- Department of Forestry and Natural Resources, Purdue University, West Lafayette, IN, United States
Basal area is a key measure of forest stocking and an important proxy of forest productivity in the face of climate change. Black walnut (Juglans nigra) is one of the most valuable timber species in North America. However, little is known about how the stocking of black walnut would change with differed bioclimatic conditions under climate change. In this study, we projected the current and future basal area of black walnut. We trained different machine learning models using more than 1.4 million tree records from 10,162 Forest Inventory and Analysis (FIA) sample plots and 42 spatially explicit bioclimate and other environmental attributes. We selected random forests (RF) as the final model to estimate the basal area of black walnut under climate change because RF had a higher coefficient of determination (R2), lower root mean square error (RMSE), and lower mean absolute error (MAE) than the other two models (XGBoost and linear regression). The most important variables to predict basal area were the mean annual temperature and precipitation, potential evapotranspiration, topology, and human footprint. Under two emission scenarios (Representative Concentration Pathway 4.5 and 8.5), the RF model projected that black walnut stocking would increase in the northern part of the current range in the USA by 2080, with a potential shift of species distribution range although uncertainty still exists due to unpredictable events, including extreme abiotic (heat, drought) and biotic (pests, disease) occurrences. Our models can be adapted to other hardwood tree species to predict tree changes in basal area based on future climate scenarios.
Introduction
In recent decades, climate change has modified the growth of forests, mainly due to increasing temperature and altered precipitation patterns (Kirilenko and Sedjo, 2007; Li et al., 2015). Several biogeographical models demonstrate a poleward shift of boreal and hardwood tree species distribution at a higher rate than in past glacial times (Solomon and Kirilenko, 1997; Woodall et al., 2009). Due to climate change, some models project a 50% loss in the geographic range of some tree species by 2100 (Iverson et al., 2019), resulting in diversity loss and impact on forest productivity. In addition, rising air temperatures decrease cloud covers and increase solar radiation, which may enhance several critical constraints to plant growth (Li et al., 2015). In eastern U.S. forests, environmental variability owing to climate change could potentially affect temporal changes in species richness, demographic rates, and biomass (Fei et al., 2017; Iverson et al., 2019; Ma et al., 2020). Despite that prediction, more profound knowledge of which climate variables might have the most significant impact on tree growth is needed.
A primary step to predicting the impacts of climate change on tree growth is to understand how a particular species’ performance (growth or dispersal) is influenced by climate variables (Li et al., 2015). Climate, soils, topography, and anthropogenic factors could influence tree growth directly (Ares and Brauer, 2004; Ashraf et al., 2015). Climate change affects soil water content and increases the growing season length (Ashraf et al., 2015). Subsequently, unpredictable environmental factors such as high temperature and severe drought, pests, disease, and anthropogenic activities affect forest ecosystems, transforming forestland into cropland or municipalities. Present-day forests cannot be replaced quickly, especially with significant climate changes that endanger tree habitats and their environments. Consequently, existing forest development should be monitored and measured using efficient models while considering current climate data to predict growth changes and future bioclimatic variables.
Evaluating tree growth is used to establish a norm for forest management, reforestation, harvest time estimation, and prediction of forest production (Ares and Brauer, 2004; Schlamadinger et al., 2007; Da Cunha et al., 2016) since functional traits such as tree growth directly affect its performance under environmental changes (Li et al., 2015). Thus, many forestry programs’ goals are to enhance tree growth and develop more timber (Ares and Brauer, 2004; McKenna and Coggeshall, 2018) and strengthen the adaptability of trees to cope with climate change consequences (Brang et al., 2014). Traditionally, tree growth is predicted by measuring tree height and DBH (Diameter at Breast Height) (Stage, 1975; Myers and Fischer, 1991; Ares and Brauer, 2004; Ercanlı, 2020). However, with increasing forest complexity, quantifying basal area metrics is critical for measuring forest productivity (Schmidt, 1998; Jevšenak and Skudnik, 2021) and predicting future changes as a result of climate change.
Tree growth modeling facilities adaptive forest management in the face of climate change (Iverson et al., 2019). For this type of application, machine learning-based models are often tested to be more accurate than linear regression and other statistical models (Jevšenak et al., 2018; Ma et al., 2020). For basal area (a measure of the cross-sectional area of a single tree at diameter breast height), machine learning was used to develop a model to evaluate the feasibility/capability of machine learning for tree species (Kindermann et al., 2008). Machine learning, especially using random forests, has also been applied to predict basal area, stem density, and habitat or land suitability in order to facilitate adaptive forest management in response to climate change in eastern USA forests (Iverson et al., 2019; Vahdati et al., 2019).
Black walnut (Juglans nigra) is a native species of eastern North America, widespread in various climates of riparian zones from the southern part of Ontario to northern Florida and central Texas. Black walnut is one of the most valuable timber species in North America. It provides habitat to several wildlife species, including squirrels, raccoons, and bears, and it is used for commercial nut production for human consumption (Michler et al., 2009). Black walnut covers 5,600 ha across its range, which constitutes approx. 13% of the forest area (Michler et al., 2009) in the United States. Black walnut is distributed in a broad range of habitats, and its variability in growth can be used as an ideal model to evaluate growth and climate relationship. Black walnut is known for its premium timber quality, with a timber value of 6,500 $/mbf (Log Dia > 28) that dwarfs the value of most other hardwood timber species in North America (Michler et al., 2009; Nicolescu et al., 2020).1 The cost of saw-timber (stumpage) increased from 150$/m3 in 2000 to 480$/m3 in 2020 (Nicolescu et al., 2020). Eleven states currently have the most significant volume of black walnut growing stock on timberland (Shifley, 2004), with 126.7 million m3, 1.0% of total US hardwood growing stock (Nicolescu et al., 2020). The United States is exporting walnut lumber to 67 countries (on average $40 million per year) and walnut logs to 49 countries (on average $37 million per year) (USDA-FAS, 2004) and the net volume is increasing by 3.0 million m3 per year.2
Little is known, however, about the future of stocking of this ecologically and commercially important species under climate change. Black walnut is sensitive to warmer-drier summers and extreme weather events (Gauthier and Jacobs, 2011). In addition, the threat of thousand-canker disease in black walnut may be increased under future climate scenarios (Williams and Ginzel, 2021). Therefore, developing a model to predict the stocking of black walnut with current and future climate data is important for prioritizing adaptive forest management in response to climate change. To do this, we tested different machine-learning models to (i) estimate the current black walnut basal area to identify the important predictor variables and (ii) predict future basal areas under two emission scenarios (RCP 4.5 and 8.5). The findings of this study provide meaningful recommendations/methodology for black walnut species management in the future.
Materials and methods
Tree and forest data
We compiled black walnut basal area data from the Forest Inventory and Analysis (FIA) (Burrill et al., 2018). FIA is a nationwide strategic forest survey, providing periodically collected information on trees and species data from permanent sample plots. The plot design uses 7.30-m radius subplots in which trees with a diameter at breast height (DBH) greater than 12 cm are measured. The age of the trees is not recorded. The data was collected from a 1968 to 2020 cycle of inventory. This study extracted 12,499 plots of black walnut (Juglans nigra) information as the first approach. Black walnut is the only dominant species of the Juglans genus that grows in the eastern forest in the United States and does not hybridize naturally with other native species (Ebrahimi et al., 2019). Black walnut grows almost exclusively in natural forests with plantations (afforestation) making up a small fraction of black walnut growing stock (Shifley, 2004). Black walnut occurs in mixed hardwood stands and typically comprises about 11% of the total volume in stands where it occurs (Shifley, 2004).
Due to management practices, misnamed or overlapped plots were removed from the analysis. Finally, 10,162 plots were used to evaluate the basal area for each plot and inventory year. We calculated the total black walnut basal area (m2/ha) based on DBH. The basal area value outside the 99th percentile range was removed to avoid plots with substantial measurement errors. Dead and harvested trees were excluded, and only growing trees were used for the analysis. We then aggregated the FIA plot data into a hexagon-based map. The hexagons are 2,700 ha in size, covering the entire eastern USA based on the ecoregions defined by Olson et al. (2001) (Supplementary Table 1).
Climate and environmental data
We compiled 42 predictor variables to train machine learning models (see section “Basal area modeling and model evaluation”). We obtained 21 climate variables (Hijmans et al., 2005; Trabucco and Zomer, 2009), 12 topographic variables (Amatulli et al., 2018), five soil variables (Batjes, 2016), and four anthropogenic variables (Venter et al., 2016). These predictor variables are all derived from open-source satellite-based remote sensing and were extracted to the centroids of hexagons. Detailed information about each variable is available in Table 1. The data was extracted using the SP, SF, raster, and rgdal R packages (Bucklin and Basille, 2018).
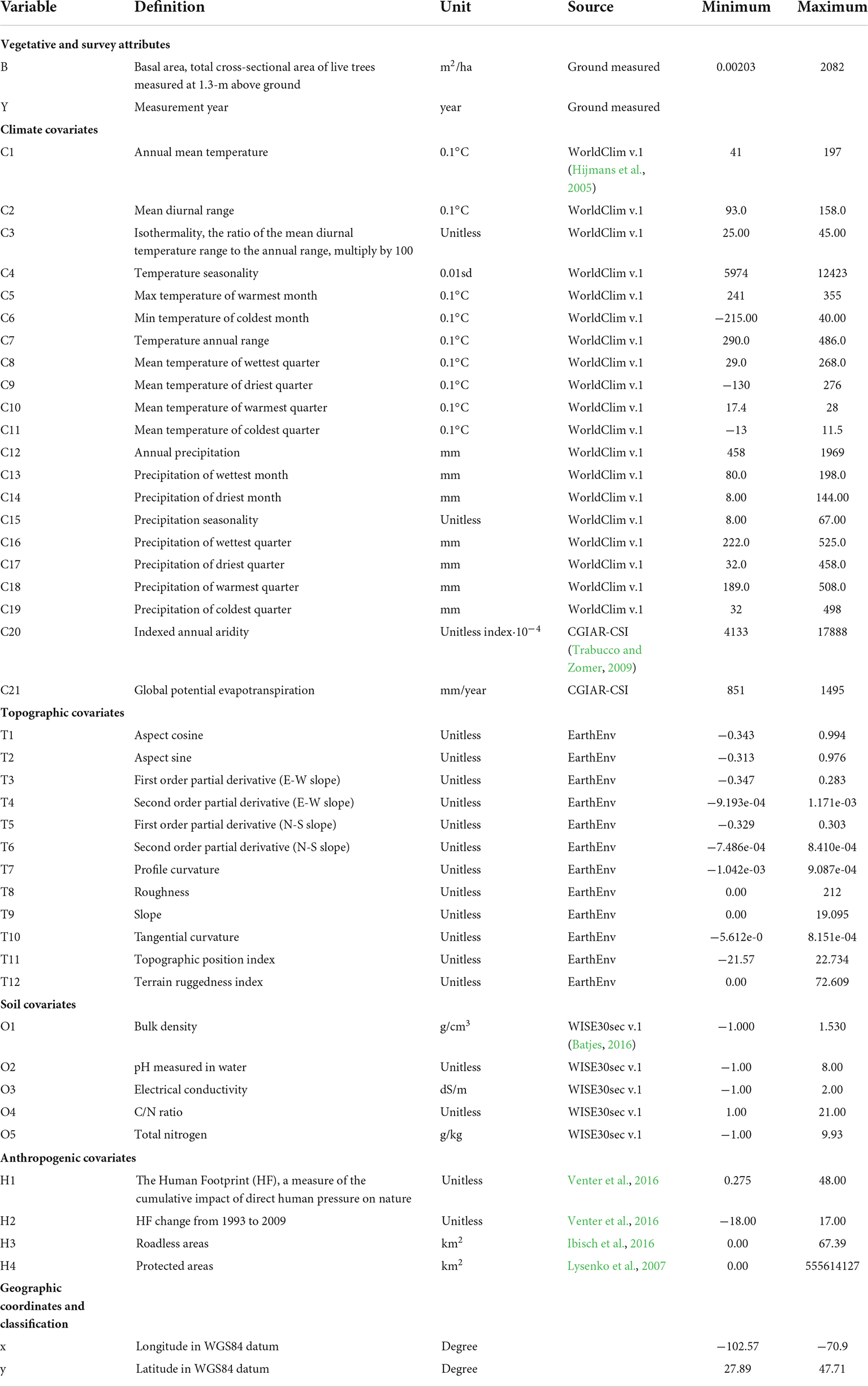
Table 1. Definition and units of the environmental and bioclimatic covariates (Hijmans et al., 2005) extracted to the FIA plot locations.
We also obtained future bioclimate variables to predict future black walnut basal areas. For the 19 bioclimate variables (C1–C19) (Table 1), we obtained predicted values under two emission scenarios [Representative Concentration Pathways (RCP) 4.5 and 8.5] averaged between 2061 and 2080 (Karger et al., 2017). The RCP 4.5 is a stabilization scenario in which total radiative forcing is stabilized shortly after 2100, without overshooting the long-run radiative forcing target level. The RCP 8.5 is characterized by increasing greenhouse gas emissions over time, representing the high greenhouse gas concentration levels (Bernstein et al., 2008). All future bioclimate data are in 1 km-resolution and capture spatially specific trends of future climates. In this study, we took the average of five climate models developed during the phase 5 of CMIP (CMIP5): CESM1-BGC, MPI-ESM-MR, ACCESS 1.0, MIROC5, and IPSL-CM5A-MR (Sanderson et al., 2015; Karger et al., 2017). We selected these five models to minimize the interdependency between models (Sanderson et al., 2015).
Basal area modeling and model evaluation
To estimate the current black walnut basal area across the eastern USA, we considered three candidate machine learning models based on 42 predictor variables. The three candidate models were random forests, XGBoost, and multiple linear regression. Random forests are a non-parametric ensemble learning approach (Breiman, 2001), which generates a variant of regression trees to add an additional level of randomness by bootstrapping sub-data and using different sets of predictor variables to mitigate the multicollinearity issues. The XGBoost model is a decision-tree-based ensemble machine learning algorithm that uses a gradient boosting framework (Nielsen, 2016). To assess the performance of candidate models in estimating black walnut basal area, we used Monte Carlo cross-validation, which creates multiple random splits of the dataset into training (90%) and validation data (10%). We calculated the R2 (coefficient of determination), MAE (mean absolute error), and RMSE (root mean square error) as indicators of the explained variance to compare the performance of the three models. These indices were calculated based on Wang et al. (2018). We tuned two hyperparameters for random forests to maximize the performance while minimizing the computational time: the number of regression trees to grow (ntree) and the number of randomly selected evidential features at each node (mtry). We used RMSE to determine the optimal ntree and mtry values (Supplementary Figure 1). We determined the optimal ntree = 20 and mtry = 10. With random forests, we also quantified the importance value of all the predictor variables based on Gini impurity (Grabmeier and Lambe, 2007). Finally, future basal area was predicted using the final model and future climate projection data as a new dataset. Non-bioclimate variables were maintained the same as current values for future prediction due to lack of data under future emission scenarios. Nevertheless, the impact of this is minimal as changes in topography and soil are not expected to be as drastic as changes in climate.
We used “randomForest” package to conduct random forests and predict future basal area in R (Liaw and Wiener, 2002), “xgboost” package to conduct XGBoost (Nielsen, 2016), and ‘corrplot’ package to create a correlation matrix of the predictor variables (Wei et al., 2017).
Results
Basal area modeling and model evaluation
Random forests model outperformed multiple linear regression and XGBoost models in terms of the coefficient of determination (R2), root mean square error (RMSE), and mean absolute error (MAE) (Figure 1 and Table 2). Therefore, we chose random forests as a final model to estimate black walnut basal area. The R2 of random forests model was 2,450% and 6.3% higher than multiple linear regression and XGBoost models, respectively (Figure 1 and Table 2). Furthermore, random forests showed 27.4% lower MAE and 32.6% lower RMSE than multiple linear regression and 3.9% lower MAE and 3.2% lower RMSE than XGBoost (Figure 1 and Table 2), which indicates the highest accuracy of random forests among the three candidate models. The proportion of variation explained in our final random forests was 75%. Random forests was also used to predict future basal area.
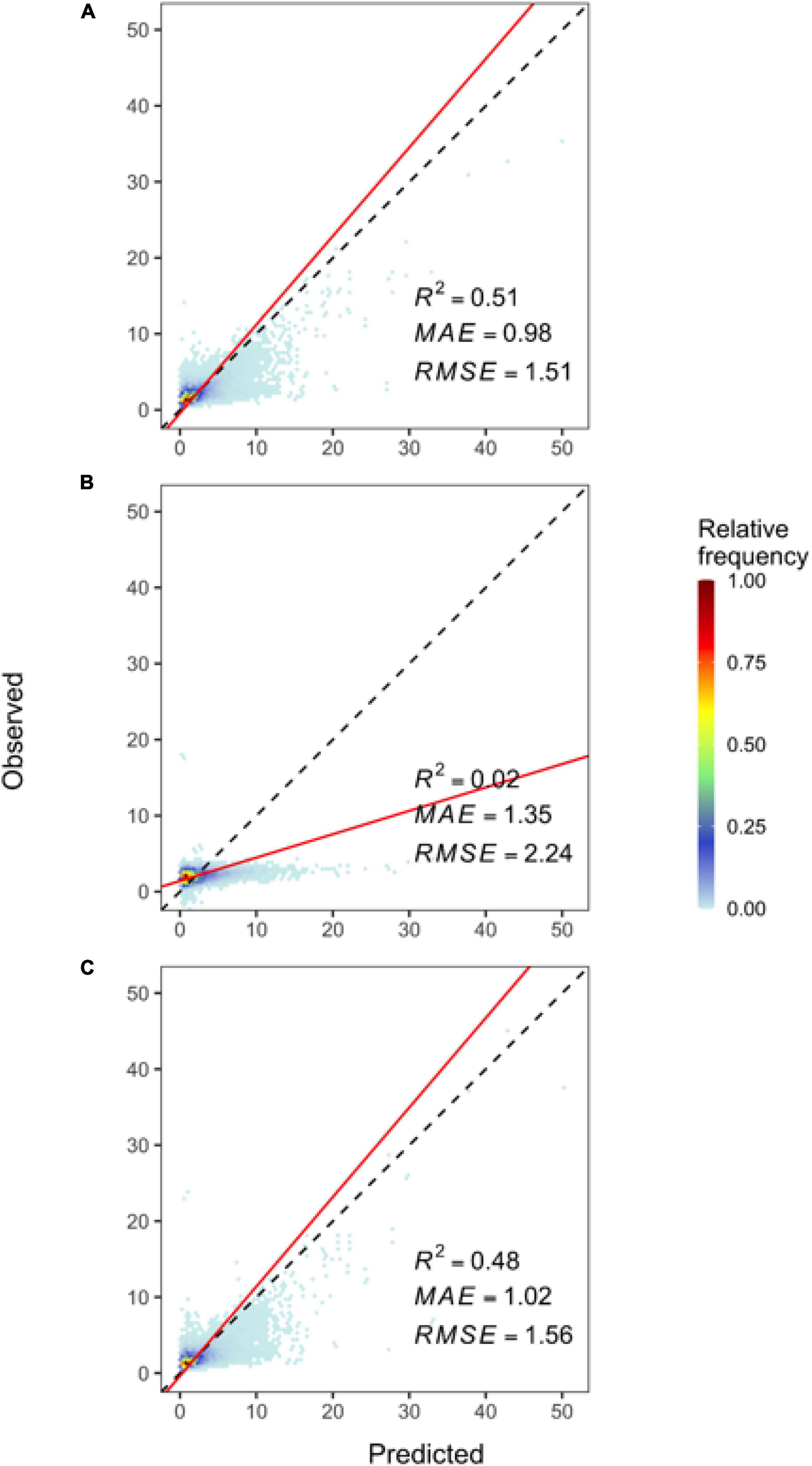
Figure 1. Cross-validation of random forests model (A) versus multiple Linear Regression (B) and XGBoost (C). Scatter plots show observed (vertical axis) vs. predicted (horizontal axis) basal area values (m2/ha), from which we calculated mean absolute error (MAE), root-mean-squared error (RMSE), and the coefficient of determination (R2) of the trendlines (red) between the predicted and observed values. The broken lines represent the perfect fit where predicted values match observed values.
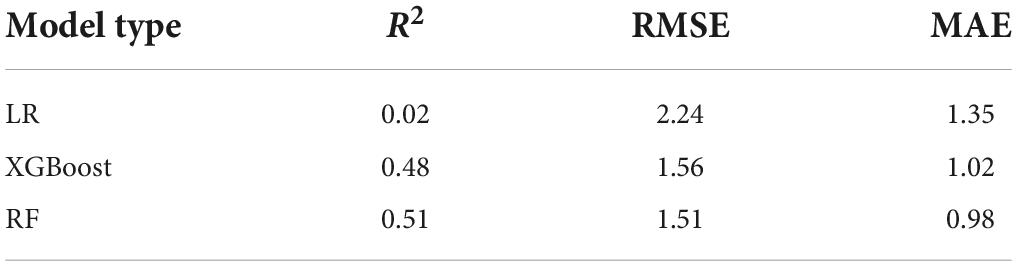
Table 2. Statistical comparison of random forests (RF) model, Linear Regression (LR), and XGBoost model to predict random forests using bioclimatic variables statistics characteristics show the coefficient of determination (R2), root means square error (RMSE), and mean absolute error (MAE).
Important variables to predict the basal area
Based on our random forests model, some topography and climate variables had a profound influence on the prediction of basal area in black walnut species (Figure 2). Aspect sine and cosine (T1, T2), topographic position index (T11), temperature seasonality (C4), annual Precipitation (C12), indexed annual aridity (C20), global potential evapotranspiration (C21), and human footprint (H1) were the most important predictor variables for black walnut basal area (Figure 2 and Supplementary Figure 2). Black walnut grows in areas/regions with annual precipitation varied from 500 to 2,000 mm (Table 1 and Supplementary Figure 3). The annual precipitation (C12) from 500 to 1,000 mm increased the basal area of black walnut across its range. The amount of precipitation above 1,000 mm can decrease the basal area. Global potential evapotranspiration (C21) can decrease the basal area if the amount of evapotranspiration is high. The human footprint plays a significant role in increasing basal area, revealing that managed forests can increase the basal area in black walnut. Soil variables, maximum and minimum temperature of the warmest and coldest month (C5, C6), mean temperature of warmest quarter (C10), precipitation of wettest and driest month (C13, C14), and precipitation seasonality (C15) had the lowest impact on the basal area (Figure 2).
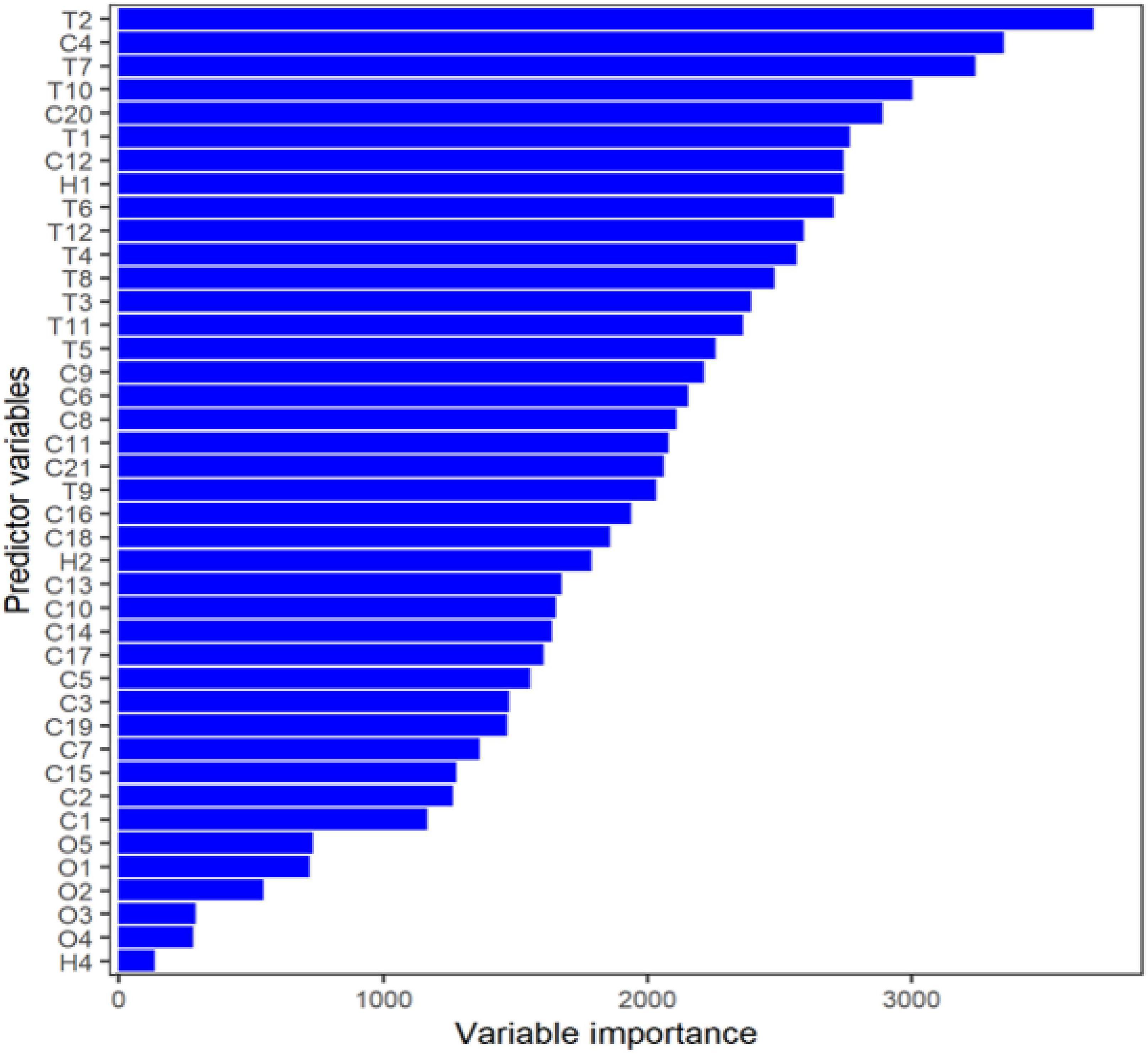
Figure 2. Variable importance of the predictor of the basal area in black walnut based on the Gini model (IncNodePurity: variables that split at each node) using random forests machine learning The value was calculated based on the Gini model for each of the 42 independent variables. See Table 1 for definitions of each predictor variable.
Future changes in black walnut basal area
We predicted the current and future distribution of basal area of black walnut over the study region based on the FIA database (Figure 3) and the random forests model. The estimated basal area of black walnut was on average 3.99 m2/ha across the eastern USA (with a maximum of 43.01 m2/ha) with current climate data, 24.55 m2/ha (with a maximum of 116.45 m2/ha) with RCP 4.5, and 29.26 m2/ha (with a maximum of 119.53 m2/ha) with RCP 8.5 (Table 3). While current basal area was estimated to be distributed between 35 and 42 degrees latitude (Figure 4A), future distribution was estimated to shift up to 50 degrees (Figures 4B,C). In some parts of the eastern USA, basal area was estimated to exceed 100 m2/ha—more than double the current basal area—such as in western Midwest with RCP 4.5 (Figure 4B) and in eastern Midwest with RCP 8.5 (Figure 4C). In contrast, we estimated that basal area will largely decline in lower latitudes (Figures 4B,C).
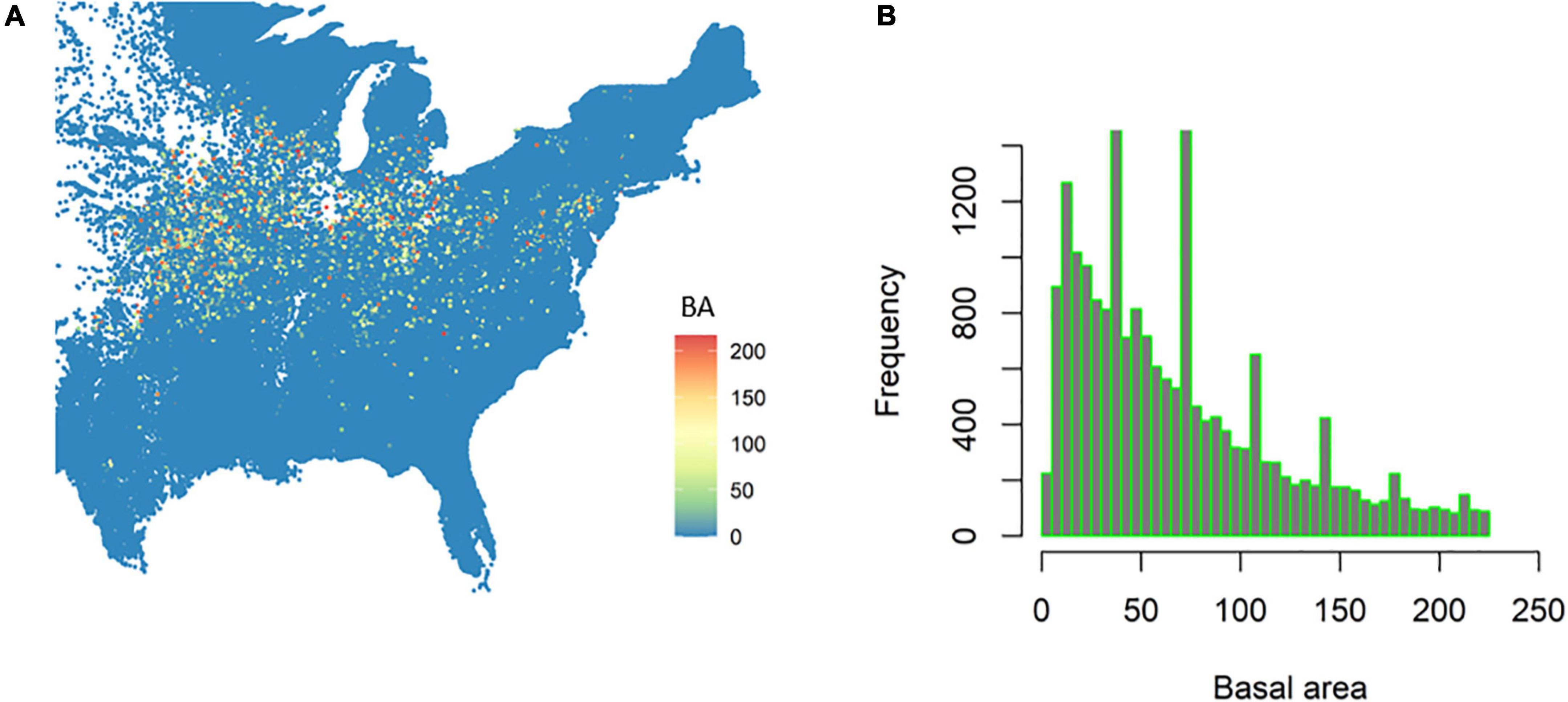
Figure 3. The distribution of black walnut in the United States (A) and associated basal area (BA, m2/ha) frequency distribution (B).

Table 3. Absolute basal area (m2/ha) and prediction of the basal area with current and future prediction of two scenarios (RCP 45 and RCP 8.5).
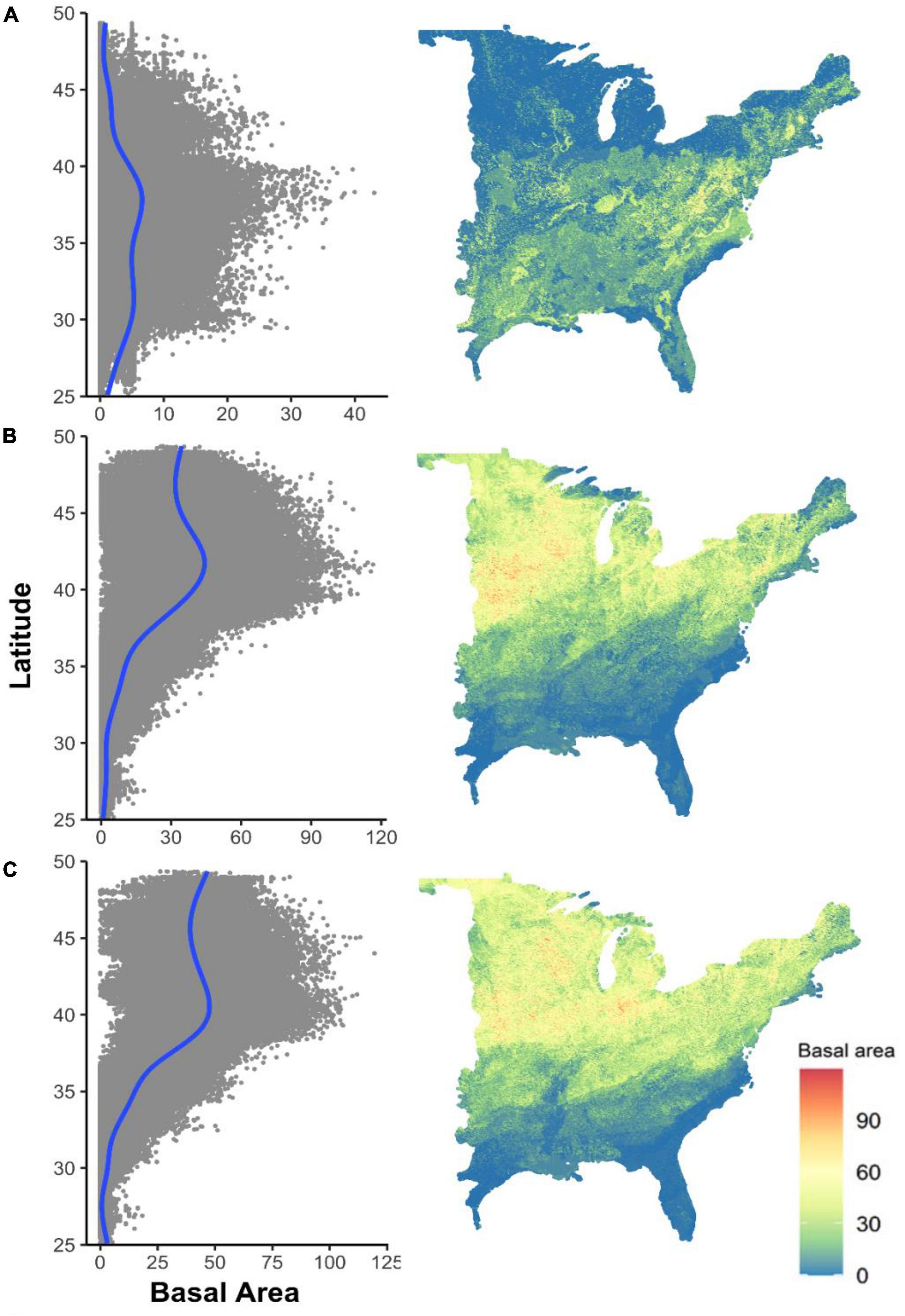
Figure 4. Predicted local basal area (m2/ha) of black walnut based on current climate (A) and future climate scenario RCP4.5 (B) and RCP8.5 (C). Right panels map the geographic distribution of the predicted basal area, with latitudinal occurrences (gray points) and associated latitudinal trendlines (blue curves) shown in the left panels.
Discussion
Black walnut is distributed in a wide range of climates, making it an important species to evaluate the climate-stocking relationship with random forests models. Black walnut grows in regions with a minimum temperature between −45 and 4°C (Williams, 1990), representing growth variation, inheritance variability, and natural adaptation across latitudes. Additionally, the regions of black walnut distribution have experienced a common land disturbance history, leading to an equal or higher abundance of trees for large vs. small diameter classes across regions and suggesting that the age distribution in our models was relatively similar (Shifley, 2004). Our study shows black walnut basal area starts to decline in regions of lower than 35° and higher than 45° latitude in the northern part of Wisconsin and Minnesota due to severe cold temperatures. Therefore, black walnut is absent in the northern part of Wisconsin, Michigan, New York, Minnesota, and Maine based on the plot measured by FIA. Other studies reported that black walnut could not grow at less than −45°C (Williams, 1990).
In our study, some covariates, such as aspect and topography, had the greatest impact on the basal area of black walnut. Topography was previously determined as a key factor of black walnut growth in natural stands (Schultz and DeLoach, 2004). The positive interaction of aspect and the topology on basal area was previously reported by Jevšenak and Skudnik (2021). However, another study reported a negative effect of slope on basal area (Rohner et al., 2018). Since black walnut is a wind-pollinated species, the topology can ease the pollen movement and increase the regeneration of this species.
Temperature and precipitation influence black walnut basal area. In a provenance test of black walnut, the annual mean temperatures of seed resource’s location play a significant role in the accumulation of tree growth in a common garden (Onofrio et al., 2021). Jevšenak (2019) reported a significant climate-growth relationship on the European tree-ring network. Hence, increasing temperature and changing precipitation patterns due to rapid climate change could influence black walnut basal area in the future. The impact of temperature seasonality and annual precipitation in our study on basal area represents how climate change can influence future black walnut regeneration and stocking. A study evaluating five tree species in the eastern USA showed that seasonal temperature and precipitation positively impact growth rate compared to annual mean temperature (Rollinson et al., 2016), which is consistent with our study’s findings.
In this study, annual precipitation of 500–1,000 mm can increase the basal area; in contrast, precipitation above 1,000 mm can decrease the basal area in black walnut. Therefore, given the increase of flood events in the Midwestern USA in the last decades and the prospective continuation of this trend, black walnut stocking may be affected in the future. Extreme or higher precipitation in specific seasons negatively affected basal area in various tree species in Europe (Jevšenak and Skudnik, 2021) or caused severe seedling mortality in black walnut (Coggeshall and Schlarbaum, 2007). This reveals that changing precipitation patterns, especially frequent flooding events, can negatively affect the future survival rate and basal areas in black walnut. A provenance study of black walnut planted in Indiana, USA revealed that partial flooding events increase the rate of seedling mortality compared with other sites without flooding events (Onofrio et al., 2021). Evapotranspiration and index annual aridity were the other two important variables in our study. Above 1,100 mm/year can influence basal area since the water content of soils is positively correlated with water uptake and evapotranspiration, especially in the summer. Evapotranspiration is highly correlated with mean annual temperature (Liang et al., 2016), which indicates that increasing temperatures in the future can influence basal areas in black walnut. Rollinson et al. (2016) identified evapotranspiration and temperature as the main factors influencing basal area in several tree species.
Soil variables did not impact basal area, which is not surprising since the soil type in the Midwestern USA could be consistent or at least provides enough minerals for black walnut to grow across its range. Some studies showed that soil nutrients do not significantly influence black walnut growth but can be slightly influenced by soil texture, soil depth, and pH (Geyer and Ponder, 2004; Schultz and DeLoach, 2004). Another study reported that other tree species growing in poor or rocky soil types or distributed across different continents do not have consistent growth potential in a random forests model (Jevšenak and Skudnik, 2021). Some studies reported that soil content was the least important variable for pine forest richness (Jin et al., 2021). This suggests that black walnut stocking may not be affected by soil content or nutrition, although the depth of the soil can be an important factor. Black walnut prefers loamy, moist, but well-drained soils typically found in riparian zones (McKenna and Coggeshall, 2018). Evaluating four tree species in the Amazon rain forests, Da Cunha et al. (2016) suggested that similar R2 values for all species represent the consistent soil conditions for increasing the accuracy of the models to predict basal area. Based on our studies and a previous report (Iverson et al., 2019), black walnut will migrate to the northern range in the future. Therefore, the type of soils in areas like northern Wisconsin, Minnesota, Northern Michigan, New York, and Maine need to be considered in future studies. Black walnut cannot tolerate dry, rocky, limestone soils and even ruderal sites (Pike et al., 2021). The northern range of black walnut has more hills and rocky soils that may not be suitable for black walnut and may threaten the habitat of this species in the future.
Our results showed that the human footprint is an important variable in predicting basal area. Black walnut has been used for gunstocks, bowls, and furniture because of its high-quality logs, beautiful grain, and ease of working with veneer. Therefore, black walnut has a higher prize timber quality than other hardwood tree species (McKenna and Coggeshall, 2018), which encourages foresters to remove competitors to increase tree growth and timber quality of shade-intolerant black walnut. Thinning/harvesting had a positive effect on the basal area for other tree species (Rohner et al., 2018). The history of human influence on modern tree species goes back to millions of years ago when they tried to conserve specific species and harvest unwanted/undesirable trees (Rohner et al., 2018).
The CO2 concentration of air is expected to increase exponentially by 2080 (Kirilenko and Sedjo, 2007), affecting forest productivity (Kirilenko and Sedjo, 2007; Arab et al., 2020). The basal area can be affected due to elevated temperature or changing precipitation patterns as a result of climate change. Our study found that the basal area may increase under both emission scenarios compared with the projection under constant climate. Albert and Schmidt, 2010 reported that in conditions where the precipitation is not limited, rising temperatures in the growing season would positively affect forest productivity. Thus, increasing the basal area in our study RCP 8.5 scenarios could result from rising temperatures in the future and changing precipitations patterns. Increasing growth with elevated CO2 was previously reported in other plant species (Polley et al., 2003). In addition, elevated CO2 could affect the chilling requirement of tree species (Hajinia et al., 2021). Responses to climate change are, however, a result of complex interactions among multiple factors, which require future interpolation specific to black walnut.
Based on future climate data, black walnut may migrate to a northern range and gain a higher value of basal area mainly based on the RCP 8.5 scenario. This finding agrees with the climate change atlas published by the USDA Forest Service (Iverson et al., 2019), which showed that under RCP 4.5 and RCP 8.5 scenarios, trees can migrate to a northern part of the region within 100 years. Tree migration to a northern range will induce range shifts, bottlenecks, fragmentation of populations, or changes in the habitat of other species. However, with increasing temperature and precipitation patterns in the last 30 years, black walnut may migrate more than 100 km to a northern range to grow in a more favorable condition (Iverson et al., 2019). Fei et al. (2017) also reported at least 25 km per decade of tree migration of black walnut to the northern range, which is 250 times faster than the past-glacial migration time reported for two American hardwood species (McLachlan et al., 2005). Therefore, with the rapidly changing climate, black walnut distribution may shift in the future to its northern range, especially in northern Wisconsin, Minnesota, Northern Michigan, New York, and Maine through the Canadian border. Consequently, in the face of climate change, migrating black walnut populations may be threatened by climate-related stressors, including extraordinarily high or cold temperatures, late spring frost, or drought events that have become more frequent in the last decade. The role of adaptive responses to climate change should therefore be considered for tree migration since evolution occurs more slowly than climate change (Davis et al., 2005). In addition, local, regional, and global changes in temperature and precipitation can influence the occurrence, frequency, and intensity of disturbances, significantly enhancing massive insects and pathogen outbreaks (Dale et al., 2001). Thus, since temperature and precipitation directly and indirectly affect forest disturbances and forest range expansion, the interaction of temperature-precipitation variability with other factors such as disease or pest outbreaks and anthropogenic changes should be considered in future studies.
Our results also showed black walnut abundance would decline in southern and central latitudes under RCP 4.5 and 8.5 scenarios. This result is consistent with a study in Europe that reported a remarkable decrease in tree species’ abundance and genetic diversity in southern and central forests and increasing abundance and genetic diversity in the northern range (Buras and Menzel, 2019). Since black walnut is currently distributed mainly in the center of the eastern USA forest, a declining abundance of black walnuts might endanger the habitat of this species.
In summary, we developed machine learning models to study the future of black walnut stocking across the United States and assess the impact of climate change on basal area. We selected random forests (RF) as a final model to estimate the basal area of black walnut under climate change, because RF had a higher coefficient of determination (R2), lower root mean square error (RMSE), and lower mean absolute error (MAE) than the other two models (XGBoost and linear regression). The most important variables to predict basal area were the mean annual temperature and precipitation, global evapotranspiration, topology, and human footprint. Under two emission scenarios (Representative Concentration Pathway 4.5 and 8.5), the RF model projected that black walnut stocking would increase in the northern part of the current range in the USA, with a potential shift of species distribution range. This model can be adapted to predict tree stocking for other hardwood tree species based on future climate scenarios.
Data availability statement
The datasets presented in this study can be found in online repositories. The names of the repository/repositories and accession number(s) can be found below: https://apps.fs.usda.gov/fia/datamart/datamart.html.
Author contributions
AE developed the original idea, implemented the computations, data analysis and mapping, interpreted and visualized the results, and wrote the manuscript. AA contributed to the analytical methods, interpretation of the results, supported R coding, and edited and revised the manuscript. JL coordinated tree-level data compilation with the F.I.A. data, conceptualized the study, supported and supervised R coding, data analysis, and mapping, taking the lead throughout, and edited and revised the manuscript. DJ supervised the study and edited and revised the manuscript. All authors contributed to the article and approved the submitted version.
Funding
We acknowledge financial support from the National Science Foundation Center for Advanced Forestry Systems award number 1916587, the van Eck Forest Foundation, and the Department of Forestry and Natural Resources at Purdue University to support this study.
Acknowledgments
We thank Bee Redfield, Port Townsend Marine Science Center and graduate student at Purdue University, for editing the primary version of the manuscript and Sven Nelson Director of Plant Science, Heliponix, LLC and graduate student at Purdue University, for consulting in analyzing and supporting R coding. We also thank Rosen Center for Advanced Computing (RCAC) at Purdue University for the high-performance computing support. This manuscript was developed based on a term project for the course FNR59800 Big Data, AI, and Forests, which is offered at Purdue University each spring semester.
Conflict of interest
The authors declare that the research was conducted in the absence of any commercial or financial relationships that could be construed as a potential conflict of interest.
Publisher’s note
All claims expressed in this article are solely those of the authors and do not necessarily represent those of their affiliated organizations, or those of the publisher, the editors and the reviewers. Any product that may be evaluated in this article, or claim that may be made by its manufacturer, is not guaranteed or endorsed by the publisher.
Supplementary material
The Supplementary Material for this article can be found online at: https://www.frontiersin.org/articles/10.3389/ffgc.2022.970379/full#supplementary-material
Supplementary Figure 1 | The number of regression trees (ntree) and the number of randomly selected evidential features at each node (mtry) based on the fine-tuned model to predict basal area in black walnut.
Supplementary Figure 2 | Pearson correlation coefficients between all pairs of bioclimate variables across the black walnut range in the eastern USA (see the details of variables in Table 1).
Supplementary Figure 3 | Partial dependence plot of the most important environment, topography, and anthropogenic covariates affecting the basal area in black walnut in the eastern USA (For the units, please see Table 1). A partial dependence plot shows the effect of one predictor variable (horizontal axis) on predicted basal area of black walnut (vertical axis), while all other predictors are kept constant at their sample mean.
Footnotes
- ^ https://www.treefarmsystem.org
- ^ https://www.americanhardwood.org/en/american-hardwood/American-walnut
References
Albert, M., and Schmidt, M. (2010). Climate-sensitive modeling of site-productivity relationships for Norway spruce (Picea abies (L.) Karst.) and common beech (Fagus sylvatica L.). Forest Ecol. Manag. 259, 739–749.
Amatulli, G., Domisch, S., Tuanmu, M. N., Parmentier, B., Ranipeta, A., Malczyk, J., et al. (2018). A suite of global, cross-scale topographic variables for environmental and biodiversity modeling. Sci. Data 5, 1–15. doi: 10.1038/sdata.2018.40
Arab, M. M., Marrano, A., Abdollahi-Arpanahi, R., Leslie, C. A., Cheng, H., Neale, D. B., et al. (2020). Combining phenotype, genotype, and environment to uncover genetic components underlying water use efficiency in Persian walnut. J. Exp. Bot. 71, 1107–1127. doi: 10.1093/jxb/erz467
Ares, A., and Brauer, D. (2004). Growth and nut production of black walnut in relation to site, tree type and stand conditions in south-central USA. Agroforest. Syst. 63, 83–90.
Ashraf, M. I., Meng, F. R., Bourque, C. P. A., and MacLean, D. A. (2015). A novel modelling approach for predicting forest growth and yield under climate change. PLoS One 10:e0132066. doi: 10.1371/journal.pone.0132066
Batjes, N. H. (2016). Harmonized soil property values for broad-scale modelling (WISE30sec) with estimates of global soil carbon stocks. Geoderma 269, 61–68.
Bernstein, L., Bosch, P., Canziani, O., Chen, Z., Christ, R., and Riahi, K. (2008). IPCC, 2007: Climate Change 2007: Synthesis Report. Geneva: IPCC.
Brang, P., Spathelf, P., Larsen, J. B., Bauhus, J., Boncčìna, A., Chauvin, C., et al. (2014). Suitability of close-to-nature silviculture for adapting temperate European forests to climate change. Forestry 87, 492–503.
Bucklin, D., and Basille, M. (2018). Rpostgis: Linking R with a PostGIS Spatial Database. R. J. 10, 251–268.
Buras, A., and Menzel, A. (2019). Projecting tree species composition changes of European forests for 2061–2090 under RCP 4.5 and RCP 8.5 scenarios. Front. Plant Sci. 9:1986. doi: 10.3389/fpls.2018.01986
Burrill, E. A., Wilson, A. M., Turner, J. A., Pugh, S. A., Menlove, J., Christiansen, G., et al. (2018). The Forest Inventory and Analysis Database: Database Description and User Guide Version 8.0 for Phase 2. Washington, DC: US Department of Agriculture, Forest Service.
Coggeshall, M. V., and Schlarbaum, S. E. (2007). “Genotypic variation in flood tolerance of black walnut and three southern bottomland oaks,” in Proceedings, 15th Central Hardwood Forest Conference. e-Gen. Tech. Rep. SRS-101, eds D. S. Buckley and W. K. Clatterbuck (Southern Research Station: US Department of Agriculture, Forest Service), 629–637.
Da Cunha, T. A., Finger, C. A. G., and Hasenauer, H. (2016). Tree basal area increment models for Cedrela, Amburana, Copaifera and Swietenia growing in the Amazon rain forests. Forest Ecol. Manag. 365, 174–183.
Dale, V. H., Joyce, L. A., McNulty, S., Neilson, R. P., Ayres, M. P., Flannigan, M. D., et al. (2001). Climate change and forest disturbances: climate change can affect forests by altering the frequency, intensity, duration, and timing of fire, drought, introduced species, insect and pathogen outbreaks, hurricanes, windstorms, ice storms, or landslides. BioScience 51, 723–734.
Davis, M. B., Shaw, R. G., and Etterson, J. R. (2005). Evolutionary responses to changing climate. Ecology 86, 1704–1714.
Ebrahimi, A., Mathur, S., Lawson, S. S., Labonte, N. R., Lorch, A., Coggeshall, M. V., et al. (2019). Microsatellite Borders and Micro-sequence Conservation in Juglans. Sci. Rep. 9:3748. doi: 10.1038/s41598-019-39793-z
Ercanlı, Ý (2020). Innovative deep learning artificial intelligence applications for predicting relationships between individual tree height and diameter at breast height. Forest Ecosyst. 7:12.
Fei, S., Desprez, J. M., Potter, K. M., Jo, I., Knott, J. A., and Oswalt, C. M. (2017). Divergence of species responses to climate change. Sci. Adv. 3;e1603055.
Gauthier, M. M., and Jacobs, D. F. (2011). Walnut (Juglans spp.) ecophysiology in response to environmental stresses and potential acclimation to climate change. Ann. Forest Sci. 68, 1277–1290.
Geyer, W. A., and Ponder, F. Jr. (2004). “Site relationships and black walnut height growth in natural stands in eastern Kansas,” in Proceedings of the 6th Walnut Council Research Symposium; Gen. Tech. Rep. NC-243, eds C. H. Michler, P. M. Pijut, J. W. Van Sambeek, M. V. Coggeshall, J. Seifert, K. Woeste, et al. (St. Paul, MN: U.S. Department of Agriculture, Forest Service, North Central Research Station), 77–80.
Grabmeier, J. L., and Lambe, L. A. (2007). Decision trees for binary classification variables grow equally with the Gini impurity measure and Pearson’s chi-square test. Int. Jo. Bus. Intelligence Data Min. 2, 213–226.
Hajinia, Z., Sarikhani, S., and Vahdati, K. (2021). Exploring low-chill genotypes of Persian walnut (Juglans regia L.) in west of Iran. Genet. Resour. Crop Evol. 68, 2325–2336.
Hijmans, R. J., Cameron, S. E., Parra, J. L., Jones, P. G., and Jarvis, A. (2005). Very high-resolution interpolated climate surfaces for global land areas. Int. J. Climatol. 25, 1965–1978.
Ibisch, P. L., Hoffmann, M. T., Kreft, S., Pe’er, G., Kati, V., Biber-Freudenberger, L., et al. (2016). A global map of roadless areas and their conservation status. Science 354, 1423–1427. doi: 10.1126/science.aaf7166
Iverson, L. R., Prasad, A. M., Peters, M. P., and Matthews, S. N. (2019). Facilitating adaptive forest management under climate change: A spatially specific synthesis of 125 species for habitat changes and assisted migration over the eastern USA. Forests 10:989.
Jevšenak, J. (2019). Daily climate data reveal stronger climate-growth relationships for an extended European tree-ring network. Quat. Sci. Rev. 221:105868.
Jevšenak, J., Levanič, T., and Džeroski, S. (2018). Comparison of an optimal regression method for climate reconstruction with the compare_methods () function from the dendroTools R package. Dendrochronologia 52, 96–104.
Jevšenak, J., and Skudnik, M. (2021). A random forest model for basal area increment predictions from national forest inventory data. Forest Ecol. Manag. 479:118601.
Jin, W. T., Gernandt, D. S., Wehenkel, C., Xia, X. M., Wei, X. X., and Wang, X. Q. (2021). Phylogenomic and ecological analyses reveal the spatiotemporal evolution of global pines. Proc. Natl. Acad. Sci. 118:e2022302118. doi: 10.1073/pnas.2022302118
Karger, D. N., Conrad, O., Böhner, J., Kawohl, T., Kreft, H., Soria-Auza, R. W., et al. (2017). Climatologies at high resolution for the Earth land surface areas. Sci. Data 4:170122. doi: 10.1038/sdata.2017.122
Kindermann, G., Obersteiner, M., Sohngen, B., Sathaye, J., Andrasko, K., Rametsteiner, E., et al. (2008). Global cost estimates of reducing carbon emissions through avoided deforestation. Proc. Natl. Acad. Sci. U.S.A. 105, 10302–10307.
Kirilenko, A. P., and Sedjo, R. A. (2007). Climate change impacts on forestry. Proc. Natl. Acad Sci. 104, 19697–19702.
Li, R., Zhu, S., Chen, H. Y., John, R., Zhou, G., Zhang, D., et al. (2015). Are functional traits a good predictor of global change impacts on tree species abundance dynamics in a subtropical forest? Ecol. Lett. 18, 1181–1189. doi: 10.1111/ele.12497
Liang, J., Crowther, T. W., Picard, N., Wiser, S., Zhou, M., Alberti, G., et al. (2016). Positive biodiversity-productivity relationship predominant in global forests. Science 354:6309. doi: 10.1126/science.aaf8957
Lysenko, I., Besançon, C., and Savy, C. (2007). 2007 UNEP-WCMC Global List of Transboundary Protected Areas. Global Transboundary Conservation Network. Cambridge: UNEP-WCMC.
Ma, W., Lin, G., and Liang, J. (2020). Estimating dynamics of central hardwood forests using random forests. Ecol. Model. 419:108947.
McKenna, J. R., and Coggeshall, M. V. (2018). The Genetic Improvement of Black Walnut for Timber Production. Plant Breed. Rev. 41, 263–290.
McLachlan, J. S., Clark, J. S., and Manos, P. S. (2005). Molecular indicators of tree migration capacity under rapid climate change. Ecology 86, 2088–2098.
Michler, C. H., Pijut, P. M., Meilan, R., Smagh, G., Liang, X., and Woeste, K. E. (2009). “Black walnut,” in Compendium Transgenic Crop Plants Ed. C. Kole (Hoboken, NJ: Wiley), 263–278.
Myers, R. K., and Fischer, B. C. (1991). “Black walnut tree growth in a mixed species, upland hardwood stand in southern Indiana,” in Proceedings, 8th Central Hardwood Forest Conference; 1991 March 4-6; University Park, PA. Gen. Tech. Rep. NE-148, Vol. 148, eds L. H. McCormick and K. W. Gottschalk (Radnor, PA: US Department of Agriculture, Forest Service, Northeastern Forest Experiment Station), 388–394.
Nicolescu, V. N., Rédei, K., Vor, T., Bastien, J. C., Brus, R., Benčat’, T., et al. (2020). A review of black walnut (Juglans nigra L.) ecology and management in Europe. Trees 34, 1087–1112.
Nielsen, D. (2016). Tree Boosting with xgboost-Why Does xgboost win” every” Machine Learning Competition?. Master’s thesis, Trondheim: NTNU.
Olson, D. M., Dinerstein, E., Wikramanayake, E. D., Burgess, N. D., Powell, G. V., Underwood, E. C., et al. (2001). Terrestrial Ecoregions of the World: A New Map of Life on EarthA new global map of terrestrial ecoregions provides an innovative tool for conserving biodiversity. BioScience 51, 933–938.
Onofrio, L., Hawley, G., and Leites, L. P. (2021). Ecological genetics of Juglans nigra: Differences in early growth patterns of natural populations. Ecol. Evol. 11, 7399–7410. doi: 10.1002/ece3.7571
Pike, C. C., Williams, M., Brennan, A., Woeste, K., Jacobs, J., Hoban, S., et al. (2021). Save our species: a blueprint for restoring butternut (Juglans cinerea) across eastern North America. J. Forest., 196–206.
Polley, H. W., Johnson, H. B., and Tischler, C. R. (2003). Woody invasion of grasslands: evidence that CO2 enrichment indirectly promotes establishment of Prosopis glandulosa. Plant Ecol. 164, 85–94.
Rohner, B., Waldner, P., Lischke, H., Ferretti, M., and Thürig, E. (2018). Predicting individual-tree growth of central European tree species as a function of site, stand, management, nutrient, and climate effects. Eur. J. Forest Res. 137, 29–44.
Rollinson, C. R., Kaye, M. W., and Canham, C. D. (2016). Interspecific variation in growth responses to climate and competition of five eastern tree species. Ecology 97, 1003–1011.
Sanderson, B. M., Knutti, R., and Caldwell, P. (2015). A Representative Democracy to Reduce Interdependency in a Multimodel Ensemble. J. Clim. 28, 5171–5194.
Schlamadinger, B., Bird, N., Johns, T., Brown, S., Canadell, J., Ciccarese, L., et al. (2007). A synopsis of land use, land-use change and forestry (LULUCF) under the Kyoto Protocol and Marrakech Accords. Environ. Sci. Policy 10, 271–282.
Schmidt, T. L. (1998). “Black walnut in the USA,” in Nut Production Handbook for Eastern Black Walnut, eds J. E. Jones, R. Mueller, and J. W. Van Sambeek (Jefferson, MO: Southwest Missouri Resources, Conservation & Development, Inc), 5–21.
Schultz, E. B., and DeLoach, W. M. III (2004). Site suitability and economic aspects of black walnut (Juglans nigra L.) in Mississippi. S. J. Appl. Forest. 28, 123–131.
Shifley, S. R. (2004). “The black walnut resource in the United States,” in Proceedings of the 6th Walnut Council Research Symposium; Gen. Tech. Rep. NC-243, eds C. H. Michler, P. M. Pijut, J. W. Van Sambeek, M. V. Coggeshall, J. Seifert, K. Woeste, et al. (St. Paul, MN: U.S. Department of Agriculture, Forest Service, North Central Research Station), 168–176.
Solomon, A. M., and Kirilenko, A. P. (1997). Climate change and terrestrial biomass: what if trees do not migrate? Glob. Ecol. Biogeogr. Lett. 6, 139–148.
Stage, A. R. (1975). Prediction of Height Increment for Models of Forest Growth. Washington, DC: US Department of Agriculture.
Trabucco, A., and Zomer, R. J. (2009). Global aridity index (global-aridity) and global potential evapo-transpiration (global-PET) geospatial database. CGIAR Consort. Spatial Inform. 89, 1–2.
Vahdati, K., Massah Bavani, A. R., Khosh-Khui, M., Fakour, P., and Sarikhani, S. (2019). Applying the AOGCM-AR5 models to the assessments of land suitability for walnut cultivation in response to climate change: a case study of Iran. PLoS One 14:e0218725. doi: 10.1371/journal.pone.0218725
Venter, O., Sanderson, E. W., Magrach, A., Allan, J. R., Beher, J., Jones, K. R., et al. (2016). Global terrestrial Human Footprint maps for 1993 and 2009. Sci. Data 3, 1–10. doi: 10.1038/sdata.2016.67
Wang, B., Waters, C., Orgill, S., Cowie, A., Clark, A., Li Liu, D., et al. (2018). Estimating soil organic carbon stocks using different modelling techniques in the semi-arid rangelands of eastern Australia. Ecol. Indic. 88, 425–438.
Wei, T., Simko, V., Levy, M., Xie, Y., Jin, Y., and Zemla, J. (2017). Package ‘corrplot’. Statistician 56, 316–324.
Williams, G. M., and Ginzel, M. D. (2021). Competitive advantage of Geosmithia morbida in low-moisture wood may explain historical outbreaks of thousand cankers disease and predict the future fate of Juglans nigra within its native range. Front. Forests Glob. Change 8:725066. doi: 10.3389/ffgc.2021.725066
Keywords: forest, climate change, black walnut (Juglans nigra L.), random forest (bagging) and machine learning, basal area, tree migration
Citation: Ebrahimi A, Abbasi AO, Liang J and Jacobs DF (2022) Spatiotemporal trends of black walnut forest stocking under climate change. Front. For. Glob. Change 10:984725. doi: 10.3389/ffgc.2022.970379
Received: 15 June 2022; Accepted: 31 August 2022;
Published: 20 September 2022.
Edited by:
Erwin Dreyer, INRA Centre Nancy-Lorraine, FranceReviewed by:
Salim Heddam, University of Skikda, AlgeriaQin Ma, Nanjing Normal University, China
Kourosh Vahdati, University of Tehran, Iran
Copyright © 2022 Ebrahimi, Abbasi, Liang and Jacobs. This is an open-access article distributed under the terms of the Creative Commons Attribution License (CC BY). The use, distribution or reproduction in other forums is permitted, provided the original author(s) and the copyright owner(s) are credited and that the original publication in this journal is cited, in accordance with accepted academic practice. No use, distribution or reproduction is permitted which does not comply with these terms.
*Correspondence: Douglass F. Jacobs, djacobs@purdue.edu