
Initially, 210 Scots pine individuals were sampled from eight populations located in southern Middle Siberia (Russia) on a vast territory covering the mountain and foothill forests of the Western Sayan Mts., Kuznetsk Alatau Mts. and forest-steppe and steppe landscapes of the Minusinsk basin, Todzha basin and Central Tuva basin (Table 1; Figure 1). Due to amplification failures, the number of studied individuals was reduced to 169.
2.2. DNA extraction and microsatellite analysis
Total genomic DNA was isolated according to the CTAB method (Doyle and Doyle, 1990). The quality and quantity of the extracted DNA was measured with a Nanodrop 8,000 spectrophotometer (Thermo Fisher Scientific, United States), then adjusted to a concentration of 10 ng/μL. Seven nuclear microsatellite primers were selected: Psyl16, Psyl42, Psyl44, Psyl57 (Sebastiani et al., 2012); PtTX2146 (Elsik et al., 2000); lw_isotig04306, lw_isotig07383 (Fang et al., 2014). All primers were combined into two multiplex sets: set 1 consisted of Psyl44, Psyl57 and lw_isotig04306; set 2 comprised of Psyl16, Psyl42, PtTX2146, and lw_isotig07383. Reverse primers were labelled with a fluorescent dye. Polymerase chain reaction (PCR) was performed in a 10 μL reaction volume using Qiagen Multiplex PCR Kits (Qiagen, Germany) under the manufacturer’s instructions. Amplification was performed in a Corbett thermal cycler (Corbett Research, Australia) with the following cycling parameters: an initial denaturation at 95°C for 5 min, followed by 32 cycles of denaturation at 95°C for 30 s, annealing at 58°C for 90 s, extension at 72°C for 50 s and a final extension at 72°C for 7 min. Then, the amplified fragments were run on a GenomeLab GeXP genetic analyzer (Beckman Coulter, Fullerton, CA) with an internal size standard. Genotyping was performed with the GenomeLab GeXP software (Version 10.2, Beckman Coulter, Fullerton, CA).
2.3. Data analysis
Genetic diversity per locus and population was estimated by using GenAlEx v. 6.5 software (Peakall and Smouse, 2006). The number of alleles (NA); number of effective alleles (NE); inbreeding coefficient of an individual relative to the subpopulation (FIS); inbreeding coefficient of an individual relative to the total population (FIT); genetic differentiation coefficient (FST); observed heterozygosity (HO); expected heterozygosity (HE) were calculated. The allelic richness (AR) was computed in R (R Core Team, 2013) using the “hierfstat” package (Goudet, 2005). Presence of null alleles and possible genotyping errors was checked using Micro-Checker software (Van Oosterhout et al., 2006). No evidence of null alleles or genotyping errors was found in the populations.
BOTTLENECK software v.1.2.02 (Piry et al., 1999) was used to test for recent population bottlenecks on the basis of the infinite allele model (IAM). Statistical significance was determined by two-tailed Wilcoxon signed-rank test with 1,000 iterations.
Analysis of molecular variance (AMOVA) was performed using the software GenAlEx v. 6.5. The significance of differences was tested by 999 permutations. The unweighted pair-group method with arithmetic mean (UPGMA) was applied to perform cluster analysis on the Nei’s genetic distances data (Nei, 1978) and a Principal Coordinates Analysis (PCoA) was conducted using the “FactoMineR” package in R (Lê et al., 2008) to compare genetic differentiation among populations.
A Bayesian clustering approach implemented in STRUCTURE v.2.3.4 was used to estimate the number of clusters in the nSSR dataset (Pritchard et al., 2000). The analysis was performed with an admixture model with correlated allele frequencies and a LOCPRIOR setup. K value was set to 1–10 with a burn-in period of 100,000 iterations followed by 500,000 Monte Carlo Markov repetitions. Twenty repetitions were set for each run. The number of clusters was estimated using ΔK parameter according to Evanno et al. (2005) using the STRUCTURE HARVESTER program (Earl and Vonholdt, 2012). To average the results of the replicated runs, the CLUMPP software v.1.1.2. was used (Jakobsson and Rosenberg, 2007).
SAMOVA software v.2.0 (Dupanloup et al., 2002) was used to identify groups of populations that are maximally differentiated from each other. Runs were conducted with the number of groups set from two to seven, performing 100 independent simulated annealing processes. The maximum FCT value was chosen as the indicator of the best grouping.
Followed by the detection of genetic clusters, Genetic Landscape Shape Interpolation analysis was carried out, as implemented in Alleles in Space (Miller, 2005), to produce a surface plot that shows major genetic discontinuities, indicating probable contact areas between the detected genetic clusters. On the surface plot, positive peaks indicate areas with high genetic discontinuities (high genetic distances) and negative peaks represent of areas with genetic similarities (low genetic distances).
To detect the presence of isolation by distance (IBD), the correlation between geographical distances and genetic distances between population pairs was tested with Mantel test (Mantel, 1967). The test was performed with the “adegenet” R package with 1,000 bootstrap replicates (Jombart, 2008).
To test whether climatic variation contributed to the patterns of genetic differentiation, i.e., to test the isolation-by-environment (IBE) hypothesis (Wang and Bradburd, 2014), three different approaches were taken. First, in addition to the genetic and geographic distances calculated for IBD, Euclidean climatic distances were calculated from recent (c. 1950–2000) climate data using 19 bioclimatic variables (Supplementary Table S1) which were extracted from the global climate layer data using a grid size of 30 arc-seconds and downloaded from the WorldClim v.1.4 database.1 After, genetic, geographic, and the climatic distances were used in Mantel, partial-Mantel and MMRR (Multiple Matrix Regression with Randomization) regression analyses. The partial-Mantel test was conducted using the “vegan” package (Oksanen et al., 2022), while the MMRR carried out using the custom script of Wang (2013). The MMRR R script is deposited in the Dryad Data Repository under DOI:10.5061/dryad.kt71r.
3. Results
Using seven nuclear microsatellite loci, we identified a total of 49 alleles in the 169 individuals (Supplementary Table S2). The average number of alleles per locus (NA) was seven. FIS ranged from-0.055 (Psyl42) to 0.249 (Psyl44). The values of genetic differentiation (FST) varied in the range between 0.028 (lw_isotig07383) to 0.111 (Psyl42).
The mean number of alleles present per population varied from 3.571 (BAL-2) to 5.143 (PER-1 and SAR-1) (Table 2). Effective number of alleles (NE) ranged between 2.191 in KER population and 2.910 in PER-2 population, with an average of 2.517 per population. Shannon Information Index (I) varied from 0.891 (BAL-1) to 1.118 (PER-2) population. The BAL-2 population had the lowest values for allelic richness (AR = 3.571) and PER-1 population had the highest value (AR = 4.764). The values of expected heterozygosity (HE) ranged from 0.472 (BAL-1) to 0.565 (PER-2). The inbreeding coefficient (FIS) values were between-0.161 and 0.229, but in general placed around zero in most of the populations. The value of the number of migrants per generation was high (Nm = 5.690) indicates high gene flow between populations. The ratio of observed and expected heterozygosity was balanced (mean HO: HE = 0.510: 0.518).
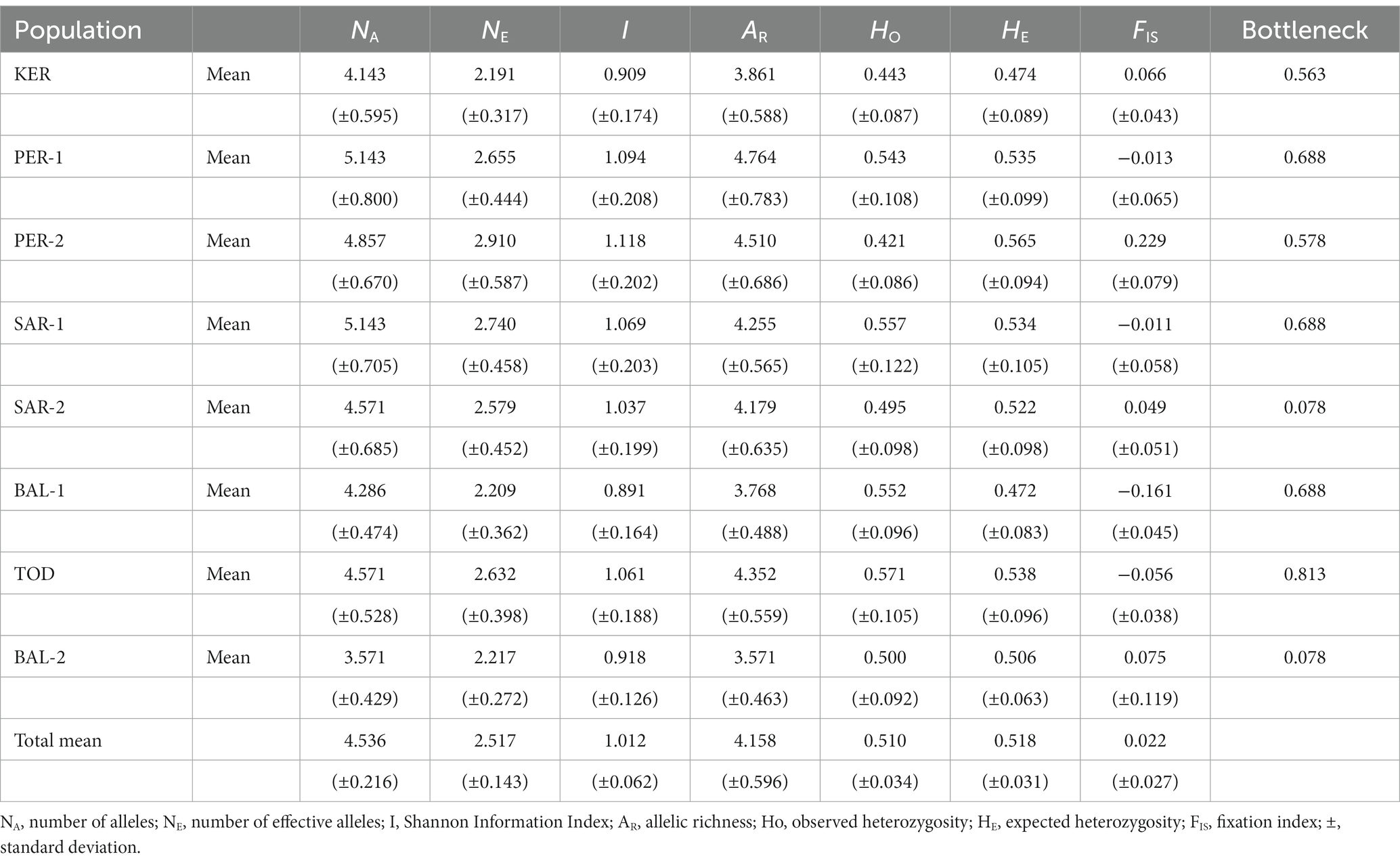
Table 2. Genetic statistics averaged across seven microsatellite loci for each Scots pine population.
The population stability analysis revealed no evidence for recent bottlenecks in the studied populations.
We performed AMOVA among and within Scots pine populations (Supplementary Table S3) and the results showed that the genetic variation among populations was 7%, whereas most of genetic variation occurred within populations (93%, p < 0.001).
The matrix of pairwise FST values (Figure 2A) revealed that the highest differentiation apparent between KER, PER-1, PER-2 and SAR-1, SAR-2 and BAL-1 populations, while the lowest between KER, PER-1, PER-2 populations. Similarly, the UPGMA clustering indicated two groups. The first group consisted of PER-1, PER-2 and KER populations and the second group was composed of the five remaining Scots pine populations (Figure 2B).
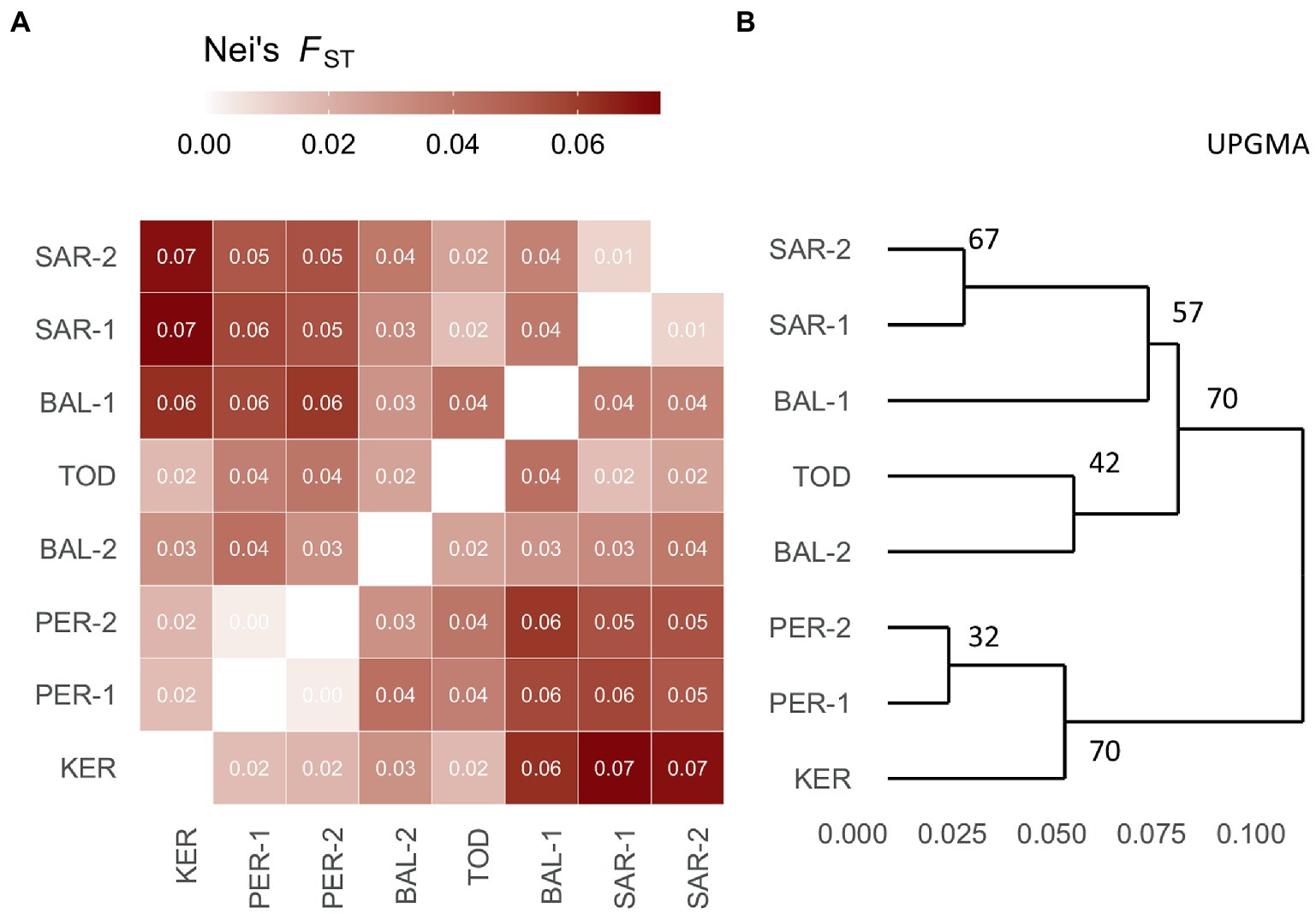
Figure 2. Heatmap of pairwise Fst (Nei, 1978) values (A) and bootstrapped UPGMA tree (B) of the eight Scots pine populations investigated.
The Bayesian STRUCTURE analysis revealed two Scots pine gene pools in the south of Middle Siberia (Figure 3), based on the Mean L(K) (±SD) and ΔK values. Group I included PER-1, PER-2 and KER populations, whereas Group II included all remaining populations.
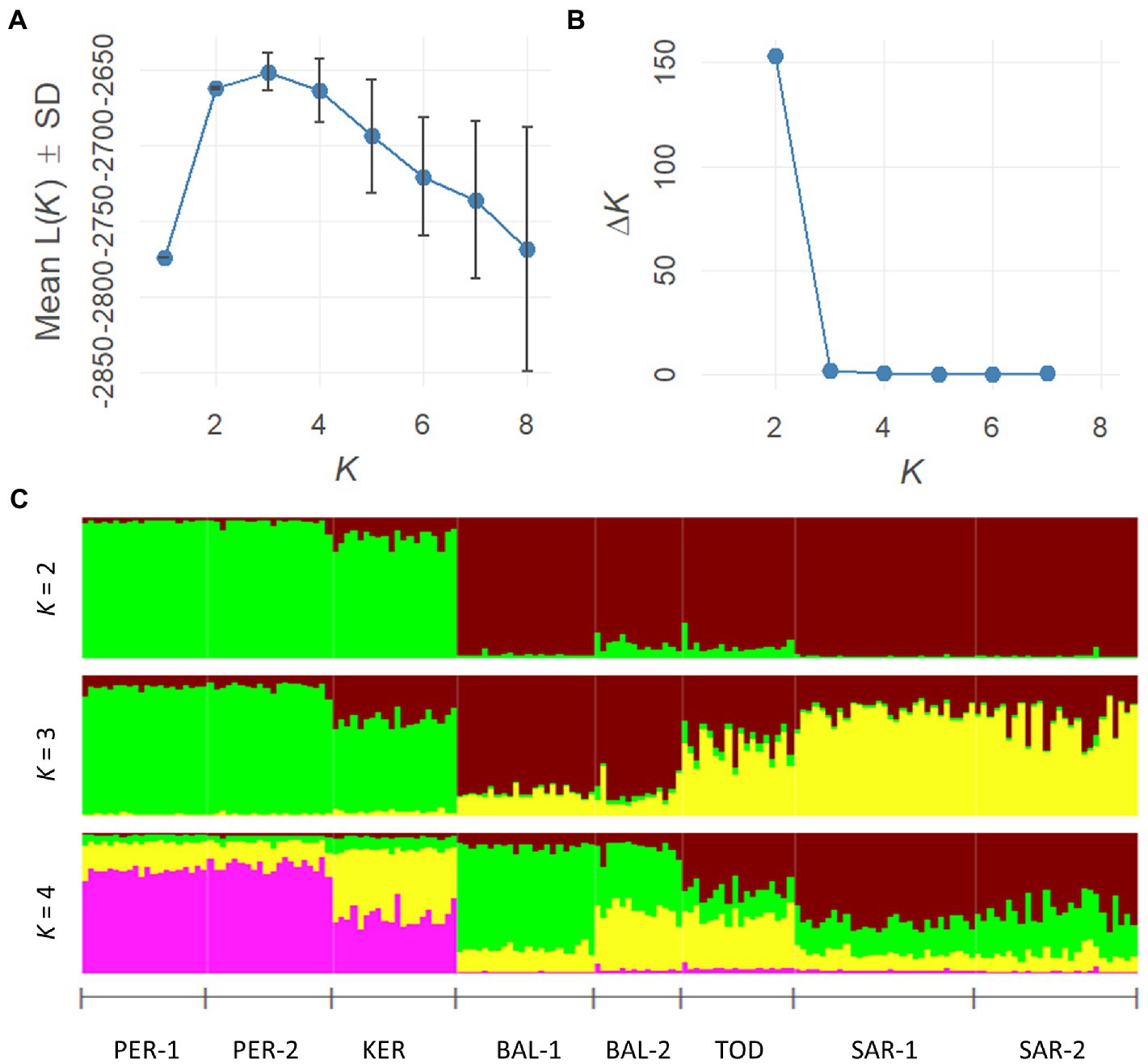
Figure 3. Estimation of the best subpopulation numbers based on Mean L(K) (±SD) and ΔK values (A,B). Genetic structural plot of eight Scots pine populations (acronyms are as in Table 1) (C).
Principal Coordinates Analysis (PCoA) based on the FST values identified two major groups (Supplementary Figure S1) at Dim. 1 vs. Dim. 2, explaining jointly 74.01% of the total variation for the nSSR markers. One group included populations from the Minusinsk basin (PER-1 and PER-2) and the Western Sayan Mts. (KER). The second group contained five populations from the Kuznetsk Alatau Mts. (SAR-1 and SAR-2), the Central Tuva basin (BAL-1 and BAL-2) and the Todzha Basin (TOD). The second and third axes (Dim. 2 vs. Dim. 3) explained much less, only 31.69% of the total variation and grouped SAR-1 and SAR-2 with PER-1 and PER-2 populations.
Genetic Landscape Spatial Interpolation has detected a significant barrier to gene flow in the form of a genetic discontinuity in the contact zone between the genetic lineages in this region (Figure 4). The estimation of the contribution of genotypes in each population showed that the PER-1, PER-2 and KER populations contained a higher proportion of genotypes originated from the Minusinsk basin, compared to other samples from the Kuznetsk Alatau Mts. (SAR-1 and SAR-2), the Central Tuva basin (BAL-1 and BAL-2) and the Todzha basin (TOD).
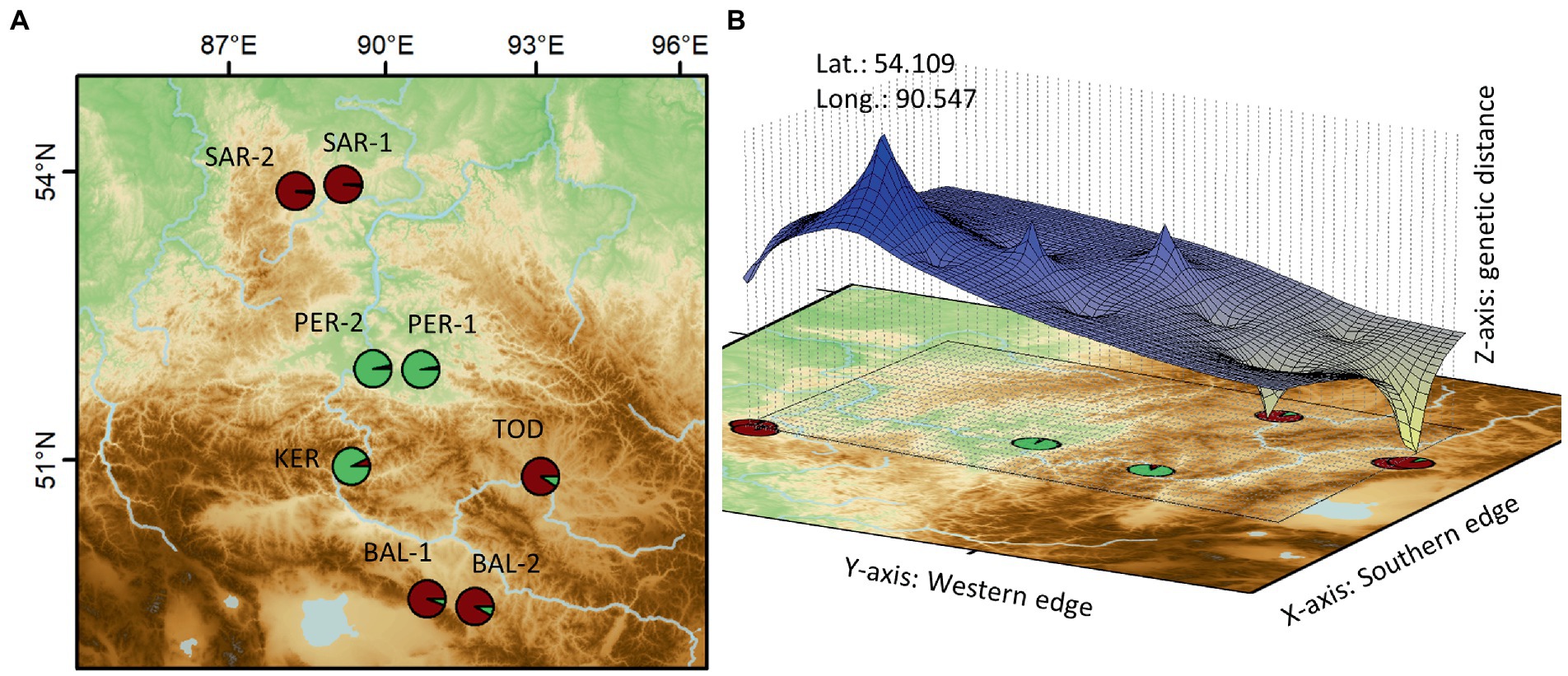
Figure 4. Spatial extent of the detected genetic clusters (A) and the genetic discontinuity revealed by the Genetic Landscape Shape Interpolation analysis (B) (for the acronyms, see Table 1).
Spatial analysis of molecular variance (SAMOVA) produced values of FCT ranging from 0.0305 (K = 3) to a maximal value of 0.03336 (K = 6), which indicated number 6 to be the preferred number of genetically homogenous clusters for the whole dataset (Supplementary Table S4). The six defined clusters contained the following populations: (I) PER-1 and PER-2; (II) BAL-1; (III) BAL-2; (IV) SAR-1 and SAR-2; (V) KER; (VI) TOD.
We further analyzed the correlation between genetic distance and geographic distance for the studied populations using the Mantel test. The results showed no correlation between genetic differentiation and geographical distance among Scots pine populations (R2 = 0.043, p = 0.163). In addition, none of the matrix regression approaches (Mantel, partial-Mantel, and MMRR) to investigate IBE, were able to find significant relationships between the genetic, geographic and climatic distances (Supplementary Table S5).
4. Discussion
Forest fragmentation is constantly increasing in southern Siberia due to intensive logging, livestock grazing, as well as drought-and fire-related forest declines, and has been detected for many forest species (Wirth et al., 1999; González de Andrés et al., 2022). Fragmentation of forest landscapes causes isolation, and poses genetic and ecological threats to populations (Gustafson et al., 2010; Khansaritoreh et al., 2018; Erasmi et al., 2021). Conservation and rational use of Siberian forest genetic resources is one of the most important environmental and economic tasks. The effectiveness of solving this problem depends on the degree of knowledge of forest genetic resources. Unfortunately, despite some success, Siberian forest genetic resources have not been studied enough (Tarakanov and Krutovsky, 2016). Here, we assessed the genetic diversity and structure of eight southern Siberian Scots pine populations.
Our results showed that, despite the detectable effects of fragmentation, the level of genetic diversity in Scots pine populations in southern Middle Siberia is high (HE = 0.518) and is similar to the other Scots pine populations in Middle Siberia (HE = 0.514) (Sheller et al., 2023). At the same time, our estimates are lower than those observed in other studies of Scots pine from Italy (HE = 0.81) (Scalfi et al., 2009), Romania and Hungary (HE = 0.55) (Bernhardsson et al., 2016), Turkey (HE = 0.772) (Bilgen and Nuray, 2017), and Lithuania (HE = 0.59) (Kavaliauskas et al., 2022). The highest level of genetic diversity and allelic richness were detected in PER-2 and PER-1 populations (HE = 0.565 and AR = 4.764 respectively) located in the Minusinsk basin. PER-1 and PER-2 populations represent geographically isolated pine forests, which are unique in their origin and ecological functions (Polyakova, 2008; Tatarintsev et al., 2015). The Minusinsk basin populations are distributed on the territory of the National Park “Sushenshky Forest,” which is specially protected natural area of national importance. The extrazonal ribbon-like Scots pine forests are particularly valuable natural objects of the National Park “Sushenshky Forest” (Pate, 2020). The obtained results indicate the need to maintain the genetic variability of these valuable Scots pine forests in the Minusinsk basin. In the study by Ekart et al., 2014 the highest values of heterozygosity (Ho and HE) were also found in Scots pine population from the Minusinsk basin.
Gene flow is one of the most important factors that maintains genetic diversity and improves population fitness and/or adaptive potential and decrease extinction probability especially in small populations (Berthaud et al., 2001; O’Connell et al., 2007). We detected high number of migrants (Nm) indicating substantial gene flow among the studied populations, also the ration of expected and observed heterozygosity was balanced, indicating neither inbreeding nor isolation-breaking effects. This hypothesis was further supported by the inbreeding coefficient, with an overall mean of FIS = 0.022, which generally indicated random mating of individuals.
However, toward the south the pattern of genetic diversity changes, and considerably lower levels of genetic diversity were found. Expected heterozygosity and allelic richness in two southernmost populations, BAL-1 and BAL-2, were HE = 0.472 and AR = 3.571, respectively. These populations are located in the Central Tuva basin and belong to Balgazyn relict pine forest. It is possible that the adverse effects of the extremely continental climate of Central Asia and the anthropogenic factors in the area of the Balgazyn pine forest, which is steadily shrinking, contributed to the decreased diversity. For instance, due to fires, natural forests decreased by four times in the last 25 years (1988–2014) (Kuular et al., 2015). Decrease of population size, due to fires and logging, diseases and environmental pollution leads to the reduction of genetic diversity (Ellstrand and Elam, 1993). In the study by Sheller et al. (2021) the lowest level of chloroplast DNA haplotype diversity was also detected in one of the Balgazyn Scots pine populations, confirming the need the conservation of these genetic resources.
Our estimate on total genetic variation occurring among populations showed a 7% applying AMOVA, which is considerably high, by taking into account the relatively short geographical distances among some of the populations (from 3 to 593 km). In similar microsatellite studies, but on a larger geographical scales, Shuvaev et al. (2022) found a FST value of only 0.026 in Scots pine populations in Krasnoyarsk region (Middle Siberia) and Sheller et al. (2023) found a FST value of 0.097 among distant Scots pine populations in Russia. Based on allozyme analysis, Sannikov and Petrova (2012) revealed that the genetic differentiation of Scots pine populations in the southern part of the range (south of 52°–53° latitude) in Central and Eastern Siberia is 2–4 times higher than in the contiguous forest zone. By decomposing our FST value among populations, based on pairwise estimates, majority of differentiation was found between populations located in the Kuznetsk Alatau Mts. (SAR-1 and SAR-2), the Minusinsk basin (PER-1 and PER-2), and the Western Sayan (KER).
The results obtained through the utilization of regression approaches (Mantel, partial-Mantel, MMRR) did not reveal a significant association between genetic differentiation and either geographic or climatic distances. This finding suggests that genetic differentiation may be attributed to past demographic events, rather than the specific environmental conditions of the region where the population resides.
Our Bayesian and the spatial clustering approaches (STRUCTURE and SAMOVA) were consistent with the FST estimates. The STRUCTURE analysis divided the studied populations into two main groups (K = 2), with PER-1, PER-2 and KER forming one group and the remaining five populations forming another group. The Minusinsk basin and the Western Sayan populations (PER-1, PER-2 and KER) wedged among the members of the other genetic group. However, toward the south they showed mixing, albeit at an extremely low proportion. This was evident also on our pairwise FST estimates, because lower FST values were typical here than toward the north, in the direction of the West Siberian Plain. We assume that this genetic differentiation across the landscape provided evidence of a contact zone of distinct genetic lineages, and a sharp boundary limiting gene flow, of a wedged population group. The genetic specificity of many population groups in marginal parts of the species’ distribution range was also observed in Iberia, Transcaucasia, Asia Minor and Eastern Siberia (Prus-Glowacki and Stephan, 1994; Sinclair et al., 1999; Sannikov et al., 2005; Cheddadi et al., 2006; Naydenov et al., 2007; Pihäjärvi et al., 2008; Dering et al., 2017, 2021; Sheller et al., 2023). At the same time, a significant homogeneity of populations was revealed within certain regions of Scandinavia, Central, Western and Eastern Europe, Siberia (Goncharenko et al., 1993; Zhelev et al., 1994; Sinclair et al., 1999; Robledo-Arnuncio et al., 2005; Cheddadi et al., 2006; Pihäjärvi et al., 2008; Semerikov et al., 2018; Sheller et al., 2021, 2023).
It should be noted, as an interesting fact, that SAMOVA indicated six different groups, and dissected the population groups in the same order as FST decreases. First separated the Minusinsk basin and the Western Sayan populations (PER-1, PER-2, and KER), from the remaining ones, and so on. This shows that the primary barrier to restriction of gene flow is located here. Our Genetic Landscape Spatial Interpolation concurs with this, having identified a significant barrier to gene flow in the form of a genetic discontinuity in the contact zone between the genetic lineages in this region.
However, this pattern changes as we move toward the south. It is known, that an increase in FST indicates a decrease in number of migrants (Nm) and vice versa (Whitlock and McCauley, 1999). In line with this, we observed that there was less differentiation to the south, potentially indicating increased gene flow. Historical and contemporary gene flow between genetic lineages, of the same species, has been described in many species (McDermott and McDonald, 1993; Ottenburghs, 2020), and it has been known to cause introgression (i.e., admixture), through hybridization and backcrossing (Petit and Excoffier, 2009). However, in this case increased genetic diversity is expected (Pazouki et al., 2016; Dering et al., 2017). In contrast, our observations revealed a discrepancy in the southern populations. Despite the notable degree of differentiation, the level of genetic diversity was found to be low, for example in BAL-1 and BAL-2 (described above). It is plausible that these populations are relict, since the legacy of long-term isolation is characterized by low genetic diversity. Such relict populations generally harbor low levels of allelic and haplotypic variation, have limited adaptive potential for range expansion and increased risk of extinction (Rinaldi et al., 2019; Urbaniak et al., 2019; Méndez-Cea et al., 2023). However, to rule out misclassification of these southern populations, further investigations targeting past demography, gene flow and introgression are required.
5. Conclusion
In our study, we assessed the genetic diversity and population structure of eight Scots pine populations in southern Middle Siberia using seven nuclear SSR markers. The study has revealed genetic heterogeneity of Scots pine populations near the southern boundaries of the species distribution in Middle Siberia. Despite fragmentation, the studied populations preserved high genetic diversity. The highest level of genetic diversity and allelic richness was detected in two populations from the Minusinsk basin while the lowest level of genetic diversity and allelic richness was found in two southernmost populations, which belong to a relict Balgazyn pine forest in the Central Tuva basin. However, to confirm a relictary status of Balgazyn pine forest additional studies should be carried out. Two clustering methods showed that the Minusinsk basin and the Western Sayan populations formed a distinct genetic group. The pattern of genetic diversity suggests a different origin of the studied Scots pine populations. However, further investigation is needed to study the evolutionary history of Scots pine populations in southern Siberia.
Data availability statement
The original contributions presented in the study are included in the article/Supplementary material, further inquiries can be directed to the corresponding author.
Author contributions
MS and AC designed the experiment. EC performed laboratory work. MS and ET analyzed the data. MS wrote the manuscript. ET reviewed and edited the manuscript. SK, AT, NK, AI, TS, NM, and PM collected the samples. All authors contributed to the article and approved the submitted version.
Funding
This research was carried out within the State Assignment (theme Fundamental principles of forest protection from entomo-and phyto-pests in Siberia no. FEFE 2020–0014) supported by the Ministry of Education and Science of the Russian Federation.
Acknowledgments
We would like to acknowledge the administration of the National Park “Shushenski Forest” and the Sayano-Shushenski Nature Reserve for helping us with the sampling.
Conflict of interest
The authors declare that the research was conducted in the absence of any commercial or financial relationships that could be construed as a potential conflict of interest.
Publisher’s note
All claims expressed in this article are solely those of the authors and do not necessarily represent those of their affiliated organizations, or those of the publisher, the editors and the reviewers. Any product that may be evaluated in this article, or claim that may be made by its manufacturer, is not guaranteed or endorsed by the publisher.
Supplementary material
The Supplementary material for this article can be found online at: https://www.frontiersin.org/articles/10.3389/ffgc.2023.1152850/full#supplementary-material
Footnotes
References
Bazhina, E. V. (2010). On the factors of Abies sibirica drying in the southern Siberia mountains. News Irkutsk State Univ. 3, 20–25.
Belletti, P., Ferrazzini, D., Ducci, F., De Rogatis, A., and Mucciarelli, M. (2017). Genetic diversity of Italian populations of Abies alba. Dendrobiology 77, 147–159. doi: 10.12657/denbio.077.012
Bernhardsson, C., Floran, V., Ganea, S. L., and García-Gil, M. R. (2016). Present genetic structure is congruent with the common origin of distant scots pine populations in its Romanian distribution. For. Ecol. Manag. 361, 131–143. doi: 10.1016/j.foreco.2015.10.047
Berthaud, J., Clément, J. C., Emperaire, L., Louette, D., Pinton, F., Sanou, J., et al. (2001). “The role of local level gene flow in enhancing and maintaining genetic diversity” in Broadening the genetic base of crop production. eds. H. D. Cooper, C. Spillane, and T. Hodgkin (New York, NY: CABI Publishing in Association with FAO and IPGRI), 81–103.
Bilgen, B. B., and Nuray, K. (2017). Genetic diversity among Pinus sylvestris L. populations and its implications for genetic conservation: Comparison of nuclear and chloroplast microsatellite markers. Fresenius Environ. Bull. 26, 6873–6881.
Cheddadi, R., Vendramin, G. G., Litt, T., François, L., Kageyama, M., Lorentz, S., et al. (2006). Imprints of glacial refugia in the modern genetic diversity of Pinus sylvestris. Glob. Ecol. Biogeogr. 15, 271–282. doi: 10.1111/j.1466-822X.2006.00226.x
Dering, M., Baranowska, M., Beridze, B., Chybicki, I. J., Danelia, I., Iszkuło, G., et al. (2021). The evolutionary heritage and ecological uniqueness of scots pine in the Caucasus ecoregion is at risk of climate changes. Sci. Rep. 11:22845. doi: 10.1038/s41598-021-02098-1
Dering, M., Kosiński, P., Wyka, T. P., Pers-Kamczyc, E., Boratyński, A., Boratyńska, K., et al. (2017). Tertiary remnants and Holocene colonizers: Genetic structure and phylogeography of scots pine reveal higher genetic diversity in young boreal than in relict Mediterranean populations and a dual colonization of Fennoscandia. Divers. Distrib. 23, 540–555. doi: 10.1111/ddi.12546
Dupanloup, I., Schneider, S., and Excoffier, L. (2002). A simulated annealing approach to define the genetic structure of populations. Mol. Ecol. 11, 2571–2581. doi: 10.1046/j.1365-294X.2002.01650.x
Earl, D. A., and Vonholdt, B. M. (2012). Structure harvester: A website and program for visualizing STRUCTURE output and implementing the Evanno method. Conserv. Genet. Resour. 4, 359–361. doi: 10.1007/s12686-011-9548-7
Ekart, A. K., Larionova, A. Y., Kravchenko, A. N., Tikhonova, I. V., Zatsepina, K. G., Tarakanov, V. V., et al. (2014). Genetic diversity and differentiation of scots pine populations in southern Siberia and Mongolia. Contemp. Probl. Ecol. 7, 52–59. doi: 10.1134/S1995425514010041
Ellstrand, N. C., and Elam, D. R. (1993). Population genetic consequences of small population size: Implications for plant conservation. Annu. Rev. Ecol. Syst. 24, 217–242. doi: 10.1146/annurev.es.24.110193.001245
Elsik, C. G., Minihan, V. T., Hall, S. E., Scarpa, A. M., and Williams, C. G. (2000). Low-copy microsatellite markers for Pinus taeda L. Genome 43, 550–555. doi: 10.1139/g00-002
Erasmi, S., Klinge, M., Dulamsuren, C., Schneider, F., and Hauck, M. (2021). Modelling the productivity of Siberian larch forests from Landsat NDVI time series in fragmented forest stands of the Mongolian forest-steppe. Environ. Monit. Assess. 193, 1–18. doi: 10.1007/s10661-021-08996-1
Evanno, G., Regnaut, S., and Goudet, J. (2005). Detecting the number of clusters of individuals using the software STRUCTURE: A simulation study. Mol. Ecol. 14, 2611–2620. doi: 10.1111/j.1365-294X.2005.02553.x
Fang, P., Niu, S., Yuan, H., Li, Z., Zhang, Y., Yuan, L., et al. (2014). Development and characterization of 25 454 EST-SSR markers in Pinus sylvestris var. mongolica (Pinaceae). Appl. Plant Sci. 2:455. doi: 10.3732/apps.1300057
Floran, V., Sestras, R. E., and Garcia-Gil, M. R. (2011). Organelle genetic diversity and Phylogeography of scots pine (Pinus sylvestris L.). Not. Bot. Horti Agrobot. Cluj-Napoca 39, 317–322. doi: 10.15835/nbha3916103
Goncharenko, G. G., Silin, A. E., and Padutov, V. E. (1993). Investigation of the genetic structure and level of differentiation in Pinus sylvestris L. in central and regional populations of Eastern Europe and Siberia. Genetics 29, 2019–2037.
González de Andrés, E., Shestakova, T. A., Scholten, R. C., Delcourt, C. J., Gorina, N. V., and Camarero, J. J. (2022). Changes in tree growth synchrony and resilience in Siberian Pinus sylvestris forests are modulated by fire dynamics and ecohydrological conditions. Agric. For. Meteorol. 312:108712. doi: 10.1016/j.agrformet.2021.108712
Goudet, J. (2005). Hierfstat, a package for R to compute and test hierarchical F-statistics. Mol. Ecol. Notes 5, 184–186. doi: 10.1111/j.1471-8286.2004.00828.x
Gustafson, E. J., Shvidenko, A. Z., Sturtevant, B. R., and Scheller, R. M. (2010). Predicting global change effects on forest biomass and composition in south-central Siberia. Ecol. Appl. 20, 700–715. doi: 10.1890/08-1693.1
Hoban, S., Bruford, M., D’Urban, J. J., Lopes-Fernandes, M., Heuertz, M., Hohenlohe, P. A., et al. (2020). Genetic diversity targets and indicators in the CBD post-2020 global biodiversity framework must be improved. Biol. Conserv. 248:108654. doi: 10.1016/j.biocon.2020.108654
Jakobsson, M., and Rosenberg, N. A. (2007). CLUMPP: A cluster matching and permutation program for dealing with label switching and multimodality in analysis of population structure. Bioinformatics 23, 1801–1806. doi: 10.1093/bioinformatics/btm233
Jombart, T. (2008). Adegenet: An R package for the multivariate analysis of genetic markers. Bioinformatics 24, 1403–1405. doi: 10.1093/bioinformatics/btn129
Kavaliauskas, D., Danusevičius, D., and Baliuckas, V. (2022). New insight into genetic structure and diversity of scots pine (Pinus sylvestris L.) populations in Lithuania based on nuclear, chloroplast and mitochondrial DNA markers. Forests 13:1179. doi: 10.3390/f13081179
Khansaritoreh, E., Schuldt, B., and Dulamsuren, C. (2018). Hydraulic traits and tree-ring width in Larix sibirica Ledeb. as affected by summer drought and forest fragmentation in the Mongolian forest steppe. Ann. For. Sci. 75, 1–12.
Kuular, H. B., Namzyn, S. A., and Hertek, S. B. (2015). Monitoring of the Balgazyn forest according to remote sensing data. News of higher educational institutions. Geodesy Aerial Photogr. 3, 67–72.
Lê, S., Josse, J., and Husson, F. (2008). FactoMineR: An R package for multivariate analysis. J. Stat. Softw. 25, 1–18. doi: 10.18637/jss.v025.i01
Mantel, N. A. (1967). The detection of disease clustering and a generalized regression approach. Cancer Res. 27, 209–220.
McDermott, J. M., and McDonald, B. A. (1993). Gene flow in plant pathosystems. Annu. Rev. Phytopathol. 31, 353–373. doi: 10.1146/annurev.py.31.090193.002033
Méndez-Cea, B., García-García, I., Gazol, A., Camarero, J. J., de Andrés, E. G., Colangelo, M., et al. (2023). Weak genetic differentiation but strong climate-induced selective pressure toward the rear edge of mountain pine in North-Eastern Spain. Sci. Total Environ. 858:159778. doi: 10.1016/j.scitotenv.2022.159778
Miller, M. P. (2005). Alleles in space (AIS): computer software for the joint analysis of interindividual spatial and genetic information. J. Hered. 96, 722–724. doi: 10.1093/jhered/esi119
Naydenov, K., Senneville, S., Beaulieu, J., Tremblay, F., and Bousquet, J. (2007). Glacial variance in Eurasia: Mitochondrial DNA evidence from scots pine for a complex heritage involving genetically distinct refugia at mid-northern latitudes and in Asia minor. BMC Evol. Biol. 7, 233–235. doi: 10.1186/1471-2148-7-233
Nei, M. (1978). Estimation of average heterozygosity and genetic distance from a small number of individuals. Genetics 89, 583–590. doi: 10.1093/genetics/89.3.583
Novikova, T. N., and Zhamyansuren, S. (2012). Scotch pine seed quality variability near southern limits of its spread in Siberia and Mongolia. Bull. KrasSAU 4, 102–107.
O’Connell, L. M., Mosseler, A., and Rajora, O. P. (2007). Extensive long-distance pollen dispersal in a fragmented landscape maintains genetic diversity in white spruce. J. Hered. 98, 640–645. doi: 10.1093/jhered/esm089
Oksanen, J., Blanchet, F.G., Friendly, M., Kindt, R., Legendre, P., McGlinn, D., et al. (2022). Package ‘vegan’: community ecology package. Available at: https://cran.r-project.org/web/packages/vegan/index.html
Ottenburghs, J. (2020). Ghost introgression: Spooky gene flow in the distant past. BioEssays 42:2000012. doi: 10.1002/bies.202000012
Pate, E. Y. (2020) Stories of the Shushensky forest. Verkhny Tagil: Ural Provincial Publishing House, 207.
Pavlov, I. N., Barabanova, O. A., and Ageev, A. A. (2011). Resistance reduction of fir-cedar forests of eastern Sayan Mountains to root pathogens. Russ. For. J. 4, 40–45.
Pazouki, L., Shanjani, P. S., Fields, P. D., Martins, K., Suhhorutšenko, M., Viinalass, H., et al. (2016). Large within-population genetic diversity of the widespread conifer Pinus sylvestris at its soil fertility limit characterized by nuclear and chloroplast microsatellite markers. Eur. J. For. Res. 135, 161–177. doi: 10.1007/s10342-015-0928-5
Peakall, R., and Smouse, P. E. (2006). GenAlEx 6: Genetic analysis in excel. Population genetic software for teaching and research. Mol. Ecol. Notes 6, 288–295. doi: 10.1111/j.1471-8286.2005.01155.x
Petit, R. J., and Excoffier, L. (2009). Gene flow and species delimitation. Trends Ecol. Evol. 24, 386–393. doi: 10.1016/j.tree.2009.02.011
Pihäjärvi, T., Salmela, M. J., and Savolainen, O. (2008). Colonization routes of Pinus sylvestris inferred from distribution of mitochondrial DNA variation. Tree Genet. Genomes 4, 247–254. doi: 10.1007/s11295-007-0105-1
Pimenov, A.V. (2015). Biodiversity of the scots pine (Pinus sylvestris L.) in contrasting ecotopes of the south of Siberia. dissertation/doctoral thesis. Krasnoyarsk: Sukachev Forest Institute of the Siberian Branch of the Russian Academy of Sciences.
Piry, S., Luikart, G., and Cornuet, J. M. (1999). BOTTLENECK: A computer program for detecting recent reductions in the effective population size using allele frequency data. J. Hered. 90, 502–503. doi: 10.1093/jhered/90.4.502
Polyakova, M. A. (2008). Vegetation of ribbon forests of the Minusinsk basin. dissertation/PhD thesis. Novosibirsk: Central Botanical Garden SB RAS.
Pritchard, J., Stephens, M., and Donnelly, P. (2000). Inference of population structure using multilocus genotype data. Genetics 155, 945–959. doi: 10.1093/genetics/155.2.945
Prus-Glowacki, W., and Stephan, B. R. (1994). Genetic variation of Pinus sylvestris from Spain in relation to other European populations. Silvae Genet. 43, 7–14.
R Core Team. (2013). R: A language and environment for statistical computing. Vienna: R Foundation for Statistical Computing.
Rinaldi, R., Cafasso, D., Strumia, S., Cristaudo, A., Sebastiani, F., and Fineschi, S. (2019). The influence of a relict distribution on genetic structure and variation in the Mediterranean tree, Platanus orientalis. AoB Plants. 11:plz002. doi: 10.1093/aobpla/plz002
Robledo-Arnuncio, J. J., Collada, C., Alia, R., and Gil, L. (2005). Genetic structure of montane isolates of Pinus sylvestris L. in a Mediterranean refugial area. Biogeographica 32, 595–605. doi: 10.1111/j.1365-2699.2004.01196.x
Sannikov, S. N., and Petrova, I. V. (2012). Phylogenogeography and genotaxonomy of Pinus sylvestris L. populations. Ecology 4, 252–260.
Sannikov, S. N., Petrova, I. V., Sannikova, N. S., and Philippova, T. V. (2005). Gradient genogeographic analysis of Pinus sylvestris L. populations in Europe. Ecology 6, 415–420.
Scalfi, M., Piotti, A., Rossi, M., and Piovani, P. (2009). Genetic variability of Italian southern scots pine (Pinus sylvestris L.) populations: The rear edge of the range. Eur. J. Forest Res. 128, 377–386. doi: 10.1007/s10342-009-0273-7
Sebastiani, F., Pinzauti, F., Kujala, S. T., González-Martínez, S. C., and Vendramin, G. G. (2012). Novel polymorphic nuclear microsatellite markers for Pinus sylvestris L. Conserv. Genet. Resour. 4, 231–234. doi: 10.1007/s12686-011-9513-5
Semerikov, V. L., Semerikova, S. A., Dymshakova, O. S., Zatsepina, K. G., Tarakanov, V. V., Tikhonova, I. V., et al. (2014). Microsatellite loci polymorphism of chloroplast DNA of scots pine (Pinus sylvestris L.) in Asia and Eastern Europe. Russ. J. Genet. 50, 577–585. doi: 10.1134/S1022795414040127
Semerikov, V., Semerikova, S., Putintseva, J., Tarakanov, V., Tikhonova, I., Vidyakin, A., et al. (2018). Colonization history of scots pine in Eastern Europe and North Asia based on mitochondrial DNA variation. Tree Genet. Genomes 14, 1–7. doi: 10.1007/s11295-017-1222-0
Sheller, M., Ciocîrlan, E., Mikhaylov, P., Kulakov, S., Kulakova, N., Ibe, A., et al. (2021). Chloroplast DNA diversity in populations of P. sylvestris L. from Middle Siberia and the Romanian Carpathians. Forests 12:1757. doi: 10.3390/f12121757
Sheller, M., Tóth, E. G., Ciocîrlan, E., Mikhaylov, P., Kulakov, S., Kulakova, N., et al. (2023). Genetic diversity and population structure of scots pine (Pinus sylvestris L.) in middle Siberia. Forests 14:119. doi: 10.3390/f14010119
Shuvaev, D. N., Ibe, A. A., Shcherba, Y. E., Sukhikh, T. V., Shilkina, E. A., Sheller, M. A., et al. (2022). Molecular genetic characteristics of scots pine populations in Krasnoyarsk region by panel of 15 nuclear microsatellite loci. Forestry 5, 530–539.
Sinclair, W. T., Morman, J. D., and Ennos, R. A. (1999). The postglacial history of scots pine (Pinus sylvestris L.) in western Europe: Evidence from mitochondrial DNA variation. Mol. Ecol. 8, 83–88. doi: 10.1046/j.1365-294X.1999.00527.x
Spielman, D., Brook, B. W., and Frankham, R. (2004). Most species are not driven to extinction before genetic factors impact them. Proc. Natl. Acad. Sci. U. S. A. 101, 15261–15264. doi: 10.1073/pnas.0403809101
Stavnikov, D. (2013). Analysis of sanitary and forest pest status of forests in republic of Buryatia. Environ. Manage. Cadastres 31, 67–73.
Tarakanov, V. V., and Krutovsky, K. V. (2016). Conservation of Siberian forest genetic resources: A brief review of the materials of the IV international meeting (Barnaul, august 24-29, 2015). Sib. For. J. 5, 7–11.
Tatarintsev, A. I., Kalenskaya, O. P., and Bublikov, A. G. (2015). On the issue of root rot infestation of pine forests of the Minusinsk basin. Conif. Boreal Zone 5, 240–247.
The Plant List. (2023). Available at: (http://www.theplantlist.org/).
Tretyakova, I. N., Bazhina, E. V., Pakharkova, N. V., and Storozhev, V. N. (2008). The condition of fir-cedar forests of the Ergaki Nature Park and their fluorescent diagnostics. Conif. Boreal Zone 3, 237–243.
Urbaniak, L., Wojnicka-Półtorak, A., Celiński, K., Lesiczka, P., Pawlaczyk, E., and Aučina, A. (2019). Genetic resources of relict populations of Pinus sylvestris (L.) in Western Carpathians assessed by chloroplast microsatellites. Biologia 74, 1077–1086. doi: 10.2478/s11756-019-00255-6
Van Oosterhout, C., Weetman, D., and Hutchinson, W. F. (2006). Estimation and adjustment of microsatellite null alleles in nonequilibrium populations. Mol. Ecol. Notes 6, 255–256. doi: 10.1111/j.1471-8286.2005.01082.x
Voronin, V. I., Morozova, T. I., Stavnikov, D. Y., Nechesov, I. A., Oskolkov, V. A., Buyantuev, V. A., et al. (2013). Bacterial damage of Siberian stone pine forests in the Baikal region. Forestry 3, 39–41.
Wang, I. J. (2013). Examining the full effects of landscape heterogeneity on spatial genetic variation: A multiple matrix regression approach for quantifying geographic and ecological isolation. Evolution 67, 3403–3411. doi: 10.1111/evo.12134
Wang, I. J., and Bradburd, G. S. (2014). Isolation by environment. Mol. Ecol. 23, 5649–5662. doi: 10.1111/mec.12938
Whitlock, M. C., and McCauley, D. E. (1999). Indirect measures of gene flow and migration: FST≠ 1/(4Nm+ 1). Heredity 82, 117–125. doi: 10.1038/sj.hdy.6884960
Wirth, C., Schulze, E. D., Schulze, W., von Stunzner-Karbe, D., Ziegler, W., Miljukova, I. M., et al. (1999). Above-ground biomass and structure of pristine Siberian scots pine forests as controlled by competition and fire. Oecologia 121, 66–80. doi: 10.1007/s004420050908
World Flora Online (2023). Available at: http://www.worldfloraonline.org. (Accessed January 21, 2023).
Zhelev, P., Longauer, R., Paule, L., and Gömöry, D. (1994). Genetic variation of the indigenous scots pine (Pinus sylvestris L.) population from the Rhodopi mountains. Nauka-za-go-rata. 31, 68–76.
Keywords: Scots pine, Pinus sylvestris L., nuclear microsatellite markers (nSSR), genetic diversity, genetic structure, Middle Siberia
Citation: Sheller M, Tóth EG, Ciocîrlan E, Mikhaylov P, Tatarintsev A, Kulakov S, Kulakova N, Melnichenko N, Ibe A, Sukhikh T and Curtu AL (2023) Genetic legacy of southern Middle Siberian mountain and foothill populations of Scots pine (Pinus sylvestris L.): Diversity and differentiation. Front. For. Glob. Change. 6:1152850. doi: 10.3389/ffgc.2023.1152850
Edited by:
Lucian Dinca, National Institute for Research and Development in Forestry “Marin Dracea”, RomaniaReviewed by:
Maria Glushkova, Bulgarian Academy of Sciences, BulgariaAdriana F. Sestras, University of Agricultural Sciences and Veterinary Medicine of Cluj-Napoca, Romania
Copyright © 2023 Sheller, Tóth, Ciocîrlan, Mikhaylov, Tatarintsev, Kulakov, Kulakova, Melnichenko, Ibe, Sukhikh and Curtu. This is an open-access article distributed under the terms of the Creative Commons Attribution License (CC BY). The use, distribution or reproduction in other forums is permitted, provided the original author(s) and the copyright owner(s) are credited and that the original publication in this journal is cited, in accordance with accepted academic practice. No use, distribution or reproduction is permitted which does not comply with these terms.
*Correspondence: Marina Sheller, bWFyYWxleHNoZWxsZXJAbWFpbC5ydQ==
†ORCID: Endre György Tóth orcid.org/0000-0001-7060-924X