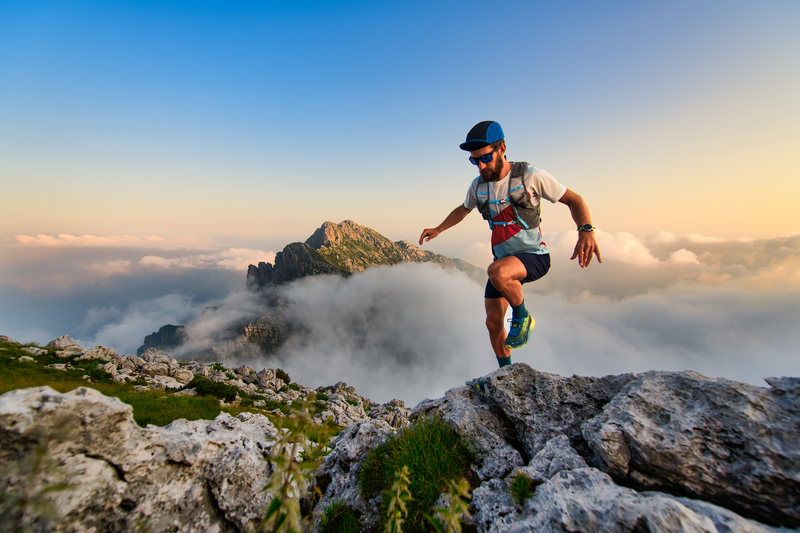
94% of researchers rate our articles as excellent or good
Learn more about the work of our research integrity team to safeguard the quality of each article we publish.
Find out more
ORIGINAL RESEARCH article
Front. For. Glob. Change , 10 February 2023
Sec. Fire and Forests
Volume 6 - 2023 | https://doi.org/10.3389/ffgc.2023.1071484
This article is part of the Research Topic Wildfire Management and Decision Support View all 7 articles
The use of fire simulation tools has become a regular feature of support systems for fuel management decisions at landscape level. Considering the spatial nature of fire in the evaluation of risk and the definition of fire mitigation goals is an ongoing research topic in forest management planning. By combining a fire simulation tool, a growth and yield simulator and an optimization module, it is possible to minimize the negative impact of fire over time and maximize the yield of various ecosystem services. Specific requirements for a fire simulator adapted to support tactical forest planning include a level of accuracy, the possibility of exploring diverse fire scenarios, the computational capability to simulate multiple fires and the flexibility to generate different outputs or metrics depending on the specific requirements of the planning problem under study. The present article addresses the requirements of fire simulators for their inclusion on forest tactical planning. The Cell2Fire simulator is adapted for use with fuel models more commonly employed in Europe and the United States, and to simulate the generation and spread of crown fires. Already able to solve static fire mitigation problems in its original version, this new adaptation, known as Cell2Fire_SB, has been developed with the more ambitious goal of being integrated into a decision support system that simultaneously considers fire behavior forest dynamics and allocation of management actions in order to solve temporal dynamic tactical forest problems.
Preventive management of wildfire risk through traditional fuel reduction management practices (Finney, 2004; Agee and Skinner, 2005) or fire-adapted forest management (González-Olabarria and Pukkala, 2011) involves assessing the probability of a fire anywhere within the study area, in order to determine the potential damage to values at risk and evaluate the costs and benefits of the preventive management systems under consideration (Calkin et al., 2014). Any such system should take into account the explicit spatial and temporal nature of fires since most of the components defining when, where and how large a fire becomes, including ignition point, fuel arrangement, topography and fire weather, will vary across time and space.
The choice of fuel management strategies for fire mitigation is not a straightforward issue and can be tackled at the operational, tactical or strategic planning levels. Operational planning should focus on defining how specific managerial operations can be implemented across a landscape to reduce fire spread and damage, without considering the evolution of fuels over time. Strategic planning aims to define management policies that take into account how fire regimes impact large areas of study over long periods of time. It is often the case, however, that the planning process does not consider the landscapes’ temporal evolution, concentrating rather on evaluating and setting general strategies and policies where defining specific management actions is not a goal. As for tactical planning, its role is to define management alternatives across medium-sized landscapes and over medium time spans.
The inclusion of fire risk through fire simulation at any of the three planning levels poses different challenges depending on their respective objectives and timeframes. Strategic planning relies on two different types of fire spread simulators, either fire succession models if the impact of time is to be addressed, or more accurate fire spread models if the temporal evolution of landscapes is not a factor. Landscape fire succession models used to assess forest dynamics under management regimes and disturbance regimes over long periods of time such as LANDIS (He and Mladenoff, 1999; Krofcheck et al., 2019), DRYADES (Mailly et al., 2000), SELES (Fall and Fall, 2001), or MEDFIRE (Duane et al., 2016) are often based on simplified fire spread rules and fuel approximations in order to reduce computational requirements stemming from the need to simulate multiple fires over an evolving landscape. Although, the accuracy and methodological approach of landscape fire succession models, may change, a common characteristic of these systems is their focus on understanding processes or test hypothesis (Scheller and Mladenoff, 2007) on how landscapes will evolve and their interaction with fire, identifying management as a driver rather than as a decision that requires to be optimized. More accurate fire spread models and fuel characteristics adapted to simulate multiple fire events can also be employed in strategic planning where the goal is to set management priorities and the values at risk on a static landscape. This is done, for example, in FSim (Thompson et al., 2013), FSpro (Thompson et al., 2016), FlamMap (Alcasena et al., 2018, 2019), and WildfireAnalyst (Gonzalez-Olabarria et al., 2019). The use of fire spread simulators in operational planning to evaluate which specific fuel management operations are to be implemented across a landscape is quite similar to its use in strategic planning on a static landscape, but in the former case the objective is to conduct the evaluations for the landscape at selected locations across it (Phillips et al., 2006). Typically, once priority areas in a landscape have been identified, the operational aspects of the planning process rely on non-spatial fire behavior models to determine the effectiveness of specific fuel treatments (Waldrop et al., 2010; Piqué and Domènech, 2018) at the stand level.
Tactical forest planning, when associated with fire mitigation goals or even if only using risk assessment to reduce uncertainty on goods and services yield, entails considerable challenges due to its intrinsic nature. At the landscape level, it aims at defining a schedule of management activities over space and time that is most likely to achieve a desired set of objectives. As regards fire assessment using spatially explicit fire simulations, tactical planning requires a simulation system that is able to produce risk information based on multiple fire events across the landscape over the planning period. The system would also have to be sensitive to changes in the landscape, due not only to the natural evolution of the forest ecosystems but also to management operations included in the management schedules to be evaluated (González-Olabarria and Pukkala, 2011). In the past, methods for integrating fire simulations into tactical forest planning relied on over-simplifications, either by confining itself to very simple forest landscapes (Konoshima et al., 2008), limiting the number of fires during the planning period (Bettinger, 2009; Kim et al., 2009) or creating a fire spread simulator based on very simple fire propagation rules (González-Olabarria and Pukkala, 2011). More recently, innovative approaches have been studied that add complexity to the problem, such as combining the fuel-related capacities of the Forest Vegetation Simulator (Reinhardt and Crookston, 2003) with proxies of the expected fire probability provided by FlamMap in order to optimize the allocation of fuel treatments across time and space (Chung et al., 2013). The latter work, however, analyzes the impact of fuel management across time and space on potential fire behavior and expected loss without considering other outcomes from forest management, and thus does not meet the requirements of tactical forest planning. Barreiro et al. (2021), on the other hand, use a fire hazard index generated from multiple fire simulations at the start of a planning period as the basis for evaluating and optimizing forest and fuel management across an evolving landscape. While this is a clear example of tactical forest planning incorporating fire risk considerations, it still uses fire simulations at the start of the planning period to provide the spatial component of fire risk, relying thereafter on stand-level indicators linked to forest growth to propagate fire hazard over time. Finally, Ager et al. (2020) presented the LSim, a fully operational linkage between the Forest Vegetation System (Reinhardt and Crookston, 2003) to FSim (Finney et al., 2011). The LSim system was used to simulate forest growth, forest management and forest fires over a 50 years period, prioritizing allocation of management operations according to multiple criteria, and in contrast to Chung et al. (2013) produced outputs not only related to fire related variables, but also on extracted timber volumes. In principle, it can be said that Ager et al. (2020) created an appropriate solution for integrating fire spread and impact simulations into forest management planning, although the use of standing volume proxies to maximize harvested volume, and the lack of goals related to for example economic performance of the forest plan, are shortcoming that should be considered in the future to obtain a complete tactical system.
An ideal fire simulation system that can serve as a basis for integrating fire risk and fire behavior features into tactical forest planning should have the following attributes: (1) incorporate knowledge and rules on the influence of all factors modifying fire behavior (characteristics and arrangement of surface and canopy fuels, weather and fuel moisture, topography); (2) be able to simulate one or multiple fires across a landscape and retrieve useful information on fire behavior, area burn probability, fire impact and any other metric considered relevant to planning problems that may arise; (3) integrate a landscape’s temporal and spatial evolution under different management scenarios; and (4) be able to assess the impact of forest management on fire risk over a planning period, and the combined impacts of management and fire risk on the achievement of a defined planning objective.
Currently, there do exist fire simulation systems that fulfill the first two requirements, generating relevant information for fuel management planning. Mention should be made of the family of fire simulation tools developed by the Missoula Fire Sciences Laboratory [FlamMap (Finney, 2006), FSIM (Finney et al., 2011), or FSPro (Noonan-Wright et al., 2011)], which have proven their usefulness in generating information needed to plan fuel management strategies. By linking them to a growth and yield simulator, such as FVS (Reinhardt and Crookston, 2003), the possibility of assessing the impact of forest management on dynamic landscapes has been solved, and new possibilities to optimize management schedules on time and space, according to defined objectives, is quite realistic possibility (Ager et al., 2020; Young et al., 2022).
Recently, a fire spread simulator based on the Canadian Forest Fire Behavior Prediction (FBP) Systems has been developed that is designed to support short term forest planning decisions (Pais et al., 2021a). Known as Cell2Fire, the new simulator overcomes certain limitations of the Prometheus spatial growth model (Tymstra et al., 2010), offering increased computational capacity, the ability to simulate multiple fire events, automatic generation of different metrics, maps of risk and fire propagation patterns. Another important feature from Cell2Fire is its smooth integration with decision-making algorithms. Cell2Fire is a system developed in C++, under the object-oriented programming paradigm, whose implementation allows each landscape cell to be built from a “cell class,” which implies, in computational terms, that each cell itself is an instance of such a class, structurally containing: “attributes” and “methods.” Among the most important methods included in the “cell class” for fuel management planning is the ability to change the state of a cell dynamically (e.g., when a management is assigned to a specific cell: firebreak allocation, fuel conversion, etc.). This feature makes Cell2Fire different from other dedicated systems for these purposes, because Cell2Fire does not need to incur the effort of modifying the input fuel layers to apply an action on a cell or portion of the landscape (Pais et al., 2021a). The capacity to simulate and evaluate different management actions across the landscape, allows to create an objective function that could be optimized using different kinds of algorithms ranging from Metaheuristics (Zuuring et al., 1995; Chung et al., 2013), Simulation-based Optimization (Rytwinski and Crowe, 2010), to more advanced techniques that have taken advantage these days such as Reinforcement Learning techniques Lauer et al. (2017).
Albeit developed having in mind its use for forest and fuel planning purposes, Cell2Fire has not yet being considered as a part of a multitemporal planning system that solves the difficulty of linking forest evolution under different management schedules to fuel conditions (Botequim et al., 2017; Parsons et al., 2018). Combining Cell2Fire to a forest growth and yield simulator able to predict the evolution of fuels across a landscape under different management policies or scenarios, and an optimization module that would explore combinations of management alternatives across time and space, can be the basis of a new decision system oriented to integrate fire into tactical forest planning (Figure 1). While there is a prototype of growth and yield simulator (Gr4Tree, unpublished) based on the same set of models used by González-Olabarria and Pukkala (2011), including new additional models and rules to generate variables required by traditional fire behavior models (Krsnik et al., 2020), these variables are not the currently applied by Cell2Fire. Being the growth and yield simulator based on empirical forest dynamic models, and linking functions, for the region of Catalonia (NE Spain), the adjustment of fuel models depending on forest state and evolution were set match Scott and Burgan (2005).
Figure 1. Conceptual idea for a DSS to solve landscape level tactical planning problems, integrating the fire growth and mapping system (Cell2Fire_SB), the forest growth and management simulator (Growth4tree) and an optimization/planning solver.
Therefore, a first step before developing a complete decision support system for tactical forest planning, as the conceptually proposed in Figure 1, should be to develop a fire spread simulator called Cell2Fire_SB based on Pais et al. (2021a). This simulator while providing all the advantages for fuel management planning associated to Cell2Fire, should be able to apply those rules for surface and canopy fire spread, that are commonly used in Europe and USA. By using these rules while simulating fire spread, and the input variables required to run them, we will ensure the future linkage between simulators proposed in Figure 1.
In order to simulate a fire, the proposed Cell2Fire_SB system requires information for each cell of a landscape on topography (slope steepness and aspect), weather conditions (wind speed and direction), fuel humidity scenarios, fuel information (fuel behavior models, and for forested areas also the canopy base height, the canopy bulk density, and crown canopy cover), plus one or multiple ignition points.
The system is able to simulate surface and crown fires across heterogeneous landscapes. It models surface fire behavior using a subset of the standard fire behavior models proposed by Scott and Burgan (2005). Following the two authors’ approach, we simplified the mathematical relationships between rate of spread, wind speed and fuel humidity content by defining four different fuel humidity content scenarios: D4 L4 for very high moisture content, D3 L3 for moderate moisture content, D2 L2 for low moisture content, and D1 L1for very low moisture content in both dead and living fuel components. Once the scenario is chosen, fire propagation speed and flame length are estimated for a wide range of wind speeds (0, 5, 10, 20, 30, 40, 50, 60 km/h) with BehavePlus (Andrews, 2014). The information generated by these simulations is then inputted as metadata to the Curve Fitting Toolbox in MATLAB r2022a, which calibrates the mathematical relationships using non-linear least squares (Marquardt, 1963). For head rate of spread (HROS), the relationship to be estimated is:
where the variable x is the wind speed (Km/h) and the parameters p1, p2, p3 depend on the fuel type in each of the climatic scenarios considered (see Supplementary Tables 1–4). Slope is assumed to be zero. Based on the estimated HROS, the rules in Alexander (1985) are applied to define the back ROS (BROS) using Eqs. (2), (3), and (4) below, and the ROS in any other direction using the elliptical fire growth model formulated by Eqs. (5) and (6). Thus,
where LB is the length-to-breadth ratio, HB the head-to-back ratio, x the wind speed (Km/h), ROS(ϕ) the rate of spread for angle ϕ, and a, b, c, and e the main parameters of the proposed ellipse. All rates are estimated in meters per minute. Each landscape cell has eight immediate neighbors known as adjacent cells, so a burning cell can potentially propagate a fire in eight directions (0, 45, 90, 135, 180, 225, 270, 315°). Fire is assumed to propagate between cells in a straight line from the center of a given cell to the center of an adjacent one [for more details, see Pais et al. (2021a)].
All previously estimated spread rates consider a humidity scenario and the effects of wind speed and direction for each fuel model through the choices of corresponding parameters in Eq. (1). The slope effect is captured by adding Eqs. (7), (8, from Julio et al., 1995), and (9) to adjust the spread rate between point i and each of its neighbors (indexed j), as follows:
where SLOPEij is the slope between adjacent cells i and j determined by their elevations ELEVi and ELEVj and the distance between their centers, SEij is the slope effect, and ROSij is the updated surface rate of spread between adjacent cells after taking the slope effect into account.
In similar fashion to the calculation of HROS, the outputs from the BehavePlus fire simulations are used to calibrate the flame length relationship (FL) in meters for a given fuel model and wind speed as given by:
where the factor q = (q1, q2, q3) depends on the fuel type and moisture scenario (see Supplementary Tables 5–8). Fireline intensity (IB) (kW/m) is also introduced to describe the rate of energy released per unit length of the fire front. IB and FL are related through the following equation (Alexander, 1982):
Based on the fireline intensity concept, Wagner (1977) developed one of the earliest and most widely accepted models to derive the conditions for the initiation of crown fires, which we have adopted in Cell2Fire_SB. The model assumes that the threshold for the transition to a crown fire [I0, Eq. (12)] depends on the trees’ canopy base height (CBH in meters) and the foliar moisture content (FMC in %) in the various fuel moisture scenarios. It is formulated as:
where the proposed FMC percentage values are 60, 80, 100, and 120 in the D1L1, D2L2, D3L3, and D4L4 scenarios, respectively. Although, the system also allows to set user defined moistures if necessary (i.e., simulate D1L1 with FMC = 90).
A set of rules was also added to the simulator to incorporate the possibility of fire reaching the canopies and spreading through them. The rules include a crown initiation criterion that assumes fire will ignite the canopies if IB ≥ I0 (Wagner, 1977). Under this rule, if fire reaches the canopies, a crown rate of spread (CROS) is estimated using Rothermel (1991) correlation, replacing the previously estimated surface ROS in the simulations for the period during which fire is spreading actively through the canopies. Thus,
where (ROSFM10)40% is the spread rate predicted by the surface fire model (FM10) due to Rothermel (1972) with a wind reduction factor of 0.4. However, an active crown fire is not assumed to maintain itself indefinitely so a rule based on Wagner (1977) and Alexander (1988) is applied to identify the conditions under which it will continue. According to the rule, a fire will spread through canopies as long as CROS multiplied by the canopy bulk density (CBD in kg/m3) equals or exceeds a value of 3.0, and scale down again to a surface fire only once the value falls below 3.0. The product CROS × CBD is the horizontal mass-flow rate of fuel into the flaming zone and the constant 3.0 is the critical value of this flow as defined in Alexander (1988).
In a simulation there are three sets of conditions under which the spread of a fire will terminate: (1) there is no forest fuel or the propagation front reaches the forest frontier; (2) the ROS along the main available axes falls below some empirical threshold (set at 0.1 m/min); (3) a burning cell does not have any available adjacent cells the fire can spread to. This last condition depends on the initial landscape conditions but also considers that during a single fire simulation, a previously burned cell changes its state to unavailable. The rules can be modified to fit the tool’s intended application, as for example by setting a maximum number of hours per fire event.
To explore potential improvements of the simulation system rules, we designed an automatic parameter calibration tool based on historical fire data. The tool enables the simulator to learn and save certain parameters of interest for later use that will improve its performance. It is built around a derivative-free optimization (DFO) technique set out below that solves an optimization problem in which the variables are the parameters to be calibrated and the objective function represents the error in the simulation relative to the fire it seeks to reproduce.
Let be a fire scar produced by the simulator with input parameters and F be an observed (i.e., real) scar. To find the best parameters such that the simulated and real scars are similar, we first formulate the following optimization problem:
where LU and UB are the lower and upper bounds, respectively.
The tool is used to calibrate parameters related to crown fire propagation in order to improve the estimates of CROS [Eq. (13)]. For this purpose, we added the ability to evaluate and parametrise the influence of canopy cover fraction (CCF) and crown bulk density (CBD) on the active rate of spread while a fire is considered to be an active crown fire. This is done by modifying the initial values of the coefficients (αCCF = 0, and αCBD = 0) until the difference between the simulated fire perimeter and historical fires is minimized, as is shown in Figure 2.
Figure 2. Automatic adjustment of simulation parameters by comparing the outputs of simulations and real fire instances.
Following the methodology proposed by Carrasco et al. (2019), Eq. (14) is minimized to calibrate the parameters using the BOBYQA (bound optimization by quadratic approximation) algorithm developed by Powell (2009), which seeks the least value of a non-linear objective function subject to bound constraints without differentiating it. The fire scar Sk (see Figure 3) depends on the parameters and obtained in the k-th iteration. At the (k+1)-th iteration, the parameters are modified by in order to reduce the error :
Figure 3. Valbona 2016 fire analysis. The historical fire scar (left) and the adjusted simulation after 100 iterations of the DFO module (right) are compared. Each color represents a different fuel type, while the brown color was reserved to represent the cells burned by the respective (simulated) fires.
where . The algorithm stops when it reaches a maximum number of iterations k = N or the error falls to a tolerance level () predefined by the user.
To apply this methodology, we selected five fires of medium to large size for which all initial conditions and its evolution were known (ignition allocation and time, fire-weather conditions, final fire perimeter, and stopping time). Another key criterion for the selection was the date of fire occurrence, which had to post-date the landscape data on the arrangement of surface and canopy fuels given in Krsnik et al. (2020). The fires were named by combining the municipality and year of occurrence; thus, the five were identified as La Jonquera 2012, Naut Aran 2017, Odena 2015, Valbona 2016, and Vilopriu 2013.
The application of the methodology identified impacts of canopy cover fraction (CCF) and crown bulk density (CBD) in three of the studied fires (Table 1). The improvement regarding final fire perimeter was especially clear in the case of the Valbona fire (Figure 3). The newly found optimal parameters were recorded and stored for future simulations and easy use as starting points for further fine-tuning.
Table 1. Coefficients reflecting the impact of canopy characteristics on the spread of crown fires, for each historical fire.
The usability of the proposed simulator and its role as a tool to support forest management decision-making depend on its ability to generate meaningful outputs that can be given a definite interpretation and integrated into a forest management planning problem. As a single-event simulator, Cell2Fire_SB can reproduce the final shape of a fire, identifying which cells were affected by it and which ones escaped unburned. It can also determine other fire behavior variables embedded in it that may be useful in evaluating the virulence of a fire such as its rate of spread, fire propagation trees (Figure 4), and whether it has spread along the surface or through crowns. This information can be used not only to optimize suppression activities (Minas et al., 2015) but also to implement medium-to-long term adaptive planning based on a rolling horizon simulation (Feng et al., 2010) by linking it with a growth and yield simulator and a fire damage model such as the one due to González et al. (2007).
Figure 4. Representation of a simulated fire. The propagation tree shows the fire contagion patterns or the paths followed by fire as it spreads across the landscape from a burning cell to its adjacent neighbors.
The results of multiple fire simulators across a landscape can provide useful metrics for forest plans aimed at mitigating the impact of fires even before they occur (Figure 5). Two such metrics are fire occurrence probability and expected burned area, both required for estimating potential losses and the effectiveness of mitigation strategies (González-Olabarria and Pukkala, 2011). Other, more novel metrics generated by Cell2Fire_SB can assist in defining specific policies to mitigate fire impacts by identifying which landscape cells are most prone to acting as fire corridors. As described in Pais et al. (2021a), the Cell2Fire simulator records the shortest propagation paths during simulation time thanks to its ability to generate propagation graphs known as fire propagation trees in which the full spread dynamic is represented including the ROS, traveling times and other relevant information associated with the cells involved in a fire. The union of these individual graphs produces a multi-digraph (or a global propagation tree) containing all the fire dynamics information generated by multiple stochastic simulations. This information can be used to facilitate the localization of fuel management plans for mitigating future fire losses. Pais et al. (2021b) introduces a prioritization metric called downstream protection value and finds that its effectiveness for firebreak location is superior to measures based on probability of occurrence (Pais et al., 2021b), betweenness centrality (Gray and Dickson, 2016) or fire protection value (Palma et al., 2007). The metric measures the value of a cell based on a characteristic or value-at-risk provided by the decision-maker such as the total volume of timber or the number of cells that can be protected by treating a certain section of the forest with the aim of disrupting the fire propagation paths. Decision-makers can thus easily identify the most critical sections of the forest in terms of expected losses aligned with their specific value function, information which in turn supports their resource allocation process and landscape management decisions.
Figure 5. Examples of metrics that can be obtained from 1,000 stochastic simulations with various ignition points and weather scenarios. (A) Burn probability; (B) downstream protection value; (C) betweenness centrality; (D) global propagation tree.
The Cell2Fire_SB system is capable alone of evaluating an array of different fire behavior and fire risk metrics used in forest and landscape planning, whether for one or multiple fire events. Its full potential, however, will be reached once it has been effectively linked to a growth and yield simulation system that can predict the evolution of the forest and the factors influencing fire behavior (fuel models, canopy structure). There already exists a growth and yield simulator prototype known as Growth4Tree based on individual tree models that produces outputs on the evolution of multiple stands under different management schedules and estimates the yield of multiple ecosystem services (see Figure 1). In addition to the aforementioned traditional features based on the size and composition of trees in a stand, Growth4Tree can estimate certain input variables required to run the fire simulator such as bush coverage, canopy characteristics and a standard fuel model. The assignment of the Scott and Burgan fuel models based on forest characteristics follows the rules given in Krsnik et al. (2020).
When multiple fire simulations are run for a given landscape, ideally based on an adequate number and well-chosen distribution of fire ignitions and climate (wind and humidity) scenarios defined by the historical or expected fire regime and readjusted to fit the duration of the study period, the simulator will generate useful information. For example, it is able to produce metrics on the most common fire paths and fire behavior variables distributions, which can then be used to identify critical locations where stand management would have the greatest impact in disrupting fire spread (Finney and Cohen, 1998), thus improving the economic effectiveness of fire prevention measures (Loehle, 2004). Like other simulators, ours is capable of generating fire probability maps, one of the most useful types of information for forest planning purposes given that the probability of disturbance occurrence when combined with a damage model provides an estimate of expected losses. At present, the damage model applied by the system is a stand-and-tree level mortality model developed by González et al. (2007), which is adjusted for tactical planning purposes (González-Olabarria et al., 2017) depending basically on stand characteristics prior to a fire and is independent of fire virulence. Since the simulation system produces a distribution of fire behavior variables (intensity, rate of spread, flame height) for each simulation cell, it will be possible in the near future to approximate fire severity and modify the existing damage model, especially for areas where crown fires are recurrent, by incorporating some of the principles of traditional mortality models [e.g., Ryan and Reinhardt (1988) and Fernandes et al. (2008)]. Applying the impact of fire on tree mortality, will allow to identify the impact of fire on timber production (Ager et al., 2020; Young et al., 2022), and on other ecosystem services depending on the allocation and structure of the stands.
The proposed tactical planning system (see Figure 1) still is in a conceptual phase of development, with the linkage between the presented fire spread simulator and the growth and yield simulator being currently implemented, but the decision space format defined by the outputs of the combined simulations not being explored, keeping at hold the design of the optimization module. Still, once completed, the system is expected to provide a robust platform to support decisions on designing more resistant and resilient forested landscapes, considering not only fire mitigation objectives but also the provision of multiple ecosystem services.
There are a number of other possibilities for improving the reliability of the simulator’s results in terms of a better adjustment to the reality of fire spread. One of these is understanding and predicting fuel accumulation over long periods in heterogeneous landscapes. This is not an easy task, however (He and Mladenoff, 1999), and together with uncertainty regarding future weather and fire initiation conditions has traditionally prevented the use of fire spread simulators in long-term forest planning (Martell, 2001). The availability of new remote sensing tools such as airborne LiDAR has enabled the mapping of fuel over space, but even though there is ongoing progress in this regard (González-Olabarria et al., 2012; Gonzalez-Olabarria et al., 2019), it still has to rely on adjustment by fire experts to represent reality more accurately and avoid the sort of over-simplification produced by statistical models (Krsnik et al., 2020). Therefore, even if current fuel adjustment methods are adequate for tactical planning problems, any future improvements in modeling of fuel accumulation and its relation to forest evolution and management should be incorporated into the forest growth and fire simulation system.
The application of machine learning tools to adjust the fire behavior rules so that they better match specific conditions based on how multiple historic fires have spread in the past is another source of improvements that has proved to be a flexible approach. In particular, the tools can be used to modify well-established rules so that they coincide more closely with the reality of fire spread across complex landscapes. Our use of five fires with well-known ignition points, duration times, final fire perimeters, and fuel and fire-weather conditions is sufficient for an initial rule adjustment, but does not bring out the full potential of these techniques to better understand fire behavior. For example, the importance of crown fires cannot be overlooked in terms of their impact on forest and non-forest goods and services, or on the fire suppression capacities of firefighters. Therefore, an improvement on existing crown fire rules is imperative. Existing empirical models are often based on limited observations and rather uniform forests in terms of structure and composition (Hoffman et al., 2016) and thus are not well suited to more complex forest lands. Using another set of fires with higher variability forest conditions will improve the robustness of the DFO adjustments proposed here, and if those historic fires have additional information on fire isochrones and when a fire started or stopped being a crown fire, the automatic learning process may be able to produce rules on propagation of active crown fires that are far more complex and realistic than the currently existing ones. Furthermore, new machine learning tools may be the way to address the challenge of deepening our understanding of the factors behind the occurrence and behavior of megafires (Tedim et al., 2018). Finally, not yet considered in the simulator are processes such as the effectiveness of fire suppression efforts (Plucinski, 2019) and the capacity of a fire to jump over fuel barriers though spotting (Plana et al., 2005). Including them in the system will improve its effectiveness and should therefore be a priority for future work. If the necessary data and models are not available, expert knowledge from forest firefighters should be exploited to create credible approximations.
Publicly available datasets were analyzed in this study. Data on fuel and weather information used in this study can be found at https://previncat.ctfc.cat/. The fire simulation system can be found at https://github.com/fire2a/C2FSB.
JG-O wrote the manuscript and participated in the selection of fire spreading rules. JC, CP, AW, DP-M, and RM-R worked on the definition and formulation of the relationships between the spread rules and wrote the first version of the fire spread simulation software. JG-G, OP, and JG-O were responsible for defining and evaluating rules for the growth and yield simulation software. All authors contributed to the conception and design of the study, and to the editing and revising of the manuscript edition and the final approval of the submitted version.
This project received funding from the European Union’s Horizon 2020 Research and Innovation Programme under grant agreements Nos. 101037419–FIRE-RES and 101007950–DecisioES. National funding was also provided by the Spanish Ministry of Science and Innovation (PID2020-120355RB-I00). AW, CP, and JC acknowledge the support of the Agencia Nacional de Investigación y Desarrollo (ANID), Chile under project FONDEF ID20I10137. JC acknowledges the support of ANID, Chile under funding from Postdoctoral Fondecyt project No. 3210311. AW acknowledges the support of ANID, Chile under project PIA/APOYO AFB 180003. JG-O acknowledges the receipt of a fellowship from the OECD Co-operative Research Programme: Sustainable Agricultural and Food Systems 2020.
We would like to acknowledge the assistance of Eduard Busquets of the CTFC and the Bombers-GRAF, Fire Department, Government of Catalonia for providing the historical fires data used to test the machine learning adjustments.
The authors declare that the research was conducted in the absence of any commercial or financial relationships that could be construed as a potential conflict of interest.
All claims expressed in this article are solely those of the authors and do not necessarily represent those of their affiliated organizations, or those of the publisher, the editors and the reviewers. Any product that may be evaluated in this article, or claim that may be made by its manufacturer, is not guaranteed or endorsed by the publisher.
The Supplementary Material for this article can be found online at: https://www.frontiersin.org/articles/10.3389/ffgc.2023.1071484/full#supplementary-material
Agee, J. K., and Skinner, C. N. (2005). Basic principles of forest fuel reduction treatments. For. Ecol. Manag. 211, 83–96. doi: 10.1016/j.foreco.2005.01.034
Ager, A. A., Barros, A. M., Houtman, R., Seli, R., and Day, M. A. (2020). Modelling the effect of accelerated forest management on long-term wildfire activity. Ecol. Model. 421:108962. doi: 10.1016/j.ecolmodel.2020.108962
Alcasena, F. J., Ager, A. A., Bailey, J. D., Pineda, N., and Vega-García, C. (2019). Towards a comprehensive wildfire management strategy for Mediterranean areas: Framework development and implementation in Catalonia, Spain. J. Environ. Manag. 231, 303–320. doi: 10.1016/j.jenvman.2018.10.027
Alcasena, F. J., Ager, A. A., Salis, M., Day, M. A., and Vega-Garcia, C. (2018). Optimizing prescribed fire allocation for managing fire risk in central Catalonia. Sci. Total Environ. 621, 872–885. doi: 10.1016/j.scitotenv.2017.11.297
Alexander, M. E. (1982). Fire behaviour in aspen slash fuels .as related to the Canadian fire weather index. Can. J. For. Res. 12, 1028–1029. doi: 10.1139/x82-150
Alexander, M. E. (1985). “Estimating the length-to-breadth ratio of elliptical forest fire patterns,” in Proceedings of the 8th Conference on FIRE and Forest Meteorology, (Bethesda, MD: Society of American Foresters), 287–304.
Alexander, M. E. (1988). “Help with making crown fire hazard assessments,” in Protecting People and Homes from Wildfire in the Interior West, eds W. C. Fischer and S. F. Arno (Ogden, GA: USDA Forest Service).
Andrews, P. L. (2014). Current status and future needs of the BehavePlus Fire Modeling System. Int. J. Wildland Fire 23, 21–33. doi: 10.1071/WF12167
Barreiro, S., Benali, A., Rua, J. C., Tomé, M., Santos, J. L., and Pereira, J. M. (2021). Combining landscape fire simulations with stand-level growth simulations to assist landowners in building wildfire-resilient landscapes. Forests 12:1498. doi: 10.3390/f12111498
Bettinger, P. (2009). A prototype method for integrating spatially-referenced wildfires into a tactical forest planning model. Res. J. For. 3, 8–22. doi: 10.3923/rjf.2009.8.22
Botequim, B., Fernandes, P. M., Garcia-Gonzalo, J., Silva, A., and Borges, J. G. (2017). Coupling fire behaviour modelling and stand characteristics to assess and mitigate fire hazard in a maritime pine landscape in Portugal. Eur. J. For. Res. 136, 527–542. doi: 10.1007/s10342-017-1050-7
Calkin, D. E., Cohen, J. D., Finney, M. A., and Thompson, M. P. (2014). How risk management can prevent future wildfire disasters in the wildland-urban interface. Proc. Natl. Acad. Sci. U.S.A. 111, 746–751. doi: 10.1073/pnas.1315088111
Carrasco, J., Pais, C., Shen, Z. J. M., and Weintraub, A. (2019). Adjusting rate of spread factors through derivative-free optimization: A new methodology to improve the performance of forest fire simulators. arXiv [Preprint]. doi: 10.48550/arXiv.1909.05949
Chung, W., Jones, G., Krueger, K., Bramel, J., and Contreras, M. (2013). Optimising fuel treatments over time and space. Int. J. Wildland Fire 22, 1118–1133. doi: 10.1071/WF12138
Duane, A., Aquilué, N., Gil-Tena, A., and Brotons, L. (2016). Integrating fire spread patterns in fire modelling at landscape scale. Environ. Model. Softw. 86, 219–231. doi: 10.1016/j.envsoft.2016.10.001
Fall, A., and Fall, J. (2001). A domain-specific language for models of landscape dynamics. Ecol. Model. 141, 1–18. doi: 10.1016/S0304-3800(01)00334-9
Feng, Y., D’Amours, S., and Beauregard, R. (2010). Simulation and performance evaluation of partially and fully integrated sales and operations planning. Int. J. Prod. Res. 48, 5859–5883. doi: 10.1080/00207540903232789
Fernandes, P. M., Vega, J. A., Jimenez, E., and Rigolot, E. (2008). Fire resistance of European pines. For. Ecol. Manag. 256, 246–255. doi: 10.1016/j.foreco.2008.04.032
Finney, M. A. (2004). Landscape fire Simulation and Fuel Treatment Optimization. Methods for Integrating Modeling Of Landscape Change: Interior Northwest Landscape Analysis System. General Technical Report PNW-GTR-610. Portland, OR: US Department of Agriculture, Forest Service, Pacific Northwest Research Station, 117–131.
Finney, M. A. (2006). “An overview of FlamMap fire modeling capabilities,” in Fuels Management-How To Measure Success. Proceedings RMRS-P-41, eds P. L. Andrews and B. W. Butler (Portland, OR: USDA Forest Service).
Finney, M. A., and Cohen, J. D. (1998). “Expectation and evaluation of fuel management objectives,” in Proceedings of the Fire, Fuel Treatments, and Ecological Restoration, (Fort Collins, CO: USDA Forest Service, Rocky Mountain Research Station), 353–366.
Finney, M. A., McHugh, C. W., Grenfell, I. C., Riley, K. L., and Short, K. C. (2011). A simulation of probabilistic wildfire risk components for the continental United States. Stoch. Environ. Res. Risk Assess. 25, 973–1000. doi: 10.1007/s00477-011-0462-z
González, J. R., Trasobares, A., Palahi, M., and Pukkala, T. (2007). Predicting stand damage and tree survival in burned forests in Catalonia (North-East Spain). Ann. For. Sci. 64, 733–742. doi: 10.1051/forest:2007053
González-Olabarria, J. R., and Pukkala, T. (2011). Integrating fire risk considerations in landscape-level forest planning. For. Ecol. Manag. 261, 278–287. doi: 10.1016/j.foreco.2010.10.017
González-Olabarria, J. R., Garcia-Gonzalo, J., Mola-Yudego, B., and Pukkala, T. (2017). Adaptive management rules for Pinus nigra Arnold ssp. salzmannii stands under risk of fire. Ann. For. Sci. 74:52. doi: 10.1007/s13595-017-0649-7
Gonzalez-Olabarria, J. R., Reynolds, K. M., Larrañaga, A., Garcia-Gonzalo, J., Busquets, E., and Piqué, M. (2019). Strategic and tactical planning to improve suppression efforts against large forest fires in the Catalonia region of Spain. For. Ecol. Manag. 432, 612–622. doi: 10.1016/j.foreco.2018.09.039
González-Olabarria, J. R., Rodríguez, F., Fernández-Landa, A., and Mola-Yudego, B. (2012). Mapping fire risk in the Model Forest of Urbión (Spain) based on airborne LiDAR measurements. For. Ecol. Manag. 282, 149–156. doi: 10.1016/j.foreco.2012.06.056
Gray, M. E., and Dickson, B. G. (2016). Applying fire connectivity and centrality measures to mitigate the cheatgrass-fire cycle in the arid West, USA. Landsc.Ecol. 31, 1681–1696. doi: 10.1007/s10980-016-0353-2
He, H. S., and Mladenoff, D. J. (1999). Spatially explicit and stochastic simulation of forest-landscape fire disturbance and succession. Ecology 80, 81–99. doi: 10.1890/0012-9658
Hoffman, C. M., Canfield, J., Linn, R. R., Mell, W., Sieg, C. H., Pimont, F., et al. (2016). Evaluating crown fire rate of spread predictions from physics-based models. Fire Technol. 52, 221–237. doi: 10.1007/s10694-015-0500-3
Julio, G., Pedernera, P., and Castillo, E. (1995). Diseño Funcional del Simulador de incendios forestales, en Actas del Taller Internacional sobre Prognosis y Gestión en Control de Incendios Forestales. Santiago de Chile: Ministerio de Agricultura, 182–204.
Kim, Y. H., Bettinger, P., and Finney, M. (2009). Spatial optimization of the pattern of fuel management activities and subsequent effects on simulated wildfires. Eur. J. Oper. Res. 197, 253–265. doi: 10.1016/j.ejor.2008.05.025
Konoshima, M., Montgomery, C. A., Albers, H. J., and Arthur, J. L. (2008). Spatial-endogenous fire risk and efficient fuel management and timber harvest. Landsc. Econ. 84, 449–468. doi: 10.3368/le.84.3.449
Krofcheck, D. J., Remy, C. C., Keyser, A. R., and Hurteau, M. D. (2019). Optimizing forest management stabilizes carbon under projected climate and wildfires. J. Geophys. Res. Biogeosci. 124, 3075–3087. doi: 10.1029/2019JG005206)
Krsnik, G., Busquets Olivé, E., Piqué Nicolau, M., Larrañaga, A., Cardil, A., García-Gonzalo, J., et al. (2020). Regional level data server for fire hazard evaluation and fuel treatments planning. Remote Sens. 12:4124. doi: 10.3390/rs12244124
Lauer, C. J., Montgomery, C. A., and Dietterich, T. G. (2017). Spatial interactions and optimal forest management on a fire-threatened landscape. For. Policy Econ. 83, 107–120. doi: 10.1016/j.forpol.2017.07.006
Loehle, C. (2004). Applying landscape principles to fire hazard reduction. For. Ecol. Manag. 198, 261–267. doi: 10.1016/j.foreco.2004.04.010
Mailly, D., Kimmins, J. P., and Busing, R. T. (2000). Disturbance and succession in a coniferous forest of northwestern North America: Simulations with DRYADES, a spatial gap model. Ecol. Model. 127, 183–205. doi: 10.1016/S0304-3800(99)00208-2
Marquardt, D. W. (1963). An algorithm for least-squares estimation of nonlinear parameters. J. Soc. Indust. Appl. Math. 11, 431–441. doi: 10.1137/0111030
Martell, D. L. (2001). “Forest fire management,” in ForestFires: Behaviour and Ecological Effects, eds E. A. Johnson and K. Miyanishi (New York, NY: Academic Press), 527–583.
Minas, J., Hearne, J., and Martell, D. (2015). An integrated optimization model for fuel management and fire suppression preparedness planning. Ann. Oper. Res. 232, 201–215. doi: 10.1007/s10479-012-1298-8
Noonan-Wright, E. K., Opperman, T. S., Finney, M. A., Zimmerman, G. T., Seli, R. C., Elenz, L. M., et al. (2011). Developing the US wildland fire decision support system. J. Combust. 168473:14. doi: 10.1155/2011/168473
Pais, C., Carrasco, J., Martell, D. L., Weintraub, A., and Woodruff, D. L. (2021a). Cell2Fire: A cell-based forest fire growth model to support strategic landscape management planning. Front. For. Glob. Change 4:692706. doi: 10.3389/ffgc.2021.692706
Pais, C., Carrasco, J., Moudio, P. E., and Shen, Z. J. M. (2021b). Downstream protection value: Detecting critical zones for effective fuel-treatment under wildfire risk. Comput. Oper. Res. 131:105252. doi: 10.1016/j.cor.2021.105252
Palma, C. D., Cui, W., Martell, D. L., Robak, D., and Weintraub, A. (2007). Assessing the impact of stand-level harvests on the flammability of forest landscapes. Int. J. Wildland Fire 16, 584–592. doi: 10.1071/WF06116
Parsons, R. A., Pimont, F., Wells, L., Cohn, G., Jolly, W. M., de Coligny, F., et al. (2018). Modeling thinning effects on fire behaviour with STANDFIRE. Ann. For. Sci. 75:7. doi: 10.1007/s13595-018-0696-8
Phillips, R. J., Waldrop, T. A., and Simon, D. M. (2006). “Assessment of the FARSITE model for predicting fire behaviour in the southern Appalachian Mountains”, in Proceedings of the 13th Biennial Southern Silvicultural Research Conference. Gen. Tech. Rep. SRS-92, (Asheville, NC: US Department of Agriculture, Forest Service, Southern Research Station), 521–525.
Piqué, M., and Domènech, R. (2018). Effectiveness of mechanical thinning and prescribed burning on fire behaviour in Pinus nigra forests in NE Spain. Sci. Total Environ. 618, 1539–1546. doi: 10.1016/j.scitotenv.2017.09.316
Plana, E., Cerdan, R., and Castellnou, M. (2005). “Developing firebreaks,” in Forest Restoration in Landscapes, eds S. Mansourian, D. Valluri, and N. Dudley (New York, NY: Springer), 269–273.
Plucinski, M. P. (2019). Contain and control: Wildfire suppression effectiveness at incidents and across landscapes. Curr. For. Rep. 5, 20–40. doi: 10.1007/s40725-019-00085-4
Powell, M. J. (2009). The BOBYQA Algorithm for Bound Constrained Optimization without Derivatives. Cambridge NA Report NA2009/06. Cambridge: University of Cambridge, 26–46.
Reinhardt, E., and Crookston, N. L. (2003). The Fire and Fuels Extension to the Forest Vegetation Simulator. Gen. Tech. Rep. RMRS-GTR-116. Ogden, UT: U.S. Department of Agriculture, Forest Service, Rocky Mountain Research Station, 209.
Rothermel, R. C. (1972). A Mathematical Model for Predicting Fire Spread in Wildland Fuels. Res. Pap. INT-115. Ogden, UT: U.S. Department of Agriculture, Intermountain Forest and Range Experiment Station, 40.
Rothermel, R. C. (1991). Predicting Behaviour and Size Of Crown Fires in the Northern Rocky Mountains. Res. Pap. INT-RP-438. Ogden, UT: U.S. Department of Agriculture, Forest Service, Intermountain Research Station, 46.
Ryan, K. C., and Reinhardt, E. D. (1988). Predicting postfire mortality of seven western conifers. Can. For. Res. 18, 1291–1297. doi: 10.1139/x88-199
Rytwinski, A., and Crowe, K. A. (2010). A simulation-optimization model for selecting the location of fuel-breaks to minimize expected losses from forest fires. For. Ecol. Manage. 260, 1–11. doi: 10.1016/j.foreco.2010.03.013
Scheller, R. M., and Mladenoff, D. J. (2007). An ecological classification of forest landscape simulation models: Tools and strategies for understanding broad-scale forested ecosystems. Landsc. Ecol. 22, 491–505. doi: 10.1007/s10980-006-9048-4
Scott, J. H., and Burgan, R. E. (2005). Standard Fire Behaviour Fuel Models: A Comprehensive Set for use with Rothermel’s surface Fire Spread Model. Gen. Tech. Rep. RMRS-GTR-153. Fort Collins, CO: U.S. Department of Agriculture, 72.
Tedim, F., Leone, V., Amraoui, M., Bouillon, C., Coughlan, M., Delogu, G., et al. (2018). Defining extreme wildfire events: Difficulties, challenges, and impacts. Fire 1:9. doi: 10.3390/fire1010009
Thompson, M. P., Scott, J., Helmbrecht, D., and Calkin, D. E. (2013). Integrated wildfire risk assessment: Framework development and application on the Lewis and Clark National Forest in Montana, USA. Integr. Environ. Assess. Manag. 9, 329–342. doi: 10.1002/ieam.1365
Thompson, M., Calkin, D., Scott, J. H., and Hand, M. (2016). “Uncertainty and probability in wildfire management decision support: an example from the United States,” in Natural Hazard Uncertainty Assessment: Modeling and Decision Support, Geophysical Monograph 223 (First Edition), eds K. Riley, P. Webley, and M. Thompson (Washington, DC: American Geophysical Union), 31–41. doi: 10.1002/9781119028116.ch4
Tymstra, C., Bryce, R. W., Wotton, B. M., Taylor, S. W., and Armitage, O. B. (2010). Development and Structure of Prometheus: The Canadian Wildland Fire Growth Simulation Model. Information Report NOR-X-417. Edmonton, AB: Canadian Forest Service, 102.
Wagner, C. V. (1977). Conditions for the start and spread of crown fire. Can. J. For. Res. 7, 23–34. doi: 10.1139/x77-004
Waldrop, T., Phillips, R. A., and Simon, D. A. (2010). Fuels and predicted fire behaviour in the southern Appalachian Mountains after fire and fire surrogate treatments. For. Sci. 56, 32–45. doi: 10.1093/forestscience/56.1.32
Young, J. D., Ager, A. A., and Thode, A. E. (2022). Using wildfire as a management strategy to restore resiliency to ponderosa pine forests in the southwestern United States. Ecosphere 13:e4040. doi: 10.1002/ecs2.4040
Keywords: fire simulator, tactical forest planning, derivative-free optimization, fire growth simulation, wildfire
Citation: Gonzalez-Olabarria JR, Carrasco J, Pais C, Garcia-Gonzalo J, Palacios-Meneses D, Mahaluf-Recasens R, Porkhum O and Weintraub A (2023) A fire spread simulator to support tactical management decisions for Mediterranean landscapes. Front. For. Glob. Change 6:1071484. doi: 10.3389/ffgc.2023.1071484
Received: 16 October 2022; Accepted: 24 January 2023;
Published: 10 February 2023.
Edited by:
Chad M. Hoffman, Colorado State University, United StatesReviewed by:
Nicholas Adam Povak, Pacific Southwest Research Station, Forest Service (USDA), United StatesCopyright © 2023 Gonzalez-Olabarria, Carrasco, Pais, Garcia-Gonzalo, Palacios-Meneses, Mahaluf-Recasens, Porkhum and Weintraub. This is an open-access article distributed under the terms of the Creative Commons Attribution License (CC BY). The use, distribution or reproduction in other forums is permitted, provided the original author(s) and the copyright owner(s) are credited and that the original publication in this journal is cited, in accordance with accepted academic practice. No use, distribution or reproduction is permitted which does not comply with these terms.
*Correspondence: Jose Ramon Gonzalez-Olabarria, anIuZ29uemFsZXpAY3RmYy5jYXQ=
Disclaimer: All claims expressed in this article are solely those of the authors and do not necessarily represent those of their affiliated organizations, or those of the publisher, the editors and the reviewers. Any product that may be evaluated in this article or claim that may be made by its manufacturer is not guaranteed or endorsed by the publisher.
Research integrity at Frontiers
Learn more about the work of our research integrity team to safeguard the quality of each article we publish.