- 1Department of Ecology, Evolution, and Marine Biology, University of California, Santa Barbara, Santa Barbara, CA, United States
- 2Environmental Studies Department, University of California, Santa Barbara, Santa Barbara, CA, United States
- 3Bren School of Environmental Science and Management, University of California, Santa Barbara, Santa Barbara, CA, United States
- 4University of California Cooperative Extension, Oakland, CA, United States
Big-cone Douglas-Fir (Pseudotsuga macrocarpa, hereafter BCDF) is an endemic, fire-adapted conifer found throughout the mountains of southern California. Because recent large high intensity wildfires have resulted in loss of BCDF, understanding how environmental factors, such as topography, fuels, climate, and weather, impact BCDF survivorship is important for informing restoration and conservation efforts. Here, we used randomForest (RF) and accumulated local effects (ALE) plots to examine how environmental variables contribute to the occurrence of both fire refugia and high fire-induced mortality of BCDF stands during two large wildfires. Additionally, we explored how the influence of these variables changed between the use of two different response variables: (1) visually-assessed mortality evaluated through estimation of canopy survival using Google Earth imagery and (2) RdNBR. This comparison allows us to evaluate the potential that RdNBR overestimates BCDF mortality because it is highly indicative of understory conditions post-fire, rather than direct changes to BCDF trees. We found that pre-fire fuel was one of the most influential variables contributing to both fire refugia and high mortality; sparse and oak dominant understories contributed to fire refugia, while chaparral contributed to high mortality. We also found that the role of certain variables was not consistent across the two fires. For example, areas of the landscape with hotter temperature and higher vapor pressure deficit (VPD) during the fire experienced high BCDF mortality in the Zaca Fire, but had the inverse effect in the Thomas Fire. Lastly, we found that our two metrics of response resulted in significantly different classification of BCDF stands: RdNBR resulted in more stands being classified as high intensity and fewer low severity/unburned areas, supporting our concern that it can overestimate high severity impact in some ecosystems. However, the two model types resulted in relatively similar explanatory environmental variable selections, although different rankings.
Introduction
Fire plays an important role in shaping species distributions (Zackrisson, 1977; Smith, 2000; Bond and Keeley, 2005; Kelly et al., 2017). Two prominent vegetation types in California, conifer forests and chaparral shrublands, have been particularly affected by fire regimes altered by anthropogenic factors. Forest fires have become larger and burn more land at higher severity than historic norms (Nigro and Molinari, 2019), likely a result of a mixture of climate change (Abatzoglou and Williams, 2016) and fire suppression (Steel et al., 2015). This will impact future species composition as many coniferous species are limited by the distance to a seed source to regenerate (Donato et al., 2009; Welch et al., 2016). Population density and human ignitions have increased fire frequency in chaparral (Syphard et al., 2007; Safford and Van de Water, 2014), which can lead to vegetation type conversion as chaparral species are sensitive to short fire return intervals (Haidinger and Keeley, 1993; Syphard et al., 2018; Park and Jenerette, 2019). Big-cone Douglas-Fir (Pseudotsuga macrocarpa, hereafter BCDF), a conifer endemic to southern California that often grows on north-facing canyons or ravines, and can be associated with Quercus chrysolepis (Horton, 1960; Minnich, 1976). In mesic, lower elevation sites BCDF primarily grows in small stands within chaparral. Extensive fire-scar analyses suggest that current stands had experienced recurrent fire at perhaps 30 year intervals prior to the 1930s (Lombardo et al., 2009). BCDF is one of the few conifers in California that exists from within low elevation chaparral to high elevation mixed conifer forests. Therefore, this species could be affected by the altered fire regimes in both chaparral and conifer ecosystems (Minnich, 1988) which puts additional strain on the long-term persistence of this regionally restricted species. Identifying fire refugia of the species may be critical for the conservation planning of this geographically limited species particularly as climate change alters fuel aridity, fire regimes and post fire regeneration potential.
BCDF is a fire tolerant conifer that can resprout from epicormic buds after wildfires (Gause, 1966). Despite this, stands are susceptible to wildfire impacts. Historic sources have noted the considerable impact of wildfires on BCDF populations (Leiberg, 1899; U.S. Geologic Survey, 1899), with one researcher describing “large tracts…laid waste” after a wildfire (U.S. Geologic Survey, 1899). Even contemporary research has noted the loss of BCDF in lower montane habitats (Minnich, 1999). While the area of BCDF that has been lost to stand replacing fires is not known (Stephenson and Calcarone, 1999), Minnich (1999) estimated that 18% (approximately 6,000 acres) has been lost in the San Bernardino Mountains since 1938 as a result of stand replacing fires. After stand replacing fires, BCDF stands are typically replaced with chaparral (Stephenson and Calcarone, 1999), particularly Ceanothus or Cercocarpus [now Fragula] species (Leiberg, 1899; U.S. Geologic Survey, 1899). Furthermore, Minnich (1980) found no regeneration in deforested stands 19 years after a high intensity wildfire suggesting stands are susceptible to extirpation in an era of increasing fire severity. Additionally, the species does not have serotinous cones or seeds that can be wind dispersed long distances so a nearby seed source is required for regeneration. Because BCDF trees tend to grow in relatively isolated stands, a severely burned stand has limited ability to regenerate due isolation from other seed sources (Minnich, 1980). It is thought that the isolated growth patterns of BCDF “represent the remnants of a more extensive forest” (U.S. Geologic Survey, 1899). It is possible that over millennia fire has continuously whittled down expansive BCDF forests into the isolated patches seen today.
Refugia have been essential for the persistence of species during changing climates (Tzedakis et al., 2002; Hampe and Petit, 2005) and are receiving increasing attention as it becomes clear that climate and fire refugia need to overlap in order for some species to persist across landscapes into the future (Meddens et al., 2018). Identifying the environmental components that contribute to fire refugia of BCDF is critical given the limited geographic distribution of the species and the altered fire regimes in conifer forests and chaparral shrublands that will affect the distribution of BCDF populations. Additionally, identification of controls on refugia of BCDF will help inform management efforts, including placement of protective fuel breaks and restoration siting, and ensure that these species will continue to provide valuable ecosystem service such as providing shade, food, and vertical structure within shrublands (Meretsky and Snyder, 1992; Vander Wall et al., 2006; Minnich, 2007; Sawyer et al., 2009). While the term “fire refugia” has been defined differently throughout the literature (Meddens et al., 2018), we use the definition from Kolden et al. (2017) that refugia are patches that are unburned or experienced little to no change in live tree cover despite the surrounding area burning, a definition that appears to be broadly used (see Meddens et al., 2018). Because any of the three elements of the fire behavior triangle (weather, fuels, topography) can contribute to the existence of refugia, but only topography is a permanent or stable feature, it is important to distinguish whether it has a significant role and whether this is consistent across different fires in the same vegetation types. Wind-driven fires may create “stochastic” refugia, refugia that are not formed by permanent landscape features like rockiness and topography but rather are the result of the vagaries of particular wind and fire events (Krawchuk et al., 2016). Distinguishing the elements contributing to persistent vs. ephemeral refugia thus helps managers to anticipate future fire impacts and regions where species may persist.
Here, we used ensemble learning methods to compare the environmental associations of high and low mortality (refugia) stands of BCDF burned in two large fires in southern California using two distinct estimates of fire impacts. The assessment of environmental drivers of fire severity including both low and high mortality areas can inform management efforts (see Yin et al., 2021), including where to prioritize restoration for the best long-term outcomes given changes in climate and fire regimes. Ensemble learning is used to better understand the complex relationship between environmental variables like topography, weather, climate, and fuels and fire impacts on vegetation; this approach has been a frequently used method in similar areas of research (Haire and McGarigal, 2009; Holden et al., 2009; Thompson and Spies, 2009; Dillon et al., 2011; Cansler and McKenzie, 2014; Birch et al., 2015; Fang et al., 2015; Harris and Taylor, 2015, 2017; Kane et al., 2015a; Viedma et al., 2015; Estes et al., 2017; Parks et al., 2018). Most of these studies have used a remotely sensed metric (e.g., dNBR, RdNBR, or RBR) to determine how variables contribute to burn severity or to the existence of refugia. Few studies use other metrics (but see Thompson and Spies, 2009; Chapman et al., 2020). We compare and contrast the insights gained by using RdNBR vs. visually-assessed stand mortality (hereafter, “mortality”) as the response variable. Remote sensing burn severity metrics directly measure the change in greenness (Miller and Thode, 2007), which should be associated with mortality or canopy loss (Miller and Thode, 2007). However, in some forest ecosystems canopy trees and understory species can vary in their fire tolerance. Thus, burn severity estimates, or regrowth estimates, may be capturing the effect of fire on understory vegetation rather than the canopy trees, especially if the canopy trees are sparse but the understory is dense and fire susceptible, or vice versa. This could also affect the accuracy of assigning a landscape location to be a refugia. As a result, modeling of the direct impact of wildfire on conifer mortality as assessed visually using aerial imagery may produce different results in some settings than using RdNBR.
The purpose of this study was to determine how elements of the fire behavior triangle affected mortality of BCDF at the stand level scale after two, large mixed severity wildfires in Los Padres National Forest. We ask the following specific questions: (1) What variables contribute to fire refugia (i.e., low mortality) and to high fire-induced mortality of BCDF and are these environmental variables consistent in their rankings across the two large fires? We predict that they should not be consistent across the fires because although the fires were similar in size and habitats affected, the two fires burned under very different weather and climate conditions. We predict that refugia in the second fire, which was strongly wind-driven, will be more stochastic and less affected by permanent landscape features like topography. (2) How does the choice of response variable affect model accuracy and predictor variable strength and ranking? Specifically, does using visually assessed mortality result in different explanatory variables for low (refugia) and high fire impacts and model accuracy, compared to using the more traditional RdNBR as the response variable? This question will help guide future researchers in obtaining a reliable understanding of long-term fire impacts across complex landscapes.
Materials and methods
Study area and fires
We examined mortality and burn severity within BCDF stands in two large fire scars—the 2007 Zaca Fire and the 2017 Thomas Fire (Figure 1). Both fires burned within the Los Padres National Forest in southern California, in chaparral, oak associated, and conifer ecosystems. The Zaca Fire burned 97,208 ha in the summer (July and August) of 2007 and was classified as a non-katabatic fire (Kolden and Abatzoglou, 2018) meaning it was not driven by either Sundowner or Santa Ana wind conditions although a few periods of higher winds did occur within the broad fire window. It was a topography and vegetation-driven fire that burned for almost 2 months under moderate weather conditions with low humidity (Keeley et al., 2009). It burned vegetation that had not burned in >80 years. Approximately 10 years later, the Thomas Fire burned 114,078 ha during December 2017. The Thomas Fire occurred at the tail end of the severe 2012–2018 drought, which killed millions of trees in California (U.S. Department of Agriculture, 2017; Fettig et al., 2019). It was a largely wind-driven fire that occurred during an unprecedented, 12-day Santa Ana wind event (Fovell and Gallagher, 2018; Kolden and Abatzoglou, 2018) and burned through a variety of stand ages. The Zaca Fire affected more BCDF stands (4,348 ha) than the Thomas Fire (1,014 ha). BCDF grows throughout the Zaca Fire scar with a median elevation of 1,344 m (range 857–1,921 m). It has a more limited range in the Thomas Fire because this fire burned in more coastal regions where BCDF is less common. The Thomas Fire affected more low elevation BCDF stands (median 1,289 m; range 440–1,723 m).
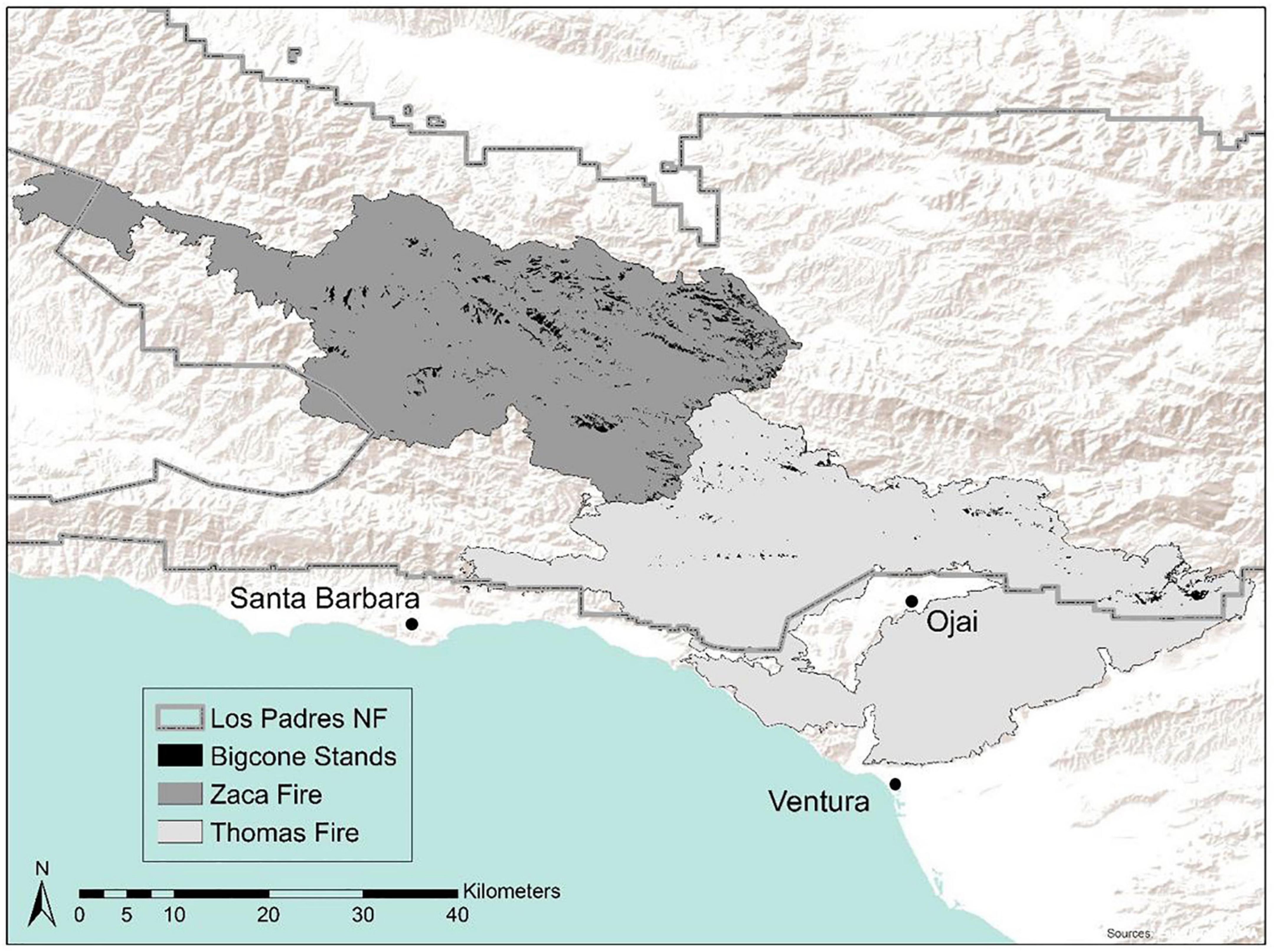
Figure 1. Big-cone Douglas-Fir (BCDF) stands in the Zaca and Thomas Fires. BCDF stands were manually identified from pre-fire Google Earth imagery using methodology from Post-Leon et al. (2022). 4,348 ha of BCDF were impacted during the 2007 Zaca Fire and 1,014 ha impacted during the 2017 Thomas Fire.
Data
We assessed the relationship between mortality or burn severity and environmental variables categorized into variables associated with each of the axes of the landscape fire triangle (topography, weather/climate, fuel). For each variable, the mean was calculated for a given stand, except where noted. Variables associated with each axis are described below.
Burn severity and mortality
BCDF stands were manually identified in the Zaca and Thomas Fire scars following the methodology from Post-Leon et al. (2022). In total, 2,389 stands in the Zaca Fire and 655 stands in the Thomas fire were identified, but only stands greater than 0.118 ha were included in the model (Zaca: n = 1,794; Thomas: n = 478). Mortality for each BCDF stand was manually classified into four bins using Google Earth imagery: unburned (0%), low (1–25%), moderate (25–75%), and high (75–100%) (Table 1). Because BCDF can resprout after wildfires, imagery from several years post-fire was used to classify mortality. 2013–2017 imagery was used to classify mortality after the 2007 Zaca Fire and 2021 imagery was used to classify mortality after the 2017 Thomas Fire; these dates coincide with the 2012–2018 drought, so extra care was taken to ensure mortality estimates were a result of wildfire and not drought.
RdNBR was calculated using 30 m resolution imagery from the Landsat Thematic Mapper; the earliest available cloud-free pre-fire (Zaca Fire: June 21, 2007; Thomas Fire: November 23, 2017) and post-fire (Zaca Fire: August 25, 2007; Thomas Fire: January 26, 2018) images were used. Mean burn severity was calculated for each stand and was classified into unburned (RdNBR < 68), low (RdNBR 69–315), moderate (RdNBR 316–640), and high (RdNBR > 641) bins using classification from Miller and Thode (2007).
We compare how using visually classified mortality as the response compared to RdNBR, influenced VI and the relationship between fire, controlling variables and BCDF. Ultimately, we conducted this analysis to determine if the more time consuming and cost-intensive manual classification of mortality produces higher accuracy models or identifies more distinct importance variables and is thus worth the effort compared to using the more simple and cost-effective method of calculating and using RdNBR.
Topography data
All topographic data were derived from a 30 m Digital Elevation Model (DEM) (U.S. Geological Survey, 2017). Preliminary topographic variables included elevation, annual solar radiation, aspect, maximum flow accumulation, maximum topographic wetness index (TWI), and mean, minimum, and maximum topographic position index (TPI). TPI was calculated for several different neighborhoods (Supplementary material 1) using Topography Tools from Dilts (2015).
Climate and weather data
We considered weather data to be either annual, monthly, or daily. Annual data include annual precipitation from PRISM and values are calculated for the water year. Monthly CWD was provided by Park et al. (2021), and monthly PDSI was extracted from Climate Engine (Abatzoglou, 2013). Daily maximum temperature and daily maximum vapor pressure deficit (VPD) during the fire were downloaded from PRISM, and daily minimum relative humidity from gridMET (Abatzoglou, 2013). Daily fire progression polygons were clipped to daily weather rasters.
Climate data are represented by 30-year averages (1981–2010). Climatic water deficit (CWD), maximum August temperature, maximum December temperature, precipitation were obtained from the Basin Characterization Model (BCM) at 270 m resolution (Flint and Flint, 2014). Maximum August and December VPD was acquired from PRISM at 800 m resolution (Daly et al., 2008).
Fuel data
The vegetation understory and vegetation surrounding (aka vegetation association) each stand was manually classified using Google Earth imagery mostly pre-fire, however, both pre and post fire imagery were used when necessary due to varying levels of image clarity. The dominant vegetation class, besides BCDF, was recorded. Vegetation classification bins included: sparse vegetation (defined as bare ground or boulders/bedrock), oak, chaparral, approximately even mixture of chaparral and oaks (aka chaparral/oak). A joint chaparral, oak, and conifer group was included as an option for classifying vegetation outside of stands since some large stands bordered multiple veg types including other BCDF stands that might have been on the other side of a ridge or slight discontinuity. NDVI, EVI, and NDMI were calculated using pre-fire Landsat imagery from (Zaca Fire: June 21, 2007; Thomas Fire: November 23, 2017).
Other data
Daily burn perimeters (also known as fire progression perimeters) were downloaded from the National Interagency Fire Center (NIFC) FTP site. Daily area burned for each stand was determined by calculating the total hectares burned per day and clipping the daily fire progression layer to the BCDF stands. Reliable daily or hourly wind data were not available on public platforms, so daily area burned was included as a potential explanatory variable as an indirect indicator for daily wind conditions. Time since last fire (TSLF) was also included as a potential explanatory variable.
Random forest models
We used RF (Breiman, 2001), a decision tree algorithm, from the RF package in R (R Core Team, 2019), to identify how environmental variables contributed to either low mortality/refugia or high mortality/burn severity of BCDF stands. RandomForest (RF) does not impose assumptions on the variable’s distributions nor does it overfit the data (Breiman, 2001; Kane et al., 2015b). Unlike other statistical models, such as logistic regressions, RF is not affected by multicollinearity (Bi and Chung, 2011). For these reasons, RF has been increasingly used in studies to disentangle complex ecological processes.
Four binary classification RF models were created for each fire. The first two models were generated to explain correlates with “refugia” using either low mortality (0–25%) or low RdNBR (<315) (see Miller and Thode, 2007), as the response variable. These were classified against all other non-refugia patches. The second two models evaluated patterns of occurrence of high burn severity (RdNBR > 641) and high mortality (>75%) stands compared to all other stands. Moderate RdNBR (315–640)/moderate mortality (26–74%) stands were included with the “other”/non-target vegetation type in each model.
While an important concept regarding refugia is that they can serve as seed sources of future BCDF stands, our analysis excluded examination of the hundreds of stands that experienced moderate (25–75%) mortality. These stands could still have sufficient living adult trees to repopulate the burned portions of the stand over time and also to contribute to the continued existence of BCDF on the landscape. However, per typical definitions of refugia (Blomdahl et al., 2019; Mackey et al., 2021), we focus on stands that experienced minimal change (Krawchuk et al., 2016; Kolden et al., 2017) thereby excluding stands experiencing medium severity since the fate of those is more questionable than either low or high burn severity stands.
Before each model run, the sample size of the largest class was restricted to match the number of observations of the smallest class; this process is known as downsampling (Chen et al., 2004; Evans and Cushman, 2009). This was done because RF classification models can be biased to classes with the larger samples which ultimately skews the out-of-bag (OOB) error (Chen et al., 2004; Khoshgoftaar et al., 2007).
Variable selection
We used a combination of objective and subjective methods to select the final variables for each model. We used VSURF to objectively narrow down the preliminary variables. VSURF is commonly used to select optimal variables for RF and similar models and has been shown to outperform similar programs (Speiser et al., 2019). VSURF uses a stepwise forward approach to select the most important variables based on RF variable importance (VI) permutation scores while simultaneously reducing redundancy. VSURF reduces variables in three steps—the first (i.e., threshold) step removes irrelevant variables, the second (i.e., interpretation) step selects variables important for interpreting the relationship with the response variable, and the third (i.e., prediction) step removes any redundant variables selected in the second step. Since the goal of this analysis is to interpret how environmental variables contribute to BCDF mortality or burn severity, the variables output in the interpretation step were selected as some of the preliminary set of final variables for the model (Table 2). VSURF, particularly the prediction step, was also especially useful to help reduce variables that measured similar features, such as the TPI variables which captured the local topography at different scales.
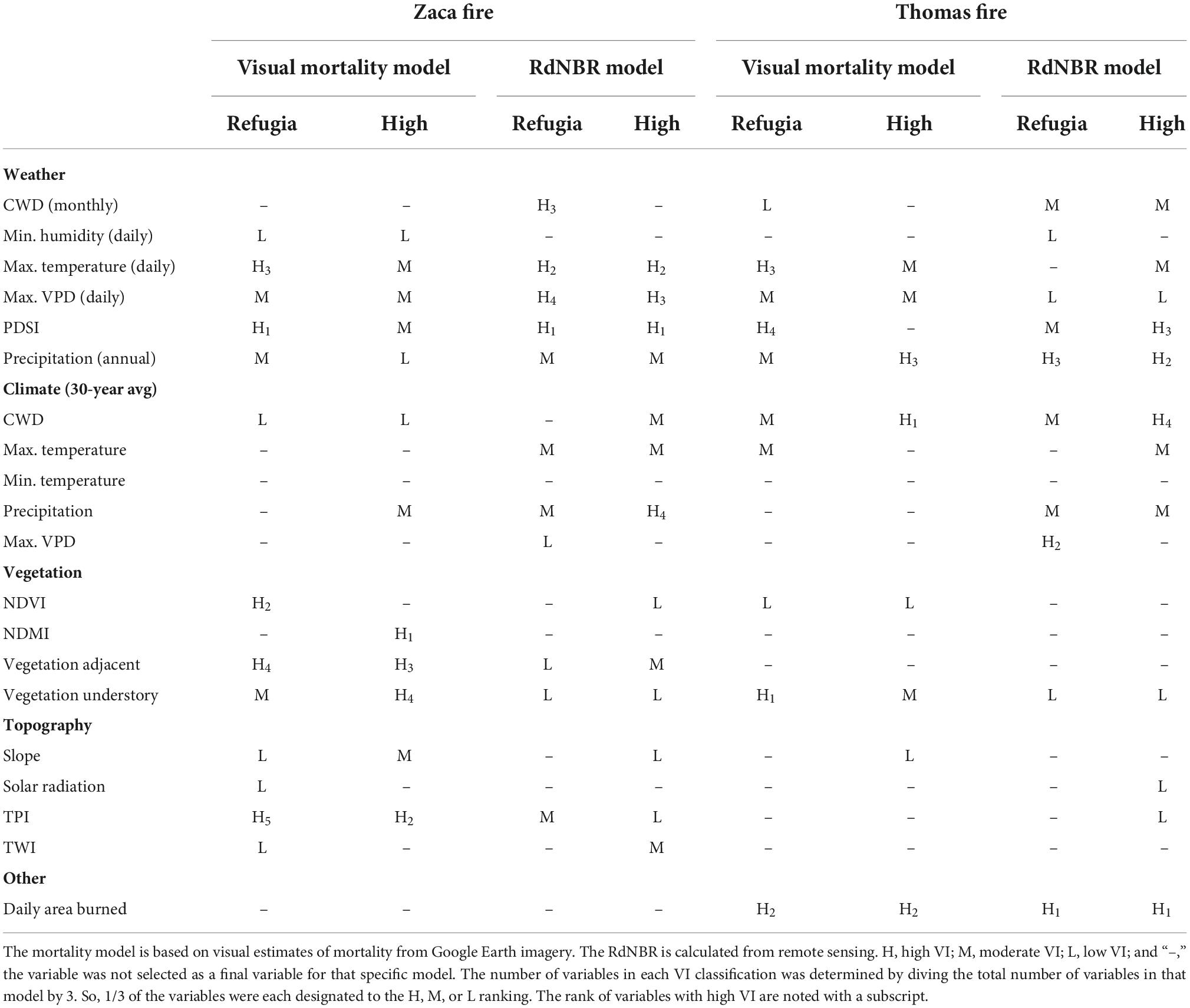
Table 2. Variable importance (VI) rankings for the final variables selected for each of the 4 models.
In some models there were too many correlated variables selected at the interpretation step which ultimately resulted in inconsistent VI rankings. In this case, highly correlated variables and redundant variables were systematically removed until the VI ranking was more or less consistent across model runs. Variables output in the prediction step were used to help determine which correlated or redundant variables should be tested for removal. Additionally, sometimes VSURF did not select all of the non-redundant variables that had informative relationships with the response variable. In this case, we employed some subjectivity in deciding which variables to include as final variables. We took this approach because the purpose of the model is interpretation and hypothesis generation and not strictly identification of an optimal, parsimonious set of variables. Variables that had informative relationships with the response variable (based on ALE plots), continued to decrease model OOB error and increase area under the ROC curve (AUC) while also maintaining stable VI rankings were included as final variables. The exception to this was for TPI variables. If one or no TPI variables were output in the VSURF interpretation step, then no other TPI variables were explored for inclusion in the final model. Through this approach, only two to three additional variables were added to a few models.
Model performance and model interpretation
One feature of the RF package is calculating VI. We used mean decrease accuracy (henceforth referred to as VI) to rank the influence of the explanatory variables on mortality/burn severity. RF estimates VI by quantifying how much prediction error increases when data for that variable is permuted while all others are left unchanged (Liaw and Wiener, 2002). Model OOB error and AUC were used to assess model performance. OOB is the misclassification rate of the samples RF withholds for use as testing data (Breiman, 2001).
Accumulated local effect (ALE) plots were then used to visualize the average relationship between the environmental variables and the response (Apley and Zhu, 2020). ALE plots are robust when modeling correlated variables as the variables are not extrapolated to unrealistic values, like in partial dependence plots (Apley and Zhu, 2020).
Visual mortality vs. RdNBR analysis
We used a chi-square test to determine if there was a significant difference between the number of stands grouped into the visual mortality vs. RdNBR classification bins. We then followed the same variable selection process and used the same metrics of model performance mentioned above. Model AUC, OOB error, class accuracy, and VI rankings were used to compare models. We also compared the ranking of the variables selected for input into the model and compared the ALE plot interpretations to conclude if one method provides more accurate results.
Results
Environmental contributions to low mortality (fire refugia)
Variables falling within all three features of the fire triangle—weather, vegetation, and topography—were important in understanding the location of refugia across the Zaca Fire landscape as one or more highly ranked variables were in each explanatory variable class (Table 2). We relied on the quantity of variables in each explanatory variable class (weather, climate, topography, and fuels) to draw conclusions about the relative importance of each variable class in each of the models. For example, if all topography variables were ranked as low (or not included at all as variables in the model), then we concluded that topography was of low importance in that model for that mortality class. If many of the highly ranked variables were in the vegetation explanatory class, then we concluded that vegetation was the most important variable class for understanding mortality in that model. In the Thomas Fire, topography played no explanatory role, but the other features of the fire triangle—weather and vegetation—were important explanatory classes. Additionally, daily area burned, which is a proxy for wind conditions, was one of the most important variables in the Thomas Fire.
Weather variables acted in different ways to explain refugia in the two fires. Low daily maximum temperatures and low daily VPD were associated with refugia in the Zaca Fire, while higher temperatures and high VPD were associated with refugia in the Thomas Fire (Figure 2).
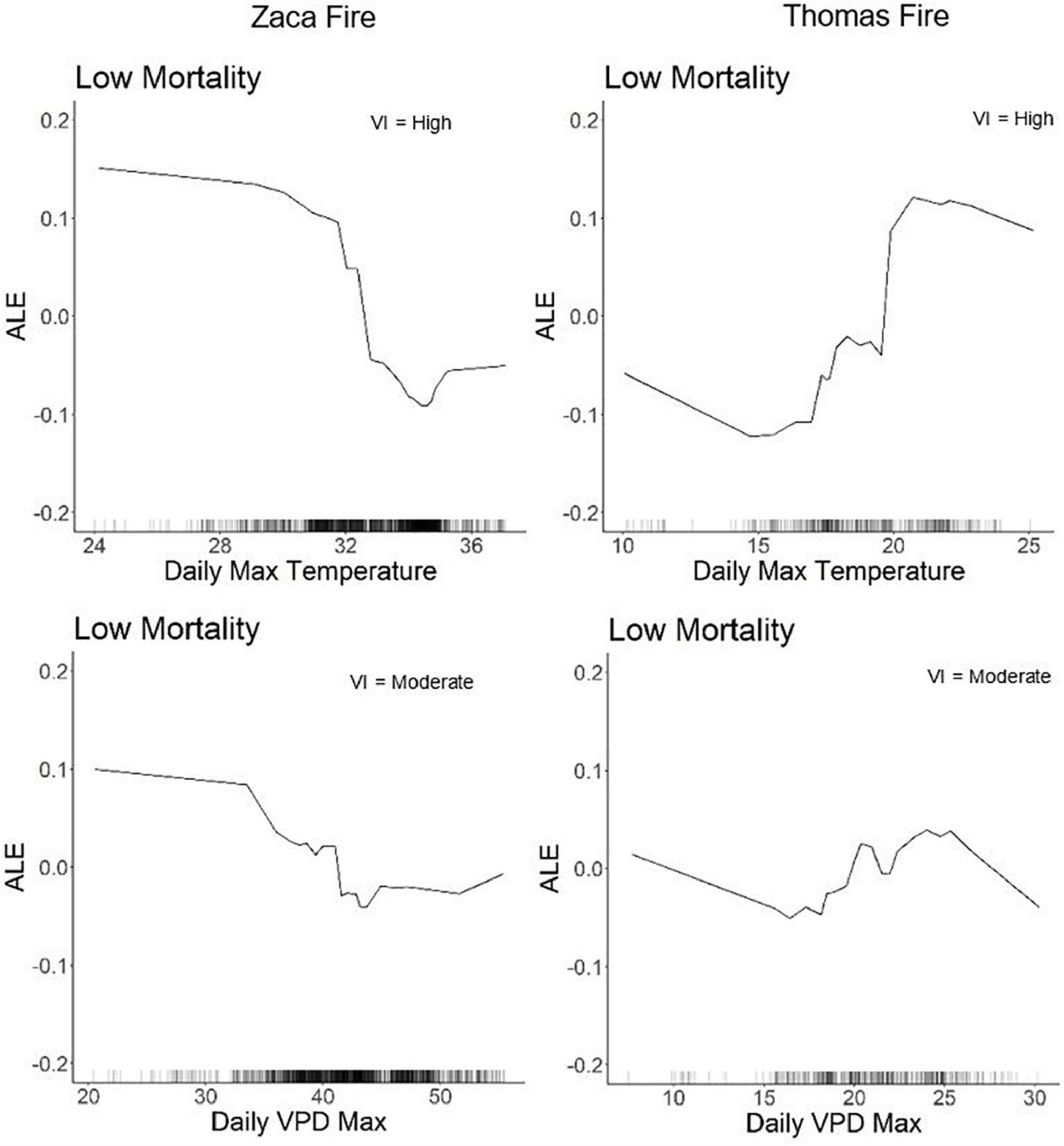
Figure 2. ALE plots for daily maximum temperature and daily VPD in the Zaca and Thomas Fire low mortality (e.g., refugia) models. The black tick marks on the x-axis represent the distribution of values for that variable. Positive values indicate increased likelihood of that mortality class, negative values indicate a decreased likelihood of that mortality class, and values near zero indicate that those values have no effect on classification for that mortality class. The VI ranking of that variable is listed in the top right corner.
Water-related variables (i.e., annual precipitation, monthly CWD, 30-year average CWD, PDSI, and 30 year precipitation) overall refugia were associated with drier, more drought-stressed regions across the landscape, but sometimes had conflicting relationships with mortality between the two fire events and within the same fire event. For example, more negative PDSI (drier conditions) and lower 30-year CWD (less drought stressed vegetation) were associated with refugia in the Zaca Fire, but less negative PDSI (less dry conditions) and higher 30 year CWD (more drought stressed vegetation) were associated with refugia in the Thomas Fire (Figure 3). Some of the water variables had non-linear relationships with refugia locations; very low and very high values of annual precipitation were associated with refugia in the Zaca and Thomas Fires (Supplementary materials 2, 3). Of the two climate variables selected in the Zaca Fire they had conflicting relationships: lower CWD (i.e., lower drought stress) and lower precipitation were associated with refugia. These variables were highly correlated (R2 = 0.847). While weather variables both showed consensus that drier and more drought stressed vegetation were associated with refugia. In the Thomas Fire, the annual weather variables had opposing effects: lower annual precipitation and lower PDSI (i.e., lower drought stress).
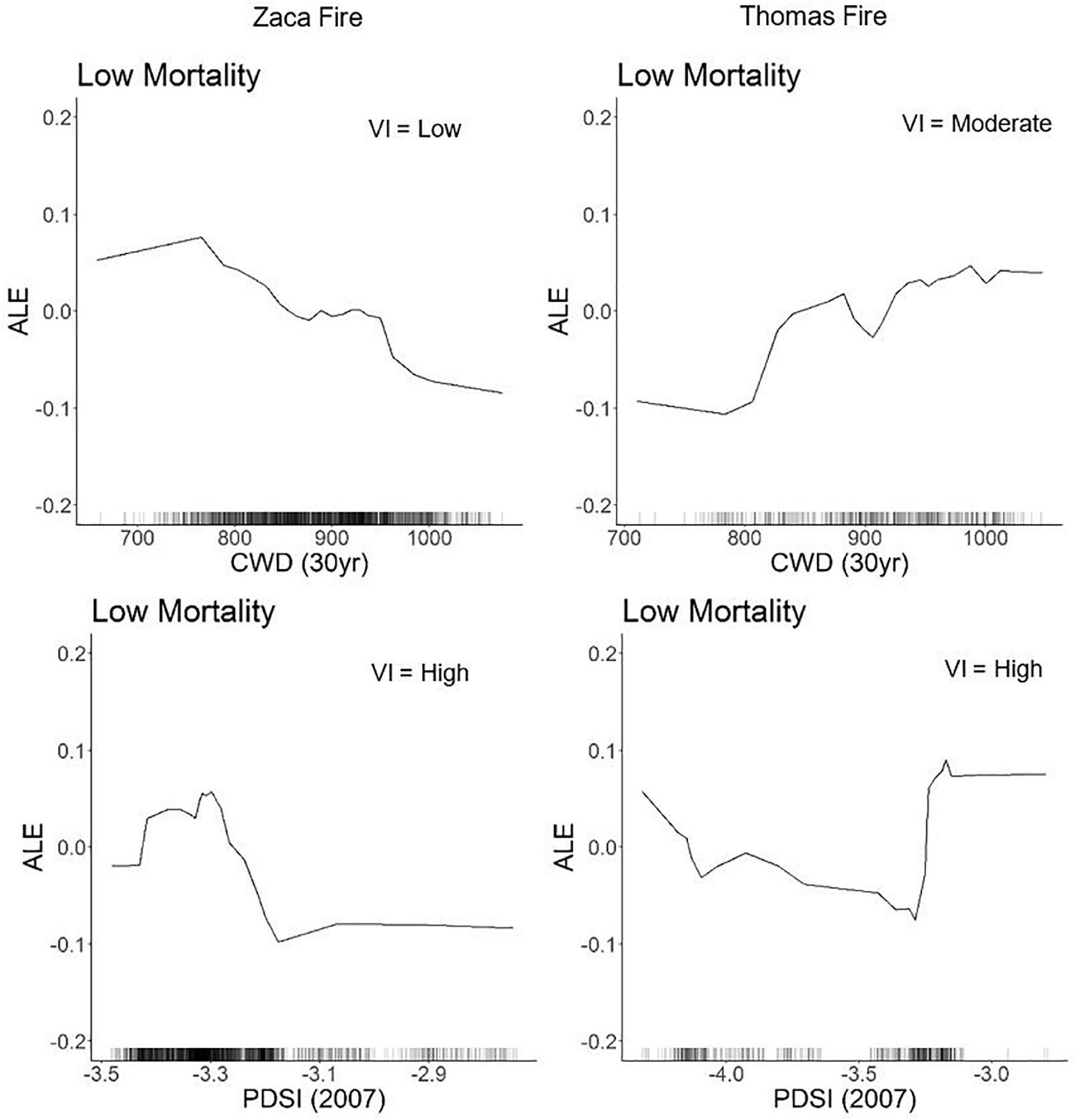
Figure 3. ALE plots for 30-year CWD, a climate variable, and PDSI, a weather variable, have conflicting relationship within and across fire events. The VI ranking of each variable is listed in the top right corner.
The impact of vegetation in our models was high in both fire events, however, the understory vegetation was more important in the Thomas Fire than the Zaca Fire and the adjacent vegetation was more important in the Zaca Fire than the Thomas Fire considering this variable was not selected as a final variable in the latter model (Table 2). A dominant oak or sparse (i.e., bare ground) understory was positively associated with refugia in both fires, and adjacent sparse or chaparral/oak vegetation were positively associated with refugia in the Zaca Fire (Figure 4). If the adjacent vegetation was chaparral, it was a negative predictor of refugia with either response metric. This negative association of refugia with chaparral was also seen in the Thomas Fire, but only when chaparral was inside the stand at the time of fire.
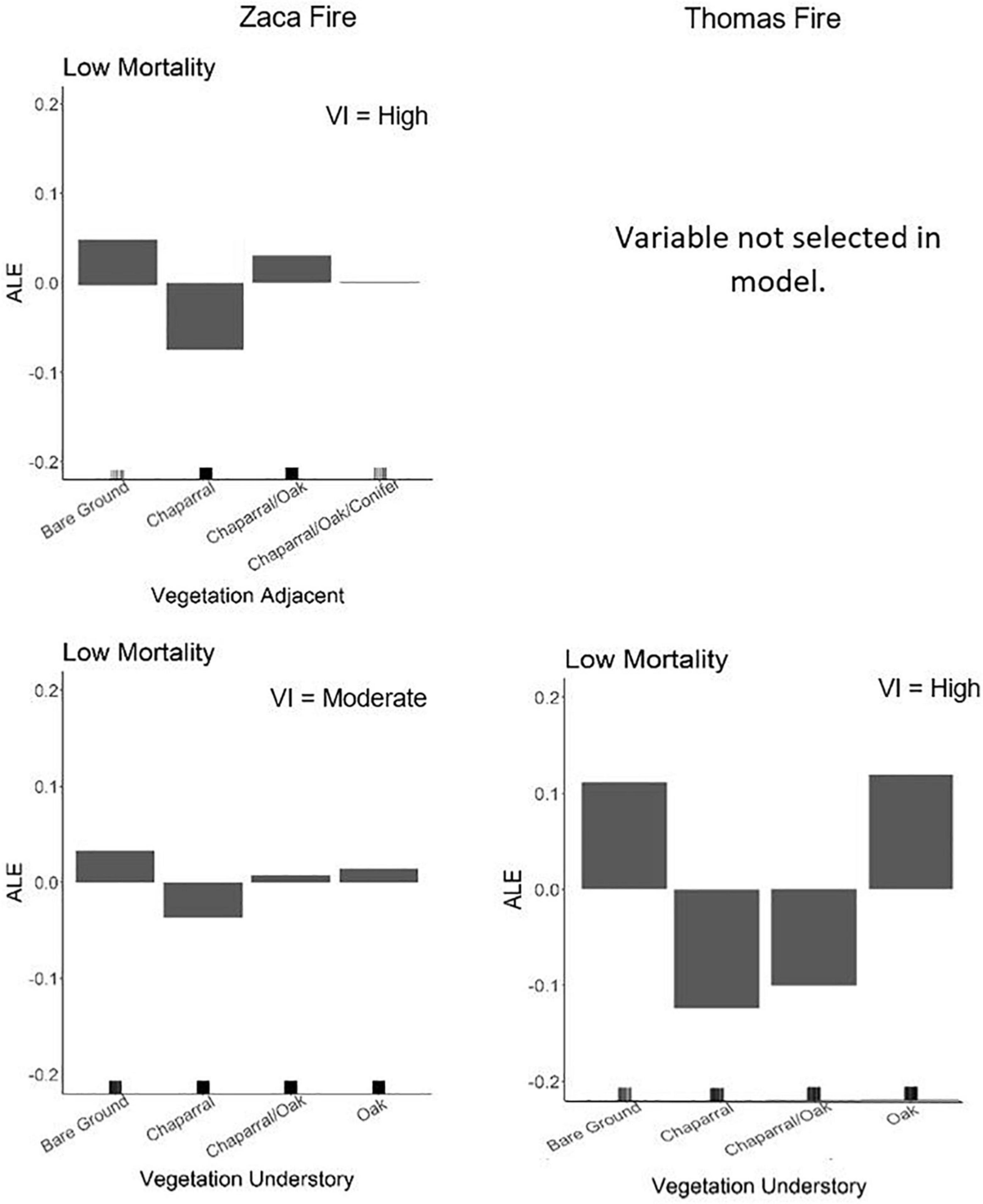
Figure 4. ALE plots for vegetation association and vegetation understory in the Zaca Fire and vegetation understory in the Thomas Fire. Vegetation association was not selected as a final variable in the Thomas Fire and so is not shown. The VI ranking of that variable is listed in the top right corner.
Topography played a role in the fire impact on BCDF in the Zaca Fire, but not the Thomas Fire (Table 2). A stand with high TWI was more likely to act as a fire refugia in the Zaca Fire (Supplementary material 2). Additionally, refugia were associated with steep slopes and valleys (i.e., low TPI) within that fire perimeter.
Environmental contributions to high mortality
Vegetation had a controlling effect on high BCDF mortality in the Zaca Fire (Table 2). In the Thomas Fire there was no dominant explanatory variable class, instead weather and climate were both important while vegetation was moderately important in understanding high mortality (Table 2). The understory vegetation behaved similarly across fire events (Table 2)—a dominant chaparral understory was strongly associated with high fire-driven mortality although this effect was stronger in the Thomas than in the Zaca Fire (Supplementary materials 2, 3). Likewise, if chaparral was adjacent to stands, they had a higher chance of higher mortality in the Zaca Fire (Supplementary material 2).
Similar to the relationship between refugia and BCDF, daily maximum temperature also had inconsistent relationships with high mortality of BCDF across the two fires: high mortality locations were strongly associated with higher maximum temperature in the Zaca Fire and cooler maximum temperatures in the Thomas Fire (Supplementary materials 2, 3).
Several water-related variables were selected for the Zaca Fire: PDSI, annual precipitation, 30 year CWD, and NDMI (Table 2). For these variables, wetter and less drought stressed vegetation were associated with high BCDF mortality except for 30-year CWD which more drought stressed vegetation was associated with high mortality. In contrast, only 30-year CWD was selected in the Thomas Fire to explain high mortality (Table 2), which showed that lower drought stress was associated with high mortality in the Thomas Fire (Supplementary material 3).
High mortality sites were associated with flat or low gradient slopes and non-valleys (i.e., high TPI) in the Zaca Fire (Supplementary material 2). No topographic variable contributed to mortality in the Thomas Fire. Lastly, larger area burned in a day was associated with high mortality in the Thomas Fire and was one of the strongest importance variables (Figure 5). Yet daily burned area was not a relevant variable in understanding mortality in the Zaca Fire (Table 2).
Visual mortality vs. RdNBR
Using RdNBR appears to favor identification of higher burn severity locations: in both fire scars significantly more BCDF stands were classified as having moderate burn severity [Zaca: X2(3) = 556.9, p < 0.0001; Thomas: X2(3) = 193.4, p < 0.0001] or high burn severity [Zaca: X2(3) = 556.9, p < 0.0001; Thomas: X2(3) = 193.4, p < 0.0001] using RdNBR than using the visual mortality approach to classify the stands. In both the Zaca and Thomas Fires, significantly more BCDF stands were classified as having no mortality [Zaca: X2(3) = 556.9, p < 0.0001; Thomas: X2(3) = 193.4, p < 0.0001] and low mortality [Zaca: X2(3) = 556.9, p < 0.0001; Thomas: X2(3) = 193.4, p < 0.0001] using visual mortality classification compared to when using RdNBR (Figure 6).
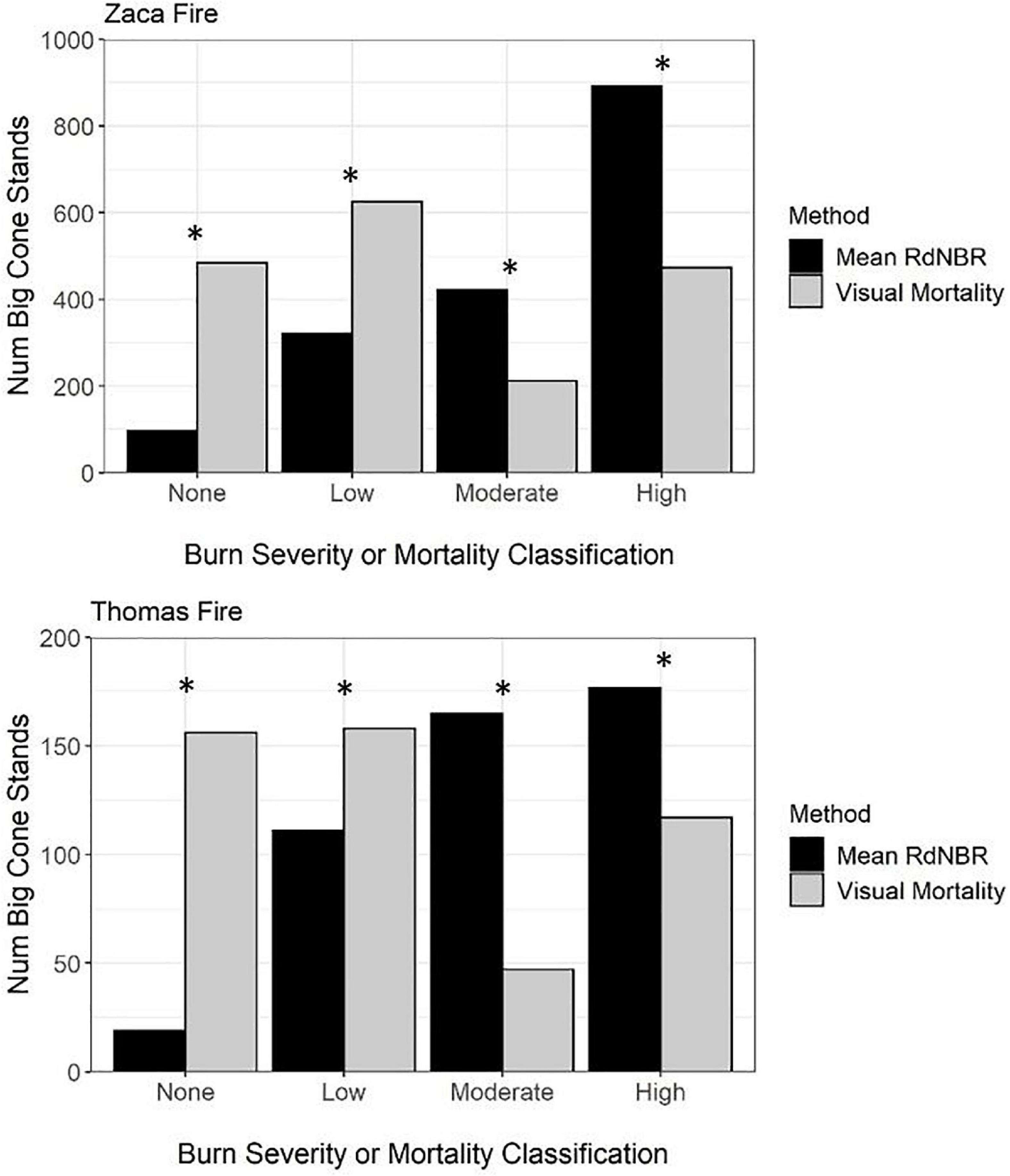
Figure 6. Comparison of the number of BCDF stands classified as having unchanged, low, moderate, or high mortality using both visually assessed mortality estimates or burn severity (RdNBR) estimates using Landsat. A chi-square test was used to determine if there was a significant difference in the number of BCDF stands classified in the various bins using the two different classification methods. *Denotes when there was a significant difference in the number of BCDF stands classified in the bins.
Despite the similarities in the selected variables, VI rankings tended to differ between the models according to response metric, particularly for Zaca Fire (Table 2). In the Zaca Fire, weather was the most influential variable class for explaining both low and high burn severity when RdNBR was the response, while when visual mortality was the response metric, all variable classes were important in understanding low mortality, and vegetation was the most important variable class for understanding high mortality. In fact, for the two mortality/severity classes, vegetation was consistently less important as an explanatory variable when RdNBR was the response metric in both fire scars.
In both Zaca and Thomas Fire refugia models, when RdNBR was the response variable, models had higher AUC and higher OOB (error), but lower class classification accuracy than when visual mortality was used as the response variable (Table 3). Model performance metrics, however, were not consistent across the two fires for the high fire-impact response variables. In the Zaca fire, the high burn severity (RdNBR) model outperformed the visual high mortality model in all three metrics. In contrast, the visual mortality model assessing high mortality in the Thomas Fire outperformed the corresponding RdNBR model in all three metrics (Table 3).
Lastly, where the final variables were consistent in both visual mortality and RdNBR models for each fire, direct comparisons of the trends with the response could be made. Overall, trends remained consistent for both the fire scars; even changes in trend lines generally occurred at the same threshold points (Supplementary materials 2–5). However, there was one main exception to this observation. The relationship between bare ground and mortality/severity was flipped or negated in the Zaca Fire (Supplementary material 4), which was especially apparent with the vegetation understory variable (Figure 7). The role of oaks and chaparral, however, was consistent across response metrics.
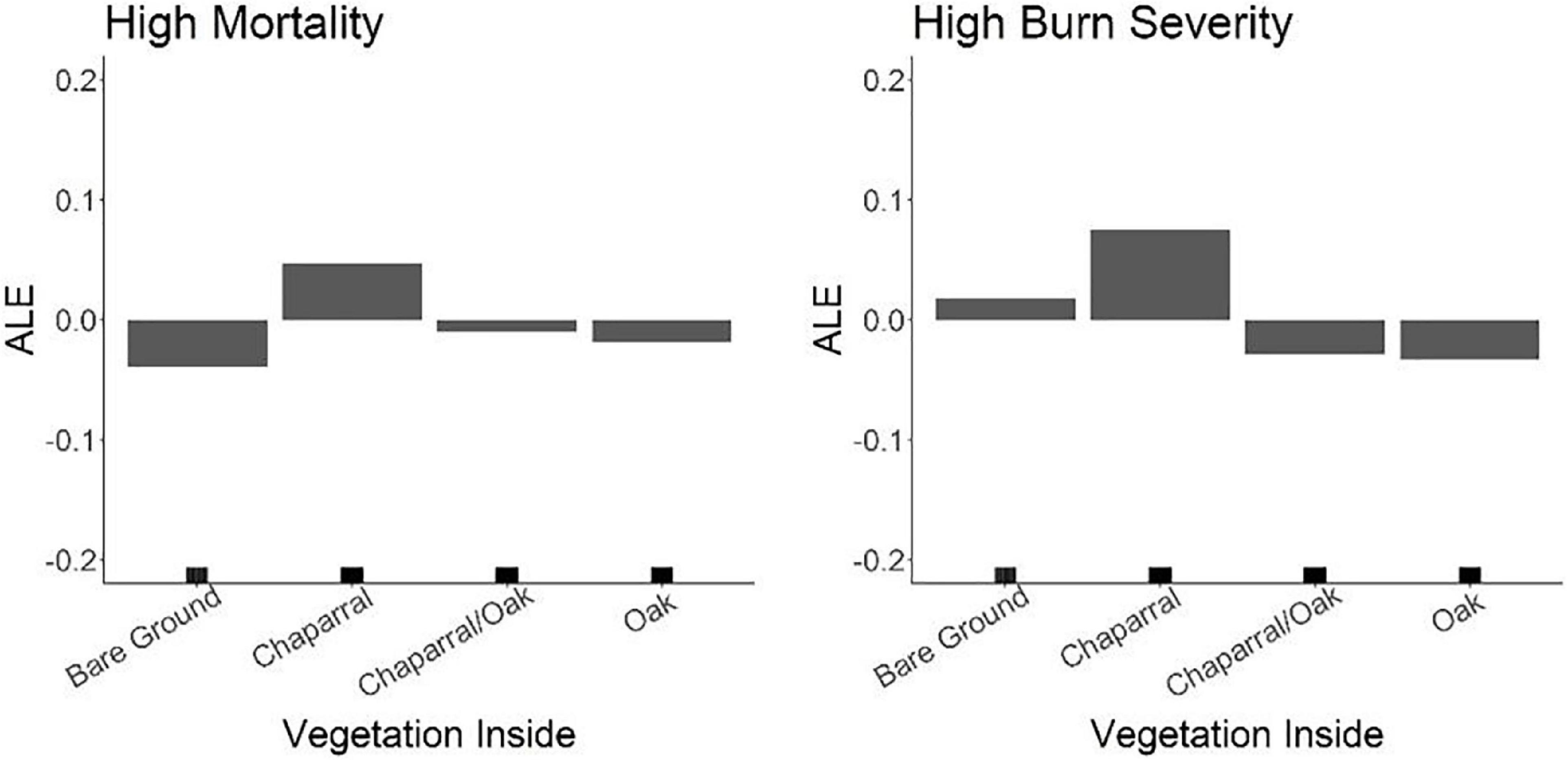
Figure 7. ALE results showing the relationship of bare ground on fire refugia in the Zaca Fire. Depending on the response metric, bare ground contributed to fire refugia when visual mortality was the response, but had the inverse effect when RdNBR was the response.
Discussion
Environmental contributions to fire refugia and high mortality
Fire exerts a controlling influence on BCDF distributions (McDonald, 1990) overall and while BCDF trees are able to resprout after some fires with individual trees recording a history of fire scars (Lombardo et al., 2009), high intensity wildfires can have lasting effects on entire populations (Leiberg, 1899; U.S. Geologic Survey, 1899; Minnich, 1999; Kauffmann et al., 2017). Here we found that refugia exist in both a wind and non-wind driven fire and that some aspects of these refugia are consistent across fires, suggesting that fire refugia are likely to persist into the future. However, severe stressors, like drought, occurring concurrently, preceding, or after a fire can affect BCDF persistence as well as restoration in fire-impacted sites (Post-Leon et al., 2022).
Modeling refugia can be difficult as it can be hard to determine if some unburned or minimally impacted stands are persistent refugia that will continue to provide mitigating effects during future fires. These would contrast with transient refugia that occur because of a sudden change in weather conditions that altered fire behavior or direction in just that one fire (Bradstock et al., 2005; Krawchuk et al., 2016). Several studies identifying refugia use many fires to separate persistent refugia (e.g., Krawchuk et al., 2016; Rogeau et al., 2018; Meigs et al., 2020). However, given the limited distribution of BCDF we could not do that. Instead, by comparing results from a fuel driven fire burning under moderate conditions (i.e., the Zaca Fire) to a wind driven fire burning under extreme weather conditions (i.e., the Thomas Fire) we hoped to generate data to build hypotheses to better predict refugia for BCDF. Our analysis identified common landscape scale refugial characteristics consistent across both types of fire.
Our modeling results demonstrated the landscape features that contributed to low mortality (i.e., fire refugia) of BCDF during both moderate (Zaca) and extreme (Thomas) burning conditions including association with oaks, sparse understories, and xeric sites. Additionally, our models showed that during moderate burning conditions in the vegetation driven Zaca Fire, steep slopes, valleys, low temperatures, low VPD, and high minimum relative humidity also contributed to refugia. In contrast, topography did not really influence mortality or refugia in the wind-driven Thomas Fire. These results are consistent with other research showing that extreme weather conditions can mute the protective effect of landscape features like topography during a wildfire (Collins et al., 2019; Mackey et al., 2021).
There was little consistency in environmental contributions to high mortality across fire scars, except that wetter, less drought stressed regions were more likely to be associated with high mortality. In the Zaca Fire high temperatures, high VPD, low humidity, flat and moderate slopes, and stands in non-valleys or along ridgelines were associated with high stand mortality. In contrast to the Zaca Fire that burned under non-katabatic wind conditions, in the Santa Ana wind driven Thomas Fire (Kolden and Abatzoglou, 2018), denser vegetation, and larger daily area burned, which is an indirect measure of daily wind conditions, were highly important in explaining high BCDF mortality. Daily weather had unexpected relationships with high mortality and refugia, and weaker associations with high mortality, in this fire perhaps because wind driven fires are less predictable in the paths that they burn making refugia more stochastic and harder to predict (Meddens et al., 2018).
Relationship between vegetation, fire refugia, and high mortality
Vegetation association and understory had an enormous impact on BCDF mortality in both fires. While other studies have included fuels data in their modeling efforts (Cansler and McKenzie, 2014; Birch et al., 2015; Viedma et al., 2015; Estes et al., 2017; Harris and Taylor, 2017), typically from LANDFIRE, few studies had as high of resolution for vegetation classification fuels data as our study (Fang et al., 2018). That high resolution analysis from our visual evaluation of Google Earth imagery, complemented our RdNBR analyzes in bringing insight into the role of fuel type and structure. For example, during our Google Earth classification of visual mortality, we frequently observed that fire had burned around a BCDF stand if oaks were growing in or around the stand. Indeed, our modeling confirmed that an oak dominated understory, and in the case of the Zaca Fire oaks around/adjacent to the BCDF stand, contributed to fire refugia. BCDF growing with oaks has been described elsewhere as experiencing lower burn severity (Minnich, 1977, 1980; McDonald, 1990). Such vegetation associations may ultimately be driven by moisture-related growing conditions as Q. chrysolepis is known to be associated with more mesic canyons (Plumb and Gomez, 1983; Boyd, 1999). That oak trees in similar California habitats, may not burn as hot or completely as other vegetation in southern California has been reported elsewhere (e.g., Bendix and Cowell, 2010). It thus seems likely that association of BCDF with Quercus imparts protection from fire mortality and that this is related to fuel moisture.
That chaparral results in high mortality and greatly impacts survivorship of BCDF is clear in analyses for both fires. Because fires in intact chaparral typically burn as active crown fires and these are typically very high intensity (Keeley et al., 2008), it is not surprising that this vegetation is associated with the highest mortality of BCDF stands. While the chaparral in most areas of the Zaca Fire perimeter had not burned in at least 80 years, the chaparral in the Thomas Fire was much younger (most burned in the 1980s) and yet was still associated with high mortality of Big-cone. Thus, our results generally suggest that a mature chaparral understory of any age can result in high mortality for BCDF during fire.
A somewhat surprising finding was that more sparse vegetation, which here occurred in more xeric regions, was associated with low mortality. Rogeau et al. (2018) also found that the proportion of non-fuel (rocks, open soil) in a pixel was a strong predictor of fire refugia in northern conifer forests. In our study, both sparse understories and low NDVI were associated with refugia in our visual inspection of mortality. More xeric sites have less dense vegetation and potentially less fuel continuity. Some researchers have suggested that approximately 10–30% of the landscape needs fuel for a fire to spread (Finney et al., 2006). More xeric sites thus potentially act as areas of fire refugia because they lack fuel continuity and denser fuel loads more typical of mesic sites, and this would result in lower burn intensity (Cochrane et al., 2012). Chaparral by contrast, was consistently associated with high mortality. Because high intensity crown fire is the norm in chaparral (Keeley, 2007), when chaparral stands are adjacent to BCDF they likely contribute to extreme temperatures and high flame lengths within the adjacent or overstory BCDF stands. While BCDF is considered to be fire adapted (Lombardo et al., 2009), its bark may not be thick enough to protect the cambium from severe damage in an extremely hot fire such as occurs in dense chaparral (Stevens et al., 2020).
Relationship between weather, climate, fire refugia, and high mortality
Climate has been found to have a mixed effect on burn severity and fire behavior throughout the literature (Thompson and Spies, 2009; Dillon et al., 2011; Birch et al., 2015; Parks et al., 2018). This may in part be due to the many scales at which climate and weather operate. Weather and climate act at multiple scales from local and short-term during a fire, to pre-fire weather conditions (affecting NMDI and LFM), vs. more regional and longer term [via an influence on long-term (30-year) drought stress and ultimately vegetation patterns]. While climate is typically not important during the fire itself (a very short-term event), longer term climate, along with other local factors like topography, exerts a dominant control on landscape scale vegetation patterns (Cansler and McKenzie, 2014; Estes et al., 2017). This is likely because climate (30-year averages) reflects long-term trends that will dictate vegetation composition (Zerbo et al., 2016), while short-term weather (here, weather was at monthly or annual scales) better reflects the conditions or health of the vegetation that is currently present. Overall, we did not find that climate had a particularly controlling or consistent effect on mortality in the Zaca and Thomas Fires.
While the relationship between daily weather and mortality was not similar between the two fires, the relative importance of weather in the refugia and high mortality models was similar despite the bulk of the area that burned during the Thomas Fire burned in a short period of time (25 days) during overall cooler winter temperatures compared to the long burning (50 days) hot summer temperatures of the Zaca Fire. The Thomas Fire burned when strong Santa Ana winds were the driving force of fire behavior. Also, Moritz (2003) demonstrated that Santa Ana winds could drive fire through almost any age of vegetation in Southern California diminishing influences like daily temperature, live fuel moisture, or fuel structure. We could not include wind as a variable in the spatial modeling because reliable, high resolution wind data were not available on public platforms, but we included daily area burned (windier days would spread the fire further) as a proxy. The fact that it was one of the two most important variables driving mortality in the Thomas Fire models (Table 2) is consistent with the dominant role these winds play in driving fire patterns across the region (Kolden and Abatzoglou, 2018) overriding influences of short term and local scale climate. By contrast, daily area burned was not selected as a relevant variable that contributed to mortality in the Zaca Fire. Keeley et al. (2009) concluded that the Zaca Fire was a topography and vegetation driven fire that burned under moderate weather conditions, which is reflected in our modeling results where weather, topography, and vegetation were relatively equally important contributors to refugia and vegetation was the dominant variable class in understanding high mortality.
Lastly, we did not expect our results to overall indicate that drier regions would be associated with fire refugia and wetter regions to be associated with high mortality. As mentioned above, sparser vegetation was found to be in the more xeric locations in our study area demonstrating the link between broader climate and weather patterns and local vegetation density and live fuel moisture, which then ultimately impacts fire behavior.
Relationship between topography, fire refugia, and high mortality
The literature commonly describes suitable BCDF habitat as north facing canyons and ravines, steeper slopes, and/or on ridgelines (Bolton and Vogl, 1969; Minnich, 1980; McDonald, 1990). Our RF results from the Zaca Fire indicated that these stable topographic features may provide suitable refugia for BCDF protecting the stands from fire altogether or reducing the severity of fire. Areas where water accumulates (i.e., high TWI and low TPI), such as canyons, ravines, and valleys, should have higher soil moisture and thus higher live fuel moistures. Additionally, despite some researchers finding that steeper slopes were associated with higher fire mortality (Holden et al., 2009; Estes et al., 2017), steep slopes were actually areas of refuge for BCDF in this study. Reduced fuel continuity on steep slopes likely contributed to them acting as refugia. Lastly, the Zaca model indicated that areas with higher TPI, which indicated non-valleys, ridgelines, or areas closer to ridgelines, were associated with high mortality. However, ridgelines can provide a topographic barrier that limits fire spread as there is often less vegetation to burn or discontinuity in fuel (McDonald and Littrell, 1976; Rogeau et al., 2018). Upon visual inspection, several low mortality BCDF stands on ridgelines had sparse understories. Sparse understories, which included stands growing in or around boulders, were associated with fire refugia, meaning ridgelines likely acted as fire refugia for BCDF if they lacked vegetation cover. The lack of understory or adjacent vegetation reduces the likelihood of high intensity crown fire.
Since topography is commonly found to be an important predictor in fire modeling studies (Haire and McGarigal, 2009; Dillon et al., 2011; Birch et al., 2015; Harris and Taylor, 2015; Estes et al., 2017), it was unexpected that only one topographic variable contributed to mortality in one Thomas Fire model. We believe that because of the extreme wind conditions that occurred during this fire, the typical refugial properties of different portions of the landscape were negated: strong winds drive wide distribution of embers and fire brands, and extreme fire behavior (Gill and Allan, 2008). This observation suggests that otherwise stable landscape features associated with refugia may not be reliable under extreme conditions.
Visual mortality vs. RdNBR as the response variable
Burn severity metrics such as dNBR, RdNBR, and RBR are commonly used in post-fire models to examine the relationship between burn severity and environmental variables (Dillon et al., 2011; Birch et al., 2015; Viedma et al., 2015; Harris and Taylor, 2017; Fang et al., 2018; Parks et al., 2018). Here we wanted to explore a more direct relationship between wildfire and conifer mortality and so conducted the visual mortality assessment with Google Earth imagery. When comparing the number of BCDF stands that were classified into their respective mortality and burn severity classes, we found that the RdNBR burn severity classes commonly did not associate with the same mortality classification. In both fires, remotely sensed RdNBR significantly overestimated the number of stands with high and moderate mortality and significantly underestimated the number of stands with no and low mortality compared to visual assessment, perhaps due to the sensitivity of RdNBR to fire impacts on the understory (Kibler et al., 2019).
This fundamental difference in classification led us to predict that explanatory variables would behave differently depending on the response variable. Despite differences in stand classification, when the same variables were selected for both models, the overarching variable trends were consistent (Supplementary materials 2–5), with one exception. The vegetation adjacent and understory variables had conflicting results in just the Zaca Fire models—sparse vegetation association and sparse vegetation understory (i.e., high soil exposure) were associated with fire refugia when visual mortality was the response variable, but sparse adjacent vegetation had a negative association with low burn severity and no association with high burn severity and sparse understory was associated with high burn severity when RdNBR was the response variable. The former makes more ecological sense as a lack of vegetation disrupts fuel continuity leading to lower burn severity and thus lower mortality. The results in the RdNBR model may be a byproduct of how RdNBR is calculated: while RdNBR takes into account pre-fire cover (Miller and Thode, 2007), high soil cover can be associated with unusually high RdNBR values (Huete et al., 1984). This is one case using RdNBR would have led to incorrect conclusions about the association of vegetation and BCDF stand survival.
Based on model assessment metrics it was difficult to definitively conclude whether one response metric was superior to another (Table 3) since AUC and OOB value comparisons often went in different directions. Overall, all models resulted in AUC values in the 0.8–0.9 range. This range is considered to be very good for predictive values of a model (Hosmer et al., 2013). In both the Zaca and Thomas Fires, visual mortality resulted in better (lower) OOB but poorer (lower) AUC when predicting refugia. This was true for predicting high mortality locations in the Zaca Fire, but the opposite was true for the Thomas Fire. Hence accuracy alone does not suggest that one response metric or one accuracy method, is consistently better than another.
When considering the feasibility of acquiring the response metrics, RdNBR is less time consuming because Landsat imagery is freely available, pre-processed and relatively little work is needed to acquire usable imagery for a broad region. By contrast, manually classifying mortality was a time, labor, and cost intensive process that cannot be easily or quickly conducted over large geographic areas. BCDF stands first had to be identified because there were no reliable maps showing BCDF populations, which is likely true for many species, including those with discontinuous distributions like BCDF. Then mortality had to be visually classified for over 3,000 stands. Yet, without this approach it would not have been possible to classify the underlying and surrounding vegetation composition, which provided valuable insight into the casual relationship between fire and BCDF mortality including the protective effects of oak associations. Vegetation association was only of low to moderate importance in the RdNBR models likely because of the biased influence of understory on RdNBR measurements. Nonetheless, the biggest contrast between the modeled outcomes was the stronger role of weather and long-term climate variables in the RdNBR models compared to the mortality models.
We conclude that using RdNBR in ensemble models may be preferable when VI ranking is not the primary goal because it is more cost and time effective and accuracies are not consistently better with one or the other. This may be particularly true for consistently, or densely forested conifer ecosystems where the RdNBR may be less influenced by a fire sensitive, dense understory like chaparral. By contrast, if obtaining the VI rankings for use in management is the primary goal of a post-fire analysis, then it must be understood that RdNBR may misrepresent fire severity relationships in some areas. One such situation is when vegetation is a patchwork of shrubland and different types of woodland and forest, in which case visual classification of mortality can provide valuable management insights.
Restoration implications
Our results indicate that restoration of BCDF should focus in areas that have at least one of the three following vegetation characteristics: low chaparral density, high oak density, or sparse understory. For practical reasons, areas with high oak density may be the most suitable for restoration. BCDF stands with sparse understory, although unlikely to burn at high intensity, are not ideal restoration sites because these areas are more arid. Conifer seedling establishment is sensitive to precipitation patterns (Varmola et al., 2000; Brown and Wu, 2005; Rother et al., 2015) so drier sites may have a lower chance of long-term survival of outplanted seedlings. By contrast, canyons and valleys are ideal restoration sites particularly if oaks are present, due to the increased access to water, which will be necessary for sapling survival.
BCDF stands that suffered the highest mortality, while seeming to be most in need of restoration, are those that were likely within chaparral before fire. Since high fire intensity is associated with higher post-fire shrub densities (Russell et al., 1998; Nagel and Taylor, 2005) regardless of pre-fire vegetation composition, these sites will quickly regenerate to chaparral. These chaparral seedlings may prove competitive for BCDF seedlings. In addition, restoring within a vegetation type that is likely to eventually reburn at high intensity should be a low priority.
Lastly, our results can be used to prioritize fuel management work. Thinning chaparral around or under BCDF stands that meet other fire refugia criteria could increase the survivability of a valued stand. Such stands could be ones that harbor high genetic diversity and offer a large source of seed to surrounding areas, or areas that are culturally valuable for other reasons. Additionally, managers could consider planting Quercus chrysolepis individuals simultaneously with outplanting of BCDF seedlings since this association seems to aid survival of BCDF during fire.
Conclusion
Given the rapid pace of anthropogenic climate and fire regime change, the identification and protection of refugia are increasingly important conservation priorities in forested landscapes (Morelli et al., 2020). For regionally restricted species such BCDF, these concerns are pressing, as refugia will be important for maintaining the presence of this species in the increasingly dry and fire-prone habitats of southern California. High intensity fires are likely to continue to destroy BCDF stands while drought is likely to limit regeneration success (Runte et al., in press). Fire refugia will be increasingly important for the persistence of rare and geographically limited species, such as BCDF, as anthropogenic factors continue to alter historic fire regimes (McKenzie et al., 2004).
Our results contribute to the growing literature showing that places that are refugia in a fuel driven fire may not be refugia in a wind driven fire (Collins et al., 2019; Mackey et al., 2021). This was demonstrated when several topographic variables were contributors to refugia in the Zaca Fire, yet they were not important in the Thomas Fire presumably because topography was overridden by high wind conditions during the Thomas Fire. One consistent contributor to refugia across fires was understory vegetation, thus demonstrating that even under severe wind conditions vegetation can behave predictably—chaparral will contribute to high mortality and oaks will contribute to refugia.
The key to the long-term persistence of BCDF will be identifying where fire, drought, and climate refugia overlap. Not all areas of fire refugia will also be drought or climate refugia. Our modeling efforts have demonstrated that canyons and valleys are suitable habitat and this may be in part because these features decrease burn severity. These features have the added benefit of being areas of climate and drought refugia since they have reduced evaporative demand and more access to water (i.e., higher TWI) and maybe areas of cold air drainage. Regions with sparse vegetation and low NDVI were identified as fire refugia, but these attributes tend to be located in the drier, more drought-stressed regions of the landscape thus they may not be long term climate refugia. While the vegetation in these regions appear to be less affected by drought than higher elevation sites today (Post-Leon et al., 2022), the higher aridity and low precipitation of these areas would make them challenging for active restoration should stands there be impacted by fire.
Data availability statement
The original contributions presented in this study are included in the article/Supplementary material, further inquiries can be directed to the corresponding author/s.
Author contributions
A-MP, CD’A, and MM: study conception and design and analysis and interpretation of results. A-MP: data collection. A-MP and CD’A: draft manuscript preparation. All authors contributed to the article and approved the submitted version.
Funding
This research was funded by the National Fish and Wildlife Foundation (NFWF, ID: 64532) grants to CD’A and MM.
Acknowledgments
We thank C. Kibler and I. Park for their assistance with modeling methodology and to N. Molinari (USFS) for feedback and encouragement throughout. Numerous volunteers helped with evaluation of understory cover from Google Earth images. We thank R. Hazard, Santa Barbara County Fire Battalion Chief, for sharing his knowledge on the local weather conditions during the Zaca and Thomas Fires. Finally, we also thank two reviewers for comments that helped improve the clarity of this manuscript.
Conflict of interest
The authors declare that the research was conducted in the absence of any commercial or financial relationships that could be construed as a potential conflict of interest.
Publisher’s note
All claims expressed in this article are solely those of the authors and do not necessarily represent those of their affiliated organizations, or those of the publisher, the editors and the reviewers. Any product that may be evaluated in this article, or claim that may be made by its manufacturer, is not guaranteed or endorsed by the publisher.
Supplementary material
The Supplementary Material for this article can be found online at: https://www.frontiersin.org/articles/10.3389/ffgc.2022.995537/full#supplementary-material
References
Abatzoglou, J. T. (2013). Development of gridded surface meteorological data for ecological applications and modelling. Int. J. Climatol. 33, 121–131. doi: 10.1002/joc.3413
Abatzoglou, J. T., and Williams, A. P. (2016). Impact of anthropogenic climate change on wildfire across western US forests. Proc. Natl. Acad. Sci. U.S.A. 113, 11770–11775. doi: 10.1073/pnas.1607171113
Apley, D. W., and Zhu, J. (2020). Visualizing the effects of predictor variables in black box supervised learning models. J. R. Stat. Soc. Ser. B 82, 1059–1086. doi: 10.1111/rssb.12377
Bendix, J., and Cowell, C. M. (2010). Impacts of wildfire on the composition and structure of riparian forests in southern California. Ecosystems 13, 99–107. doi: 10.1007/s10021-009-9303-z
Bi, J., and Chung, J. (2011). Identification of drivers of overall liking – Determination of relative importances of regressor variables. J. Sensory Stud. 26, 245–254. doi: 10.1111/j.1745-459X.2011.00340.x
Birch, D. S., Morgan, P., Kolden, C. A., Abatzoglou, J. T., Dillon, G. K., Hudak, A. T., et al. (2015). Vegetation, topography and daily weather influenced burn severity in central Idaho and western Montana forests. Ecosphere 6:17.
Blomdahl, E. M., Kolden, C. A., Meddens, A. J., and Lutz, J. A. (2019). The importance of small fire refugia in the central Sierra Nevada, California, USA. For. Ecol. Manage. 432, 1041–1052. doi: 10.1016/j.foreco.2018.10.038
Bolton, R. B. Jr., and Vogl, R. J. (1969). Ecological requirements of Pseudotsuga macrocarpa in the Santa Ana Mountains, California. J. Forestry 67, 112–116. doi: 10.1093/jof/67.2.112
Bond, W. J., and Keeley, J. E. (2005). Fire as a global ‘herbivore’: The ecology and evolution of flammable ecosystems. Trends Ecol. Evol. 20, 387–394. doi: 10.1016/j.tree.2005.04.025
Boyd, S. (1999). Vascular flora of the Liebre Mountains, western Transverse Ranges, California. Aliso. 18, 93–139.
Bradstock, R. A., Bedward, M., Gill, A. M., and Cohn, J. S. (2005). Which mosaic? A landscape ecological approach for evaluating interactions between fire regimes, habitat and animals. Wildl. Res. 32, 409–423. doi: 10.1071/WR02114
Brown, P. M., and Wu, R. (2005). Climate and disturbance or episodic tree recruitment in a southwestern ponderosa pine landscape. Ecology 86, 3030–3038. doi: 10.1890/05-0034
Cansler, C., and McKenzie, D. (2014). Climate, fire size, and biophysical setting control fire severity and spatial pattern in the northern Cascade Range, USA. Ecol. Appl. 24, 1037–1056. doi: 10.1890/13-1077.1
Chapman, T. B., Schoennagel, T., Veblen, T. T., and Rodman, K. C. (2020). Still standing: Recent patterns of post-fire conifer refugia in ponderosa pine-dominated forest of the Colorado Front Rage. PLoS One 15:e0226926. doi: 10.1371/journal.pone.0226926
Cochrane, M. A., Moran, C. J., Wimberly, M. C., Baer, A. D., Finney, M. A., Beckendorf, K. L., et al. (2012). Estimation of wildfire size and risk changes due to fuels treatments. Int. J. Wildl. Fire 21, 357–367. doi: 10.1071/WF11079
Collins, L., Bennett, A. F., Leonard, S. W. J., and Penman, T. D. (2019). Wildfire refugia in forests: Severe fire weather and drought mute the influence of topography and fuel age. Glob. Change Biol. 25, 3829–3843. doi: 10.1111/gcb.14735
Daly, C., Halbleib, M., Smith, J. I., Gibson, W. P., Doggett, M. K., Taylor, G. H., et al. (2008). Physiographically sensitive mapping of climatological temperature and precipitation across the conterminous United States. Int. J. Climatol. 28, 2031–2064. doi: 10.1002/joc.1688
Dillon, G. K., Holden, Z. A., Morgan, P., Crimmins, M. A., Heyerdahl, E. K., and Luce, C. H. (2011). Both topography and climate affected forest and woodland burn severity in two regions of the western US, 1984 to 2006. Ecosphere 2:art130. doi: 10.1890/ES11-00271.1
Dilts, T. E. (2015). Topography Tools for ArcGIS 10.1. ArcGIS. Available online at: https://www.arcgis.com/home/item.html?id=b13b3b40fa3c43d4a23a1a09c5fe96b9
Donato, D. C., Fontaine, J. B., Campbell, J. L., Robinson, W. D., Kauffman, J. B., and Law, B. E. (2009). Conifer regeneration in stand-replacement portions of a large mixed-severity wildfire in the Klamath–Siskiyou Mountains. Can. J. For. Res. 39, 823–838. doi: 10.1139/X09-016
Estes, B. L., Knapp, E. E., Skinner, C. N., Miller, J. D., and Preisler, H. K. (2017). Factors influencing fire severity under moderate burning conditions in the Klamath Mountains, northern California, USA. Ecosphere 8:e01794. doi: 10.1002/ecs2.1794
Evans, J. S., and Cushman, S. A. (2009). Gradient modeling of conifer species using random forests. Landsc. Ecol. 24, 673–683. doi: 10.1007/s10980-009-9341-0
Fang, L., Yang, J., White, M., and Liu, Z. (2018). Predicting potential fire severity using vegetation, topography and surface moisture availability in a Eurasian boreal forest landscape. Forests 9:130. doi: 10.3390/f9030130
Fang, L., Yang, J., Zu, J., Li, G., and Zhang, J. (2015). Quantifying influences and relative importance of fire weather, topography, and vegetation on fire size and fire severity in a Chinese boreal forest landscape. For. Ecol. Manage. 356, 2–12. doi: 10.1016/j.foreco.2015.01.011
Fettig, C. J., Mortenson, L. A., Bulaon, B. M., and Foulk, P. B. (2019). Tree mortality following drought in the central and southern Sierra Nevada, California, U.S. For. Ecol. Manage. 432, 164–178. doi: 10.1016/j.foreco.2018.09.006
Finney, M. A., Seli, R. C., McHugh, C. W., Ager, A. A., Bahro, B., and Agee, J. K. (2006). “Simulation of long-term landscape-level fuel treatment effects on large wildfires,” in Fuels Management-How to Measure Success: Conference Proceedings, eds P. L. Andrews and B. W. Butler (Fort Collins, CO: Rocky Mountain Research Station, U.S. Forest Service), 28–30.
Flint, L. E., and Flint, A. L. (2014). California basin characterization model: A dataset of historical and future hydrologic response to climate change, (ver. 1.1, May 2017): U.S. Geological Survey Data. Available online at: https://doi.org/10.5066/F76T0JPB
Fovell, R., and Gallagher, A. (2018). Winds and Gusts during the Thomas Fire. Fire 1:47. doi: 10.3390/fire1030047
Gause, G. W. (1966). Silvical characteristics of bigcone Douglas-fir. Berkeley, CA: Pacific Southwest Forest and Range Experiment Station.
Gill, A. M., and Allan, G. (2008). Large fires, fire effects and the fire-regime concept. Int. J. Wildl. Fire 17, 688–695. doi: 10.1071/WF07145
Haidinger, T. L., and Keeley, J. E. (1993). Role of high fire frequency in destruction of mixed chaparral. Madroño 40, 141–147.
Haire, S. L., and McGarigal, K. (2009). Changes in fire severity across gradients of climate, fire size, and topography: A landscape ecological perspective. Fire Ecol. 5, 86–103. doi: 10.4996/fireecology.0502086
Hampe, A., and Petit, R. J. (2005). Conserving biodiversity under climate change: The rear edge matters. Ecol. Lett. 8, 461–467. doi: 10.1111/j.1461-0248.2005.00739.x
Harris, L., and Taylor, A. H. (2015). Topography, Fuels, and Fire Exclusion Drive Fire Severity of the Rim Fire in an Old-Growth Mixed-Conifer Forest, Yosemite National Park, USA. Ecosystems 18, 1192–1208. doi: 10.1007/s10021-015-9890-9
Harris, L., and Taylor, A. H. (2017). Previous burns and topography limit and reinforce fire severity in a large wildfire. Ecosphere 8:e02019. doi: 10.1002/ecs2.2019
Holden, Z. A., Morgan, P., and Evans, J. S. (2009). A predictive model of burn severity based on 20-year satellite-inferred burn severity data in a large southwestern US wilderness area. For. Ecol. Manage. 258, 2399–2406. doi: 10.1016/j.foreco.2009.08.017
Horton, J. S. (1960). Vegetation types of the San Bernardino Mountains. Tech. Rep. PSW-44. Berkeley, CA: Pacific Southwest Forest and Range Experiment Station. doi: 10.5962/bhl.title.70233
Hosmer, D. W., Lemeshow, S., and Sturdivant, R. X. (2013). Applied logistic regression. Oxford: Wiley. doi: 10.1002/9781118548387
Huete, A. R., Post, D. F., and Jackson, R. D. (1984). Soil spectral effects on 4-space vegetation discrimination. Remote Sens. Environ. 15, 155–165. doi: 10.1016/0034-4257(84)90043-9
Kane, V. R., Cansler, C. A., Povak, N. A., Kane, J. T., McGaughey, R. J., Lutz, J. A., et al. (2015a). Mixed severity fire effects within the Rim fire: Relative importance of local climate, fire weather, topography, and forest structure. For. Ecol. Manage. 358, 62–79. doi: 10.1016/j.foreco.2015.09.001
Kane, V. R., Lutz, J. A., Alina Cansler, C., Povak, N. A., Churchill, D. J., Smith, D. F., et al. (2015b). Water balance and topography predict fire and forest structure patterns. For. Ecol. Manage. 338, 1–13. doi: 10.1016/j.foreco.2014.10.038
Kauffmann, M., Evens, J., and Ratchford, J. (2017). Bigcone Douglas-Fir mapping and inventorying in the Angeles National Forest. Fremontia 45, 30–41.
Keeley, J. E., Brennan, T., and Pfaff, A. H. (2008). Fire severity and ecosystem responses following crown fires in California shrublands. Ecol. Appl. 18, 1530–1546. doi: 10.1890/07-0836.1
Keeley, J. E., Safford, H., Fotheringham, C. J., Franklin, J., and Moritz, M. (2009). The 2007 Southern California wildfires: Lessons in complexity. J. Forestry 107, 287–296. doi: 10.1093/jof/107.6.287
Kelly, L. T., Haslem, A., Holland, G. J., Leonard, S. W. J., MacHunter, J., Bassett, M., et al. (2017). Fire regimes and environmental gradients shape vertebrate and plant distributions in temperate eucalypt forests. Ecosphere 8:e01781. doi: 10.1002/ecs2.1781
Khoshgoftaar, T. M., Golawala, M., and Hulse, J. V. (2007). “An empirical study of learning from imbalanced data using random forest,” in Proceedings of the 19th IEEE International Conference on Tools with Artificial Intelligence (ICTAI 2007), Vol. 2, NW Washington, DC, 310–317. doi: 10.1109/ICTAI.2007.46
Kibler, C. L., Parkinson, A. M. L., Peterson, S. H., Roberts, D. A., D’Antonio, C. M., Meerdink, S. K., et al. (2019). Monitoring post-fire recovery of chaparral and conifer species using field surveys and landsat time series. Remote Sens. 11:2963. doi: 10.3390/rs11242963
Kolden, C. A., and Abatzoglou, J. T. (2018). Spatial distribution of wildfires ignited under katabatic versus non-katabatic winds in Mediterranean Southern California USA. Fire 1:19. doi: 10.3390/fire1020019
Kolden, C. A., Bleeker, T. M., Smith, A. M. S., Poulos, H. M., and Camp, A. E. (2017). Fire Effects on historical wildfire Refugia in contemporary wildfires. Forests 8:400. doi: 10.3390/f8100400
Krawchuk, M. A., Haire, S. L., Coop, J., Parisien, M. A., Whitman, E., Chong, G., et al. (2016). Topographic and fire weather controls of fire refugia in forested ecosystems of northwestern North America. Ecosphere 7:e01632. doi: 10.1002/ecs2.1632
Leiberg, J. B. (1899). The San Bernardino forest reserve. Twentieth Annual report of the United States Geological Survey, 1898- 1899: Part V - Forest reserves. (Washington, DC: U.S. Government Publishing Office), 29–454. doi: 10.3133/ar20_5
Liaw, A., and Wiener, M. (2002). Classification and Regression by randomForest. R News. Available online at: https://cran.r-project.org/doc/Rnews/ (accessed May 21, 2019).
Lombardo, K. J., Swetnam, T. W., Baisan, C. H., and Borchert, M. I. (2009). Using bigcone douglas-fir fire scars and tree rings to reconstruct interior chaparral fire history. Fire Ecol. 5, 35–56. doi: 10.4996/fireecology.0503035
Mackey, B., Lindenmayer, D., Norman, P., Taylor, C., and Gould, S. (2021). Are fire refugia less predictable due to climate change? Environ. Res. Lett. 16:114028. doi: 10.1088/1748-9326/ac2e88
McDonald, P. M. (1990). “Pseudotsuga macrocarpa (Vasey) Mayr bigcone Douglas-fir,” in Silvics of North America. Volume 1. Conifers. Agricultural Handbook. 654, eds R. M. Burns and B. H. Honkala (Washington, DC: U.S. Department of Agriculture, Forest Service), 520–526.
McDonald, P. M., and Littrell, E. E. (1976). The Bigcone Douglas-Fir—Canyon Live Oak Community in Southern California. Madroño 23, 310–320.
McKenzie, D., Gedalof, Z. E., Peterson, D. L., and Mote, P. (2004). Climatic change, wildfire, and conservation. Conserv. Biol. 18, 890–902. doi: 10.1111/j.1523-1739.2004.00492.x
Meddens, A. J. H., Kolden, C. A., Lutz, J. A., Smith, A. M. S., Cansler, C. A., Abatzoglou, J. T., et al. (2018). Fire Refugia: What are they, and Why do they matter for global change? BioScience 68, 944–954. doi: 10.1093/biosci/biy103
Meigs, G. W., Dunn, C. J., Parks, S. A., and Krawchuk, M. A. (2020). Influence of topography and fuels on fire refugia probability under varying fire weather conditions in forests of the Pacific Northwest, USA. Can. J. For. Res. 50, 636–647. doi: 10.1139/cjfr-2019-0406
Meretsky, V. J., and Snyder, N. F. R. (1992). Range use and movements of California condors. Condor 94, 313–335. doi: 10.2307/1369205
Miller, J. D., and Thode, A. E. (2007). Quantifying burn severity in a heterogeneous landscape with a relative version of the delta Normalized Burn Ratio (dNBR). Remote Sens. Environ. 109, 66–80. doi: 10.1016/j.rse.2006.12.006
Minnich, R. A. (1976). “Vegetation of the San Bernardino Mountains,” in Proceedings of the plant communities of southern California; 1974 May 4, ed. J. Latting (Fullerton, CA: California Native Plant Society), 99–124.
Minnich, R. A. (1977). “The geography of fire and big-cone Douglas-fir, Coulter pine and western conifer forests in the east transverse ranges, southern California,” in Proceedings of the symposium on the environmental consequences of fire and fuel management in Mediterranean ecosystems; 1977 August 1-5; Palo Alto, CA. Gen. Tech. Rep. WO-3, eds H. A. Mooney and C. E. Conrad (Washington, DC: U.S. Department of Agriculture, Forest Service), 443–450.
Minnich, R. A. (1980). “Wildfire and the geographic relationships between canyon live oak, coulter pine, and Bigcone Douglas- fir Forests,” in Proceedings of the Symposium on the Ecology, Management, and Utilization of California Oaks, (Albany, CA: USDA Forest Service).
Minnich, R. A. (1988). The biogeography of fire in the san bernardino mountains of California: A historical study, Vol. 28. Berkeley, CA: University of California Press.
Minnich, R. A. (1999). “Vegetation, fire regimes, and forest dynamics,” in Oxidant air pollution impacts in the montane forests of Southern California. Ecological Studies, Vol. 134, eds P. R. Miller and J. R. McBride (New York, NY: Springer). doi: 10.1007/978-1-4612-1436-6_4
Minnich, R. A. (2007). “Southern California conifer forests,” in Terrestrial Vegetation of California, 3rd Edn, eds M. G. Barbour, T. Keeler-Wolf, and A. A. Shoenedder (Berkeley, CA: University of California Press), 502–538.
Morelli, T. L., Barrows, C. W., Ramirez, A. R., Cartwright, J. M., Ackerly, D. D., Eaves, T. D., et al. (2020). Climate-change refugia: Biodiversity in the slow lane. Front. Ecol. Environ. 18, 228–234. doi: 10.1002/fee.2189
Moritz, M. A. (2003). Spatiotemporal analysis of controls on shrubland fire regimes: age dependency and fire hazard. Ecology 84, 351–361.
Nagel, T. A., and Taylor, A. H. (2005). Fire and Persistence of Montane Chaparral in Mixed Conifer Forest Landscapes in the Northern Sierra Nevada, Lake Tahoe Basin, California, USA. J. Torrey Bot. Soc. 132, 442–457.
Nigro, K., and Molinari, N. (2019). Status and trends of fire activity in southern California yellow pine and mixed conifer forests. For. Ecol. Manage. 441, 20–31. doi: 10.1016/j.foreco.2019.01.020
Park, I. W., and Jenerette, G. D. (2019). Causes and feedbacks to widespread grass invasion into chaparral shrub dominated landscapes. Landsc. Ecol. 34, 459–471. doi: 10.1007/s10980-019-00800-3
Park, I. W., Mann, M. L., Flint, L. E., Flint, A. L., and Moritz, M. (2021). Relationships of climate, human activity, and fire history to spatiotemporal variation in annual fire probability across California. PLoS One 16:e0254723. doi: 10.1371/journal.pone.0254723
Parks, S. A., Holsinger, L. M., Panunto, M. H., Jolly, W. M., Dobrowski, S. Z., and Dillon, G. K. (2018). High-severity fire: Evaluating its key drivers and mapping its probability across western US forests. Environ. Res. Lett. 13:044037. doi: 10.1088/1748-9326/aab791
Plumb, T. R., and Gomez, A. P. (1983). Five southern California oaks: Identification and postfire management. Gen. Tech. Rep. PSW-71. Berkeley, CA: Pacific Southwest Forest and Range Experiment Station.
Post-Leon, A., Dryak, M., Zhu, E., De Guzman, M., Salladay, R., Moritz, M., et al. (2022). Integration of landscape-level remote sensing and tree-level ecophysiology reveals drought refugia for a rare endemic, bigcone Douglas-fir. Front. Forests Glob. Change. 5:946728. doi: 10.3389/ffgc.2022.946728
Rogeau, M. P., Barber, Q. E., and Parisien, M. A. (2018). Effect of topography on persistent fire refugia of the Canadian Rocky Mountains. Forests 9:285.
Rother, M. T., Veblen, T. T., and Furman, L. G. (2015). A field experiment informs expected patterns of conifer regeneration after disturbance under changing climate conditions. Can. J. For. Res. 45, 1607–1616. doi: 10.1139/cjfr-2015-0033
Russell, W. H., McBride, J., and Rowntree, R. (1998). Revegetation after four stand-replacing fires in the Lake Tahoe basin. Madroño (USA). Available online at: https://agris.fao.org/agris-search/search.do?recordID=US1999004807 (accessed August 14, 2017).
Safford, H. D., and Van de Water, K. M. (2014). Using fire return interval departure (FRID) analysis to map spatial and temporal changes in fire frequency on national forest lands in California (PSW-RP-266; p. PSW-RP-266). Albany, CA: U.S. Department of Agriculture, Forest Service, Pacific Southwest Research Station. doi: 10.2737/PSW-RP-266
Sawyer, J. O., Keeler-Wolf, T., and Evens, J. M. (2009). A manual of California vegetation. Sacramento, CA: California Native Plant Society.
Smith, J. K. (2000). Wildland fire in ecosystems: Effects of fire on fauna (RMRS-GTR-42-V1; p. RMRS-GTR-42-V1). Fort Collins, CO: Rocky Mountain Research Station, U.S. Forest Service. doi: 10.2737/RMRS-GTR-42-V1
Speiser, J. L., Miller, M. E., Tooze, J., and Ip, E. (2019). A comparison of random forest variable selection methods for classification prediction modeling. Expert Syst. Appl. 134, 93–101. doi: 10.1016/j.eswa.2019.05.028
Steel, Z. L., Safford, H. D., and Viers, J. H. (2015). The fire frequency-severity relationship and the legacy of fire suppression in California forests. Ecosphere 6:art8. doi: 10.1890/ES14-00224.1
Stephenson, J. R., and Calcarone, G. M. (1999). Southern California mountains and foothills assessment: Habitat and species conservation issues (PSW-GTR-172; p. PSW-GTR-172). Berkeley, CA: Pacific Southwest Research Station. doi: 10.2737/PSW-GTR-172
Stevens, J. T., Kling, M. M., Schwilk, D. W., Varner, J. M., and Kane, J. M. (2020). Biogeography of fire regimes in western US conifer forests: A trait-based approach. Glob. Ecol. Biogeogr. 29, 944–955.
Syphard, A. D., Brennan, T. J., and Keeley, J. E. (2018). “Chaparral landscape conversion in Southern California,” in Valuing chaparral: Ecological, socio-economic, and management perspectives, eds E. C. Underwood, H. D. Safford, N. A. Molinari, and J. E. Keeley (Cham: Springer International Publishing), 323–346. doi: 10.1007/978-3-319-68303-4_12
Syphard, A. D., Radeloff, V. C., Keeley, J. E., Hawbaker, T. J., Clayton, M. K., Stewart, S. I., et al. (2007). Human influence on California fire regimes. Ecol. Appl. 17, 1388–1402. doi: 10.1890/06-1128.1
Thompson, J. R., and Spies, T. A. (2009). Vegetation and weather explain variation in crown damage within a large mixed-severity wildfire. For. Ecol. Manage. 258, 1684–1694. doi: 10.1016/j.foreco.2009.07.031
Tzedakis, P. C., Lawson, I. T., Frogley, M. R., Hewitt, G. M., and Preece, R. C. (2002). Buffered tree population changes in a quaternary Refugium: Evolutionary implications. Science 297, 2044–2047. doi: 10.1126/science.1073083
U. S. Geologic Survey (1899). Nineteenth Annual Report of the United States Geological Survey to the Secretary of the Interior 1897—1898. Washington, DC: Government Printing Office. doi: 10.3133/ar19
U.S. Department of Agriculture (2017). Drought and tree mortality in the Pacific Southwest region. Department of Agriculture, Forest Service, Pacific Southwest Station. Available online at: https://www.fs.usda.gov/psw/topics/tree_mortality/california/documents/DroughtFactSheet_R5_2017.pdf (accessed November 01, 2021).
U.S. Geological Survey (2017). 3DEP products and services: The National Map, 3D Elevation Program Web page. Available online at: https://nationalmap.gov/3DEP/3dep_prodserv.html (accessed August, 2018).
Vander Wall, S. B., Borchert, M. I., and Gworek, J. R. (2006). Secondary Dispersal of big cone Douglas-fir (Pseudotsuga macrocarpa) Seeds. Acta Oecol. 30, 100–106. doi: 10.1016/j.actao.2006.02.004
Varmola, M., Salminen, H., Rikala, R., and Kerkelä, M. (2000). Survival and early development of Lodgepole pine. Scand. J. For. Res. 15, 410–423. doi: 10.1080/028275800750172619
Viedma, O., Quesada, J., Torres, I., De Santis, A., and Moreno, J. M. (2015). Fire Severity in a Large Fire in a Pinus pinaster Forest is Highly Predictable from Burning Conditions, Stand Structure, and Topography. Ecosystems 18, 237–250. doi: 10.1007/s10021-014-9824-y
Welch, K. R., Safford, H. D., and Young, T. P. (2016). Predicting conifer establishment post wildfire in mixed conifer forests of the North American Mediterranean-climate zone. Ecosphere 7:e01609. doi: 10.1002/ecs2.1609
Yin, C., Xing, M., Yebra, M., and Liu, X. (2021). Relationships between burn severity and environmental drivers in the temperate coniferous Forest of Northern China. Remote Sens. 13:5127. doi: 10.3390/rs13245127
Zackrisson, O. (1977). Influence of forest fires on the North Swedish Boreal Forest. Oikos 29, 22–32. doi: 10.2307/3543289
Keywords: Pseudotsuga macrocarpa, RdNBR, randomForest, ALE plots, wildfire, chaparral understory
Citation: Parkinson A-ML, D’Antonio CM and Moritz MA (2022) Influence of topography, vegetation, weather, and climate on Big-cone Douglas-Fir fire refugia and high fire-induced mortality after two large mixed-severity wildfires. Front. For. Glob. Change 5:995537. doi: 10.3389/ffgc.2022.995537
Received: 16 July 2022; Accepted: 25 October 2022;
Published: 17 November 2022.
Edited by:
Andres Holz, Portland State University, United StatesReviewed by:
Francisco Mauro, Universidad de Valladolid, SpainMaría Menéndez-Miguélez, Instituto Nacional de Investigación y Tecnología Agroalimentaria (INIA), Spain
Copyright © 2022 Parkinson, D’Antonio and Moritz. This is an open-access article distributed under the terms of the Creative Commons Attribution License (CC BY). The use, distribution or reproduction in other forums is permitted, provided the original author(s) and the copyright owner(s) are credited and that the original publication in this journal is cited, in accordance with accepted academic practice. No use, distribution or reproduction is permitted which does not comply with these terms.
*Correspondence: Anne-Marie L. Parkinson, aparkinson@bren.ucsb.edu