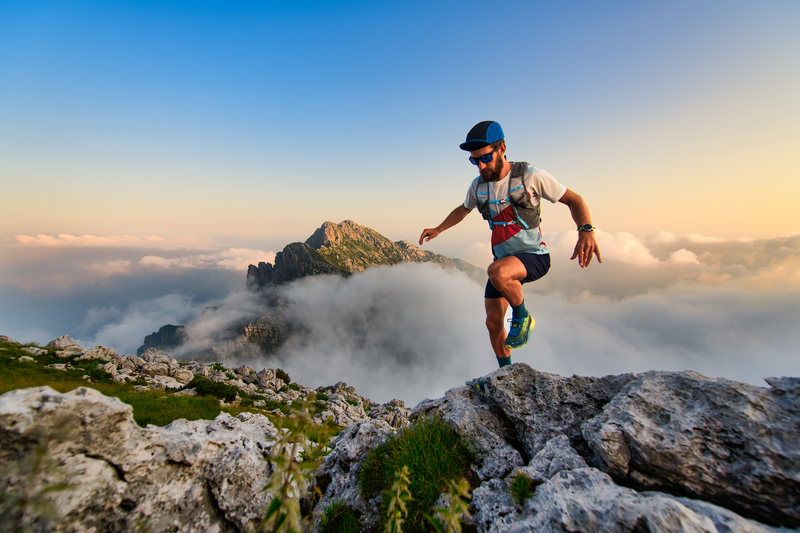
95% of researchers rate our articles as excellent or good
Learn more about the work of our research integrity team to safeguard the quality of each article we publish.
Find out more
ORIGINAL RESEARCH article
Front. For. Glob. Change , 25 October 2022
Sec. Fire and Forests
Volume 5 - 2022 | https://doi.org/10.3389/ffgc.2022.880963
This article is part of the Research Topic Transformation of Tropical Forests Through Fire View all 6 articles
The Amazon forest represents one of the world’s largest terrestrial carbon reservoirs. Here, we evaluated the role of soil texture, climate, vegetation, and distance to savanna on the distribution and stocks of soil pyrogenic carbon (PyC) in intact forests with no history of recent fire spanning the southern Amazonia forest-Cerrado Zone of Transition (ZOT). In 19 one hectare forest plots, including three Amazonian Dark Earth (ADE, terra preta) sites with high soil PyC, we measured all trees and lianas with diameter ≥ 10 cm and analyzed soil physicochemical properties, including texture and PyC stocks. We quantified PyC stocks as a proportion of total organic carbon using hydrogen pyrolysis. We used multiple linear regression and variance partitioning to determine which variables best explain soil PyC variation. For all forests combined, soil PyC stocks ranged between 0.9 and 6.8 Mg/ha to 30 cm depth (mean 2.3 ± 1.5 Mg/ha) and PyC, on average, represented 4.3% of the total soil organic carbon (SOC). The most parsimonious model (based on AICc) included soil clay content and above-ground biomass (AGB) as the main predictors, explaining 71% of soil PyC variation. After removal of the ADE plots, PyC stocks ranged between 0.9 and 3.8 Mg/ha (mean 1.9 ± 0.8 Mg/ha–1) and PyC continued to represent ∼4% of the total SOC. The most parsimonious models without ADE included AGB and sand as the best predictors, with sand and PyC having an inverse relationship, and sand explaining 65% of the soil PyC variation. Partial regression analysis did not identify any of the components (climatic, environmental, and edaphic), pure or shared, as important in explaining soil PyC variation with or without ADE plots. We observed a substantial amount of soil PyC, even excluding ADE forests; however, contrary to expectations, soil PyC stocks were not higher nearer to the fire-dependent Cerrado than more humid regions of Amazonia. Our findings that soil texture and AGB explain the distribution and amount of soil PyC in ZOT forests will help to improve model estimates of SOC change with further climatic warming.
The Amazon rainforest is one of the world’s largest terrestrial above- and below-ground carbon (C) pools (Feldpausch et al., 2012; Ometto et al., 2014). With intensifying land-use change and severe drought, C emissions due to fire are increasing (Aragão et al., 2018), thereby reversing a multi-decadal C sink (Phillips et al., 2009; Brienen et al., 2015; Feldpausch et al., 2016). The central and northwestern regions of Amazonia, with high annual precipitation and short or no dry season, are resistant to burning in non-drought years (Pontes-Lopes et al., 2021), but in the transitional forests of southern Amazonia, a combination of lower annual precipitation, a pronounced dry season, and proximity to flammable Cerrado vegetation, increase the probability of fire in the presence of ignition sources (Balch et al., 2008; Nogueira et al., 2019; Prestes et al., 2020). The conditions promoting fire and the proportion of closed-canopy forest cover have varied over time, with palaeo-records for south and southwestern Amazonia indicating drier conditions during the mid-Holocene (Burbridge et al., 2004; Kury et al., 2021), followed by an expansion of closed-canopy forest over the past 2000 years (Burbridge et al., 2004).
Across the southern Amazon forest-Cerrado zone of ecological tension (ZOT), therefore, fire has been a long-term driver of vegetation dynamics (Mayle et al., 2000, 2007; Kury et al., 2021) and has contributed to the transformation of a proportion of above-ground biomass (AGB) into charcoal (Koele et al., 2017). The development of soil pyrogenic carbon (PyC), a soil organic carbon (SOC) fraction formed by the incomplete combustion of plant biomass, represents a SOC fraction resistant to decomposition that may persist for millennia (Bird et al., 2015). Determining the drivers of variation in soil PyC stocks is important for understanding past fire history, the contribution of PyC to total SOC stocks, and determining SOC sensitivity to current and future fire regimes and climate change.
Global estimates suggest an annual PyC production of 50–270 Tg (Kuhlbusch and Crutzen, 1995; Wei et al., 2018), with vegetation burning being responsible for up to 28 Tg year–1. Estimated inputs of PyC by atmospheric deposition from fossil fuel and biomass burning aerosols in Amazonia (∼6 kg km–2 yr–1) are low (Coppola et al., 2019), indicating many thousands of years would be required to develop the current Amazonian soil PyC stock, estimated to be 2.67 Pg over 0–100 cm soil depth (Koele et al., 2017). Therefore, the majority of soil PyC found in forests with no history of recent fire is likely due to in situ historical fires and/or local edaphic or topographic conditions favoring the long-term accumulation of soil PyC. Radiocarbon dating of macro-charcoal fragments from the soil indicates Amazon forests have periodically burned throughout the Holocene (Sanford et al., 1985; Goulart et al., 2017; McMichael and Bush, 2019; Feldpausch et al., 2022), with some old-growth forests having fire return intervals of hundreds of years and having experienced no fire for the last ∼800 years (Feldpausch et al., 2022).
The PyC fraction of SOC is more resistant to degradation than other SOC forms due to its refractory polyaromatic composition, as well as potential interactions with the clay mineral matrix through the formation of organo-mineral associations (Czimczik and Masiello, 2007). However, there is still uncertainty about how long PyC persists in soils, or how global warming will affect soil PyC stocks. Some studies have shown that most of the larger PyC particles remain in the soil after fire (Suman et al., 1997; Gustafsson and Gschwend, 1998; Masiello, 2004). Depending on the temperature of formation and organic matter of origin of PyC, mineral phase, and soil texture, PyC can remain in the soil much longer than other forms of SOC, and in some cases for thousands of years (Singh et al., 2014; Bird et al., 2015). Dissolved and fine particulate PyC fractions may be translocated to deeper soil depths and lost to erosion over years to decades, especially with heavy rainfall (Major et al., 2010), and these effects may be greater in sandy soils and forests with steeper slopes (Rumpel et al., 2006).
The ZOT between the two largest Brazilian biomes, the Amazon forests and the Cerrado, is located in a seasonal climate, with a dry period of up to 6 months, average temperature above 25°C, and annual precipitation predominantly between 1400 and 2000 mm (Silva et al., 2008). While an increase in temperatures with global warming (IPCC, 2018) is likely to increase the rate of respiration of SOC to the atmosphere (Davidson et al., 2006), for recalcitrant PyC fractions losses could be much lower since PyC stocks are more resistant to degradation in response to warming (Lehmann et al., 2008). The more open vegetation and the low stratum that dries during the dry season, that are typical of the Cerrado (Ribeiro and Walter, 2008), favor the entry of fires in transition forests at higher frequency than moist forests (Balch et al., 2008) and therefore, likely the production of PyC. Although these seasonal evergreen forests show relative fire resistance, this resistance significantly changes with an increase in fire frequency, and intensity, exacerbated by extreme weather events and land-use change (Balch et al., 2008; Brando et al., 2020). Thus, these factors, combined with the precipitation gradient and differences in vegetation structure such as height (Barbier et al., 2010; Feldpausch et al., 2011), biomass (Fauset et al., 2015), and composition (Morandi et al., 2016) across the transition may interact to affect soil PyC stocks.
Some factors could also act ambiguously to affect SOC and PyC stocks across the ZOT. For example, AGB stocks are generally greater with higher precipitation, providing more fuel for fire and potentially more charcoal during burning (Fearnside et al., 2001). However, higher AGB and higher precipitation can maintain higher sub-canopy moisture, preventing combustion of dead leaves and small branches that act as fine fuel and thereby reduce the capacity of these components to carry a fire (Ray et al., 2005). Likewise, soils with more clay can protect PyC from decomposition through the formation of organo-mineral compounds (Glaser et al., 2003); however, the lower moisture content (Metcalfe et al., 2007) of sandier soils may slow the decomposition of SOC and PyC. Therefore, a combination of edaphic, climatic, and vegetation factors may interact to affect soil PyC. Thus, we hypothesize that forests located in regions with lower annual precipitation, combined with more clayey soils, will have higher PyC than sandy soils located in regions with higher precipitation. Furthermore, we hypothesize that PyC stocks will vary with AGB, as AGB directly reflects the amount of material that can be converted to PyC during burning (Fearnside et al., 2001; Preston and Schmidt, 2006). Our study aims to evaluate the interaction of multiple drivers in controlling the distribution and stocks of forest PyC across the Amazon-Cerrado transition. We address the following questions: (1) How much soil PyC is stored in forests across the Amazon-Cerrado transition? (2) How do PyC stocks relate to soil texture, forest AGB, and climate? (3) How does the distance of each sampled location to the current Cerrado biome border influence forest soil PyC?
We conducted this study in 19 permanent forest plots (forests of the Amazon-Cerrado transition), representing structurally intact forests with no history of recent fire (at least since the availability of satellite data) in the southeastern and southwestern border zone of the Amazon Basin, with sixteen plots located in Brazil and three in Bolivia (Table 1 and Figure 1). Plots in Brazil’s forests, in southeastern Amazonia, are distributed over two states: Mato Grosso, covering the municipalities of Nova Xavantina, Ribeirão Cascalheira, Querência, São Félix do Araguaia, Porto Alegre do Norte, Santa Terezinha, Gaúcha do Norte and Alta Floresta; and in Pará, in the municipality of Santana do Araguaia. The plots in Bolivia in southwestern Amazonia, are located in the Department of Santa Cruz, bordering Mato Grosso/Brazil.
Table 1. Forest plot codes (RAINFOR codes), states (MT, Mato Grosso; PA, Pará; SC, Santa Cruz province), Country (BR, Brazil and BO, Bolivia), geographic location, annual precipitation (PA), average annual temperature (TA), soil types (Soil Survey Staff, 1999), and soil PyC/Total Organic Carbon (TOC) of areas sampled along the Amazon-Cerrado transition.
Figure 1. The location of the permanent forest plots along the Amazon-Cerrado transition. The blue line represents the limit of the Legal Amazon, the black line country boundaries, and the pink line the limit of the Cerrado biome.
We collected vegetation and soil data following the standard RAINFOR protocol (Phillips et al., 2010); all plot data are archived and managed through ForestPlots.net (Lopez-Gonzalez et al., 2009, 2011) and contribute to regional and international plot networks (ForestPlots.net et al., 2021). The average annual precipitation of the region varies between 1,451 and 2,353 mm and the average temperature from 23.9 to 26.9°C (Worldclim 1.4, Hijmans et al., 2005; Table 1).
The forests of the Amazon-Cerrado transition grow on deep, highly weathered soils, mainly represented by Ultisols and Oxisols with low pH and cation exchange capacity (CEC), as well as largely occluded P due to the high levels of Fe and Al oxide minerals (Quesada et al., 2011). As a result of extensive sandstone and granitic rocks (Sombroek, 1966), the Brazilian soils are quite sandy and have low fertility (Reatto et al., 2008). The Ultisols comprise soils consisting of mineral material, with the presence of a textural B horizon of clay of low or high activity combined with low base saturation (EMBRAPA, 2006). Their striking feature is an increase in clay from the surface horizon A to the subsurface B. The depth ranges from shallow to deep (IBGE, 2015).
Oxisols are very weathered, deep and well-drained soils. In general, they are strongly acidic, with low base saturation, dystrophic or aluminum-rich soils (EMBRAPA, 2006). Together with the Ultisols, they are the most extensive soils in Brazil (IBGE, 2015). Many different vegetation types are found on this soil type, ranging from more open formations, such as campo cerrado, to forest formations such as cerradão (Marimon-Junior and Haridasan, 2005), to seasonal evergreen and ever wet forests (Ivanauskas et al., 2008). Pre-Columbian land-use also modified vegetation and soils through the production of Anthrosols (Amazon Dark Earths, ADE, terra preta), small ancient agricultural patches of up to several hectares of soil highly enriched in PyC that are now covered by mature forests (Oliveira et al., 2020). While ADEs are found throughout Amazonia (Palace et al., 2017), estimates suggest they only represent a small fraction (∼3%) of Amazonian soils (McMichael et al., 2014). Pre-Columbian land-use and fire management appears to have declined sharply across Amazonia following the Great Dying after 1492 (Goulart et al., 2017; McMichael and Bush, 2019; Feldpausch et al., 2022) due to the introduction of Old-World diseases during post-Columbian European colonization (Dull et al., 2010).
We selected 19 one hectare forest plots that were within the forest-cerrado ZOT, with plots in intact forest at maximum and minimum distances of 52.2 and 0.2 km from cerrado. The Alta Floresta plot (ALF-02) is geographically the farthest into the Amazon forest biome and the Nova Xavantina plot (VCR-02) closest to the Cerrado biome. The plots span a 1540 km length of the ZOT representing large-scale variations in vegetation and environment.
For the soil analysis, we collected soil samples at 0–5, 5–10, 10–20, and 20–30 cm depth distributed over five random points in each plot using a Edelman soil auger. We stored samples in plastic bags and dried them in the laboratory. After drying, we analyzed the samples for textural characteristics (granulometry), including sand, silt, and clay, using the analysis protocol for assessing soil of Empresa Brasileira de Pesquisa Agropecuária (Silva et al., 1998; EMBRAPA, 2011). To quantify soil PyC abundance, we prepared soil for analysis by grinding 10 g of soil with a ball mill. Using hydrogen pyrolysis (Meredith et al., 2012), we quantified the polycyclic aromatic component of PyC as a proportion of total organic carbon (in g/dm3). Initially, ∼1 g of ground soil was catalyst loaded, subsequently pressurized to 150 bar of hydrogen gas and heated from 250 to 300°C over 1 min, then ramped at 8°C per minute to a final temperature of 550°C which was maintained for 2 min (Koele et al., 2017). This technique is assumed to remove all non-PyC from the sample and elemental analysis of the original and treated samples provide an accurate estimate of the abundances of of SOC and PyC. PyC results from this technique are highly reproducible, with determinations from triplicate analyses within ±2% across all samples (Meredith et al., 2012).
We quantified the PyC stocks (0–30 cm) using mean bulk density by FAO major soil grouping (Batjes, 1996), and converted this to tons per hectare (Mg/ha) for each forest. We determined the biomass for each plot using the pantropical allometric model of Chave et al. (2005), AGB = 0.0509 × ρD2H; where the coefficient F (0.0509) is dependent on the diameter (D): height (H) ratio, ρ is the wood density (g/cm3), compiled from the DRYAD global database (Chave et al., 2009; Zanne et al., 2009), D is the individual diameter of each tree (cm) at breast height or above deformities or buttresses and H (m) is the total tree height. We estimated the height for each tree from a pantropical allometric equation H = a(1−exp(−bDc)) (Feldpausch et al., 2012), where the coefficients a, b, and c were determined for each region. Together, these equations minimize the error in biomass estimation, as they consider geographic location, forest environment, and structure (see Feldpausch et al., 2011, 2012).
Data for temperature (maximum and minimum), average annual precipitation, coefficient of precipitation variation (seasonality), and soil water deficit in the dry season were compiled from meteorological stations between 1950 and 2000, with a spatial resolution of approximately 1 km (30 s) using WorldClim (Hijmans et al., 2005). We extracted climate data using the raster (Hijmans, 2016) and rgdal (Bivand et al., 2016) packages in R (R Core Team, 2020). We calculated potential evapotranspiration for all plots from the Tropical Rainfall Monitoring Mission (TRMM) data, version 3B42, V7, mainly derived from satellite data (NASA, 2011) with a range of 50° North to 50° South, using the same packages mentioned above and also vegan (Oksanen et al., 2016).
The Maximum Climatological Water Deficit (MCWD) (Aragão et al., 2007) was estimated for each plot as the value of the cumulative water deficit in all months of the year, starting in the rainy season and ending in the dry season, using monthly data from TRMM (NASA, 2011). This index is based on the approximate monthly transpiration of tropical forests (100 mm/month). When this value is less than 100 mm, the forest is assumed to be in water deficit, which is then accumulated monthly. We generated these data using the raster and rgdal packages (Bivand et al., 2016; Hijmans, 2016).
We measured the shortest distance from each forest plot to the Cerrado biome (savanna environment) using Google Earth Pro. To reduce uncertainties associated with anthropogenically altered areas between the biomes, we used the transition band delimited by Marques (2016) as a kmz extension and superimposed this layer on the Google Earth image as boundaries for the two biomes. We used this distance in the present study as a predictor of fires, as the closer to the Cerrado biome, which has characteristics that favor the entry of fire (Balch et al., 2008; Ribeiro and Walter, 2008), the greater the chances of exposure to fire in prehistory and in modern times, mainly due to land-use change.
To test which predictor variables best explain soil PyC variation, we applied Multiple Linear Regression (OLS) models for (1) the distance from the forests (plots) to the Cerrado Biome; (2) the climate (MCWD, evapotranspiration of the warmest months, TA, PA, coefficient of variation of precipitation and average temperature of the warmest months); (3) soil texture (silt, sand, and clay) and (4) current AGB of each plot.
To identify collinearity and remove predictor variables with low correlations to the response variable (r < 0.1), we applied Pearson’s correlation (Supplementary Table 3). We removed the collinearity by removing from our models the variables with r > 0.8 (Supplementary Tables 1, 2). With the remaining variables, we applied planned models (ICtab function) of corrected Akaike and corrected Akaike Information Criterion corrected (AICc) (Anderson and Burnham, 2002). This argument creates a table by fitting the models in ascending order, with the best model (most parsimonious), with the smallest delta (ΔAICc = 0), and the greatest weight (WEIGHT, measures the weight in favor of each model) at the top.
We also tested the multi-collinearity of the complete models selected by AICc from the Variance Inflation Factor (VIF), where the variables should present values less than 10 (Quinn and Keough, 2002). As we did not observe VIF greater than 10, we continued with the remaining variables in the models. We also evaluated the impact of the possible presence of very discrepant data in the model by the diagnosis of Bonferroni outliers. As outliers were detected, we applied the tsclean function and replaced the outliers with linear interpolated values (Box–Cox) (Hyndman, 2016).
To confirm the independence of the data (p > 0.05), we tested the Spatial Autocorrelation (SAC) of the residuals of the models, complete, selected (obtained by selecting the AICc models), the response variable, and the Geographically Weighted Regression-GWR by the Moran index (Legendre and Legendre, 1998; Diniz-Filho et al., 2003). We also tested the assumptions of multiple linear regression by evaluating the normality of the residuals (Shapiro–Wilk) and by graphically displaying the values predicted by the model vs. residues for homoscedasticity and linearity (Legendre and Legendre, 1998; Supplementary Figures 1, 2). As we did not observe significant SAC and the assumptions of the analysis were not violated, we continued using the OLS multiple linear regression model.
We previously tested for possible local effects on the response variable by comparing the OLS and GWR models (Fotheringham et al., 2002), with the Gaussian spatial function. As there was no increase in the GWR regression model on the OLS (F1.4, 6.4–effective parameter number = 0.54, R2 = 0.19, AICc = –42.6, p = 0.63 and F1.5, 5–effective parameter number = 0.43, R2 = 0.11, AICc = –52.5, p = 0.78), we chose to continue the analyses using only the OLS and assumed an absence of local effects.
To assess which sets of variables best explain the variation in the soil PyC response, we divided the variables into pure climatic, edaphic, and environmental fractions (biomass and distance), fractions shared between them, and the residue. Since the response variable is a single vector, we applied a partial regression model based on linear regression. This analysis divides the variation in the response variable into components explained in two or more data sets and the fractions combined between them (Legendre and Legendre, 1998). The significance of pure fractions A, B, and C were tested by Redundancy analysis – RDA, using 999 permutations with a significance level of 0.05.
All analyses were done using the R program (R Core Team, 2020). We performed all the procedures for the GWR analysis with the spgwr package (Bivand and Yu, 2017), OLS and partial linear regression with the vegan package (Oksanen et al., 2016), Spatial Autocorrelation-ACE (Moran Index) with the spacemakeR package (Dray, 2013), AICc model selection with the bbmle package (Bolker and Team, 2016), VIF and data discrepancy test (Bonferroni test) with the car package (Fox and Weisberg, 2011), and the correction of outliers with the forecast package (Hyndman, 2016). All of the above packages were used together with the vegan package (Oksanen et al., 2016). We standardized all data using the normalized argument (make margin sum of squares equal to one) of the decostand function (Oksanen et al., 2016) and used a significance level of 5% for all analyses. Maps and figures were produced with the packages RdoBy, lattice, plyr, reshape, boot, zoo, ggplot2, maps, GISTools, mapdata, shape, and maptools (Davison and Hinkley, 1997; Zeileis and Grothendieck, 2005; Wickham, 2007, 2009, 2011; Sarkar, 2008; Brunsdon and Chen, 2014; Soetaert, 2014; Becker et al., 2016; Bivand and Lewin-Koh, 2016; Canty and Ripley, 2016; Richard et al., 2016).
The PyC stocks in the soils of the forests spanning the ZOT ranged between 0.9 and 6.8 Mg ha−1 to 0–30 cm depth (with an average of 2.3 ± 1.5) (Figure 2), with PyC representing 4.3% of the total soil organic carbon (SOC) to 30 cm depth. The most parsimonious model, based on AICc, included soil clay and above-ground biomass as the main predictors of PyC, and these together explained 71% of the variation [F(2, 16) = 20; r2 = 0.71; p < 0.001, intercept = −2.01] (Table 2). When evaluating these variables in isolation, only clay explained a significant part of the model variance, however, with a loss in the explanatory power [F(1, 17) = 13.46; r2 = 0.44; p = 0.001, intercept = 0.30] and in the estimative value, of 0.07 to 0.06 Mg of PyC for each unit of clay in the model.
Figure 2. Spatial variation of pyrogenic carbon (PyC) to 0–30 cm depth along the Amazon-Cerrado transition. Circle colors represent the amount of PyC per hectare; the blue line represents the limit of the Legal Amazon. The plot locations on the map were shifted slightly to avoid overlap with nearby plots.
Next, we evaluated the effect of removing the ADE plots from the models. As these sites are known to have been altered by pre-Columbian peoples, the ADE plots represent outliers compared to the PyC stocks found in non-ADE soils, and non-ADE plots represent the vast majority of soils across the ZOT. After the removal of the ADE plots, soil PyC stocks ranged between 0.9 and 3.8 (mean 1.9 ± 0.8 Mg/ha PyC) to 0–30 cm depth. The PyC, on average, continued to represent ∼ 4% of the total soil carbon inventory to 30 cm depth (Supplementary material 1). The most parsimonious model included soil sand content and biomass as the main predictors of PyC and these explained 72% (r2) of the variation. When evaluating these variables in isolation, only sand explained a significant part of the model variance (Table 2), with a slight loss in the explanatory power and estimation (−0.81 to −0.004) of the model [F(1,14) = 26.14; r2 = 0.65; p < 0.001, intercept = 4.42, Table 2 and Figure 3]. That is, there is a gain in the predictive power of PyC when evaluating the sand in the presence of the variable biomass; however, the variable sand has a predictive capacity three times that of biomass to explain the amount of PyC.
Figure 3. Linear regression indicates the variation in soil pyrogenic carbon explained by the variation in soil sand content of 16 forest sites (excluding Amazonian Dark Earth) along the Amazon-Cerrado transition.
None of the components (climatic, environmental, or edaphic), pure or shared, was important in explaining soil PyC variation with or without ADE plots based on the partial regression models (Figures 4A,B and Table 3). However, when we removed the ADE plots, there was a small increase in explanatory power of the pure edaphic component (Figure 4B). The fractions not explained by the models (residuals) were relatively high (Figures 4A,B).
Figure 4. Variance partitioning between the different components to explain the variation in soil PyC in forests in the Amazon-Cerrado transition. (A) With Amazon Dark Earth forests and (B) without these forests. Pure fractions: A = Climate (precipitation + MCWD + evapotranspiration in the driest month), B = Environment. (biomass + distance) and C = Edaphic (clay + silt); Shared fractions = D, E, F, and G; Values = 0 were not shown. Pearson’s correlation table (collinearity) can be found in the Supplementary Table 3.
Studies over the past decade have begun to reveal the effects of historical fire on structurally intact forests with no history of recent fire throughout the Amazon Basin (Feldpausch et al., 2022), including higher than predicted PyC stocks (Bird et al., 2015; Koele et al., 2017). However, there remains large uncertainty about how soil PyC varies spatially (Carvalho et al., 2018), and the drivers of spatial variability, especially throughout the ZOT where fire risk is greater and fire has potentially affected vegetation and soils for millennia. Here, we show that soil PyC storage in the southern Amazon ZOT varies approximately eight fold (Figure 2), at local and regional scales of 1540 km, and these stocks are, on average, similar to humid regions of Amazonia where historical fire may have been less prevalent (Koele et al., 2017). Edaphic factors were one of the strongest predictors of soil PyC stocks, which follows expectations based on studies of biochar application and the capacity of clay to protect PyC through the formation of organo-mineral complexes (Czimczik and Masiello, 2007). Although there was substantial variation in TA (4°C) and PA (900 mm/yr) across the geographical focus on the ZOT, which are known to affect soil respiration rates (Davidson and Janssens, 2006; Lehmann et al., 2008), these variables were unimportant in predicting soil PyC. The inferred main drivers of soil PyC stocks differed when including the ADE plots rich in PyC, with biomass and soil clay (−88 correlation with sand, Supplementary material 3) content then being important drivers. This was due to biomass stocks on average being higher in ADE than the surrounding non-ADE forests in southern, but not eastern, Amazonia (Oliveira et al., 2020).
We hypothesized that soil PyC stocks would be higher in drier forests. On average, soil PyC content across the seasonally dry and fire-prone ZOT was similar to that found in more humid regions of Amazonia (Koele et al., 2017). This suggests that historical fire regimes may have not differed substantially from other regions of Amazonia. Alternatively, historical fire may have differed but the mechanisms that produced and preserved PyC may have differed, e.g., there could have been differences in the degree of recalcitrance of PyC formed during burning, which may have affected preservation potential, or differences in past climate may have affected either the incidence of fire or the preservation of PyC in the soil. For example, in biochar (purposefully carbonized biomass for agricultural use), the recalcitrance or resistance to decomposition is related to the production temperature (Zhao et al., 2013).
The fraction of PyC in soils varies globally and with vegetation type and historic land-use. A study evaluating PyC in ADE and non-ADE forests in central and eastern Amazonia found that PyC represented 35% of the SOC in ADE soils and 14% in adjacent Oxisol (Glaser et al., 2001). A literature-based inventory and on a wider scale indicates that PyC may represent up to 50% of total SOC (Reisser et al., 2016). High amounts of PyC (20%) as a fraction of total SOC were observed in Australian soils (Lehmann et al., 2008). As expected, we observed the highest values of soil PyC in ADE plots in our study, representing 5.07% (see Supplementary Table 1) of the total SOC. However, since ADE likely only covers a small fraction of the total area of Amazonia (∼3%, McMichael et al., 2014), the higher PyC content of ADE soils would not substantially increase SOC stocks across Amazonian soils, which are dominated by lower PyC soil contents. That soil PyC stocks were not substantially higher in the ZOT will be important to consider when modeling PyC and estimating the effects of climate change on SOC.
Increases in global temperature are significantly and positively correlated with changes in soil respiration (Bond-Lamberty and Thomson, 2010). There is a forecast for an increase in global temperature of up to 3°C (IPCC, 2018) and therefore also in soil carbon emissions, mainly caused by increased microbial activity, decomposition of organic matter, and root respiration (Davidson et al., 2006; Lehmann et al., 2008). There is evidence PyC is resistant to decomposition (Maestrini et al., 2015), with turnover times on the order of 1,300–2,600 years (Lehmann et al., 2008; Jones et al., 2019), and with models showing the presence of PyC in soils buffering losses of SOC under future climate warming. While PyC storage has been shown to decrease in warmer climates, no relationship was found between temperature and PyC mineralization across a climatic gradient of mean annual temperatures of 3.9–17.2, suggesting that warmer or cooler periods may have little impact on the stability of PyC currently in soils (Cheng et al., 2008). Egli et al. (2004) observed in a controlled laboratory experiment that increasing temperature reduces the amount of SOC storage. Despite this, the effects of increasing global temperature on the PyC stock in tropical forest soils remains uncertain.
In the ever wet forests of the Amazon, PyC in the soil usually results from ancient fires, both natural and those caused by people from pre-Columbian civilizations (Masiello, 2004; Lehmann et al., 2008; Goulart et al., 2017; McMichael and Bush, 2019; Feldpausch et al., 2022), as inputs from aerosols are relatively small (Coppola et al., 2019). There is evidence for ancient and frequent fires in closed canopy forest in Amazonia (Hammond et al., 2007), especially during the Middle Holocene (Mayle and Power, 2008), with historical fire return intervals of ∼450 years (Feldpausch et al., 2022). However, as there was an advance of the Amazon transitional forests over savanna formations in more recent times (Mayle et al., 2000, 2004; Marimon et al., 2006; Mayle and Power, 2008; Morandi et al., 2015), our plots that are currently forested could have previously supported savanna vegetation with lower biomass (Marimon et al., 2006). Therefore the rate of conversion of biomass to soil PyC may have been lower, resulting in only a moderate amount of PyC delivered to upper soil as observed in the present study. Furthermore, fire severity and fuel conversion efficiency likely varied greatly, as they do today. During modern fires, the conversion of forest AGB to PyC during deforestation varies between 3 and 10% (Fearnside et al., 1993; Preston and Schmidt, 2006).
Sand content (negative effect) was selected as the main predictors of PyC in non-ADE soils. It is possible that greater aeration of soils with a more sandy texture may have been responsible for the loss of PyC (Glaser et al., 2001) and resulting in lower soil PyC. More PyC may have also been illuviated downward through coarse-grained soils (Major et al., 2010). The model including the ADE soils indicated clay as one of the main predictors, which is inversely related to sand content (Supplementary material 3). We expect that the higher clay content explains part of the PyC variation in the soil due to the greater capacity of clay-rich soils to form organo-mineral compounds, together with organic matter, capable of protecting the charcoal from decomposition (Glaser et al., 2003). Another factor was the tendency of forests with higher AGB values (selected by the best model when including ADE sites) to explain part of this variation. It is predicted that increased aboveground biomass (Preston and Schmidt, 2006) leads to a larger amount of charcoal that can be formed during burning. Alternatively, greater biomass may be due to greater soil fertility in soils with high PyC, e.g., for forests growing on ADE soils in southern Amazonia, biomass is higher than the surrounding forests (Oliveira et al., 2020).
The selection of pure edaphic (texture) variables to explain the amount of soil PyC may have been due to the subtle edaphic differences along this extensive ecological zone of tension (Ab’Saber, 2002; Reatto et al., 2008; Silva et al., 2008; Quesada et al., 2011). However recent studies have shown that the extent and frequency of drought events in Amazonia have increased (Marengo et al., 2011), reducing tree growth and increasing mortality (Feldpausch et al., 2016), which may affect fire fuel loads. Models suggest that dry season stress will increase across the ZOT and that future climate may be more seasonal but still capable of supporting forests rather than becoming savanna-like (Malhi et al., 2009), which may affect biomass storage, fire regimes, and PyC stocks.
Contrary to expectations, we did not find higher soil PyC stocks in areas closer to the Cerrado biome. Evidence shows that when a fire reaches forests geographically close to savanna environments, many tree individuals die and allow light to enter, changing the microclimate (Balch et al., 2008) and favoring the entry of grasses (Silvério et al., 2013). Thus, the chance of new fires also increases, especially in warmer and drier years (Zarin et al., 2005), reinforcing a positive fire feedback and thereby potentially increasing the soil PyC stock over time. Whether more PyC is formed would also depend on fuel loads, fuel availability (moisture content), and fire behavior. A possible explanation for the absence of an effect of distance to savanna may lie in the soil texture, as, in the more sandy soils characteristic of the region closer to the savanna, PyC is more readily oxidized (Lima, 2014) or leached (Abney and Berhe, 2018) with losses from the surface through deeper translocation (Major et al., 2010). It may be that the modern transitional forest sites had Cerrado on them in the past and retain the PyC from those times (Marimon et al., 2006; Wright et al., 2020). Furthermore, biochar (PyC type) in the soil can also be transformed through the action of enzymes of microorganisms and environmental factors such as temperature (Madari et al., 2009). As a result, PyC may be chemically altered over time and thus not detected in the analytical window used by hydrogen pyrolysis.
Geographically, our study sites cover an extensive part of the Forest-Cerrado ZOT, with a focus on closed-canopy, but transitional, forests; however, there are other forest types throughout the region (e.g., cerradão and patches of cerrado within a forest matrix; Marimon-Junior and Haridasan, 2005) that may have different PyC stocks and drivers of soil PyC distribution. Because we made use of existing plots, we were unable to use predefined points to systematically assess the effect of distance from the cerrado on soil PyC. The proportion of variance (residuals) unexplained by our models was relatively high, highlighting the importance of including new areas to fully explain the likely variation that exists across the expansive ZOT region. The ZOT forests, also known as the ‘Arc of Deforestation,’ are threatened by the rapidly advancing agricultural frontier (Nogueira et al., 2007), placing the SOC stocks in these forests at risk. Continued research is needed to evaluate how SOC fractions, including PyC, will respond to climate change and to the landscape-scale changes in land-use.
This study shows that there is only a moderate amount of PyC (∼ 4% of total SOC) in the forest soils in the southern Amazonian ZOT. A much greater fraction of soil PyC in the ADE plots reinforces the importance of long-term conservation of ADE areas as a recalcitrant carbon stock. Soil PyC stocks in non-ADE forest plots are largely driven by edaphic properties. A sharp reduction in soil PyC in sandier soils and higher PyC in clayey soils highlights the physicochemical role of soil in the mineralization or the protection of soil PyC and its loss from the upper soil by illuviation to deeper soil levels. Biomass combined with edaphic variables explained part of the soil PyC variation, but only when including ADE forests. It will be important to include new forest types and soil samples at greater depths to more fully understand the variation observed in this study. Our surprising findings that soil PyC stocks are no greater in the ZOT than in the wetter regions of Amazonia, and that PyC stocks are not greater near the fire-dependent cerrado, require further investigation to understand variation in past fire history and factors that affect PyC formation and loss. This study improves understanding of the drivers of PyC across the ZOT, providing important new information to manage and conserve soils and to improve models to assess the impact of climate change on SOC.
The original contributions presented in this study are included in the article/Supplementary material, further inquiries can be directed to the corresponding author/s.
EO, TF, BM, and BM-J designed the study. EO analyzed the data. EO, AM, CAQ, LA, and PM analyzed the sampled soil. MB analyzed the soil PyC. CAQ analyzed the soil physicochemical properties. BM, PM, LA, OLP and AM contributed to the plot vegetation data. EO wrote the manuscript with TF, BM, and BM-J and with input from all co-authors. All authors contributed to the article and approved the submitted version.
This research was supported by the Coordination for the Improvement of Higher Education Personnel (CAPES) through the Science without Borders Program (Project PVE 177/2012) and UK Natural Environment Research Council (NERC, NE/N011570/1). We thank the University of Exeter, United Kingdom for sponsoring a visit by EO. To the National Council for Technological and Scientific Development (CNPq) for the research productivity scholarship to BM-J and BM and for the financial support of the PELD projects (441244/2016-5, 441572/2020-0), PPBio (457602/2012-0), and FAPEMAT (Proc. 0346321/2021). EO thanks the Coordination of Improvement of Personnel in Higher Education, Brazil (CAPES) for a postdoctoral Fellowship (Finance Code 001, Fellowship CAPES/Brazil 88887.504562/2020-00), and the CNPq, Brazil, for a postdoctoral scholarship (151226/2021-0). This project has been supported by ForestPlots.net, approved Project “Pyrogenic carbon in soils in southern Amazonia: interaction between soils and climate”.
We thank the Laboratório de Ecologia Vegetal (LABEV) team for their logistical and intellectual support in the field and the data processing. We thank L.C.S. Carvalho for help with PyC extrapolation calculations.
The authors declare that the research was conducted in the absence of any commercial or financial relationships that could be construed as a potential conflict of interest.
All claims expressed in this article are solely those of the authors and do not necessarily represent those of their affiliated organizations, or those of the publisher, the editors and the reviewers. Any product that may be evaluated in this article, or claim that may be made by its manufacturer, is not guaranteed or endorsed by the publisher.
The Supplementary Material for this article can be found online at: https://www.frontiersin.org/articles/10.3389/ffgc.2022.880963/full#supplementary-material
Supplementary Figure 1 | Test of the assumptions of the multiple regression model for the variables with Amazon Dark Earth forests sampled along with the Amazon-Cerrado transition.
Supplementary Figure 2 | Test of the assumptions of the multiple regression model for the variables without Amazon Dark Earth forests sampled along with the Amazon-Cerrado transition.
Ab’Saber, A. N. (2002). Bases para o estudo dos ecossistemas da Amazônia brasileira. Estud. Avançados 16, 5–30. doi: 10.1021/la800835k
Abney, R. B., and Berhe, A. A. (2018). Pyrogenic Carbon Erosion: Implications for Stock and Persistence of Pyrogenic Carbon in Soil. Front. Earth Sci. 6:26. doi: 10.3389/feart.2018.00026
Anderson, D. R. B., and Burnham, K. P. (2002). Model Selection and Multimodel Inference. 2nd. ed. New York, NY: Springer. doi: 10.1016/j.fishres.2014.k07.015
Aragão, L. E. O. C., Anderson, L. O., Fonseca, M. G., Rosan, T. M., Vedovato, L. B., Wagner, F. H., et al. (2018). 21st Century drought-related fires counteract the decline of Amazon deforestation carbon emissions. Nat. Commun. 9:536. doi: 10.1038/s41467-017-02771-y
Aragão, L. E. O. C., Malhi, Y., Roman-Cuesta, R. M., Saatchi, S., Anderson, L. O., and Shimabukuro, Y. E. (2007). Spatial patterns and fire response of recent Amazonian droughts. Geophys. Res. Lett. 34, 1–5. doi: 10.1029/2006GL028946
Balch, J. K., Nepstad, D. C., Brando, P. M., Curran, L. M., Portela, O., Carvalho, O. D., et al. (2008). Negative fire feedback in a transitional forest of southeastern Amazonia. Glob. Chang. Biol. 14, 2276–2287. doi: 10.1111/j.1365-2486.2008.01655.x
Barbier, N., Couteron, P., Proisy, C., Malhi, Y., and Gastellu-Etchegorry, J. P. (2010). The variation of apparent crown size and canopy heterogeneity across lowland Amazonian forests. Glob. Ecol. Biogeogr. 19, 72–84. doi: 10.1111/j.1466-8238.2009.00493.x
Batjes, N. H. (1996). Total carbon and nitrogen in the soils of the world. European J. Soil Sci. 47, 151–163.
Becker, R. A., Wilks, A. R., and Brownrigg, R. (2016). mapdata: Extra Map Databases. R package. Version 2.3.0.
Bird, M. I., Wynn, J. G., Saiz, G., Wurster, C. M., and McBeath, A. (2015). The Pyrogenic Carbon Cycle. Annu. Rev. Earth Planet Sci. 43, 273–298. doi: 10.1146/annurev-earth-060614-105038
Bivand, R., and Lewin-Koh, N. (2016). maptools: Tools for Reading and Handling Spatial Objects. R Package. Version 1.1-4.
Bivand, R., and Yu, D. (2017). Spgwr: Geographically Weighted Regression. R Package. Version 0.6-35.
Bivand, R., Keitt, T., and Rowlingson, B. (2016). rgdal: Bindings for the Geospatial Data Abstraction Library. R package. Version 1.5-32.
Bolker, B., and R Development Core Team (2016). bbmle: Tools for General Maximum Likelihood Estimation. R package. Version 1.0.25.*
Bond-Lamberty, B., and Thomson, A. (2010). Temperature-associated increases in the global soil respiration record. Nature 464, 579–582. doi: 10.1038/nature08930
Brando, P. M., Soares-Filho, B., Rodrigues, L., Assunção, A., Morton, D., Tuchschneider, D., et al. (2020). The gathering firestorm in southern Amazonia. Sci. Adv. 6:eaay1632. doi: 10.1126/sciadv.aay1632
Brienen, R. J., Phillips, O. L., Feldpausch, T. R., Gloor, E., Baker, T. R., Lloyd, J., et al. (2015). Long-term decline of the Amazon carbon sink. Nature 519, 344–348. doi: 10.1038/nature14283
Brunsdon, C., and Chen, H. (2014). GISTools: Some further GIS capabilities for R. R package. Version 0.7-4
Burbridge, R. E., Mayle, F. E., and Killeen, T. J. (2004). Fifty-thousand-year vegetation and climate history of Noel Kempff Mercado National Park. Bolivian Amazon. Q. Res. 61, 215–230. doi: 10.1016/j.yqres.2003.12.004
Canty, A., and Ripley, B. (2016). boot: Bootstrap R (S-Plus) Functions. R package. Version:1.3-28. doi: 10.1002/9781118445112.stat06177.pub2
Carvalho, L. C. S., Fearnside, F. M., Nascimento, M. T., and Barbosa, R. I. (2018). Amazon soil charcoal: Pyrogenic carbon stock depends of ignition source distance and forest type in Roraima Brazil. Glob. Change Biol. 9, 4122–4130. doi: 10.1111/gcb.14277
Chave, J., Andalo, C., Brown, S., Cairns, M. A., Chambers, J. Q., Eamus, D., et al. (2005). Tree allometry and improved estimation of carbon stocks and balance in tropical forests. Oecologia 145, 87–99. doi: 10.1007/s00442-005-0100-x
Chave, J., Coomes, D., Jansen, S., Lewis, S. L., Swenson, N. G., and Zanne, A. E. (2009). Towards a worldwide wood economics spectrum. Ecol. Lett. 12, 351–366. doi: 10.1111/j.1461-0248.2009.01285.x
Cheng, C. H., Lehmann, J., Thies, J. E., and Burton, S. D. (2008). Stability of black carbon in soils across a climatic gradient. J. Geophys. Res. 113:G02027. doi: 10.1029/2007JG000642
Coppola, A. I., Seidel, M., Ward, N. D., Viviroli, D., Nascimento, G. S., Haghipour, N., et al. (2019). Marked isotopic variability within and between the Amazon River and marine dissolved black carbon pools. Nat. Commun. 10:4018. doi: 10.1038/s41467-019-11543-9
Czimczik, C. I., and Masiello, C. A. (2007). Controls on black carbon storage in soils. Glob. Biogeochem. Cycles. 21:GB3005 doi: 10.1029/2006GB002798
Davidson, E. A., Janssens, I. A., Marks, D., Murdock, M., Ahl, R. S., Woods, S. W., et al. (2006). Temperature sensitivity of soil carbon decomposition and feedbacks to climate change. Nature 440, 165–173. doi: 10.1038/nature04514
Davidson, E. A., and Janssens, I. A. (2006). Temperature sensitivity of soil carbon decomposition and feedbacks to climate change. Nature 440, 165–173. doi: 10.1038/nature04514
Davison, A. C., and Hinkley, D. V. (1997). Bootstrap Methods and Their Applications. Cambridge: Cambridge University Press. doi: 10.1017/CBO9780511802843
Diniz-Filho, J. A. F., Bini, L. M., and Hawkins, B. A. (2003). Spatial autocorrelation and red herrings in geographical ecology. Glob. Ecol. Biogeogr. 12, 53–64. doi: 10.1046/j.1466-822X.2003.00322.x
Dull, R. A., Nevle, R. J., Woods, W. I., Bird, D. K., Avnery, S., and Denevan, W. M. (2010). The Columbian encounter and the little ece age: abrupt land use change, fire, and greenhouse forcing. Ann. Assoc. Amer. Geogr. 100, 755–771. doi: 10.1080/00045608.2010.502432
Egli, M., Hitz, C., Fitze, P., and Mirabella, A. (2004). Experimental determination of climate-change effects on above-ground and below-ground organic matter in alpine grasslands by translocation of soil cores. J. Plant Nutr. Soil Sci. 167, 457–470. doi: 10.1002/jpln.200321333
EMBRAPA (2006). Sistema Brasileiro de Classificação de Solos, 2nd Edn. Rio de Janeiro: Centro Nacional de Pesquisa de Solos, 286.
Fauset, S., Johnson, M. O., Gloor, M., Baker, T. R., Monteagudo, M. A., Brienen, R. J. W., et al. (2015). Hyperdominance in Amazonian forest carbon cycling. Nat. Commun. 6:6857. doi: 10.1038/ncomms7857
Fearnside, P. M. Filho, N. L., and Fernandes, F. M. (1993). Rainforest burning and the global carbon budget: Biomass, combustion efficiency, and charcoal formation in the Brazilian Amazon. J. Geophys. Res. 98, 16733–16743. doi: 10.1029/93JD01140
Fearnside, P. M., Graça, P. M. L. A., and Rodrigues, F. J. A. (2001). Burning of Amazonian rainforests: burning efficiency and charcoal formation in forest cleared for cattle pasture near Manaus, Brazil. Forest Ecol. Manag. 146, 115–128. doi: 10.1016/S0378-1127(00)00450-3
Feldpausch, T. R. T., Lloyd, J., Lewis, S. L., Brienen, R. J. W., Gloor, M., Monteagudo, A., et al. (2012). Tree height integrated into pantropical forest biomass estimates. Biogeosciences 9, 3381–3403. doi: 10.5194/bg-9-3381-2012
Feldpausch, T. R., Banin, L., Phillips, O. L., Baker, T. R., Lewis, S. L., Quesada, C. A., et al. (2011). Height-diameter allometry of tropical forest trees. Biogeosciences 8, 1081–1106. doi: 10.5194/bg-8-1081-2011
Feldpausch, T. R., Carvalho, L., Macario, K., Ascough, P. L., Flores, C. F., Honorio Coronado, E. N., et al. (2022). Forest fire history in Amazonia inferred from intensive soil charcoal sampling and radiocarbon dating. Front. For. Glob. Change. 5:815438. doi: 10.3389/ffgc.2022.815438
Feldpausch, T. R., Phillips, O. L., Brienen, R. J. W., Gloor, E., Lloyd, J., Lopez-Gonzalez, G., et al. (2016). Amazon forest response to repeated droughts. Glob. Biogeochem. Cycles 30, 964–982. doi: 10.1002/2015GB005133
ForestPlots.net, Blundo, C., Carilla, J., Grau, R., Malizia, A., Malizia, L., et al. (2021). Taking the pulse of Earth’s tropical forests using networks of highly distributed plots. Biol. Conserv. 260:108849. doi: 10.1016/j.biocon.2020.108849
Fotheringham, A. S., Brunsdon, C., and Charlton, M. (2002). Geographically Weighted Regression: The Analysis of Spatially Varying Relationships. Hoboken, NJ: JohnWiley&Sons.
Fox, J., and Weisberg, S. (2011). An {R} Companion to Applied Regression. 2nd Edition. Thousand Oaks, CA: Sage
Glaser, B., Guggenberger, G., and Zech, W. (2001). “Black carbon in sustainable soils of the Brazilian Amazon region,” in Understanding and Managing Organic Matter in Soils, Sediments and Waters, (St. Paul, MINN: International Humic Substances Society), 359–364. doi: 10.1007/s00248-010-9689-3
Glaser, B., Guggenberger, G., Zech, W., and Ruivo, M. L. (2003). “Soil organic matter stability in Amazonian Dark Earths,” in Amazonian Dark Earths: Origin, Properties, and Management, eds J. Lehmann, D. C. Kern, B. Glaser, and W. I. Wodos (London, ENG: Kluwer Academic Publishers), 141–158. doi: 10.1007/1-4020-2597-1
Goulart, A. C., Macario, K. D., Scheel-Ybert, R., Alves, E. Q., Bachelet, C., Pereira, B. B., et al. (2017). Charcoal chronology of the Amazon forest: A record of biodiversity preserved by ancient fires. Q. Geochronol. 41, 180–186. doi: 10.1016/j.quageo.2017.04.005
Gustafsson, O., and Gschwend, P. M. (1998). Sequestration of black carbon in continental shelf sediments. Mineral. Mag. 62A, 555–556. doi: 10.1180/minmag.1998.62A.1.293
Hammond, D. S., Ter Steege, H., and Van Der Borg, K. (2007). Upland soil charcoal in the wet tropical forests of central Guyana. Biotropica 39, 153–160. doi: 10.1111/j.1744-7429.2006.00257.x
Hijmans, R. J., Cameron, S. E., Parra, J. L., Jones, P. G., and Jarvis, A. (2005). Very high resolution interpolated climate surfaces for global land areas. Int. J. Climatol. 25, 1965–1978. doi: 10.1002/joc.1276
Hyndman, R. J. (2016). forecast: Forecasting Functions for Time Series and Linear Models. R package. Version 8.16.
IBGE (2015). Manual Técnico de Pedologia, Coordenação de Recursos Naturais e Estudos Ambientais, 3rd Edn. Rio de Janeiro: IBGE, 430.
IPCC (2018). “Global Warming of 1.5C. An IPCC Special Report on the impacts of global warming of 1.5C above pre-industrial levels and related global greenhouse gas emission pathways”, in the Context of Strengthening the Global Response to the Threat of Climate Change, Sustainable Development, And Efforts To Eradicate Poverty, eds V. Masson-Delmotte, P. Zhai, H.-O. Pörtner, D. Roberts, J. Skea, P. R. Shukla, et al. (Geneva: Intergovernmental Panel on Climate Change).
Ivanauskas, N. M., Monteiro, R., and Rodrigues, R. R. (2008). Classificação fitogeográfica das florestas do Alto Rio Xingu. Acta Amaz. 38, 387–402. doi: 10.1590/S0044-59672008000300003
Jones, M. W., Santín, C., van der Werf, G. R., and Doerr, S. H. (2019). Global fire emissions buffered by the production of pyrogenic carbono. Nat. Geosci. 12, 742–747. doi: 10.1038/s41561-019-0403-x
Koele, N., Bird, M. I., Haig, J., Marimon-Junior, B. H., Marimon, B. S., Phillips, O. L., et al. (2017). First estimate of soil pyrogenic carbon stocks to 2 m in the Amazon Basin. Geoderma 306, 237–243. doi: 10.1016/j.geoderma.2017.07.029
Kuhlbusch, T. A. J., and Crutzen, P. J. (1995). Toward a global estimate of black carbon in residues of vegetation fires representing a sink of atmospheric CO 2 and a source of O 2. Glob. Biogeochem. Cycles 9, 491–501. doi: 10.1029/95GB02742
Kury, M. S., Moreira, L. S., Cordeiro, R. C., Sifeddine, A., Turcq, B., Stríkis, N. M., et al. (2021). Late-Holocene palaeoenvironmental reconstruction from a lake in the Amazon Rainforest-Tropical Savanna (Cerrado) boundary in Brazil using a multi-proxy approach. Holocene 31, 1417–1429. doi: 10.1177/09596836211019091
Lehmann, J., Skjemstad, J., Sohi, S., Carter, J., Barson, M., Falloon, P., et al. (2008). Australian climate-carbon cycle feedback reduced by soil black carbon. Nat. Geosci. 1, 832–835. doi: 10.1038/Ngeo358
Lima, L. B. (2014). Desempenho Agronômico Da Soja, Fertilidade e Dinâmica da Matéria Orgânica em Solo Sob Aplicação do Biochar no Cerrado Brasileiro. Goiânia: Universidade Federal de Goiás.
Lopez-Gonzalez, G., Lewis, S. L., Burkitt, M., and Phillips, O. L. (2011). ForestPlots.net: A web application and research tool to manage and analyze tropical forest plot data. J. Veg. Sci. 22, 610–613. doi: 10.1111/j.1654-1103.2011.01312.x
Lopez-Gonzalez, G., Lewis, S. L., Burkitt, M., Baker, P. J., and Phillips, O. L. (2009). ForestPlots.net Database [WWW Document]. Available online at: www.forestplots.net (Accessed on Oct 9 15).
Madari, B. E., Cunha, T. J. F., Novotny, E. H., Milori, D. M. B. P., Martin Neto, L., Benites, V. D. M., et al. (2009). “Matéria Orgânica dos Solos Antrópicos da Amazônia (Terra Preta de Índio): Suas Características e Papel na Sustentabilidade da Fertilidade do Solo,” in As Terras Pretas de Índio Da Amazônia: Sua Caracterização E Uso Deste Conhecimento Na Criação de Novas Áreas, ed W. G. Teixeira, D. C. Kern, B. E. Madari, H. N. Lima, W. Woods (Manaus: Embrapa Amazônia Ocidental), 172–188.
Maestrini, B., Nannipieri, P., and Abiven, S. (2015). A meta-analysis on pyrogenic organic matter induced priming effect. Glob. Change Biol. Bioenergy 7, 577–590. doi: 10.1111/gcbb.12194
Major, J., Lehmann, J., Rondon, M., and Goodale, C. (2010). Fate of soil-applied black carbon: downward migration, leaching and soil respiration. Glob. Change Biol. 4, 1366–1379. doi: 10.1111/j.1365-2486.2009.02044.x
Malhi, Y., Aragão, L. E. O. C., Galbraith, D., Huntingfordc, C., Fisherd, R., Zelazowskia, P., et al. (2009). Exploring the likelihood and mechanism of a climate-change-induced dieback of the Amazon rainforest. PNAS USA 106, 20610–20615. doi: 10.1073/pnas.0804619106
Marengo, J. A., Tomasella, J., Alves, L. M., Soares, W. R., and Rodrigues, D. A. (2011). The drought 800 of 2010 in the context of historical droughts in the Amazon region. Geophys. Res. Lett. 38:12703. doi: 10.1029/2011gl047436
Marimon, B. S. S., Lima, E. S., Duarte, T. G. G., Chieregatto, L. C. C., Ratter, J. A., Lima, E., et al. (2006). Observations on the Vegetation of Northeastern Mato Grosso. Brazil. Iv. an Analysis of the Cerrado–Amazonian Forest Ecotone. Edinburgh J. Bot. 63:323. doi: 10.1017/S0960428606000576
Marimon-Junior, B. H., and Haridasan, M. (2005). Comparação da vegetação arbórea e características edáficas de um cerradão e um cerrado sensu stricto em áreas adjacentes sobre solo distrófico no leste de Mato Grosso. Brasil. Acta Bot. Brasilica 19, 913–926. doi: 10.1590/S0102-33062005000400026
Marques, E. Q. (2016). Redefinindo os limites Amazônia-Cerrado no Brasil: quanto e o que estamos perdendo?. Cavalhada : Universidade do Estado de Mato Grosso.
Masiello, C. A. (2004). New directions in black carbon organic geochemistry. Mar. Chem. 92, 201–213. doi: 10.1016/j.marchem.2004.06.043
Mayle, F. E., and Power, M. J. (2008). Impact of a drier Early-Mid-Holocene climate upon Amazonian forests. Philos. Trans. R. Soc. B 363, 1829–1838. doi: 10.1098/rstb.2007.0019
Mayle, F. E., Beerling, D. J., Gosling, W. D., and Bush, M. B. (2004). Responses of Amazonian ecosystems to climatic and atmospheric carbon dioxide changes since the last glacial maximum. Philos. Trans. R. Soc. Lond. B. 359, 499–514. doi: 10.1098/rstb.2003.1434
Mayle, F. E., Burbridge, R., and Killeen, T. J. (2000). Millennial-scale dynamics of southern Amazonian rain forests. Science 290, 2291–2294. doi: 10.1126/science.290.5500.2291
Mayle, F. E., Langstroth, R. P., Fisher, R. A., and Meir, P. (2007). Long-term forest–savannah dynamics in the Bolivian Amazon: implications for conservation. Philos. Trans. R. Soc. B 362, 291–307. doi: 10.1098/rstb.2006.1987
McMichael, C. H., Palace, M. W., Bush, M. B., Braswell, B., Hagen, S., Neves, E. G., et al. (2014). Predicting pre-Columbian anthropogenic soils in Amazonia. Proc. R. Soc. B 281:20132475. doi: 10.1098/rspb.2013.2475
McMichael, C. N., and Bush, M. B. (2019). Spatiotemporal patterns of pre-Columbian people in Amazonia. Q. Res. 92, 53–69. doi: 10.1017/qua.2018.152
Meredith, W., Ascough, P. L., Bird, M. I., Large, D. J., Snape, C. E., Sun, Y., et al. (2012). Assessment of hydropyrolysis as a method for the quantification of black carbon using standard reference materials. Geochim. Cosmochim. Acta 97, 131–147. doi: 10.1016/j.gca.2012.08.037
Metcalfe, D. B., Meir, P., Aragao, L. E. O. C., Malhi, Y., da Costa, A. C. L., Braga, A., et al. (2007). Factors controlling spatio-temporal variation in carbon dioxide efflux from surface litter, roots, and soil organic matter at four rain forest sites in the eastern Amazon. J. Geophys. Res. 112, 1–9. doi: 10.1029/2007JG000443
Morandi, P. S., Marimon, B. S., Eisenlohr, P. V., Marimon-Junior, B. H., Oliveira-Santos, C., Feldpausch, T. R., et al. (2016). Patterns of tree species composition at watershed-scale in the Amazon ‘arc of deforestation’: implications for conservation. Environ. Conserv. 43, 317–326. doi: 10.1017/S0376892916000278
Morandi, P. S., Marimon-Junior, B. H., de Oliveira, E. A., Reis, S. M., Valadão, M. B. X., Forsthofer, M., et al. (2015). Vegetation succession in the Cerrado-Amazonian Forest transition zone of Mato Grosso state, Brazil. Edinburgh J. Bot. 1, 1–11. doi: 10.1017/S096042861500027X
NASA (2011). Trmm (Tmpa/3b43) Rainfall Estimate L3 1 Month 0.25-Degree X 0.25 Degree V7, Version. Greenbelt, MD: Goddard Earth Sciences Data and Information Services Center (GES DISC)
Nogueira, D. S., Marimon, B. S., Marimon-Junior, B. H., Oliveira, E. A., Morandi, P. S., Reis, S. M., et al. (2019). Impacts of fire on forest biomass dynamics at the southern amazon edge. Environ. Conserv. 4, 285–292. doi: 10.1017/S0376892919000110
Nogueira, E. M., Fearnside, P. M., Nelson, B. W., and Franca, M. B. (2007). Wood density in forests of Brazil’s “arc of deforestation”: Implications for biomass and flux of carbon from land-use change in Amazonia. For. Ecol. Manag. 248, 119–135. doi: 10.1016/j.foreco.2007.04.047
Oksanen, J., Blanchet, F. G., Friendly, M., Kindt, R., Legendre, P., McGlinn, D., et al. (2016). vegan: Community Ecology Package. R package. Version 2.6-2.
Oliveira, E. A., Marimon-Junior, B. H., Marimon, B. S., Iriarte, J., Morandi, P. S., Maezumi, S. Y., et al. (2020). Legacy of Amazonian Dark Earth soils on forest structure and species composition. Glob. Ecol. Biogeogr. 29, 1458–1473. doi: 10.1111/geb.13116
Ometto, J. P., Aguiar, A. P., Assis, T., Soler, L., Valle, P., Tejada, G., et al. (2014). Amazon forest biomass density maps: Tackling the uncertainty in carbon emission estimates. Clim. Change 124, 545–560. doi: 10.1007/s10584-014-1058-7
Palace, M. W., McMichael, C. N. H., Braswell, B. H., Hagen, S. C., Bush, M. B., Neves, E., et al. (2017). Ancient Amazonian populations left lasting impacts on forest structure. Ecosphere 8:e02035. doi: 10.1002/ecs2.2035
Phillips, O. L., Aragão, L. E. O. C., Lewis, S. L., Fisher, J. B., Lloyd, J., López-Gonzàlez, et al. (2009). Drought sensitivity of the Amazon rainforest. Science 323, 1344–1347. doi: 10.1126/science.1164033
Phillips, O. L., Baker, T. R., Brienen, R., and Feldpausch, T. R. (2010). Field Manual for Plot Establishment and Remeasurement. Available online at: https://www.forestplots.net (Accessed on Dec 10 2016)
Pontes-Lopes, A., Silva, C. V. J., Barlow, J., Rincón, L. M., Campanharo, W. A., Nunes, C. A., et al. (2021). Drought-driven wildfire impacts on structure and dynamics in a wet Central Amazonian forest. Proc. R. Soc. B 288:20210094. doi: 10.1098/rspb.2021.0094
Prestes, N. C. C. S., Massi, K. G., Silva, E. A., Nogueira, D. S., Oliveira, E. A., and Freitag, R. (2020). Fire Effects on Understory Forest Regeneration in Southern Amazonia. Front. For. Glob. Change 2020, 31–15. doi: 10.3389/ffgc.2020.00010
Preston, C. M., and Schmidt, M. W. I. (2006). Black (pyrogenic) carbon: a synthesis of current knowledge and uncertainties with special consideration of boreal regions. Biogeosciences 3, 397–420. doi: 10.5194/bg-3-397-2006
Quesada, C. A., Lloyd, J., Anderson, L. O., Fyllas, N. M., Schwarz, M., and Czimczik, C. I. (2011). Soils of Amazonia with particular reference to the RAINFOR sites. Biogeosciences 8, 1415–1440. doi: 10.5194/bg-8-1415-2011
Quinn, G. P., and Keough, M. J. (2002). Experimental Design and Data Analysis for Biologists. Edinburgh: Cambridge University Press. doi: 10.1017/CBO9780511806384
R Core Team (2020). R: A Language and Environment for Statistical Computing. Vienna, Aus: R Foundation for Statistical Computing.
Ray, D., Nepstad, D., and Moutinho, P. (2005). Micrometeorological and canopy controls of fire susceptibility in a forested Amazon landscape. Ecol. Appl. 15, 1664–1678. doi: 10.1890/05-0404
Reatto, A., Correia, J. R., Spera, S. T., and Martins, E. S. (2008). “Solos do Bioma Cerrado,” in Cerrado: Ecologia E Flora, eds S. M. Sano, S. P. Almeida, and J. F. Ribeiro (Brasília: Embrapa Cerrados), 406.
Reisser, M., Purves, R. S., Schmidt, M. W. I., and Abiven, S. (2016). Pyrogenic carbon in soils: a literature-based inventory and a global estimation of its content in soil organic carbon and stocks. Front. Earth Sci. 4:80. doi: 10.3389/feart.2016.00080
Ribeiro, J. F., and Walter, B. M. T. (2008). “As Principais Fitofisionomias do bioma Cerrado,” in Cerrado: Ecologia E Flora, eds S. M. Sano, S. P. Almeida, and J. F. Ribeiro (Brasília-DF: Embrapa Cerrados: Embrapa Informação Tecnologia), 406.153–212.
Richard, A. B., Wilks, A. R., Minka, T. P., and Deckmyn, A. (2016). maps: Draw Geographical Maps. R package Version 3.4.0.
Rumpel, C., Chaplot, V., Planchon, O., Bernadou, J., Valentin, C., and Mariotti, A. (2006). Preferential erosion of black carbon on steep slopes with slash and burn agriculture. Catena 65, 30–40. doi: 10.1016/j.catena.2005.09.005
Sanford, R. L. Jr., Saldarriaga, J., Clark, K. E., Uhl, C., and Herrera, R. (1985). Amazon Rain-Forest Fires. Science 227:1985. doi: 10.1126/science.227.4682.53
RADAMBRASIL (1982). Levantamento dos Recursos Naturais–Folhas SF 23/24, Vol. 32. Rio de Janeiro: Ministério de Minas e Energia, 780.
Sarkar, D. (2008). Lattice: Multivariate Data Visualization with R. New York, NY: Springer. doi: 10.1007/978-0-387-75969-2
Silva, F. A. M., Assad, E. D., and Evangelista, B. A. (2008). “Caracterização Climática do Bioma Cerrado,” in Cerrado: Ecologia E Flora, eds S. M. Sano, S. P. Almeida, and J. F. Ribeiro (Brasília-DF: Embrapa Cerrados), 69–88.
Silva, F. C., Eira, P. A., Barreto, W. O., Pérez, D. V., and Silva, C. A. (1998). “Análises químicas para avaliação da fertilidade do solo: Métodos usados na embrapa solos,” in Manual de análises químicas de solos, plantas e fertilizantes, (Rio de Janeiro: EMBRAPA), 1–40.
Silvério, D. V., Brando, P. M., Balch, J. K., Putz, F. E., Nepstad, D. C., Oliveira-Santos, C., et al. (2013). Testing the Amazon savannization hypothesis: fire effects on invasion of a neotropical forest by native cerrado and exotic pasture grasses. Philos. Trans. R. Soc. B 368:8. doi: 10.1098/rstb.2012.0427
Singh, N., Abiven, S., Maestrini, B., Bird, J. A., Torn, M. S., and Schmidt, M. W. I. (2014). Transformation and stabilization of pyrogenic organic matter in a temperate forest field experiment. Glob. Change Biol. 20, 1629–1642. doi: 10.1111/gcb.12459
Soetaert, K. (2014). shape: Functions for plotting graphical shapes, colors. R package. Version: 1.4.6.
Sombroek, W. G. (1966). Amazon Soil: a Reconnaissance of the Soils of the Brazilian Amazon Region. Wageningen: Centre for Agricultural Publications and Documentation (PUDOC).
Suman, D. O., Kuhlbusch, T. A. J., and Lim, B. (1997). “Marine sediments: a reservoir for black carbon and their use as spatial and temporal records of combustion,” in NATO ASI Series I: Global Environmental Change, eds J. S. Clark, H. Cachier, J. G. Goldammer, and B. Stocks (Berlin: Springer), 271–293. doi: 10.1007/978-3-642-59171-6_12
Wei, X., Hayes, D. J., Fraver, S., and Chen, G. (2018). Global Pyrogenic Carbon Production During Recent Decades Has Created the Potential for a Large, Long-Term Sink of Atmospheric CO 2. J. Geophys. Res. 123, 3682–3696. doi: 10.1029/2018JG004490
Wickham, H. (2007). Reshaping data with the reshape package. J. Stat. Softw. 21, 1–20. doi: 10.18637/jss.v021.i12
Wickham, H. (2009). ggplot2: Elegant Graphics for Data Analysis. New York, NY: Springer-Verlag. doi: 10.1007/978-0-387-98141-3
Wickham, H. (2011). The Split-Apply-Combine Strategy for Data Analysis. J. Stat. Softw. 40, 1–29. doi: 10.18637/jss.v040.i01
Wright, J. L., Bomfim, B., Wong, C. I., Marimon-Junior, B. H., Marimon, B. S., and Silva, L. C. R. (2020). Sixteen hundred years of increasing tree cover prior to modern deforestation in Southern Amazon and Central Brazilian savannas. Glob. Change Biol. doi: 10.1111/gcb.15382
Zanne, A. E., Lopez-Gonzalez, G., Coomes, D. A., Ilic, J., Jansen, S., Lewis, S. L., et al. (2009). Global Wood Density Database. Dryad. IDENTIFIER. Available Online at: http://hdl.handle.net/ 10255/dryad.235. (Accessed on Feb 21 2022)
Zarin, D. J., Davidson, E. A., Brondizio, E., Vieira, I. C. G., Sá, T., Feldpausch, T. R., et al. (2005). Legacy of fire slows carbon accumulation in Amazonian forest regrowth. Front. Ecol. Environ. 3:365–369. doi: 10.1890/1540-92952005003[0365:LOFSCA]2.0.CO;2
Zeileis, A., and Grothendieck, G. (2005). Zoo : S3 Infrastructure for Regular and Irregular Time Series. J. Stat. Softw. 14, 1–27. doi: 10.18637/jss.v014.i06
Keywords: fire, biomass, drought, Holocene, Zone of Transition, Cerrado, soil organic carbon, fire fuel
Citation: de Oliveira EA, Feldpausch TR, Marimon BS, Morandi PS, Phillips OL, Bird M, Murakami AA, Arroyo L, Quesada CA and Marimon-Junior BH (2022) Soil pyrogenic carbon in southern Amazonia: Interaction between soil, climate, and above-ground biomass. Front. For. Glob. Change 5:880963. doi: 10.3389/ffgc.2022.880963
Received: 22 February 2022; Accepted: 28 June 2022;
Published: 25 October 2022.
Edited by:
Andrew R. Zimmerman, University of Florida, United StatesReviewed by:
Jessica R. Miesel, Michigan State University, United StatesCopyright © 2022 de Oliveira, Feldpausch, Marimon, Morandi, Phillips, Bird, Murakami, Arroyo, Quesada and Marimon-Junior. This is an open-access article distributed under the terms of the Creative Commons Attribution License (CC BY). The use, distribution or reproduction in other forums is permitted, provided the original author(s) and the copyright owner(s) are credited and that the original publication in this journal is cited, in accordance with accepted academic practice. No use, distribution or reproduction is permitted which does not comply with these terms.
*Correspondence: Edmar Almeida de Oliveira, ZWRtYXJkaW5laUBob3RtYWlsLmNvbQ==; Ted R. Feldpausch, VC5SLkZlbGRwYXVzY2hAZXhldGVyLmFjLnVr; Ben Hur Marimon-Junior, YmhtanVuaW9yQGdtYWlsLmNvbQ==
Disclaimer: All claims expressed in this article are solely those of the authors and do not necessarily represent those of their affiliated organizations, or those of the publisher, the editors and the reviewers. Any product that may be evaluated in this article or claim that may be made by its manufacturer is not guaranteed or endorsed by the publisher.
Research integrity at Frontiers
Learn more about the work of our research integrity team to safeguard the quality of each article we publish.