- 1Department of Biology, Sonoma State University, Rohnert Park, CA, United States
- 2Environmental Change Institute, School of Geography and the Environment, University of Oxford, Oxford, United Kingdom
- 3Department of Geography, Environment, and Planning, Sonoma State University, Rohnert Park, CA, United States
- 4Center for Interdisciplinary Geospatial Research, Sonoma State University, Rohnert Park, CA, United States
- 5Pepperwood Preserve, Santa Rosa, CA, United States
- 6Geography and Environment, San Francisco State University, San Francisco, CA, United States
- 7Department of Geography, University College London, London, United Kingdom
- 8NERC National Centre for Earth Observation, Leicester, United Kingdom
While fire is an important ecological process, wildfire size and severity have increased as a result of climate change, historical fire suppression, and lack of adequate fuels management. Ladder fuels, which bridge the gap between the surface and canopy leading to more severe canopy fires, can inform management to reduce wildfire risk. Here, we compared remote sensing and field-based approaches to estimate ladder fuel density. We also determined if densities from different approaches could predict wildfire burn severity (Landsat-based Relativized delta Normalized Burn Ratio; RdNBR). Ladder fuel densities at 1-m strata and 4-m bins (1–4 m and 1–8 m) were collected remotely using a terrestrial laser scanner (TLS), a handheld-mobile laser scanner (HMLS), an unoccupied aerial system (UAS) with a multispectral camera and Structure from Motion (SfM) processing (UAS-SfM), and an airborne laser scanner (ALS) in 35 plots in oak woodlands in Sonoma County, California, United States prior to natural wildfires. Ladder fuels were also measured in the same plots using a photo banner. Linear relationships among ladder fuel densities estimated at broad strata (1–4 m, 1–8 m) were evaluated using Pearson’s correlation (r). From 1 to 4 m, most densities were significantly correlated across approaches. From 1 to 8 m, TLS densities were significantly correlated with HMLS, UAS-SfM and ALS densities and UAS-SfM and HMLS densities were moderately correlated with ALS densities. Including field-measured plot-level canopy base height (CBH) improved most correlations at medium and high CBH, especially those including UAS-SfM data. The most significant generalized linear model to predict RdNBR included interactions between CBH and ladder fuel densities at specific 1-m stratum collected using TLS, ALS, and HMLS approaches (R2 = 0.67, 0.66, and 0.44, respectively). Results imply that remote sensing approaches for ladder fuel density can be used interchangeably in oak woodlands, except UAS-SfM combined with the photo banner. Additionally, TLS, HMLS and ALS approaches can be used with CBH from 1 to 8 m to predict RdNBR. Future work should investigate how ladder fuel densities using our techniques can be validated with destructive sampling and incorporated into predictive models of wildfire severity and fire behavior at varying spatial scales.
Introduction
There is an urgent need in the western United States to reduce wildfire hazard and restore wildfire’s historic role as a beneficial ecological process (Stephens et al., 2012; Duff et al., 2013, 2019). Surface fuels, often < 1 m in height and mostly horizontal in orientation, carry fire across the ground when there is continuity of litter, slash, herbaceous vegetation, shrubs, small conifers, and downed woody material (Brown, 1982; Schmidt et al., 2008). Ladder fuels, which are live and dead vegetation that bridge the gap between the surface and the canopy, can provide a conduit for a low-severity surface fire to become a high-severity canopy fire (Menning and Stephens, 2007; Ottmar et al., 2007). Management targeted at reducing surface and ladder fuels can effectively mitigate wildfire intensity and burn severity (Agee and Skinner, 2005; Ritchie et al., 2007; Safford et al., 2012; Prichard et al., 2013). Due to the scale of the area that requires treatment, targeted management must be informed by mapping the spatial distribution of ladder fuels to help prevent high-severity fires.
It is generally time consuming and ineffective to quantify ladder fuel loads in the field using traditional forestry methods (e.g., Keane et al., 2005). Sometimes, fuels are measured indirectly via canopy base height (CBH), the average distance between the bottom of the canopy and the ground. An alternative approach is to estimate fuel structure via remote sensing technology. Relative to field-based techniques, remote sensing allows for measurements across large and inaccessible areas at a potentially lower cost, depending on scale of measurements (Gale et al., 2021). Sensing can be from a top-down or downward (typically airborne or spaceborne) or a bottom-up or upward (typically ground-based) view of forest structure (Skowronski et al., 2011). Depending on photon flux at a particular wavelength, biochemistry, and three-dimensional (3D) structure, downward sensing will generally detect more upper-canopy components due to progressive attenuation of photons from the top of the canopy to the surface, whereas, for the same reasons, upward sensing will be relatively sensitive to lower-canopy components.
From the downward sensing perspective, the use of airborne laser scanners (ALS), or LiDAR, has been used to estimate spatially explicit fuel parameters over landscape to regional scales (Andersen et al., 2005; Jakubowski et al., 2013; Kelly and Di Tommaso, 2015; González-Ferreiro et al., 2017), and can contribute to reliable and robust estimates of modeled forest fire behavior (Kelly et al., 2017). For example, in oak woodlands of northern California, ALS data were used to estimate canopy cover, canopy height, and ladder fuels at 1-m resolution at a county scale (∼458,000 ha; Green et al., 2020).
At plot to stand scales (i.e., 1 to 50 ha), unoccupied aerial systems (UAS; Joyce et al., 2021) can be outfitted to acquire LiDAR (i.e., active sensing) or digital aerial images (i.e., passive sensing) at lower costs relative to airplane-mounted sensors, which is useful for repeated forest monitoring (Campbell et al., 2020; Hillman et al., 2021a,b). When UAS are flown to capture images with sufficient overlap (e.g., 75–85%), Structure from Motion (SfM) data processing can generate 3D point clouds of vegetation structure, which has the potential to quantify fuel loads. Although UAS-SfM generally provides highly variable or unresolved data of below-canopy vegetation structure (Wallace et al., 2016; Graham et al., 2019; Hillman et al., 2021a,b; Reilly et al., 2021), the technology has been used to successfully estimate canopy height and cover, DBH, and stem count (Wallace et al., 2016; Shin et al., 2018; Puliti et al., 2020; Reilly et al., 2021).
From the upward sensing perspective, terrestrial laser scanning (TLS) is a ground-based form of LiDAR mounted on a tripod. This technology has been used successfully to estimate plot-scale variables related to the spread of canopy fires, subtle fire-induced change, and forest fuels structural metrics (García et al., 2011; Gupta et al., 2015; Chen et al., 2016; Hillman et al., 2021a,b), with millimeter accuracy and precision (Disney, 2019). The use of TLS allows for fine-scale and high-resolution measurement of forest structure, enabling studies to measure quantitative changes in fuels over time (Wallace et al., 2016; Singh et al., 2018).
Handheld-mobile laser scanners (HMLS), also used from the upward sensing perspective, are a lightweight LiDAR about 30% the cost of a TLS. HMLS have been used to accurately estimate tree height under 25 m (Hyyppä et al., 2020) and diameter at breast height (DBH; Chudá et al., 2020; Hyyppä et al., 2020) with less variation than field measurements. In addition, HMLS technology typically requires less processing time compared to TLS and reduces the issues of occlusion that occur with TLS sampling on a fixed grid due to HMLS being one single walking scan. This allows for many different scan angles and locations, albeit with the compromise of lower range and precision compared to TLS, particularly in scanning the upper canopy (Ryding et al., 2015; Almeida et al., 2019; Soma et al., 2021). Currently, few studies have investigated the use of HMLS to examine forest structure parameters (Marselis et al., 2016; Donager et al., 2021), and we are unaware of any studies that have focused on HMLS and ladder fuels.
While remote sensing approaches are valuable, calibration and validation of remote sensing data with ground-based data is crucial. There are very few studies to date that validate fuels measured by remote sensing with ground-based direct measurements, such as destructive sampling or intercept methods (Hillman et al., 2019). Kramer et al. (2016) presented a low-cost ground-based photographic technique (referred to hereafter as “photo banner”) to visually measure ladder fuels from 1 to 4 m. Importantly, photo banner measurements were found to correlate with ladder fuel density developed using ALS data from 1 to 8 m (Kramer et al., 2016). Currently, land managers and conservation groups in Sonoma County, California have explored machine learning models to predict wildfire severity that include ladder fuel point densities from 1 to 4 m via ALS measurements (Green et al., 2020). The higher the density of shrubs and forest ladder fuels, the higher the canopy damage observed following wildfires.
Given the important predictive role of ladder fuels estimated by ALS data, but the often prohibitive cost and effort required for repeat monitoring with ALS data, the purpose of this study was to compare a suite of remote sensing approaches (TLS, HMLS, UAS-SfM, and ALS) and field measurements (photo banner) to measure plot-scale ladder fuels in oak woodlands in the same region. In addition, we compared the use of various measurements of ladder fuel densities to predict wildfire burn severity in an effort to provide alternative options to ALS data. Specifically, we aimed to answer the following questions: (1) What is the linear relationship between ladder fuel densities estimated using TLS, HMLS, UAS-SfM, ALS, and photo banner methods and do the strength of these correlations change in plots with different forest structure (i.e., mean CBH)?; (2) For each method, can ladder fuels be used to predict wildfire burn severity (i.e., Landsat-based Relativized delta Normalized Burn Ratio; RdNBR) at a plot scale? If so, which ladder fuel density strata from 1 to 8 m is the most important predictor variable?; and, (3) When predicting burn severity, do different methods of estimating ladder fuel densities or including CBH lead to different predictive capabilities?
We hypothesized that ladder fuel densities from different approaches would be correlated to each other if their measurement approach was similar (i.e., terrestrial or airborne perspectives, laser or image based). In addition, we hypothesized that TLS and HMLS collected data would most accurately predict burn severity (RdNBR) due to high point density, closely followed by ALS. We predicted UAS-SfM and the photo banner would not be able to significantly predict burn severity (estimated using RdNBR; Miller and Thode, 2007) due to the lack of below canopy detection of UAS-SfM and 4-m height limit of the banner. We hypothesized that the most important predictor of burn severity would be ladder fuel density strata from 1 to 4 m, as it was highly significant in the Green et al. (2020) model.
Materials and Methods
Data Collection and Processing
Remote sensing and field data related to forest structure were collected from two study sites, Pepperwood Preserve (3,200 acres; 38° 34′ 57.5″ N, 122° 42′ 37.3″ W) and Saddle Mountain Open Space Preserve (960 acres; 38° 30′ 3.3″ N, 122° 37′ 44.6″ W), both in the Mayacamas Mountains in Sonoma County 10-20 km outside of Santa Rosa, California, United States (Figure 1 and Supplementary Figure 1).
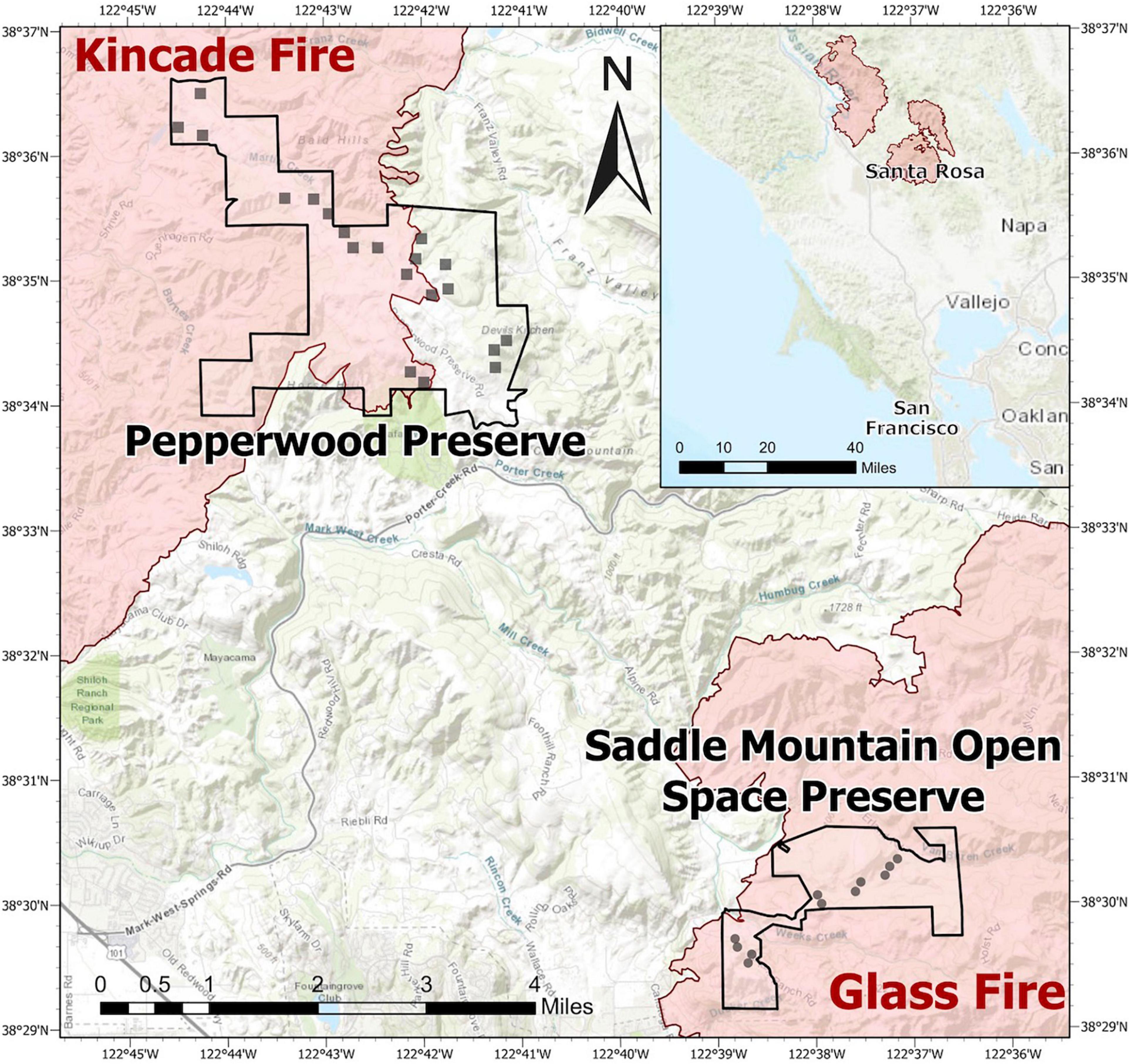
Figure 1. Map of our study locations (Pepperwood Preserve and Saddle Mountain Open Space Preserve) and plots (indicated by gray squares and circles) in Sonoma County, California. The perimeter of each wildfire that occurred during our research study is shown in red.
The most prominent community at Pepperwood is oak woodlands, with rolling hills also consisting of Douglas-fir and redwood conifer forests, chaparral shrublands, and grassland (Evett et al., 2013; Ackerly et al., 2020). Saddle Mountain primarily consists of mixed hardwood and conifer forest, oak woodland, grassland, and chaparral. Across the two study sites, the top five species in both abundance and basal area are Pseudotsuga menziesii (PSME), Quercus agrifolia (QUAG), Q. garryana (QUGA), Q. kelloggi (QUKE), and Umbellularia californica (UMCA; Supplementary Figure 2). While PSME has the most basal area (33%), it is the third most abundant based on density (17%). QUAG and QUGA are the second most basal area dominant species and have the greatest abundance, comprising 23% and 17% of the trees across all plots, respectively. Between the two sites, CBH, DBH, and tree height were similar in distribution, although the number of trees per plot was greater at Saddle Mountain compared to Pepperwood (Supplementary Figure 3).
In September 2019, Pepperwood Preserve data were collected from 24 plots (20 × 20 m, 0.04 ha) that are part of a Sentinel site plot network and have had demographic measurements collected since 2013 (Ackerly et al., 2013). In 2017, the Tubbs Fire burned through Pepperwood, creating a dramatic gradient in wildfire severity throughout all plots. Immediately following the measurement survey in September 2019, 60% of Pepperwood Preserve re-burned in the Kincade Fire including 14 of our 24 study plots.
In August 2020, we established 11 plots (circular with a radius of 11.3 m, 0.04 ha) at Saddle Mountain Open Space Preserve. In September 2020, immediately following the measurement survey, the Glass Fire burned all of the plots.
At each site, a variety of approaches were used to generate ladder fuel densities at different height strata from 1 to 8 m (Table 1). All measurements (TLS, HMLS, UAS-SfM, photo banner) were taken at the phenologically same time each year at each site (Pepperwood = September 2019; Saddle Mountain = August 2020), except ALS which was collected between September and November 2013 at both sites. Since ALS data collection occurred prior to both the Tubbs and Glass Fires, changes in both forest structure and ladder fuels could be expected by the time of our field and remote sensing surveys. Reilly et al. (2021) compared canopy metrics at Pepperwood between 2019 UAS-SfM data to the 2013 ALS data and found significant relationships between these methods for unburned sites. However, they did detect changes in canopy attributes with increasing wildfire severity (RdNBR). We interpret our results in light of this time lag and disturbance history. Below, we first describe the field-based methods used (photo banner, canopy base height), and then follow with descriptions of the remote sensing approaches (TLS, HMLS, UAS-SfM, ALS).
Photo Banner
We adopted Kramer et al.’s (2016) photo banner to measure ladder fuels at a plot-level (see Supplementary Material for more details). In each plot, the photo banner (Supplementary Figure 4) was placed at the plot edge or corner and a digital photograph was taken facing the corner from the plot center (Supplementary Figure 5), providing a total of 4 pictures per plot.
Ladder fuel banner photos were analyzed by finding the percent area of all parts of vegetation covering the photo banner via ImageJ (Kramer et al., 2016; National Institute of Health, Maryland, United States, version 1.52). First, the image was converted into an 8-bit grayscale image. The measured area was set to a single 1 × 0.5-m rectangle and the intensity, or lightness, threshold was adjusted to select the darker parts. This selection included any vegetation in front of the photo banner and could include shadows. This selection was then converted into percent area. The photos were independently analyzed by two people and results were averaged per 1 × 0.5-m area section to account for human error. The photo banner pictures were taken at 34 out of 35 total plots and 24 out of 25 burned plots.
Canopy Base Height
Canopy base height (CBH), a distance that influences vertical propagation of fire and is easily measured in the field or by remote sensing is the variable most often used to model the transition of surface to crown fires, as opposed to ladder fuels (García et al., 2011). However, the definition of CBH varies across the literature. From the point of view of fire behavior, CBH is a fuel metric that defines the distance between the bottom of the canopy and ground. According to some authors (e.g., Scott and Reinhardt, 2001), it is the lowest height in a stand at which there is a sufficient amount of forest canopy fuel to propagate fire vertically into the canopy, i.e., the height above ground level at which the bulk density reaches a specified minimum value (Sando and Wick, 1972). When this definition is used, ladder fuels such as lichen, dead branches and small trees must be included in the calculation of CBH. However, other authors (e.g., Cruz et al., 2003) have indicated that CBH can be calculated as the average vertical distance between the ground surface and the live canopy fuel layer. The advantage of this definition is that it is compatible with the canopy fuel stratum characteristics used in the crown fire initiation and propagation model developed by Van Wagner (1977).
For this study, we defined CBH as the average distance between the bottom of the canopy and ground. Specifically, tree crown base height was defined as the lowest live crown with at least two branches at the same height and less than a 1-m gap from the continuous main crown. Crown base height was measured for each tree > 10 cm DBH in each plot. A tape measure was used to measure from the lowest live crown to the ground when possible. In cases when a tape measure could not reach, a laser hypsometer was used. Then, to determine canopy base height (CBH), the crown base heights for each tree within each plot were averaged. The CBH values were binned evenly into four CBH categories supporting a visual inspection of the data distribution as follows: low (0–3 m), medium (3.01–6 m), high (6.01–9 m), and very high (>9 m). The sample size for the number of plots in each CBH category varied and was n = 8 (low), n = 8–12 (medium), n = 6–7 (high) and n = 4–5 (very high).
Terrestrial Laser Scanner
A Riegl VZ-400i TLS (RIEGL Laser Measurement Systems GmbH) was used to scan each plot. The VZ-400i is a discretized multiple-return LiDAR scanner with a 1550-nm wavelength (shortwave infrared), 1200 kHz pulse rate and a 0.35-mrad beam divergence (Wilkes et al., 2017; Gonzalez de Tanago et al., 2018). This TLS has a maximum scan range of 800 m with view angles of 360° in azimuth and 100° in zenith angles (Lau et al., 2019). Within each plot, there were 9 scan positions 10-11.3 m apart based on a grid (Supplementary Figure 5), as recommended by Wilkes et al. (2017) to capture uniform point density throughout the plot and canopy. No significant relative difference in point density was found between the two sites. At each scan position, both an upright position scan and a 90° tilt scan were taken to get complete coverage of the ground and the upper canopy. These scans were taken at times of the day when it was not windy (usually between 7 am and 4 pm PST). If there was a gust of wind, the scan was canceled and taken again once the wind slowed to prevent a ghosting effect in the point cloud.
Processing for TLS data occurred in RiSCAN PRO (Riegl Laser Measurement Systems GmbH, Horn, Austria, version 2.8.2). This software allowed us to align all scans within each plot to each other to create one plot-level scan. First, a coarse registration was applied using automatic registration. Automatic registration used voxels with a resolution of 0.50 m and an on-board Global Navigation Satellite System (GNSS) to align the scans to each other in space. Then, plane files were generated for a Multi-Station Adjustment (MSA), which properly aligned the planes to each other for the most accurate registration by adjusting the orientation and position of each scan position in several iterations to calculate the best overall fit. Using GNSS, data were georeferenced in NAD83(2011)/UTM Zone 10N and geoid 12B for accurate height data. Once registered and georeferenced, TLS plot-level data were manually aligned to ALS data for accurate georeferencing in RiSCAN PRO. Following registration and alignment, Lidar360 (GreenValley International, Berkeley, California, version 4.1) was used to create a 0.25-m raster DEM interpolated from a triangular irregular network (TIN) from TLS data for each plot. This DEM was then used to height-normalize plot-level TLS data. TLS data were collected at all 35 plots and all 25 burned plots. The plot center was determined from visual examination of the ALS-adjusted TLS point cloud and used for determining the plot location and aerial extent for all methods.
Handheld-Mobile Laser Scanner
We used a GeoSLAM ZEB-Revo (GeoSLAM, Nottingham, United Kingdom) HMLS system, which has a 905 nm laser (near infrared), scan range of 360° in azimuth and 270° in zenith angles, and maximum range of 15 to 20 m. We walked the HMLS for 5–10 min throughout each plot to collect data points. The path of the HMLS was a closed loop around the perimeter while pointing the instrument toward the center of the plot and then walking smaller loops inward in a haphazard pattern to scan areas that could not be collected from the perimeter (Bauwens et al., 2016; Supplementary Figure 6).
Using the GeoSLAM software (GeoSLAM, Nottingham, United Kingdom), HMLS data were automatically generated for each plot. The resulting point clouds were then imported into RiSCAN PRO and aligned to the ALS-aligned TLS data using MSA. This allowed georeferencing of HMLS data in NAD83(2011)/UTM Zone 10N with geoid 12B. Lidar360 was then used to height normalize HMLS data by creating a TIN-generated DEM (see TLS) for each plot. HMLS data were collected at all 35 plots and all 25 burned plots.
Unoccupied Aerial System
A SenseFly eBee X fixed-wing UAS with a MicaSense RedEdge-MX sensor, which collects blue, green, red, red edge, and near-IR spectral bands, was flown during the same time period that TLS, HMLS, and photo banner data were collected. A DJI Matrice 200 quadcopter UAS with the same MicaSense RedEdge-MX sensor was used for two plots at Saddle Mountain due to accessibility issues. Pepperwood was split into eleven zones with average size of 33 ha and total area of 332 ha. Saddle Mountain was split into five zones with an average size of 6 ha and total area of 29 ha. Each zone had two autonomous flights at 120-m above-canopy height, 75% along- and across-track overlap in a crosshatch grid pattern, and a nadir view angle for the sensor. For more detailed information about UAS methods see Reilly et al. (2021).
Pix4Dmapper (Pix4D, Lausanne, Switzerland, version 4.4.12 and 4.6.4) was used to create reflectance orthomosaics for each spectral band with an 8-cm resolution for each zone and perform SfM point cloud processing for each flight zone using the green spectral band. Using Lidar360’s ICP registration, UAS-SfM data were aligned to ALS data. Due to the accuracy of the ALS DEM (see below), it was used to height-normalize UAS-SfM data using the R lidR and raster packages (Hijmans et al., 2019; R Core Team, 2020; Roussel et al., 2020). UAS-SfM data were collected at 33 out of 35 plots, but only 29 of these plots (17 out of 25 burned plots) had data below 8 m due to UAS limitations on below canopy vegetation detection. Two plots were not flown due to overhead cable obstructions.
Airborne Laser Scanner
Airborne laser scanner (ALS) data were downloaded from existing data collected in 2013 for Sonoma County (QL1/2013). The imagery was collected using Leica ALS50 and ALS70 sensors at 5054 m altitude on a Beechcraft Airliner twin turboprop aircraft. These sensors have 1064 nm (NIR) lasers. The maximum RMSE for the georeferencing of this data was 0.2 cm due to the use of 9,685 ground control points (Watershed Sciences, 2016). Data can be found at http://sonomavegmap.org/data-downloads/.
Most pre-processing was already complete for the ALS dataset. Watershed Sciences (2016) used TerraSolid to classify ground points and a statistical surface algorithm to refine their classification. For each UAS zone, ALS ground points were used to create a 1-m raster DEM using the R lidR (Roussel et al., 2020) and raster packages and TIN interpolation. The DEM was then used to height-normalize the ALS point cloud for each zone. ALS data were collected at all 35 plots and all 25 burned plots.
Wildfire Burn Severity
We defined burn severity using the Relativized delta Normalized Burn Ratio (RdNBR) applied to pre- and post-fire Landsat 8 multispectral satellite imagery (Miller and Thode, 2007). RdNBR measures the amount of dead vegetation post-fire in relation to the amount of alive pre-fire vegetation. Therefore, a positive value indicates a decrease in vegetation cover. In California, RdNBR has been well-calibrated with field measurements of wildfire severity (Miller et al., 2009), and Landsat-based time series of RdNBR have been used to track regional shifts in fire severity (Mallek et al., 2013; Coppoletta et al., 2016; Stevens et al., 2017; Nigro and Molinari, 2019). This metric measures the change in vegetation as a result of wildfire, rather than the energy output or other environmental responses, such as soil (Keeley, 2009).
We used Landsat 8 Collection 2 Level-2 data from USGS EarthExplorer. These data were atmospherically corrected reflectance measurements with a 30-m pixel resolution. The Kincade fire started on October 23, 2019 and burned until November 6, 2019. For pre-fire data, a Landsat image from October 17, 2019 was used. For post-fire data, a November 2, 2019 image was used as the fire was 72% contained by then and was no longer burning in the study plots on that date. The Glass fire started on September 27, 2020 and burned until October 20, 2020. A Landsat image from September 17, 2020 was used for pre-fire data and an October 19, 2020 image was used for post-fire data as the fire was no longer burning in the study plots on that date. To determine dNBRoffset, which accounts for seasonal phenology changes between pre- and post-fire images, the mean dNBR in a 1-km area around each fire perimeter was calculated and focused on the native forests as defined by the Sonoma County Lifeform Project.1
A raster layer was created for each calculation and, using TLS plot centers, the average of the closest 9 cells (3 × 3 cells; 90 × 90 m), including the cell where the plot center was located, were used to measure the continuous RdNBR value for each plot, following the techniques of Miller and Thode (2007). Plot RdNBR values were binned into four burn severity classes, using breaks determined with the dNBR offset (Miller and Thode, 2007): no change (-500-0), low (69–315), moderate (316–640), and high (640–1200). Due to a small sample size for high severity plots (n = 1), the high severity plot was grouped into a moderate and above group (316–1200). Categorical data for RdNBR were created for exploratory analyses but were not used in the final models presented here (model results can be found in Supplementary Table 1).
Data Analyses
Ladder Fuels
For all point clouds from each method, a standardized vector polygon was used to cut each point cloud to either a 20 × 20-m square (Pepperwood) or 11.3-m radius circle (Saddle Mountain) plot. Ladder fuel densities were then extracted at the plot level from TLS, HMLS, UAS-SfM, and ALS point-cloud data using the lidR package in R (Roussel et al., 2020). The mean CBH across all plots (5.0 m) plus one standard deviation (2.7 m) was used to determine the maximum height for ladder fuels (8.0 m). That is, we chose not to calculate ladder fuel strata > 8 m as we did not want to consistently include living crowns in our measurements. It should be noted, however, that in plots with low or medium CBH, ladder fuel strata > 6 m might include parts of the living crowns of trees. Fuels below 1 m are often defined as surface fuels (Keane et al., 2012; Kramer et al., 2016), thus we used 1 m as the minimum height for ladder fuel strata. Using Equation 1 (Kramer et al., 2016, Table 2), the density of points within all 1-m strata from 1 to 8 m were calculated using points above 0 m in the denominator (Table 1). We also computed ladder fuel densities in 1-4 m and 1-8 m strata.
Comparing Ladder Fuel Densities Across Methods
Ladder fuel density data were log transformed for normality (Supplementary Figure 7) and Pearson’s product moment correlation (r) values were used to compare ladder fuel densities from 1–4 m to 1–8 m between each method. Since the photo banner was only 4-m tall, photo banner data were not included in analyses using the 1–8 m strata. Plots were grouped into CBH bins and individual correlations were conducted on these separate classes to determine if overall plot forest canopy structure influenced the strength and significance of the correlations. Note that for this study, CBH data were only measured in the field and not via remote sensing methods. To discuss the results of Pearson’s correlation heat maps, the following classifications were used: negative (–1 to –0.01), very low (0 to 0.30), low (0.31 to 0.50), moderate (0.51 to 0.70), and high (0.71 to 1.00).
Modeling the Relationship Between Ladder Fuels and Burn Severity
To determine the effect of ladder fuels densities on RdNBR, and interactions with CBH, generalized linear models were selected using proc GLMSELECT in SAS (SAS Institute Inc, Cary, North Carolina, version 9.4) using forward stepwise variable selection based on minimizing Schwarz’s Bayesian Criteria (SBC). Model residuals were visually assessed for approximate normality and homoscedasticity. The global model contained each ladder fuel density strata at discrete 1-m increments, CBH, and the interaction between CBH and each ladder fuel density strata. The model selection process started with the intercept only model and the variables with the most explanatory power that decreased the SBC were added until adding the next variable gave an equivalent SBC. Both burn severity continuous data and binned categories were analyzed, but only CBH categories were used in the final model as they had significantly more predictive power. The coefficient of determination (R2) for continuous values and the likelihood ratios (LR) for categorical values were used to evaluate model performance and SBC was used to evaluate the explanatory power of the selected variables. The greater the value for R2 or LR, the higher the predictive power of the model, but the lower the SBC, the higher the explanatory power of the variables.
Results
Sensitivity to Ladder Fuel Density Among Methods
Data collected by TLS, HMLS, UAS-SfM, and ALS had average plot-level point densities of 399,064 pts/m2, 16,378 pts/m2, 330 pts/m2, and 17 pts/m2, respectively. For each method, there was a high percentage of points between 0 and 1 m and a decrease in the percentage of points between 1 and 2 m (Table 2). While our ladder fuel density strata were between 1 and 8 m, we also included the point density between 0 and 1 m in our discussion as this measurement was included in the calculation of each ladder fuel density strata. The TLS and HMLS data gradually decreased in point density with increasing strata height, due to bottom-up scanning of the forest (Table 2). On average, 84% of TLS plot data were below 8 m, and 40.7% were between 1 and 8 m (Table 3). On average, 96.7% of HMLS plot data were below 8 m, and 32.5% were between 1 and 8 m (Table 3). The inability of HMLS to sense above 8 m is clearly seen for representative plots with mean or high CBH when compared to ALS (Figure 2). In contrast, point density in UAS-SfM and ALS data gradually diminished with decreasing strata height (not including 1–2 m for UAS-SfM, which had 1.2% more points than 2–3 m), due to the top-down views of those sensors (Table 2). Data collected using UAS-SfM had the highest point density at the top of the canopy, but the technique did not capture as many understory or ground points as TLS or HMLS (Figure 2). Only 13.3% of UAS-SfM data were between 1 and 8 m, while 28.2% were between 0 and 8 m (Table 3). Data collected by ALS were comparatively sparse, but had better canopy penetration than UAS-SfM, with an average of 35.9% of points between 0 and 8 m, 19.6% of points between 1 and 8 m, and 2.8% of points in each stratum between 1 and 8 m (Figure 2 and Table 3).
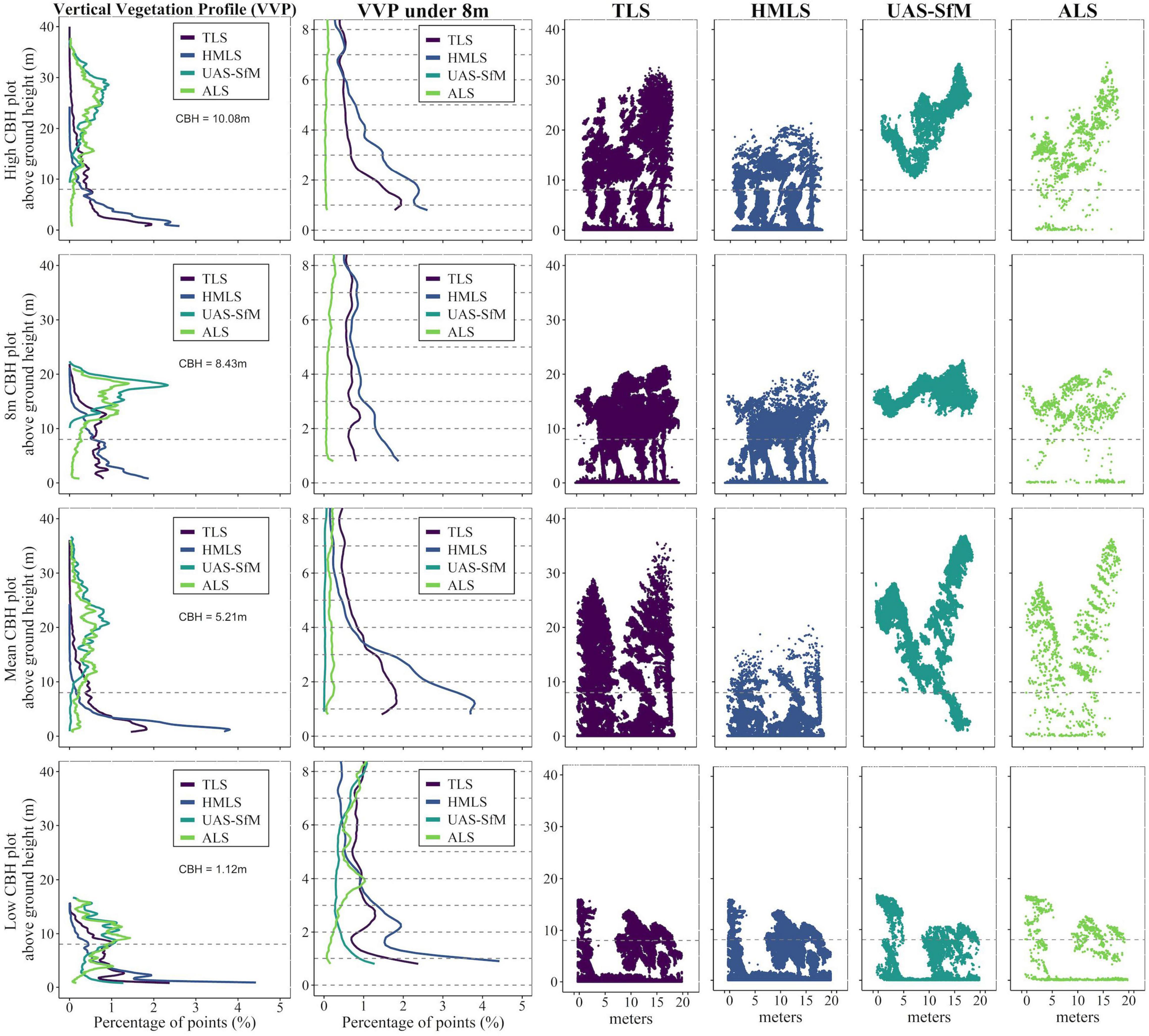
Figure 2. Vertical profile of vegetation (VVP) generated by each remote sensing method, separated into bins based on CBH for a representative plot. The CBH categories used are as follows: low (0–3 m), medium (3.01–6 m), high (6.01–9 m), and very high (>9 m). We included a plot with a CBH of ∼8 m as this is the mean plus one standard deviation and used as the maximum height for our ladder fuels strata. The percentage of points at each height was found by dividing the number of points at each height by the total number of points (above 0.5 m) for each method. VVP under 8 m shows the same data as the whole VVP, but zoomed in. The gray dashed line shown in the VVP represents 8 m, the maximum height we used for ladder fuels, the gray dashed lines shown in the VVP under 8 m, are in 1m increments and represent our ladder fuel densities.
Comparing Ladder Fuel Densities Across Methods
The largest significant correlation for ladder fuel densities from 1 to 4 m was between TLS and ALS (r = + 0.87, p < 0.001; Figure 3). The only non-significant correlation was between UAS-SfM and the photo banner (r = + 0.33, p > 0.1). All other methods were at least marginally significantly correlated (r from +0.49 to +0.84, p from 0.10 to <0.001) and TLS had the largest and most significant correlations with other methods (average r = + 0.76, average p < 0.001). The greatest correlation for ladder fuels strata 1-8 m was between TLS and ALS (r = + 0.71, p < 0.001) and TLS had the largest and most significant correlations with other methods (average r = + 0.69, average p < 0.001). The least significant and lowest correlation was between UAS-SfM and HMLS (r = + 0.30, p = 0.1).
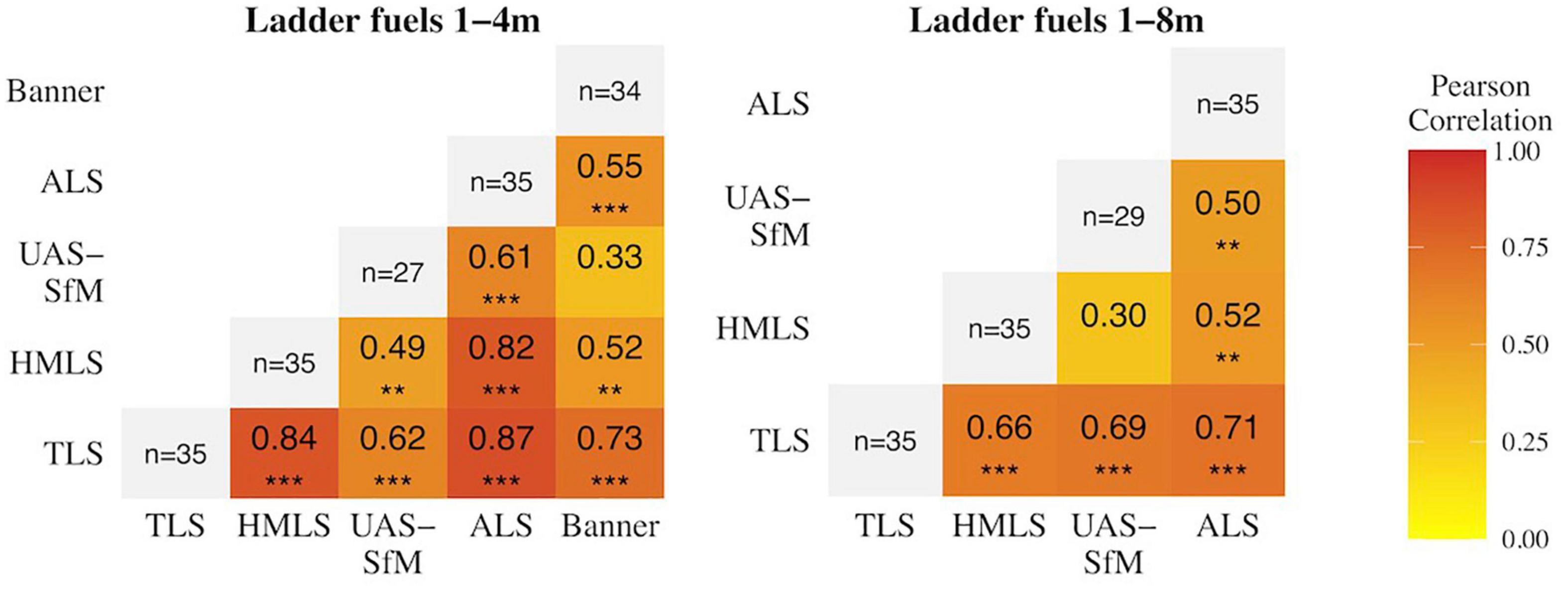
Figure 3. Heatmap of Pearson correlation coefficients to compare ladder fuel densities 1–4 m and 1–8 m across methods. Significance is as follows: *** p-value ≤ 0.001 and ** 0.001 > p-value ≤ 0.01.
Comparing Ladder Fuel Densities Across Methods Within Canopy Base Height Categories
Terrestrial laser scanner (TLS) and HMLS were not significantly correlated for ladder fuel density strata 1–4 m in very high (r = + 0.66, p = 0.2) CBH plots, but were significantly correlated in low (r = + 0.72, p = 0.04), medium (r = + 0.94, p < 0.001), and high CBH plots (r = + 0.91, p = 0.004; Figure 4). TLS and ALS had high correlations in all CBH plots (r from + 0.70 to + 0.96, p from 0.1 to < 0.001). TLS and ALS were significantly correlated in medium (r = + 0.96, p < 0.001) and high CBH plots (r = + 0.96, p < 0.001). UAS-SfM was not significantly correlated with TLS or HMLS at any CBH class (note removal of UAS-SfM from very high CBH class). HMLS and ALS were significantly correlated in low (r = + 0.74, p = 0.04), medium (r = + 0.88, p < 0.001), and high (r = + 0.85, p = 0.02). UAS-SfM and ALS were significantly correlated for low CBH plots (r = + 0.72, p < 0.04) and high CBH plots (r = + 0.86, p = 0.03). The photo banner only had significant correlations with TLS (r = + 0.84, p = 0.001), HMLS (r = + 0.75, p = 0.008), and ALS (r = + 0.62, p = 0.04) in medium CBH plots and TLS (r = + 0.94, p = 0.001) and ALS (r = + 0.88, p = 0.008) in high CBH plots. The photo banner had the lowest correlations overall, and was the only method to have negative correlations. While the plot sample size per CBH category was small (n = 4 to n = 12), there were some patterns observed (Table 4). Relative to full data correlations, the separation of plots by CBH categories generally increased the strength of the correlations in medium and high CBH plots (most so for the banner and UAS correlations) and decreased the strength of the correlations in low and very high CBH plots (especially when using photo banner data).
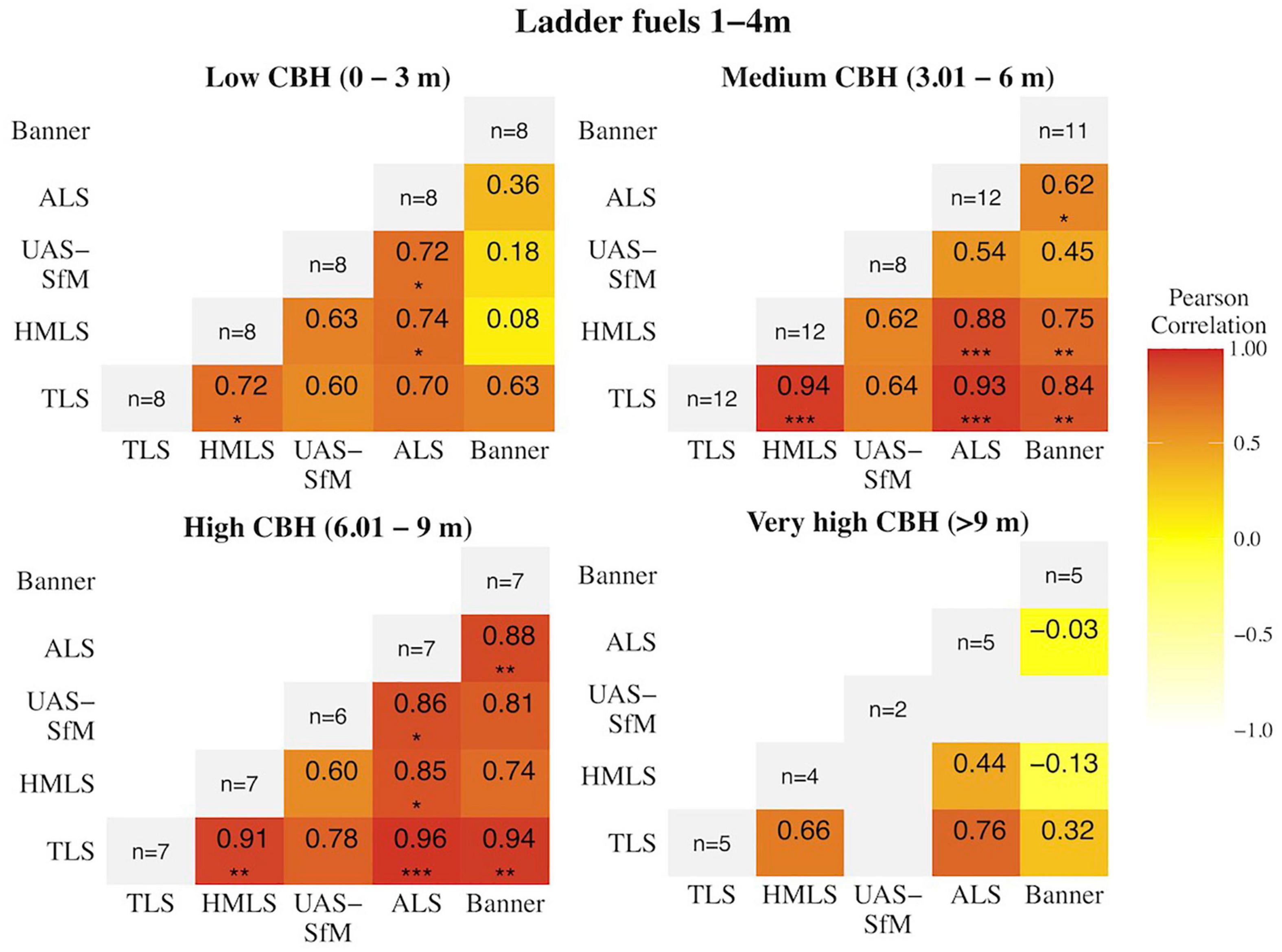
Figure 4. Heatmap of Pearson correlation coefficients for ladder fuel density 1–4 m by CBH category across all methods. The CBH categories used are as follows: low (0–3 m), medium (3.01–6 m), high (6.01–9 m), and very high (> 9 m). Significance is as follows: *** p-value ≤ 0.001, ** 0.001 > p-value ≤ 0.01, * 0.01 > p-value ≤ 0.05.
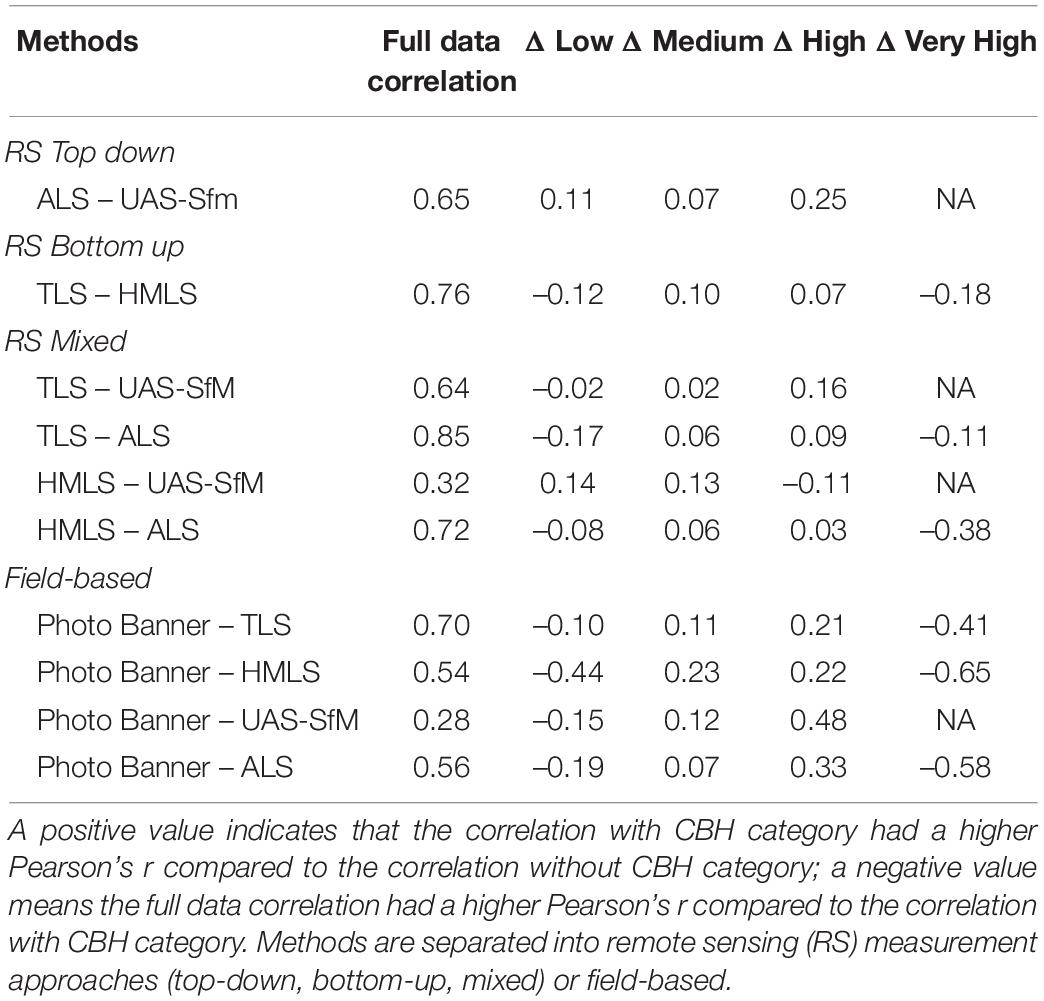
Table 4. Differences (Δ) between the Pearson correlation values of ladder fuel density from 1 to 4 m strata calculated without (full data) and with canopy base height (CBH) categories (low, medium, high and very high).
Modeling the Relationship Between Ladder Fuels and Burn Severity
The ladder fuel stratum/strata selected for each model to best predict RdNBR varied among methods (Table 5). TLS and ALS data produced the best models with very similar explanatory (R2 = 0.67 and 0.66, respectively) and predictive (SBC = 255.4 and 252.7, respectively) power. Both models included fuel strata 1–2 m, with the interaction of CBH. The TLS model also included CBH, and the ALS model also included fuel strata 3–4 m and fuel strata 5–6 m with the CBH interaction. The UAS-SfM model had the next highest predictive power (R2 = 0.53) and included fuel strata 7–8 m and fuel strata 3–4 m with a CBH interaction. However, the lack of below canopy detection caused this model to have a lower sample size than the other models, which artificially increases R2 and does not allow the SBC value to be comparable to other models. The HMLS model included fuel strata 7–8 m with a CBH interaction, with less explanatory and predictive power (R2 = 0.44, SBC = 259.0) than the other models. The photo banner model did not include any ladder fuels data and had no predictive power. Parameter estimates from the model can be found in Supplementary Table 2 and model fit criteria are shown in Supplementary Figure 8.
Discussion
Due to the immense economic and ecological impact of wildfires and the significant relationship between canopy damage and ladder fuel density found in previous studies (Dennison et al., 2014; Balch et al., 2018; Green et al., 2020; Hernandez, 2020), we evaluated different remote sensing and traditional field-based approaches for measuring ladder fuel density. In addition, we aimed to determine if ladder fuels, and which ladder fuel stratum in particular, could be used to predict wildfire burn severity in our study forests. Indeed, an assessment of 3D fuel structure is an important planning tool to assist in designing effective forest fuels management actions in light of wildfire (Hillman et al., 2021a,b).
Comparing Ladder Fuel Densities Across Methods
Estimates of ladder fuel densities were generated using TLS, HMLS, UAS-SfM, ALS, and photo banner methods, using sub-canopy height stratification techniques similar to other studies (Skowronski et al., 2007; Kramer et al., 2016; Rowell et al., 2020). While it was not possible to field validate the ladder fuel density measured by each method in this study (i.e., through destructive sampling), our results imply that all methods, except UAS-SfM and the photo banner from 1 to 4 m and UAS-SfM and HMLS from 1 to 8 m (note that the photo banner is not included in this binned strata), can be used interchangeably to quantify ladder fuel density in 1-4 or 1-8 m strata. These results support work by Kramer et al. (2016) who found that the photo banner could be used to validate ALS measurements of ladder fuels. The non-ALS approaches explored in our study are easier and less expensive to collect than ALS data at the plot scale. Thus, these alternative methods can be completed at a high temporal resolution, and might be beneficial for land managers to describe changes in ladder fuel density over time. In addition, it is possible that since there was a strong, significant correlation between TLS, HMLS, and ALS based density estimates, these approaches may be able to assist in the calibration and validation of spaceborne optical, LiDAR, and SAR missions (e.g., GEDI, ICESAT-2, NISAR, BIOMASS; Levick et al., 2021; Leite et al., 2022), or a state-wide forest monitoring system such as the California Forest Observatory, which estimates ladder fuels and CBH from multispectral satellite imagery calibrated with ALS.
While we found various strong relationships between methods in ladder fuel densities, the magnitude of each measurement across techniques was not the same, primarily due to differences from the top-down (ALS, UAS-SfM), bottom-up (TLS, HMLS) or 2D ground-based view (photo banner) of the canopy. Since TLS and HMLS had 84% and 97% of their collected points below 8 m, respectively, HMLS and TLS ladder fuels were significantly correlated. In contrast, only about a third of UAS and ALS points were below 8 m (28% and 36%, respectively), with UAS points heavily concentrated in the upper canopy or in canopy gaps (Figure 2). Consequently, ladder fuel correlations between UAS and ALS were generally weaker than TLS and HMLS. These findings corroborate other studies which have found that TLS are more sensitive to 3D structure in the lower canopy than ALS and UAS LiDAR (García et al., 2011; Brede et al., 2019; Hillman et al., 2021a,b; Levick et al., 2021).
Similar to Hillman et al. (2021b), UAS-SfM methods were unable to consistently detect sub-canopy structure when compared to TLS, particularly in forests with closed canopies. We explored this technology due to its relatively low cost compared to airborne small-footprint lidar, mounted either on a plane or UAS. Despite its limitations, we found that UAS-SfM was significantly correlated with ALS across a range of ladder fuel strata, and correlations strengthened considerably with ALS and TLS when considering CBH classes. Thus, while patterns in metrics were correlated between sensors, variation in the ladder fuel percentage values due to top-down vs. bottom-up measurement approaches, in addition to factors such as laser scan overlap, scan angles, wavelength, and power, could explain differential significance of correlations among ladder fuels in our results, and ultimately their utility in subsequent fire behavior or burn-severity modeling.
While we used density-based approaches to estimate ladder fuels, future studies should explore the use of voxel-based metrics or standardization of density metrics using voxels (Hillman et al., 2021a,b). While Green et al. (2020) and Atchley et al. (2021) and found significant results using density methods, voxels, a unit which defines 3D space, could be an alternative way of calculating ladder fuels. Wilson et al. (2021) found voxels to effectively explain the effects of logging and wildfire on vertical fuel continuity by using these methods to explain forest structure.
Modeling the Relationship Between Ladder Fuels and Burn Severity
Measurements of ladder fuel densities using various remote sensing approaches were able to moderately estimate wildfire burn severity. When we examined the utility of ladder fuel density and CBH to predict RdNBR, the most common ladder fuels strata included were 1–2 m, 3–4 m and 7–8 m, even though in some plots it is possible that 7–8 m included data from tree canopies (Figure 2, Low CBH). These results support results by Green et al. (2020) who showed that ladder fuels from 1 to 4 m measured by ALS were a significant predictor of post-fire canopy damage, along with other with additional topographic variables (R2 = 0.63 for non-wind driven fires; R2 = 0.56 for wind-driven fires). Our models that included TLS- and ALS-based data had the highest predictive power, followed by the model using HMLS data. TLS provides very detailed data of forest structure beyond 8 m (Figure 2; Disney, 2019), and thus was expected to be a useful approach for collecting data to predict RdNBR.
Models using ladder fuel densities collected from HMLS and UAS-SfM approaches were not as useful to predict RdNBR. While Bauwens et al. (2016) found HMLS outperformed TLS when estimating forest inventory metrics, HMLS is less reliable for density metrics, as point density varies based on length of scan and the walking path taken by the user. Also, we observed nearly complete loss of information beyond 8 m height with HMLS. Although the RdNBR UAS-SfM model did not perform as well as TLS or ALS, it had an R2 from 0.50 to 0.53, slightly better than HMLS (although the UAS-SfM model had fewer total plots, as previously mentioned). We thus find that there is some value in UAS-SfM, particularly in forests with gaps in the canopy or relatively low CBH, such as oak woodlands or managed stands, where some lower-canopy points are detected in the SfM process. In addition, our previous research found multispectral UAS-SfM to be useful for monitoring changes in upper-canopy structure and greenness after wildfires (Reilly et al., 2021). We also note that multi-angle views can improve detection of sub-canopy structure with UAS-SfM (Lamping et al., 2021), a factor that we could not explore as we were constrained to a nadir view by our UAS and sensor equipment.
Importantly, the strength of ladder fuel densities to predict RdNBR were stronger in all analyses when CBH was included. This finding agrees with those of Fernández-Guisuraga et al. (2021) who found that severe ecosystem damage was mainly driven by vegetation structure rather than topography or patch size, with different roles of pre-fire fuel structure parameters. Many studies have accurately estimated CBH from ALS data (Andersen et al., 2005; Kelly et al., 2017; Luo et al., 2018; Moran et al., 2020; Stefanidou et al., 2020; Chamberlain et al., 2021), and a few studies have estimated CBH with TLS data (García et al., 2011; Novotny et al., 2021), so ideally these forest structure variables could be estimated via remote sensing instead of a field-based approach, to maintain a continuity in data collection.
In the future, additional forest structure variables which have been shown to be important in predicting burn severity in our ecosystem (i.e., canopy height, spatial context of surrounding vegetation types, and topography), could be included into a predictive model (Green et al., 2020). Further, climate variables, especially annual mean vapor-pressure deficit, wind speed, and burning index (Chen et al., 2021), as well as those related to weather and fire propagation, could also help inform future models as they may exert dominant control over burn severity in relation to topography (Viedma et al., 2015; García-Llamas et al., 2020), particularly under extreme climatic conditions (Turner and Romme, 1994). With a more robust model that includes these additional forest structure, topographic and climate variables collected over more plots, the role of ladder fuels can be further assessed and extrapolated to broader spatial scales which has significant applications to scaling fire risk.
Conclusion
Remote sensing methods allow for opportunities to discover new pathways to estimate forest fuels and predict wildfire severity. Our study illustrated the utility of diverse remote sensing techniques to measure ladder fuel density, and results indicated that land managers who do not have access to all of these approaches can use different approaches interchangeably across the same spatial extents. Our results also stress the importance of forest canopy structure to compare ladder fuels across methods or predict burn severity and suggest that methods for ladder fuel estimation should include canopy structure to account for differences in measurement techniques. We expect that these remote sensing approaches at the plot-level will contribute to future research to incorporate ladder fuel density into predictive models of wildfire severity and fire behavior at varying spatial scales.
Data Availability Statement
The datasets presented in this study can be found in online repositories. The names of the repository/repositories and accession number(s) can be found below: https://doi.org/10.2737/RDS-2021-0101, FS Data Research Data Archive.
Author Contributions
BF: conceptualization, data curation, formal analysis, funding acquisition, investigation, methodology, resources, software, supervision, visualization, writing—original draft, and writing—review and editing. SR: conceptualization, data curation, formal analysis, funding acquisition, investigation, methodology, software, visualization, and writing—review and editing. MC: conceptualization, data curation, formal analysis, funding acquisition, investigation, methodology, resources, software, supervision, validation, and writing—review and editing. RF: data curation, investigation and methodology. AK: data curation, investigation, software, and writing—review and editing. PK: data curation, investigation, and methodology. CM, MO’N, and MV: investigation. MD and PW: funding acquisition, investigation, and writing—review and editing. LPB: conceptualization, data curation, funding acquisition, investigation, methodology, project administration, resources, supervision, validation, visualization, writing—original draft, and writing—review and editing. All authors contributed to the article and approved the submitted version.
Funding
This research was funded by the CAL FIRE Forest Health and Forest Legacy Program (8GG18806) to LPB and MC, California State University Agricultural Research Institute (20-01-106) to LPB and MC, and a Joint Fire Science Program Graduate Research Innovation Grant (20-1-01-20) to BF and LPB. MD received capital funding from NERC via UCL Geography and NCEO. SR was funded by the Rhodes Trust and through the University of Oxford Environmental Change Institute Small Grant Scheme.
Conflict of Interest
The authors declare that the research was conducted in the absence of any commercial or financial relationships that could be construed as a potential conflict of interest.
Publisher’s Note
All claims expressed in this article are solely those of the authors and do not necessarily represent those of their affiliated organizations, or those of the publisher, the editors and the reviewers. Any product that may be evaluated in this article, or claim that may be made by its manufacturer, is not guaranteed or endorsed by the publisher.
Acknowledgments
We thank students in SSU’s Research Experience in Biology class from Fall 2020: Christopher Aguilar, Eloisa Burke, Stef Delgado, Mia Furman, Angeline Gorenflo, Shannon Manhoso, Frida Pereira, Rosa Ramirez and undergraduate researchers Sarah Arroyo-Chavez, Jolene Markarian, and Lauren Webster for their analysis of photo banner data. We are grateful to Daniel Crocker for his assistance with statistical analyses and to Tadashi Moody and Sean Place for their thoughtful comments on the manuscript. We appreciate the hard work of the 3DForests field crew for data collection including Elise Piazza and Catherine Seel. We additionally thank the Ag and Open Space District, Pepperwood Preserve, and Dr. David Ackerly for granting us access to their sites and facilitating our research data collection.
Supplementary Material
The Supplementary Material for this article can be found online at: https://www.frontiersin.org/articles/10.3389/ffgc.2022.818713/full#supplementary-material
Footnotes
References
Ackerly, D. D., Kling, M. M., Clark, M. L., Papper, P., Oldfather, M. F., Flint, A. L., et al. (2020). Topoclimates, Refugia, and Biotic Responses to Climate Change. Front. Ecol. Environ. 18, 288–297. doi: 10.1002/fee.2204
Ackerly, D. D., Oldfather, M. F., Britton, M., Halbur, M., and Micheli, L. (2013). Establishment of Woodland Vegetation Research Plots at Pepperwood Preserve. Palo Alto, CA: The Moore Foundation, doi: 10.6084/m9.figshare.1122453.v1
Agee, J. K., and Skinner, C. N. (2005). Basic Principles of Forest Fuel Reduction Treatments. Forest Ecol. Manag. 211, 83–96. doi: 10.1016/j.foreco.2005.01.034
Almeida, D. R. A., Stark, S. C., Chazdon, R., Nelson, B. W., Cesar, R. G., Meli, P., et al. (2019). The Effectiveness of Lidar Remote Sensing for Monitoring Forest Cover Attributes and Landscape Restoration. Forest Ecol. Manag. 438, 34–43. doi: 10.1016/j.foreco.2019.02.002
Andersen, H.-E., McGaughey, R. J., and Reutebuch, S. E. (2005). Estimating Forest Canopy Fuel Parameters using LIDAR Data. Remote Sens. Environ. 94, 441–449. doi: 10.1016/j.rse.2004.10.013
Atchley, A. L., Linn, R., Jonko, A., Hoffman, C., Hyman, J. D., Pimont, F., et al. (2021). Effects of fuel spatial distribution on wildland fire behaviour. Int. J. Wildland Fire 30, 179–189. doi: 10.1071/WF20096
Balch, J., Schoennagel, T., Williams, A., Abatzoglou, J., Cattau, M., Mietkiewicz, N., et al. (2018). Switching on the Big Burn of 2017. Fire 1:17. doi: 10.3390/fire1010017
Bauwens, S., Bartholomeus, H., Calders, K., and Lejeune, P. (2016). Forest Inventory with Terrestrial LiDAR: A Comparison of Static and Hand-Held Mobile Laser Scanning. Forests 7:127. doi: 10.3390/f7060127
Brede, B., Calders, K., Lau, A., Raumonen, P., Bartholomeus, H. M., Herold, M., et al. (2019). Non-Destructive Tree Volume Estimation through Quantitative Structure Modelling: Comparing UAV Laser Scanning with Terrestrial LIDAR. Remote Sens. Environ. 233:111355. doi: 10.1016/j.rse.2019.111355
Brown, J. K. (1982). Handbook for Inventorying Surface Fuels and Biomass in the Interior West. Ogden, US: US Department of Agriculture, 129.
California Forest Observatory (2020). A Statewide Tree-Level Forest Monitoring System. San Francisco, CA: Salo Sciences, Inc.
Campbell, M. J., Dennison, P. E., Tune, J. W., Kannenberg, S. A., Kerr, K. L., Codding, B. F., et al. (2020). A Multi-Sensor, Multi-Scale Approach to Mapping Tree Mortality in Woodland Ecosystems. Remote Sens. Environ. 245:111853. doi: 10.1016/j.rse.2020.111853
Chamberlain, C. P., Sánchez Meador, A. J., and Thode, A. E. (2021). Airborne lidar provides reliable estimates of canopy base height and canopy bulk density in southwestern ponderosa pine forests. Forest Ecol. Manag. 481:118695. doi: 10.1016/j.foreco.2020.118695
Chen, B., Jin, Y., Scaduto, E., Moritz, M. A., Goulden, M. L., and Randerson, J. T. (2021). Climate, Fuel, and Land Use Shaped the Spatial Pattern of Wildfire in California’s Sierra Nevada. J. Geophys. Res. Biogeosci. 126:e2020JG005786. doi: 10.1029/2020JG005786
Chen, Y., Zhu, X., Yebra, M., Harris, S., and Tapper, N. (2016). Strata-Based Forest Fuel Classification for Wild Fire Hazard Assessment using Terrestrial LiDAR. J. Appl. Remote Sens. 10:046025. doi: 10.1117/1.JRS.10.046025
Chudá, J., Hunčaga, M., Tuček, J., and Mokroš, M. (2020). The Handheld Mobile Laser Scanners as a Tool for Accurate Positioning Under Forest Canopy. Int. Arch. Photogramm. Remote Sens. Spatial Inf. Sci. 2020, 211–218. doi: 10.5194/isprs-archives-XLIII-B1-2020-211-2020
Coppoletta, M., Merriam, K. E., and Collins, B. M. (2016). Post-fire vegetation and fuel development influences fire severity patterns in reburns. Ecol. Appl. 26, 686–699. doi: 10.1890/15-0225
Cruz, M. G., Alexander, M. E., and Wakimoto, R. H. (2003). Assessing canopy fuel stratum characteristics in crown fire prone fuel types of western North America. Int. J. Wildland Fire 12:39. doi: 10.1071/WF02024
Dennison, P. E., Brewer, S. C., Arnold, J. D., and Moritz, M. A. (2014). Large Wildfire Trends in the Western United States, 1984-2011. Geophys. Res. Lett. 41, 2928–2933. doi: 10.1002/2014GL059576
Disney, M. (2019). Terrestrial LiDAR: A Three-Dimensional Revolution in How We Look at Trees. N. Phytol. 222, 1736–1741. doi: 10.1111/nph.15517
Donager, J. J., Meador, A. J. S., and Blackburn, R. C. (2021). Adjudicating Perspectives on Forest Structure: How Do Airborne, Terrestrial, and Mobile Lidar-Derived Estimates Compare? Remote Sens. 13:2297. doi: 10.3390/rs13122297
Duff, T. J., Bell, T. L., and York, A. (2013). Predicting Continuous Variation in Forest Fuel Load using Biophysical Models: A Case Study in South-Eastern Australia. Int. J. Wildland Fire 22, 318–332. doi: 10.1071/WF11087
Duff, T., Cawson, J., and Penman, T. D. (2019). Determining Burnability: Predicting Completion Rates and Coverage of Prescribed Burns for Fuel Management. For. Ecol. Manage. 433, 431–440. doi: 10.1016/j.foreco.2018.11.009
Evett, R. R., Dawson, A., and Bartolome, J. W. (2013). Estimating Vegetation Reference Conditions by Combining Historical Source Analysis and Soil Phytolith Analysis at Pepperwood Preserve, Northern California Coast Ranges, U.S.A: Estimating Vegetation Reference Conditions. Restor. Ecol. 21, 464–473. doi: 10.1111/j.1526-100X.2012.00912.x
Fernández-Guisuraga, J. M., Suárez-Seoane, S., García-Llamas, P., and Calvo, L. (2021). Vegetation Structure Parameters Determine High Burn Severity Likelihood in Different Ecosystem Types: A Case Study in a Burned Mediterranean Landscape. J. Environ. Manag. 288:112462. doi: 10.1016/j.jenvman.2021.112462
Gale, M. G., Cary, G. J., Van Dijk, A. I. J. M., and Yebra, M. (2021). Forest Fire Fuel Through the Lens of Remote Sensing: Review of Approaches, Challenges and Future Directions in the Remote Sensing of Biotic Determinants of Fire Behaviour. Remote Sens. Environ. 255:112282. doi: 10.1016/j.rse.2020.112282
García, M., Danson, F. M., Riaño, D., Chuvieco, E., Ramirez, F. A., and Bandugula, V. (2011). Terrestrial Laser Scanning to Estimate Plot-Level Forest Canopy Fuel Properties. Int. J. Appl. Earth Observ. Geoinform. 13, 636–645. doi: 10.1016/j.jag.2011.03.006
García-Llamas, P., Suárez-Seoane, S., Fernández-Manso, A., Quintano, C., and Calvo, L. (2020). Evaluation of Fire Severity in Fire Prone-Ecosystems of Spain under Two Different Environmental Conditions. J. Environ. Manage. 271:110706. doi: 10.1016/j.jenvman.2020.110706
Gonzalez, de Tanago, J., Lau, A., Bartholomeus, H., Herold, M., Avitabile, V., et al. (2018). Estimation of Above-Ground Biomass of Large Tropical Trees with Terrestrial LiDAR. Methods Ecol. Evol. 9, 223–234. doi: 10.1111/2041-210X.12904
González-Ferreiro, E., Arellano-Pérez, S., Castedo-Dorado, F., Hevia, A., Vega, J. A., Vega-Nieva, D., et al. (2017). Modelling the Vertical Distribution of Canopy Fuel Load using National Forest Inventory and Low-Density Airbone Laser Scanning Data. PLoS One 12:e0176114. doi: 10.1371/journal.pone.0176114
Graham, A., Coops, N., Wilcox, M., and Plowright, A. (2019). Evaluation of Ground Surface Models Derived from Unmanned Aerial Systems with Digital Aerial Photogrammetry in a Disturbed Conifer Forest. Remote Sens. 11:84. doi: 10.3390/rs11010084
Green, K., Tukman, M., Loudon, D., Gaffney, K., and Clark, M. (2020). Sonoma County Complex Fires of 2017: Remote Sensing Data and Modeling to Support Ecosystem and Community Resiliency. California, CA: California Fish and Wildlife, 14–45.
Gupta, V., Reinke, K., Jones, S., Wallace, L., and Holden, L. (2015). Assessing Metrics for Estimating Fire Induced Change in the Forest Understorey Structure Using Terrestrial Laser Scanning. Remote Sens. 7, 8180–8201. doi: 10.3390/rs70608180
Hernandez, M. (2020). Interactions Among Sudden Oak Death, Fire Fuel Loads, and Climate in Sonoma County Oak Woodlands. Ph.D thesis, Sonoma CA: Sonoma State University.
Hijmans, R. J., van Etten, J., Sumner, M., Cheng, J., Bevan, A., Bivand, R., et al. (2019) Raster: Geographic Data Analysis and Modeling, Vienna: R Foundation for Statistical Computing.
Hillman, S., Wallace, L., Reinke, K., Hally, B., Jones, S., and Saldias, D. S. (2019). A method for validating the structural completeness of understory vegetation models captured with 3D remote sensing. Remote Sens. 11:2118. doi: 10.3390/rs11182118
Hillman, S., Wallace, L., Reinke, K., and Jones, S. (2021a). A Comparison between TLS and UAS LiDAR to Represent Eucalypt Crown Fuel Characteristics. ISPRS J. Photogr. Remote Sens. 181, 295–307. doi: 10.1016/j.isprsjprs.2021.09.008
Hillman, S., Wallace, L., Lucieer, A., Reinke, K., Turner, D., and Jones, S. (2021b). A Comparison of Terrestrial and UAS Sensors for Measuring Fuel Hazard in a Dry Sclerophyll Forest. Int. J. Appl. Earth Observ. Geoinform. 95:102261. doi: 10.1016/j.jag.2020.102261
Hyyppä, E., Yu, X., Kaartinen, H., Hakala, T., Kukko, A., Vastaranta, M., et al. (2020). Comparison of Backpack, Handheld, Under-Canopy UAV, and Above-Canopy UAV Laser Scanning for Field Reference Data Collection in Boreal Forests. Remote Sens. 12:3327. doi: 10.3390/rs12203327
Jakubowski, M. K., Guo, Q., and Kelly, M. (2013). Tradeoffs between lidar pulse density and forest measurement accuracy. Remote Sens. Environ. 130, 245–253. doi: 10.1016/j.rse.2012.11.024
Joyce, K. E., Anderson, K., and Bartolo, R. E. (2021). Of Course We Fly Unmanned—We’re Women!. Drones 5:21. doi: 10.3390/drones5010021
Keane, R. E., Gray, K., Bacciu, V., and Leirfallom, S. (2012). Spatial scaling of wildland fuels for six forest and rangeland ecosystems of the northern Rocky Mountains, USA. Landsc. Ecol. 27, 1213–1234. doi: 10.1007/s10980-012-9773-9
Keane, R. E., Reinhardt, E. D., Scott, J., Gray, K., and Reardon, J. (2005). Estimating forest canopy bulk density using six indirect methods. Can. J. For. Res. 35, 724–739. doi: 10.1139/x04-213
Keeley, J. E. (2009). Fire intensity, fire severity and burn severity: a brief review and suggested usage. Int. J. Wildland Fire 18:116. doi: 10.1071/WF07049
Kelly, M., and Di Tommaso, S. (2015). Mapping Forests with Lidar Provides Flexible, Accurate Data with Many Uses. Cal Ag. 69, 14–20. doi: 10.3733/ca.v069n01p14
Kelly, M., Su, Y., Di Tommaso, S., Fry, D., Collins, B., Stephens, S., et al. (2017). Impact of Error in Lidar-Derived Canopy Height and Canopy Base Height on Modeled Wildfire Behavior in the Sierra Nevada, California, USA. Remote Sens. 10:10. doi: 10.3390/rs10010010
Kramer, H., Collins, B., Lake, F., Jakubowski, M., Stephens, S., and Kelly, M. (2016). Estimating Ladder Fuels: A New Approach Combining Field Photography with LiDAR. Remote Sens. 8:766. doi: 10.3390/rs8090766
Lamping, J. E., Zald, H. S. J., Madurapperuma, B. D., and Graham, J. (2021). Comparison of Low-Cost Commercial Unpiloted Digital Aerial Photogrammetry to Airborne Laser Scanning across Multiple Forest Types in California. USA. Remote Sens. 13:4292. doi: 10.3390/rs13214292
Lau, A., Martius, C., Bartholomeus, H., Shenkin, A., Jackson, T., Malhi, Y., et al. (2019). Estimating Architecture-Based Metabolic Scaling Exponents of Tropical Trees using Terrestrial LiDAR and 3D Modelling. Forest Ecol. Manage. 439, 132–145. doi: 10.1016/j.foreco.2019.02.019
Leite, R. V., Silva, C. A., Broadbent, E. N., Do Amaral, C. H., Liesenberg, V., De Almeida, D. R. A., et al. (2022). Large scale multi-layer fuel load characterization in tropical savanna using GEDI spaceborne lidar data. Remote Sens. Environ. 268:112764. doi: 10.1016/j.rse.2021.112764
Levick, S. R., Whiteside, T., Loewensteiner, D. A., Rudge, M., and Bartolo, R. (2021). Leveraging TLS as a Calibration and Validation Tool for MLS and ULS Mapping of Savanna Structure and Biomass at Landscape-Scales. Remote Sens. 13:257. doi: 10.3390/rs13020257
Luo, L., Zhai, Q., Su, Y., Ma, Q., Kelly, M., and Guo, Q. (2018). Simple Method for Direct Crown Base Height Estimation of Individual Conifer Trees using Airborne LiDAR Data. Opt. Exp. 26, A562–A578. doi: 10.1364/OE.26.00A562
Mallek, C., Safford, H., Viers, J., and Miller, J. (2013). Modern departures in fire severity and area vary by forest type, Sierra Nevada and southern Cascades, California, USA. Ecosphere 4:12. doi: 10.1890/ES13-00217.1
Marselis, S. M., Yebra, M., Jovanovic, T., and van Dijk, A. I. J. M. (2016). Deriving Comprehensive Forest structure Information from Mobile Laser Scanning Observations Using Automated Point Cloud Classification. Environ. Model. Softw. 82, 142–151. doi: 10.1016/j.envsoft.2016.04.025
Menning, K. M., and Stephens, S. L. (2007). Fire Climbing in the Forest: A Semiqualitative, Semiquantitative Approach to Assessing Ladder Fuel Hazards. West. J. Appl. Forestry 22, 88–93. doi: 10.1093/wjaf/22.2.88
Miller, J. D., Knapp, E. E., Key, C. H., Skinner, C. N., Isbell, C. J., Creasy, R. M., et al. (2009). Calibration and Validation of the Relative Differenced Normalized Burn Ratio (RdNBR) to Three Measures of Fire Severity in the Sierra Nevada and Klamath Mountains, California, USA. Remote Sens. Environ. 113, 645–656. doi: 10.1016/j.rse.2008.11.009
Miller, J. D., and Thode, A. E. (2007). Quantifying Burn Severity in a Heterogeneous Landscape with a Relative Version of the Delta Normalized Burn Ratio (dNBR). Remote Sens. Environ. 109, 66–80. doi: 10.1016/j.rse.2006.12.006
Moran, C. J., Kane, V. R., and Seielstad, C. A. (2020). Mapping Forest Canopy Fuels in the Western United States with LiDAR–Landsat Covariance. Remote Sens. 12:1000. doi: 10.3390/rs12061000
Nigro, K., and Molinari, N. (2019). Status and trends of fire activity in southern California yellow pine and mixed conifer forests. Forest Ecol. Manag. 441, 20–31. doi: 10.1016/j.foreco.2019.01.020
Novotny, J., Navratilova, B., Albert, J., Cienciala, E., Fajmon, L., and Brovkina, O. (2021). Comparison of Spruce and Beech Tree Attributes from Field Data, Airborne and Terrestrial Laser Scanning using Manual and Automatic Methods. Remote Sens. Appl. 23:100574. doi: 10.1016/j.rsase.2021.100574
Ottmar, R. D., Sandberg, D. V., Riccardi, C. L., and Prichard, S. J. (2007). An Overview of The Fuel Characteristic Classification System—Quantifying, Classifying, and Creating Fuelbeds for Resource Planning. Can. J. For. Res. 37, 2383–2393. doi: 10.1139/x07-077
Prichard, S. J., Sandberg, D. V., Ottmar, R. D., Eberhardt, E., Andreu, A., Eagle, P., et al. (2013). Fuel Characteristic Classification System Version 3.0. doi: 10.2737/PNW-GTR-887
Puliti, S., Dash, J. P., Watt, M. S., Breidenbach, J., and Pearse, G. D. (2020). A comparison of UAV Laser Scanning, Photogrammetry and Airborne Laser Scanning for Precision Inventory of Small-Forest Properties. Forestry 93, 150–162. doi: 10.1093/forestry/cpz057
R Core Team (2020). R: A Language and Environment for Statistical Computing. Vienna: R Foundation for Statistical Computing.
Reilly, S., Clark, M. L., Bentley, L. P., Matley, C., Piazza, E., and Oliveras Menor, I. (2021). The Potential of Multispectral Imagery and 3D Point Clouds from Unoccupied Aerial Systems (UAS) for Monitoring Forest Structure and the Impacts of Wildfire in Mediterranean-Climate Forests. Remote Sens. 13:3810. doi: 10.3390/rs13193810
Ritchie, M. W., Skinner, C. N., and Hamilton, T. A. (2007). Probability of Tree Survival After Wildfire in an Interior Pine Forest of Northern California: Effects of Thinning and Prescribed Fire. Forest Ecol. Manage. 247, 200–208. doi: 10.1016/j.foreco.2007.04.044
Roussel, J.-R., Auty, D., Coops, N. C., Tompalski, P., Goodbody, T. R. H., Meador, A. S., et al. (2020). lidR: An R package for analysis of Airborne Laser Scanning (ALS) data. Remote Sens. Environ. 251:112061. doi: 10.1016/j.rse.2020.112061
Rowell, E., Loudermilk, E. L., Hawley, C., Pokswinski, S., Seielstad, C., and Queen, et al. (2020). Coupling Terrestrial Laser Scanning with 3D Fuel Biomass Sampling for Advancing Wildland Fuels Characterization. Forest Ecol. Manage. 462:117945. doi: 10.1016/j.foreco.2020.117945
Ryding, J., Williams, E., Smith, M., and Eichhorn, M. (2015). Assessing Handheld Mobile Laser Scanners for Forest Surveys. Remote Sens. 7, 1095–1111. doi: 10.3390/rs70101095
Safford, H. D., Stevens, J. T., Merriam, K., Meyer, M. D., and Latimer, A. M. (2012). Fuel Treatment Effectiveness in California Yellow Pine and Mixed Conifer Forests. Forest Ecol. Manage. 274, 17–28. doi: 10.1016/j.foreco.2012.02.013
Sando, R. W., and Wick, C. H. (1972). “A method of evaluating crown fuels in forest stands,” in Research Paper NC-84. (St. Paul, MN: U.S. Dept. of Agriculture). doi: 10.1890/07-1747.1
Schmidt, D. A., Taylor, A. H., and Skinner, C. N. (2008). The influence of fuels treatment and landscape arrangement on simulated fire behavior, Southern Cascade range, California. Forest Ecol. Manage. 255, 3170–3184. doi: 10.1016/j.foreco.2008.01.023
Scott, J. H., and Reinhardt, E. D. (2001). “Assessing crown fire potential by linking models of surface and crown fire behaviour,” in USDA Forest Service Res Pap RMRS-RP-29. (Fort Collins, CO: U.S. Department of Agriculture).
Shin, P., Sankey, T., Moore, M. M., and Thode, A. E. (2018). Evaluating Unmanned Aerial Vehicle Images for Estimating Forest Canopy Fuels in a Ponderosa Pine Stand. Remote Sens. 10:1266. doi: 10.3390/rs10081266
Singh, J., Levick, S. R., Guderle, M., Schmullius, C., and Trumbore, S. E. (2018). Variability in Fire-Induced Change to Vegetation Physiognomy and Biomass in Semi-Arid Savanna. Ecosphere 9:e02514. doi: 10.1002/ecs2.2514
Skowronski, N., Clark, K., Nelson, R., Hom, J., and Patterson, M. (2007). Remotely Sensed Measurements of Forest Structure and Fuel Loads in the Pinelands of New Jersey. Remote Sens. Environ. 108, 123–129. doi: 10.1016/j.rse.2006.09.032
Skowronski, N. S., Clark, K. L., Duveneck, M., and Hom, J. (2011). Three-dimensional canopy fuel loading predicted using upward and downward sensing LiDAR systems. Remote Sens. Environ. 115, 703–714. doi: 10.1016/j.rse.2010.10.012
Soma, M., Pimont, F., and Dupuy, J. L. (2021). Sensitivity of Voxel-Based Estimations of Leaf Area Density with Terrestrial LiDAR to Vegetation Structure and Sampling Limitations: A Simulation Experiment. Remote Sens. Environ. 257:112354. doi: 10.1016/j.rse.2021.112354
Stefanidou, A., Gitas, I. Z., Korhonen, L., Stavrakoudis, D., and Georgopoulos, N. (2020). LiDAR-Based Estimates of Canopy Base Height for a Dense Uneven-Aged Structured Forest. Remote Sens. 12:1565. doi: 10.3390/rs12101565
Stephens, S. L., McIver, J. D., Boerner, R. E. J., Fettig, C. J., Fontaine, J. B., Hartsough, B. R., et al. (2012). The Effects of Forest Fuel-Reduction Treatments in The United States. Bioscience 62, 549–560. doi: 10.1525/bio.2012.62.6.6
Stevens, J. T., Collins, B. M., Miller, J. D., North, M. P., and Stephens, S. L. (2017). Changing spatial patterns of stand-replacing fire in California conifer forests. Forest Ecol. Manag. 406, 28–36. doi: 10.1016/j.foreco.2017.08.051
Turner, M. G., and Romme, W. H. (1994). Landscape Dynamics in Crown Fire Ecosystems. Landsc. Ecol. 9, 59–77. doi: 10.1007/BF00135079
Van Wagner, C. V. (1977). Conditions for the start and spread of crown fire. Can. J. For. Res. 7, 23–34. doi: 10.1139/x77-004
Viedma, O., Quesada, J., Torres, I., De Santis, A., and Moreno, J. M. (2015). Fire Severity in a Large Fire in a Pinus pinaster Forest is Highly Predictable from Burning Conditions, Stand Structure, and Topography. Ecosystems 18, 237–250. doi: 10.1007/s10021-014-9824-y
Wallace, L., Gupta, V., Reinke, K., and Jones, S. (2016). An Assessment of Pre- and Post Fire Near Surface Fuel Hazard in an Australian Dry Sclerophyll Forest Using Point Cloud Data Captured Using a Terrestrial Laser Scanner. Remote Sens. 8:679. doi: 10.3390/rs8080679
Watershed Sciences (2016). Sonoma County Vegetation Mapping and Lidar Program. [Technical Report]. Available online at: https://sonomaopenspace.egnyte.com/dl/8DukhuIXgT/ [accessed on Jun 20, 2021].
Wilkes, P., Lau, A., Disney, M., Calders, K., Burt, A., and Gonzalez, et al. (2017). Data Acquisition Considerations for Terrestrial Laser Scanning of Forest Plots. Remote Sens. Environ. 196, 140–153. doi: 10.1016/j.rse.2017.04.030
Keywords: ladder fuels, terrestrial laser scanner (TLS), handheld-mobile laser scanner (HMLS), unoccupied aerial system (UAS), airborne laser scanner (ALS), Structure from Motion (SfM), wildfire burn severity
Citation: Forbes B, Reilly S, Clark M, Ferrell R, Kelly A, Krause P, Matley C, O’Neil M, Villasenor M, Disney M, Wilkes P and Bentley LP (2022) Comparing Remote Sensing and Field-Based Approaches to Estimate Ladder Fuels and Predict Wildfire Burn Severity. Front. For. Glob. Change 5:818713. doi: 10.3389/ffgc.2022.818713
Received: 19 November 2021; Accepted: 28 February 2022;
Published: 06 April 2022.
Edited by:
Olga Viedma Sillero, University of Castilla La Mancha, SpainReviewed by:
Leonor Calvo Galvan, Universidad de León, SpainFernando Castedo Dorado, Universidad de León, Spain
Copyright © 2022 Forbes, Reilly, Clark, Ferrell, Kelly, Krause, Matley, O’Neil, Villasenor, Disney, Wilkes and Bentley. This is an open-access article distributed under the terms of the Creative Commons Attribution License (CC BY). The use, distribution or reproduction in other forums is permitted, provided the original author(s) and the copyright owner(s) are credited and that the original publication in this journal is cited, in accordance with accepted academic practice. No use, distribution or reproduction is permitted which does not comply with these terms.
*Correspondence: Brieanne Forbes, YnJpZWFubmUuay5mb3JiZXNAZ21haWwuY29t