- 1Jiyang College, Zhejiang A&F University, Zhuji, Zhejiang, China
- 2Department of Forestry and Environmental Conservation, Clemson University, Clemson, SC, United States
- 3Ecological Technical Research Institute, China International Engineering Consulting Corporation (CIECC), Beijing, China
- 4Department of Life Science and Health, Huzhou College, Huzhou, Zhejiang, China
Background: Pinus massoniana is an important timber species with high ecological and economic value in southern China and a pioneer species for the reforestation of barren mountains. The structure and function of the existing Masson pine ecosystem have been seriously affected by worsening habitats under current climate change. Its suitable habitat is likely to change greatly in the near future.
Methods: To estimate the potential geographic distribution of P. massoniana and its response to climate change, the Maxent model was selected to simulate the potentially suitable habitat and corresponding changes in the distribution pattern of P. massoniana under current and future climate scenarios (SSP1-2.6, SSP2-4.5, and SSP5-8.5) with two periods (2050s and 2090s).
Results: Under current climate conditions, the total suitable habitat area of P. massoniana was 2.08 × 106 km2, including 0.76 × 106 km2 of highly suitable habitat, concentrated mainly in Jiangxi and Zhejiang, central and southeastern Hunan, northern Fujian, central and western Chongqing, southern Anhui, central and surrounding areas of Guangdong, central and eastern Guangxi, and northern Taiwan. The areas of moderately suitable habitat and poorly suitable habitat were 0.87 × 106 km2 and 0.45 × 106 km2, respectively. Temperature and precipitation appear to be the most important predictors: precipitation of the driest month (14.7–215.6 mm), minimum temperature of the coldest month (−3.5–13.8°C), annual temperature range (8.1–32.9°C), and mean temperature of the warmest quarter (23.6–34.7°C). P. massoniana are predicted to expand their potential distribution under future climate change: by the end of this century, their total suitable habitat area increased 0.26 × 106 km2 (10.61%) and 0.45 × 106 km2 (17.05%) under the most moderate (SSP1-2.6) and severe (SSP5-8.5) warming scenarios, respectively, by mainly extending northward.
Conclusion: Under the different future climate scenarios, the total suitable habitat area of P. massoniana increased by mainly extending northward. Overall, our study clarifies the potential habitat distribution of P. massoniana and provides a critical empirical reference for future P. massoniana conservation and planting practices.
1. Introduction
Pinus massoniana Lamb. (Masson pine), a common and dominant tree species characterized by strong tolerance and adaptability to depleted soil, with a northern boundary of the natural distribution of the southern Henan and Shandong Province in China. It has been used as an important afforestation tree species in southern China considering its high economic (e.g., timber resource) and ecological (e.g., carbon sequestration, water, and soil conservation, forest development) values (Li H. et al., 2019; Shao et al., 2022), which accounting for 20% of the total afforestation area (National forestry and Grassland administration, 2019). However, P. massoniana is sensitive to climate change (Huang et al., 2021), for example, absolute minimum temperature, precipitation, and soil acidity-alkalinity (Jiang et al., 2019). Due to human or environmental factors, P. massoniana has presented problems such as low productivity and poor ecological function in the current situation in China.
Climate, especially temperature and precipitation, are confirmed the main environmental variables affecting the geographical distribution and growth of species worldwide (Jochum et al., 2007; Dyderski et al., 2018). The global mean temperature is predicted to increase by at least 0.3°C and up to 4.8°C by the end of this century as global warming continues (Stocker et al., 2013). There was enough evidence that current climate warming has caused many plants to migrate to higher altitudes and latitudes (Mckenney et al., 2007; Bertin, 2008; Thurm et al., 2018). Meanwhile, seasonal drought and heavy rain, hot summer and severe winter are occurring more and more frequently in subtropical areas, which may expose species to extinction, regional biodiversity and ecological security patterns change, and ecosystem functions reduction, forcing them to adapt to new condition or shift of their geographic distributions (Alexander et al., 2018; Feeley et al., 2020; He et al., 2021; Jian et al., 2022; Zhang et al., 2022). Hence, under the context of global change, increasing global warming and frequency of extreme events (extreme heat, cold, drought, etc.), how P. massoniana changes its distribution area to adapt to future climate change is a problem worth discussing. These changes will challenge forest management, utilization and preservation in the future (Dyderski et al., 2018).
Species distribution models (SDMs) or Ecological Niche Models (ENM), are mathematical models that reflect the distribution of suitable habitats of species on a large scale based on species presence or richness data and environmental factor data, which include but are not limited to genetic algorithms for rule-set production (GARP) (Qin et al., 2015), generalized linear models (GLM) (Lopatin et al., 2016), classification and regression tree (CART) (Cao et al., 2005), Bioclim (Zhu et al., 2020b), random forest (RF) (Kolli et al., 2022) and maximum entropy (Maxent) (Yan et al., 2020). The Maxent model is one of the most widely used models because of its good performance in accuracy and friendly interface, even if there are a few occurrence points or training data (Elith et al., 2006; Hernandez et al., 2006; Phillips et al., 2006; Kaky et al., 2020). It has been widely used in potential habitat predicting or geographic distribution migration under global change of invasive species, Chinese Herbs, commercial crops, desert plants, and endangered species (Bose et al., 2016; Guo et al., 2017; Li J. et al., 2019; Liu et al., 2019; Guan et al., 2022), covering plants, animals, bacteria, and fungi (Lin et al., 2019; Prevéy et al., 2020; Ye et al., 2022), and achieved satisfactory results.
In recent years, studies on P. massoniana have been conducted in physiological and ecological processes (Lei et al., 2022; Wang et al., 2022; Yuan et al., 2022), nutrient cycle (Dong et al., 2021), and forest management (Wang et al., 2019, 2021), little attention has been paid to the range shift of this widespread species in China. While this task will be a key step in the development of climate change adaptation strategies and forest management for P. massoniana. For this purpose, we used the Maxent model and ArcGIS to predict the potential suitable habitats for P. massoniana under current and various possible future climatic conditions according to P. massoniana distribution data and associated environmental variables. The main objectives of this research included the following: (1) predicting the potential suitable habitat for P. massoniana under the current climate condition; (2) screening the main environmental variables limiting the geographic distribution of P. massoniana; (3) predicting the potential suitable habitats for P. massoniana under future climate conditions and exploring changes in geographic distribution patterns of P. massoniana in the present and future. Our results provide practical guidance for the future management conservation and planting practices of P. massoniana in China.
2. Materials and methods
2.1. Species occurrence data collection
The distribution records of P. massoniana we used were obtained from the Global Biodiversity Information Facility (GBIF)1 and the National Plant Specimen Resource Center (CVH)2. These data were integrated into the present dataset, and only the records within the territory of China were kept. We collected 371 P. massoniana distribution records after deleting invalid and duplicate records. Only one distribution record was chosen from each 2.5’ × 2.5’ grid to minimize sampling bias. Ultimately, 264 useful records were selected to create the Maxent model (Figure 1).
2.2. Environmental variables and processing
Besides climatic factors, there are many other factors that influence the geographic distribution of species, such as topography and soil factors (Bradie and Leung, 2017). Numerous studies have used only climatic variables for simulation, yet these variables cannot fully describe all of the complex processes that affect species distribution (Thuiller et al., 2019). Although these models perform well, their predicted species distributions may have large biases from the true distributions and carry some prediction risk (Beale et al., 2008). Thus, a total of 37 environmental variables, including bioclimatic, topographic, and soil variables, were considered in the prediction model to better identify which variables have a greater impact on the potential distribution of P. massoniana (Table 1). The 19 bioclimatic variables were obtained with a resolution of 2.5’ from the Worldclim database3 for both current (1970–2000) and future (2050s, 2090s) scenarios. For future scenarios, 2050s bioclimatic data represented the average of 2041 to 2060, and 2090s data represented the average of 2081 to 2100. To estimate future climate change, we used general circulation model (GCM) forecasts under Shared Socio-economic Pathways (SSPs) scenarios, which were started by the IPCC’s Coupled Model Intercomparison Project Phase 6 (CMIP6) (Popp et al., 2017). The CMIP6 models have improved the simulation capability of the climatological precipitation distribution and East Asian summer monsoon (EASM) climate patterns, and also have strong simulation capability in Chinese climate indices (Xin et al., 2020; Zhu et al., 2020a). The GCM we selected for the present study was the BCC-CSM2-MR climate system model created by the National Climate Center, which performs best in reproducing the anomalous rainfall pattern in eastern China with the highest spatial correlation and can realistically simulate the rainfall anomalies in eastern China (Xin et al., 2020). It includes the following three emission scenarios: SSP1-2.6, SSP2-4.5, and SSP5-8.5. (Fick and Hijmans, 2017). SSP1-2.6 is a sustainability scenario with low levels of greenhouse gas emissions. SSP2-4.5 indicates that greenhouse gas emissions are at a moderate level, which means that the future socio-economic development pattern will continue to follow the current pattern. In contrast, SSP5-8.5 is a rapid socio-economic development scenario with high levels of greenhouse gas emissions (Eyring et al., 2016). Digital elevation model data were obtained from Geospatial Data Cloud4 in order to extract elevation, slope, and aspect data. Soil variables were derived from the Harmonized World Soil Database,5 including 15 basic soil indicators.
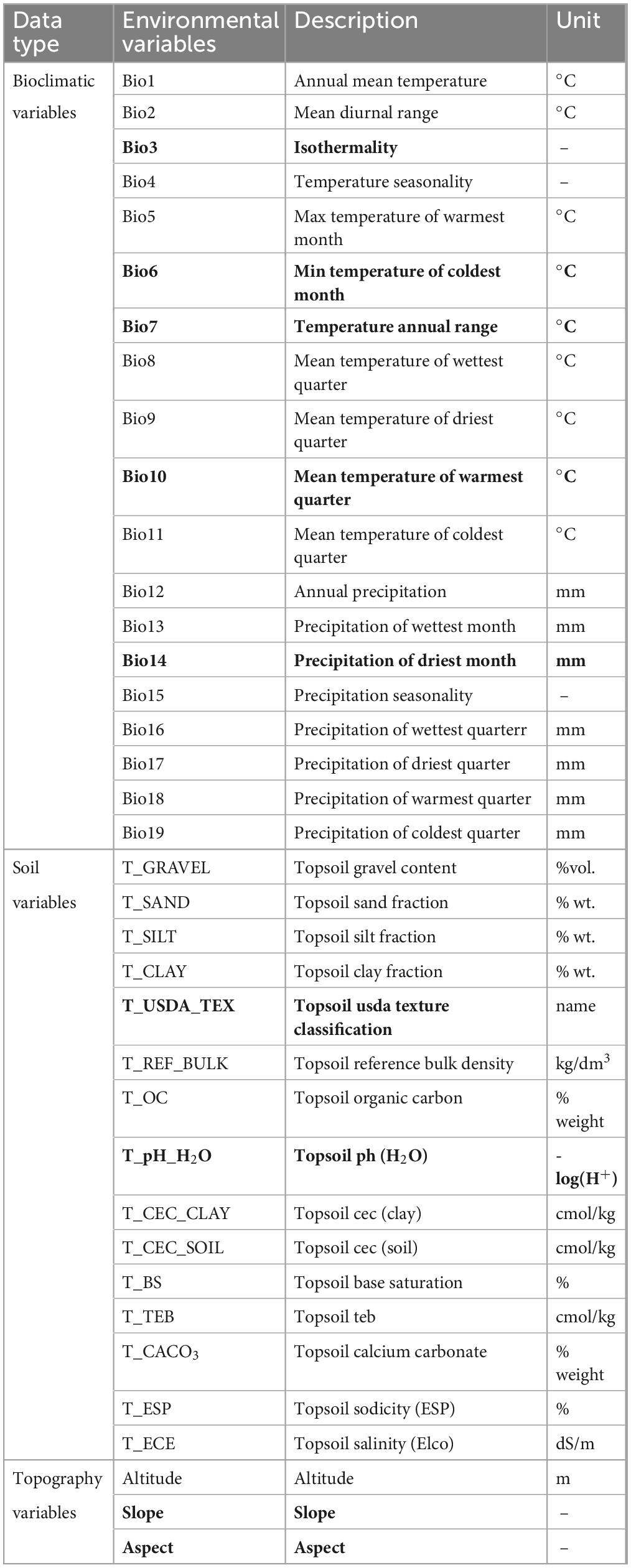
Table 1. Environmental variables considered for modeling the potentially suitable habitat of Pinus massoniana (the nine variables selected for model development are shown in bold).
Potential correlations between environmental variables may affect the accuracy of prediction (Pearson et al., 2006). Studies that ignore the redundant information that highly correlated variables introduced during species distribution modeling typically have low accuracy (Duflot et al., 2018). Eliminating redundant variables can reduce multicollinearity among variables and thus give the model stronger predictive power (Ashcroft et al., 2011; Yi et al., 2016). To improve the accuracy of the model, we selected environmental variables according to the following principles: (1) the variables imported into Maxent with contribution rates < 1% were removed; (2) after calculation of Pearson correlation coefficients between the remaining variables, those with correlation coefficients (r) less than 0.8 were retained, while variable with less contribution was eliminated when the r ≥ 0.8 (Lu et al., 2020). This approach effectively eliminated the environmental variables with low contribution and high correlation. Ultimately, nine main environmental variables were selected for modeling (Table 1).
2.3. Parameter setting and accuracy verification of Maxent model
The Maxent model was created and replicated 10 times based on the environmental variables and distribution data; 25% of the distribution data were randomly selected for model testing, while the rest 75% were utilized for model training (Phillips, 2008). The replicated run type was set as “Cross-validate,” the maximum iteration was set to 500, and the output format was logistic. To identify the key environmental variables affecting P. massoniana distribution, the jackknife test and response curves were used to assess the importance of environmental variables and their effects on P. massoniana distribution (Narouei-Khandan et al., 2016).
We evaluated the model performance using the area under the receiver operating curve (AUC) (Qin et al., 2017). In this evaluation, poor performance falls in 0.5 < AUC ≤ 0.7, moderate performance falls in 0.7 < AUC ≤ 0.9, and excellent performance falls in 0.9 < AUC ≤ 1 (Peterson et al., 2011).
2.4. Division of suitable habitat grades
We used ArcGIS 10.4 to process the suitability classification of P. massoniana. We quantified the potential distribution probabilities (P) of P. massoniana from 0 to 1 and classified them according to the method used by Zhao et al. (2021), and the habitat suitability of P. massoniana was classified into four categories: unsuitable habitat (P < 0.1), poorly suitable habitat (0.1 ≤ P < 0.3), moderately suitable habitat (0.3 ≤ P < 0.5), and highly suitable habitat (0.5 ≤ P < 1).
We utilized the SDMToolbox in ArcGIS to visualize habitat changes of P. massoniana in different climate conditions, and we compared current and future potential habitat distributions (Brown, 2014; Hu et al., 2017). The results were divided into three components: decreased area, increased area, and invariant area (Liu et al., 2022). The centroids of suitable habitats and their migration distances were also calculated for current and future climate scenarios using the SDM Toolbox in ArcGIS.
3. Results
3.1. Model performance and major environmental variables
Our validation results showed a high accuracy of the P. massoniana distribution prediction in China (Figure 2), with an AUC value of 0.914 for habitat suitability maps. Thus, the model could be used to predict the suitable habitats of P. massoniana in China.
The contributions of the nine environmental variables predicted by the Maxent model are shown in Table 2. The four environmental variables with the highest contribution rate were precipitation of the driest month (Bio14, 70.5%), minimum temperature of the coldest month (Bio6, 15%), isothermality (Bio3, 4.9%), and annual temperature range (Bio7, 4.2%). When only a single variable was used for modeling, the four variables with the highest regularized training gain were minimum temperature of the coldest month (Bio6), precipitation of the driest month (Bio14), annual temperature range (Bio7), and mean temperature of the warmest quarter (Bio10). The regularized training gains for the four variables mentioned above were higher than others significantly, indicating that these variables contain information absent from other variables (Figure 3).
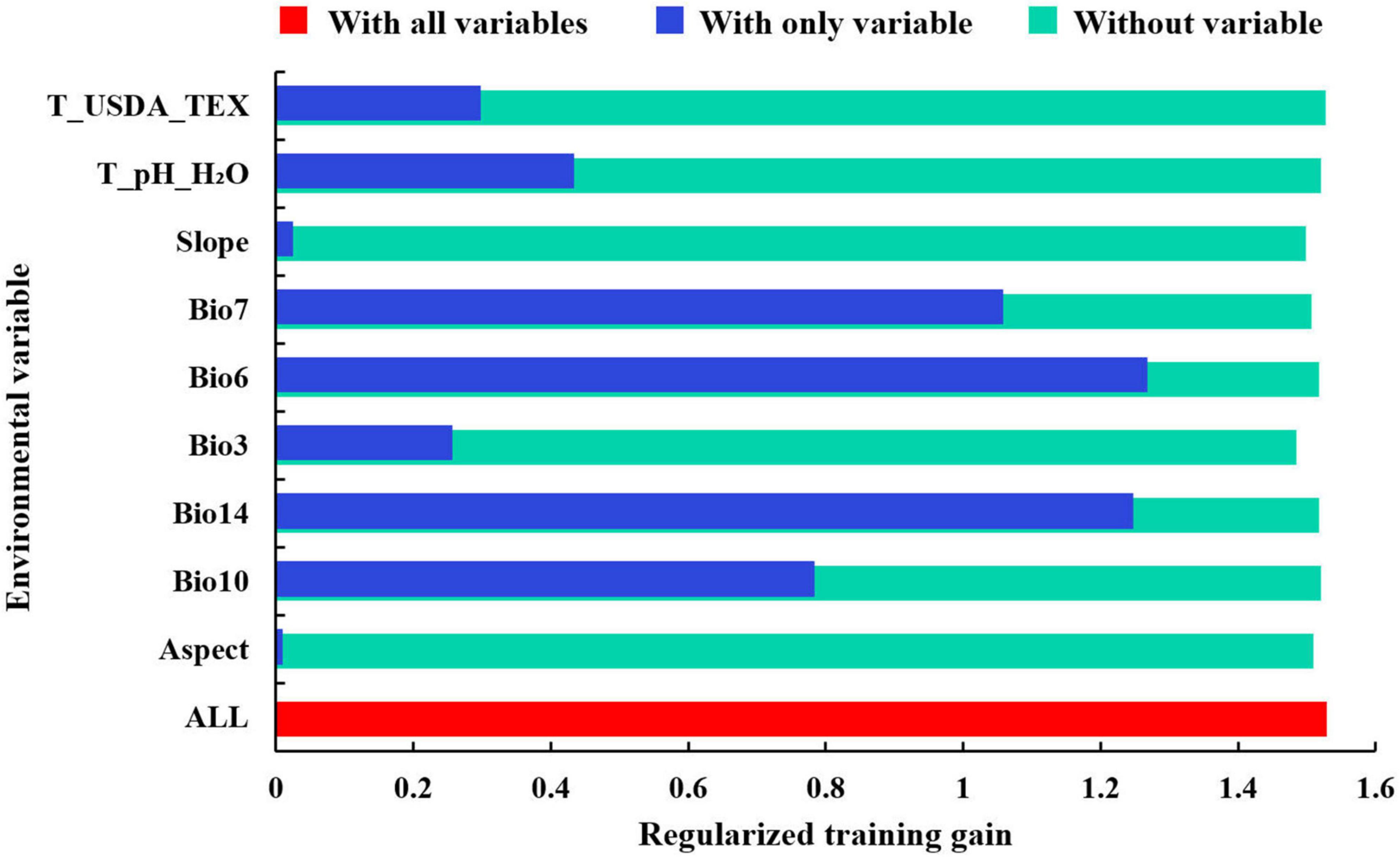
Figure 3. Jackknife test of variable importance for Pinus massoniana (“T_USDA_TEX” is topsoil USDA texture classification; “T_pH_H2O” is topsoil pH (H2O); “Slope” is slope; “Bio7” is annual temperature range; “Bio6” is minimum temperature of the coldest month; “Bio3” is isothermality; “Bio14” is precipitation of the driest month; “Bio10” is mean temperature of the warmest quarter; “Aspect” is aspect).
We used Maxent to plot response curves with single environmental variables (Figure 4) to elucidate the relationship between the probability of P. massoniana existence and key environmental variables, as well as the range of suitability of environmental variables determined by applying a threshold based on the moderately suitable habitat. The suitable habitat conditions relating to the key environmental variables for P. massoniana are: precipitation of the driest month (Bio14), 14.7–215.6 mm (Figure 4A); minimum temperature of the coldest month (Bio6), –3.5 to 13.8°C (Figure 4B); annual temperature range (Bio7), 8.1–32.9°C (Figure 4C); mean temperature of the warmest quarter (Bio10), 23.6–34.7°C (Figure 4D).
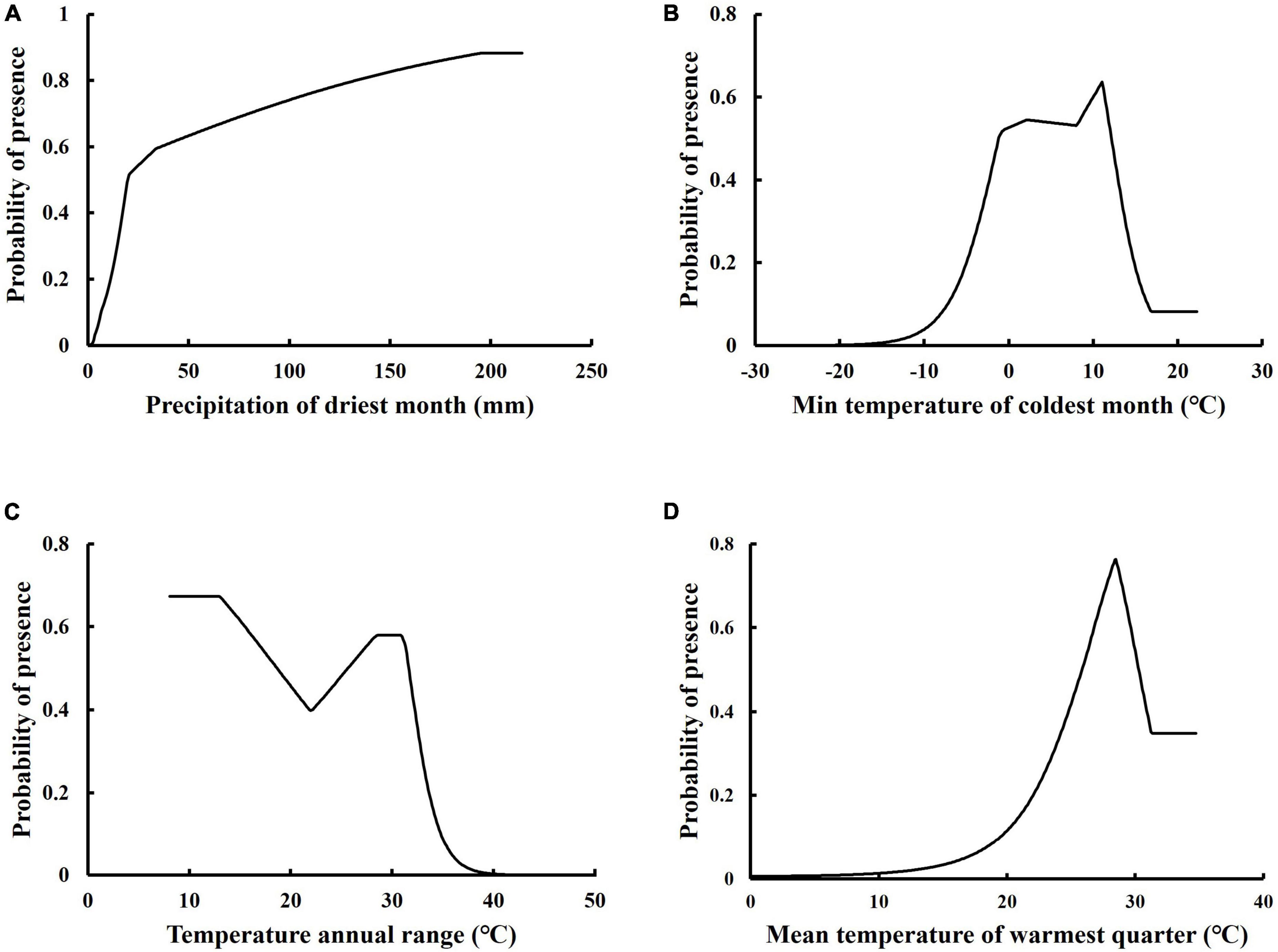
Figure 4. Response curves of presence probability of Pinus massoniana to precipitation of driest month (A), min temperature of coldest month (B), temperature annual range (C), and mean temperature of warmest quarter (D).
3.2. Potential distribution under current climate conditions
The potentially suitable habitat for P. massoniana distribution under the current climatic scenarios is shown in Figure 5. The total suitable habitat area was estimated to be 2.08 × 106 km2, accounting for 21.67% of the whole study area. The highly suitable habitat was mainly located in Jiangxi and Zhejiang, central and southeastern Hunan, northern Fujian, central and western Chongqing, southern Anhui, central and surrounding areas of Guangdong, central and eastern Guangxi, and northern Taiwan, with a combined area of 0.76 × 106 km2, accounting for 7.92% of the study area. The moderately suitable habitat was mainly located in Hubei and Guizhou, southern Fujian, eastern Guangdong, western Guangxi, eastern Sichuan, southern Shaanxi, central Anhui, and central Taiwan, with a combined area of 0.87 × 106 km2, accounting for 9.06% of the study area. The poorly suitable habitat was mainly located in Jiangsu, western Guizhou, southeastern Tibet, western Guangxi, southern Gansu, central Shaanxi, central and southwestern Henan, eastern Shandong, southern Taiwan, and northern Hainan, with a combined area of 0.45 × 106 km2, accounting for 4.69% of the study area.
3.3. Potential distribution under future climate conditions
The suitability of P. massoniana was classified to obtain predictions of suitable habitats for P. massoniana in the 2050s and 2090s under future climate scenarios (Figure 6 and Supplementary Figure 1). Overall, the total area of suitable habitats under future climate scenarios was predicted to increase compared to the suitable habitat under current climate scenarios, with a significant increase in the highly and poorly suitable habitats and a decrease in the moderately suitable habitat.
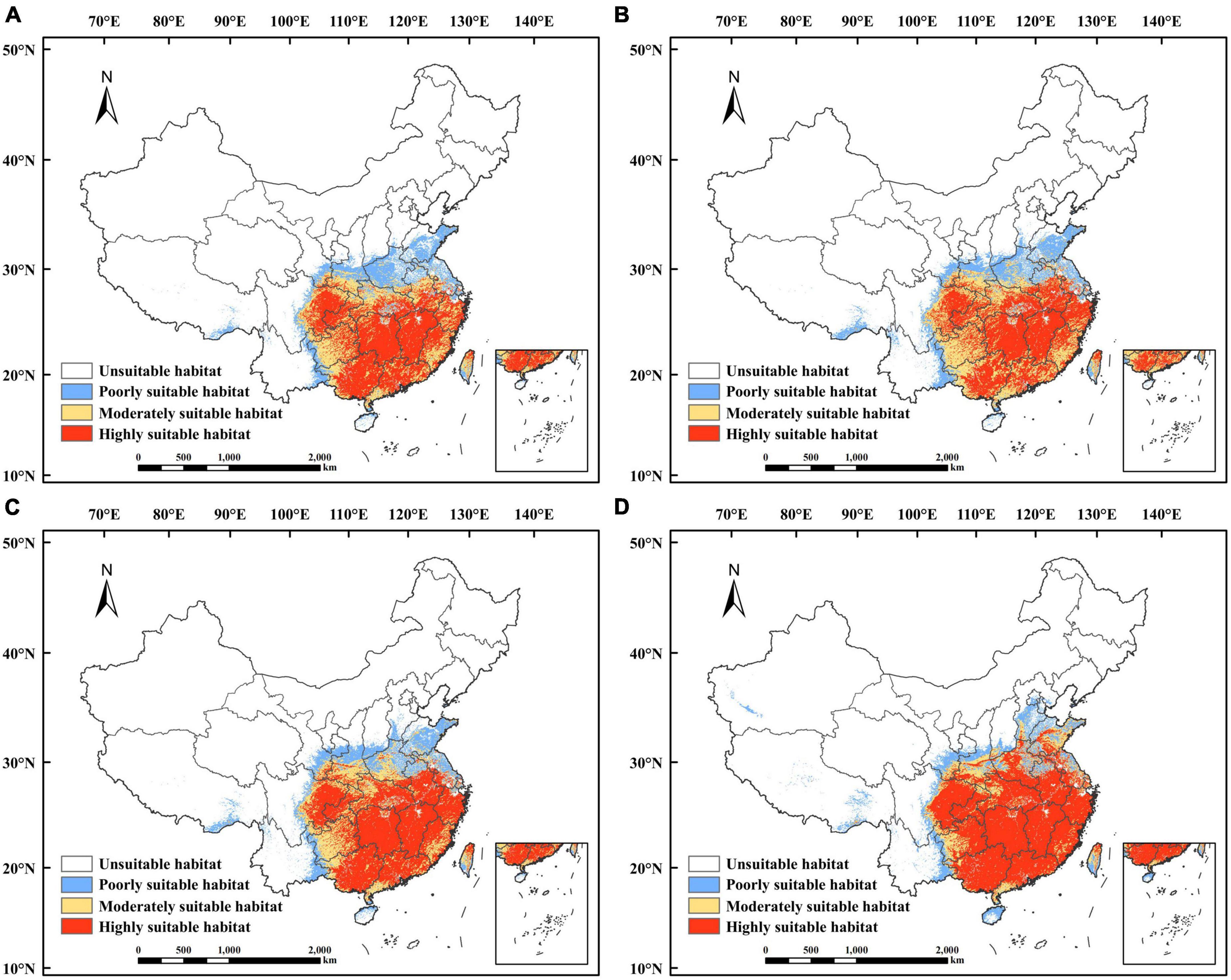
Figure 6. Potential suitable habitat of Pinus massoniana in China under future climate scenarios. Under the SSP1-2.6 scenario in (A) the 2050s and (B) the 2090s. Under the SSP5-8.5 scenario in (C) the 2050s and (D) the 2090s.
Under the SSP1-2.6 climate scenario, the total suitable habitat for P. massoniana in the 2050s was predicted to be 2.22 × 106 km2, accounting for 23.13% of the whole study area. The areas with highly, moderately, and poorly suitable habitats were predicted to be 1.09 × 106 km2, 0.62 × 106 km2, and 0.51 × 106 km2, respectively, accounting for 11.35, 6.46, and 5.31% of the study area. The highly and poorly suitable habitat areas were predicted to increase by 0.32 × 106km2 and 0.061 × 106 km2, respectively, in comparison to the corresponding suitable habitat areas in the current climate, whereas the moderately suitable habitat area was predicted to decrease by 0.25 × 106 km2. By the 2090s, under the SSP1-2.6 climate scenario, the total suitable habitat for P. massoniana was predicted to be 2.33 × 106 km2, accounting for 24.27% of the whole study area. The areas with highly, moderately, and poorly suitable habitats were predicted to be 1.08 × 106 km2, 0.72 × 106km2, and 0.53 × 106 km2, respectively, accounting for 11.25, 7.5, and 5.52% of the study area. The highly and poorly suitable habitat areas were predicted to increase by 0.32 × 106 km2 and 0.083 × 106 km2, respectively, relative to the suitable habitat areas in the current climate, whereas the moderately suitable habitat area was predicted to decrease by 0.15 × 106 km2 (Figure 6).
Under the SSP5-8.5 climate scenario, the total suitable habitat for P. massoniana in the 2050s was predicted to be 2.40 × 106 km2, accounting for 25% of the whole study area. The areas with highly, moderately, and poorly suitable habitats were predicted to be 1.31 × 106 km2, 0.58 × 106 km2, and 0.51 × 106 km2, respectively, accounting for 13.65, 6.04, and 5.31% of the study area. The highly and poorly suitable habitat areas were predicted to increase by 0.55 × 106 km2 and 0.064 × 106 km2, respectively, in comparison to the corresponding suitable habitat areas in the current climate, whereas the moderately suitable habitat area was predicted to decrease by 0.29 × 106 km2. By the 2090s, under the SSP5-8.5 climate scenario, the total suitable habitat for P. massoniana was predicted to be 2.53 × 106 km2, accounting for 26.35% of the whole study area. The areas with highly, moderately, and poorly suitable habitats were predicted to be 1.66 × 106 km2, 0.44 × 106km2, and 0.43 × 106 km2, respectively, accounting for 17.29, 4.58, and 4.48% of the study area. The highly suitable habitat area was predicted to increase by 0.90 × 106 km2, relative to the suitable habitat areas in the current climate, whereas the moderately and poorly suitable habitat areas were predicted to decrease by 0.43 × 106 km2 and 0.016 × 106 km2, respectively (Figure 6).
3.4. Potential habitat changes under future climate conditions
The projections indicated that the total suitable habitats for P. massoniana in the future periods showed an increasing trend compared to the current potential distribution, with a general expansion to the north (Figure 7 and Supplementary Figure 2). The newly suitable habitat areas were mainly located in Shandong and Hebei, in addition, northern Jiangsu, northeastern Henan, northern Anhui, southwestern Shanxi, central Shaanxi, southeastern Gansu, especially Xinjiang and Tibet gradually suitable for P. massoniana distribution (Figure 7D). The decreased area was small and mainly concentrated in a small part of Hainan. The predicted suitable habitat for P. massoniana differed less between the two different future periods under the same SSP climate scenarios, while it differed more between the different SSP climate scenarios within the same future periods. The suitable habitat changed more substantially under the SSP5-8.5 climate scenario.
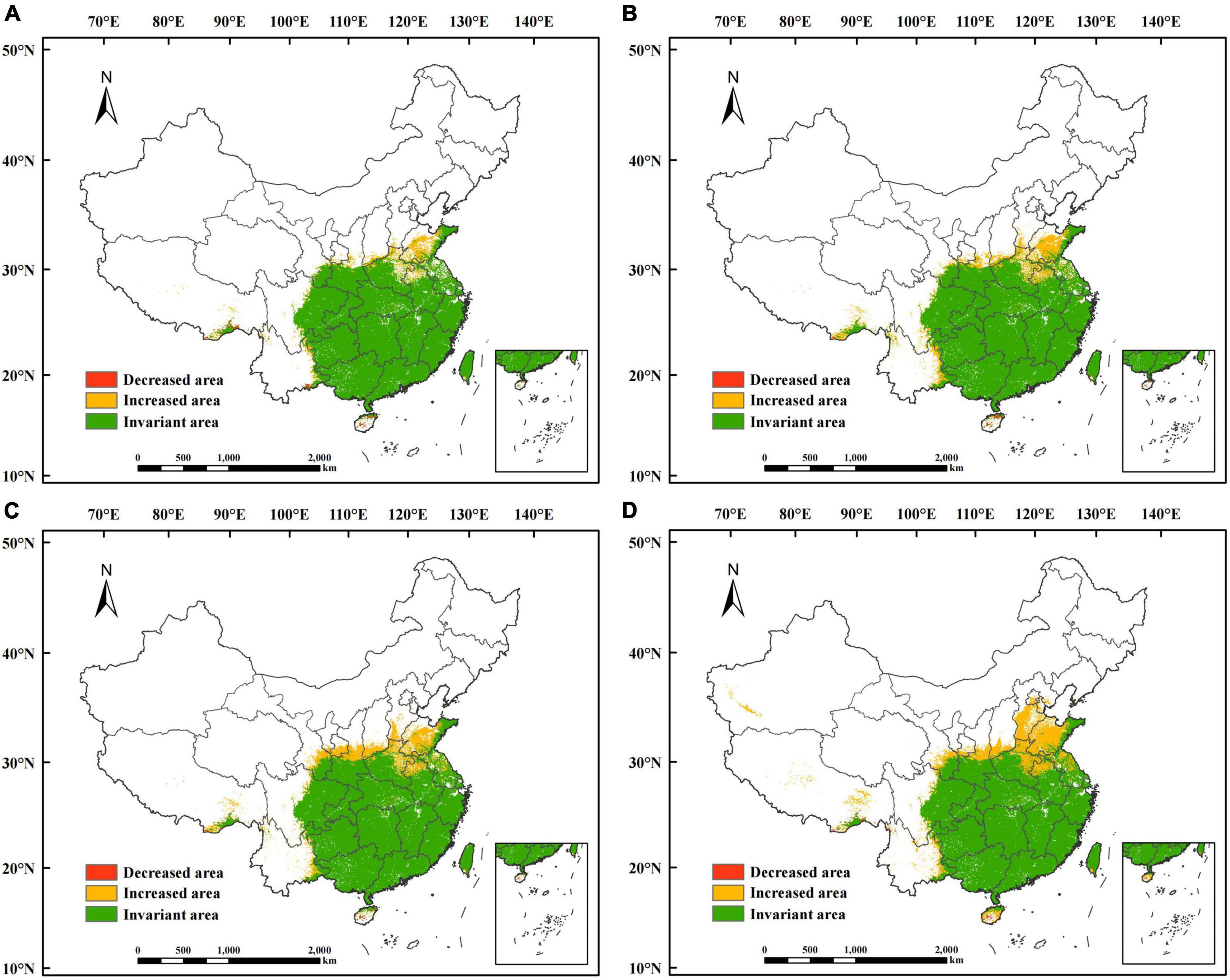
Figure 7. Change in the potential suitable habitat of Pinus massoniana under future climate scenarios. Under the SSP1-2.6 scenario in (A) the 2050s and (B) the 2090s. Under the SSP5-8.5 scenario in (C) the 2050s and (D) the 2090s.
To further evaluate the effects of different climatic scenarios on the potential geographic distribution of P. massoniana in the 2050s and 2090s, the area of suitable habitat change was predicted for each of the periods and each of the scenarios (Table 3). Under the SSP1-2.6 climate scenario, the increased area, decreased area, invariant area, and total change in the 2050s were predicted to be about 0.15 × 106, 0.015 × 106, 2.18 × 106, and 0.14 × 106 km2, accounting for 6.44, 0.64, 93.56, and 6.01% of the total suitable area, respectively. By the 2090s, under the same climate scenario, the increased area, decreased area, invariant area, and total change were predicted to be about 0.26 × 106, 0.0076 × 106, 2.19 × 106, and 0.25 × 106 km2, accounting for 10.61, 0.31, 89.39, and 10.20% of the total suitable area, respectively.
Under the SSP5-8.5 climate scenario, the increased area, decreased area, invariant area, and total change in the 2050s were predicted to be about 0.32 × 106, 0.0073 × 106, 2.19 × 106, and 0.31 × 106 km2, accounting for 12.75, 0.29, 87.25, and 12.35% of the total suitable area, respectively. By the 2090s, under the same climate scenario, the increased area, decreased area, invariant area, and total change were predicted to be about 0.45 × 106, 0.0087 × 106, 2.19 × 106, and 0.44 × 106 km2, accounting for 17.05, 0.33, 82.95, and 16.67% of the total suitable area, respectively (Table 3).
3.5. Suitable habitat centroid trends under future climate conditions
The suitable habitat of P. massoniana in different periods and different climate scenarios was extracted by ArcGIS, and the location and migration distance of the total suitable habitat centroids were calculated accordingly (Figure 8). The centroid of the suitable area under the current climate was located at 111.79° E, 28.6° N. Under the SSP1-2.6 climate scenario, the centroid of suitable habitat was predicted to move 42.29 km northeast (111.92° E, 29.01° N) by the 2050s and then 21.95 km northwest (111.76° E, 29.16° N) by the 2090s. By the 2090s, the centroid would overall be displaced by 61.98 km to the north.
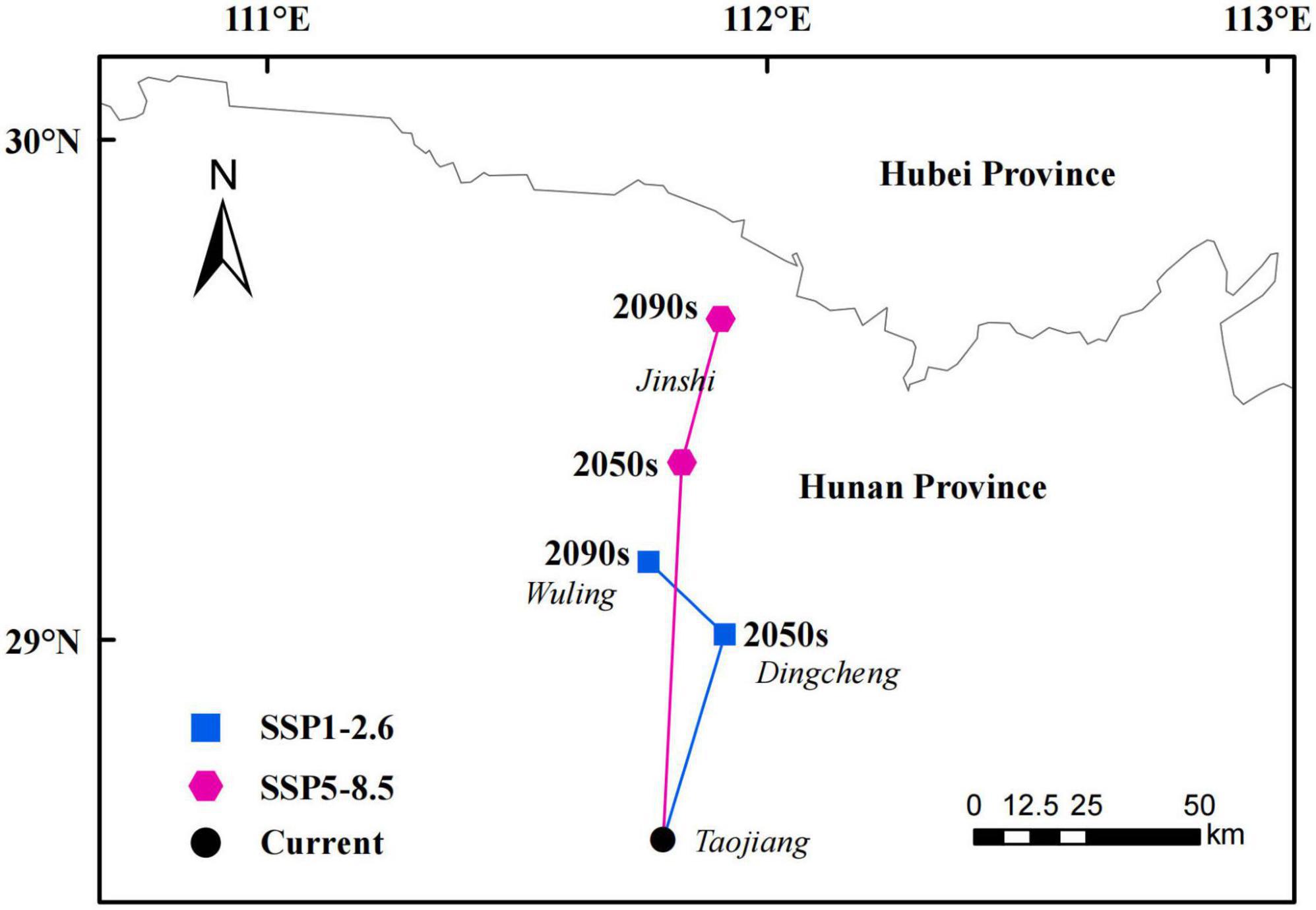
Figure 8. Total suitable habitat centroid distribution shifts under climate change for Pinus massoniana.
Under the SSP5-8.5 climate scenario, the centroid of suitable habitat was predicted to move 84.22 km north (111.83° E, 29.36° N) by the 2050s and then 32.75 km northeast (111.91° E, 29.64° N) by the 2090s. By the 2090s, the centroid would overall be displaced by 116.57 km to the north. Thus, the distribution center of suitable habitat for P. massoniana is predicted to shift northward (i.e., higher latitudes) over the coming decades. Furthermore, under the SSP 5-8.5 future climate scenario, the suitable habitat would shift further north.
4. Discussion
4.1. Main environmental variables affecting the occurrence of P. massoniana
According to the Maxent model predictions, climatic variables are the main environmental variables affecting the potential geographic distribution of P. massoniana. The response curves of environmental variables were used to illustrate the impacts of the main environmental variables on the potential geographic distribution of P. massoniana, and it was concluded that the minimum temperature of the coldest month and the precipitation of the driest month (i.e., temperature and precipitation in winter) were the key environmental variables influencing the distribution of P. massoniana. Physiological ecology studies of P. massoniana have come to similar conclusions that higher winter temperatures and adequate winter precipitation play a critical role in tree growth. Low winter temperatures can lead to wilting of needle tips and may also reduce the activity of tree roots and buds, or even cause more damage to tree roots, buds or branches, to the detriment of plant growth (Pederson et al., 2004; Zhu et al., 2009; Shi et al., 2010). On the contrary, higher winter temperatures can avoid frost damage to foliar tissues and ensure normal metabolic activities of trees, and also enable trees to enter the growing season earlier, thus extending the growing season and improving photosynthetic efficiency, which is beneficial to tree growth (Northcote and Hartman, 2008; Chen et al., 2016). On the other hand, sufficient winter precipitation increases the soil water content, which facilitates the accumulation of carbohydrates, thus providing more nutritional support for the cambium activities of trees in the following year and for tree growth in the early part of the next growing season (Jiao et al., 2021), which is also consistent with its growth in warm and moist environments (Yan et al., 2019). We assessed the contributions of the main environmental variables, finding that the contributions related to temperature and precipitation were 20.2 and 70.5%, respectively, indicating that the effect of precipitation was greater than that of temperature. This supports previous findings that precipitation plays a large impact on the potential distribution of P. massoniana than temperature (Zhang et al., 2018; Jiang et al., 2019). Water availability is essential for plant seed germination, and both long-term and short-term water deficits can constrain the growth and development of trees and seed germination (Han and Ding, 2012). Under drought stress, P. massoniana suffers from different degrees of injury, resulting in corresponding physiological and biochemical changes. Water deficit significantly affects root vigor and photosynthetic rate, which eventually causes changes in external morphology and inhibits the growth of P. massoniana (Tan et al., 2017). Therefore, precipitation should be considered a critical environmental variable informing the introduction and cultivation of P. massoniana.
4.2. Analysis of current potential suitable habitat
The main potential habitat areas of P. massoniana in our study are south of the Qinling Mountains–Huaihe River Line, and spread outward in progressively decreasing habitat classes toward northern and northwestern China. The Qinling Mountains–Huaihe River Line is the dividing line in China between the warm temperate and subtropical zones as well as the humid and semi-humid zones, with distinctly different climate characteristics (such as temperature and precipitation) between the areas north and south. The suitable areas of P. massoniana are dominated by subtropical monsoon or subtropical monsoon humid climates, which are characterized by hot summers, warm winters, and four distinct seasons (Xu et al., 2019). The warm and humid climatic factors may be the main factors affecting the distribution of P. massoniana. In addition, the jackknife test showed that soil type and soil pH were second only to climatic variables in their importance to the P. massoniana distribution (Figure 3). P. massoniana is a species that prefers acidic soil and is intolerant of saline soils (Wu et al., 2022). Most of the soil types in the suitable areas mentioned above are dominated by red, yellow, or brown loams, with varying levels of aluminum in the soil resulting in neutral to acidic soil pH values, conditions that are favorable for the growth of P. massoniana (Meng and Shi, 2017). In addition, soil salinization mainly occurs in arid areas lacking moisture or in low-lying coastal areas subject to seawater intrusion (Lin and Tang, 2005; Guo, 2018), and salinized lands in China are mainly distributed in the northwest, northeast, north, and coastal areas of China, with relatively little land salinization occurring in inland areas south of the Qinling Mountains–Huaihe River Line, and less salinized soils are also favorable for the growth of P. massoniana. This suggests that soil variables also influence the potential distribution of P. massoniana to some extent, which is consistent with our findings. In summary, it may be the suitable climate and soil environment that have ultimately influenced the formation of the modern geographical distribution pattern of P. massoniana. Meanwhile, we suggest the development of P. massoniana forests in the inland areas south of the Qinling Mountains–Huaihe River Line. However, P. massoniana afforestation should not be attempted in coastal areas, despite their suitable climatic conditions, because soil salinization may not be conducive to the growth of P. massoniana.
4.3. Analysis of future potential suitable habitat
Under future climate scenarios, the geographic distribution pattern of P. massoniana habitat generally showed a northward migration trend. The area of suitable habitat tended to increase compared to the current period, particularly under the SSP5-8.5 climate scenario in the 2090s, in which the predicted area of new suitable habitat was the largest. Numerous studies have shown that species distribution changes are closely related to climate warming and that climate change may lead to significant changes in species distribution patterns (Parmesan and Yohe, 2003; Broennimann et al., 2006; Chen et al., 2011; Li J. et al., 2019), resulting in their migration to the north (Hickling et al., 2006; Bertrand et al., 2011). Long-term climate observations suggest that under future climate conditions, the annual mean precipitation in China will increase (0–20%), especially in northern and northwestern China; moreover, the annual mean surface temperature in China is expected to increase by 2.7–2.9°C (Jiang and Fu, 2012). Additionally, the temperature increase would be more pronounced under the SSP5-8.5 climate scenario with the highest greenhouse gas emissions, which would further promote the northward migration of tree species (Li J. et al., 2019; Xie et al., 2021). Under the three future climate scenarios considered (i.e., SSP1-2.6, SSP2-4.5, and SSP5-8.5), the new suitable habitats in the 2050s and 2090s would be located mainly in northern and northwestern China. These results indicate that these regions will be affected by climate change with increased temperature and precipitation, resulting in an increase in the total suitable area for P. massoniana and a shift toward higher latitudes, consistent with its preference for warm and humid environments.
Therefore, in the context of climate change, it is important to achieve the reasonable utilization of P. massoniana resources in different suitable habitats. We should adopt a more active management mode to promote the development and utilization of P. massoniana resources in highly suitable habitats. Whereas in low suitable areas, we should control the exploitation of P. massoniana to ensure ecological benefits, considering masson pines of poor growth are more susceptible to diseases, such as Bursaphelenchus xylophilus. While expanding the forest resources of P. massoniana, the ecological service function should be improved to finally achieve a win-win situation for both economic and ecological benefits (Chen et al., 2022).
The predicted spatial patterns of change in this study were based only on the response of P. massoniana to future climate change without taking into account other factors that limit its migration. Migration capacity has important effects on the adaptation of tree species to future climate change (Corlett and Westcott, 2013), which is limited by plant community competition, geographic barriers, human activities, and land use (Zhang et al., 2020, 2021). However, it is currently difficult to integrate all influencing factors into one model to simulate the potential distribution of species (Guan et al., 2022). On the other hand, introducing too many variables into the model may lead to more collinearity-related problems, the goodness-of-fit of the model may not be better than the current model, and the effects of key variables may be weakened (Liu et al., 2022). In addition, wind dispersal and animal dispersal are the main modes of seed dispersal in P. massoniana, and their spread may be limited (Yang et al., 2013). Seeds are affected by wind speed, wind direction and topography during wind dispersal (Pan et al., 2014). Seed dispersal is also related to the behavior of dispersal animals, and the movement patterns of dispersal animals can vary in different habitats, thus affecting the distribution pattern of seeds (Barraquand and Benhamou, 2008; Van Loon et al., 2011). Because we did not take these constraints into account, the increase in suitable habitat predicted by the model and the distance that suitable habitat centroids migrate may have been overestimated. Nevertheless, the migration changes in suitable habitats predicted by this study in future periods are consistent with its growth habit and point the way to future migration trends of P. massoniana. Consequently, this study is still an important reference value for the potential suitable habitat migration of P. massoniana in the context of climate change. However, these limiting factors should be accounted for in future studies to more accurately predict the distribution of P. massoniana.
4.4. Conservation and cultivation recommendations
Climate change has already led to reduced resistance and resilience of boreal and tropical forests (Forzieri et al., 2022), and increased tree mortality in Australia (Bauman et al., 2022). The two most dominant factors of climate change, uneven precipitation distribution and increased temperature, are simultaneously the key factors affecting the distribution of P. massoniana in our predicted results. Under the background of global change, extreme events become frequently in the northern hemisphere, such as extreme low-temperature in the northern hemisphere in winter (especially the coldest month in the middle and high latitudes) and high temperature (mean temperature of warmest quarter increase) and drought (precipitation of driest month decrease) in summer (especially in subtropical China) (Voosen, 2021). Therefore, we have reasonable grounds to suspect that in the near future, the potentially suitable habitats for P. massoniana in China will be threatened (northward expansion limited by low temperature in the coldest month and southward expansion limited by high temperature and drought in summer). The potential distribution maps we simulated can serve as an important but relatively conservative reference for the protection strategy of P. massoniana, and can also protect its potential habitat more effectively (Carroll et al., 2017).
The present study supports three main recommendations for the protection and cultivation of P. massoniana. (1) Based on the potentially suitable habitat under the current climatic scenarios, P. massoniana should be protected by preserving its local hydrological and geological conditions. (2) Based on the predicted future distribution pattern of P. massoniana, we should strengthen monitoring and reduce human interference in the potential new habitats to protect the gradual migration of P. massoniana. (3) Planting activities should be tailored to moderately suitable habitats and highly suitable habitats; specifically, considering the influence of different habitats on the growth potential of plantations, we recommended developing short-term and small-diameter wood plantings in moderately suitable habitats and cultivating high-quality and large-diameter wood in highly suitable habitats.
5. Conclusion
In this study, the Maxent model was used to predict the potentially suitable habitat of P. massoniana in China based on 264 distribution records and nine environmental variables. Under the current climatic conditions, the potentially suitable habitat of P. massoniana was mainly distributed in the subtropical region south of the Qinling Mountains–Huaihe River Line. The main environmental variables that affect the potential distribution of P. massoniana were temperature and precipitation, including precipitation of the driest month, minimum temperature of the coldest month, annual temperature range, and mean temperature of the warmest quarter. Under future climate conditions, the area of potentially suitable habitat for P. massoniana was projected to increase, and the centroid of suitable habitat would thus move to higher latitudes. Under the SSP5-8.5 climate scenario, climate warming would increase due to higher greenhouse gas emissions, further promoting the migration of P. massoniana to even higher latitudes. Our study on the potential distribution of P. massoniana can serve as an important empirical reference for future conservation and planting of P. massoniana. Moreover, the methodology of this study can be applied to predict the potentially suitable habitats for other species worldwide.
Data availability statement
The original contributions presented in this study are included in the article/Supplementary material, further inquiries can be directed to the corresponding authors.
Author contributions
YC: writing—original draft, validation, investigation, and data curation. YHan and SJ: conceptualization, methodology, writing—review and editing, and funding acquisition. MZ: methodology. GW: writing—review and editing. PJ, YHu, PS, ZW, AF, and PQ: investigation and data curation. All authors contributed to the article and approved the submitted version.
Funding
This research was supported by the National Key Research and Development Program of China (2019YFE0118900), National Natural Science Foundation of China (31971641), the Natural Science Foundation of Zhejiang Province (LQ22C160002), and Jiyang College of Zhejiang A&F University under Grant (05251700031).
Acknowledgments
We thank the reviewers for their time and efforts to review and improve this work.
Conflict of interest
The authors declare that the research was conducted in the absence of any commercial or financial relationships that could be construed as a potential conflict of interest.
Publisher’s note
All claims expressed in this article are solely those of the authors and do not necessarily represent those of their affiliated organizations, or those of the publisher, the editors and the reviewers. Any product that may be evaluated in this article, or claim that may be made by its manufacturer, is not guaranteed or endorsed by the publisher.
Supplementary material
The Supplementary Material for this article can be found online at: https://www.frontiersin.org/articles/10.3389/ffgc.2023.1144401/full#supplementary-material
Footnotes
- ^ http://www.gbif.org
- ^ http://www.cvh.ac.cn/
- ^ http://www.worldclim.org/
- ^ http://www.gscloud.cn
- ^ http://www.fao.org/
References
Alexander, J. M., Chalmandrier, L., Lenoir, J., Burgess, T. I., Essl, F., Haider, S., et al. (2018). Lags in the response of mountain plant communities to climate change. Glob. Change Biol. 24, 563–579. doi: 10.1111/gcb.13976
Ashcroft, M. B., French, K. O., and Chisholm, L. A. (2011). An evaluation of environmental factors affecting species distributions. Ecol. Model. 222, 524–531. doi: 10.1016/j.ecolmodel.2010.10.003
Barraquand, F., and Benhamou, S. (2008). Animal movements in heterogeneous landscapes: identifying profitable places and homogeneous movement bouts. Ecology 89, 3336–3348. doi: 10.1890/08-0162.1
Bauman, D., Fortunel, C., Delhaye, G., Malhi, Y., Cernusak, L. A., Bentley, L. P., et al. (2022). Tropical tree mortality has increased with rising atmospheric water stress. Nature 608, 528–533. doi: 10.1038/s41586-022-04737-7
Beale, C. M., Lennon, J. J., and Gimona, A. (2008). Opening the climate envelope reveals no macroscale associations with climate in European birds. Proc. Natl. Acad. Sci. 105, 14908–14912. doi: 10.1073/pnas.0803506105
Bertin, R. I. (2008). Plant phenology and distribution in relation to recent climate change. J. Torrey Bot. Soc. 135, 126–146. doi: 10.3159/07-RP-035R.1
Bertrand, R., Lenoir, J., Piedallu, C., Riofrio-Dillon, G., de Ruffray, P., Vidal, C., et al. (2011). Changes in plant community composition lag behind climate warming in lowland forests. Nature 479, 517–520. doi: 10.1038/nature10548
Bose, R., Munoz, F., Ramesh, B., and Pélissier, R. (2016). Past potential habitats shed light on the biogeography of endemic tree species of the Western Ghats biodiversity hotspot, South India. J. Biogeogr. 43, 899–910. doi: 10.1111/jbi.12682
Bradie, J., and Leung, B. (2017). A quantitative synthesis of the importance of variables used in MaxEnt species distribution models. J. Biogeogr. 44, 1344–1361. doi: 10.1111/jbi.12894
Broennimann, O., Thuiller, W., Hughes, G., Midgley, G. F., Alkemade, J. R., and Guisan, A. (2006). Do geographic distribution, niche property and life form explain plants’ vulnerability to global change? Glob. Change Biol. 12, 1079–1093. doi: 10.1111/j.1365-2486.2006.01157.x
Brown, J. L. (2014). SDMtoolbox: a python-based GIS toolkit for landscape genetic, biogeographic and species distribution model analyses. Methods Ecol. Evol. 5, 694–700. doi: 10.1111/2041-210X.12200
Cao, M., Zhou, G., and Weng, E. (2005). Application and comparison of generalized models and classification and regression tree in simulating tree species distribution. Acta Ecol. Sin. 25, 2031–2040.
Carroll, C., Roberts, D. R., Michalak, J. L., Lawler, J. J., Nielsen, S. E., Stralberg, D., et al. (2017). Scale-dependent complementarity of climatic velocity and environmental diversity for identifying priority areas for conservation under climate change. Glob. Change Biol. 23, 4508–4520. doi: 10.1111/gcb.13679
Chen, D., Fang, K., Li, Y., Dong, Z., Zhang, Y., and Zhou, F. (2016). Response of Pinus taiwanensis growth to climate changes at its southern limit of Daiyun Mountain, mainland China Fujian Province. Sci. China Earth Sci. 59, 328–336. doi: 10.1007/s11430-015-5188-1
Chen, I.-C., Hill, J. K., Ohlemüller, R., Roy, D. B., and Thomas, C. D. (2011). Rapid range shifts of species associated with high levels of climate warming. Science 333, 1024–1026. doi: 10.1126/science.1206432
Chen, Y.-G., Xg, L., Chen, Y.-H., Cheng, W.-X., Jg, D., Zhong, Q.-L., et al. (2022). Identification of the potential distribution area of Cunninghamia lanceolata in China under climate change based on the MaxEnt model. J. Appl. Ecol. 33, 1207–1214.
Corlett, R. T., and Westcott, D. A. (2013). Will plant movements keep up with climate change? Trends Ecol. Evol. 28, 482–488. doi: 10.1016/j.tree.2013.04.003
Dong, H., Ge, J., Sun, K., Wang, B., Xue, J., Wakelin, S. A., et al. (2021). Change in root-associated fungal communities affects soil enzymatic activities during Pinus massoniana forest development in subtropical China. For. Ecol. Manag. 482:118817. doi: 10.1016/j.foreco.2020.118817
Duflot, R., Avon, C., Roche, P., and Bergès, L. (2018). Combining habitat suitability models and spatial graphs for more effective landscape conservation planning: an applied methodological framework and a species case study. J. Nat. Conserv. 46, 38–47. doi: 10.1016/j.jnc.2018.08.005
Dyderski, M. K., Paź, S., Frelich, L. E., and Jagodziński, A. M. (2018). How much does climate change threaten European forest tree species distributions? Glob. Change Biol. 24, 1150–1163. doi: 10.1111/gcb.13925
Elith, J., Graham, C. H., Anderson, R. P., Dudík, M., Ferrier, S., Guisan, A., et al. (2006). Novel methods improve prediction of species’ distributions from occurrence data. Ecography 29, 129–151. doi: 10.1111/j.2006.0906-7590.04596.x
Eyring, V., Bony, S., Meehl, G. A., Senior, C. A., Stevens, B., Stouffer, R. J., et al. (2016). Overview of the coupled model intercomparison project phase 6 (CMIP6) experimental design and organization. Geosci. Model Dev. 9, 1937–1958. doi: 10.5194/gmd-9-1937-2016
Feeley, K., Bravo-Avila, C., Fadrique, B., Perez, T., and Zuleta, D. (2020). Climate-driven changes in the composition of New World plant communities. Nat. Clim. Change 10, 965–970. doi: 10.1038/s41558-020-0873-2
Fick, S. E., and Hijmans, R. J. (2017). WorldClim 2: new 1-km spatial resolution climate surfaces for global land areas. Int. J. Climatol. 37, 4302–4315. doi: 10.1002/joc.5086
Forzieri, G., Dakos, V., McDowell, N. G., Ramdane, A., and Cescatti, A. (2022). Emerging signals of declining forest resilience under climate change. Nature 608, 534–539. doi: 10.1038/s41586-022-04959-9
Guan, L., Yang, Y., Jiang, P., Mou, Q., Gou, Y., Zhu, X., et al. (2022). Potential distribution of Blumea balsamifera in China using MaxEnt and the ex situ conservation based on its effective components and fresh leaf yield. Environ. Sci. Pollut. Res. Int. 29, 44003–44019. doi: 10.1007/s11356-022-18953-1
Guo, Y., Li, X., Zhao, Z., Wei, H., Gao, B., and Gu, W. (2017). Prediction of the potential geographic distribution of the ectomycorrhizal mushroom Tricholoma matsutake under multiple climate change scenarios. Sci. Rep. 7, 1–11. doi: 10.1038/srep46221
Guo, Z. (2018). Analysis of Soil Salinization Characteristics in Tianjin Binhai and Its Surrounding Areas. Tianjin: Tianjin University.
Han, W., and Ding, G. (2012). The responses of water stress on germination of Pinus massoniana seeds from different provenance. Seed 31, 81–86.
He, Y., Huang, W., Zhao, X., Lv, P., and Wang, H. (2021). Review on the impact of climate change on plant diversity. J. Desert Res. 41:59.
Hernandez, P. A., Graham, C. H., Master, L. L., and Albert, D. L. (2006). The effect of sample size and species characteristics on performance of different species distribution modeling methods. Ecography 29, 773–785. doi: 10.1111/j.0906-7590.2006.04700.x
Hickling, R., Roy, D. B., Hill, J. K., Fox, R., and Thomas, C. D. (2006). The distributions of a wide range of taxonomic groups are expanding polewards. Glob. Change Biol. 12, 450–455. doi: 10.1111/j.1365-2486.2006.01116.x
Hu, X.-G., Wang, T., Liu, S.-S., Jiao, S.-Q., Jia, K.-H., Zhou, S.-S., et al. (2017). Predicting future seed sourcing of Platycladus orientalis (L.) for future climates using climate niche models. Forests 8:471. doi: 10.3390/f8120471
Huang, X., Dai, D., Xiang, Y., Yan, Z., Teng, M., Wang, P., et al. (2021). Radial growth of Pinus massoniana is influenced by temperature, precipitation, and site conditions on the regional scale: a meta-analysis based on tree-ring width index. Ecol. Indic. 126:107659. doi: 10.1016/j.ecolind.2021.107659
Jian, S., Zhu, T., Wang, J., and Yan, D. (2022). The current and future potential geographical distribution and evolution process of Catalpa bungei in China. Forests 13:96. doi: 10.3390/f13010096
Jiang, D., and Fu, Y. (2012). Climate change over China with a 2°C global warming. J. Atmos. Sci. 36, 234–246.
Jiang, Y., Li, M., Liu, Y., and Liu, F. (2019). Impact of climate change on suitable habitats of Pinus massoniana in hunan province. J. Nanjing For. Univ. 62:94.
Jiao, L., Xue, R., Qi, C., Chen, K., and Liu, X. (2021). Comparison of the responses of radial growth to climate change for two dominant coniferous tree species in the eastern Qilian Mountains, northwestern China. Int. J. Biometeorol. 65, 1823–1836. doi: 10.1007/s00484-021-02139-4
Jochum, G. M., Mudge, K. W., and Thomas, R. B. (2007). Elevated temperatures increase leaf senescence and root secondary metabolite concentrations in the understory herb Panax quinquefolius (Araliaceae). Am. J. Bot. 94, 819–826. doi: 10.3732/ajb.94.5.819
Kaky, E., Nolan, V., Alatawi, A., and Gilbert, F. (2020). A comparison between ensemble and MaxEnt species distribution modelling approaches for conservation: a case study with Egyptian medicinal plants. Ecol. Inform. 60:101150. doi: 10.1016/j.ecoinf.2020.101150
Kolli, M. K., Pham, Q. B., Thi Thuy Linh, N., Ngoc Hoai, P., Costache, R., and Anh, D. T. (2022). Assessment of change in the extent of mangrove ecosystems using different spectral indices in Google Earth Engine based on random forest model. Arab. J. Geosci. 15, 1–12. doi: 10.1007/s12517-022-10158-7
Lei, L., Xiao, W., Zeng, L., Liu, C., Zhu, J., and Li, M.-H. (2022). Phenological season-dependent temperature effects on soil respiration in a subtropical Pinus massoniana forest. Agric. For. Meteorol. 323:109086. doi: 10.1016/j.agrformet.2022.109086
Li, H., Liu, X., Fu, Y., and Wang, J. (2019). Study on suitable bioclimatic characteristics of Pinus massoniana in chongqing by using MaxEnt model. Ecol. Sci. [Epub ahead of print].
Li, J., Chang, H., Liu, T., and Zhang, C. (2019). The potential geographical distribution of Haloxylon across Central Asia under climate change in the 21st century. Agric. For. Meteorol. 275, 243–254. doi: 10.1016/j.agrformet.2019.05.027
Lin, N., and Tang, J. (2005). Study on the environment evolution and the analysis of causes to land salinization and desertification in Songnen plain. Q. Sci. 25, 474–485.
Lin, W., Xu, M., Quan, Y., Liao, L., and Gao, L. (2019). Potential geographic distribution of Spodoptera frugiperda in China based on MaxEnt model. Plant Quarantine 33, 69–73.
Liu, J., Xu, Y., Sun, C., Wang, X., Zheng, Y., Shi, S., et al. (2022). Distinct ecological habits and habitat responses to future climate change in three east and southeast Asian Sapindus species. For. Ecol. Manag. 507:119982. doi: 10.1016/j.foreco.2021.119982
Liu, Y., Huang, P., Lin, F., Yang, W., Gaisberger, H., Christopher, K., et al. (2019). MaxEnt modelling for predicting the potential distribution of a near threatened rosewood species (Dalbergia cultrata Graham ex Benth). Ecol. Eng. 141:105612. doi: 10.1016/j.ecoleng.2019.105612
Lopatin, J., Dolos, K., Hernández, H., Galleguillos, M., and Fassnacht, F. (2016). Comparing generalized linear models and random forest to model vascular plant species richness using LiDAR data in a natural forest in central Chile. Remote Sens. Environ. 173, 200–210. doi: 10.1016/j.rse.2015.11.029
Lu, S., Chen, Y., Zhou, S., and Yin, X. (2020). Responses of Pinus species to climate change in Southwestern China. J. For. Environ. 40, 466–477.
Mckenney, D. W., Pedlar, J. H., Lawrence, K., Campbell, K., and Hutchinson, M. F. (2007). Potential impacts of climate change on the distribution of North American trees. BioScience 57, 939–948. doi: 10.1641/B571106
Meng, Y., and Shi, L. (2017). Stability of aluminum in mineral conditioners and amelioration on acid soil. Soils 49, 345–349.
Narouei-Khandan, H. A., Halbert, S. E., Worner, S. P., and van Bruggen, A. H. C. (2016). Global climate suitability of citrus huanglongbing and its vector, the Asian citrus psyllid, using two correlative species distribution modeling approaches, with emphasis on the USA. Eur. J. Plant Pathol. 144, 655–670. doi: 10.1007/s10658-015-0804-7
National forestry and Grassland administration (2019). China Forest Resources Report. Beijing: China Forestry Publishing House.
Northcote, T. G., and Hartman, G. (2008). Fishes and Forestry: Worldwide Watershed Interactions and Management. New York, NY: John Wiley & Sons.
Pan, Y., Wang, S., Wang, C., Zhu, X., Ren, P., Hu, M., et al. (2014). Comparison of seed weed-dispersed characteristics between Pinus yunnanensis and Keteleeria evelyniana. Plant Divers. Resour. 36, 403–410.
Parmesan, C., and Yohe, G. (2003). A globally coherent fingerprint of climate change impacts across natural systems. Nature 421, 37–42. doi: 10.1038/nature01286
Pearson, R. G., Raxworthy, C. J., Nakamura, M., and Townsend Peterson, A. (2006). Original article: predicting species distributions from small numbers of occurrence records: a test case using cryptic geckos in Madagascar. J. Biogeogr. 34, 102–117. doi: 10.1111/j.1365-2699.2006.01594.x
Pederson, N., Cook, E. R., Jacoby, G. C., Peteet, D. M., and Griffin, K. L. (2004). The influence of winter temperatures on the annual radial growth of six northern range margin tree species. Dendrochronologia 22, 7–29. doi: 10.1016/j.dendro.2004.09.005
Peterson, A. T., Soberón, J., Pearson, R. G., Anderson, R. P., Martínez-Meyer, E., Nakamura, M., et al. (eds) (2011). “Ecological niches and geographic distributions (MPB-49),” in Ecological Niches and Geographic Distributions (MPB-49), (Princeton, NJ: Princeton University Press). doi: 10.23943/princeton/9780691136868.001.0001
Phillips, S. J. (2008). Transferability, sample selection bias and background data in presence-only modelling: a response to Peterson et al. (2007). Ecography 31, 272–278. doi: 10.1111/j.0906-7590.2008.5378.x
Phillips, S. J., Anderson, R. P., and Schapire, R. E. (2006). Maximum entropy modeling of species geographic distributions. Ecol. Model. 190, 231–259. doi: 10.1016/j.ecolmodel.2005.03.026
Popp, A., Calvin, K., Fujimori, S., Havlik, P., Humpenöder, F., Stehfest, E., et al. (2017). Land-use futures in the shared socio-economic pathways. Glob. Environ. Change 42, 331–345. doi: 10.1016/j.gloenvcha.2016.10.002
Prevéy, J. S., Parker, L. E., Harrington, C. A., Lamb, C. T., and Proctor, M. F. (2020). Climate change shifts in habitat suitability and phenology of huckleberry (Vaccinium membranaceum). Agric. For. Meteorol. 280:107803. doi: 10.1016/j.agrformet.2019.107803
Qin, A., Liu, B., Guo, Q., Bussmann, R. W., Ma, F., Jian, Z., et al. (2017). Maxent modeling for predicting impacts of climate change on the potential distribution of Thuja sutchuenensis Franch., an extremely endangered conifer from southwestern China. Glob. Ecol. Conserv. 10, 139–146. doi: 10.1016/j.gecco.2017.02.004
Qin, Z., Zhang, J.-E., Di Tommaso, A., Wang, R.-L., and Wu, R.-S. (2015). Predicting invasions of Wedelia trilobata (L.) Hitchc. with Maxent and GARP models. J. Plant Res. 128, 763–775. doi: 10.1007/s10265-015-0738-3
Shao, C., Duan, H., Ding, G., Luo, X., Fu, Y., and Lou, Q. (2022). Physiological and biochemical dynamics of Pinus massoniana lamb. seedlings under extreme drought stress and during recovery. Forests 13:65. doi: 10.3390/f13010065
Shi, J., Cook, E. R., Lu, H., Li, J., Wright, W. E., and Li, S. (2010). Tree-ring based winter temperature reconstruction for the lower reaches of the Yangtze River in southeast China. Clim. Res. 41, 169–175. doi: 10.3354/cr00851
Stocker, T., Qin, D., Plattner, G. K., Tignor, M., Allen, S. K., Boschung, J., et al. (2013). IPCC, 2013: Climate Change 2013: The Physical Science Basis. Contribution of Working Group I to the Fifth Assessment Report of the Intergovernmental Panel on Climate Change. Cambridge, MA: Cambridge University Press.
Tan, J., Tang, S., and Chen, H. (2017). Advances of drought resistance in Pinus massoniana. Guangxi For. Sci. 46, 1–7.
Thuiller, W., Guéguen, M., Renaud, J., Karger, D. N., and Zimmermann, N. E. (2019). Uncertainty in ensembles of global biodiversity scenarios. Nat. Commun. 10, 1–9. doi: 10.1038/s41467-019-09519-w
Thurm, E. A., Hernandez, L., Baltensweiler, A., Ayan, S., Rasztovits, E., Bielak, K., et al. (2018). Alternative tree species under climate warming in managed European forests. For. Ecol. Manag. 430, 485–497. doi: 10.1016/j.foreco.2018.08.028
Van Loon, A., Soomers, H., Schot, P., Bierkens, M., Griffioen, J., and Wassen, M. (2011). Linking habitat suitability and seed dispersal models in order to analyse the effectiveness of hydrological fen restoration strategies. Biol. Conserv. 144, 1025–1035. doi: 10.1016/j.biocon.2010.12.021
Voosen, P. (2021). Global temperatures in 2020 tied record highs. Science 371, 334–335. doi: 10.1126/science.371.6527.334
Wang, H., Liu, S., Song, Z., Yang, Y., Wang, J., You, Y., et al. (2019). Introducing nitrogen-fixing tree species and mixing with Pinus massoniana alters and evenly distributes various chemical compositions of soil organic carbon in a planted forest in southern China. For. Ecol. Manag. 449:117477. doi: 10.1016/j.foreco.2019.117477
Wang, H., Wu, F., Li, M., Zhu, X., Shi, C., Shao, C., et al. (2022). Structure and chlorophyll fluorescence of heteroblastic foliage affect first-year growth in Pinus massoniana Lamb. seedlings. Plant Physiol. Biochem. 170, 206–217. doi: 10.1016/j.plaphy.2021.12.006
Wang, X., Li, S., Huang, S., Cui, Y., Fu, H., Li, T., et al. (2021). Pinus massoniana population dynamics: driving species diversity during the pioneer stage of ecological restoration. Glob. Ecol. Conserv. 27:e01593. doi: 10.1016/j.gecco.2021.e01593
Wu, F., Zhu, P., and Ji, K. (2022). Response of masson pine (Pinus massoniana) distribution pattern to future climate change. J. Nanjing For. Univ. 46, 196–204.
Xie, C., Huang, B., Jim, C. Y., Han, W., and Liu, D. (2021). Predicting differential habitat suitability of Rhodomyrtus tomentosa under current and future climate scenarios in China. For. Ecol. Manag. 501:119696. doi: 10.1016/j.foreco.2021.119696
Xin, X., Wu, T., Zhang, J., Yao, J., and Fang, Y. (2020). Comparison of CMIP6 and CMIP5 simulations of precipitation in China and the East Asian summer monsoon. Int. J. Climatol. 40, 6423–6440. doi: 10.1002/joc.6590
Xu, D., Zhuo, Z., Wang, R., Ye, M., and Pu, B. (2019). Modeling the distribution of Zanthoxylum armatum in China with MaxEnt modeling. Glob. Ecol. Conserv. 19:e00691. doi: 10.1016/j.gecco.2019.e00691
Yan, H., Feng, L., Zhao, Y., Feng, L., Wu, D., and Zhu, C. (2020). Prediction of the spatial distribution of Alternanthera philoxeroides in China based on ArcGIS and MaxEnt. Glob. Ecol. Conserv. 21:e00856. doi: 10.1016/j.gecco.2019.e00856
Yan, Y., Cen, Y., Zhang, P., Zhang, Y., Liu, X., Li, C., et al. (2019). Predicting distribution pattern and 399 future change of Pinus massoniana in China based on MaxEnt model. Chinese J. Ecol. 38, 2896–2901.
Yang, Q., Yang, H., and Liu, H. (2013). Dispersal modes of plant seeds and their adaptability. J. Jiaying Univ. 31, 50–59.
Ye, X., Zhang, M., Yang, Q., Ye, L., Liu, Y., Zhang, G., et al. (2022). Prediction of suitable distribution of a critically endangered plant Glyptostrobus pensilis. Forests 13:257. doi: 10.3390/f13020257
Yi, Y.-J., Cheng, X., Yang, Z.-F., and Zhang, S.-H. (2016). Maxent modeling for predicting the potential distribution of endangered medicinal plant (H. riparia Lour) in Yunnan, China. Ecol. Eng. 92, 260–269. doi: 10.1016/j.ecoleng.2016.04.010
Yuan, Z., Jin, X., Xiao, W., Wang, L., Sun, Y., Guan, Q., et al. (2022). Comparing soil organic carbon stock and fractions under natural secondary forest and Pinus massoniana plantation in subtropical China. Catena 212:106092. doi: 10.1016/j.catena.2022.106092
Zhang, J., Liu, J., Yu, K., Zeng, Q., Zheng, W., Ai, J., et al. (2018). Prediction of geographical distribution of main conifer species under climate change in Sanming City. J. Fujian Agric. For. Univ. 47, 74–81.
Zhang, J.-H., Li, K.-J., Liu, X.-F., Yang, L., and Shen, S.-K. (2021). Interspecific variance of suitable habitat changes for four alpine Rhododendron species under climate change: implications for their reintroductions. Forests 12:1520. doi: 10.3390/f12111520
Zhang, L., Zhu, L., Li, Y., Zhu, W., and Chen, Y. (2022). Maxent modelling predicts a shift in suitable habitats of a subtropical evergreen tree (Cyclobalanopsis glauca (Thunberg) Oersted) under climate change scenarios in China. Forests 13:126. doi: 10.3390/f13010126
Zhang, Y., Liu, Y., Zhang, X., Qin, H., Wang, G., and Wang, W. (2020). Impacts of climate change on the suitable habitats and spatial migration of Xanthoceras sorbifolia. China Environ. Sci. 40, 4597–4606.
Zhao, Y., Deng, X., Xiang, W., Chen, L., and Ouyang, S. (2021). Predicting potential suitable habitats of Chinese fir under current and future climatic scenarios based on Maxent model. Ecol. Inform. 64:101393. doi: 10.1016/j.ecoinf.2021.101393
Zhu, H., Fang, X., Shao, X., and Yin, Z. (2009). Tree ring-based February–April temperature reconstruction for Changbai Mountain in Northeast China and its implication for East Asian winter monsoon. Clim. Past 5, 661–666. doi: 10.5194/cp-5-661-2009
Zhu, H., Jiang, Z., Li, J., Li, W., Sun, C., and Li, L. (2020a). Does CMIP6 inspire more confidence in simulating climate extremes over China? Adv. Atmos. Sci. 37, 1119–1132. doi: 10.1007/s00376-020-9289-1
Keywords: Pinus massoniana Lamb., Maxent, potential distribution, climate change, suitable habitat
Citation: Chi Y, Wang GG, Zhu M, Jin P, Hu Y, Shu P, Wang Z, Fan A, Qian P, Han Y and Jin S (2023) Potentially suitable habitat prediction of Pinus massoniana Lamb. in China under climate change using Maxent model. Front. For. Glob. Change 6:1144401. doi: 10.3389/ffgc.2023.1144401
Received: 14 January 2023; Accepted: 27 February 2023;
Published: 14 March 2023.
Edited by:
Hongfang Zhao, East China Normal University, ChinaReviewed by:
Chongyang Xu, Hebrew University of Jerusalem, IsraelHongzhang Kang, Shanghai Jiao Tong University, China
Copyright © 2023 Chi, Wang, Zhu, Jin, Hu, Shu, Wang, Fan, Qian, Han and Jin. This is an open-access article distributed under the terms of the Creative Commons Attribution License (CC BY). The use, distribution or reproduction in other forums is permitted, provided the original author(s) and the copyright owner(s) are credited and that the original publication in this journal is cited, in accordance with accepted academic practice. No use, distribution or reproduction is permitted which does not comply with these terms.
*Correspondence: Yini Han, hanyini@zafu.edu.cn; Songheng Jin, shjin@zafu.edu.cn