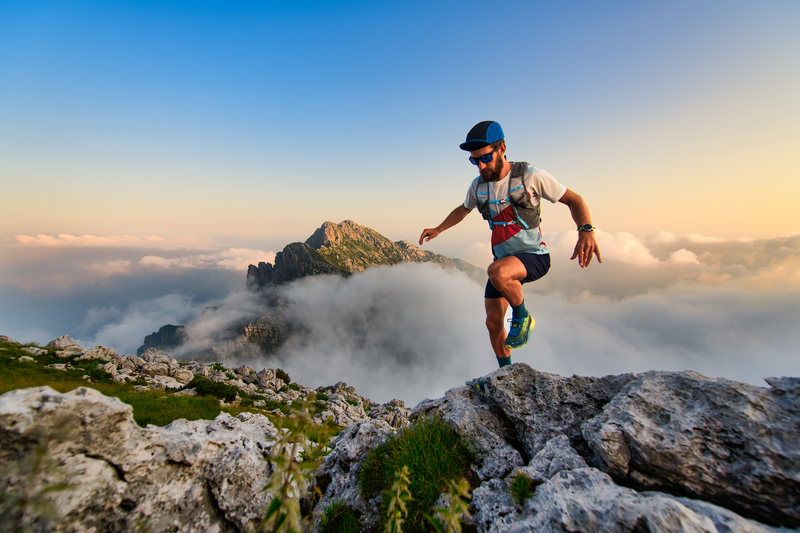
95% of researchers rate our articles as excellent or good
Learn more about the work of our research integrity team to safeguard the quality of each article we publish.
Find out more
ORIGINAL RESEARCH article
Front. For. Glob. Change , 06 February 2023
Sec. Forest Management
Volume 6 - 2023 | https://doi.org/10.3389/ffgc.2023.1124677
This article is part of the Research Topic Mountainous Forest Ecosystems: Challenges and Management Implications View all 16 articles
Soil erosion is a major environmental problem that affects land and w ater resources. It has many negative implications that lead to deforestation, poor agricultural practices, loss of soil fertility, and siltation that hinder socio-economic development. In view of this, the present study was conducted with the aim of estimating soil loss in relation to long-term land use/land cover change (LULC) in the Dehar watershed, Himachal Himalaya, North India. The study was carried out using Landsat and Sentinel imageries for the years 1999, 2010, and 2020. A GIS-based Revised Universal Soil Loss Equation (RUSLE) model was applied to assess the potential soil risk. The parameters used as input for computing the spatiotemporal changes of soil loss were rainfall erosivity, soil erodibility, topographic, crop management, and conservation support practice factors. The results showed a mean soil loss of 63.71, 60.99, and 66.71 t/ha/yr for the years 1999, 2010, and 2020, respectively. In the LULC class defined as Built-up Land, the mean soil loss decreased from 32.19 t/ha/yr in 1999 to 18.77 t/ha/yr in 2010, and in the year 2020 the mean soil loss slightly increased to 20.15 t/ha/yr. Moreover, the LULC class Barren Land registered a decrease in mean soil loss for the years 1999, 2010, and 2020 of 86.43, 74.60, and 73.19 t/ha/yr, respectively. Regarding the Agriculture Land class, the rate of mean soil loss slightly increased from 32.55 t/ha/yr in 1999 to 33.35 t/ha/yr in 2010, and the mean soil loss decreased to 25.43 t/ha/yr in the year 2020. Areas covered under Forest Land experienced an increase in mean soil loss from 65.30 t/ha/yr in 1999 to 65.87 in 2010 and 74.72 t/ha/yr in 2020. The study demonstrated that LULC changes apparently influenced the soil loss in the Dehar watershed. Therefore, urgent interventions are required with the involvement of scientists, policymakers, and the general public for conservation and management of soil resources.
Land use/land cover change (LULC) change is one of the leading global environmental problems and poses an alarming threat to humankind. LULC change is a decisive factor in most of the initiatives carried out by the public, as can be evidenced by various development activities taken up in almost all parts of the globe (Steffen et al., 2001; Glaeser and Kahn, 2004; Lambin et al., 2010; Ayele et al., 2014; Haregeweyn et al., 2015). Land use change is a key factor that contributes to soil erosion and is of prime concern in environmental studies (Senanayake et al., 2020). Moreover, LULC changes are considered to affect the hydrological characteristics of a watershed and enhance land degradation problems like soil erosion and sedimentation if they are not addressed scientifically (Abdulkareem et al., 2019; Mohammadi et al., 2021). Hence, knowing LULC dynamics and their implications on soil erosion can help policymakers in decision-making processes (Waltner et al., 2020). Extensive soil erosion results in soil erosion hazards, which influence landscape processes including land productivity, hydrological activity, and eventual human wellbeing. Therefore, soil erosion assessment is important for understanding the dynamics of landscape processes.
Soil erosion plays a pivotal role in land degradation and is considered a serious environmental hazard (Eswaran et al., 2001; Panagos et al., 2017; Poesen, 2018; Steinmetz et al., 2018). In recent times, land degradation has become a major environmental concern in many regions of the world, particularly in developing countries where agriculture is a main occupation (Krishna Bahadur, 2009; Xu et al., 2013; Rawat et al., 2016; Samanta et al., 2016; Saha et al., 2018). Further, it has many negative impacts including the loss of soil fertility that hinders socio-economic development on a global scale (Kouli et al., 2009).
At a global level, nearly 85% of land degradation is mainly caused by soil erosion (Tang et al., 2015). Soil erosion has become a serious issue in almost all parts of the globe (Pimentel and Burgess, 2013; Prashanth et al., 2021, 2022). Moreover, LULC change is affecting the availability of natural resources mainly soil resources in most regions of the world (Abdulkareem et al., 2019; Mohammadi et al., 2021; Zhu et al., 2022; Moisa et al., 2023). Generally, soil erosion is a natural process but has become a significant environmental issue in the last century due to anthropogenic interventions (Alkharabsheh et al., 2013). The problem is expected to exacerbate in the 21st century (Hurni et al., 2005). Out of the different types of soil erosion, the effects caused by water erosion are of more concern, as this disturbs soil texture, structure, and quality, as well as endangers other natural resources, such as land and water, which mankind depend upon for survival (Pimentel et al., 1993; Hrissanthou et al., 2010; Yang et al., 2013; Keno and Suryabhagavan, 2014; Srinivasan et al., 2019; Kolli et al., 2021). The immediate on-the-spot effect is a decrease in soil productivity, and ex-situ impacts include sediment deposition that triggers floods (Meshesha et al., 2012; Negash et al., 2021). Further, anthropogenic interference often intensifies the process of soil erosion on the steep slopes of highly elevated areas particularly in a mountainous ecosystem, because of various unsustainable activities such as deforestation, forest fires, intensive farming, and improper land management practices (Mandal and Sharda, 2013; Jayasekara et al., 2018).
Stocking (1993) depicted land degradation as a global environmental problem and found it to be a major environmental crisis in the developing world. Land degradation is one of the ecologically sensitive issues in India, and increasing populations, increasing urbanization, and over-exploitation of natural resources are the main drivers of land degradation. Moreover, Singh and Panda (2017) observed that out of the total geographical area of India, nearly 47.7% of the area suffers from land degradation. Further, the National Bureau of Soil Survey and Land Use Planning (NBSS and LUP, 2005) estimated that almost 146.8 Mha of land in India is degraded. Ravi et al. (2010) viewed soil erosion as one of the major processes of land degradation spreading in arid and semi-arid regions of India.
In India, soil erosion due to water is considered to be the most severe land degradation aspect that affects the topsoil and landscape of an area in general and Himalaya in particular (Mandal and Sharda, 2011; Bhattacharyya et al., 2015; Mahapatra et al., 2018). Himalaya is considered a highly land-degraded region due to various factors including soil erosion, which poses a major challenge to mountain ecosystems (Stoddart, 1969; Chalise et al., 2019). Most of the climatic regions of the world, including mountain areas such as Himalaya, are prone to soil erosion (e.g., Garcia-Ruiz et al., 2015). The main drivers of soil erosion in Himalaya are thought to be associated with both natural and anthropogenic activities (Hamilton, 1987; Jain et al., 2001; Prashanth et al., 2021, 2022). Thus, soil erosion assessment in highly ecologically sensitive Himalayan watersheds will be supportive to land degradation evaluation and to minimize the stress on sustainable agricultural practices (Singh and Singh, 2018).
Oliveira et al. (2019) observed that runoff from mountain regions toward regions of lower elevations increases the rate of erosion of an area. Further, regular land-use changes stimulate runoff and soil productivity loss, thus resulting in land degradation (Ang and Oeurng, 2018). Sharma (2008) assessed that out of the total degraded land in the Himalaya region, 79% is related to water erosion and is mostly confined to the river catchments. Significantly, lower Himalaya is more prone to soil erosion resulting in land degradation and making the land unproductive (Kaiser, 2004). About 54% of the state of Himachal Pradesh, Himachal Himalaya (North India) is prone to soil erosion, out of which, 98% is mainly because of water erosion (Kumar et al., 2014). The uneven complex dissected terrain, tectonic pressure, and diverse climatological influences supported by anthropogenic interventions have increased stress on natural resources, mainly land resources in lower Himalaya (Prashanth et al., 2021). Hence, under these circumstances, the estimation of soil loss with emphasis on land-degraded areas is quite necessary for sustainable planning and effective conservation of natural resources in Himalaya (Yadav and Sidhu, 2010).
Several models have been developed to predict soil erosion, including: empirical models such as the Universal Soil Loss Equation (USLE) developed by Wischmeier and Smith (1978); a revised model of the USLE known as the Revised Universal Soil Loss Equation (RUSLE) (Renard et al., 1997); and process-based models like the USDA-Water Erosion Prediction Project (WEPP) (Nearing et al., 1989), European Soil Erosion Model (EUROSEM) (Morgan et al., 1998), and Large Scale Catchment Model LASCAM (Viney and Sivapalan, 1999). Though many models are used for predicting soil erosion, the most widely used model for soil erosion estimation studies is the RUSLE (Shrestha, 1997; Angima et al., 2003; Prasannakumar et al., 2011, 2012; Mandal and Sharda, 2013; Wang et al., 2013; Pan and Wen, 2014; Erol et al., 2015; Uddin et al., 2016; Panditharathne et al., 2019). For assessing soil erosion, the combined application of geospatial technologies such as geographical information systems (GIS), remote sensing, and RUSLE techniques are quite economical, time-saving, and more precise than most other models (Wang et al., 2003; Prasannakumar et al., 2011; Galdino et al., 2016; Gelagay and Minale, 2016; Zerihun et al., 2018; Adongo et al., 2019; Kumar et al., 2020; Kumar and Hole, 2021; Sandeep et al., 2021). Integrating soil erosion models with GIS acts as a potential tool in land degradation assessment practices. RUSLE is one of the soil erosion models applied for calculating and predicting the spatial and temporal differences in soil erosion in a GIS environment (Islam et al., 2020). It is used to estimate long-term annual average soil loss in certain areas with specific ground slope characters (Boggs et al., 2001). It is found to be a simple and pertinent model with limited data requirements and is widely used all over the globe because of its suitability and easy computational approaches (Jha and Paudel, 2010). Further, it is mostly an applied model for hilly terrains (Ganasri and Ramesh, 2016; Dissanayake et al., 2019) to assess erosion risk with potential application capabilities (Boggs et al., 2001). The primary objectives of the present study are to: (1) evaluate the long-term LULC changes of a Himalayan watershed; (2) assess the soil erosion due to LULC changes in the watershed using remote sensing, GIS, and RUSLE techniques; and (3) understand the potential risks of soil erosion caused by changing land-use patterns. The study will help to suggest suitable measures required for the conservation, planning, and sustainable management of soil resources in the study area and watersheds of similar settings.
The Dehar river is a tributary of the Beas River system. The Dehar watershed is located between the boundaries of the Kangra and Chamba districts of the state of Himachal Pradesh between latitudes 32°5′21′′ N to 32°23′47′′ N and longitudes 76°0′25′′’ E to 76°12′29′′ E. In the course of its journey, the Dehar river traverses several places, such as Trilokpur, Kotla, Kuther, Sihunta, Thulel, and Jawali, before joining the Pong Reservoir also known as Maharana Pratap Sagar (Figure 1). The Dehar watershed is covered by the Survey of India (SOI) top-sheet maps nos. 52D/3, 52D/4, and 52D/7 with 1:50000 scales. It covers an area of 450 km2 and with a minimum and maximum elevation of 395 and 4080 m above mean sea level, respectively. Geographically, the area is located in Himachal Himalaya and consists of almost parallel hills disconnected by longitudinal valleys. The area experiences a varied climate, sub-tropical at lower elevations and temperate at higher altitudes. The area experiences a maximum temperature of 38°C and a minimum temperature of 0°C with an average temperature of 22°C. It has a normal annual rainfall of 2500 mm. The dominant soil varieties that cover the watershed are sandy-skeletal, coarse-loamy, fine-loamy and mesic-loamy soils (Sidhu et al., 1997). Geologically, the watershed is covered by rocks of the Lower Himalaya in the North and Siwaliks (Outer Himalaya) in the South (Srikantia and Bhargava, 1998). From north to south, it is covered by various geological formations, such as Dhauladhar Granites, Mandi Darla Volcanics, Shali Formation, Dharamshala Formation, Siwalik Group, and fluvio-glacial deposits. The Lesser Himalayan zone is represented by major rock types: Precambrian gneissic and granitic rocks (the Dhauladhar Group); slate, schist, phyllite, and limestone (Salooni Formation); older rocks include slate, quartzite, schist, basic lava flows, marl, salt, and dolomites (Sundernagar, Jutogh, and Shali Formations). The lesser Himalaya is represented by the Subathu Group which includes rocks of Cenozoic age (green shales and fossiliferous limestones), and the Siwalik Group, with sedimentary rocks consisting of shale, conglomerate, clay, and sandstone (Srikantia and Bhargava, 1998). The area is tectonically active and traversed by several northeast-southwest trending major tectonic faults/lineaments, the Chail Thrust, the MBT, the Drini Thrust, and the Jwalamukhi Thrust, which separate different geological units by bordering them (Kumar and Nanda, 1989; Srikantia and Bhargava, 1998; Kumar and Mahajan, 2001).
Figure 1. Location maps. (A) Map of India showing the location of the Himachal Pradesh state (shaded by yellow color). (B) Map of the Himachal Pradesh state showing area (shaded by yellow color) covered by the Dehar watershed. (C) Enlarged map showing the Dehar watershed.
Shuttle Radar Topographic Mission (SRTM) digital elevation model data of 30 m resolution was used in the extraction of basin boundaries and for determining topographic factors (LS factors) for RUSLE modeling using ArcGIS 10.8 software (Table 1). Time series data of LANDSAT-7, LANDSAT-5, and SENTINAL-2 for the years 1999, 2010, and 2020, respectively, were used for preparing LULC maps and determining crop management (C) and conservation practice (P) factors used in RUSLE modeling (Table 1). The soil maps required to determine soil classes and soil erodibility (K factor) for the study were obtained from the database of NBSS and LUP (2005). Rainfall data procured from the India Meteorological Department (IMD) of 11 nearby rain gauge stations for the duration of 1999–2020 was used in determining the rainfall erosivity (R) factor (Table 1). All factors used in RUSLE modeling for determining soil loss were derived independently.
To estimate soil erosion, various parameters such as precipitation, soil categories, terrain characters, and land cover changes are required (Kouli et al., 2009; Vijith et al., 2018). The annual average soil loss per unit area per year was estimated as per the following equation (Eq. 1) given by Renard et al. (1997) using the required parameters generated through DEM, rainfall data, soil types, and satellite images (Figure 2):
Where A denotes the average annual soil loss (t/ha/year), R represents the rainfall erosivity factor (MJ mm/ha/hr/year), K refers to the soil erodibility factor (Mg h/MJ/mm), LS indicates the topological factor expressed as slope length and steepness factor, C is crop management, and P signifies the conservation support practice factor (Figure 2). Of all these factors, LS, C, and P factors are dimensionless.
To determine the LULC changes in the study area, the satellite imageries of LANDSAT-7, LANDSAT-5, and SENTINAL-2 for the years 1999, 2010, and 2020, respectively, were used. Image enhancement techniques were adopted to categorize the LULC features. All the downloaded images were in TIFF format and therefore exported to .img format via application of a layer stack function using ERDAS Imagine 2020. These stacked images were georeferenced into the same map projection of the World Geodetic System 1984 Zone 43N (WGS 1984-43N). All images were subset (sub-mapped) with reference to the boundaries of the watershed for marking the whole study area. Red, Green, Blue (RGB) band composition was used for all the images to interpret and classify the surface features with more precision (Teng et al., 2016). False color composite (FCC) of satellite images were generated for the years 1999, 2010, and 2020 by displaying different band combinations such as band 4 (NIR), band 3 (red), and band 2 (green). Various LULC classes were identified in the study area, specifically, Built-Up, Agricultural Land, Forest, Barren, and Water Bodies. With the aim to check the level of accuracy of the generated LULC layers, nearly 200 randomly collected sample points were selected from each of the created LULC layers. These layers were again opened and overlaid in Google Earth Pro software to validate the generated LULC layers for accuracy. Further, a confusion matrix was computed with the validated data.
R factor is the measure of the intensity of rainfall in a certain place based on the quantum of soil erosion (Koirala et al., 2019). Incessant rainfall data is required to compute the R factor, as it is valuable in knowing the influence of rainfall intensity on soil erosion triggered by water erosion (Wischmeier and Smith, 1978). From the literature, it was noticed that a multitude of equations have been developed to quantify the R factor (Kouli et al., 2009; Khosrokhani and Pradhan, 2014). Nevertheless, the formula (Eq. 2) given by Singh et al. (1981) was used for discerning the rainfall erosivity factor. In addition, the equation illustrates the relationship between the rainfall erosivity factor and the average annual precipitation, which has been found to be more reliable for soil erosion studies in India (Saha et al., 2018; Sandeep et al., 2021; Sangeetha and Ambujam, 2021). Monthly rainfall data was procured from the IMD for the 11 rain gauge stations located in and around the Dehar watershed for the duration of 1999 to 2020. Further, the mean annual rainfall data (for the duration of 1999 to 2020) of the rain gauge stations, which is essential for the computation of the R factor, was drawn from the collected rainfall data sets (Figure 3). The R factor was computed by inverse distance weighting (IDW) interpolation method and converted to a raster data set of 30 m cell grid size (Figure 9).
Figure 3. Maps of the Dehar watershed showing the average annual rainfall for the years 1999, 2010, and 2020.
Figure 8. Bar graph showing the change in different LULC classes for the years 1999, 2010, and 2020 of the Dehar watershed.
Where Rn denotes the average annual rainfall expressed in mm.
The soil erodibility factor (K) describes the characteristic eroding capacity of soils. It measures the susceptibility of soils to detach and be transported predominantly caused by rainfall and overland flow. The K factor refers to the impact of the physical and chemical properties of soils on erodibility during precipitation events in an elevated region (Wischmeier and Smith, 1978). The K factor is affected by various soil properties that comprise soil texture, structure, organic matter, permeability, porosity, and soil profile (Prasannakumar et al., 2011). For the present study, the soil map was procured from the NBSS and LUP (2005). The three different soil classes, sandy-skeletal, coarse-loamy, and fine-loamy soils, were recognized in the watershed (Figure 4; Sidhu et al., 1997). The K-values for these three soil classes were assigned considering the K-values proposed by Jain et al. (2010) (Table 2). The soil map was initially generated in vector format to decipher the soil classes considering K-values and later converted into raster format with a 30 m × 30 m grid (Figure 10).
Table 2. K-values for different soil types (after Jain et al., 2010).
The topographic factor (LS) is generated from the two significant aspects of slope-length factor (L) and slope-gradient factor (S), and these factors are primarily created from the Digital Elevation Method (DEM). Zhang et al. (2013) determined that the LS factor is an essential component of the RUSLE model soil erosion estimation as gravitational forces play a major role in overland flow. The LS factor revealed that the landscape setting of terrain with steep slopes and heavy precipitations is more vulnerable to soil erosion. Paul et al. (2021) viewed that if the length and steepness of an area are higher, the velocity and runoff volume escalates, leading to soil erosion. The DEM is considered to be the best option for the calculation of the LS factor in a particular area (Panagos et al., 2015). The LS factor was calculated from SRTM DEM of 30 m spatial (Figure 5). The LS factor map was generated by adopting equation (Eq. 3) (Wischmeier and Smith, 1978) and using a tool developed in Arc Macro Language by Hickey (2001). The tool takes DEM in ASCII format as input to calculate the LS factor (Figure 11).
Where, LS represents the slope length and steepness factor, l is cumulative slope length in meters, and β is the downhill slope angle.
The crop management factor (C factor), also known as the cover management factor, shows the influence of cropping and other allied practices on soil erosion (Chalise et al., 2019; Sandeep et al., 2021). It also expresses the response of vegetative cover to water erosion. Areas with unprotected vegetative cover are commonly prone to high water erosion. On the other hand, soil erosion is low in the areas where the land is protected by vegetative cover. Nearing et al. (2004) viewed the C factor as sensitive to spatiotemporal variations, as it depends on vegetative growth and rainfall conditions. The C factor is represented as the erosion-weighted ratio of soil loss from crop/cover land to the corresponding loss from bare fallow or unprotected soil cover (Wischmeier and Smith, 1978). This factor holds a dimensionless number and the values range between 0 and 1, where 0 signifies the area has vegetative cover and is erosion-protected, whereas 1 represents the area is bare, uncovered, and prone to soil erosion. Significantly, thick vegetation protects from soil erosion by reducing runoff intensity and raindrop influence on the soil-covered ground surface (Ranzi et al., 2012). Therefore, vegetative cover is known to be a crucial factor along with the topographic factor in controlling the rate of soil loss (Zhou et al., 2008; Li et al., 2019).
In this study, an LU/LC-related method was adopted for calculating C factor values of the area. The C factor values for the respective years were generated from Normalized Differential Vegetative Index (NDVI) derived from LANDSAT-07, LANDSAT-05, and SENTINAL-2 images for the years 1999, 2010, and 2020, respectively (Figure 6). For calculating the C factor, the equation (Eq. 4) proposed by van der Knijff et al. (2000) was adopted.
Where α and β are unitless parameters that fix the shape of the NDVI and the C factor curve. This equation has been widely used for Indian mountainous terrains (Kumar et al., 2014).
The conservation practice factor reveals the positive outcome of soil and water conservation processes of soil erosion in relation to agricultural practices. Wischmeier and Smith (1978) viewed the P factor as the percentage of erosion during the period of conservation and protective conditions compared to the usual circumstances of erosion occurrence.
The P factor values range between 0 and 1, where the values falling near to 0 signify the area is under healthy soil and water conservation practices, whereas the values close to 1 represent that the area lacks appropriate conservation practices (Table 3). In this study, the P factor map was developed by adopting the existing P factor values of the related land-cover classes collected from published sources (Wischmeier and Smith, 1978; Wanielista and Yousef, 1993; Londhe et al., 2010).
Table 3. P-values for different LULC classes (complied after Wischmeier and Smith, 1978; Wanielista and Yousef, 1993; Londhe et al., 2010).
It was inferred from the analysis of the LULC classes that Built-Up areas increased from 2.3 km2 in the year 1999 to 3.96 km2 in 2010 and 5.36 km2 in 2020. Agricultural Land increased from 48.16 km2 in the year 1999 to 61.09 km2 in 2010 and 64.28 km2 in 2020. Conversely, Barren Land decreased from 50.15 km2 in the year 1999 to 34.75 km2 in 2010 and 26.85 km2 in 2020. Forest Land increased from 342.1 km2 in 1999 to 343.2 km2 in 2010 and 346.2 km2 in 2020. However, in the case of Water Bodies, there was a decrease from 5.89 km2 in the year 1999 to 5.6 km2 in 2010, and an increase 5.91 km2 in 2020 (Table 4 and Figures 7, 8). The results of the area under very low, low, moderate, high, very high, and extremely severe erosion classes during the years 1999, 2010, and 2020 with respect to different LULC classes such as Built-Up area, Agriculture Land, Forest Land, and Barren Land are presented in Table 7. The confusion matrix resulted in an overall accuracy of 86.84% for 1999, 87.43% for 2010, and 88.05% for 2020 with a Kappa coefficient value of 0.827, 0.835, and 0.842 for the years 1999, 2010, and 2020, respectively (Table 5). It was inferred from the obtained results that the image classification was performed with accuracy.
The erosivity rate is directly related to the total amount of precipitation that occurred in a particular area (Sandeep et al., 2021). The R-values of the study area varied between 713 and 985 MJ mm/ha/hr/year in 2020, 663 to 845 MJ mm/ha/hr/year in 2010, and 672 to 874 MJ mm/ha/hr/year in 1999 (Figure 9). The watershed receives high rainfall from July to September during the southwest monsoon season. The eastern part of the watershed has a high R-value in comparison to the western part (Figure 9). Thus, this indicated that high R factor values influence the rate of soil erosion in the watershed.
The prominent soil types of the area are sandy-skeletal, coarse-loamy, and fine-loamy soils. Almost 57% of the total geographical area is covered by sandy-skeletal soil followed by coarse-loamy soil (32%), then fine-loamy soil (11%) (Figure 4). The soil erodibility (K factor) values of the study area vary from 0.020 to 0.042 (Table 2). The northern part of the watershed is covered by sandy-skeletal soil signifying the highest K factor value (Figure 10), while the central and southern parts of the watershed are covered by coarse- and fine-loamy soils with lower K factor values.
The elevation of the Dehar watershed ranges from 395 to 4080 m with a relief of 3,695 m. The LS factor was calculated using DEM and equation (Eq. 3), by considering the interface between flow accumulation and topographic features (Figure 5). The LS factor of the watershed ranged from 0 to 98 with a mean value of 5.38 (Figure 11).
The vegetative cover factor (C) was determined using the equation (Eq. 4) for the years 1999, 2010, and 2020 (Figure 12). The NDVI values for the years 1999, 2010, and 2020 ranged from−0.41 to 0.63, −0.38 to 0.67, and −0.19 to 0.55, respectively (Figure 6). The C factor values generated from the NDVI ranged from 0.03 to 1.78 for 1999, 0.01 to 1.74 for 2010, and 0.07 to 1.39 for 2020 (Figure 12). The derived C factor values revealed that higher values were found in barren land and lower values were reported in vegetative cover areas.
The P factor value of the study area varied from 0 to 1 (Figure 13 and Table 3). The lowest value (0) was assigned to a water body where conservation measures were undertaken, while a P factor value (1) was given to vegetation, barren land, built-up area, and agriculture land (less than 3% and more than 25% slope) where appropriate soil-water conservation strategies were not adopted. The different agricultural land use classes (with slopes varying from 3–8, 8–12, 12–16, 16–20, and 20–25%) were assigned P-values ranging from 0.5 to 0.9.
The different RUSLE parameters were multiplied in an ArcGIS 10.8 environment by applying a raster calculator tool to find the geoenvironmental conditions in the Dehar watershed by knowing the spatiotemporal changes and the rate of soil erosion during the years 1999, 2010, and 2020. The assessed rate of soil erosion was grouped into five classes: very low (0–1 t/ha/y), low (1–5 t/ha/y), moderate (5–10 t/ha/y), high (10–20 t/ha/y), very high (20–50 t/ha/y), and extreme (>50 t/ha/y), as shown in Table 6 (Dabral et al., 2008). The very low class (0–1 t/ha/yr) showed a higher area in percentage with respect to soil loss followed by the extremely severe class (>50 t/ha/yr) for all three years (1999, 2010, and 2020) of the LULC settings (Figure 14).
Table 6. Area under different soil loss potential classes and mean soil loss for the year 1999, 2010, and 2020.
Table 7. LULC class-wise area in percentage under different soil loss potential classes for the years 1999, 2010, and 2020.
Figure 14. Maps showing soil erosion trends of the Dehar watershed for the years 1999, 2010, and 2020.
In 1999, the percentages of areas that experienced very low, low, moderate, high, very high, and extremely severe erosion were 46.42, 0.45, 2.53, 5.05, 10.81, and 34.74%, respectively (Table 6). Whereas in 2010, the percentages of areas that experienced very low, low, moderate, high, very high, and extremely severe erosion were 46.52, 1.32, 3.31, 5.91, 11.69, and 31.25%, respectively. Similarly, in 2020, the areas prone to very low, low, moderate, high, very high, and extremely severe erosion were 46.1, 0.56, 2.71, 4.68, 10.03, and 35.92%, respectively. The results representing areas under very low, low, moderate, high, very high, and extremely severe erosion classes and mean soil loss within different land use classes are presented in Tables 6–8.
In the present study, the influence of LULC changes on soil loss dynamics in the Dehar watershed was estimated using the empirical model RUSLE in the GIS environment. RUSLE is widely applied in mountain terrains and very suitable for the Himalayan mountains system (Cevik and Topal, 2003; Dissanayake et al., 2019; Thapa, 2020; George et al., 2021; Kumar and Hole, 2021). It was inferred from the study that a subsequent rise in Built-Up and Agricultural Land was observed in the years 2010 and 2020 and, conversely, Barren Land decreased in the years 2010 and 2020 due to the rise in the population and expansion of habitats and agricultural fields in the lesser and Sub-Himalayan zone in recent years, leading to the conversion of Barren Land to Built-Up and Agricultural fields. The class Forest also showed a minor rise in the years 2010 and 2020 due to sustainable forest conservation and management practices (Table 4). In the Built-Up class of land use, the mean soil loss decreased from 32.19 in 1999 to 18.77 t/ha/yr in 2010, but in the year 2020 the mean soil loss slightly increased to 20.15 t/ha/yr (Table 8). Further, Barren Land registered a decrease in the mean soil loss from 86.43 t/ha/yr in the year 1999 to 74.60 t/ha/yr in 2010 and 73.19 t/ha/yr in the year 2020. Regarding the Agriculture class, the rate of mean soil loss slightly increased from 32.55 t/ha/yr in 1999 to 33.35 t/ha/yr in 2010, but then decreased to 25.43 t/ha/yr in 2020. The area covered under Forest experienced an increase in mean soil loss, from 65.30 t/ha/yr in 1999 to 65.87 in 2010 and 74.72 t/ha/yr in 2020. From the current study, it is inferred that the Barren Land and Forest classes experienced a higher rate of soil erosion in comparison to other classes because the Dehar watershed is prone to high precipitation and its presence in a high altitudinal area with hilly terrain, steep to very steep slopes, incidents of forest fires, and unsustainable forest management practices. In the Sub-Himalayas, the Barren and Forest areas experience a high rate of soil erosion due to their spread on high elevations and steep slopes (Kumar et al., 2014; Fayas et al., 2019; Uddin et al., 2019).
Rainfall erosivity (R factor) indicated a rise in the mean soil loss between the years 2010 and 2020. Further, the R factor clearly illustrated the importance of rainfall erosivity in soil loss estimation, as its values increased between the years 2010 and 2020. Interestingly, the area experienced a decrease in total mean soil loss between the years 1999 and 2010 and an increase in the total mean soil loss between the years 2010 and 2020 (Table 6).
This study evaluated the influence of LULC changes on soil erosion in a hilly watershed in the northern western Himalaya region in the state of Himachal Pradesh, India, for the years 1999, 2010, and 2020. The methodology adopted was to apply the RUSLE method for soil loss estimation in a GIS environment to create and compare soil loss estimation maps of 1999, 2010, and 2020. Moreover, the main aim was to identify soil loss changes that occurred due to LULC change. The RUSLE model in combination with the GIS technique was applied to estimate the rate of soil erosion in different LULC classes. The RUSLE model was successfully employed by giving due importance to different factors (R, K, LS, P, and C). The results yielded mean soil loss of 63.71, 60.99, and 66.71 t/ha/yr for the year 1999, 2010, and 2020, respectively, indicating that the LULC changes considerably influenced the rate of soil loss in the Dehar watershed. Overall, the present study clearly shows that soil erosion is a serious geoenvironmental issue and it needs the urgent attention of scientists, policymakers, and the general public for sustainable management of soil resources. Additionally, the data used in the present study was of coarser resolution and of a larger scale. There is wider scope for further studies at micro-watershed levels using high-resolution satellite imageries and more accurate rainfall data sets derived from different sources and validated with field-based models. More precise soil-loss estimations would help various stakeholders in taking suitable measures for the prevention of soil erosion.
The raw data supporting the conclusions of this article will be made available by the author, without undue reservation.
MP: investigation, visualization, supervision and formal analysis, writing – original draft, and reviewing and editing. AK: conceptualization, methodology, investigation, data collection and data generation, writing, and reviewing and editing. SD: conceptualization, methodology, investigation, writing, and reviewing and editing. OV, SR, and BK: writing and reviewing and editing. All authors contributed to the article and approved the submitted version.
The authors declare that the research was conducted in the absence of any commercial or financial relationships that could be construed as a potential conflict of interest.
All claims expressed in this article are solely those of the authors and do not necessarily represent those of their affiliated organizations, or those of the publisher, the editors and the reviewers. Any product that may be evaluated in this article, or claim that may be made by its manufacturer, is not guaranteed or endorsed by the publisher.
Abdulkareem, J. H., Pradhan, B., Sulaiman, W. N. A., and Jamil, N. R. (2019). Prediction of spatial soil loss impacted by long-term land-use/land-cover change in a tropical watershed. Geosci. Front. 10, 389–403. doi: 10.1016/j.gsf.2017.10.010
Adongo, T. A., Agyare, W. A., Abagale, F. K., and Buffour, N. K. (2019). Spatial soil loss estimation using an integrated GIS-based revised universal soil loss equation. Int. J. Sci. Technol. 11, 58–71. doi: 10.4314/ijest.v11i4.6
Alkharabsheh, M. M., Alexandridis, T. K., Bilas, G., Misopolinos, N., and Silleos, N. (2013). Impact of land cover change on soil erosion hazard in northern Jordan using remote sensing and GIS. Procedia Environ. Sci. 19, 912–921. doi: 10.1016/j.proenv.2013.06.101
Ang, R., and Oeurng, C. (2018). Simulating streamflow in an ungauged catchment of Tonlesap Lake basin in Cambodia using soil and water assessment tool (SWAT) model. Water Sci. 32, 89–101. doi: 10.1016/j.wsj.2017.12.002
Angima, S. D., Stott, D. E., O’neill, M. K., Ong, C. K., and Weesies, G. A. (2003). Soil erosion prediction using RUSLE for central Kenyan highland conditions. Agric. Ecosyst. Environ. 97, 295–308. doi: 10.1016/S0167-8809(03)00011-2
Ayele, K. F., Suryabhagavan, K. V., and Sathishkumar, B. (2014). Assessment of habitat changes in Holeta watershed, central Oromiya, Ethiopia. Int. J. Earth Sci. Eng. 7, 1370–1375.
Bhattacharyya, R., Ghosh, B. N., Mishra, P. K., Mandal, B., Rao, C. S., Sarkar, D., et al. (2015). Soil degradation in India: Challenges and potential solutions. Sustainability 7, 3528–3570. doi: 10.3390/su7043528
Boggs, G., Devonport, C., Evans, K., and Puig, P. (2001). GIS-based rapid assessment of erosion risk in a small catchment in the wet/dry tropics of Australia. Land Degrad. Dev. 12, 417–434. doi: 10.1002/ldr.457
Cevik, E., and Topal, T. (2003). GIS-based landslide susceptibility mapping for a problematic segment of the natural gas pipeline, Hendek (Turkey). Environ. Geol. 44, 949–962. doi: 10.1007/s00254-003-0838-6
Chalise, D., Kumar, L., and Kristiansen, P. (2019). Land degradation by soil erosion in Nepal: A review. Soil Syst. 3:12. doi: 10.3390/soilsystems3010012
Dabral, P. P., Baithuri, N., and Pandey, A. (2008). Soil erosion assessment in a hilly catchment of North Eastern India using USLE, GIS and remote sensing. Water Resour. Manag. 22, 1783–1798. doi: 10.1007/s11269-008-9253-9
Dissanayake, D. M. S. L. B., Morimoto, T., and Ranagalage, M. (2019). Accessing the soil erosion rate based on RUSLE model for sustainable land use management: A case study of the Kotmale watershed, Sri Lanka. Model. Earth Syst. Environ. 5, 291–306. doi: 10.1007/s40808-018-0534-x
Erol, A., Koşkan, Ö., and Başaran, M. A. (2015). Socioeconomic modifications of the universal soil loss equation. Solid Earth 6, 1025–1035. doi: 10.5194/se-6-1025-2015
Eswaran, H., Lal, R., and Reich, P. F. (2001). “Land degradation: An overview,” in Proceedings of the 2nd international conference on land degradation and desertification. Responses to land degradation, eds E. Bridges, I. Hannam, L. Oldeman, F. Penning de Vries, S. Scherr, and S. Sompatpanit (Thailand: Oxford Press), 20–35. doi: 10.1201/9780429187957-4
Fayas, C. M., Abeysingha, N. S., Nirmanee, K. G. S., and Samaratunga, M. D. (2019). A Soil loss estimation using rusle model to prioritize erosion control in KELANI river basin in Sri Lanka. Int. Soil Water Conserv. Res. 7, 130–137. doi: 10.1016/j.iswcr.2019.01.003
Galdino, S., Sano, E. E., Andrade, R. G., Grego, C. R., Nogueira, S. F., Bragantini, C., et al. (2016). Large-scale modeling of soil erosion with RUSLE for conservationist planning of degraded cultivated Brazilian pastures. Land Degrad. Dev. 27, 773–784. doi: 10.1002/ldr.2414
Ganasri, B., and Ramesh, H. (2016). Assessment of soil erosion by RUSLE model using remote sensing and GIS-A case study of Nethravathi Basin. Geosci. Front. 7, 953–961. doi: 10.1016/j.gsf.2015.10.007
Garcia-Ruiz, J. M., Beguería, S., Nadal-Romero, E., Gonzalez-Hidalgo, J. C., Lana-Renault, N., and Sanjuán, Y. (2015). A meta-analysis of soil erosion rates across the world. Geomorphology 239, 160–173. doi: 10.1016/j.geomorph.2015.03.008
Gelagay, H. S., and Minale, A. S. (2016). Soil loss estimation using GIS and remote sensing techniques: A case of Koga watershed, Northwestern Ethiopia. Int. Soil Water Conserv. Res. 4, 126–136. doi: 10.1016/j.iswcr.2016.01.002
George, K. J., Kumar, S., and Hole, R. M. (2021). Geospatial modelling of soil erosion and risk assessment in Indian Himalayan region—A study of Uttarakhand state. Environ. Adv. 4:100039.
Glaeser, E. L., and Kahn, M. E. (2004). “Sprawl and urban growth,” in Handbook of regional and urban economics, cities and geography, Vol. 4, eds J. V. Henderson and J. E. Thisse (Amsterdam: Elsevier), 2481–2527. doi: 10.1016/S1574-0080(04)80013-0
Hamilton, L. S. (1987). What are the impacts of Himalayan deforestation on the Ganges-Brahmaputra lowlands and delta? Assumptions and facts. Mt. Res. Dev. 7, 256–263. doi: 10.2307/3673202
Haregeweyn, N., Tsunekawa, A., Nyssen, J., Poesen, J., Tsubo, M., Meshesha, D., et al. (2015). Soil erosion and conservation in Ethiopia: A review. Prog. Phys. Geogr. 39, 750–774. doi: 10.1177/0309133315598725
Hickey, R. (2001). Slope angle and slope length solutions for GIS. Cartography 29, 1–8. doi: 10.1080/00690805.2000.9714334
Hrissanthou, V., Delimani, P., and Xeidakis, G. (2010). Estimate of sediment inflow into Vistonis Lake, Greece. Int. J. Sediment Res. 25, 161–174. doi: 10.1016/S1001-6279(10)60035-2
Hurni, H., Tato, K., and Zeleke, G. (2005). The implications of changes in population, land use, and land management for surface runoff in the upper Nile basin area of Ethiopia. Mt. Res. Dev. 25, 147–154. doi: 10.1659/0276-4741(2005)025[0147:TIOCIP]2.0.CO;2
Islam, M. R., Jaafar, W. Z. W., Hin, L. S., Osman, N., and Karim, M. R. (2020). Development of an erosion model for Langat River Basin, Malaysia, adapting GIS and RS in RUSLE. Appl. Water Sci. 10, 1–11. doi: 10.1007/s13201-020-01185-4
Jain, M. K., Mishra, S. K., and Shah, R. B. (2010). Estimation of sediment yield and areas vulnerable to soil erosion and deposition in a Himalayan watershed using GIS. Curr. Sci. 98, 213–221.
Jain, S. K., Kumar, S., and Varghese, J. (2001). Estimation of soil erosion for a Himalayan watershed using GIS technique. Water Resour. Manag. 15, 41–54. doi: 10.1023/A:1012246029263
Jayasekara, M. J. P. T. M., and Kadupitiya, H. K., and Vitharana, U. W. A. (2018). Mapping of soil erosion hazard zones of Sri Lanka. Trop. Agric. Res. 29, 135–146. doi: 10.4038/tar.v29i2.8284
Jha, M. K., and Paudel, R. C. (2010). Erosion predictions by empirical models in a mountainous watershed in Nepal. J. Spat. Hydrol. 10, 89–102.
Kaiser, J. (2004). Wounding earth’s fragile skin. Science 304, 1616–1618. doi: 10.1126/science.304.5677.1616
Keno, K., and Suryabhagavan, K. V. (2014). Multi-temporal remote sensing of landscape dynamics and pattern change in Dire district, Southern Ethiopia. J. Geomorphol. 8, 189–194. doi: 10.4172/2157-7617.1000226
Khosrokhani, M., and Pradhan, B. (2014). Spatio-temporal assessment of soil erosion at Kuala Lumpur metropolitan city using remote sensing data and GIS. Geomat. Nat. Hazards Risk 5, 252–270. doi: 10.1080/19475705.2013.794164
Koirala, P., Thakuri, S., Joshi, S., and Chauhan, R. (2019). Estimation of soil erosion in Nepal using a RUSLE modeling and geospatial tool. Geosciences 9:147. doi: 10.3390/geosciences9040147
Kolli, M. K., Opp, C., and Groll, M. (2021). Estimation of soil erosion and sediment yield concentration across the Kolleru Lake catchment using GIS. Environ. Earth Sci. 80:161. doi: 10.1007/s12665-021-09443-7
Kouli, M., Soupios, P., and Vallianatos, F. (2009). Soil erosion prediction using the revised universal soil loss equation (RUSLE) in a GIS framework, Chania, Northwestern Crete, Greece. Environ. Geol. 57, 483–497. doi: 10.1007/s00254-008-1318-9
Krishna Bahadur, K. C. (2009). Mapping soil erosion susceptibility using remote sensing and GIS: A case of the Upper Nam Wa Watershed, Nan Province, Thailand. Environ. Geol. 57, 695–705. doi: 10.1007/s00254-008-1348-3
Kumar, A., Devi, M., and Deshmukh, B. (2014). Integrated remote sensing and geographic information system based RUSLE modelling for estimation of soil loss in western Himalaya, India. Water Resour. Manag. 28, 3307–3317. doi: 10.1007/s11269-014-0680-5
Kumar, R., and Nanda, A. C. (1989). Sedimentology of the middle Siwalik subgroup of Mohand area, Dehra Dun valley, India. J. Geol. Soc. India 34, 597–616.
Kumar, S., and Hole, R. M. (2021). Geospatial modelling of soil erosion and risk assessment in Indian Himalayan region—A study of Uttarakhand state. Environ. Adv. 4:100039. doi: 10.1016/j.envadv.2021.100039
Kumar, S., and Mahajan, A. K. (2001). Seismotectonics of the Kangra region, northwest Himalaya. Tectonophysics 331, 359–371. doi: 10.1016/S0040-1951(00)00293-6
Kumar, T., Jhariya, D. C., and Pandey, H. K. (2020). Comparative study of different models for soil erosion and sediment yield in Pairi watershed, Chhattisgarh, India. Geocarto Int. 35, 1245–1266. doi: 10.1080/10106049.2019.1576779
Lambin, E. F., Turner, B. L., Geist, H. J., Agbola, S. B., Angelsen, A., Bruce, J. W., et al. (2010). Land degradation in dry lands: Interactions among hydrologic-aeolian erosion and vegetation dynamics. Geomorphology 116, 236–245. doi: 10.1016/j.geomorph.2009.11.023
Li, Y., Qi, S., Liang, B., Ma, J., Cheng, B., Ma, C., et al. (2019). Dangerous degree forecast of soil loss on highway slopes in mountainous areas of the Yunnan–Guizhou Plateau (China) using the Revised Universal Soil Loss Equation. Nat. Hazards Earth Syst. Sci. 19, 757–774. doi: 10.5194/nhess-19-757-2019
Londhe, S., Nathawat, M. S., and Subudhi, A. P. (2010). Erosion susceptibility zoning and prioritization of mini-watersheds using geomatics approach. Int. J. Geomat. Geosci. 1, 511–528.
Mahapatra, S. K., Reddy, G. O., Nagdev, R., Yadav, R. P., Singh, S. K., and Sharda, V. N. (2018). Assessment of soil erosion in the fragile Himalayan ecosystem of Uttarakhand, India using USLE and GIS for sustainable productivity. Curr. Sci. 115, 108–121. doi: 10.18520/cs/v115/i1/108-121
Mandal, D., and Sharda, V. N. (2011). Assessment of permissible soil loss in India employing a quantitative bio-physical model. Curr. Sci. 100, 383–390.
Mandal, D., and Sharda, V. N. (2013). Appraisal of soil erosion risk in the Eastern Himalayan region of India for soil conservation planning. Land Degrad. Dev. 24, 430–437.
Meshesha, D. T., Tsunekawa, A., Tsubo, M., and Haregeweyn, N. (2012). Dynamics and hotspots of soil erosion and management scenarios of the Central Rift Valley of Ethiopia. Int. J. Sediment Res. 27, 84–99. doi: 10.1016/S1001-6279(12)60018-3
Mohammadi, M., Khaledi Darvishan, A. K., Spalevic, V., Dudic, B., and Billi, P. (2021). Analysis of the impact of land use changes on soil erosion intensity and sediment yield using the intErO model in the Talar watershed of Iran. Water 13:881. doi: 10.3390/w13060881
Moisa, M. B., Babu, A., and Getahun, K. (2023). Integration of geospatial technologies with RUSLE model for analysis of soil erosion in response to land use/land cover dynamics. A case of Jere watershed, Western Ethiopia. Sustain. Water Resour. Manag. 9:13. doi: 10.1007/s40899-022-00805-y
Morgan, R. P. C., Quinton, J. N., Smith, R. E., Govers, G., Poesen, J. W. A., Auerswald, K., et al. (1998). The European soil erosion model (EUROSEM): A dynamic approach for predicting sediment transport from fields and small catchments. Earth Surf. Process. Landf. 23, 527–544. doi: 10.1002/(SICI)1096-9837(199806)23:6<527::AID-ESP868>3.0.CO;2-5
NBSS, and LUP (2005). Annual report 2005. Nagpur: National Bureau of Soil Survey and Land Use Planning.
Nearing, M. A., Foster, G. R., Lane, L. J., and Finkner, S. C. (1989). A process-based soil erosion model for USDA-water erosion prediction project technology. Trans. ASAE 32, 1587–1593. doi: 10.13031/2013.31195
Nearing, M. A., Pruski, F. F., and O’neal, M. R. (2004). Expected climate change impacts on soil erosion rates: A review. J. Soil Water Conserv. 59, 43–50.
Negash, D. A., Moisa, M. B., Merga, B. B., Sedeta, F., and Gemeda, D. O. (2021). Soil erosion risk assessment for prioritization of sub-watershed: The case of Chogo Watershed, Horo Guduru Wollega, Ethiopia. Environ. Earth Sci. 80:589. doi: 10.1007/s12665-021-09901-2
Oliveira, M. L., Saikia, B. K., da Boit, K., Pinto, D., Tutikian, B. F., and Silva, L. F. (2019). River dynamics and nanopaticles formation: A comprehensive study on the nanoparticle geochemistry of suspended sediments in the Magdalena River, Caribbean Industrial Area. J. Clean. Prod. 213, 819–824. doi: 10.1016/j.jclepro.2018.12.230
Pan, J., and Wen, Y. (2014). Estimation of soil erosion using RUSLE in Caijiamiao watershed, China. Nat. Hazards 71, 2187–2205. doi: 10.1007/s11069-013-1006-2
Panagos, P., Borrelli, P., and Meusburger, K. (2015). A new European slope length and steepness factor (LS-Factor) for modeling soil erosion by water. Geosciences 5, 117–126. doi: 10.3390/geosciences5020117
Panagos, P., Borrelli, P., Meusburger, K., Yu, B., Klik, A., Jae, L. K., et al. (2017). Global rainfall erosivity assessment based on high-temporal resolution rainfall records. Sci. Rep. 7:4175. doi: 10.1038/s41598-017-04282-8
Panditharathne, D. L. D., Abeysingha, N. S., Nirmanee, K. G. S., and Mallawatantri, A. (2019). Application of revised universal soil loss equation (RUSLE) model to assess soil erosion in “Kalu Ganga” River Basin in Sri Lanka. Appl. Environ. Soil Sci. 2019:4037379. doi: 10.1155/2019/4037379
Paul, S. S., Li, J., Li, Y., and Shen, L. (2021). Assessing land use–land cover change and soil erosion potential using a combined approach through remote sensing, RUSLE and random forest algorithm. Geocarta Int. 36, 361–375. doi: 10.1080/10106049.2019.1614099
Pimentel, D., Allen, J., Beers, A., Guinand, L., Hawkins, A., Linder, R., et al. (1993). “Soil erosion and agricultural productivity,” in World soil erosion and conservation, ed. D. Pimentel (Cambridge: Cambridge University Press), 277–292. doi: 10.1017/CBO9780511735394.014
Pimentel, D., and Burgess, M. (2013). Soil erosion threatens food production. Agriculture 3, 443–463. doi: 10.3390/agriculture3030443
Poesen, J. (2018). Soil erosion in the Anthropocene: Research needs. Earth Surf. Process. Landf. 43, 64–84. doi: 10.1002/esp.4250
Prasannakumar, V., Shiny, R., Geetha, N., and Vijith, H. J. E. E. S. (2011). Spatial prediction of soil erosion risk by remote sensing, GIS and RUSLE approach: A case study of Siruvani river watershed in Attapady valley, Kerala, India. Environ. Earth Sci. 64, 965–972. doi: 10.1007/s12665-011-0913-3
Prasannakumar, V., Vijith, H., Abinod, S., and Geetha, N. J. G. F. (2012). Estimation of soil erosion risk within a small mountainous sub-watershed in Kerala, India, using Revised Universal Soil Loss Equation (RUSLE) and geo-information technology. Geosci. Front. 3, 209–215. doi: 10.1016/j.gsf.2011.11.003
Prashanth, M., Kumar, A., Dhar, S., Verma, O., and Gogoi, K. (2022). Hypsometric analysis for determining erosion proneness of Dehar watershed, Himachal Himalaya, North India. J. Geosci. Res. 7, 86–94.
Prashanth, M., Kumar, A., Dhar, S., Verma, O., and Sharma, S. (2021). Morphometric characterization and prioritization of sub-watersheds for assessing soil erosion susceptibility in the Dehar watershed (Himachal Himalaya), Northern India. Him. Geol. 42, 345–358.
Ranzi, R., Le, T. H., and Rulli, M. C. (2012). A RUSLE approach to model suspended sediment load in the Lo River (Vietnam): Effects of reservoirs and land use changes. J. Hydrol. 422–423, 17–29. doi: 10.1016/j.jhydrol.2011.12.009
Ravi, S., Breshears, D. D., Huxman, T. E., and D’Odorico, P. (2010). Land degradation in dry lands: Interactions among hydrologic–aeolian erosion and vegetation dynamics. Geomorphology 116, 236–245.
Rawat, K. S., Mishra, A. K., and Bhattacharyya, R. (2016). Soil erosion risk assessment and spatial mapping using LANDSAT-7 ETM+, RUSLE, and GIS—a case study. Arab. J. Geosci. 9:288. doi: 10.1007/s12517-015-2157-0
Renard, K., Foster, G., Weesies, G., McCool, D., and Yoder, D. (1997). Predicting soil erosion by water: A guide to conservation planning with the Revised Universal Soil Loss Equation (RUSLE). Agric. Handb. 703, 65–100.
Saha, A., Ghosh, P., and Mitra, B. (2018). GIS based soil erosion estimation using Rusle model: A case study of upper Kangsabati watershed, West Bengal, India. J. Environ. Sci. Nat. Resour. 13, 119–126. doi: 10.19080/IJESNR.2018.13.555871
Samanta, S., Koloa, C., Pal, D. K., and Palsamanta, B. (2016). Estimation of potential soil erosion rate using RUSLE and E30 model. Model. Earth Syst. Environ. 2:149. doi: 10.1007/s40808-016-0206-7
Sandeep, P., Kumar, K. C., and Haritha, S. (2021). Risk modelling of soil erosion in semi-arid watershed of Tamil Nadu, India using RUSLE integrated with GIS and Remote Sensing. Environ. Earth Sci. 80:511. doi: 10.1007/s12665-021-09800-6
Sangeetha, R., and Ambujam, N. K. (2021). The over shadow of the human evolvement process in the dynamics of soil drift of an agricultural watershed in Nilgiri Hills, India. Soil Water Res. 16, 103–111. doi: 10.17221/105/2020-SWR
Senanayake, S., Pradhan, B., Huete, A., and Brennan, J. (2020). Assessing soil erosion hazards using land-use change and landslide frequency ratio method: A case study of Sabaragamuwa Province, Sri Lanka. Remote Sens. 12:1483. doi: 10.3390/rs12091483
Sharma, J. C. (2008). “Soil and water conservation in river catchments in Himalayan region,” in Land resources management in river valley and flood prone areas of Himalayan region, eds J. C. Sharma, I. P. Sharma, and J. N. Raina (Dehradun: Bishen Singh Mahendra Pal Singh), 113–123.
Shrestha, D. P. (1997). Assessment of soil erosion in the Nepalese Himalaya: A case study in Likhu Khola Valley, Middle Mountain Region. Land Husb. 2, 59–80.
Sidhu, G. S., Rana, K. P. C., Larsem, L., Sehgal, J., and Velayutham, M. (1997). Soils of Himachal Pradesh for optimizing land use, India, Bulletin 57(b). Nagpur: National Bureau of Soil Survey and Land Use Planning, 20–25.
Singh, G., Babu, R., and Chandra, S. (1981). Soil loss prediction research in India, Bulletin No. T-12/D9. Dehradun: Central Soil and Water Conservation Research and Training Institute.
Singh, G., and Panda, R. K. (2017). Grid-cell based assessment of soil erosion potential for identification of critical erosion prone areas using USLE, GIS and remote sensing: A case study in the Kapgari watershed, India. Int. Soil Water Conserv. Res. 5, 202–211. doi: 10.1016/j.iswcr.2017.05.006
Singh, O., and Singh, J. (2018). Soil erosion susceptibility assessment of the lower Himachal Himalayan Watershed. J. Geol. Soc. India 92, 157–165. doi: 10.1007/s12594-018-0975-x
Srikantia, S. V., and Bhargava, O. N. (1998). Geology of Himachal Pradesh. Bangalore: Geological Society of India.
Srinivasan, R., Singh, S. K., Nayak, D. C., Hegde, R., and Ramesh, M. (2019). Eurasian journal of soil science. Eurasian J. Soil Sci. 8, 321–328. doi: 10.18393/ejss.598120
Steffen, W., Stone, G. D., Svedin, U., Veldkamp, T. A., Vogel, C., and Xu, J. (2001). The causes of land-use and land-cover change: Moving beyond the myths. Glob. Environ. Change 11, 261–269. doi: 10.1016/S0959-3780(01)00007-3
Steinmetz, A. A., Cassalho, F., Caldeira, T. L., Oliveira, V. A. D., Beskow, S., and Timm, L. C. (2018). Assessment of soil loss vulnerability in data-scarce watersheds in southern Brazil. Ciênc. Agrotec. 42, 575–587. doi: 10.1590/1413-70542018426022818
Stocking, M. (1993). Soil erosion in developing countries: Where geomorphology fears to tread. Norwich: School of Development Studies University of East Anglia, 241.
Stoddart, D. R. (1969). “World erosion and sedimentation in water,” in Water, earth and man, ed. R. J. Chorley (London: Methuen), 43–64. doi: 10.4324/9781003170181-5
Tang, Q., Xu, Y., Bennett, S. J., and Li, Y. (2015). Assessment of soil erosion using RUSLE and GIS: A case study of the Yangou watershed in the Loess Plateau, China. Environ. Earth Sci. 73, 1715–1724. doi: 10.1007/s12665-014-3523-z
Teng, H., Rossel, R. A. V., Shi, Z., Behrens, T., Chappell, A., and Bui, E. (2016). Assimilating satellite imagery and visible–near infrared spectroscopy to model and map soil loss by water erosion in Australia. Environ. Model. Softw. 77, 156–167. doi: 10.1016/j.envsoft.2015.11.024
Thapa, P. (2020). Spatial estimation of soil erosion using RUSLE modeling: A case study of Dolakha district, Nepal. Environ. Syst. Res. 9:15. doi: 10.1186/s40068-020-00177-2
Uddin, K., Matin, M. A., and Maharjan, S. (2019). Assessment of land cover change and its impact on changes in soil erosion risk in Nepal. Sustainability 10:4715. doi: 10.3390/su10124715
Uddin, K., Murthy, M. S. R., Wahid, S. M., and Matin, M. A. (2016). Estimation of soil erosion dynamics in the Koshi basin using GIS and remote sensing to assess priority areas for conservation. PLoS One 11:e0150494. doi: 10.1371/journal.pone.0150494
van der Knijff, J. M., Jones, R. J. A., and Montanarella, L. (2000). Soil erosion risk assessment in Europe, EUR 19044 EN. Luxembourg: Office for official publications of the European Communities, 1–34.
Vijith, H., Seling, L. W., and Dodge-Wan, D. (2018). Estimation of soil loss and identification of erosion risk zones in a forested region in Sarawak, Malaysia, Northern Borneo. Environ. Dev. Sustain. 20, 1365–1384. doi: 10.1007/s10668-017-9946-4
Viney, N. R., and Sivapalan, M. (1999). A conceptual model of sediment transport: Application to the Avon River Basin in Western Australia. Hydrol. Process. 13, 727–743. doi: 10.1002/(SICI)1099-1085(19990415)13:5<727::AID-HYP776>3.0.CO;2-D
Waltner, I., Saeidi, S., Grósz, J., Centeri, C., Laborczi, A., and Pásztor, L. (2020). Spatial assessment of the effects of Land cover change on soil erosion in Hungary from 1990 to 2018. ISPRS Int. J. Geo Inf. 9:667. doi: 10.3390/ijgi9110667
Wang, G., Gertner, G., Fang, S., and Anderson, A. B. (2003). Mapping multiple variables for predicting soil loss by geostatistical methods with TM images and a slope map. Photogramm. Eng. Remote Sens. 69, 889–898. doi: 10.14358/PERS.69.8.889
Wang, L., Huang, J., Du, Y., Hu, Y., and Han, P. (2013). Dynamic assessment of soil erosion risk using Landsat TM and HJ satellite data in Danjiangkou Reservoir area, China. Remote Sens. 5, 3826–3848. doi: 10.3390/rs5083826
Wanielista, M. P., and Yousef, Y. A. (1993). Stormwater management. New York, NY: John Wiley & Sons, 399–410.
Wischmeier, W. H., and Smith, D. D. (1978). Predicting rainfall erosion losses: A guide to conservation planning (No. 537). Department of agriculture, science and education administration, agriculture handbook. Washington, DC: U.S. Department of Agriculture.
Xu, L., Xu, X., and Meng, X. (2013). Risk assessment of soil erosion in different rainfall scenarios by RUSLE model coupled with information diffusion model: A case study of Bohai Rim, China. Catena 100, 74–82. doi: 10.1016/j.catena.2012.08.012
Yadav, R. P., and Sidhu, G. S. (2010). Assessment of soil erosion in Himachal Pradesh. J. Indian Soc. Soil Sci. 58, 212–220.
Yang, X., Gao, W., Shi, Q., Chen, F., and Chu, Q. (2013). Impact of climate change on the water requirement of summer maize in the Huang-Huai-Hai farming region. Agric. Water Manag. 124, 20–27. doi: 10.1016/j.agwat.2013.03.017
Zerihun, M., Mohammedyasin, M. S., Sewnet, D., Adem, A. A., and Lakew, M. (2018). Assessment of soil erosion using RUSLE, GIS and remote sensing in NW Ethiopia. Geoderma Reg. 12, 83–90. doi: 10.1016/j.geodrs.2018.01.002
Zhang, H., Yang, Q., Li, R., Liu, Q., Moore, D., He, P., et al. (2013). Extension of a GIS procedure for calculating the RUSLE equation LS factor. Comput. Geosci. 52, 177–188. doi: 10.1016/j.cageo.2012.09.027
Zhou, P., Luukkanen, O., Tokola, T., and Nieminen, J. (2008). Effect of vegetation cover on soil erosion in a mountainous watershed. Catena 75, 319–325. doi: 10.1016/j.catena.2008.07.010
Keywords: soil erosion, land use/land cover, GIS, RUSLE, Himachal Himalaya, North India
Citation: Prashanth M, Kumar A, Dhar S, Verma O, Rai SK and Kouser B (2023) Land use/land cover change and its implication on soil erosion in an ecologically sensitive Himachal Himalayan watershed, Northern India. Front. For. Glob. Change 6:1124677. doi: 10.3389/ffgc.2023.1124677
Received: 15 December 2022; Accepted: 09 January 2023;
Published: 06 February 2023.
Edited by:
Shreekar Pant, Baba Ghulam Shah Badshah University, IndiaReviewed by:
Madhusudhana Reddy, Dr. B.R. Ambedkar Open University, IndiaCopyright © 2023 Prashanth, Kumar, Dhar, Verma, Rai and Kouser. This is an open-access article distributed under the terms of the Creative Commons Attribution License (CC BY). The use, distribution or reproduction in other forums is permitted, provided the original author(s) and the copyright owner(s) are credited and that the original publication in this journal is cited, in accordance with accepted academic practice. No use, distribution or reproduction is permitted which does not comply with these terms.
*Correspondence: Manthena Prashanth, bXByYXNoYW50aEBpZ25vdS5hYy5pbg==; Arun Kumar,
YXJ1bmRoYXdhbjkyQHlhaG9vLmNvbQ==
Disclaimer: All claims expressed in this article are solely those of the authors and do not necessarily represent those of their affiliated organizations, or those of the publisher, the editors and the reviewers. Any product that may be evaluated in this article or claim that may be made by its manufacturer is not guaranteed or endorsed by the publisher.
Research integrity at Frontiers
Learn more about the work of our research integrity team to safeguard the quality of each article we publish.