- 1National Institute for Space Research (INPE), São José dos Campos, Brazil
- 2Geoprocessing Laboratory Applied to the Environment, Federal University of Acre (UFAC), Cruzeiro do Sul, Brazil
- 3ECOS Consult, Santa Cruz de la Sierra, Bolivia
- 4University of Exeter, Exeter, United Kingdom
- 5General Coordination for Research and Development, National Center for Monitoring and Early Warning of Natural Disasters, São José dos Campos, Brazil
Fires are among the main drivers of forest degradation in Amazonia, causing multiple socioeconomic and environmental damages. Although human-ignited sources account for most of the fire events in Amazonia, extended droughts may magnify their occurrence and propagation. The southwestern Amazonia, a transnational region shared by Brazil, Peru, and Bolivia and known as the MAP region, has been articulating coordinated actions to prevent disasters, including fire, to reduce their negative impacts. Therefore, to understand the fire patterns in the MAP region, we investigated their main drivers and the changes in the suitability of fire occurrence for the years 2005, 2010, 2016, and 2020. We used a maximum entropy (MaxEnt) model approach based on active fire data from satellites, climatic data, and land use and land cover mapping to spatially quantify the suitability of fire occurrence and its drivers. We used the year 2015 to calibrate the models. For climatic data and active fire count, we only considered grid cells with active fire count over the third quartile. All our models had a satisfactory performance, with values of the area under the curve (AUC) above 0.75 and p < 0.05. Additionally, all models showed sensitivity rates higher than 0.8 and false positive rates below 0.25. We estimated that, on average, 38.5% of the study region had suitable conditions for fire occurrence during the study period. Most of the fire-prone areas belong to Acre, representing approximately 74% of the entire MAP region. The percentage of deforested areas, productive lands, forest edges, and high temperatures were the main drivers of fire occurrence in southwestern Amazonia, indicating the high vulnerability of fragmented landscapes extreme climatic conditions to fire occurrence. We observed that the modeling approach based on Maxint is useful for useful for evaluating the implications of climatic and anthropogenic variables on fire distribution. Furthermore, because the model can be easily employed to predict suitable and non-suitable locations for fire occurrence, it can to prevent potential impacts associated with large-scale wildfire in the future at regional levels.
1. Introduction
Fires are an increasing cause of forest degradation in Amazonia, representing great socioeconomic and environmental damage (Faria et al., 2017). Land use change, such as deforestation for the expansion of agricultural activities and urban development, is a key driver of fire dynamics in tropical forests (Knorr et al., 2016). In addition, climate change, which can exacerbate the incidence of extreme droughts, may provide suitable conditions for magnifying fire occurrence and its propagation (Krawchuk and Moritz, 2011; Moritz et al., 2012).
Over the past 40 years, deforestation has been responsible for increasing forest fires in Amazonia, which can contribute up to 25% of total gross carbon emissions related to land use changes (Aragão et al., 2014). It has been quantified that forest fires, edge effects, and timber extraction degraded over 36 × 104 km2 of Amazonian forests between 2001 and 2018, an area that was 12% greater than the total area deforested in that period. By including extreme droughts, the degraded forest area increases from 5.5 to 38% of the remaining forest (Lapola et al., 2023). Tropical forests are an important carbon sink that assimilate large amounts of carbon through the photosynthesis process, building a stable carbon pool in their biomass (Carvalho et al., 2010). However, fire-affected forests in Amazonia can have, on average, 24.8 ± 6.9% less carbon stocks than undisturbed forests. This is because of the imbalance caused by increased tree mortality induced by fire, which generates carbon emissions that overcome forest carbon sequestration (Anderson et al., 2015; Silva et al., 2018, 2020). Moreover, fires directly impact biodiversity and ecosystem services provided by tropical forests, such as climate regulation (Flannigan et al., 2009). Finally, fires strongly impact the regional economy and the health of the population, contributing to the increased incidence of respiratory diseases (Carmo et al., 2013; Aragão et al., 2016a; Campanharo et al., 2019, 2021; Rocha and Sant'anna, 2022).
The probability of fire occurrence relates to factors that lead to the ignition, propagation, and extinction of fires (Chuvieco et al., 2014). Despite the need for fuel material (e.g., biomass) to generate fire, in high biomass regions, the influence of climate is notorious. For instance, a drier climate and low moisture content in soil and vegetation provide suitable conditions for the burning and spreading of fire over large areas of forest (Moritz et al., 2005; Krawchuk and Moritz, 2011). Thus, directional changes in climate (e.g., warmer and drier) act as driving forces to increase the probability of a fire occurring in different areas of the planet (Krawchuk et al., 2009; Moritz et al., 2012; Faria et al., 2017). Several studies have shown that extreme climate events have occurred in Amazonia, including the droughts of 2005, 2010, and 2015/2016. These droughts were strongly influenced by the anomalous warming of the Atlantic and Pacific oceans. In 2005, the positive mode of the Atlantic multidecadal oscillation (AMO) was a key determinant of the drought. In 2010 and 2015/16, the droughts were related to the occurrence of an El Niño, measured by the Multivariate ENSOUL Index (MED) combined with a positive AMS (Marengo et al., 2008; Zeng et al., 2008; Lewis et al., 2011; Aragão et al., 2018). During these extreme droughts, the number of recorded fires was higher than that in regular years, affecting large areas in Amazona (Brown et al., 2006; Lewis et al., 2011; Aragão et al., 2018; Silva et al., 2018). These events highlight the potential of climate to maximize the threat of fire on forest dynamics and the well-being of the population (Silva et al., 2018). Therefore, the increase in fires already evidenced during drought years and the probability of a drier future climate may put the efficacy of forest conservation and restoration programs planned in international agreements and policy initiatives for the coming years at risk, such as Reducing Emissions from Deforestation and forest Degradation (REDD+) and Sendai Framework for Disaster Risk Reduction 2015–2030 (UNDRR, 2015).
There are large uncertainties yet to be understood about how land use and land cover changes combined with long-term climate change affect fire dynamics in different political contexts, especially when fires are related to transboundary negative impacts that pose more challenges for Amazonia's fire governance and management (Pismel et al., 2023). This is particularly true for southwestern Amazonia, a region known as MAP, spanning from Madre de Dios (Peru), Acre (Brazil), and Pando (Bolivia). During regular climatic years, fire-related economic losses in Acre reached approximately 0.51% of Acre's GDP and can be as high as 7% during anomalous drought years (Campanharo et al., 2019). In 2005, the extreme dry season that affected the western Amazonian region maximized the effect of human-ignited fire over more than 300,000 hectares of standing forests. Nevertheless, it is worth noting that the effect was notably greater in the Brazilian portion, which experienced over US$50 million of direct economic losses and had more than 250,000 hectares of old-growth forests affected by fire (Brown et al., 2006). In subsequent years, the MAP region was exposed to increased deforestation rates and the emergence of a considerable increase in the burned area, particularly during the prolonged droughts of 2015/2016 (Silveira et al., 2022). Lastly, during the dry season of 2019 and 2020, an extensive fire crisis occurred, related to the sustained increase of deforestation rates, but no clear evidence of extreme droughts similar to those in 2010 and 2015/2016 were reported across Amazonia (Silveira et al., 2020). Despite a range of information on fire impact in the MAP region, further investigation is required for quantifying fire conditioning factors in the MAP region to support fire management.
Fire prediction in Amazonia based on the maximum entropy method has already been performed in different contexts by Fonseca et al. (2016, 2019); however, understanding the complex effect of landscape fragmentation combined with climatic drivers, such as water deficit, is still lacking. In a transnational context, present at the MAP region, modeling fire dynamics becomes even more challenging because, despite the environmental similarities, clear distinct political and socioeconomic vulnerabilities exist and are likely to affect fire patterns. Promoting the prediction of fire behavior and coping with the complexity of the MAP region, becomes, hence, an important governance strategy since the damage to natural and human systems is not limited to administrative and political boundaries. Moreover, transnational efforts from institutions in Brazil, Bolivia, and Peru are underway, aiming to coordinate and implement actions to prevent and mitigate fire damages (Pismel et al., 2023). Understanding the contribution of land use and extreme climate events and the effect these factors have on the risk of fire occurrence are, therefore, critical for socioeconomic and environmental planning in the MAP region.
In this study, our main goal was to investigate spatially-explicit changes in fire suitability across the MAP region for 4 years with different intensities and extent of the dry season during this century, including the years 2005, 2010, 2016, and 2020. To achieve our main goal, we aimed to predict the spatial distribution of the suitability of fire occurrence across the MAP region to answer two specific questions: (i) What is the contribution of the environmental and human forces driving the spatial distribution of fire suitability? and (ii) Has the threat of fire increased in recent years?
2. Materials and methods
2.1. Study area
The study area encompasses the transnational frontier between Madre de Dios (Peru), Acre (Brazil) and Pando (Bolivia), known as the MAP region (Figure 1). Located in the southwest flank of the Amazonia, the region is composed of approximately 91% of primary forests and hosts substantial natural resources. This region is one of the most biodiverse ecosystems in the world (Myers et al., 2000). It is represented by tropical rainforest formations composed of dense and open tropical rainforests with flooded and non-flooded areas. Additionally, the region hosts at least 30 different ethnicities of indigenous people (Mendoza et al., 2007).
Fire occurrence has a marked intra-annual seasonality in the region. During normal meteorological years, the fire season occurs from July to September when rainfall is below 100 mm per month. However, during extreme dry years, fires can start in June and prolong until October (Aragão et al., 2008; Carvalho et al., 2021). Along with dry conditions, anomalously high temperatures above the long-term mean during the fire season maximize the risk of major fires (Anderson et al., 2018; Silva Junior et al., 2019).
Because the area is a cross-border region straddling the three countries, it is under the influence of different socioeconomic contexts and political interests. This characteristic makes the MAP region strategic for studies that focus on forest conservation and environmental change mitigation programs with transboundary implications, contemplating fire risk and assessment of its impacts. The MAP region economy is based on the extraction of wood, nuts, and livestock, mainly in the states/departments of Pando and Madre de Dios; therefore, the lack of alignment of country-specific public policies, combined with distinct political interests in this transnational region, may directly impact these activities, magnifying the advancement of deforestation and forest fire degradation (Souza et al., 2006; Marsik et al., 2011; Perz et al., 2012; Michaelsen et al., 2013). For instance, the annual total deforested area in the states/departments of Acre and Madre de Dios increased by 137 and 103%, respectively, in 2020 compared to 2012 (INPE-National Institute for Space Research, 2022a; RAISG-Amazon Network of Georeferenced Socio-Environmental Information, 2022). In the department of Pando, the annual increment of deforestation rate in 2020 was the same as that in 2012, representing more than 45,000 km2 of the newly deforested areas in 2020 alone (RAISG-Amazon Network of Georeferenced Socio-Environmental Information, 2022).
2.2. Data preparation
We used different types of input data to represent fire events and their driving forces, including active fire data, land use and land cover (LULC), and climate data. All input data are listed and described in Table 1.
We used daily active fire data from September 2015 to indicate the presence of fire activity for each grid cell in the region. For this study, we combined active fire data detected by all available satellite sensors from the Center for Weather Forecast and Climate Studies—CPTEC of the Brazilian National Institute for Space Research—INPE (available at http://www.inpe.br/queimadas) to obtain the most complete coverage of active fires since cloud cover is high in the region, and it may contribute considerably to fire occurrence underestimation. The satellites used in this study passed over the study area at different times of the day, and they had different revisit periods over the same area (Anderson et al., 2017), which improved the information on the persistence of the fire event in the landscape through time. In this sense, the use of several sensors with different revisit periods and spatial resolution guarantees a better characterization of fire events spatially and over time without introducing bias.
From LULC and climatic data, we calculated 10 different metrics to represent direct or indirect predictor variables of fire (Figure 2). These predictor variables were selected according to criteria described in the Section 2.3, and they were used to provide a wide range of indicators of suitable conditions for fire occurrence. The details are presented below.
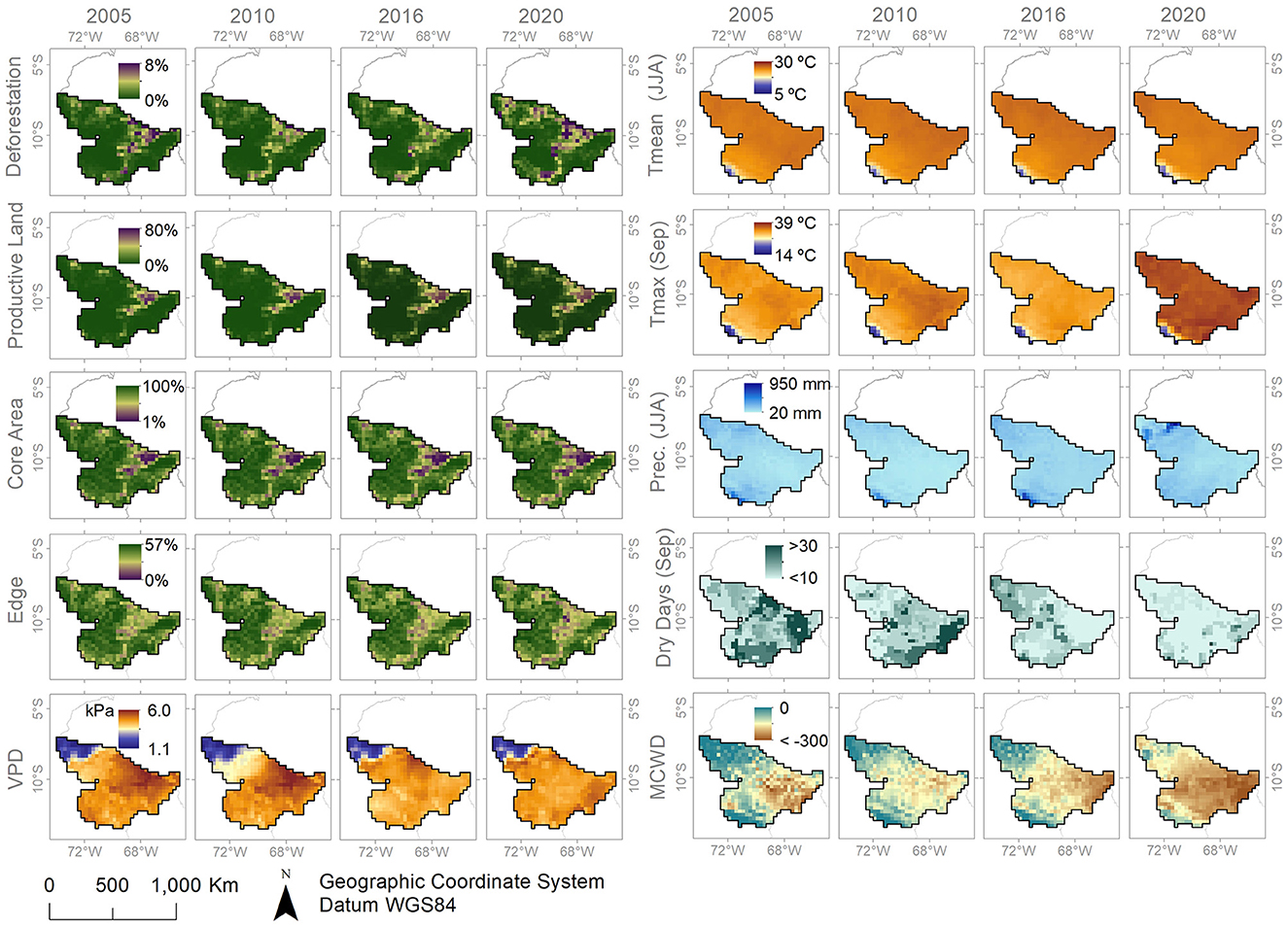
Figure 2. Spatial distribution of land use and climate predictor variables. “Productive Land”: percentage of productive areas, “Core”: core area, “Deforestation”: annual percentage of deforested area, “Edge”: forest edge area, “MCWD (Sep)”: MCWD index for the month of September, “Dry Days (Sep)”: number of consecutive days with precipitation below 1 mm of September, “Prec. (JJA)”: total precipitation of June, July, and August, “Tmax (Sep)”: maximum temperature of September, “Tmed (JJA)”: average temperature of June, July, and August, “VPD (Sep)”: vapor pressure deficit of September.
The LULC data were used as a measure of human-induced drivers, and they were collected from MapBiomas Amazonia Project Collection 3.0 (available at https://amazonia.mapbiomas.org/). As LULC-related variables, we included (i) the annual percentage of deforestation, calculated by considering the yearly transition areas of forest or flooded forest to farming, urban infrastructure, other non-vegetated areas, mining, or grassland; (ii) the annual percentage of agricultural land, calculated by considering all productive LULC classes such as farming and a mosaic of agriculture and pasture, grassland, and mining; (iii) the annual percentage of the forested core area; and (iv) the annual percentage of forest edges, bothcalculated by using the Morphological Spatial Pattern Analysis (MSPA) tool implemented in the open-access software GUIDOS toolbox from the European Commission's Joint Research Center (Soille and Vogt, 2009). In this study, we considered the innermost area of a forest fragment as the core area, and the forest edges perimeter was defined as 300 m (Laurance et al., 2018).
Climatic-related metrics were used as a measure of water and temperature stress in forests. In this study, we included (v) the total precipitation of June, July, and August; (vi) the mean temperature of June, July, and August; (vii) the maximum temperature of September (peak of fire season), obtained from the European Center for Medium-Range Weather Forecasts—Copernicus Climate Change Service—ECMWF (available at https://cds.climate.copernicus.eu/); (viii) the number of days with precipitation of <1 mm, obtained from the Brazilian Center for Early Warning and Monitoring for Natural Disasters—CAMDEN (Rozante et al., 2010); (ix) the maximum cumulative water deficit (MAC) of September; and (x) the vapor pressure deficit (VPD) of September.
Variables that represent a period of extended droughts such as total precipitation of June, July, and August, the mean temperature of June, July, and August, and the number of days with precipitation of <1 mm are expected to better represent the condition of the vegetation in a water stress state, which can cause tree mortality (Phillips et al., 2009) and favors the propagation of fire due to the accumulation of dry fuel material (Silvério et al., 2022). Silva Junior et al. (2019) have demonstrated that extended drought periods cause an increase in fire occurrences. Also, Anderson et al. (2018) have shown that the vulnerability of forests to drought has increased.
The climate ECMWF products used in this study were obtained from ERA5 atmospheric reanalysis data of the global climate. Reanalysis combines data from climate models with observations from multiple satellite data sources around the globe to estimate a continuous and consistent grid of the surface temperature to a height of 2 m and monthly total precipitation (Hersbach et al., 2021). These products are available from 1979 onwards with a spatial resolution of 0.25°. Generally, using reanalysis data allows us to overcome the temporal and spatial non-representativeness of conventional meteorological stations or lack of data due to high cloud cover when only satellite data are used for tropical forest regions. In addition, temperature and precipitation data derived from ECMWF products have good agreement with local meteorological stations for the Amazonia region, diverging on average by approximately 68 mm and 1.1°C, respectively (Gatti et al., 2021).
The MCWD metric was calculated by following the method adopted by Aragão et al. (2007), which relates the amount of evapotranspiration and total precipitation to generate an indicator of water deficit in the vegetation. Here, we used the total monthly precipitation and assumed 100 mm as the monthly average evapotranspiration for Amazonian forests (Von Randow et al., 2004; Maeda et al., 2017; Silva Júnior et al., 2017). Also, we used VPD as an indicator of atmospheric humidity since it relates indirectly to the amount of water vapor in the environment given the temperature (Murray, 1967). To calculate VPD, we used daily air temperature and dew point data collected from the Copernicus Climate Change Service—ECMWF. Both MCWD and VPD have previously shown great influence on Amazonian conditions for fire occurrence (Silvestrini et al., 2011; Ribeiro et al., 2022; Silveira et al., 2022).
2.3. Data analysis and model calibration
To calibrate the fire suitability model, we chose September as the reference period of fire occurrence because it is the peak of the fire season in southwestern Amazonia, extending from July to October (Carvalho et al., 2021), and more than 50% of yearly fire occurrence takes place then (Supplementary Figure 1). The third quartile of the distribution of active fire counting per 0.25° grid cell was used as a threshold to indicate the presence of samples for fire occurrence across the region. The year 2015 was chosen for the model calibration based on previous analysis of precipitation and temperature anomalies carried out from 2003 to 2020 (Supplementary Figure 2). In September 2015, both precipitation and temperature anomalies were severe across all three regions (Madre de Dios, Acre, and Pando), unlike other analyzed years when such events were not concomitantly severe or occurred with greater intensity in just one of the regions. Thus, September 2015 better represented the greatest range of values across the entire MAP region to allow a more comprehensive characterization of the amplitude of conditions that could lead to fires in each of the regions.
Initially, we resampled all data to a 0.25° spatial resolution using a regular grid. For each grid cell, we calculated the mean value of the climatic variables and the proportion of area covered by each of the LULC categories. Next, we calculated Pearson's correlation coefficient to test the statistical strength of the linear association between the variables by combining two different approaches. First, the coefficients between all predictor variables and fires were calculated to select the most correlated. For this, we used a threshold higher than 0.8 from Pearson's linear coefficient (Parisien and Moritz, 2009). Then, by analysing only the independent variables, new coefficients were calculated to select the predictor variables to verify whether these variables were associated with each other. All variables that presented coefficients lesser than 0.8 were marked as lacking correlation between the variables and thus removed. The variables that were finally considered in this analysis are presented in Table 1.
To model the spatial fire suitability distribution, we used MaxEnt software version 3.4.1. The algorithm has been commonly used for species distribution and habitat modeling, and it is based on the probabilistic inference method to estimate the maximum entropy distribution from incomplete datasets (Phillips et al., 2006, 2017). The method has been successfully applied to estimate the occurrence of fires in the landscape (Parisien and Moritz, 2009; Fonseca et al., 2016) and the aboveground biomass distribution (Saatchi et al., 2011; Ferreira et al., 2023).
The calibrated models were produced by using 70% of the sample subset, and the bootstrap resampling technique with 100 replicates and 1,000 iterations, generating an average estimate of the probability values between 0 and 1. Subsequently, the calibrated model was projected to the four different drought years, 2005, 2010, 2016, and 2020, considering the human and environmental conditions, to generate one continuous surface of spatially explicit fire suitability distribution for each year. We chose these years because they presented the greatest extent of rainfall anomalies in Amazonia, according to Silveira et al. (2022), in the last two decades. Model validation was performed using the remaining 30% of the samples not used to calibrate the model.
Finally, the results were evaluated according to the area under the curve (AUC) adjustment index, which reflects the model's performance in correctly classifying an area compared to a random classification from background points (Phillips et al., 2006). In this study, models with an AUC >0.7 were considered to have a satisfactory performance (Phillips et al., 2006; Elith et al., 2011). Furthermore, we performed a visual assessment of active fire occurrence across-grid to compare the real events of fire and the potential areas for fire occurrence.
3. Results
In September of the years 2005, 2010, 2016, and 2020, a total of approximately 75,400 active fires were detected, of which 37.5% were detected in 2005 alone. Moreover, in September 2020, the monthly active fire counting was the highest since 2010, considering the studied period and highlighting that 2005, 2010, and 2016 were major drought years in Amazonia. On average, Acre alone had witnessed almost three-quarters (73.9%) of active fire detected throughout the years in the MAP region, followed by Pando with 15.5% and Madre de Dios with 10.7%. In 2020, although Acre still held the record for the highest number of active fires (72%), the configuration changed for Madre de Dios and Pando, representing 15 and 13% of total active fires, respectively.
The results showed that, between 148 to 163 grid cells, representing ca. 31 ± 1.17% of the MAP region, had 25 or more fire counts, defined based on the third quartile used for calibrating the model. The patterns found followed the historical geography of fire occurrence in this region (Perz et al., 2012; Baraloto et al., 2015). Fires were concentrated in the most anthropized regions, in the northwest, extending along the northern borders, reaching the northeast flank and central east portions of the study area. This pattern is clear by analysing the third quartile distribution map for fire occurrence and its corresponding model of fire-prone grid cells (Figure 3).
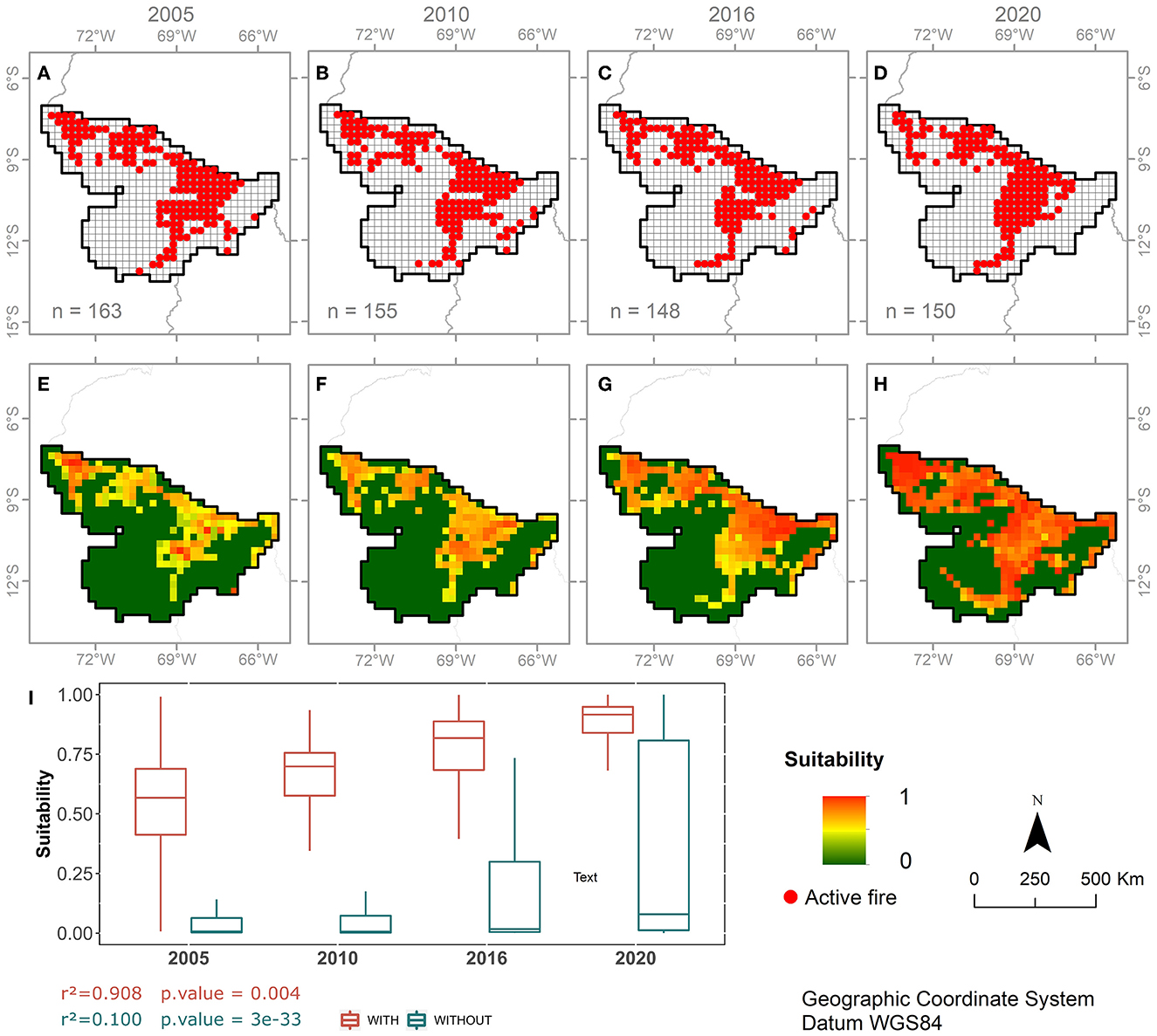
Figure 3. Distribution of grid cells for the month of September with 25 or more hot pixels (A–D), “n” represents the grid-cell count) for 2005, 2010, 2016, and 2020, and their corresponding model of risk of fire occurrence (E–H). The boxplot (I) shows the distribution of probability for fire occurrence in grid cells with and without the presence of 25 or more hot pixels, where the horizontal lines that split the box in two are the median value of each distribution, the lower and upper horizontal lines of the box are the 25th percentile and 75th percentile, and the vertical lines are the minimum and maximum range of the distribution. The probability values ranged from 0 to 1, with 1 representing the most fire-prone area and 0 the least fire-prone area.
The projected suitability areas for fire occurrence (Figures 3E–H) showed a satisfactory model performance (AUC > 0.7 and p <0.05) for all maximum entropy-calibrated models in the MAP region. The mean value of the AUC test and its standard deviation was 0.78 ± 0.02. All projected models showed consistency with the distribution of fire count in each studied year, except for 2020 when it predicted fires in a larger area compared to other years (Figure 3I). For all years, grid cells containing 25 or more fire counts consistently had median suitability values above 0.50, while grid cells with <25 fire counts had median suitability values below 0.10 (Figures 3A–D). This pattern clearly shows the separation between high-risk areas, associated with fire incidence larger than 25 fire counts, and the low-risk areas, associated with <25 fire counts, or no fire identified in that specific month. The analysis of the boxplot (Figure 3I) also showed the largest variation of suitability values in grid cells with <25 fire counts for the 2020 model. Fire-prone areas detected by the 2020 model had a lower number of fires than expected. This indicates that, for this specific year, another variable not considered by the model forced the reduction of fire, even with a suitable condition for its occurrence. Additionally, we noticed a significant increase in the suitability values over time in cells with fire presence (r2 = 0.9 and p < 0.05), with a median value of 0.54 in 2005 and 0.88 in 2020 (Figure 3I).
Generally, the predicted areas with a higher risk of fire were found next to the main highways of Madre de Dios (Interoceanic Highway/Iñapari-Mazuko), Acre (BR-364/Rio Branco-Cruzeiro do Sul and BR-317/Assis Brasil-Boca do Acre), and Pando (RN-13/Porvenir-Puerto Rico, RN-16/Porvenir-Chivé, and RN-18/Zofra-Nareuda). Conversely, the lower-risk areas were found in the least fragmented areas of each state or department, as expected (Figure 1).
The relative importance of each predictive variable is shown in Figure 4. The results indicated a contrasting variability between the human and climatic variables' importance. The percentage area within the grid cell of deforestation, productive land, and forest edge were the variables with the highest gain; therefore, they contained the most useful information to explain the fire occurrence in the MAP region compared to the other variables used to generate all four models. On average, all human-influenced predictive variables (productive land, core area, deforestation, and edge) showed higher relative importance to the models when compared to the climatic variables. Among the climatic variables, the mean temperature of June, July, and August and the maximum temperature of September explain the most fire occurrence in the studied region. Contrary to expectations, the MCWD presented the smallest AUC value (0.52); hence, this variable contributes the least to the predictive models.
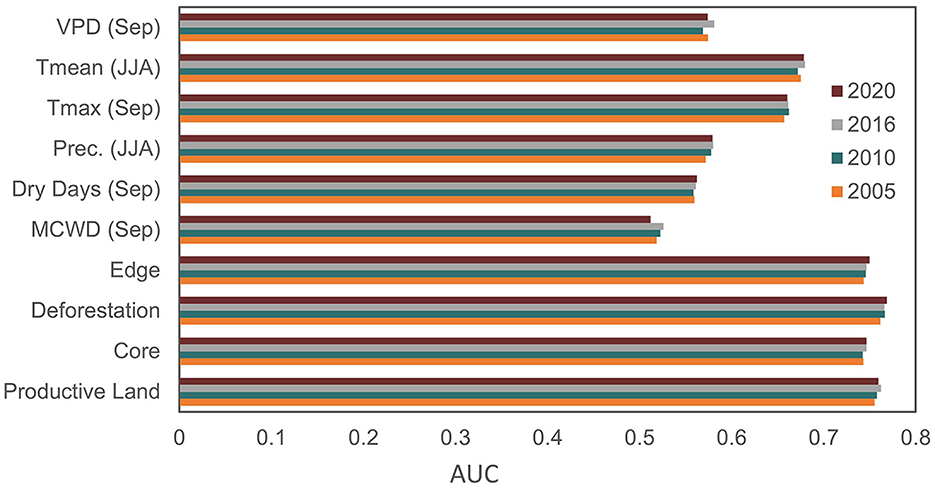
Figure 4. Relative importance based on the AUC test of each predictive variable for the fire probability models in 2005, 2010, 2016, and 2020. “Productive Land”: percentage of productive areas, “Core”: core area, “Deforestation”: annual percentage of deforested area, “Edge”: forest edge area, “MCWD (Sep)”: MCWD index for the month of September, “Dry Days (Sep)”: number of consecutive days with precipitation below 1 mm of September, “Prec. (JJA)”: total precipitation of June, July, and August, “Tmax (Sep)”: maximum temperature of September, “Tmed (JJA)”: average temperature of June, July, and August, “VPD (Sep)”: vapor pressure deficit of September.
The threshold analysis showed that the false positive rate (FPR) decreases as the threshold increases (Figure 5). Also, the sensitivity of the model decreases as the threshold increases. The chosen thresholds to maximize the sensitivity and maintain the low false positive rate were 0.45 ± 0.08 (Supplementary Figure 3). Based on this configuration, all models showed sensitivity higher than 0.8 and FPR lower than 0.25.
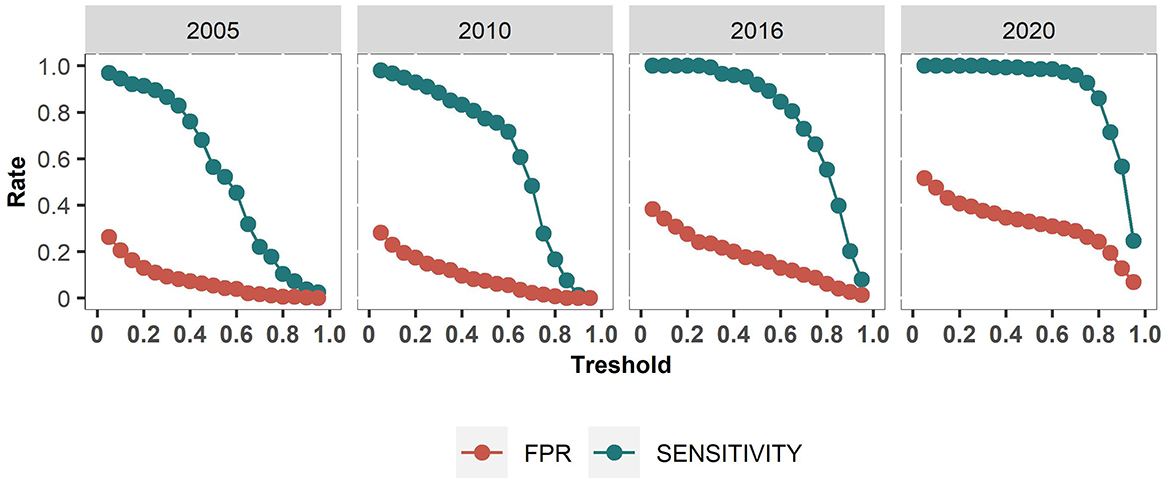
Figure 5. Threshold analysis of maximum entropy models for fire occurrence in 2005, 2010, 2016, and 2020.
The proposed maximum entropy models showed areas with great human and climatic suitability for the occurrence of fire, although there may be no record of active fires in some of these locations (Figure 3). One possible explanation is the absence of drivers leading to fire ignition. Figure 6A shows the contrasting percentage of areas with real fire occurrence and areas with fire-prone conditions. For example, even though fire occurrence was detected in 31.2% of the studied area on average, the region showed environmental and human suitability for fire occurrence in approximately 38.5% of its territory for all years on average. From that area, the Acre region alone contributed to ca. 75% of fire-prone areas found all over the MAP region, followed by Pando with ca. 19%, and Madre de Dios with 6.5%. In 2005 and 2010, our respective models estimated ca. 33% of fire-prone areas, a similar percentage for real occurrence (33.1 and 31.5% respectively). Additionally, we noticed that the total fire-prone area increased from 36.9% in 2016 to 51.5% in 2020 across the studied region. In fact, Madre de Dios and Pando presented the largest fire-prone area in 2020 compared to the other modeled years (6.7 and 9.9% respectively). Additionally, Figure 6B shows the percentage of grid cells with fire suitability >0 that overlap with actual fires. On average, our models estimated fire suitability in 96 ± 3% of actual fires in Acre, 73 ± 14% in Madre de Dios, and 75 ± 20% in Pando.
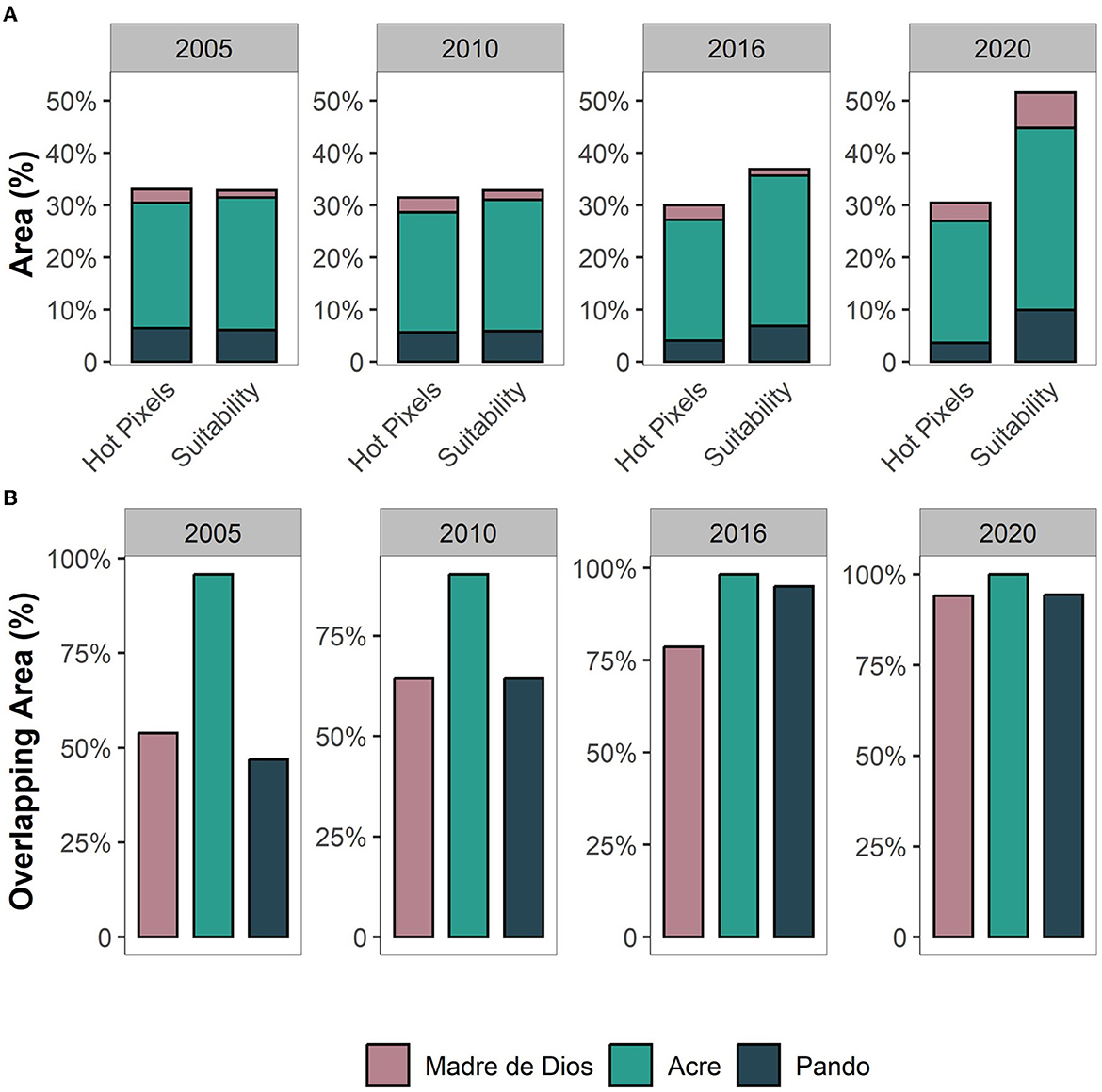
Figure 6. Comparison between models and actual fires. (A) Percentage of fire-prone areas and fire records in the MAP region. (B) Percentage of grid cells with fire risk that overlaps actual fires.
In this study, we noted that the risk of fire is directly related to the intensity of human activity (Figure 4) even though climatic variables also play an important role in occurrence by accentuating the effects of human actions. Accordingly, Figure 7 shows the response curves of fire conditions for each of the predictor variables related to the calibrated models for 2015. Therefore, fire-prone areas are expected to increase as the agricultural activity, the percentage of deforested area, and the percentage of forest edge increases and the core area decreases. Nevertheless, the increase in temperature, vapor pressure deficit, and maximum accumulated water deficit could maximize suitable conditions for fire occurrence over the entire studied area. In addition, Figure 7 shows that the risk of fire is expected to decrease as the total precipitation of June, July, and August tends to increase.
In Figure 8, we provide an annual overview of each variable used as predictors in our study for the three states of the MAP region. The time-series information along with the relative importance of each predictor variable (Figure 4) helps us to understand how all drivers influence the fire occurrence patterns through time and space throughout the period. From Figure 4 we can derive that deforestation, productive land, and forest edge have the highest relative importance to the models; therefore, an increase in these events would result in higher chances of fire occurrence. In fact, we reported an increase in the percentage of deforestation and forest edges for Acre in the last few years, a closely related magnitude for Madre de Dios, and a decrease for Pando. A similar increase-decrease pattern related to the percentage of productive land was reported for all three states. The year 2020 presented the driest September among all years regarding MCWD even though the total precipitation for June, July, and August did not differ greatly from other years. Moreover, 2020 presented the highest values for maximum temperature in September and the vapor pressure deficit for all three states was as high as the other years.
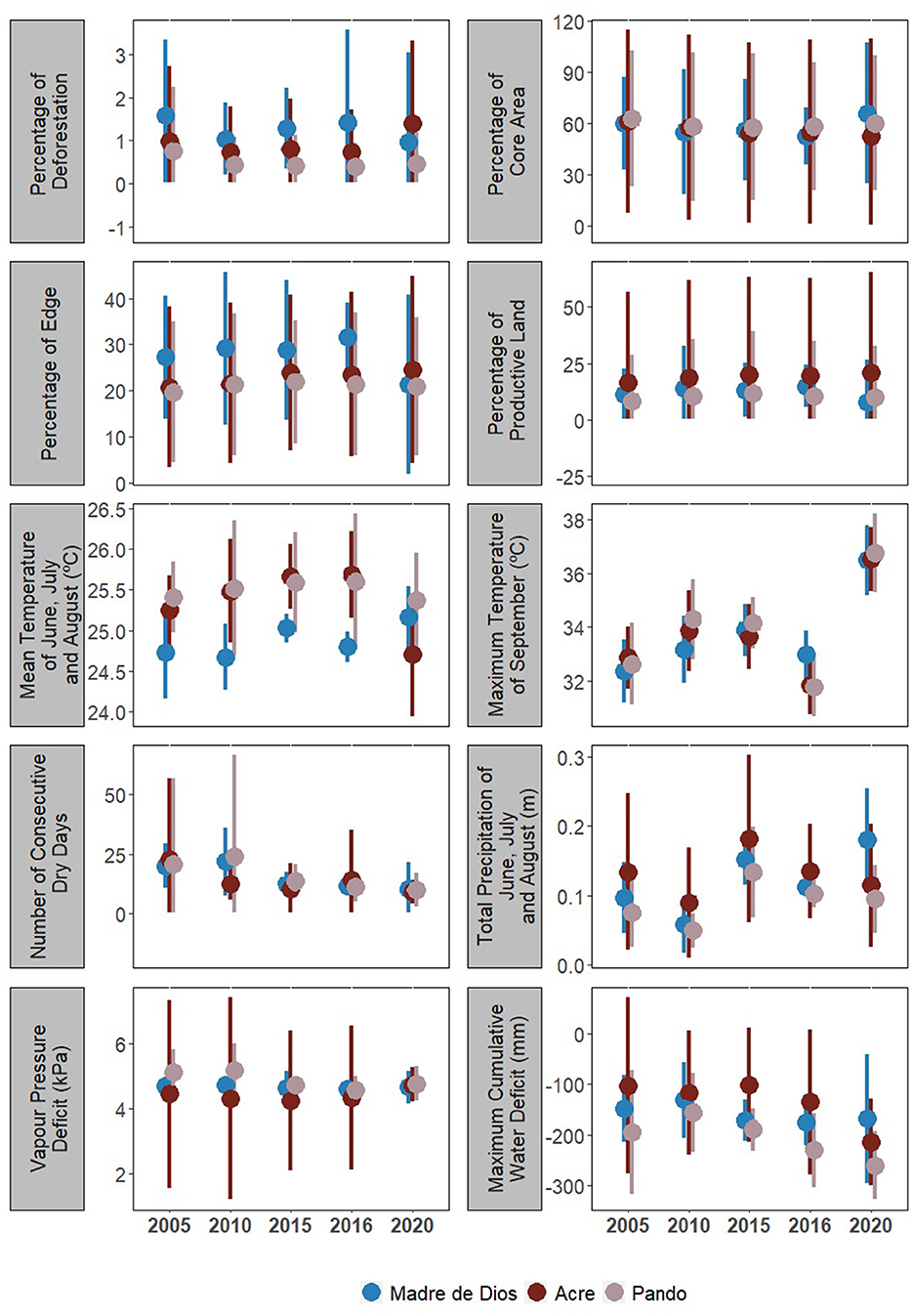
Figure 8. Distribution of human and climatic drivers for fire occurrence in the MAP region for 2005, 2010, 2015, 2016, and 2020. The colored points represent the median value of each MAP region distribution, and the vertical lines are the minimum and maximum range of the distribution.
4. Discussion
4.1. Fire drivers and impacts
In this study, we have investigated the changes in suitability for fire occurrence in southwestern Amazonia for the years 2005, 2010, 2016, and 2020 by proposing a maximum entropy model based on 2015 data. The method has demonstrated a good performance in the studied region by presenting mean AUC values of >0.7 and p-values of <0.05 for all models. Similar results have been documented by other authors (Devisscher et al., 2016; Kim et al., 2019). Based on the visual assessment of the active fire occurrence, we found additional evidence that our models were able to simulate the spatial patterns distribution of fire-prone areas in most of the years, which were concentrated near human settlements and along the major roads (Baraloto et al., 2015; Nascimento et al., 2021). Additionally, our results for the threshold analysis agree with the findings of Fonseca et al. (2016, 2017) for the Brazilian Amazonia, although we have used a different set of metrics.
Our models have advanced our understanding of how the synergy of human and climatic driving factors can affect fire dynamics in Amazonia, supporting decision-makers in the development of new strategies for the management of fire risk, including the use of model results to put in place preventive measures for tropical forest protection. Unlike other studies based on the maxent method to investigate fire dynamics in Amazonia (Fonseca et al., 2016, 2019), our study considered forest fragmentation metrics such as forest edges and core area as fire predictor variables. Forest edges are intimately related to patterns of fire occurrence in tropical forests since fires tend to occur more frequently next to edges due to proximity to human occupancy and microclimate stress such as desiccation (Armenteras et al., 2013, 2017; Silva-Junior et al., 2022). On the other hand, core areas act as an indicator of vulnerability to fire because they are sensitive regions to environmental disturbances and therefore need to be preserved (Vedovato et al., 2016).
We found that anthropic drivers such as deforestation, productive land, and percentage of forest edge contribute the most to explaining the fire distribution in the MAP region. Similarly, recent deforestation and proximity to human settlements were pointed as variables increasing fire occurrence in the Bolivian region by Devisscher et al. (2016). Furthermore, our results are in accordance with several other studies that have modeled fire risk dynamics for Amazonia positively associated with human activities (Andela et al., 2017; Silveira et al., 2020; Silva-Junior et al., 2022).
Even though climatic drivers have not been pointed out as the main drivers by our models, climate is an important factor to understand fire distribution in Amazonia (Le Page et al., 2017), and it may explain up to one-quarter of fire distribution modeling in the Brazilian portion of Amazonia (Oliveira et al., 2022). The study region has important interannual climate differences and effects from the major droughts were localized (da Silva et al., 2018); therefore, generalized emphazise analyses on the effect of climate on fire occurrence can mask the real importance of such drivers. Accordingly, our models showed a great contribution of mean and maximum temperature among the climatic variables, where, in 2020, the average maximum temperature for September was two to four degrees higher than in other years, showing worrying signs for the future. Amazonia is sensitive to high temperatures and reduced precipitation levels, and recurrent extreme droughts events negatively impact forest resilience by decreasing and changing the photosynthetic capacity, increasing tree mortality, and reducing tree growth rates (Anderson et al., 2018; Panisset et al., 2018). At this point, the exposure to extreme dry events is expected to aggravate considerably the risk of fire spread due to the amount of dry woody material. Silva Junior et al. (2019) demonstrated that the 2010 and 2015/2016 droughts caused an anomalous increase in fire occurrence, emitting together 0.47 Pg CO2.
Even though climatic conditions can increase forest flammability (Anderson et al., 2015, 2022; Silva Junior et al., 2019), we demonstrated that fire distribution and the increase of suitability for fire occurrence in Amazonia are mostly related to the increase of human presence such as the intensification of land use changes. With that in mind, it is important to note that deforestation rates have been increasing in the MAP region for the past 10 years (INPE-National Institute for Space Research, 2022a; RAISG-Amazon Network of Georeferenced Socio-Environmental Information, 2022), raising concerns for future trends of increased fire.
In Amazonia, deforestation of either primary or secondary forests is often associated with the clearing of new areas for agricultural and cattle ranching expansion (Aragão et al., 2016b; Heinrich et al., 2021). Along with these activities, the use of fire is a common practice adopted in the Amazonia region for preparing (renovation or clearing) the land for crop plantation or pasture management (Morton et al., 2008; Pivello, 2011; Silveira et al., 2022). In some countries such as Brazil, fire usage goes against national regulations, except for subsistence purposes, which intensifies the risk of accidental and uncontrolled fires escaping into the forest. In the case of Bolivia, since 2013, eight national laws enabled the expansion of the agricultural and livestock production frontier. Among these, Law 1171 has enabled the use of fire to clear land for agriculture and livestock purposes with fire use permits lasting up to 3 and 5 years (Villalobos, 2020). Effects can be more extreme in highly fragmented and degraded landscapes where the vegetation is exposed to extensive edge effects, leading to decreased core areas and increased tree mortality rates (Laurance et al., 2018; Silva-Junior et al., 2022). As a result, the great amount of fuel (dry biomass) at edges becomes available and exposed to drier climatic conditions such as relatively low humidity and high temperatures, providing fitting conditions for fire to spread. From 2016 to 2019 more than 550,000 ha were burned in already deforested areas in Acre, with 33% occurring in 2019 (da Silva et al., 2021).
Fires have a devastating effect on non-adapted tropical ecosystems such as Amazonian forests, putting the integrity of the ecosystem services provided (e.g., carbon cycling, soil quality, and biodiversity loss) at risk. For instance, tree mortality or physiological stress caused by the reduction of photosynthetic activity are just some of the consequences of recurrent fires on vegetation (Phillips et al., 2015; Hood et al., 2018; Oliveira et al., 2022). Additionally, frequent recurrence of fires directly affects the resilience of the ecosystem, increasing vulnerabilities to new fires as they are likely to burn again due to lower humidity on the accumulated fuel load (Pellegrini et al., 2018). According to da Silva et al. (2021), at least 40% of the total area affected by fires in Acre burned more than once between 2016 and 2019.
In addition to environmental damage, large fires cause great economic losses by compromising public health (Ahrens and Evarts, 2020; Campanharo et al., 2021), contaminating water resources, damaging infrastructure (Diaz, 2012), and decreasing inputs and outputs of commodities (De Mendonça et al., 2004). Our results emphazise that, with the expansion of productive lands and increased deforestation, the population within the MAP region will be exposed to higher fire risk areas, hence worsening air quality and its consequences. Moreover, the economic losses may slow down or even rewind investments in fire prevention and mitigation, which are crucial in our current climate change scenario.
4.2. The increasing threat of fire
We found the greatest disparity between the models and the observed fires for 2020 by predicting larger areas with suitable conditions for fire occurrence. This indicates that even though there were conditions for fires to occur, other factors not addressed in this study (e.g., ignition sources or physical barriers), may have prevented some of them from occurring or taking up larger proportions. There are many challenges in modeling fire dynamics systems, such as embodying all the physical and chemical processes necessary for perfect model performance. Thus, some estimates are expected to fall short occasionally, and uncertainties will exist. Still, the model was assertive in estimating more fires in 2020 compared to the last decade. In fact, the year 2020 presented the greatest number of active fires in the region since 2010, surpassing the fire amount detected during the 2015/2016 drought (INPE-National Institute for Space Research, 2022b).
At the state and/or department level, Acre registered the largest number of fires and fire-prone areas followed by Pando and then Madre de Dios in 2020. One possible explanation for the model's overestimation might be related to the combined set of contrasting conditions found across the MAP region in 2020, such as the increasing rates of deforestation, a high percentage of agricultural activities, high temperatures, and low water deficit indices. In addition, the alarming scenario for 2020 was maximized by the regulations that stimulate the use of fire to expand the agricultural frontier such as in the case of Pando.
All three MAP regions presented spatially higher risk of fires in 2020. Nonetheless, the suitability of fire occurrence in Madre de Dios in 2020 was the highest among the studied years and most different from the actually registered fires. In this case, it is likely assigned to deforestation rates, which leads to more fragmented areas, hence creating ideal conditions for fires, as already discussed. Since the construction and paving of the Interoceanic Highway in 2010, illegal human activities such as deforestation and gold mining have been increasing in the region (Anzualdo et al., 2022). Although the primary goal of the paving of the Interoceanic Highway was to incorporate the MAP region into the global economy, it also has enabled the exploitation of primary forests by providing access to previously isolated areas and increasing deforestation (Baraloto et al., 2015). Because the majority of the Madre de Dios territory is composed of forest, this scenario raises a worrying concern for the risk of future uncontrolled fires escaping into adjacent forested areas in the region, thus jeopardizing the integrity of the ecosystem.
In general, the alarming rates of increasing deforestation in southwestern Amazonia in recent years might be related to the history of political and socio-economic instability in Brazil, Bolivia, and Peru. For the last few years, these countries have experienced poor or weakened environmental governance, leading to non-compliance with regulatory measures, thus aggravating the widespread fire risk factors. Associated with deforestation, fire in pasture represents another important aspect to be considered as an increasing threat because beef production and the converted pasture area in Amazonia have increased sharply by 184 and 235%, respectively (França et al., 2021). In the state of Acre alone, approximately 55–65% of fires occurred in consolidated pasture areas between 2016 and 2019 (da Silva et al., 2021).
The future of the MAP region may be at risk in the face of increasing human pressures and ongoing climate change. Fu et al. (2013) warned of the increased probability of more prolonged drought events in the southwest region of Amazonia, where the MAP region is located. It is possibly already experiencing changes in the regional climate. However, if global warming above 1.5°C materializes, the effects on the fire regime could be catastrophic, with serious regional and global implications (Mckay et al., 2022).
Although our model overestimated the fire risk throughout the studied region in 2020, the outcomes should not be taken with less credit. In fact, our estimates for 2020 are a warning to the authorities about the potential fire hazard of the landscape, and domestic policies based on international commitments must be put in place and effectively implemented, especially in Brazil. The strengthening of environmental policies to combat deforestation and firefighting is necessary so that the increased risk of fires evidenced in this study will not be aggravated in the future.
4.3. Policy implications for fire threat
Specifically in Brazil and Bolivia, aggressive economic policies have been adopted, not to mention the increased number of statements made by some authorities to discredit the role of environmental agencies in complying with environmental regulations. This type of economic approach, when implemented without sustainable planning, maximizes the existent environmental threats by reducing the restrictions of environmental regulations and turning a blind eye to illegal exploitation and deforestation increasing rates (Abessa et al., 2019; Londoño and Casado, 2019; Villalobos, 2020).
It is known that fires directly impact the economy of the region, yet almost no effort is taken to enhance the budget available by the federal governments for the control and management of fire in the Brazilian Legal Amazonia, which represents on average only 1% of the entire budget intended for the Brazilian Ministry of the Environment (Morello et al., 2020). On the contrary, the Brazilian environment ministry budget has been constantly reduced since 2019. In addition to a 10% reduction in the budget for environmental inspection and firefighting programs compared to 2019, which accounted for more than BRL 20 million reduction, 2020 gathered the lowest number of environmental fines since 2012, when the Brazilian environmental regulation for the protection and conservation of natural terrestrial ecosystems was updated (Menegassi, 2021). As a result of the environmental regulation dismantling over the past few years, deforestation and forest degradation in private and public lands have been rapidly increasing (Mataveli et al., 2021). It is important to highlight that the increase in deforestation in the state of Acre accounted for 10.5K km2 in 2020 alone, a 40% increase compared to 2016 (INPE-National Institute for Space Research, 2022a).
Similarly, Bolivia and Peru have experienced the weakening of environmental governance as well as Brazil by tax pardoning and reducing financial resources (Bolivia, 2013, 2019; Perú, 2020). The major consequences of political issues take place at national and regional levels, deflagrating institutional vulnerabilities. According to Pismel et al. (2023), the MAP region has different levels of governance vulnerabilities, however, most of them are concentrated in limited inspections influenced by the deregulation of public policies and environmental legislation and reduced technical staff. Another politically related aspect pointed out by the authors was regarding constant staff turnover, which could jeopardize the investments and communication between stakeholders, scientific institutions, and public organizations.
Nevertheless, predatory development policy and lack of governance may be considered important drivers of forest degradation in the MAP region, mainly in recent years. In the case of Pando, the increase in wildfires and deforestation have a strong impact on non-timber species such as Brazil nuts (Bertholletia excelsa), a species that has contributed positively to the local economy for centuries. Almost all of Brazil nut production comes from Pando and contributes to approximately 2% of the non-traditional export products of Bolivia. Additionally, the increase in extreme climatic events (e.g., droughts, and flooding), fire, and hurricane-force winds are threats to Pando forests (Quiroz-Claros et al., 2017). Noteably, Bolivia has very low fines to penalize burning (0.20 US$ per ha), which are insufficient to discourage forest burning (Cauthin, 2022).
The lack of effectiveness with which environmental issues have been conducted in these three countries, given the weakening of environmental policies, builds a concerning high-risk scenario for wildfires in the future. Therefore, the establishment of new fire risk management strategies should be based on science rather than political interests.
5. Final consideration
Our study provided important insights about fire drivers on a human-modified landscape during years with different drought intensities and extents. For instance, our results have confirmed that anthropic activities are directly related to fire dynamics in Amazonia and can increase fire occurrence even in non-extreme drought years. Even though climate is an important variable to explain and magnify fire distribution, our models demonstrated that increasing deforestation and agricultural activities also increase the risk of fire in the MAP region. Therefore, controlling human activities is currently necessary to avoid future scenarios of forest degradation and the worsening of socioeconomic problems driven by extreme fire events in the MAP region.
We showed that the modeling approach based on maximum entropy has the potential to be a useful tool for evaluating the implications of climatic and anthropogenic variables on fire distribution. Furthermore, because the model can be easily employed to predict vulnerable and resilient locations for fire occurrence, it can help anticipatory planning to prevent potential impacts associated with large wildfires in the future at regional levels.
Finally, it is important to highlight that our models have limitations like many others because they do not fully represent the complexity of forces that fire events are driven by, which turns it into a very challenging task. Thus, to improve fire risk assessments and reduce the modeling uncertainties, we strongly suggest that future studies integrate political aspects as part of their set of predictive variables. Different political interests may influence socio-economic activities, resulting in different patterns of deforestation and agricultural productivity, leading to different patterns of fire distribution.
Data availability statement
The original contributions presented in the study are included in the article/Supplementary material, further inquiries can be directed to the corresponding author.
Author contributions
IF, LAr, and LAn conceptualized and designed the study and interpreted the results. IF and WC performed the formal analysis. IF and MB wrote the original draft. All authors contributed to reviewing and editing the manuscript draft and approved the submitted version.
Funding
This study was financed in part by the Inter-American Institute for Global Change Research (IAI), process: SGP-HW 016 and Coordination for the Improvement of Higher Education Personnel—Brazil (CAPES)—Finance Code 001. LAn thanks the Brazilian National Council for Scientific and Technological Development (CNPq) for the productivity scholarship, process no.314473/2020-3 and the São Paulo Research Foundation (FAPESP) grant number: 2020/15230-5. SS thanks CNPq for financing grant CNPq/Prevfogo-Ibama N° 33/2018 AcreQueimadas Project number 442650/2018-3. LAr thanks CNPq (grant no. 314416/2020-0) and the Brazilian Space Agency (AEB). GS thanks the PRODIGY project funded by the German Federal Ministry of Education and Research (BMBF) BioTip research program.
Acknowledgments
IF thanks the infrastructure and support provided by the National Institute for Space Research-INPE. All authors acknowledge the use of data from Centro Nacional de Monitoramento e Alertas de Desastres Naturais-CEMADEN and the use of data and/or imagery from NASA's Earth Observing System Data and Information System (EOSDIS). We also acknowledge the use of free land use and land cover dataset available from MapBiomas Amazonia Project.
Conflict of interest
GS was employed by ECOS Consult.
The remaining authors declare that the research was conducted in the absence of any commercial or financial relationships that could be construed as a potential conflict of interest.
Publisher's note
All claims expressed in this article are solely those of the authors and do not necessarily represent those of their affiliated organizations, or those of the publisher, the editors and the reviewers. Any product that may be evaluated in this article, or claim that may be made by its manufacturer, is not guaranteed or endorsed by the publisher.
Supplementary material
The Supplementary Material for this article can be found online at: https://www.frontiersin.org/articles/10.3389/ffgc.2023.1107417/full#supplementary-material
References
Abessa, D., Famá, A., and Buruaem, L. (2019). The systematic dismantling of Brazilian environmental laws risks losses on all fronts. Nat. Ecol. Evol. 3, 510–511. doi: 10.1038/s41559-019-0855-9
Ahrens, M., and Evarts, B. (2020). Fire loss in the United States during 2019. NFPA - National Fire Protection Association. Available online at: https://www.nfpa.org//-/media/Files/News-and-Research/Fire-statistics-and-reports/US-Fire-Problem/osFire Loss.pdf (accessed on November 15, 2022).
Andela, N., Morton, D. C., Giglio, L., Chen, Y., Van Derwerf, G. R., Kasibhatla, P. S., et al. (2017). A human-driven decline in global burned area. Science 356, 1356–1362. doi: 10.1126/science.aal4108
Anderson, L. O., Aragão, L. E. O. C., Gloor, M., Arai, E., Adami, M., Saatchi, S. S., et al. (2015). Disentangling the contribution of multiple land covers to fire-mediated carbon emissions in Amazonia during the 2010 drought. Global Biogeochem. Cycles 29, 1739–1753. doi: 10.1002/2014GB005008
Anderson, L. O., Burton, C., Dos Reis, J. B. C., Pessôa, A. C. M., Bett, P., Carvalho, N. S., et al. (2022). An alert system for seasonal fire probability forecast for South American protected areas. Climate Resil. Sustain. 1, e19. doi: 10.1002/cli2.19
Anderson, L. O., Neto, G. R., Cunha, A. P., Fonseca, M. G., De Moura, Y. M., Dalagnol, R., et al. (2018). Vulnerability of Amazonian forests to repeated droughts. Philos. Trans. R. Soc. B Biol. Sci. 373, 1760. doi: 10.1098/rstb.2017.0411
Anderson, L. O., Yamamoto, M., Cunningham, C., Fonseca, M. G., Fernandes, L. K., Pimentel, A., et al (2017). Utilização de Dados Orbitais de Focos de Calor Para Caracterização de Riscos de Incêndios Florestais e Priorização De Áreas Para A Tomada De Decisão. Revista Brasileira de Cartografia, 69, 1. Available online at: https://seer.ufu.br/index.php/revistabrasileiracartografia/article/view/44038. doi: 10.14393/rbcv69n1-44038
Anzualdo, V. I. T., Esenarro, D., Guillen, R., and Reyna, S. (2022). Deforestation in Peru and strategic plan for its reduce Amazonian forests. 3C Tecnol. innovación Apl. a la pyme 40–2, 97–111. doi: 10.17993/3ctecno.2022.specialissue9.97-111
Aragão, L. E. O. C., Anderson, L. O., Fonseca, M. G., Rosan, T. M., Vedovato, L. B., Wagner, F. H., et al. (2018). 21st Century drought-related fires counteract the decline of Amazon deforestation carbon emissions. Nat. Commun. 9, 1–12. doi: 10.1038/s41467-017-02771-y
Aragão, L. E. O. C., Anderson, L. O., Lima, A., and Arai, E. (2016b). “Fires in Amazonia,” in Interactions Between Biosphere, Atmosphere and Human Land Use in the Amazon Basin, eds L. Nagy, B. R. Forsberg, P. Artaxo (Heidelberg: Springer), 301–329.
Aragão, L. E. O. C., Malhi, Y., Barbier, N., Lima, A., Shimabukuro, Y., Anderson, L., et al. (2008). Interactions between rainfall, deforestation, and fires during recent years in the Brazilian Amazonia. Philos. Trans. R. Soc. B Biol. Sci. 363, 1779–1178. doi: 10.1098/rstb.2007.0026
Aragão, L. E. O. C., Malhi, Y., Roman-Cuesta, R. M., Saatchi, S., Anderson, L. O., and Shimabukuro, Y. E. (2007). Spatial patters and fire response of recent Amazonian droughts. Geophys. Res. Lett. 34, L07701. doi: 10.1029/2006gl028946
Aragão, L. E. O. C., Marengo, J. A., Cox, P. M., Betts, R. A., Costa, D., Kaye, N., et al. (2016a). Assessing the Influence of Climate Extremes on Ecosystems and Human Health in Southwestern Amazon Supported by the PULSE-Brazil Platform. Am. J. Climate Change 3, 399–416. doi: 10.4236/ajcc.2016.53030
Aragão, L. E. O. C., Poulter, B., Barlow, J. B., Anderson, L. O., Malhi, Y., Saatchi, S., et al. (2014). Environmental change and the carbon balance of Amazonian forests. Biol. Rev. 4, 913–931. doi: 10.1111/brv.12088
Armenteras, D., Barreto, J. S., Tabor, K., Molowny-Horas, R., and Retana, J. (2017). Changing patterns of fire occurrence in proximity to forest edges, roads and rivers between NW Amazonian countries. Biogeoscience 14, 2755–2765. doi: 10.5194/bg-14-2755-2017
Armenteras, D., González, T. M., and Retana, J. (2013). Forest fragmentation and edge influence on fire occurrence and intensity under different management types in Amazon forests. Biol. Conserv. 159, 73–79. doi: 10.1016/j.biocon.2012.10.026
Baraloto, C., Alverga, P., Quispe, S. B., Barnes, G., Chura, N. B., da Silva, I. B., et al. (2015). Effects of road infrastructure on forest value across a tri-national Amazonian frontier. Biol. Conserv. 191, 674–681. doi: 10.1016/j.biocon.2015.08.024
Bolivia, E. P. d. (2013). Ley no 337 de 11 de enero de 2013. Ley de Apoyo a la Producción de alimentos y restituición de bosques. Available online at: https://www.ilo.org/dyn/natlex/docs/ELECTRONIC/92465/107725/F-1108435951/BOL92465.pdf (accessed o n November 10, 2022).
Bolivia, E. P. d. (2019). Ley No 1171 de 25 de abril de 2019. Ley de uso y manejo de quemas. Available online at: https://web.senado.gob.bo/sites/default/files/LEY N°1171-2019.PDF (accessed on November 10, 2022).
Brown, I. F., Schroeder, W., Setzer, A., De Los Ríos Maldonado, M., Pantoja, N., Duarte, A., et al. (2006). Monitoring fires in southwestern Amazonia Rain Forests. Eos Trans. Am. Geophys. Union 87, 253–259. doi: 10.1029/2006EO260001
Campanharo, W., Lopes, A., Anderson, L., da Silva, T., and Aragão, L. (2019). Translating fire impacts in southwestern amazonia into economic costs. Remote Sens. 11, 764. doi: 10.3390/rs11070764
Campanharo, W. A., Morello, T., Christofoletti, M. A. M., and Anderson, L. O. (2021). Hospitalization due to fire-induced pollution in the brazilian legal Amazon from 2005 to 2018. Remote Sens. 14, 69. doi: 10.3390/rs14010069
Carmo, C. N., Alves, M. B., Hacon, S., and de, S. (2013). Impact of biomass burning and weather conditions on children's health in a city of Western Amazon region. Air Qual. Atmos. Heal. 6, 517–525. doi: 10.1007/s11869-012-0191-6
Carvalho, J. L., Avanzi, J. C., Silva, M. L. N., Mello, C. R., and Cerri, C. E. P. (2010). Potencial de sequestro de carbono em diferentes biomas do Brasil. Ver. Bras. de Ciênc. Solo 34, 277–290. doi: 10.1590/S0100-06832010000200001
Carvalho, N. S., Anderson, L. O., Nunes, C. A., Pessôa, A. C. M., Silva Junior, C. H. L., Reis, J. B. C., et al. (2021). Spatio-Temporal variation in dry season determines the Amazonian fire calendar. Environ. Res. Lett. 16, ac3aa3. doi: 10.1088/1748-9326/ac3aa3
Cauthin, M. (2022). Bolivia, el país con la multa más barata por deforestar. Available online at: https://fundacionsolon.org/2022/10/21/bolivia-el-pais-con-la-multa-mas-barata-por-deforestar-e-incendiar/ (accessed on November 10, 2022).
Chuvieco, E., Aguado, I., Jurdao, S., Pettinari, M. L., Yebra, M., Salas, J., et al. (2014). Integrating geospatial information into fire risk assessment. Int. J. Wildl. Fire 23, 606–620. doi: 10.1071/WF12052
da Silva, S. S., Fearnside, P. M., de Graça, P. M. L. A, Brown, I. F., Alencar, A., and de Melo, A. W. F. (2018). Dynamics of forest fires in the southwestern Amazon. For. Ecol. Manag. 424, 312–322. doi: 10.1016/j.foreco.2018.04.041
da Silva, S. S., Oliveira, I., Morello, T. F., Anderson, L. O., Karlokoski, A., Brando, P. M., et al. (2021). Burning in southwestern Brazilian Amazonia, 2016–2019. J. Environ. Manag. 286, 112189. doi: 10.1016/j.jenvman.2021.112189
De Mendonça, M. J. C., Vera Diaz, M. D. C., Nepstad, D., Seroa Da Motta, R., Alencar, A., Gomes, J. C., et al. (2004). The economic cost of the use of fire in the Amazon. Ecol. Econ. 49, 89–105. doi: 10.1016/j.ecolecon.2003.11.011
Devisscher, T., Anderson, L. O., Aragão, L. E. O. C., Galván, L., and Malhi, Y. (2016). Increased wildfire risk driven by climate and development interactions in the Bolivian Chiquitania, Southern Amazonia. PLoS ONE 11, e0161323. doi: 10.1371/journal.pone.0161323
Diaz, J. M. (2012). Economic impacts of wildfire. Joint Fire Science Program; Southern Fire Exchange. Available online at: https://fireadaptednetwork.org/wp-content/uploads/2014/03/economic_costs_of_wildfires.pdf (accessed on July 10, 2022).
Elith, J., Phillips, S. J., Hastie, T., Dudík, M., Chee, Y. E., and Yates, C. J. (2011). A statistical explanation of MaxEnt for ecologists. Divers. Distrib. 17, 43–57. doi: 10.1111/j.1472-4642.2010.00725.x
Faria, B. L., Brando, P. M., Macedo, M. N., Panday, P. K., Soares-Filho, B. S., and Coe, M. T. (2017). Current and future patterns of fire-induced forest degradation in amazonia. Environ. Res. Lett. 12, aa69ce. doi: 10.1088/1748-9326/aa69ce
Ferreira, I. J. M., Campanharo, W. A., Fonseca, M. G., Escada, M. I. S., Nascimento, M. T., Villela, D. M., et al. (2023). Potential aboveground biomass increase in brazilian atlantic forest fragments with climate change. Glob. Chang. Biolog. Under Review.
Flannigan, M. D., Krawchuk, M. A., De Groot, W. J., Wotton, B. M., and Gowman, L. M. (2009). Implications of changing climate for global wildland fire. Int. J. Wildl. Fire 18, 483–507. doi: 10.1071/WF08187
Fonseca, M. G., Alves, L. M., Aguiar, A. P. D., Arai, E., Anderson, L. O., Rosan, T. M., et al. (2019). Effects of climate and land-use change scenarios on fire probability during the 21st century in the Brazilian Amazon. Glob. Chang. Biol. 25, 2931–2946. doi: 10.1111/gcb.14709
Fonseca, M. G., Anderson, L. O., Arai, E., Shimabukuro, Y. E., Xaud, H. A. M., Xaud, M. R., et al. (2017). Climatic and anthropogenic drivers of northern Amazon fires during the 2015-2016 El Niño event. Ecol. Appl. 27, 2514–2527. doi: 10.1002/eap.1628
Fonseca, M. G., Aragão, L. E. O. C., Lima, A., Shimabukuro, Y. E., Arai, E., and Anderson, L. O. (2016). Modelling fire probability in the Brazilian Amazon using the maximum entropy method. Int. J. Wildl. Fire 25, 955–969. doi: 10.1071/WF15216
França, F., Solar, R., Lees, A. C., Martins, L. P., Berenguer, E., and Barlow, J. (2021). Reassessing the role of cattle and pasture in Brazil's deforestation: A response to “Fire, deforestation, and livestock: When the smoke clears.” Land use Policy 108, 105195. doi: 10.1016/j.landusepol.2020.105195
Fu, R., Yin, L., Li, W., Arias, P. A., Dickinson, R. E., Huang, L., et al. (2013). Increased dry-season lenght over southern Amazonia in recent decades and its implication for future climate projection. Proc. Nat. Acad. Sci. 110, 18110–18115. doi: 10.1073/pnas.1302584110
Gatti, L. V., Basso, L. S., Miller, J. B., Gloor, M., Domingues, L. G., Cassol, H. L. G., et al. (2021). Amazonia as a carbon source linked to deforestation and climate change. Nature 595, 388–393. doi: 10.1038/s41586-021-03629-6
Heinrich, V. H. A., Dalagnol, R., Cassol, H. L. G., Rosan, T. M., de Almeida, C. T., Silva Junior, C. H. L., et al. (2021). Large carbon sink potential of secondary forests in the Brazilian Amazon to mitigate climate change. Nat. Commun. 12, 1–11. doi: 10.1038/s41467-021-22050-1
Hersbach, H., Bell, B., Berrisford, P., Hirahara, S., Horánil, A., Muñoz-Sabater, J., et al. (2021). The ERA5 flobal reanalysis. Quart. J. Royal Meterol. Soc. 146, 1999–2049. doi: 10.1002/qj.3803
Hood, S. M., Varner, J. M., van Mantgem, P., and Cansler, C. A. (2018). Fire and tree death: understanding and improving modeling of fire-induced tree mortality. Environ. Res. Lett. 13, 113004. doi: 10.1088/1748-9326/aae,934
INPE-National Institute for Space Research (2022a). TerraBrasilis: Deter (avisos). Available online at: http://terrabrasilis.dpi.inpe.br/app/dashboard/deforestation/biomes/legal_amazon/increments (accessed on August 15, 2022).
INPE-National Institute for Space Research (2022b). Monitoramento dos Focos Ativos por Bioma. Queimadas. Available online at: http://queimadas.dgi.inpe.br/queimadas/portal-static/estatisticas_estados/ (accessed on August 15, 2022).
Kim, S. J., Lim, C. H., Kim, G. S., Lee, J., Geiger, T., Rahmati, O., et al. (2019). Multi-temporal analysis of forest fire probability using socio-economic and environmental variables. Remote Sens. 11, 86. doi: 10.3390/rs11010086
Knorr, W., Arneth, A., and Jiang, L. (2016). Demographic controls of future global fire risk. Nat. Clim. Chang. 6, 781–785. doi: 10.1038/nclimate2999
Krawchuk, M. A., and Moritz, M. A. (2011). Constraints on global fire activity vary across a resource gradient. Ecology 92, 121–132. doi: 10.1890/09-1843.1
Krawchuk, M. A., Moritz, M. A., Parisien, M.-A., Van Dorn, J., and Hayhoe, K. (2009). Global pyrogeography: the current and future distribution of wildfire. PLoS ONE 4, e5102. doi: 10.1371/journal.pone.0005102
Lapola, D. M., Pinho, P., Barlow, J., Aragão, L. E. O. C, Berenguer, E., Carmenta, R., et al. (2023). The drivers and impacts of Amazon forest degradation. Science. 279, eabp8622. doi: 10.1126/science.abp8622
Laurance, W. F., Camargo, L. C., Fearnside, P. M., Lovejoy, T. E., Williamson, G. B., Mesquita, R. C. G., et al. (2018). An Amazonian rainforest and its fragments as a laboratory of global change. Biol. Rev. 93, 223–247. doi: 10.1111/brv.12343
Le Page, Y., Morton, D., Hartin, C., Bond-Lamberty, B., Pereira, J. M. C., Hurtt, G., et al. (2017). Synergy between land use and climate change increases future fire risk in Amazon forests. Earth Syst. Dyn. 8, 1237–1246. doi: 10.5194/esd-8-1237-2017
Lewis, S. L., Brando, P. M., Phillips, O. L., Heijden, G. M. F., and van der Nepstad, D. C. (2011). The 2010 Amazon drought. Science 331, 554. doi: 10.1126/science.1200807
Londoño, E., and Casado, L. (2019). Amazon Deforestation in Brazil Rose Sharply on Bolsonaro's Watch. New York, NY: New York Times. Available online at: https://www.nytimes.com/2019/11/18/world/americas/brazil-amazon-deforestation.html (accessed on October 10 2022).
Maeda, E. E., Ma, X., Wagner, F. H., Kim, H., Oki, T., Eamus, D., et al. (2017). Evapotranspiration seasonality across the Amazon Basin. Earth Syst. Dyn. 8, 439–454. doi: 10.5194/esd-8-439-2017
Marengo, J. A., Nobre, C. A., Tomasella, J., Oyama, M. D., Oliveira, G. S., Oliveira, R., et al. (2008). The drought of amazonia in 2005. J. Climat 21, 495–516. doi: 10.1175/2007JCLI1600.1
Marsik, M., Stevens, F. R., and Southworth, J. (2011). Amazon deforestation: Rates and patterns of land cover change and fragmentation in Pando, northern Bolivia, 1986 to 2005. Prog. Phys. Geogr. 35, 353–374. doi: 10.1177/0309133311399492
Mataveli, G. A. V., de Oliveira, G., Seixas, H. T., Pereira, G., Stark, S. C., Gatti, L. V., et al. (2021). Relationship between biomass burning emissions and deforestation in amazonia over the last two decades. Forests 12, 1–19. doi: 10.3390/f12091217
Mckay, D. I. A., Stall, A., Abrams, J. F., Winkelmann, R., Sakschewski, B., Loriani, S., et al. (2022). Exceeding 1.5° C global warming could trigger multiple climate tipping point. Science. 377, 944–945. doi: 10.1126/science.abn7950
Mendoza, E., Perz, S., and Aguilar, C. (2007). The “Knowledge Exchange Train”: A model for capacity building for participatory governance in the south-western Amazon. Dev. Pract. 17, 791–799. doi: 10.1080/09614520701628451
Menegassi, D. (2021). Ministério do Meio Ambiente tem o menor orçamento das últimas duas décadas. Milwaukie, OR: OECO. Available online at: https://oeco.org.br/noticias/ministerio-do-meio-ambiente-tem-menor-orcamento-das-ultimas-duas-decadas/ (accessed on October 05, 2022).
Michaelsen, A. C., Briceño, L. H., Menis, R. F., Chura, N. B., Tito, F. V., Perz, S., et al. (2013). Regional deforestation trends within local realities: land-cover change in Southeastern Peru 1996-2011. Land 2, 131–157. doi: 10.3390/land2020131
Morello, T. F., Ramos, R. M. O, Anderson, L., Owen, N., Rosan, T. M., et al. (2020). Predicting fires for policy making: Improving accuracy of fire brigade allocation in the Brazilian Amazon. Ecol. Econ. 169, 106501. doi: 10.1016/j.ecolecon.2019.106501
Moritz, M. A., Morais, M. E., Summerell, L. A., Carlson, J. M., and Doyle, J. (2005). Wildfires, complexity, and highly optimized tolerance. Proc. Natl. Acad. Sci. U.S.A. 102, 17912–17917. doi: 10.1073/pnas.0508985102
Moritz, M. A., Parisien, M.-A., Batllori, E., Krawchuk, M. A., Van Dorn, J., Ganz, D. J., et al. (2012). Climate change and disruptions to global fire activity. Ecosphere 3, art49. doi: 10.1890/ES11-00345.1
Morton, D. C., Defries, R. S., Randerson, J. T., Giglio, L., Schroeder, W., and van der Werf, G. R. (2008). Agricultural intensification increases deforestation fire activity in Amazonia. Glob. Chang. Biol. 14, 2262–2275. doi: 10.1111/j.1365-2486.2008.01652.x
Murray, F. W. (1967). On the computation of saturation vapor pressure. J. Appl. Meteorol. 6, 203–204. doi: 10.1175/1520-0450(1967)006andlt
Myers, N., Mittermeier, R. A., Mittermeier, C. G., Fonseca, G. A. B., and Kent, J. (2000). Biodiversity hotspots for conservation priorities. Nature. 403, 853–858. doi: 10.1038/35002501
Nascimento, E., de, S., da Silva, S. S., Bordignon, L., de Melo, A. W. F., Brandão, A., et al. (2021). Roads in the southwestern amazon, state of acre, between 2007 and 2019. Land 10, 1–12. doi: 10.3390/land10020106
Oliveira, U., Soares-Filho, B., Bustamante, M., Gomes, L., Ometto, J. P., and Rajão, R. (2022). Determinants of fire impact in the Brazilian biomes. Front. For. Glob. Chang 5, 735017. doi: 10.3389/ffgc.2022.735017
Panisset, J. S., Libonati, R., Gouveia, C. M. P., Machado-Silva, F., Franca, D. A., Franca, J. R. A., et al. (2018). Contrasting patterns of the extreme drought episodes of 2005, 2010 and 2015 in the Amazon Basin. Int. J. Climatol. 38, 1096–1104. doi: 10.1002/joc.5224
Parisien, M. A., and Moritz, M. A. (2009). Environmental controls on the distribution of wildfire at multiple spatial scales. Ecol. Monogr. 79, 127–154. doi: 10.1890/07-1289.1
Pellegrini, A. F. A., Ahlström, A., Hobbie, S. E., Reich, P. B., Nieradzik, L. P., Staver, A. C., et al. (2018). Fire frequency drives decadal changes in soil carbon and nitrogen and ecosystem productivity. Nature 553, 194–198. doi: 10.1038/nature24668
Perú (2020). Presupuesto del Minam para el 2021 Asciende a S/ 665.56 Millones. Available online at: https://www.gob.pe/institucion/minam/noticias/318128-presupuesto-del-minam-para-el-2021-asciende-a-s-665-56-millones [Accessed August 15, 2022]
Perz, S. G., Cabrera, L., Carvalho, L. A., Castillo, J., Chacacanta, R., Cossio, R. E., et al. (2012). Regional integration and local change: road paving, community connectivity, and social-ecological resilience in a tri-national frontier, southwestern Amazonia. Reg. Environ. Chang 12, 35–53. doi: 10.1007/s10113-011-0233-x
Phillips, O. L., Aragão, L. E. O. C., Lewis, S. L., Lewis, S. L., Fisher, J. B., Lloyd, J., et al. (2009). Drought sensitivity of the amazon rainforest. Science 323, 1344–1347. doi: 10.1126/science.1164033
Phillips, O. L., Silva-Espejo, J. E., Alvarez-Dávila, E., Nuñez Vargas, P., Baccini, A., Brienen, R. J. W., et al. (2015). Ecosystem heterogeneity determines the ecological resilience of the Amazon to climate change. Proc. Natl. Acad. Sci. U.S.A. 113, 793–797. doi: 10.1073/pnas.1511344112
Phillips, S. J., Anderson, R. P., Dudík, M., Schapire, R. E., and Blair, M. E. (2017). Opening the black box: an open-source release of Maxent. Ecography 40, 887–893. doi: 10.1111/ecog.03049
Phillips, S. J., Anderson, R. P., and Schapire, R. E. (2006). Modelling and analysis of the atmospheric nitrogen deposition in North Carolina. Int. J. Glob. Environ. Issues 6, 231–252. doi: 10.1504/IJGENVI.2006.010156
Pismel, G., Marchezini, V., Anderson, L.o, Selaya, G., Depaula, Y., and Mendoza, E. (2023). Wildfires Governance in a tri-national frontier of southwestern Amazonia: capabilities and vulnerabilities. Int. J. Disaster Risk Reductionr 1, 103529. doi: 10.1016/j.ijdrr.2023.103529
Pivello, V. R. (2011). The use of fire in the cerrado and Amazonian rainforests of Brazil: past and present. Fire Ecol. 7, 24–39. doi: 10.4996/fireecology.0701024
Quiroz-Claros, G., Vos, V. A., Arza, L. A. M., and Benitez, F. C. (2017). Castaña, Condiciones Laborales y Medio Ambiente. Available online at: https://cipca.org.bo/docs/publications/es/3_castana-condiciones-laborales-y-medio-ambiente-1.pdf (accessed on November 10, 2022).
RAISG-Amazon Network of Georeferenced Socio-Environmental Information (2022). Mapa de la Deforestación 2001-2020. Available online at: https://www.raisg.org/en/maps/ (accessed on October 21, 2022).
Ribeiro, A. F., Brando, P. M., Santos, L., Rattis, L., Hirschi, M., Hauser, M., et al. (2022). A compound event-oriented framework to tropical fire risk assessment in a changing climate. Environ. Res. Lett.17, 6,065015. doi: 10.1088/1748-9326/ac7342
Rocha, R., and Sant'anna, A. A. (2022). Winds of fire and smoke: air pollution and health in the Brazilian Amazon. World Dev. 151, 105722. doi: 10.1016/j.worlddev.2021.105722
Rozante, J. R., Moreira, D. S., de Goncalves, L. G. G., and Vila, D. A. (2010). Combining TRMM and surface observations of precipitation: technique and validation over South America. Weather Forecast 25, 885–894. doi: 10.1175/2010WAF2222325.1
Saatchi, S. S., Harris, N. L., Brown, S., Lefsky, M., Mitchard, E. T. A., Salas, W., et al. (2011). Benchmark map of forest carbon stocks in tropical regions across three continents. Proc. Natl. Acad. Sci. U.S.A. 108, 9899–9904. doi: 10.1073/pnas.1019576108
Silva Junior, C. H. L., Anderson, L. O., Silva, A. L., Almeida, C. T., Dalagnol, R., Pletsch, M. A. J. S., et al. (2019). Fire responses to the 2010 and 2015/2016 Amazonian droughts. Front. Earth Sci. 7, 97. doi: 10.3389/feart.2019.00097
Silva Júnior, R. O., Souza, E. B., Tavares, A. L., Mota, J. A., Ferreira, D. B. S., Souza-Filho, P. W. M., et al. (2017). Three decades of reference evapotranspiration estimates for a tropical watershed in the eastern Amazon. An. Acad. Bras. Cienc. 89, 1985–2002. doi: 10.1590/0001-3765201720170147
Silva, C. V. J., Aragão, L. E. O. C., Barlow, J., Espirito-Santo, F., Young, P. J., Anderson, L. O., et al. (2018). Drought-induced Amazonian wildfires instigate a decadal-scale disruption of forest carbon dynamics. Philos. Trans. R. Soc. B Biol. Sci. 373, 43. doi: 10.1098/rstb.2018.0043
Silva, S. S., Numata, I., Fearnside, P. M., Graça, P. M. L. A., Ferreira, E. J. L., Santos, E. A., et al. (2020). Impact of fires on an open bamboo forest in years of extreme drought in southwestern Amazonia. Reg. Environ. Change 20, 1–13. doi: 10.1007/s10113-020-01707-5
Silva-Junior, C. H. L., Buna, A. T. M., Bezerra, D. S., Costa, O. S., Santos, A. L., Basson, L. O. D., et al. (2022). Forest fragmentation and fires in the Eastern Brazilian Amazon–Maranhão State, Brazil. Fire 5, 77. doi: 10.3390/fire5030077
Silveira, M. V. F., Petri, C. A., Broggio, I. S., Chagas, G. O., Macul, M. S., Leite, C. C. S. S., et al. (2020). Drivers of fire anomalies in the Brazilian amazon: lessons learned from the 2019 fire crisis. Land 9, 516–540. doi: 10.3390/land9120516
Silveira, M. V. F., Silva-Junior, C. H. L., Anderson, L. O., and Aragão, L. E. O. C. (2022). Amazon fires in the 21st century: The year of 2020 in evidence. Global Ecol. Biogeogr. 31, 2026–2040. doi: 10.1111/geb.13577
Silvério, D. V., Oliveira, R. S., Flores, B. M., Brando, P. M., Almada, H. K., Furtado, M. T., et al. (2022). Intensification of fire regimes and forest loss in the Território Indígena do Xingu. Environ. Res. Lett. 17, 4,045012. doi: 10.1088/1748-9326/ac5713
Silvestrini, R. A., Soares-Filho, B. S., Nepstad, D., Coe, M., Rodrigues, H., and Assunção, R. (2011). Simulating fire regimes in the Amazon in response to climate change and deforestation. Ecol. Appl. 21, 1573–1590. doi: 10.1890/10-0827.1
Soille, P., and Vogt, P. (2009). Morphological segmentation of binary patterns. Pattern Recognit. Lett. 30, 456–459. doi: 10.1016/j.patrec.2008.10.015
Souza, C., Veríssimo, A., Costa, A., Salomão, R., Balieiro, C., and Ribeiro, J. (2006). Dinâmica do desmatamento no Estado do Acre (1988-2004). Belém: Imazon. Available online at: https://imazon.org.br/publicacoes/dinamica-do-desmatamento-no-estado-do-acre-1988-2004/ (accessed on November 10, 2022).
Souza, C. M., Shimbo, J. Z., Rosa, M. R., Parente, L. L., Alencar, A. A., Rudorff, B. F. T., et al. (2020). Reconstructing three decades of land use and land cover changes in brazilian biomes with landsat archive and earth engine. Remote Sens. 12, 2735. doi: 10.3390/rs12172735
UNDRR (2015). Sendai Framework for Disaster Risk Reduction 2015-2030. Available online at: https://www.undrr.org/publication/sendai-framework-disaster-risk-reduction-2015-2030 (accessed on November 18, 2022).
Vedovato, L. D., Fonseca, M. G., Arai, E., Anderson, L. O., and Aragão, L. E. O. C. (2016). The extent of 2014 forest fragmentation in the Brazilian Amazon. Reg. Environ. Chang. 16, 2485–2490. doi: 10.1007/s10113-016-1067-3
Villalobos, G. M. (2020). Las leyes incendiarias en Bolivia. Available online at: https://fundacionsolon.org/2020/02/20/las-leyes-incendiarias-en-bolivia/ (accessed on November 10, 2022).
Von Randow, C., Manzi, A. O., Kruijt, B., de Oliveira, P. J., Zanchi, F. B., Silva, R. L., et al. (2004). Comparative measurements and seasonal variations in energy and carbon exchange over forest and pasture in South West Amazonia. Theor. Appl. Climatol. 78, 5–26. doi: 10.1007/s00704-004-0041-z
Keywords: fire activity, fire drivers, deforestation, climate change, human-modified landscape, machine learning
Citation: Ferreira IJM, Campanharo WA, Barbosa MLF, Silva SSd, Selaya G, Aragão LEOC and Anderson LO (2023) Assessment of fire hazard in Southwestern Amazon. Front. For. Glob. Change 6:1107417. doi: 10.3389/ffgc.2023.1107417
Received: 24 November 2022; Accepted: 23 February 2023;
Published: 31 March 2023.
Edited by:
Celso H. L. Silva-Junior, University of California, Los Angeles, United StatesReviewed by:
Carlos Antonio Da Silva Junior, State University of Mato Grosso, BrazilKatia Fernandes, University of Arkansas, United States
Copyright © 2023 Ferreira, Campanharo, Barbosa, Silva, Selaya, Aragão and Anderson. This is an open-access article distributed under the terms of the Creative Commons Attribution License (CC BY). The use, distribution or reproduction in other forums is permitted, provided the original author(s) and the copyright owner(s) are credited and that the original publication in this journal is cited, in accordance with accepted academic practice. No use, distribution or reproduction is permitted which does not comply with these terms.
*Correspondence: Igor J. M. Ferreira, aWdvcl9tYWxmZXRvbmlAaG90bWFpbC5jb20=