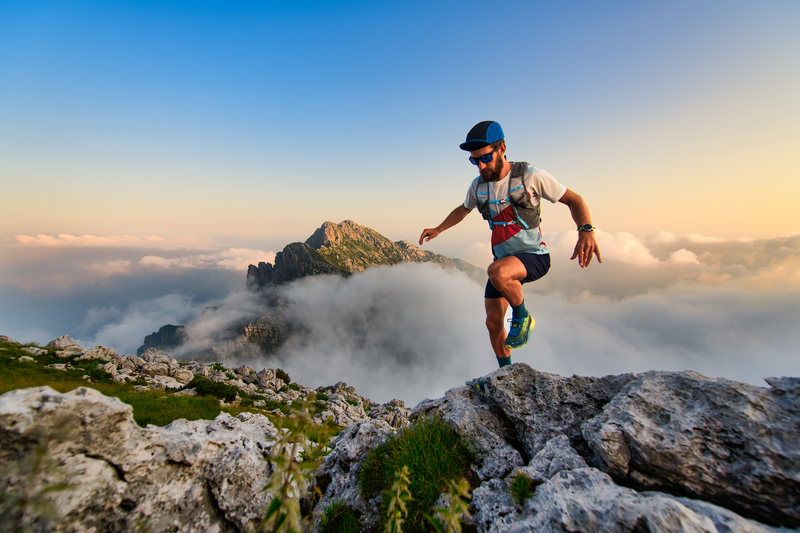
94% of researchers rate our articles as excellent or good
Learn more about the work of our research integrity team to safeguard the quality of each article we publish.
Find out more
REVIEW article
Front. For. Glob. Change , 29 November 2022
Sec. Fire and Forests
Volume 5 - 2022 | https://doi.org/10.3389/ffgc.2022.925480
This article is part of the Research Topic Forest Fire Emissions and Their Impact on Global Climate Change View all 5 articles
Forest fires are emitting substantial amounts of greenhouse gases and particulate matter into the atmosphere than assumed in state climate targets. It can play an important role in combustible environments, such as shrublands, grasslands, and forests, and contribute to climate change. Thus, forest fire, and climate change is intertwined concepts. As vegetation burns, release the carbon stored within them. This is the main reason why large-scale forest fires release atmospheric carbon dioxide (CO2) and hence, are responsible for increasing the rate of climate change to a great extent. It is extremely significant to measure the contribution of global forest fire and emissions trends of greenhouse gases. In this context, continental-scale carbon emissions assessments were primarily attempted using ground-based datasets for forest ecosystem fires. Considerable research has been published employing remote sensing data from coast to coast. While ground-based data are valuable, they have some restrictions that can be overcome by remote sensing. Ground-based fire data are primarily limited to the total burned area, with their completeness changing yearly with the location. Remote sensing can provide additional spatio-temporal fire information to improve fire emission estimates. In this paper, the factors driving forest fire, with a brief discussion on the triangular relationship between fire, land degradation, and climate change, the role of Remote Sensing and Geographic Information Systems (GIS), machine learning (ML), and a critical overview of state-of-the-art global climate change are presented.
Forests are predominantly significant because they stabilize the environment, regulate the carbon cycle, and make available habitation to thousands of existing living forms (Aju et al., 2015; Balla et al., 2021). Forest ecosystems are a grouping of topography, geology, species, and climate that are bound together by physical and biological processes specific to a single site, and are most essentially occupied by trees as the dominant undergrowth (Van Dijk, 2019; Zhumadina et al., 2021). A forest ecosystem can be as small as a tree branch microsite where various microbes, insects, and mosses interact or as large as a boreal forest that surrounds the Earth at northern latitudes (Ménard et al., 2019). The more this area is extended, the more complex the potential interactions. Forestry is one of the oldest customs of agricultural practice, resulting from the need for human inhabitants to capture and cultivate tree species to supply their basic requirements such as shelter, food, clothing, and heat (Butler and Schultz, 2019; Jhariya et al., 2019). Furthermore, “The Rio Convention,” “United Nations Framework Convention on Climate Change (UNFCCC),” “United Nations Convention on Biological Diversity (CBD),” and “United Nations Convention to Combat Desertification (UNCCD),” all eminent organizations acknowledge the important contribution of the forests. And all of them together are making constant efforts to increase the synergy of the purpose related to its conservation.
Forest fire is one of the unpredictable natural calamities and has caused tremendous damage to humans, animals and nature, as well as extinction and economic loss to the inhabitants (Mateus and Gaspar, 2018; Kizer, 2020; Singh and Suresh Babu, 2021). A tiny spark in the forest or the sun’s heat can also cause a devastating fire. A forest fire can spread rapidly throughout a forest in a second, once a fire has started it can spread at a speed of 23 km per hour, catching everything in its path (Kahanji et al., 2019; Mangiameli et al., 2021). The shifting climate could affect some areas more vulnerable to fire and increase the fire occurrence in existing hot spots. It coupled with hot, dry conditions are likely to increase the threat, timing and rigorousness of forest fires. Fire is almost certain to become a growing factor influencing the condition and durability in sensitive areas. Forest fires are an almost constant threat to life, an impact that cannot be reduced but they are also a threat to the environment and can be seen in the form of climate change (Burke et al., 2021; Ertugrul et al., 2021). Thus, forest fire and climate change is intertwined concepts.
Figure 1 shows a relational diagram created with VOS Viewer® software, using a network mechanism (based on the frequency of occurrence) on the primary issues related to climate change. Major associations with “forest fires,” “greenhouse gases,” “carbon dioxide,” and “carbon emissions.” The factors responsible for climate change are depicted in this review paper.
Figure 1. Keywords correlation map using VOS viewer software (Database: scopus and number of articles: 250 documents).
This paper is focused solely on forest fire, climate change, and carbon dioxide emissions to study its effects. Closely related research works embraced by several researchers. Seidl et al. (2014) studied increasing forest disturbances in Europe and their impact on carbon storage. Study show that this intensification can offset the effect of management strategies aiming to increase the forest carbon sink, and calculate the disturbance-related reduction of the carbon storage potential in Europe’s forests to be 503.4 Tg C in 2021–2030. Boer et al. (2020) assessed the burn area of Australian mega forest (temperate broadleaf) fires between September 2019 and early January 2020. Schneising et al. (2020), studied carbon dioxide emissions from forest fires into the atmosphere in Russia. They also explained the potential role of the model in providing possible scenarios for future forest fire events and the likelihood of its distribution in the context of climate change. Masyagina (2021), studied carbon dioxide emitted by fire-affected forest ecosystems in Siberia and local estimates of vegetation recovery. Isaev et al. (2002) used remote sensing techniques to assess Russian forest fire carbon emissions. In this paper, they discuss a remote sensing-based approach to assessing forest fire damage. Which is based on vegetation indices obtained from multi-spectral high-resolution satellite imagery. A normalized difference vegetation index (NDVI) difference image was generated from the SPOT/HRVIR and RESURS-O/MSU-E images from pre- and post-fire satellite images. The study found a close relationship between temporal NDVI values and the level of forest damage. They estimated carbon emissions from burned forest areas using forest fire extent and damage mapping. From a global scientific perspective, there is a serious requirement to focus on forest fire emissions for a sustainable environment. The great harbinger of technological development that has led to innovations in the field of forest fire and climate change studies is undoubtedly the development of remote sensing and Geographic Information Systems (GIS) (Chuvieco et al., 2010; Ahmad and Goparaju, 2018; Ahmad et al., 2019). Due to the emerging importance of the topic, this article provides a detailed review of forest fire emissions and their contribution to global climate change.
In this review, a brief overview of remote sensing, GIS applications for global forest fire monitoring is presented. Initially, a comprehensive analysis of the factors driving forest fires is presented, with a brief discussion on its relationship with biodiversity, land degradation, and climate change, and state-of-the-art monitoring based on remote sensing and GIS.
Concerning environmental aspects, those associated with fuel, weather, and topography are the most substantial drivers of forest fire ignition. In recent years, forest fire regimes have been shifting in several parts of the world with substantial variations between climatic regions (Pausas and Fernández-Muñoz, 2012; Stephens et al., 2013; Reid et al., 2016). Thus, appreciative of the connection between fires and driving factors in diverse climatic zones is essential. Fire incidence outcomes from a combination of propagation and ignition. Lightning from Cloud-to-ground and, to a minor extent, human activity is the principal ignition source in forest ecosystems (Menezes et al., 2022). There are three types of forces that typically regulate the fire spread: fuel, weather, and topography, as abbreviated by the “Fire Environment Triangle” (Krawchuk et al., 2016; Figure 2).
The fuel type itself reflects the characteristics of the fuel properties and has analytically significant implications for regulating fire-environment interactions by shifting the fuel moisture content, the flammable potential of the fuel, and fire characteristics related to the moisture present in the vegetation ecosystem. Topography also affects fires spreading in a straight line by changing wind patterns, and by controlling fuel moisture conditions through sunlight exposure and humidity pooling (Rogers, 2017). Finally, fire climate is often the major supplier for forest fire events on a disproportionate temporal scale through effects on fuel moisture content and fire explosion source. Fire hazard ranking systems, which indirectly or directly bundle climate impacts on fuel moisture, have been established to capture the broader effect of weather on potential fire escalation and to measure the likelihood of fire risk (Pook and Gill, 1993; Liu et al., 2014; He et al., 2022).
Forests cover about 30% of the world’s terrestrial surface and provide water, food, shelter, feed, nutrient cycling, and important ecosystem goods and features with cultural and divergent values (Zhou et al., 2021). Forests store carbon on their own, providing inhabitance for a wide-ranging flora-fauna species and helping to reduce land degradation (Gupta, 2019). These ecosystems help in maintaining healthy, stable soils, provide protection and habitat for the forest’s natural biodiversity, and deliver more stable carbon reserves. However, forests are increasingly vulnerable as a result of deforestation (Mahmoud et al., 2020), climate change (Tabor et al., 2018), forest fire (Schoennagel et al., 2017) and other stressors that can be associated to human actions. Climate change is highly expected to cause major forest life alterations in the near future (Zamora-Gutierrez et al., 2021). The dynamics of uncertainty, which happens when forest ecology is out of equilibrium with environment, is potentially a major characteristic of these. Forest fire, in particular, is anticipated to effect on forest biodiversity and the ability of land degradation, habitat loss and other distortion of other ecosystem services (Scholes et al., 2018). It is not wrong to say that forest fires have negative effects on forest biodiversity, land degradation and climate change (Figure 3). Forest fires show an association between weather, climate conditions and ecosystem progressions, they are closely intertwined and ultimately affecting ecosystem services.
Every year, forest fires destroy acres of land around the world, biodiversity of flora and fauna, and acting as a major source of aerosol and greenhouse gas emissions (Koristekova et al., 2020; Wokekoro, 2020; Shah, 2022). This is a very hot topic for scientists to observe the implications of forest fire emission, as its effects are increasing from time to time in the developing world due to different substantial expansions. Over the years, there has been cumulative evidence about the impact of CO2 emissions from industrialized systems on global warming (McGlade and Ekins, 2015; Allen et al., 2019). This misery has extended to other so-called greenhouse gases, particularly the chlorofluorocarbons (CFCs), methane, and nitrogen oxides (NOx) (Boettcher, 2021). Few efforts have been made to measure the emissions of these gases. Due to the burning of large forest areas globally every year, the amount of greenhouse gases emitted into the atmosphere by forest fires increases significantly. The contribution of these emissions to global warming has been found to be a significant factor (Grace, 2004; Van der Werf et al., 2010; Dong et al., 2022). Conversely, climate change due to global warming are largely responsible for the increased incidence of forest fires.
There is a need for a new indebtedness for what we are calling “pyrodiversity,” or the wide dissimilarity in the effects and fire responses (Roberts et al., 2021). Measuring spatio-temporal fire systems has many inaccuracies, a huge range of variation, and very little accuracy (Reinhardt et al., 2008; DellaSala et al., 2022), more studies are required for the full gradient of fire’s effects. Previous estimates of fire severity and amount of carbon release have often been high and perhaps in many cases underestimated (Van Der Werf et al., 2017). French et al. (2011) investigates the carbon release have been based on studies of forests in Canada and America. Most of the carbon emissions do not come directly from trees, but from other pieces of them such as brush, forest floors, leaf litter and even from under the ground. In the past few years, only a few sustained efforts have been made to very accurately assess the effects of fire on trees or on carbon dynamics (McCarthy et al., 2010). Even when a very severe fire event covers almost all trees, the trees stand still and only fall to the floor, rot, and lose their carbon very slowly over many years (Campbell et al., 2016). Grass and shrubs quickly grow back some time after a high-intensity fire, releasing some of the carbon from dead and decomposing trees. And in several scrupulous burned areas, the researchers normally observed generous tree revival, which would result in comparatively rapid retrieval of carbon uptake and storage (Smith, 1974).
It is predicted that a major fire in the near future could turn the forest from a carbon sink to a source of atmospheric carbon (Churkina et al., 2020). Since fire activities are rapid, while greenhouse gas emissions continue to rise, climate change mitigation approaches focus primarily on human-caused emissions, which will have a greater impact than those that underlie forest fires. For a more accurate memorandum, estimates of carbon impacts are in dire need to better understand the severity, the impact of non-tree responses, and the essence of below-ground processes (Hartter et al., 2020). Even though it appears that the whole thing is burning in a forest fire, it is not so. Trees do not disappear completely even during high-intensity fires, but their resilience capacity is significantly affected (Stephens et al., 2012). The destruction of fires has resulted in a short-term decline in greenhouse gases, but fire will still be an integral and essential part of the forest ecosystem on a long-term basis. The researchers supposed that global warming could lead to complex levels of forest fires and associated global carbon emissions in the future, even though there are several doubts present about how climate change will affect forest ecosystems, and there is no such warning that the incidence of forest fires will increase.
The need to study the relationship between environmental factors and forest fires is important to reduce risk (Cascio, 2018; Kim et al., 2019; Abram et al., 2021). Forest fires can be accomplished by reducing the resilience of existing ecosystems, clearing forests, and protecting the diverse flora and fauna, human life, and property that constitute the biodiversity.
Forest researchers are working with other forest fire experts to assess the representation of forest fire risk, forest vegetation, and fuels, investigating how a changing climate is shifting risk as part of comprehensive adaptation measures (Roy, 2003; Williamson et al., 2019; Cheng and Dale, 2020). Forest burning is a major cause of carbon in the atmosphere (Lasslop et al., 2019); Therefore, it is an important aspect to consider while assessing climate change at the global level. Additionally, familiarity with spatio-temporal emission patterns is essential for assessing their impact on atmospheric dynamics to advance global atmospheric models as well as to encounter the international Kyoto Protocol agreement.
Precise accounting of carbon cycling is of paramount importance for understanding and modeling global climate change. Until now, continental-scale estimates of carbon emissions were mainly made for forest ecosystem fires using ground-based fire datasets (Chuvieco et al., 2018; Desservettaz et al., 2022). There have been some attempts to employ remote sensing data from coast to coast. While ground-based data are valuable, they have some restrictions that can be overcome by remote sensing (Li et al., 2000; Wu et al., 2018; Barmpoutis et al., 2020). Ground-based fire data are primarily limited to the total burned area, their quality and completeness varying from year to year and region to region. Remote sensing can provide additional spatial and temporal fire information to improve fire emission estimates (Krylov et al., 2014; Oliveras et al., 2014; Chuvieco et al., 2020). Even though the changing aspects of global fires are driven by climatic factors (Turco et al., 2018; Teckentrup et al., 2019), several authors have ascribed the inter-annual variability of forest burning (Kelley et al., 2019). For example, in tropical regions, temperatures, and the length of the dry season can vary greatly, greatly affecting the characteristics of fires. Thus, when monitoring emissions on a continuous temporal scale, changing parameters according to seasonal anomalies would be an important step (Ware et al., 2019). Different spatio-temporal scales have also been allied in the last few years, in the direction of a more inclusive valuation of fire assessment. In addition, increasing the use of remote sensing (RS) products by atmospheric modelers involves a clear concern about adding models and observations (Chuvieco et al., 2020). Emissions estimation consists of various optimized models at several spatio-temporal scales (Biggart et al., 2020). While local assessments and dimensions are important for identifying emission mechanisms (Bhattacharjee and Chen, 2020), local and global assessments are important for measuring emission’s effects on the atmosphere and global climate patterns. Models with a high spatial resolution (Andrée et al., 2019) provide a global assessment of spatial variability that models often miss but include more comprehensive information and are problematic for specifying global scales.
The direct measurements of trace gas released during a fire have been implemented through field measurements (Andreae, 2019), as well as through remote sensing analysis of smoke components (Ichoku, 2020). Both field and remote sensing gas emissions measurements require simultaneity with active fires, either experimental or actual ones. Direct measurement of trace gas released during a fire has been instigated through field measurements (Kawa et al., 2018), as well as remote sensing analysis of smoke constituents (Ansmann et al., 2018). Active fire, as well as actual fire, are both required for emissions measurement via remote sensing. It is difficult due to operational complications to coordinate measurement operations with fire activity. The secondary approach to estimating emissions is based on a model that assimilates the input variables involved in the procedure in different ways (Talerko et al., 2021). This method makes it possible to integrate burned area maps with independent estimates of the input variable values. On the downside, these studies present more factors of uncertainty due to error propagation effects when different input variables are considered. Most of these emissions models take into account biomass weight, burning efficiency, burned areas, and combustion parameters. Remote sensing is an exceptional source of information to obtain some of the input parameters required for those models (Jaffe et al., 2020). Since remote sensors measure the same physical variables at different resolutions, the use of remotely sensed data in emission models can deliver important sustenance for spatial scaling, while considering spatial variations, mainly expanding when working with input variables at different levels (Wei and Barros, 2021). Advancement in data fusion techniques may afford a solid framework for this integration in the near future (Kalantar et al., 2020). Moreover, the temporal frequency of remote sensing interpretations can significantly improve time-domain estimates of gas emissions (Table 1).
Table 1. Application of remote sensing (RS) and geographic information system (GIS) in fire emission monitoring.
a. Quantification of greenhouse gas emission
Spatial-sequential greenhouse gas emissions, i.e., carbon, carbon dioxide (CO2), methane (CH4), nitrous oxide (N2O), NOx, and particulate matter are the major components that are estimated through the NPP. The NPP measures the ecological impact of forest fires and the associated damage in highly intensive ecosystems.
b. Release of carbon into the atmosphere
The inventory emissions of greenhouse gas emitted under forest fires are calculated using guidelines provided by the Heath et al. (2011), Baglivo et al. (2020). Seiler and Crutzen (1980), proposed a simple mathematical formula to calculate the emission of any chemical gas or particle species.
E = emission of a gas (x) or particulate matter from fire (g); BA = burned area (ha); FL = fuel density or loading (kg/ha); FF = fraction of fuel consumed (%); EF = emission factor for gas (x) or particulate matter (g/kg). In contrast, it has been an intimidating mission to acquire any of these variables from the aerial, ground, or space-borne observations. Almost none of them are insignificant to obtain from the observation platform or modeling. To effectively use different types of spatial data in raster or vector formats, GIS-based emission modeling systems have been potentially developed. For forest fire emission estimation, key fire emission characteristics can be obtained from satellites and other sources as model inputs. These include burned region, degree of burn, fuel loading, above-ground biomass, burning environments, emission factors, etc. Before the system can run, the input data desires to be attained, revised and converted as separate attribute data stratums. The system involves numerous integrated subsystems that pretend burning processes. The method needs to be able to use RS data in conjunction with conventional data to increase the assessment of carbon emissions and cycling.
c. Remote sesning monitoring and post-fire recovery
Forest fire is one of the most disturbing types around the globe. For this reason, characterizing fire disruption and monitoring post-fire restoration are appropriate topics both for ecological and management resolutions. Krawchuk et al. (2020) presented an article on models, methods, and sensors associated with this topic. Post-fire recovery analysis should be associated with an alive conception of environmental drivers that affect the retrieval processes. In this sense, the post-fire renaissance depends on biotic and abiotic influences, but slight is still recognized about how these factors are interrelated in natural retrieval procedures after fire disruption. Numerous studies have used topographical variables as drivers of post-fire undergrowth retrieval (Roula et al., 2020; Han et al., 2021; Chen et al., 2022), while some other researchers have highlighted the importance of burn rigorousness to describe renewal forms (Trumper et al., 2020). The heritage effects have also been assessed, as well as forest configuration before the fire incident. Spatial properties, such as adjacent remoteness to an unburned zone, a substitution of edge effect, or tree species features have also been recognized as important drivers of retrieval (Singh, 2015). The comparative prominence of these variables and their interface in the fire renaissance procedure remnants are still mainly unknown. Recent development in fire recovery monitoring is based on using temporal RS datasets, especially since 2008 when the US Geological Survey (USGS) released accessibility to the Landsat datasets. This fact has enhanced the examination of past trends, and it has incremented the number of approaches and submissions to illustrate the ecosystem retort to forest fires. Vegetation indexes such as NDVI (normalized ratio of red and near-infrared reflectance), and its derivatives such as dNBR, and RdNBR are widely used in forest fire monitoring. Baglivo et al. (2020) suggested using NDVI, which generally captures chlorophyll concentration and green covering, for tracing initial periods of the ancillary succession in Italy.
Understanding the consequences of forest fires is a hot topic for scientists, as its effects accumulate over time in different ways in developing countries. It is highly likely to cause major climate change shortly (Littell et al., 2016). The dynamics of imbalance, which occurs when forest ecology is out of balance with climate, is potentially a major feature of these. A large part of fire modeling work relies on deterministic, biophysical models to conclude its impact assessments (Briassoulis, 2020). Accurate fire mapping is critical for projecting climate change impacts associated with major control and eco-environmental impacts and in turn mitigation policy. The use of technological innovations such as various tools and software can support forest managers with systematic analysis desirable for sustainable management and conservation strategies. Over the past decade, machine learning (ML) approaches have revolutionized scientific discovery across disciplines, including Earth informatics, image processing-based applications (Sarker, 2021), satellite imagery (Abburu and Golla, 2015), sensor signal processing (Hong et al., 2015), big data processing (Zheng et al., 2018), etc. ML is defined as a technique that perceives patterns in datasets, and uses the exposed arrays to forecast future statistics or other consequences of interest. It is a division of AI statistics, that majorly focuses on constructing descriptive and predictive models for a given problem using specific collected datasets. ML modeling is widely used in forest fire monitoring, including all relevant physical properties, i.e., its composition, weather condition, and topography. Numerous algorithms have been developed to characterize and evaluate the responses to fire disturbances in the forest ecosystem (Šerić et al., 2018; Kalantar et al., 2020). Various empirical models have been also used to simulate post-fire foliage retrieval. Wildfire modeling forecasting techniques include physics-based simulators that rely on planners to make many important decisions regarding the allocation of scarce firefighting resources in the event of a fire. However, these physics-based simulators have some restrictions: they generally provide very low accuracy, have a likelihood bias in the areas where they are intended to be used, and often due to the large number of It is difficult to design and implement (Taylor et al., 2013). An early and reliable estimate of fire severity assessment at the field level is essential for the formulation of forest ecosystem policies and strategic plans to protect the environment. The use of ML algorithms for fire monitoring is extremely helpful in sustainability concerns (Jain et al., 2020).
Forest fire continues to end huge forest areas worldwide, mortifying ecosystem services, instigating biodiversity loss, and endangering livelihood sources. It also adds to global warming generally by freeing greenhouse gases from biomass burning and allied soil deterioration. These influences are anticipated to exacerbate by increasing fire occurrences partly intensified by climate change. Despite these major challenges, forest fire management in the area is inadequate by a lack of appropriate policy, capacity building, and mechanical equipment. This is additionally convoluted by diverse topography and antagonistic climatic conditions. This situation demands a vigorous method to fire management comprising a global comprehensive policy recommendation. A such parameter must be inclusive and adequate to embrace different fire management interferences by a dissimilar community. However, such research must be deeply rooted in empirical knowledge generated from robust scientific knowledge about forest fire dynamics, causes, and effects under climate change scenarios. This review highlights the need to understand forest fire mechanisms, risks under global environmental changes, and the use of remote sensing to achieve sustainable outcomes. Based on the verdicts, this review recommends scientific studies for forest fires: (i) Forest fire assessment helps to compile the frequency of fires, the area burned, in general, and to identify the ecological and socio-economic impacts. (ii) Evaluation of fire effectiveness capability helps management at scenario levels. (iii) Development of climate adaptive indicators including other climate disasters. (iv) Further, the review also points to the need for a more remote sensing-based approach at the community level to create awareness about forest fire management. This study is extremely important, as it can be widely used to address the increasing frequency and severity of fire disasters, and climate crises, around the world. Consequently improved satellite-derived indexes, integrating ground-based inventory into fire interference investigation and making it more accurate.
Increasing considerations today focus on climate change as a major aspect of increasing fire association, but researchers and policymakers are only looking at the role of fire emissions in the global carbon cycle as a response to the climate system. Global fire emissions are key components in appropriated carbon movement through terrestrial ecosystems into the atmosphere and other adjacent ecosystems. The increase in high-energy release fires that accompany climate change could accelerate carbon cycling from Earth’s surface to the atmosphere. The traditional monitoring technique is very laborious and expensive. Various studies have been conducted for the estimation of carbon emissions and other trace gases in many countries by combining remote sensing data with forest fire inventory. It is of utmost importance in future research to conduct field experiments to test the generality of forest fire parameters on a global scale. Estimates are slightly restricted by the spatiotemporal availability of high-resolution remote sensing data. However, coarse-resolution satellites, such as SPOT, AVHRR, and moderate resolution imaging spectroradiometer (MODIS), can provide more fire-relevant data, including emissions, severity, and combustion efficiency. Therefore improving fire detection algorithms, reducing data processing and noise as well as incorporating RS data into fire disturbance research can enable and make it more accurate. In contrast, the ML-based method is preferred for conducting fire assessment and surveillance. This integration provides beneficial information that may be useful for measuring the implications of wildfire management.
The author confirms being the sole contributor of this work and has approved it for publication.
The author declares that the research was conducted in the absence of any commercial or financial relationships that could be construed as a potential conflict of interest.
All claims expressed in this article are solely those of the authors and do not necessarily represent those of their affiliated organizations, or those of the publisher, the editors and the reviewers. Any product that may be evaluated in this article, or claim that may be made by its manufacturer, is not guaranteed or endorsed by the publisher.
Abburu, S., and Golla, S. B. (2015). Satellite image classification methods and techniques: A review. Int. J. Comput. Appl. 119, 20–25. doi: 10.5120/21088-3779
Abram, N. J., Henley, B. J., Sen Gupta, A., Lippmann, T. J., Clarke, H., Dowdy, A. J., et al. (2021). Connections of climate change and variability to large and extreme forest fires in southeast Australia. Commun. Earth Environ. 2, 1–17. doi: 10.1038/s43247-020-00065-8
Ahmad, F., and Goparaju, L. (2018). Climate change and its impact on forest fire in the state of Himachal Pradesh and Uttarakhand states of India: Remote sensing and GIS analysis. Contemp. Trends Geosci. 7, 229–246. doi: 10.2478/ctg-2018-0016
Ahmad, F., Uddin, M. M., and Goparaju, L. (2019). Analysis of forest health and socioeconomic dimension in climate change scenario and its future impacts: Remote sensing and GIS approach. Spatial Inform. Res. 27, 385–397. doi: 10.1007/s41324-019-00245-5
Aju, P. C., Iwuchukwu, J. J., and Ibe, C. C. (2015). Our forests, our environment, our sustainable livelihoods. Eur. J. Acad. Essays 2, 6–19.
Allen, M., Antwi-Agyei, P., Aragon-Durand, F., Babiker, M., Bertoldi, P., Bind, M., et al. (2019). Technical summary: Global warming of 1.5° C. An IPCC Special Report on the impacts of global warming of 1.5° C above pre-industrial levels and related global greenhouse gas emission pathways, in the context of strengthening the global response to the threat of climate change, sustainable development, and efforts to eradicate poverty. Geneva: Intergovernmental Panel on Climate Change.
Andreae, M. O. (2019). Emission of trace gases and aerosols from biomass burning–an updated assessment. Atmospheric Chem. Phys. 19, 8523–8546. doi: 10.5194/acp-19-8523-2019
Andrée, B. P. J., Chamorro, A., Spencer, P., Koomen, E., and Dogo, H. (2019). Revisiting the relation between economic growth and the environment; a global assessment of deforestation, pollution and carbon emission. Renew. Sustain. Energy Rev. 114:109221. doi: 10.1016/j.rser.2019.06.028
Ansmann, A., Baars, H., Chudnovsky, A., Mattis, I., Veselovskii, I., Haarig, M., et al. (2018). Extreme levels of Canadian wildfire smoke in the stratosphere over central Europe on 21–22 August 2017. Atmospheric Chem. Phys. 18, 11831–11845. doi: 10.5194/acp-18-11831-2018
Baglivo, M., Baronio, M., Natalini, G., Beccari, T., Chiurazzi, P., Fulcheri, E., et al. (2020). Natural small molecules as inhibitors of coronavirus lipid-dependent attachment to host cells: a possible strategy for reducing SARS-COV-2 infectivity. Acta Bio Medica 91:161. doi: 10.23750/abm.v91i1.9402
Balla, A., Silini, A., Cherif-Silini, H., Chenari Bouket, A., Moser, W. K., Nowakowska, J. A., et al. (2021). The threat of pests and pathogens and the potential for biological control in forest ecosystems. Forests 12:1579. doi: 10.3390/f12111579
Barbosa, P., Camia, A., Kucera, J., Liberta, G., Palumbo, I., San-Miguel-Ayanz, J., et al. (2008). Assessment of forest fire impacts and emissions in the European Union based on the European Forest Fire Information System. Dev. Environ. Sci. 8, 197–208. doi: 10.1016/S1474-8177(08)00008-9
Barmpoutis, P., Papaioannou, P., Dimitropoulos, K., and Grammalidis, N. (2020). A review on early forest fire detection systems using optical remote sensing. Sensors 20:6442. doi: 10.3390/s20226442
Bhattacharjee, S., and Chen, J. (2020). Prediction of satellite-based column CO2 concentration by combining emission inventory and LULC information. IEEE Trans. Geosci. Remote Sens. 58, 8285–8300. doi: 10.1109/TGRS.2020.2985047
Biggart, M., Stocker, J., Doherty, R. M., Wild, O., Hollaway, M., Carruthers, D., et al. (2020). Street-scale air quality modelling for Beijing during a winter 2016 measurement campaign. Atmospheric Chem. Phys. 20, 2755–2780. doi: 10.5194/acp-20-2755-2020
Boer, M. M., Resco de Dios, V., and Bradstock, R. A. (2020). Unprecedented burn area of Australian mega forest fires. Nat. Clim. Change 10, 171–172. doi: 10.1038/s41558-020-0716-1
Boettcher, K. (2021). Seeing red over the climate crisis: Ecosocialism as emergency brake. Doctoral dissertation. California, CA: California State University, Stanislaus.
Briassoulis, H. (2020). Analysis of land use change: Theoretical and modeling approaches. Morgantown, WV: West Virginia University.
Burke, M., Driscoll, A., Heft-Neal, S., Xue, J., Burney, J., and Wara, M. (2021). The changing risk and burden of wildfire in the United States. Proc. Natl. Acad. Sci. U.S.A. 118:e2011048118. doi: 10.1073/pnas.2011048118
Butler, W. H., and Schultz, C. A. (eds) (2019). A new era for collaborative forest management: Policy and practice insights from the Collaborative Forest Landscape Restoration Program. London: Routledge. doi: 10.4324/9781351033381
Campbell, J. L., Fontaine, J. B., and Donato, D. C. (2016). Carbon emissions from decomposition of fire-killed trees following a large wildfire in Oregon, United States. J. Geophys. Res. Biogeosci. 121, 718–730. doi: 10.1002/2015JG003165
Cascio, W. E. (2018). Wildland fire smoke and human health. Sci. Total Environ. 624, 586–595. doi: 10.1016/j.scitotenv.2017.12.086
Chen, X., Chen, W., and Xu, M. (2022). Remote-sensing monitoring of postfire vegetation dynamics in the greater Hinggan Mountain range based on long time-series data: Analysis of the effects of six topographic and climatic factors. Remote Sens. 14:2958. doi: 10.3390/rs14132958
Cheng, A. S., and Dale, L. (2020). Achieving adaptive governance of forest wildfire risk using competitive grants: Insights from the Colorado Wildfire Risk Reduction Grant Program. Rev. Pol. Res. 37, 657–686. doi: 10.1111/ropr.12379
Churkina, G., Organschi, A., Reyer, C. P., Ruff, A., Vinke, K., Liu, Z., et al. (2020). Buildings as a global carbon sink. Nat. Sustain. 3, 269–276. doi: 10.1038/s41893-019-0462-4
Chuvieco, E., Aguado, I., Salas, J., García, M., Yebra, M., and Oliva, P. (2020). Satellite remote sensing contributions to wildland fire science and management. Curr. For. Rep. 6, 81–96. doi: 10.1007/s40725-020-00116-5
Chuvieco, E., Aguado, I., Yebra, M., Nieto, H., Salas, J., Martín, M. P., et al. (2010). Development of a framework for fire risk assessment using remote sensing and geographic information system technologies. Ecol. Modell. 221, 46–58. doi: 10.1016/j.ecolmodel.2008.11.017
Chuvieco, E., Lizundia-Loiola, J., Pettinari, M. L., Ramo, R., Padilla, M., Tansey, K., et al. (2018). Generation and analysis of a new global burned area product based on MODIS 250 m reflectance bands and thermal anomalies. Earth Syst. Sci. Data 10, 2015–2031. doi: 10.5194/essd-10-2015-2018
Conard, S. G., Sukhinin, A. I., Stocks, B. J., Cahoon, D. R., Davidenko, E. P., and Ivanova, G. A. (2002). Determining effects of area burned and fire severity on carbon cycling and emissions in Siberia. Clim. Change 55, 197–211. doi: 10.1023/A:1020207710195
Cruz-López, M. I., Manzo-Delgado, L. D. L., Aguirre-Gómez, R., Chuvieco, E., and Equihua-Benítez, J. A. (2019). Spatial distribution of forest fire emissions: A case study in three mexican ecoregions. Remote Sens. 11:1185. doi: 10.3390/rs11101185
DellaSala, D. A., Baker, B. C., Hanson, C. T., Ruediger, L., and Baker, W. (2022). Have western USA fire suppression and megafire active management approaches become a contemporary Sisyphus? Biol. Conserv. 268:109499. doi: 10.1016/j.biocon.2022.109499
Desservettaz, M. J., Fisher, J. A., Luhar, A. K., Woodhouse, M. T., Bukosa, B., Buchholz, R. R., et al. (2022). Australian fire emissions of carbon monoxide estimated by global biomass burning inventories: Variability and observational constraints. J. Geophys. Res. Atmospheres 127:e2021JD035925. doi: 10.1029/2021JD035925
Dong, C., Williams, A. P., Abatzoglou, J. T., Lin, K., Okin, G. S., Gillespie, T. W., et al. (2022). The season for large fires in Southern California is projected to lengthen in a changing climate. Commun. Earth Environ. 3, 1–9. doi: 10.1038/s43247-022-00344-6
Ertugrul, M., Varol, T., Ozel, H. B., Cetin, M., and Sevik, H. (2021). Influence of climatic factor of changes in forest fire danger and fire season length in Turkey. Environ. Monit. Assess. 193, 1–17. doi: 10.1007/s10661-020-08800-6
French, N. H., de Groot, W. J., Jenkins, L. K., Rogers, B. M., Alvarado, E., Amiro, B., et al. (2011). Model comparisons for estimating carbon emissions from North American wildland fire. J. Geophys. Res. Biogeosci. 116, 1–21. doi: 10.1029/2010JG001469
Grace, J. (2004). Understanding and managing the global carbon cycle. J. Ecol. 92, 189–202. doi: 10.1111/j.0022-0477.2004.00874.x
Gupta, G. S. (2019). Land degradation and challenges of food security. Rev. Eur. Stud. 11:63. doi: 10.5539/res.v11n1p63
Han, A., Qing, S., Bao, Y., Na, L., Bao, Y., Liu, X., et al. (2021). Short-term effects of fire severity on vegetation based on sentinel-2 satellite data. Sustainability 13:432. doi: 10.3390/su13010432
Hartter, J., Hamilton, L. C., Ducey, M. J., Boag, A. E., Salerno, J. D., Christoffersen, N. D., et al. (2020). Finding common ground: Agreement on increasing wildfire risk crosses political lines. Environ. Res. Lett. 15:065002. doi: 10.1088/1748-9326/ab7ace
He, J., Loboda, T. V., Chen, D., and French, N. H. (2022). Cloud-to-Ground lightning and near-surface fire weather control wildfire occurrence in Arctic Tundra. Geophys. Res. Lett. 49:e2021GL096814. doi: 10.1029/2021GL096814
Heath, L. S., Smith, J. E., Skog, K. E., Nowak, D. J., and Woodall, C. W. (2011). Managed Forest carbon estimates for the US greenhouse gas inventory, 1990—2008. J. For. 109, 167–173.
Hong, M., Razaviyayn, M., Luo, Z. Q., and Pang, J. S. (2015). A unified algorithmic framework for block-structured optimization involving big data: With applications in machine learning and signal processing. IEEE Signal Process. Magazine 33, 57–77. doi: 10.1109/MSP.2015.2481563
Ichoku, C. (2020). African biomass burning and its atmospheric impacts. Oxford: Oxford Research Encyclopedia of Climate Science. doi: 10.1093/acrefore/9780190228620.013.523
Isaev, A. S., Korovin, G. N., Bartalev, S. A., Ershov, D. V., Janetos, A., Kasischke, E. S., et al. (2002). Using remote sensing to assess Russian forest fire carbon emissions. Clim. Change 55, 235–249. doi: 10.1023/A:1020221123884
Jaffe, D. A., O’Neill, S. M., Larkin, N. K., Holder, A. L., Peterson, D. L., Halofsky, J. E., et al. (2020). Wildfire and prescribed burning impacts on air quality in the United States. J. Air Waste Manag. Assoc. 70, 583–615. doi: 10.1080/10962247.2020.1749731
Jain, P., Coogan, S. C., Subramanian, S. G., Crowley, M., Taylor, S., and Flannigan, M. D. (2020). A review of machine learning applications in wildfire science and management. Environ. Rev. 28, 478–505. doi: 10.1139/er-2020-0019
Jhariya, M. K., Banerjee, A., Meena, R. S., and Yadav, D. K. (eds) (2019). Sustainable agriculture, forest and environmental management. Berlin: Springer. doi: 10.1007/978-981-13-6830-1
Junpen, A., Garivait, S., Bonnet, S., and Pongpullponsak, A. (2011). Spatial and temporal distribution of forest fire PM10 emission estimation by using remote sensing information. Int. J. Environ. Sci. Dev. 2:156. doi: 10.7763/IJESD.2011.V2.115
Kahanji, C., Walls, R. S., and Cicione, A. (2019). Fire spread analysis for the 2017 Imizamo Yethu informal settlement conflagration in South Africa. Int. J. Disaster Risk Reduct. 39:101146. doi: 10.1016/j.ijdrr.2019.101146
Kalantar, B., Ueda, N., Idrees, M. O., Janizadeh, S., Ahmadi, K., and Shabani, F. (2020). Forest fire susceptibility prediction based on machine learning models with resampling algorithms on remote sensing data. Remote Sens. 12:3682. doi: 10.3390/rs12223682
Kawa, S. R., Abshire, J. B., Baker, D. F., Browell, E. V., Crisp, D., Crowell, S. M., et al. (2018). Active sensing of CO2 emissions over nights, days, and seasons (ASCENDS): Final report of the ASCENDS Ad Hoc science definition team (No. NASA/TP? 2018-219034). Washington, DC: NASA.
Kelley, D. I., Bistinas, I., Whitley, R., Burton, C., Marthews, T. R., and Dong, N. (2019). How contemporary bioclimatic and human controls change global fire regimes. Nat. Clim. Change 9, 690–696. doi: 10.1038/s41558-019-0540-7
Kim, S. J., Lim, C. H., Kim, G. S., Lee, J., Geiger, T., Rahmati, O., et al. (2019). Multi-temporal analysis of forest fire probability using socio-economic and environmental variables. Remote Sens. 11:86. doi: 10.3390/rs11010086
Kizer, K. W. (2020). Extreme wildfires—a growing population health and planetary problem. JAMA 324, 1605–1606. doi: 10.1001/jama.2020.19334
Koristekova, K., Vido, J., Vida, T., Vyskot, I., Mikloš, M., Minïáš, J., et al. (2020). Evaluating the amount of potential greenhouse gas emissions from forest fires in the area of the Slovak Paradise National Park. Biologia 75, 885–898. doi: 10.2478/s11756-020-00461-7
Krawchuk, M. A., Haire, S. L., Coop, J., Parisien, M. A., Whitman, E., Chong, G., et al. (2016). Topographic and fire weather controls of fire refugia in forested ecosystems of northwestern North America. Ecosphere 7:e01632. doi: 10.1002/ecs2.1632
Krawchuk, M. A., Meigs, G. W., Cartwright, J. M., Coop, J. D., Davis, R., Holz, A., et al. (2020). Disturbance refugia within mosaics of forest fire, drought, and insect outbreaks. Front. Ecol. Environ. 18, 235–244. doi: 10.1002/fee.2190
Krylov, A., McCarty, J. L., Potapov, P., Loboda, T., Tyukavina, A., Turubanova, S., et al. (2014). Remote sensing estimates of stand-replacement fires in Russia, 2002–2011. Environ. Res. Lett. 9:105007. doi: 10.1088/1748-9326/9/10/105007
Lasslop, G., Coppola, A. I., Voulgarakis, A., Yue, C., and Veraverbeke, S. (2019). Influence of fire on the carbon cycle and climate. Curr. Clim. Change Rep. 5, 112–123. doi: 10.1007/s40641-019-00128-9
Li, Z., Nadon, S., and Cihlar, J. (2000). Satellite-based detection of Canadian boreal forest fires: Development and application of the algorithm. Int. J. Remote Sens. 21, 3057–3069. doi: 10.1080/01431160050144956
Littell, J. S., Peterson, D. L., Riley, K. L., Liu, Y., and Luce, C. H. (2016). A review of the relationships between drought and forest fire in the United States. Glob. Change Biol. 22, 2353–2369. doi: 10.1111/gcb.13275
Liu, Y., Goodrick, S., and Heilman, W. (2014). Wildland fire emissions, carbon, and climate: Wildfire–climate interactions. For. Ecol. Manag. 317, 80–96. doi: 10.1016/j.foreco.2013.02.020
Lü, A., Tian, H., Liu, M., Liu, J., and Melillo, J. M. (2006). Spatial and temporal patterns of carbon emissions from forest fires in China from 1950 to 2000. J. Geophys. Res. 111:D05313. doi: 10.1029/2005JD006198
Mahmoud, M. I., Campbell, M. J., Sloan, S., Alamgir, M., and Laurance, W. F. (2020). Land-cover change threatens tropical forests and biodiversity in the Littoral Region, Cameroon. Oryx 54, 882–891. doi: 10.1017/S0030605318000881
Mangiameli, M., Mussumeci, G., and Cappello, A. (2021). Forest fire spreading using free and open-source GIS technologies. Geomatics 1, 50–64. doi: 10.3390/geomatics1010005
Masyagina, O. V. (2021). Carbon dioxide emissions and vegetation recovery in fire-affected forest ecosystems of Siberia: Recent local estimations. Curr. Opin. Environ. Sci. Health 23:100283. doi: 10.1016/j.coesh.2021.100283
Mateus, S., and Gaspar, R. (2018). “Tweeting a tragedy: a quantitative-qualitative analysis of appraisal and coping expressions, during and after the pedrógão grande forest fire,” in Proceedings of the SRA-E-IBERIAN chapter (SRA-EI) conference, 84.
McCarthy, H. R., Oren, R., Johnsen, K. H., Gallet-Budynek, A., Pritchard, S. G., Cook, C. W., et al. (2010). Re-assessment of plant carbon dynamics at the Duke free-air CO2 enrichment site: Interactions of atmospheric [CO2] with nitrogen and water availability over stand development. New Phytol. 185, 514–528. doi: 10.1111/j.1469-8137.2009.03078.x
McGlade, C., and Ekins, P. (2015). The geographical distribution of fossil fuels unused when limiting global warming to 2 C. Nature 517, 187–190. doi: 10.1038/nature14016
Ménard, L. P., Ruel, J. C., and Thiffault, N. (2019). Abundance and impacts of competing species on conifer regeneration following careful logging in the eastern Canadian boreal forest. Forests 10:177. doi: 10.3390/f10020177
Menezes, L. S., de Oliveira, A. M., Santos, F. L., Russo, A., de Souza, R. A., Roque, F. O., et al. (2022). Lightning patterns in the Pantanal: Untangling natural and anthropogenic-induced wildfires. Sci. Tot. Environ. 820:153021. doi: 10.1016/j.scitotenv.2022.153021
Oliveras, I., Anderson, L. O., and Malhi, Y. (2014). Application of remote sensing to understanding fire regimes and biomass burning emissions of the tropical Andes. Glob. Biogeochem. Cycles 28, 480–496. doi: 10.1002/2013GB004664
Pausas, J. G., and Fernández-Muñoz, S. (2012). Fire regime changes in the Western Mediterranean Basin: From fuel-limited to drought-driven fire regime. Clim. Change 110, 215–226. doi: 10.1007/s10584-011-0060-6
Pook, E. W., and Gill, A. M. (1993). Variation of live and dead fine fuel moisture in Pinus radiata plantations of the Australian-Capital-Territory. Int. J. Wildland Fire 3, 155–168. doi: 10.1071/WF9930155
Reid, P. C., Hari, R. E., Beaugrand, G., Livingstone, D. M., Marty, C., Straile, D., et al. (2016). Global impacts of the 1980s regime shift. Glob. Change Biol. 22, 682–703. doi: 10.1111/gcb.13106
Reinhardt, E. D., Keane, R. E., Calkin, D. E., and Cohen, J. D. (2008). Objectives and considerations for wildland fuel treatment in forested ecosystems of the interior western United States. For. Ecol. Manag. 256, 1997–2006. doi: 10.1016/j.foreco.2008.09.016
Riggan, P. J., Tissell, R. G., Lockwood, R. N., Brass, J. A., Pereira, J. A. R., Miranda, H. S., et al. (2004). Remote measurement of energy and carbon flux from wildfires in Brazil. Ecol. Appl. 14, 855–872. doi: 10.1890/02-5162
Roberts, L. J., Burnett, R., and Fogg, A. (2021). Fire and mechanical forest management treatments support different portions of the bird community in fire-suppressed forests. Forests 12:150. doi: 10.3390/f12020150
Rogers, A. J. (2017). Anthropogenic modification of the natural fire landscape and its consequences for vegetation patterns on the Cape Peninsula. Master’s thesis. Rondebosch: University of Cape Town.
Roula, S. E., Bouhraoua, R. T., and Catry, F. X. (2020). Factors affecting post-fire regeneration after coppicing of cork oak (Quercus suber) trees in northeastern Algeria. Can. J. For. Res. 50, 371–379. doi: 10.1139/cjfr-2019-0181
Roy, P. S. (2003). Forest fire and degradation assessment using satellite remote sensing and geographic information system. Satellite Remote Sens. GIS Appl. Agric. Meteorol. 361:400.
Sannigrahi, S., Pilla, F., Basu, B., Basu, A. S., Sarkar, K., Chakraborti, S., et al. (2020). Examining the effects of forest fire on terrestrial carbon emission and ecosystem production in India using remote sensing approaches. Sci. Tot. Environ. 725:138331. doi: 10.1016/j.scitotenv.2020.138331
Sarker, I. H. (2021). Machine learning: Algorithms, real-world applications and research directions. SN Comput. Sci. 2, 1–21. doi: 10.1007/s42979-021-00592-x
Schneising, O., Buchwitz, M., Reuter, M., Bovensmann, H., and Burrows, J. P. (2020). Severe Californian wildfires in November 2018 observed from space: The carbon monoxide perspective. Atmospheric Chem. Phys. 20, 3317–3332. doi: 10.5194/acp-20-3317-2020
Schoennagel, T., Balch, J. K., Brenkert-Smith, H., Dennison, P. E., Harvey, B. J., Krawchuk, M. A., et al. (2017). Adapt to more wildfire in western North American forests as climate changes. Proc. Natl. Acad. Sci. 114, 4582–4590. doi: 10.1073/pnas.1617464114
Scholes, R., Montanarella, L., Brainich, A., Barger, N., ten Brink, B., Cantele, M., et al. (2018). Summary for policymakers of the assessment report on land degradation and restoration of the Intergovernmental Science-Policy Platform on Biodiversity and Ecosystem Services. Bonn: IPBES secretariat.
Seidl, R., Schelhaas, M. J., Rammer, W., and Verkerk, P. J. (2014). Increasing forest disturbances in Europe and their impact on carbon storage. Nat. Clim. Change 4, 806–810. doi: 10.1038/nclimate2318
Seiler, W., and Crutzen, P. J. (1980). Estimates of gross and net fluxes of carbon between the biosphere and the atmosphere from biomass burning. Clim. Change 2, 207–247. doi: 10.1007/BF00137988
Šerić, L., Stipaničev, D., and Krstinić, D. (2018). “ML/AI in intelligent forest fire observer network,” in Proceedings of the MMS 2018: 3rd EAI international conference on management of manufacturing systems (Ghent: European Alliance for Innovation), 126. doi: 10.4108/eai.6-11-2018.2279681
Shah, R. (2022). A comprehensive study on the effect of wasted food on greenhouse gas emissions. Mercury. Available online at: https://www.envirotech-online.com/article/environmental-laboratory/7/koehler-instrument-company/a-comprehensive-study-on-the-effect-of-wasted-food-on-greenhouse-gas-emissions/3088
Singh, B. O. (2015). The effects of grain size on morphological patterns and Land cover within boreal wildfire residual patches. Available online at: https://yorkspace.library.yorku.ca/xmlui/handle/10315/30702
Singh, S., and Suresh Babu, K. V. (2021). “Forest fire susceptibility mapping for Uttarakhand state by using geospatial techniques,” in Recent technologies for disaster management and risk reduction, eds P. K. Rai, P. Singh, and V. N. Mishra (Cham: Springer), 173–188. doi: 10.1007/978-3-030-76116-5_11
Smith, J. M. B. (1974). Origins and ecology of the non-forest flora of Mt. Wilhelm, New Guinea. Canberra: The Australian National University.
Stephens, S. L., Agee, J. K., Fule, P. Z., North, M. P., Romme, W. H., Swetnam, T. W., et al. (2013). Managing forests and fire in changing climates. Science 342, 41–42. doi: 10.1126/science.1240294
Stephens, S. L., McIver, J. D., Boerner, R. E., Fettig, C. J., Fontaine, J. B., Hartsough, B. R., et al. (2012). The effects of forest fuel-reduction treatments in the United States. BioScience 62, 549–560. doi: 10.1525/bio.2012.62.6.6
Tabor, K., Hewson, J., Tien, H., González-Roglich, M., Hole, D., and Williams, J. W. (2018). Tropical protected areas under increasing threats from climate change and deforestation. Land 7:90. doi: 10.3390/land7030090
Talerko, M., Kovalets, I., Lev, T, Igarashi, Y., and Romanenko, O. (2021). Simulation study of radionuclide atmospheric transport after wildland fires in the Chernobyl Exclusion Zone in April 2020. Atmospheric Pollut. Res. 12, 193–204. doi: 10.1016/j.apr.2021.01.010
Taylor, S. W., Woolford, D. G., Dean, C. B., and Martell, D. L. (2013). Wildfire prediction to inform fire management: Statistical science challenges. Stat. Sci. 28, 586–615. doi: 10.1214/13-STS451
Teckentrup, L., Harrison, S. P., Hantson, S., Heil, A., Melton, J. R., Forrest, M., et al. (2019). Response of simulated burned area to historical changes in environmental and anthropogenic factors: A comparison of seven fire models. Biogeosciences 16, 3883–3910. doi: 10.5194/bg-16-3883-2019
Trumper, M. L., Griffin, D., Hobbie, S. E., Howard, I. M., Nelson, D. M., Reich, P. B., et al. (2020). Century-scale wood nitrogen isotope trajectories from an oak savanna with variable fire frequencies. Biogeosciences 17, 4509–4522. doi: 10.5194/bg-17-4509-2020
Turco, M., Rosa-Cánovas, J. J., Bedia, J., Jerez, S., Montávez, J. P., Llasat, M. C., et al. (2018). Exacerbated fires in Mediterranean Europe due to anthropogenic warming projected with non-stationary climate-fire models. Nat. Commun. 9, 1–9. doi: 10.1038/s41467-018-06358-z
Van der Werf, G. R., Randerson, J. T., Giglio, L., Collatz, G. J., Mu, M., Kasibhatla, P. S., et al. (2010). Global fire emissions and the contribution of deforestation, savanna, forest, agricultural, and peat fires (1997–2009). Atmospheric Chem. Phys. 10, 11707–11735. doi: 10.5194/acp-10-11707-2010
Van Der Werf, G. R., Randerson, J. T., Giglio, L., Van Leeuwen, T. T., Chen, Y., Rogers, B. M., et al. (2017). Global fire emissions estimates during 1997–2016. Earth Syst. Sci. Data 9, 697–720. doi: 10.5194/essd-9-697-2017
Van Dijk, J. (2019). “Biodiversity and nature,” in The Netherlands and the Dutch. World regional geography book series (Cham: Springer). doi: 10.1007/978-3-319-75073-6_5
Ware, J., Kort, E. A., Duren, R., Mueller, K. L., Verhulst, K., and Yadav, V. (2019). Detecting urban emissions changes and events with a near-real-time-capable inversion system. J. Geophys. Res. Atmospheres 124, 5117–5130. doi: 10.1029/2018JD029224
Wei, T. M., and Barros, A. P. (2021). Prospects for long-term agriculture in Southern Africa: Emergent dynamics of savannah ecosystems from remote sensing observations. Remote Sens. 13:2954. doi: 10.3390/rs13152954
Williamson, T. B., Johnston, M. H., Nelson, H. W., and Edwards, J. E. (2019). Adapting to climate change in Canadian forest management: Past, present and future. For. Chronicle 95, 76–90. doi: 10.5558/tfc2019-015
Wokekoro, E. (2020). The impact of forest fires on property values and the environment. Int. J. Res. Appl. Nat. Soc. Sci. 8, 51–62.
Wu, Y., Arapi, A., Huang, J., Gross, B., and Moshary, F. (2018). Intra-continental wildfire smoke transport and impact on local air quality observed by ground-based and satellite remote sensing in New York City. Atmospheric Environ. 187, 266–281. doi: 10.1016/j.atmosenv.2018.06.006
Xu, W., He, H. S., Hawbaker, T. J., Zhu, Z., and Henne, P. D. (2020). Estimating burn severity and carbon emissions from a historic megafire in boreal forests of China. Sci. Tot. Environ. 716:136534. doi: 10.1016/j.scitotenv.2020.136534
Zamora-Gutierrez, V., Rivera-Villanueva, A. N., Martinez Balvanera, S., Castro-Castro, A., and Aguirre-Gutiérrez, J. (2021). Vulnerability of bat–plant pollination interactions due to environmental change. Glob. Change Biol. 27, 3367–3382. doi: 10.1111/gcb.15611
Zhang, Y. H., Wooster, M. J., Tutubalina, O., and Perry, G. L. W. (2003). Monthly burned area and forest fire carbon emission estimates for the Russian Federation from SPOT VGT. Remote Sens. Environ. 87, 1–15. doi: 10.1016/S0034-4257(03)00141-X
Zheng, S., Chen, S., Yang, L., Zhu, J., Luo, Z., Hu, J., et al. (2018). Big data processing architecture for radio signals empowered by deep learning: Concept, experiment, applications and challenges. IEEE Access 6, 55907–55922. doi: 10.1109/ACCESS.2018.2872769
Zhou, X., Prigent, C., and Yamazaki, D. (2021). Toward improved comparisons between land-surface-water-area estimates from a global river model and satellite observations. Water Resour. Res. 57:e2020WR029256. doi: 10.1029/2020WR029256
Keywords: forest fire, emissions, greenhouse gases, climate change, remote sensing, GIS, machine learning
Citation: Singh S (2022) “Forest fire emissions: A contribution to global climate change”. Front. For. Glob. Change 5:925480. doi: 10.3389/ffgc.2022.925480
Received: 21 April 2022; Accepted: 07 November 2022;
Published: 29 November 2022.
Edited by:
K. V. Suresh Babu, University of Cape Town, South AfricaReviewed by:
Maruti Kumar Mudunuru, Pacific Northwest National Laboratory (DOE), United StatesCopyright © 2022 Singh. This is an open-access article distributed under the terms of the Creative Commons Attribution License (CC BY). The use, distribution or reproduction in other forums is permitted, provided the original author(s) and the copyright owner(s) are credited and that the original publication in this journal is cited, in accordance with accepted academic practice. No use, distribution or reproduction is permitted which does not comply with these terms.
*Correspondence: Swati Singh, c3dhdGkuc2lrYXJ3YXIxMkBnbWFpbC5jb20=
Disclaimer: All claims expressed in this article are solely those of the authors and do not necessarily represent those of their affiliated organizations, or those of the publisher, the editors and the reviewers. Any product that may be evaluated in this article or claim that may be made by its manufacturer is not guaranteed or endorsed by the publisher.
Research integrity at Frontiers
Learn more about the work of our research integrity team to safeguard the quality of each article we publish.