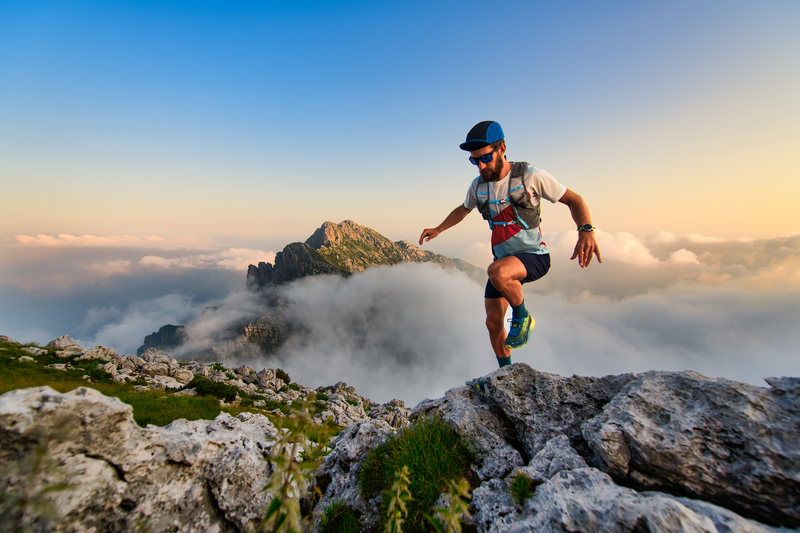
94% of researchers rate our articles as excellent or good
Learn more about the work of our research integrity team to safeguard the quality of each article we publish.
Find out more
ORIGINAL RESEARCH article
Front. For. Glob. Change , 20 September 2022
Sec. Forest Management
Volume 5 - 2022 | https://doi.org/10.3389/ffgc.2022.977691
This article is part of the Research Topic Mountainous Forest Ecosystems: Challenges and Management Implications View all 16 articles
Climate change is one of the significant factors influencing global species redistribution. As a result, a better understanding of the species' possible range change in future climate conditions is needed. Therefore, this study compiles global geographic occurrence data of a wild olive sub-species, Olea europaea subsp. cuspidate, and projected potential distribution models in current and future climate scenarios. This study using ensemble modeling predicted that the species will undergo a significant decrease in habitat suitability under future climatic conditions with a contraction ranging from ca. 41 and 42% under RCP4.5 2050 and to about 56 and 61% under RCP8.5 2070 for committee averaging and weighted mean, respectively. More specifically, there will be a decrease in habitat suitability in regions of the southeastern part of the United States in North America; coastal regions in South America; coastal regions in the majority of eastern Africa; coastal parts of Spain, France, Italy, and Greece in Europe; coastal parts of Yemen and Saudi Arabia; the southeastern parts of Pakistan and the southern part of China in Asia; and southwestern and eastern parts of Australia when compared to current habitat suitability. The results of this ensemble modeling could be extremely valuable in identifying cultivation hotspots for the effective restoration and protection of this olive lineage under future climatic conditions.
Climate change is one of the key components determining the species' range redistribution (Parmesan et al., 2011; Pacifici et al., 2015). As such, changing climatic conditions will likely expand or shrink the species' geographic ranges (Chen et al., 2011; Palmer et al., 2015). Adding to the worries are the consequences of global warming, which are expected to exacerbate in the next 50 years (Ahmad et al., 2019a). The Intergovernmental Panel on Climate Change Sixth Assessment Report (IPCC, 2021) states that the ongoing climate warming has caused the pole-ward shift of numerous plant and animal species in both the southern and the northern hemisphere and the growing season, especially in the northern hemisphere extratropics, has expanded by 2 days each decade since the 1950s. A plethora of research studies have reported that recent anthropogenic-induced ecological changes are responsible for species range shifts (Malhi et al., 2020), changes in phenology (Negi et al., 2021), and species extinctions (Román-Palacios and Wiens, 2020). However, there is still a dearth of information about the biological dynamics of these shifting climatic impacts and documentation of climate change hotspots of vulnerability and resilience (Bellard et al., 2012). Furthermore, rising global temperatures have resulted in significant climatic zone shifts in several areas around the globe, including the substantial expansion of arid climatic zones and the shrinkage of polar climatic zones (IPCC, 2019). As a result, many native species have experienced a substantial shift in their geographical ranges, abundances, and seasonality of activities (Weiskopf et al., 2020). All these negative consequences act as barriers to the management and conservation of biodiversity (Thomas et al., 2004; Butchart et al., 2010; Cook et al., 2012; IPCC, 2012; Pacifici et al., 2017; Pecl et al., 2017; Convention on Biological Diversity, 2020).
As ecology and conservation disciplines require a robust understanding of species distribution and habitat requirements, species distribution models (SDM) are, therefore, one of the essential techniques for spatio-temporal predictions of biodiversity at the biogeographic scale (Alvarado-Serrano and Knowles, 2014; Naimi, 2015; Srivastava et al., 2019). SDM projections can be used to develop sustainable management plans to help minimize the effects of climate change (Pyke and Fischer, 2005; Schorr et al., 2012; Porfirio et al., 2014). However, one major disadvantage of these distribution models is that there are already a large number of modeling algorithms available, and this number is expanding all the time, making it difficult to choose the optimal methodology (Elith et al., 2010; Ahmad et al., 2019b). To overcome this problem, the ensemble modeling technique implemented in the biomod2 package provides a valuable platform for determining the species' current distribution and predicting their future potential Spatio-temporal distributions under changing climatic scenarios (Gillard et al., 2017; Thuiller et al., 2019).
RCPs (representative concentration pathways) represent greenhouse gas emissions, atmospheric concentrations, air pollutant emissions, and land use in the twenty-first century (Vuuren et al., 2011). Based on the mitigation scenarios' trajectory, four (RCPs) have been described (IPCC, 2014). In order to identify suitable habitats for species in the 2050s and 2070s, the model was thus trained using current climate data and projected onto future climate change bioclimatic datasets for all RCPs scenarios. Therefore, to estimate the impact of climate change on species distribution, multiple scenarios based on various RCPs must be examined (Araujo and Rahbek, 2006; Parmesan, 2006; Beaumont et al., 2007; Bellard et al., 2012). This paper is based on the global distribution modeling of Olea europaea subsp. cuspidata (synonyms—Olea ferruginea, Olea africana, Olea chrysophylla) using an ensemble modeling approach. It is a wild species of olive lineage (Green, 2002) and is believed to be of Mediterranean origin (De Candolle, 1882) and a prominent part of Mediterranean vegetation (De Ollas et al., 2019). However, the species is introduced into Australia, New Zealand, and the Pacific islands as a rootstock for cultivated olive (Besnard et al., 2014). Due to over-exploitation, Ethiopia's species is extremely threatened (Negash, 2010). It is used for various purposes, including quality fuel wood and furniture (Negash, 2010), and is also a valuable source of natural antioxidants and bioactive materials (Long et al., 2010). It has numerous medicinal and anti-bacterial properties (Masoko and Makgapeetja, 2015). Therefore, climate change affects livelihoods and economic security, particularly in communities, along with its distribution range. More specifically, we aim to address the following key questions: (1) What is the current distribution of Olea europaea subsp. cuspidata? (2) What will be the future potential distribution of this species under different climate change scenarios? and (3) what are the key bioclimatic variables affecting the distribution of this species?
Occurrence data for Olea europaea subsp. cuspidata was downloaded primarily from the Global Biodiversity Information Facility (GBIF, 2021) using the gbif function from the dismo package (Hijmans et al., 2020), Botanical Information and Ecology Network Database (BIEN; accessed on 01 August 2021) using the BIEN_occurrence_species function from BIEN package (Maitner et al., 2018) and was further supplemented with intensive field surveys carried by the authors from North Western India and Herbarium records from Forest Research Institute (FRI) Dehradun and various centers of Botanical Survey of India (BSI Dehradun, BSI Kolkatta, and HAWHRC Solan). A total of 4,599 georeferenced occurrence points were obtained from the above-mentioned sources for Olea europaea subsp. cuspidata. The obtained occurrence dataset was subsequently processed to exclude any locations that fell into the oceans. As a result, 4,461 points were retained. Furthermore, it is a well-known fact that distribution data is frequently skewed toward locations that are geographically user-friendly and easily accessible (Hijmans et al., 2005). This can manifest itself in the form of geographical sample bias (Ahmad et al., 2019a). We employed spatial thinning to minimize spatial autocorrelation and sample bias by dividing the entire region into 10 × 10 km grid cells and selecting a single occurrence point from each cell with one or more occurrence points. After geographic thinning, the final dataset for modeling the distribution of Olea europaea subsp. cuspidata in this study consisted of 776 georeferenced points.
We used the current climatic factors from the WorldClim database, version 1.4 (Hijmans et al., 2005) (http://www.worldclim.org) to estimate the global current potential distribution of Olea europaea subsp. cuspidata. Between 1950 and 2000, these climatic variables indicated the minimum, maximum, and average values of monthly, quarterly, and annual ambient temperatures and precipitation data. These environmental variables had a spatial resolution of 2.5 arc seconds (~4.5 km resolution at the equator). These bioclimatic variables often show a higher degree of collinearity, resulting in poor misleading model performance (Ahmad et al., 2019a). As a result, we used Pearson's correlation analysis to choose only one variable from each pair of strongly associated variables with a correlation coefficient (i.e., r > 0.75 or −0.75) before modeling. A total of 10 variables were retained after correlation analysis for modeling the distribution of target species under present climate conditions (Table 1). The Intergovernmental Panel on Climate Change (IPCC) fifth assessment report (AR5) (Moss, 2010) provided Hadley Global Environment Model 2-Earth System (HADGEM2-ES) simulations for two representative concentration pathways (RCP4.5 and RCP8.5) for the two time periods (i.e., 2050 and 2070) for predicting the future potential distribution of the Olea europaea subsp. cuspidata. RCPs provide climate change trajectories by describing scenarios based on assumptions about socio-economic conditions, greenhouse gas emissions, and the concentration of air pollutants (Albuquerque et al., 2019). The same set of environmental variables used to estimate the current distribution of the examined species was also used to predict their future distributions.
Table 1. Bioclimatic variables selected for modeling the distribution of Olea europaea subsp. cuspidata in the present study.
We performed the ensemble distribution modeling using the nine algorithms implemented in the biomod2 package (Thuiller et al., 2009, 2020). We performed the ensemble distribution modeling using the nine algorithms as implemented in the biomod2 package (Thuiller et al., 2009, 2020), which include: the Generalized Linear Model (GLM) (McCullagh and Nelder, 1989), the Generalized Additive Models (GAM) (Hastie and Tibshirani, 1990), Generalized Boosted Models (GBM) (Ridgeway, 1999), Classification Tree Analysis (CTA) (Breiman et al., 1984), Flexible Discriminant Analysis (FDA) (Hastie et al., 1994), Artificial Neural Networks (ANN) (Ripley, 1996), Maximum Entropy (MAXENT) (Phillips et al., 2006), Random Forest (RF) (Breiman, 2001), and Surface Response Envelope (SRE) (Busby, 1991). As the different algorithms used for distribution modeling require both presence and absence data types, it is impossible to obtain the actual absence data throughout the study region. Therefore, in this study, we randomly generated an equal number of pseudo-absences to that of presence points within the study area, as recommended by Barbet-Massin et al. (2012) and Guisan et al. (2017). We built the models using 80% of the data (training set) and evaluated the model performance with the rest of the 20% of the data (evaluation set). We generated each of the models three times. We preferred two evaluation metrics to evaluate the accuracy of the models: the area under the curve (AUC) of receiver operating characteristics (ROC) and true skills statistics (TSS) (Allouche et al., 2006; Rather et al., 2022; Wani et al., 2022a). We built the final ensemble models for each climate scenario and time period based on both the committee averaging and weighted mean separately; finally, for creating the final ensemble models, only those models with a TSS score of ≥0.8 were used.
To assess the relative impact of each climate condition in determining the distribution of selected plant species, we employed the permutation approach (Elith et al., 2005). Predictions are made from a particular algorithm after changing only one target variable, while the rest of the variables are maintained statically in this method. The variable relevance estimations are obtained as the difference between the original forecast and the permuted variable prediction divided by one minus the correlation score (1-correlation score) (Ahmad et al., 2019a). As a result, high values indicate that the predictor variable is more important in the model, while a value of 0 indicates that the variable is not important in the model.
In order to visualize and measure the range change of the target plant species under future climatic conditions, we used the same biomod range size function as that implemented in the biomod2 package (Guisan et al., 2017). This function provides a summary statistic on species range change, and the prediction map shows the gain or loss of suitable conditions for the studied plant species, according to Guisan et al. (2017). Interestingly, detailed information on four absolute metrics related to “species loss” (i.e., loss of suitable habitat by the studied species under future climate change), “species absence” (i.e., amount of area not occupied by the studied species under current and future climatic scenarios), “stable” (i.e., the amount of area occupied by the studied species both under current and future climatic scenarios) and “gain” (i.e., a gain of suitable habitat by the studied species under future climate change) can be obtained (Guisan et al., 2017). Lastly, from the above four absolute metrics, three additional relative metrics can be calculated, including “percentage loss” (i.e., percentage of currently suitable areas predicted to be lost and is calculated as [loss/(loss + stable)]; “percentage gain” (i.e., percentage of new habitats predicted to be suitable when compared with the species' current distribution size and is calculated as [gain/(loss + stable)]; and “range change,” i.e., the overall output of predictions and is calculated as (percentage gain-percentage loss) (Kumari et al., 2022; Wani et al., 2022b).
The final ensemble models developed had an AUC of 0.991 and a TSS of 0.913 in terms of committee averaging. Similarly, the ensemble models developed had an AUC of 0.992 and a TSS of 0.908 in terms of weighted mean. Both of these scores indicate that our final model had predicted the distribution of the Olea europaea subsp. cuspidata with higher accuracy. When evaluated against the individual algorithms, the predictive accuracy was again excellent but varied, with RF, GBM, GLM, and GLM performing fairly well, followed by FDA, CTA, and Maxent. Phillips, when compared to the rest of the algorithms, the SRE and ANN had the lowest accuracy (Figure 1).
Figure 1. For Olea europaea subsp. cuspidata, mean model evaluation scores by algorithms according to two separate evaluation metrics, ROC (AUC) and TSS.
The performance of the selected bioclimatic variables varied significantly among the different algorithms (Table 2). In particular, the most significant variables governing the distribution of Olea europaea subsp. cuspidata were BIO-01 (annual mean temperature) with importance scores ranging from 0.13 (in the case of RF) to 0.86 (in the case of GLM) (mean score = 0.46), followed by BIO-05 (max temperature of warmest month) with importance scores ranging from 0.02 (for GBM) to 0.43 (for GLM) (mean score = 0.20) and BIO-03 (Isothermality) with importance scores ranging from 0.08 (for GAM) to 0.31 (for MAXENT.Phillips) (mean score = 0.17). The rest of the variables had diverse responses over different algorithms, therefore their influence on governing Olea europaea subsp. cuspidata potential distribution was extremely variable (Table 2).
Table 2. The relevance scores of the selected bioclimatic variables, both overall and by the algorithm.
The final ensemble model reveals that under current climatic conditions, the areas having highly suitable and optimal climatic conditions for the growth of Olea europaea subsp. cuspidata are majority parts of Mexico, the southeastern part of the United States in North America; coastal parts of Columbia, Ecuador, and Peru, major parts of Chile, central, eastern and southern parts of Argentina, majority of Uruguay, and southern and eastern parts of Brazil in South America; coastal parts of western Sahara, Morocco, Libya and Egypt, majority of Ethiopia, Kenya, almost entire of Zimbabwe, Namibia, Botswana, Madagascar, and South Africa in Africa; western coastal parts of Portugal, Spain, France, United Kingdom, Italy, and Turkey in Europe; coastal parts of Yemen and Saudi Arabia, the southeastern part of Pakistan, North Western Himalayan states of Jammu and Kashmir, Himachal Pradesh, Uttarakhand, and Arunachal Pradesh, Bhutan and Nepal, Northwestern part of Myanmar, the northern part of Thailand, the southern part of China in Asia; Central, southern, eastern, western and southern western parts of Australia and major parts of New Zealand in Australasia (Figure 2).
Figure 2. Plot showing the geographic projections using the committee average (above) and weighted mean (below) ensemble models for Olea europaea subsp. cuspidata under current climatic conditions.
The predictions of the future ensemble models showed that there will be a decrease in the habitat suitability for Olea europaea Subsp. cuspidata under all the future climatic scenarios. However, some of the currently suitable areas will consistently remain suitable in future climates also, such as the central part of Mexico, southern and central parts of the United States in North America; coastal parts of Columbia, Ecuador, and Peru, and major parts of Chile, central, eastern and southern parts of Argentina, southern and eastern parts of Brazil in South America; certain parts of Ethiopia, Kenya and Tanzania, eastern Madagascar and Southern parts of Namibia and South Africa in Africa; major parts of Spain, France, United Kingdom, Italy Germany, and Turkey in Europe; North Eastern Himalayan states of Jammu and Kashmir, Himachal Pradesh, Uttarakhand, and Arunachal Pradesh, Bhutan and Nepal, Northwestern part of Myanmar, the northern part of Thailand in Asia; southern parts of Australia and entire of New Zealand in Australasia (Figure 3).
Figure 3. Plots of the predicted habitat suitability for Olea europaea subsp. cuspidata under future climate change scenarios.
The results of the range change analysis once again indicated that Olea europaea subsp. cuspidata will undergo significant range changes under future climatic conditions and ranges from −40.52 and −42.11% under RCP4.5 2050 for committee averaging and weighted mean, respectively, to −56.16 and −60.80% under RCP8.5 2070 for committee averaging and weighted mean, respectively (Table 3). This range change will be governed mostly by habitat loss in future climatic scenarios. More specifically, there will be a reduction in suitable areas for Olea europaea Subsp. cuspidata by about 50.05 and 49.99% (under RCP4.5 2050), 54.20 and 55.04% (RCP4.5 2070), 55.90 and 56.52% (RCP8.5 2050), and 69.17 and 71.11% (under RCP8.5 for the year 2070) for committee averaging and weighted mean, respectively, when compared to current habitat suitability (Table 3). The areas that are likely to become unsuitable in the future include the majority parts of Mexico and the southeastern part of the United States in North America; Northern Chile, east and central parts of Argentina, certain parts of southern and eastern Brazil in South America; coastal parts of Ecuador and Columbia, central parts of Ethiopia, Kenya and Tanzania, parts of Madagascar and central parts of Namibia and South Africa in Africa; coastal parts of Spain, France, Italy and Greece in Europe; coastal parts of Yemen and Saudi Arabia, the southeastern part of Pakistan and southern part of China in Asia; southwestern and eastern parts of Australia (Figure 4). In contrast, some of the currently unsuitable areas become increasingly suitable for future climate with a range expansion of 9.53 and 7.88% (under RCP4.5 2050), 10.61 and 8.58% (RCP4.5 2070), 11.65 and 9.63% (RCP8.5 2050), and 13.01 and 10.31% (under RCP8.5 2070) for committee averaging and weighted mean, respectively, when compared to current habitat suitability for (Table 3). These expanding suitability areas include a certain portion of the southern United States, most of the parts of Chile, some parts of Indian Himalayan states, North Eastern Himalayan states of Jammu and Kashmir, Uttarakhand, Sikkim, and Arunachal Pradesh, as well as certain parts of Bhutan and Nepal and southern China (Figure 4).
Table 3. Summary of the range change statistics in terms of pixels for the Olea europaea subsp. cuspidata under climate change scenarios compared to current climatic conditions.
Figure 4. Plots of the predicted range changes for Olea europaea subsp. cuspidata between current and future climatic conditions.
This study employed two evaluation metrics to assess the predictive performance of our model run: threshold-independent Area under the Curve (AUC) and threshold-dependent True Skill Statistics (TSS). These criteria are frequently used in ecology to evaluate habitat modeling performance (Allouche et al., 2006). The AUC is extensively used to evaluate the accuracy of habitat suitability models, while TSS normalizes total accuracy (Allouche et al., 2006; Becerra-López et al., 2017). The threshold-independent AUC and threshold-dependent TSS performance scores for Olea europaea subsp. cuspidata were 0.991 and 0.913 for committee averaging and 0.992 and 0.908 for weighted mean, respectively. The consistent results obtained prove the model's improved performance.
Environmental conditions influence the distribution of a plant species. This impact is because climate variables such as temperature and precipitation impact species' physiological and reproductive capabilities (Sharma and Raghubanshi, 2006). Several critical biological processes of a species are synchronized by these climate-based characteristics, including dispersion ability, home range size (Bradley and Mustard, 2006), and the ability to survive under unfavorable conditions (Morris et al., 2019). However, this does not hold true for all cases, as other edaphic and topographic factors and interactions among the biotic and abiotic environments significantly impact the species distribution (Norberg et al., 2019). However, when the modeling is performed over large-scale areas such as the whole globe or continents, climatic conditions are reported as the sole determinants for evaluating the degree of distribution of the organisms (Waltari et al., 2014). Earlier SDM studies conducted on Olea europaea subsp. cuspidata in Asia have reported a loss of suitable habitat, particularly in low elevations, and a shifting distribution toward high altitudes (Ashraf et al., 2016, 2017). The other two wild olive subspecies, O. europaea subsp. europaea var. sylvestris, are predicted to increase habitat suitability, while O. europaea subsp. maroccana shows substantial contraction in future climate (Kassout et al., 2022). This suggests that plant species behave differently in future climate projections, even at the subspecies level.
The species' distribution range reflects its adaptation to the Mediterranean-type climate of the world. Such a climate is considered one of the most vulnerable zones to global warming (Almeida et al., 2022; Kassout et al., 2022). The current distribution range is stretched across many regions of all the continents, especially prominent parts of the southern hemisphere. During the last few decades, 90 percent of the net global ocean heat gain was concentrated in the southern hemisphere (Rathore et al., 2018). Several meteorological alterations have been observed in the southern hemisphere during the past several decades (Solman and Orlanski, 2016). Certain precipitation-related variables influencing this plant species' distribution have been identified (Deblauwe et al., 2016; Amiri et al., 2020). The distribution of this plant in coastal parts of Western Sahara, Morocco, Libya, and Egypt, the majority of Ethiopia, Kenya, and almost the entire country of Zimbabwe, Namibia, Botswana, Madagascar, and South Africa in Africa reflects its adaptability in the relatively low precipitation-dominated area. These findings are consistent with model-based predictions made by several other researchers, who identified temperature and precipitation-derived variables as the primary determinants of plant distribution (Woodward et al., 2004; Priyanka and Joshi, 2013; Manzoor et al., 2018; Panda and Behera, 2018; Thapa et al., 2018; Xu et al., 2021).
The study is a pioneering but crucial step in looking into the impact of climate change on the current distribution and the possible range of suitable habitats of Olea europaea subsp. cuspidata on a global scale. The study's findings show that temperature-related bioclimatic variables play a vital role in this species' distribution (Figures 2, 3), with BIO-1 (Annual Mean Temperature) being the most important explanatory variable, followed by BIO-5 (Max Temperature of Warmest Month) and BIO-3 (Annual Mean Temperature) (Isothermality). These variables are expected to alter significantly under the RCP8.5 scenario, causing substantial portions of the current distribution area to become unsuitable by 2050. This study predicts more range contraction under RCP8.5 by 2070, a comparatively more extreme scenario. On the other hand, rainfall patterns in many regions of the world are expected to result in a more widespread variable and extended dry spells. According to a recent climate precipitation trend analysis for the previous few decades, the total amount of annual precipitation is escalating along with increasing trends in consecutive dry days (CDD) (Mudelsee, 2018; Parey, 2019; Dad et al., 2021). Under the predicted importance of rainfall patterns for Olea europaea subsp. cuspidata, future extreme climate events may put additional stress on already established populations and restrict its spread to places that would be unsuitable under current climatic conditions (Kelly and Goulden, 2008).
Future climate change is expected to impair total habitat suitability for this species, with more than a quarter of suitable habitat predicted to be lost by 2070 under RCP8.5. In fact, our findings are consistent with other reports that have found a decline in the worldwide and regional species distribution as a result of climate change (Rabasa et al., 2013; Panda and Behera, 2018; Moraira et al., 2020; Zu et al., 2021). In a recent study investigating the future predictions for the spread of invasive species under the influence of climate change in South Africa, Bezeng et al. (2017) discovered a range reduction for more than 80 species. Our predicted model for the future distribution of this species corresponds with the results of Ghafoor et al. (2021), which experimentally established the reduced seed germination and vegetative growth in Olea europaea subsp. cuspidate, and predicted that climate change will significantly influence olive ecophysiology, leading to species composition changes and shifting distribution (Brito et al., 2019). In the southeast of Australia, where the species is predicted to lose its habitat substantially, vegetation type has been demonstrated to have a substantial impact on fire response to warmer temperatures, with wetter, coastal temperate forests being more likely to experience increased fire frequency (Bradstock et al., 2014). Similar observations were recorded about the distribution pattern of 292 naturalized alien species in Australia, which has been observed to be affected under future climate change scenarios (Duursma et al., 2013). Several cases of vegetation and disturbance regimes, local plant extinctions, phenological changes in reproduction, and altered biotic interactions have been observed in Australia, posing a threat to communities and endangered species (Hoffmann et al., 2018). Another most affected region in terms of habitat loss is Olea europaea subsp. cuspidata is predicted to be in sub-Saharan Africa. According to projections, countries like South Africa, Namibia, and Botswana will face temperatures greater than the global average by 2050 (Davis-Reddy and Vincent, 2017). In addition, rainfall in Southern Africa is anticipated to drop by nearly 10% by 2050 (IPCC, 2014). Our results agree with several other studies which have found that climate change has affected the habitat suitability of several other plant species (Bradley et al., 2009; Taylor et al., 2012; Wan et al., 2016; Manzoor et al., 2018). The availability of moisture and the low temperatures associated with these regions may promote future distribution feasible in the context of climate change (Chen et al., 2011; Fei et al., 2017; Panda and Behera, 2018; Rathore et al., 2018). While projected climate change may negatively shift the distribution of Olea europaea subsp. cuspidate, due to increased precipitation and temperature, moisture availability, and low temperatures associated with higher latitudes, it may also facilitate its distribution under future climate conditions. Since the majority of Olea europaea subsp. cuspidata habitat loss is predicted in the mid-latitudes of both hemispheres; the region's surface temperature changes (Tamarin-Brodsky et al., 2020) explain the warming patterns and climatic-dependent factors.
A considerable reduction of the suitable habitats for Olea europaea subsp. cuspidata will occur under future climatic conditions. As a global trend, the lower the latitude, the more areas will be lost (Sheldon, 2019); the majority of the lost areas are on the American, African, and Australian subcontinents, indicating that many mainland areas will be subjected to more severe climatic changes than coastal areas. The areas predicted for future distribution suitability currently receive less precipitation than those with current distribution. Future climatic projections foresee the emergence of new suitable areas, mostly found at higher latitudes in both the northern and southern hemispheres (Figure 4). Under the model output, such projected latitudinal migration, with Olea europaea subsp. cuspidata shifting poleward, follows one of the most common and well-documented consequences of climate change on species distribution (VanDerWal et al., 2012; Telwala et al., 2013; Rathore et al., 2018). Considering the species diverse distribution patterns and relatively wide temperature and precipitation habitat ranges (Figure 3), future estimates suggest that the plant will be severely affected by the ongoing global climate change. Other widespread plant species have exhibited probable future range contractions (Kelly and Goulden, 2008; Parmesan and Hanley, 2015; Madani et al., 2018) due to increased temperatures leading to severe heat stress (Tollefson, 2020).
The present work is the first attempt to assess global range shifts not only for a wild olive species but also for a Lamiales species in a climate change scenario. Ecophysiological features of Olea europaea subsp. cuspidata may be linked to the shrinkage of potential areas in the year 2070 compared to the present. The extraction and comparison of climatic values of gained and lost areas in relation to present and future distribution ranges revealed that Olea europaea subsp. cuspidata would tend to be displaced to locations with currently low precipitation values. As previously stated, the species appears to be unable to endure significant temperature fluctuations (Bio-1, Bio-3, and Bio-5). As a result, climatically appropriate areas for Olea europaea subsp. cuspidata are predicted to be drastically reduced by 2070, as a portion of the current potential ranges will become unsuitable due to excessive temperature changes. This is in line with worldwide trend changes, which have been anticipated to include a large increase in extreme periods of lengthy dry cycles (IPCC, 2013; Tollefson, 2020). Finally, we investigated where most suitable habitats will be lost or gained in the future due to climate change. With this ensemble modeling approach, firstly, we can predict the main current locations of Olea europaea subsp. cuspidata will still appear as suitable. Secondly, it is estimated that several diverse geographical locations where the species has not been documented will be potential colonization sites for the species in the future. Considering the ecological and economical importance, the areas predicted to be suitable for the O. europaea subsp. cuspidata may be used for the plantation of this species, while the deforested land should be restored for human welfare.
The raw data supporting the conclusions of this article will be made available by the authors, without undue reservation.
SK wrote and analyzed the manuscript. SV supervised, visualized, reviewed, and edited the manuscript. Both authors contributed to the article and approved the submitted version.
The authors appreciate the financial support from the National Mission on Himalayan Studies (NMHS) under Fellowship Grant No. GBPNI/NMHS-2018-19/HSF-24-02/153.
The authors are grateful to the Head Department of Botany, BGSB University, Rajouri, for providing necessary facilities.
The authors declare that the research was conducted in the absence of any commercial or financial relationships that could be construed as a potential conflict of interest.
All claims expressed in this article are solely those of the authors and do not necessarily represent those of their affiliated organizations, or those of the publisher, the editors and the reviewers. Any product that may be evaluated in this article, or claim that may be made by its manufacturer, is not guaranteed or endorsed by the publisher.
Ahmad, R., Khuroo, A. A., Charles, B., Hamid, M., Rashid, I., and Aravind, N. A. (2019a). Global distribution modelling, invasion risk assessment and niche dynamics of Leucanthemum vulgare (Ox-eye Daisy) under climate change. Sci. Rep. 9, 476–485. doi: 10.1038/s41598-019-47859-1
Ahmad, R., Khuroo, A. A., Hamid, M., Charles, B., and Rashid, I. (2019b). Predicting invasion potential and niche dynamics of Parthenium hysterophorus (Congress grass) in India under projected climate change. Biod. Conser. 28, 2319–2344. doi: 10.1007/s10531-019-01775-y
Albuquerque, D., Macías-Rodríguez, F. S., Búrquez, M. A., and Astudillo-Scalia, Y. (2019). Climate change and the potential expansion of buffelgrass (Cenchrus ciliaris L., Poaceae) in biotic communities of Southwest United States and northern Mexico. Biol. Invas. 21, 3335–3347. doi: 10.1007/s10530-019-02050-5
Allouche, O., Tsoar, A., and Kadmon, R. (2006). Assessing the accuracy of species distribution models: prevalence, kappa and the true skill statistic (TSS). J. Appl. Ecol. 43, 1223–1232. doi: 10.1111/j.1365-2664.2006.01214.x
Almeida, A. M., Martins, M. J., Campagnolo, M. L., Fernandez, P., Albuquerque, T., Gerassis, S., et al. (2022). Prediction scenarios of past, present, and future environmental suitability for the Mediterranean species Arbutus unedo L. Sci. Rep. 12, 84. doi: 10.1038/s41598-021-03996-0
Alvarado-Serrano, D. F., and Knowles, L. L. (2014). Ecological niche models in phylogeographic studies: applications, advances and precautions. Mol. Ecol. Resour. 14, 233–248. doi: 10.1111/1755-0998.12184
Amiri, M., Tarkeshi, M., Jafari, R., and Jetschke, G. (2020). Bioclimatic variables from precipitation and temperature records vs. remote sensing-based bioclimatic variables: which side can perform better in species distribution modeling. Ecol. Inform. 57, 1–16. doi: 10.1016/j.ecoinf.2020.101060
Araujo, M. B., and Rahbek, C. (2006). How does climate change affect biodiversity? Science 313, 1396–1397. doi: 10.1126/science.1131758
Ashraf, U. H, Ali, M. N., Chaudry, I., Ashraf, A., and Batool Saqib, Z. (2016). Predicting the potential distribution of Olea ferruginea subsp. cuspidata in Pakistan incorporating climate change by using Maxent model. Sustainability 8, 722. doi: 10.3390/su8080722
Ashraf, U., Peterson, A. T., Chaudhry, M. N., Ashraf, I., Saqib, Z., Rashid Ahmad, S., et al. (2017). Ecological niche model comparison under different climate scenarios: a case study of Olea spp. in Asia. Ecosphere 8, e01825. doi: 10.1002/ecs2.1825
Barbet-Massin, M., Jiguet, F., Albert, C. H., and Thuiller, W. (2012). Selecting pseudo-absences for species distribution models: how, where and how many? Meth. Ecol. Evol. 3, 327–338. doi: 10.1111/j.2041-210X.2011.00172.x
Beaumont, L. J., Pitman, A. J., Poulsen, M., and Hughes, L. (2007). Where will species go? Incorporating new advances in climate modelling into projections of species distributions. Glob. Chang. Biol. 13,1368-1385. doi: 10.1111/j.1365-2486.2007.01357.x
Becerra-López, J., Ramírez-Bautista, A., Romero-Méndez, U., Pavón, N., and Sánchez-Rojas, G. (2017). Effect of climate change on halophytic grasslands loss and its impact in the viability of Gopherus flavomarginatus. Nat. Conserv. 21, 39–55. doi: 10.3897/natureconservation.21.13614
Bellard, C., Bertelsmeier, C., Leadley, P., Thuiller, W., and Courchamp, F. (2012). Impacts of climate change on the future of biodiversity. Ecol. Lett. 15, 365–377. doi: 10.1111/j.1461-0248.2011.01736.x
Besnard, G., Dupuy, J., Larter, M., Cuneo, P., Cooke, D., and Chikhi, L. (2014). History of the invasive African olive tree in Australia and Hawaii: evidence for sequential bottlenecks and hybridization with the Mediterranean olive. Evol. App. 7, 195–211. doi: 10.1111/eva.12110
Bezeng, B. S., Morales-Castilla, I., Van der Bank, M., Yessoufou, K., Daru, B. H., and Davies, T. J. (2017). Climate change may reduce the spread of non-native species. Ecosphere 8, e01694. doi: 10.1002/ecs2.1694
Bradley, B. A., and Mustard, J. F. (2006). Characterizing the landscape dynamics of an invasive plant and risk of invasion using remote sensing. Ecol. Appl. 16, 1132–1147. doi: 10.1890/1051-0761(2006)016[1132:CTLDOA]2.0.CO;2
Bradley, B. A., Oppenheimer, M., and Wilcove, D. S. (2009). Climate change and plant invasions: restoration opportunities ahead? Glob. Chang. Biol. 15, 1511–1521. doi: 10.1111/j.1365-2486.2008.01824.x
Bradstock, R., Penman, T., Boer, M., Price, O., and Clarke, H. (2014). Divergent responses of fire to recent warming and drying across south-eastern Australia. Glob. Chang. Biol. 20, 1412–1428. doi: 10.1111/gcb.12449
Breiman, L., Friedman, J. H., Olshean, R. A., and Stone, C. J. (1984). Classification and Regression Trees. Belmont, CA: Wadsworth.
Brito, C., Dinis, L. T., Moutinho-Pereira, J., and Correia, C. M. (2019). Drought stress effects and olive tree acclimation under a changing climate. Plants 8, 232. doi: 10.3390/plants8070232
Busby, J. R. (1991). “BIOCLIM—a bioclimate analysis and prediction system,” in Nature Conservation: Cost Effective Biological Surveys and Data Analysis, eds Margules, C. R., and Austin, M. P. (Canberra: CSIRO), 64–68.
Butchart, S. H. M., Walpole, M., Collen, B., van Strien, A., Scharlemann, J. P. W., Almond, R. E. A., et al. (2010). Global biodiversity: indicators of recent declines. Science 328, 1164–1168. doi: 10.1126/science.1187512
Chen, I.-C., Hill, J. K., Ohlemuller, R., Roy, D. B., and Thomas, C. D. (2011). Rapid range shifts of species associated with high levels of climate warming. Science 333, 1024–1026. doi: 10.1126/science.1206432
Convention on Biological Diversity (2020). Convention on Biological Diversity Zero Draft of the Post-2020 Global Biodiversity Framework. Convention on Biological Diversity.
Cook, B. I., Wolkovich, E. M., Davies, T. J., Ault, T. R., Betancourt, J. L., Allen, J. M., et al. (2012). Sensitivity of spring phenology to warming across temporal and spatial climate gradients in two independent databases. Ecosystems 15, 1283–1294. doi: 10.1007/s10021-012-9584-5
Dad, J. M., Muslim, M., Rashid, I., Rashid, I., and Reshi, Z. A. (2021). Time series analysis of climate variability and trends in Kashmir Himalaya. Ecol. Indicat. 126, 107690. doi: 10.1016/j.ecolind.2021.107690
Davis-Reddy, C. L., and Vincent, K. (2017). Climate Risk and Vulnerability: A Handbook for Southern Africa, Second Edn. Pretoria: CSIR.
De Ollas, C., Morillón, R., Fotopoulos, V., Puértolas, J., Ollitrault, P., Gómez-Cadenas, A., et al. (2019). Facing climate change: biotechnology of iconic Mediterranean woody crops. Front. Plant Sci. 10. doi: 10.3389/fpls.2019.00427
Deblauwe, V., Droissart, V., Bose, R., Sonké, B., Blach-Overgaard, A., Svenning, J. C., et al. (2016). Remotely sensed temperature and precipitation data improve species distribution modeling in the tropics. Glob. Ecol Biogeogr. 25, 443–454. doi: 10.1111/geb.12426
Duursma, D. E., Gallagher, R. V., Roger, E., Hughes, L., Downey, P. O., and Leishman, M. R. (2013). Next-generation invaders? Hotspots for naturalised sleeper weeds in Australia under future climates. PLoS ONE 8, e84222. doi: 10.1371/journal.pone.0084222
Elith, J., Ferrier, S., Huettmann, F., and Leathwick, J. (2005). The evaluation strip: a new and robust method for plotting predicted responses from species distribution models. Ecol. Model. 186:280–289. doi: 10.1016/j.ecolmodel.2004.12.007
Elith, J., Kearney, M., and Phillips, S. (2010). The art of modelling range-shifting species. Methods Ecol. Evol. 1, 330–342. doi: 10.1111/j.2041-210X.2010.00036.x
Fei, S., Desprez, J. M., Potter, K. M., Jo, I., Knott, J. A., and Oswalt, C. M. (2017). Divergence of species responses to climate change. Sci. Adv. 3, e1603055. doi: 10.1126/sciadv.1603055
GBIF (2021). Available online at: http://www.gbif.org (accessed July 15, 2021).
Ghafoor, G. Z., Sharif, F., Khan, M. U. H., Shahid, M. G., Siddiq, Z., and Shahzad, L. (2021). Effect of climate warming on seedling growth and biomass accumulation of Acacia modesta and Olea europaea Subsp. cuspidata in a subtropical scrub forest of Pakistan. Ecoscience. 29, 133–146. doi: 10.1080/11956860.2021.1958536
Gillard, M., Thiébaut, G., Deleu, C., and Leroy, B. (2017). Present and future distribution of three aquatic plants taxa across the world: decrease in native and increase in invasive ranges. Biol. Invas. 19, 59–2170. doi: 10.1007/s10530-017-1428-y
Guisan, A., Thuiller, W., and Zimmermann, N. E. (2017). Habitat Suitability and Distribution Models With Applications in R. Cambridge: Cambridge University Press. doi: 10.1017/9781139028271
Hastie, T., Tibshirani, R., and Buja, A. (1994). Flexible discriminant analysis by optimal scoring. J. Am. Stat. Assoc. 89, 1255–1270. doi: 10.1080/01621459.1994.10476866
Hijmans, R. J., Cameron, S. E., Parra, J. L., Jones, P. G., and Jarvis, A. (2005). Very high resolution interpolated climate surfaces for global land areas. Int. J. Clim. 25, 1965–1978. doi: 10.1002/joc.1276
Hijmans, R. J., Phillips, S., Leathwick, J., and Elith, J. (2020). dismo: Species Distribution Modeling. R package version 1.3-3. Available online at: https://CRAN.R-project.org/package=dismo (accessed August 01, 2021).
Hoffmann, A. A., Rymer, P. D., Byrne, M., Ruthrof, K. X., Whinam, J., McGeoch, M., et al. (2018). Impacts of recent climate change on terrestrial flora and fauna: some emerging Australian examples. Aust. Ecol. 44, 3–27. doi: 10.1111/aec.12674
IPCC (2013). Climate Change 2013: The Physical Science Basis. Cambridge: Cambridge University Press.
IPCC (2014). “Summary for policymakers,” in Climate Change 2014: Impacts, Adaptation, and Vulnerability. Part A: global and sectoral aspects. Contribution of Working Group II to the Fifth Assessment Report of the Intergovernmental Panel on Climate Change, eds Field et al. (Cambridge and New York: Cambridge University Press).
IPCC (2019). Climate Change and Land: An IPCC Special Report on Climate Change, Desertification, Land Degradation, Sustainable Land Management, Food Security, and Greenhouse Gas Fluxes in Terrestrial Ecosystems, eds Shukla, P. R., Skea, J., Calvo Buendia, E., Masson-Delmotte, V., Pörtner, H.-O., Roberts, D. C., et al. (Cambridge: Cambridge University Press).
IPCC (2021). “Summary for policymakers,” in Climate Change 2021: The Physical Science Basis. Contribution of Working Group I to the Sixth Assessment Report of the Intergovernmental Panel on Climate Change, eds Masson-Delmotte, V., Zhai, P., Pirani, A., Connors, S. L., Péan, C., Berger, S., Caud, N., et al. (Cambridge: Cambridge University Press).
IPCC. (2012). “Managing the risks of extreme events and disasters to advance climate change adaptation,” in A Special Report of Working Groups I and II of the Intergovernmental Panel on Climate Change, eds C. B. Field, V. Barros, T. F. Stocker, D. Qin, D. J. Dokken, K. L. Ebi, M. D. Mastrandrea, K. J. Mach, G. -K. Plattner, S. K. Allen, M. Tignor, and P. M. Midgley (Cambridge; New York, NY: Cambridge University Press), p. 582.
Kassout, J., Terral, J. F., Ouahrani, A. E., Houssni, M., Ivorra, S., et al. (2022). “Species distribution based-modelling under climate change: the case of two native wild Olea europaea subspecies in Morocco, O. e. subsp. europaea var. sylvestris and O. e. subsp. Maroccana,” in Climate Change in the Mediterranean and Middle Eastern Region. Climate Change Management, eds Leal Filho, W., Manolas, E. (Cham: Springer). doi: 10.1007/978-3-030-78566-6_2
Kelly, A. E., and Goulden, M. L. (2008). Rapid shifts in plant distribution with recent climate change. Proc. Nat. Acad. Sci. USA 105, 11823–11826. doi: 10.1073/pnas.0802891105
Kumari, P., Wani, I. A., Khan, S., Verma, S., Mushtaq, S., Gulnaz, A., et al. (2022). Modeling of Valeriana wallichii habitat suitability and niche dynamics in the Himalayan Region under anticipated climate change. Biology 11, 498. doi: 10.3390/biology11040498
Long, H. S., Tilney, P. M., and Van Wyk, B. E. (2010). The ethnobotany and pharmacognosy of Olea europaea subsp. africana (Oleaceae). S. Afri. J. Bot. 76, 324–331. doi: 10.1016/j.sajb.2009.12.005
Madani, N., Kimball, J. S., Ballantyne, A. P., Affleck, D. L. R., van Bodegom, P. M., Reich, P. B., et al. (2018). Future global productivity will be affected by plant trait response to climate. Sci. Rep. 8, 2870. doi: 10.1038/s41598-018-21172-9
Maitner, B. S., Boyle, B., Casler, N., Condit, R., Donoghue, J., Durán, S. M., et al. (2018). The BIEN R package: a tool to access the botanical information and ecology network (BIEN) database. Meth. Ecol. Evol. 9, 373–379. doi: 10.1111/2041-210X.12861
Malhi, Y., Franklin, J., Seddon, N., Solan, M., Turner, M. G., Field, C. B., et al. (2020). Climate change and ecosystems: threats, opportunities and solutions. Philos. Trans. R. Soc. B Biol. Sci. 375, 20190104. doi: 10.1098/rstb.2019.0104
Manzoor, S. A., Griffiths, G., and Lukac, M. (2018). Species distribution model transferability and model grain size—finer may not always be better. Sci. Rep. 8, 1–9. doi: 10.1038/s41598-018-25437-1
Masoko, P., and Makgapeetja, D. M. (2015). Antibacterial, antifungal and antioxidant activity of Olea africana against pathogenic yeast and nosocomial pathogens. BMC Comp. Altern. Med. 15. doi: 10.1186/s12906-015-0941-8
McCullagh, P., and Nelder, J. A. (1989). Generalized Linear Models, 2nd edn. London: Chapman and Hall. doi: 10.1007/978-1-4899-3242-6
Moraira, H. S., Vitales, D., Nualart, N., et al. (2020). Global distribution patterns and niche modelling of the invasive Kalanchoe houghtonii (Crassulaceae). Sci. Rep. 10, 3143. doi: 10.1038/s41598-020-60079-2
Morris, W. F., Ehrlén, J., Dahlgren, J. P., Loomis, A. K., and Louthan, A. M. (2019). Biotic and anthropogenic forces rival climatic/abiotic factors in determining global plant population growth and fitness. Proc. Nat. Acad. Sci. USA 2019, 18363. doi: 10.1073/pnas.1918363117
Moss, R. H. (2010). The next generation of scenarios for climate change research and assessment. Nature 463, 747–756. doi: 10.1038/nature08823
Mudelsee, M. (2018). Trend analysis of climate time series: a review of methods. Earth-Sci. Rev. doi: 10.1016/j.earscirev.2018.12.005
Naimi, B. (2015). On uncertainty in Species Distribution Modelling (PhD Dissertation). Twente: University of Twente, ITC.
Negash, L. (2010). A Selection of Ethiopia's Indigenous Trees: Biology, Uses and Propagation Techniques. Addis Ababa: Addis Ababa University Press.
Negi, G. C. S., Singh, P., and Singh, S. P. (2021). Atmospheric warming-associated phenological earliness does not increase the length of growing season in Himalayan Trees. Forest Sci. 67, 694–700. doi: 10.1093/forsci/fxab040
Norberg, A., Abrego, N., Blanchet, F. G., Adler, F. R., Anderson, B. J., Anttila, J., et al. (2019). Comprehensive evaluation of predictive performance of 33 species distribution models at species and community levels. Ecol. Monogr. 89, e01370. doi: 10.1002/ecm.1370
Pacifici, M., Foden, W. B., Visconti, P., Watson, J. E. M., Butchart, S. H. M., Kovacs, K. M., et al. (2015). Assessing species vulnerability to climate change. Nat. Clim Chang. 5, 215–224. doi: 10.1038/nclimate2448
Pacifici, M., Visconti, P., Butchart, S. H. M., Watson, J. E. M., Cassola, F. M., and Rondinini, C. (2017). Species' traits influenced their response to recent climate change. Nat. Clim. Chang. 7, 205–208. doi: 10.1038/nclimate3223
Palmer, G., Hill, J. K., Brereton, T. M., Brooks, D. R., Chapman, J. W., Fox, R., et al. (2015). Individualistic sensitivities and exposure to climate change explain variation in species' distribution and abundance changes. Sci. Adv. 1, e1400220. doi: 10.1126/sciadv.1400220
Panda, R. M., and Behera, M. D. (2018). Assessing harmony in distribution patterns of plant invasions: a case study of two invasive alien species in India. Biodivers. Conserv. 28,2245–2258. doi: 10.1007/s10531-018-1640-9
Parey, S. (2019). Generating a set of temperature time series representative of recent past and near future climate. Front. Envir. Sci. 7, 99. doi: 10.3389/fenvs.2019.00099
Parmesan, C. (2006). Ecological and evolutionary responses to recent climate change. Ecol. Evol. 37, 637–669. doi: 10.1146/annurev.ecolsys.37.091305.110100
Parmesan, C., Duarte, C., Poloczanska, E., Richardson, A. J., and Singer, M. C. (2011). Overstretching attribution. Nat. Clim. Change. 1, 2–4. doi: 10.1038/nclimate1056
Parmesan, C., and Hanley, M. E. (2015). Plants and climate change: complexities and surprises. Ann. Bot. 116, 849–864. doi: 10.1093/aob/mcv169
Pecl, G. T., Araújo, M. B., Bell, J. D., Blanchard, J., Bonebrake, T. C., Chen, I.-C., et al. (2017). Biodiversity redistribution under climate change: impacts on ecosystems and human well-being. Science 355, eaai9214. doi: 10.1126/science.aai9214
Phillips, S. J., Anderson, R. P., and Schapire, R. E. (2006). Maximum entropy modelling of species geographic distributions. Ecol. Model. 190, 231–259. doi: 10.1016/j.ecolmodel.2005.03.026
Porfirio, L. L., Harris, R. M. B., Lefroy, E. C., Hugh, S., Gould, S. F., Lee, G., et al. (2014). Improving the use of species distribution models in conservation planning and management under climate change. PLoS ONE 9, e113749. doi: 10.1371/journal.pone.0113749
Priyanka, N., and Joshi, P. K. (2013). Effects of climate change on invasion potential distribution of Lantana camara. J. Earth Sci. Clim. Chang. 4, 164. doi: 10.4172/2157-7617.1000164
Pyke, C. R., and Fischer, D. T. (2005). Selection of bioclimatically representative biological reserve systems under climate change. Biol. Conserv. 121, 429–441. doi: 10.1016/j.biocon.2004.05.019
Rabasa, S. G., Granda, E., Benavides, R., Kunstler, G., Espelta, J. M., Ogaya, R., et al. (2013). Disparity in elevational shifts of European trees in response to recent climate warming. Glob. Chang. Biol. 19, 2490–2499. doi: 10.1111/gcb.12220
Rather, Z. A., Ahmad, R., Dar, T. U. H., and Khuroo, A. A. (2022). Ensemble modelling enables identification of suitable sites for habitat restoration of threatened biodiversity under climate change: a case study of Himalayan Trillium. Eco. Engin. 176, 106534. doi: 10.1016/j.ecoleng.2021.106534
Rathore, P., Roy, A., and Karnatak, H. (2018). Assessing the vulnerability of Oak (Quercus) forest ecosystems under projected climate and land use land cover changes in Western Himalaya. Biodiver. Conser. 28, 2275–2294. doi: 10.1007/s10531-018-1679-7
Ripley, B. (1996). Pattern Recognition and Neural Networks. New York, NY: Cambridge University Press. doi: 10.1017/CBO9780511812651
Román-Palacios, C., and Wiens, J. J. (2020). Recent responses to climate change reveal the drivers of species extinction and survival. Proc. Nat. Acad. Sci. USA 117, 4211–4217. doi: 10.1073/pnas.1913007117
Schorr, G., Holstein, N., Pearman, P. B., Guisan, A., and Kadereit, J. W. (2012). Integrating species distribution models (SDM) and phylogeography for two species of Alpine Primula. Ecol. Evol. 2, 1260–1277. doi: 10.1002/ece3.100
Sharma, G. P., and Raghubanshi, A. S. (2006). Tree population structure, regeneration and expected future composition at different levels of Lantana camara L. invasion in the Vindhyan tropical dry deciduous forest of India. Lyonia 11, 25–37.
Sheldon, K. S. (2019). Climate change in the tropics: ecological and evolutionary responses at low latitudes. Annu. Rev. Ecolsys. 50, 110218. doi: 10.1146/annurev-ecolsys-110218-025005
Solman, S. A., and Orlanski, I. (2016). Climate change over the extratropical southern hemisphere: The tale from an ensemble of reanalysis datasets. J. Clim. 29, 1673–1687. doi: 10.1175/JCLI-D-15-0588.1
Srivastava, V., Lafond, V., and Griess, V. C. (2019). Species distribution models (SDM): applications, benefits and challenges in invasive species management. CAB Rev. Perspect. Agric. Vet. Sci. Nutr. Nat. Resour. 2019, 1–13. doi: 10.1079/PAVSNNR201914020
Tamarin-Brodsky, T., Hodges, K., Hoskins, B. J., and Shepherd, T. G. (2020). Changes in Northern Hemisphere temperature variability shaped by regional warming patterns. Nat. Geo. doi: 10.1038/s41561-020-0576-3
Taylor, S., Kumar, L., Reid, N., and And Kriticos, D. J. (2012). Climate change and the potential distribution of an invasive shrub, Lantana camara L. PLoS ONE 7, e35565. doi: 10.1371/journal.pone.0035565
Telwala, Y., Brook, B. W., Manish, K., and Pandit, M. K. (2013). Climate-induced elevational range shifts and increase in plant species richness in a himalayan biodiversity epicentre. PLoS ONE 8, e57103. doi: 10.1371/journal.pone.0057103
Thapa, S., Chitale, V., Rijal, S. J., Bisht, N., and Shrestha, B. B. (2018). Understanding the dynamics in distribution of invasive alien plant species under predicted climate change in Western Himalaya. PLoS ONE 13, e0195752. doi: 10.1371/journal.pone.0195752
Thomas, C. D., Cameron, A., Green, R. E., Bakkenes, M., Beaumont, L. J., Collingham, Y. C., et al. (2004). Extinction risk from climate change. Nature 427, 145–148. doi: 10.1038/nature02121
Thuiller, W., Cade, B., Engler, R., and Araújo, M. B. (2009). BIOMOD a platform for ensemble forecasting of species distributions. Ecography. 32, 369–373. doi: 10.1111/j.1600-0587.2008.05742.x
Thuiller, W., Georges, D., Engler, R., and Breiner, F. (2020). biomod2:Ensemble Platform for Species Distribution Modeling. R package version 3.4.6. (Version R package version 3.4.6). Available online at: https://CRAN.R-project.org/package=biomod2
Thuiller, W., Guéguen, M., Renaud, J., Karger, D. N., and Zimmermann, N. E. (2019). Uncertainty in ensembles of global biodiversity scenarios. Nat. Commun. 10, 1–9. doi: 10.1038/s41467-019-09519-w
Tollefson, J. (2020). Why deforestation and extinctions make pandemics more likely. Nature 584, 175–176. doi: 10.1038/d41586-020-02341-1
VanDerWal, J., Murphy, H. T., Kutt, A. S., Perkins, G. C., Bateman, B. L., Perry, J. J., et al. (2012). Focus on poleward shifts in species' distribution underestimates the fingerprint of climate change. Nat. Clim. Chang. 3, 239–243. doi: 10.1038/nclimate1688
Vuuren, D. P., Van Edmonds, J., Kainuma, M., Riahi, K., Nakicenovic, N., Smith, S. J., et al. (2011). The representative concentration pathways: an overview. Clim. Chang. 109, 5–31. doi: 10.1007/s10584-011-0148-z
Waltari, E., Schroeder, R., McDonald, K., Anderson, R. P., and Carnaval, A. (2014). Bioclimatic variables derived from remote sensing: assessment and application for species distribution modelling. Meth. Ecol. Evol. 5, 1033–1042. doi: 10.1111/2041-210X.12264
Wan, J. Z., Wang, C. J., and Yu, F. H. (2016). Risk hotspots for terrestrial plant invaders under climate change at the global scale. Environ. Earth Sci. 75, 1–8. doi: 10.1007/s12665-016-5826-8
Wani, I. A., Khan, S., Verma, S., Al-Misned, F. A., Shafik, H. M., El-Serehy, H. A., et al. (2022a). Predicting habitat suitability and niche dynamics of Dactylorhiza hatagirea and Rheum webbianum in the Himalaya under projected climate change. Sci. Rep. 12, 13205. doi: 10.1038/s41598-022-16837-5
Wani, Z. A., Ridwan, Q., Khan, S., Pant, S., Siddiqui, S., Moustafa, M., et al. (2022b). Changing climatic scenarios anticipate dwindling of suitable habitats for endemic species of himalaya-predictions of ensemble modelling using Aconitum heterophyllum as a model plant. Sustainability. 14, 8491. doi: 10.3390/su14148491
Weiskopf, S. R., Rubenstein, M. A., Crozier, L. G., Gaichas, S., Griffis, R., Halofsky, J. E., et al. (2020). Climate change effects on biodiversity, ecosystems, ecosystem services, and natural resource management in the United States. Sci. Tot. Env. 773, 137782. doi: 10.1016/j.scitotenv.2020.137782
Woodward, F. I., Lomas, M. R., and Kelly, C. K. (2004). Global climate and the distribution of plant biomes. Phil. Trans. R. Soc. B Biol. Sci. 359, 1465–1476. doi: 10.1098/rstb.2004.1525
Xu, Y., Huang, Y., Zhao, H., Yang, M., Zhuang, Y., and Ye, X. (2021). Modelling the effects of climate change on the distribution of endangered Cypripedium japonicum in China. Forests 12, 429. doi: 10.3390/f12040429
Keywords: Olea europaea subsp. cuspidata, species distribution modeling, climate change, global distribution, habitat suitability
Citation: Khan S and Verma S (2022) Ensemble modeling to predict the impact of future climate change on the global distribution of Olea europaea subsp. cuspidata. Front. For. Glob. Change 5:977691. doi: 10.3389/ffgc.2022.977691
Received: 24 June 2022; Accepted: 22 August 2022;
Published: 20 September 2022.
Edited by:
Vikram S. Negi, Govind Ballabh Pant National Institute of Himalayan Environment and Sustainable Development, IndiaReviewed by:
Kailash Gaira, Govind Ballabh Pant National Institute of Himalayan Environment and Sustainable Development, IndiaCopyright © 2022 Khan and Verma. This is an open-access article distributed under the terms of the Creative Commons Attribution License (CC BY). The use, distribution or reproduction in other forums is permitted, provided the original author(s) and the copyright owner(s) are credited and that the original publication in this journal is cited, in accordance with accepted academic practice. No use, distribution or reproduction is permitted which does not comply with these terms.
*Correspondence: Susheel Verma, ZXJlbXVydXNAcmVkaWZmbWFpbC5jb20=; Sajid Khan, c2FqaWRraGFuNzE3QGdtYWlsLmNvbQ==
Disclaimer: All claims expressed in this article are solely those of the authors and do not necessarily represent those of their affiliated organizations, or those of the publisher, the editors and the reviewers. Any product that may be evaluated in this article or claim that may be made by its manufacturer is not guaranteed or endorsed by the publisher.
Research integrity at Frontiers
Learn more about the work of our research integrity team to safeguard the quality of each article we publish.