- 1U.S. Department of Agriculture-Forest Service, Pacific Southwest Research Station, Placerville, CA, United States
- 2School of Environmental and Forest Sciences, University of Washington, Seattle, WA, United States
- 3U.S. Department of Agriculture-Forest Service, Pacific Northwest Research Station, Wenatchee, WA, United States
- 4Oak Ridge Institute for Science and Education, Oak Ridge Associated Universities, Oak Ridge, TN, United States
- 5School of Civil and Environmental Engineering, University of Washington, Seattle, WA, United States
- 6Pacific Northwest National Laboratory, Richland, WA, United States
- 7Resilient Forestry, LLC, Seattle, WA, United States
Active forest management is applied in many parts of the western United States to reduce wildfire severity, mitigate vulnerability to drought and bark beetle mortality, and more recently, to increase snow retention and late-season streamflow. A rapidly warming climate accelerates the need for these restorative treatments, but the treatment priority among forest patches varies considerably. We simulated four treatment scenarios across the 3,450 km2 Wenatchee River basin in eastern Washington, United States. We used a decision support tool (DST) to assess trade-offs and synergies within and among treatments on wildfire risk and smoke emissions, water yield and snow retention, biomass production, and economic return. Treatment scenarios emphasized prescribed burning (BurnOnly), biomass production (MaxBiomass), gap-based thinning to optimize water yield (IdealWater), and a principle-based restoration scenario (RA1). Fire hazard, smoke emissions, and biomass production metrics were evaluated across scenarios using the Forest Vegetation Simulator, and water yields were modeled using the Distributed Hydrology Soil Vegetation Model. Simulations were summarized to both patch- (101–102 ha) and subwatershed- (103–104 ha) scales, and treatment effects were evaluated against an untreated baseline landscape. We used logic models to rank effect sizes by scenario across metrics along a continuum between −1 (no or weak effect) to +1 (large effect). All treatments produced benefits across one or more ecosystem services and led to synergistic benefits to water yield and wildfire hazard reduction. Tradeoffs among resource benefits were clear in wilderness where reliance on prescribed burning without mechanical treatment increased costs and eliminated the potential for biomass recovery. The BurnOnly scenario improved fire risk metrics and streamflow, but effect sizes were lower compared to other treatments. IdealWater showed the strongest benefits overall, demonstrating the ability to capture multiple resource benefits through spatially explicit thinning. Our study provides a framework for integrating strategic and tactical models that evaluate tradeoffs and synergies gained through varied management approaches. We demonstrate the utility of decision support modeling to enhance management synergies across large landscapes.
Introduction
The Challenge of Modern Land Management
Across much of the western United States (wUS), the resilience of social and ecological systems continues to be challenged by a warming climate and altered disturbance regimes (Hessburg et al., 2019). While many natural systems in this domain evolved with recurrent wildfire, insect, and pathogen disturbances, the last two decades have seen a dramatic rise in the size and severity of disturbances, with some landscapes threatened by non-recovery (Coop et al., 2020). Both empirical and theoretical research shows that many forests may be approaching ecological tipping points (Allen et al., 2015; Millar and Stephenson, 2015), highlighting the need to triage planning and land management at large spatial scales. Adaptive management approaches provide a practical method for directing forest patches toward more resilient conditions (Agee and Skinner, 2005; Schultz et al., 2012; Prichard et al., 2021), but there remains a need for tools to integrate strategic and tactical planning models across multiple scales and multiple resource benefits.
Much evidence exists for the effectiveness of restoration and fuel reduction treatments in reducing surface and canopy fuel loads, raising canopy base heights, mitigating fire severity, and slowing the spread of fire (Prichard et al., 2021). Treatments include thinning, prescribed burning, and use of managed wildfires to move forests toward conditions that are both climate change- and wildfire-adapted (Gaines et al., 2012; Hessburg et al., 2013, 2021; Furniss et al., 2022) or that are more similar to historical conditions (Stephens et al., 2021a). Prichard et al. (2021), through an extensive literature review, show the strengths and limitations of fuel reduction treatments and argue that increasing the pace and scale of management is essential to reducing the potential negative effects of large-scale wildfires. However, uncertainty in the timing and location of these events poses a challenge to land managers and decision makers when planning treatments across large landscapes (Millar et al., 2007). For example, Stevens et al. (2016) found that the desired reduction in fire extent could be attained through treating only ∼30% of their study watershed, and they show that the type and configuration of treatments had significant effects on fire size, severity, and smoke production. Directing limited resources to capture treatment benefits requires strategic decision support tools (DST) to determine the amount of area to be treated and locations where restoration treatments will have the greatest impact.
A variety of DSTs have been developed for areas across the wUS to direct management actions aimed at reducing high fire hazard (Reynolds et al., 2009; Keane et al., 2010; Hessburg et al., 2013). These studies captured data on potential fire effects, behavior, and danger based on spatially explicit fuels and weather data, which were used as inputs in a DST. DSTs can ingest a wide variety of data and evaluate them on a common numerical scale to determine the level of departure or difference from desired or targeted conditions. These scores are mapped to prioritize management actions in the areas most in need of restoration. DSTs provide a transparent, flexible, data-driven, and defensible methodology for directing management to help solve complex multidimensional environmental problems.
One of the many challenges of strategic management planning relates to the diversity of socio-ecological conditions across large planning areas. By design, management often takes an “all hands and all lands” approach; working across ownerships and land allocations to achieve a common set of objectives. Often, reducing wildfire hazard is just one of many ecological benefits that can be achieved through management (Stephens et al., 2021a). Adaptation of forested landscapes to climate change and wildfire can be made tangible by considering a handful of ecological indicators including wildfire risk and smoke emissions, resistance to insects and pathogens, improved habitat conditions (Stevens et al., 2016), and enhanced hydrologic functioning (Stephens et al., 2021b). Additionally, these socio-ecological factors are central to ensuring the long-term success of management actions on public lands (Dombeck et al., 2004), where managers must balance a variety of resource benefits. DSTs are designed to evaluate management outcomes across resource benefits and to identify the degree to which they are synergistic, where a single treatment can provide for multiple benefits, or if tradeoffs exist, where benefits to one resource or condition is produced at the expense of others.
Management impacts on landscape hydrology is an increasing concern for managers throughout the wUS, given recent droughts and declining water supply (Pierce et al., 2008; Luce and Holden, 2009; Seager et al., 2013). The temperature and quantity of water in rivers and streams is a critical factor affecting salmon recovery efforts, and water in reservoirs is of considerable economic value for agriculture and electricity generation. Water availability in the wUS is largely dependent on mountain snowpack. Mid-elevation late-season snowpack levels at the boundary between snow- and rain-dominated precipitation regimes may be most sensitive to forest canopy characteristics (Dickerson-Lange et al., 2021). Managers thus have the potential to influence snowpack and hydrologic outputs but determining where and how to apply treatments remains challenging. Previous research highlights forest management impacts on snow retention (Hamlet and Lettenmaier, 1999; Lundquist et al., 2013; Saksa et al., 2017; Goeking and Tarboton, 2020), but modeling treatment effects across a landscape is complicated. Specific treatments in some locations may improve snow retention but elsewhere have neutral or negative impacts (Dickerson-Lange et al., 2021). Accurately representing these complexities requires sophisticated computer models that can integrate the temporal dynamics and physics of snow interception, albedo, snow melt, and runoff over large landscapes. A DST must then seamlessly integrate these and other model outputs to explore tradeoffs and synergies associated with simulated alternative future conditions.
A DST framework provides a data-driven, transparent, and repeatable methodology to prioritize potential forest restoration treatments across large landscapes. In the current study, we used a DST to evaluate the benefits and trade-offs between management scenarios in the Wenatchee River Basin, in central Washington State, United States. We assessed potential benefits among four key ecosystem services: snowpack and streamflow, wildfire hazard, biomass production, and economic viability. Our objective was to demonstrate the potential for DST modeling to quantitatively evaluate tradeoffs and potential synergies within and among management scenarios, and to provide guidance to land management strategies for achieving multiple resource benefits.
Materials and Methods
Analytical Framework
We used a DST designed using the Ecosystem Management Decision Support System (EMDS; Reynolds et al., 2014) to evaluate the potential benefits among four Primary topic areas: water, fire risk, biomass, and economics. We simulated three different management scenarios based on differing treatment types: prescribed fire only (“BurnOnly”), mechanical fuel reduction and biomass production (“MaxBiomass”), and gap-based thinning designed to optimize streamflow (“IdealWater”). A fourth management scenario, Principles-Based restoration (“RA1”) was an ecologically motivated restoration scenario following the principles of landscape restoration outlined by Hessburg et al. (2015). These four scenarios were compared to a baseline “No Treatment” scenario. Scenarios were implemented with the Forest Vegetation Simulator (FVS), and potential benefits to wildfire hazard, smoke emissions, biomass recovery, and economic benefits were assessed using FVS outputs. We assessed changes in streamflow using the Distributed Hydrology Soil Vegetation Model (DHSVM; Wigmosta et al., 1994) run at a spatial resolution of 90-m over ten different water years to capture variability in weather and precipitation inputs. We then used the DST to rank the potential benefits of each treatment among each topic area at pixel, patch, and subwatershed scales. The result was a collection of wall-to-wall maps that depicted the degree to which each management strategy could optimize benefits across ecosystem services provided in the Wenatchee subbasin.
Study Area
We conducted this study in the Wenatchee River subbasin (hereafter, “Wenatchee”), a 3,450 km2 landscape in central Washington State, United States (Figure 1). Climate in the Wenatchee is continental, with hot and dry summers and cold and wet winters, with a snow-dominated precipitation regime. The Wenatchee is characteristic of much of the eastern Cascades in its steep elevational gradients (ranging from 187 to 2,870 m), mixed land ownership, large wilderness areas, and fire history. Patterns of vegetation are primarily driven by gradients in temperature and precipitation, which contribute to a broad variety of ecotypes including grass- and shrub-dominated ecosystems at low elevations, montane mixed-conifer forests at mid-elevations, and subalpine vegetation and barren alpine meadows at the highest elevations (Figure 1). Further complexity in local species distributions is a function of fine- to meso-scaled variability in terrain shape, slope, and aspect contributing to high spatial variability in plant communities.
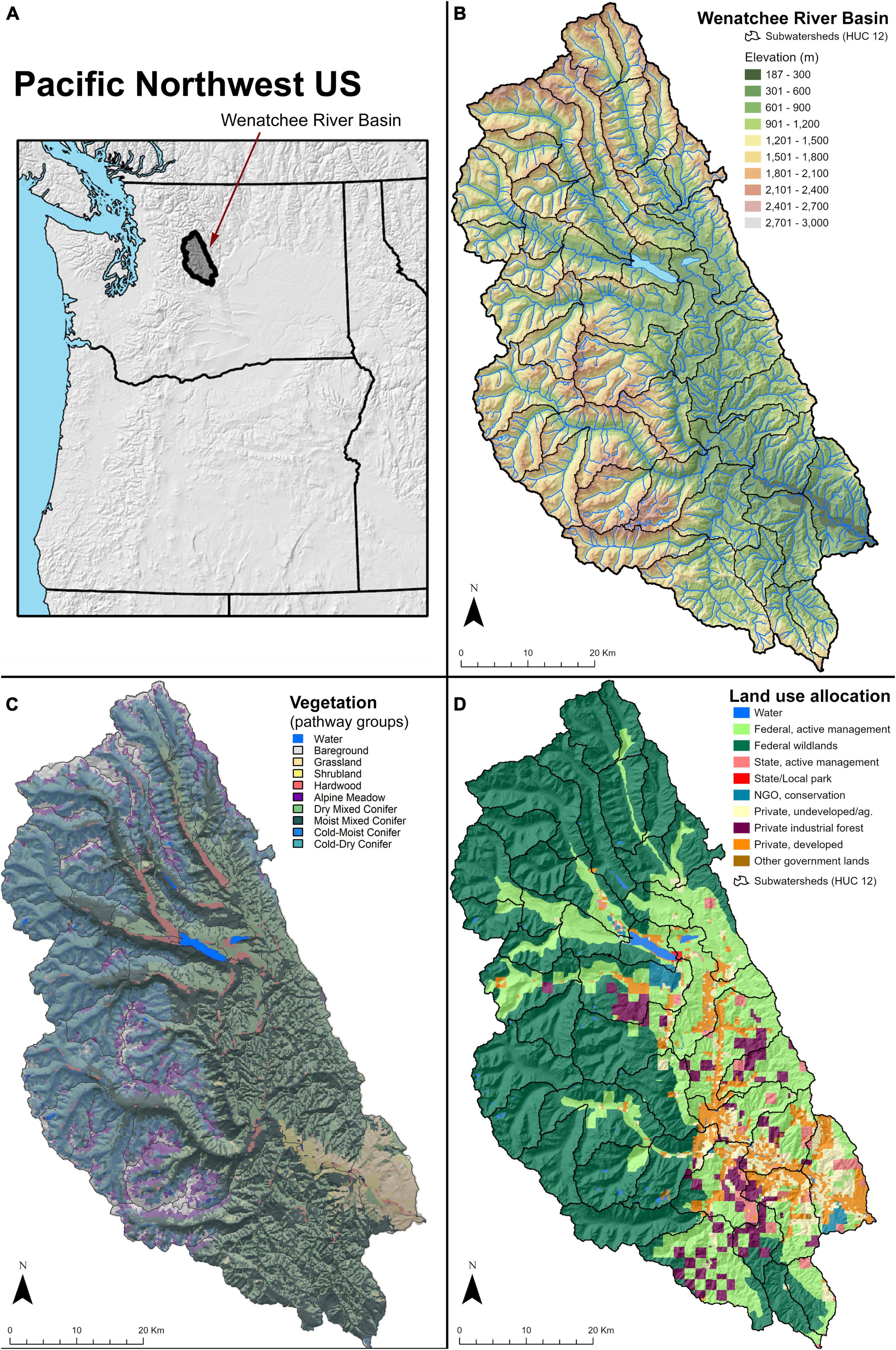
Figure 1. Location of the Wenatchee River Basin in the Cascade Mountains, north-central Washington State (A). Elevation ranges from 187 to 2,810 m (B), giving rise to diverse vegetation communities (C). Land ownership is mixed, with 56% designated as wilderness or roadless (D).
Contemporary landscapes within the Wenatchee were shaped by many centuries of antecedent fire and biological disturbances, and they continue to evolve in response to modern fires, fire exclusion by grazing and fire suppression, and timber harvest. The watershed contains both urban and rural infrastructure, and there is extensive development in the Wildland-Urban Interface (WUI). Over 80% of the land within the Wenatchee is managed by the US Forest Service, with approximately 56% of the land in designated wilderness and roadless areas. The remaining 20% of the Wenatchee is a mix of state, tribal, and private ownership. As such, wildfire risk and air quality are of great concern.
The complex terrain, vegetation, fire frequency, and land ownership patterns make the Wenatchee an interesting demonstration landscape as: (1) much of the landscape is in wilderness or roadless area, limiting opportunities for active management to prescribed burning or wildland fire use, (2) a diversity of forest types necessitates a broad range of management strategies, and (3) actively managed federal lands generally occupy mid-elevation forests, which are increasingly susceptible to early melt out, rain-on-snow events, and an intermittent snowpack, and therefore offer the greatest opportunity for management to improve snow retention and mountain hydrology.
Existing Vegetation Data Layers
We generated maps of initial vegetation for the study domain based on TreeMap, a spatially imputed forest inventory dataset developed by Riley et al. (2021). TreeMap uses machine learning to impute the nearest neighbor Forest Inventory Analysis (FIA) plot code in data space based on LANDFIRE1 topographic, biophysical, and disturbance layers. The result was a 30-m imputed raster map of FIA plots for the coterminous United States (CONUS). To generate our initial vegetation layers, we resampled this imputed map to a 90-m spatial resolution and replaced pixels that referenced FIA plots located in states outside of the Pacific Northwest using a nearest-neighbor approach. The resulting initial vegetation map was a 90-m raster containing 1860 unique FIA plot codes and represented conditions for the year 2014.
Management Patches
We developed topographically-entrained management patches using a 30-m digital elevation model (DEM) from the USGS National Elevation Data repository.2 Patches were primarily defined using a landscape topographic template as described by Hessburg et al. (2015) to spatially allocate forest restoration treatments across the study area. This template was further subdivided by hydrologic divides, land ownership, and land use allocation (LUA). Hydrologic divides were derived from nested Hydrologic Unit Codes (HUC) 10 (∼20–80,000 ha) and 12 (∼10–40,000 ha) (Seaber et al., 1987).
All raster-based input layers were resampled to 90-m resolution, and a minimum mapping unit (MMU) of 4-ha (five contiguous cells) was applied to create management treatment patches. All raw and derived input spatial layers were clipped to the study area. The spatial patches and attributes were then processed through a series of spatial intersections where the MMU was enforced at each step to best maintain the integrity of the input data.
Treatment Scenarios
We designed four treatment scenarios, which were compared to a baseline No Treatment scenario. Three of the scenarios (BurnOnly, MaxBiomass, and IdealWater) were applied to most of the landscape to better understand potential treatment effects for each treated patch across the Wenatchee. The fourth scenario (Principles Based) was an implementation of a landscape prescription that included strategic placement of burning and thinning treatments within a selection of patches across the landscape to approximate a 5- to 10-year management strategy.
We implemented treatments using the Forest Vegetation Simulator (FVS; Crookston and Dixon, 2005). Treatments were applied using an FVS application in R (R Core Team, 2021) using open-fvs.3
The four treatment scenarios were:
(1) BurnOnly: We simulated late-season controlled burns with high fuel moisture levels for large (1,000-h) fuels, and low fuel moistures for small (1-, 10-, & 100-h) fuels. Burning occurred under moderate fire weather conditions (∼80th percentile fire weather), yielding primarily low- and moderate-severity fire. Burning was applied to all patches on the landscape, including wilderness areas, but was not applied in urban areas or in non-forest environments. This resulted in treatments being applied to 73% of the raster cells within the study domain.
(2) MaxBiomass: This treatment was intended to maximize the amount of biomass removed in small- to medium-sized trees in a typical fuel reduction thinning treatment, while adhering to standards for thinning treatments on eastern Oregon and Washington National Forests (Hessburg et al., 2020, 2022). In the Hessburg et al. (2000) Interior Columbia Basin Assessment, trees <63.5 cm diameter represented the top end of the medium-sized tree size class. Accordingly, in pixels with no trees ≥63.5 cm DBH, all trees were removed. In pixels with at least one tree ≥63.5 cm DBH, all shade tolerant trees (species other than Pinus ponderosa and Larix occidentalis) <63.5 cm DBH and all trees (any species) <25.4 cm DBH were removed. No trees ≥63.5 cm DBH were ever cut. This treatment was restricted to actively managed lands (public and private) and was not applied in wilderness and roadless areas. As such, this treatment was applied to 32% of the study domain.
(3) IdealWater: This treatment optimized late-season streamflow and snow water equivalent (SWE). Thinning maximized snow accumulation by minimizing canopy interception while maintaining adequate shading for snow retention. Previous studies (e.g., Sun et al., 2018) show that small forest gaps—gap sizes proportional to mean canopy height—can enhance peak snow accumulation and duration. Following recommendations from Sun et al. (2018), this treatment created a single circular gap with a radius of 1.2 × mean canopy height within each treated 90-m pixel. The treatment was applied to the entire landscape, with the expectation that spatially explicit “thinning” could be done either mechanically (in actively managed forests) or through careful use of prescribed fire in wilderness and roadless areas. Trees were selected for treatment independent of their diameter such that large diameter trees could be removed to achieve the target gap size. This treatment was applied to 73% of the raster cells within the study domain. While its likely unrealistic that prescribed fire would reliably create the specific gap sizes intended by this treatment, we leveraged this treatment in the high elevation wilderness areas to quantify the maximum potential for treatments to optimize snow and water outputs given the crucial role these forests play in the overall water budget of the landscape.
(4) Principles-Based (RA1): The final scenario was created by mosaicking the above treatments following a principles-based approach (sensu Hessburg et al., 2015) to restore pattern and spatial complexity in a manner that would confer greater wildfire and climate resilience to landscapes. BurnOnly was applied on south-facing, flat, and ridge topographic settings in wildlands. In actively managed forest land, MaxBiomass was applied to south aspects and ridges, and IdealWater was applied to north aspects and flat areas. NoTreatment was applied to north aspects in wildlands and all valley bottoms. The resulting landscape comprised 26% non-treatable cells (water, urban, or bare ground), 38% NoTreatment, 18% BurnOnly, 14% MaxBiomass, and 4% IdealWater treatments.
Fire, Biomass, and Economics Primary Topics
We simulated all mechanical treatments and evaluated post-treatment fire effects using the FVS-Fire and Fuels Extension (FFE) extension (FVS; Reinhardt and Crookston, 2003), where pre-treatment vegetation for each cell was represented by tree lists derived from the TreeMap spatial vegetation data (Riley et al., 2021). FVS applies a broad variety of published models (e.g., growth, yield, mortality, fire behavior, insect, and disease) to simulate stand dynamics over time and can also apply silvicultural and burn treatments and model their subsequent dynamics. The FIA2FVS 2.0 extension (Shaw and Gagnon, 2020) was used to convert FIA tree lists to FVS and FFE input tables.
Resulting post-treatment fire hazard metrics included canopy bulk density (CBD), flame length (FL), and crowning index (CI). We reclassified flame length (FL) and canopy bulk density (CBD) outputs into “high” or “not-high” fire severity using published fire behavior thresholds from Keane et al. (2010). FRAGSTATS (McGarigal et al., 2012) was used to summarize spatial patterns for classified surfaces. At the patch-scale, we calculated the proportion of the landscape area (PLAND) for reclassified FL and CBD severity metrics. At the HUC-12-scale, we calculated the product of PLAND and the class level aggregation index (AI, hereafter AIPL), which reports the probability of neighboring high-severity pixels. We also used FVS-FFE to estimate smoke production from fires burned over each pixel following treatment. Metrics included total carbon released from fire and PM2.5 smoke production.
Finally, we estimated the potential costs and revenues from harvesting treatments with a first-principles modeling approach based on distance to roads, topographic slope, and biomass removed. We based our estimates of revenue from merchantable timber and chip biomass on cost tables provided by Washington State Department of Natural Resources for 2019 fuels treatment projects in the eastern Cascades. We used a simple model to estimate costs associated with harvesting, hauling, and accessing a treatment patch. Costs increased as a function of biomass removed, slope (as it can determine harvesting method), and distance to the nearest road (a proxy for costs associated with moving equipment to the site and transporting logs to the nearest mill). We estimated costs associated with prescribed fire as $371/ha ($150/ac; Aaron Rowe, Fuel Specialist, Okanogan-Wenatchee National Forest, Personal Communication).
The Distributed Hydrology Soil Vegetation Model
Distributed Hydrology Soil Vegetation Model was used to simulate the effects of soil, vegetation, and topography on water movement at and near the land surface. The model applies a two-layer forest canopy for estimating evapotranspiration rates, a two-layer energy balance model for estimating subcanopy snow accumulation and melt, a multilayer unsaturated soil model, and a saturated subsurface flow model (Wigmosta et al., 2002). The canopy snow model represents canopy processes that govern snow interception, mass release (snow sloughing), sublimation, and melt.
Surface land cover and soil properties are assigned to each pixel in the digital elevation model (DEM) where land surface vegetation, soil, and meteorological conditions are also defined, and a pixel-level, vertically coupled, energy and water balance is calculated. Moisture can then move laterally pixel by pixel on the surface or through the subsurface and be intercepted by roads and streams, which are burned into the DEM to enable flow routing. Vegetation characteristics and meteorological forcings can then be manipulated via new data and a calibrated model to evaluate the influence of changes in forest structure via treatments or disturbances, or changes in climate and weather.
Distributed Hydrology Soil Vegetation Model Inputs
Distributed Hydrology Soil Vegetation Model was run on a 90-m DEM resampled from its original 30-m resolution (Figure 1, DEM Wenatchee). The Natural Resources Conservation Service (NRCS) Soil Survey Geographic Database (SSURGO) was used to classify surface soils into texture groups for input into DHSVM (Supplementary Figure 1).
We then inventoried available meteorological, snow water equivalent, and stream flow data for the Wenatchee subbasin. Gridded meteorological data (Livneh et al., 2015; 1/16° resolution) was used to force the model. Observed snow water equivalent (SWE) from the Trinity Snow Telemetry (SNOTEL) site was used to evaluate model simulated SWE. Measured streamflow from USGS gauges on the Chiwawa River near Plain, Washington (12456500) and on Icicle Creek near Leavenworth, Washington (1245800) were used to calibrate/validate simulated water flow for the water years 1994–2003.
Distributed Hydrology Soil Vegetation Model Outputs
Changes in vegetation associated with the treatment scenarios described above were used as input to DHSVM to estimate treatment effects on patch-scale snow accumulation and melt, and streamflow response at the HUC-12 scale. We used three patch-level snow metrics as inputs to the DST system: (1) peak SWE, (2) peak SWE date, and (3) snow disappearance (melt out) date. Hydrologic response at the HUC-12 scale was evaluated with: (1) annual total flow, (2) mean monthly flow, and (3) mean late season flow (average flow in July through October).
Ecosystem Management Decision Support Modeling
To build our DST, we used EMDS software (Reynolds et al., 2014) to evaluate the impacts of each treatment scenario compared to a No Treatment baseline scenario across four Primary topic areas:
(1) Hydrology: High priority is given to locations where forest restoration has the potential to increase peak snowpack water content and duration, leading to increased annual flow volume and critical late-season low flows.
(2) Fire: High priority is given to locations where forest restoration has the potential to reduce the occurrence and spatial extent of severe wildfire and associated smoke emissions of subsequent wildfires. We also consider direct tradeoffs in total carbon and fine particulate emissions (PM2.5) that have implications for human health.
(3) Biomass: High priority is given to locations where forest restoration has the potential to yield merchantable timber and associated residue that can be collected and processed for bioenergy (wood chips in this case).
(4) Economics: High priority is given to locations where forest restoration has the potential to produce enough revenue through biomass extraction to offset operational costs.
The EMDS system is a spatially explicit application development software for integrated landscape evaluation, planning, and decision-making (Reynolds et al., 2003, 2014). The logic model component of EMDS (Rules of Thumb, Inc., North East, PA, United States) forms the mathematical basis for interpreting biophysical landscape information using fuzzy logic to evaluate social and ecological conditions against target or desired conditions. An EMDS logic model was built within the R statistical programming environment (v4.0.4, R Core Team, 2021). We used EMDS to develop ramp functions to quantify the range of expected outputs resulting from management prescriptions (Supplementary Figure 2). Ramps are mathematical transformations used to evaluate specific logical propositions (e.g., late season flow increases); where values range from −1 (no support) and +1 (full support for the proposition under evaluation). Table 1 defines Elementary topics used to evaluate the four Primary topics, the scale at which they are assessed, and the thresholds by which Elementary topics are evaluated. For each treatment scenario, we calculated the difference in the metric compared to the baseline No Treatment scenario. The treatment that exhibited the largest increase (or decrease) was chosen to establish the target values for that metric. For each metric, the 10th and 90th percentile delta values were calculated and used to represent cut-offs for no (−1) and full support (+1), for the stated propositions. Ramps were developed separately for each HUC-10 within the Wenatchee subbasin boundary to allow for spatial variability in conditions due to ecological gradients within larger catchments as well as localized ownership patterns that may influence ecological processes.
The use of fuzzy logic when building ramp functions has the advantage of evaluating the strength of evidence for a given proposition along a continuous scale rather than via a binary decision. Primary topics are then combined using fuzzy logic operators. For the current model, the Union operator (U) was used throughout, which specifies that component premises incrementally contribute to the proposition of their parent topic (e.g., low strength of evidence for one topic can be compensated by strong evidence from others). Final priority scores incorporate information across spatial scales and topics that define the ecological and economic benefits of treatments.
An additional tradeoff analysis was used to determine how the Primary topic-level spatial priorities correlated across the study area within each treatment scenario. Strong positive or negative correlations would indicate redundancy among topics while correlations near zero would indicate little shared or mutual information across topics.
Results
Canopy cover was reduced considerably across all management scenarios for forested pixels (Figure 2), and as expected, the variability in realized benefits across treatment scenarios was largely driven by the level of canopy cover reduction across the set of treated patches. That is, the level of canopy cover reduction was associated with the level of biomass removed, as well as reductions in ladder fuels, fire hazard, and smoke production. The IdealWater scenario produced the greatest canopy reduction overall (Figure 2), but BurnOnly and MaxBiomass had a greater impact in localized patches, where canopy cover was reduced by as much as 100% to re-establish meadow or shrubland conditions. The large reduction in canopy cover associated with the IdealWater treatment resulted in the highest treatment priority scores, especially in the mid- to high-elevation wilderness zones in the northern and western portions of the landscape where MaxBiomass could not be applied (Figure 3). As snow accumulation is greatest in these high-elevation forests, treatments applied in wilderness (BurnOnly and IdealWater) had the greatest potential to increase hydrologic outputs. IdealWater also led to the greatest reductions in wildfire risk, likely because this scenario resulted in significant canopy cover reduction on both north- and south-facing aspects. Patterns of canopy cover reduction associated with the BurnOnly scenario, conversely, were strongly determined by topography. That is, dense forests on north-facing aspects with abundant ladder fuels produced higher fire severities, which resulted in 80–100% canopy cover loss (Figure 2), while other stands burned at low-severity. Consequently, BurnOnly reduced overall fire risk and smoke potential, but IdealWater did this more uniformly across environments and land designations leading to higher priority scores (Figure 3). Given that the IdealWater scenario involved opportunities for biomass production and economic benefits in actively managed forests, as well as fuel reduction and increased streamflow in wilderness areas, it represented the “best” aspects of BurnOnly and MaxBiomass, and thereby received the highest overall priority scores.
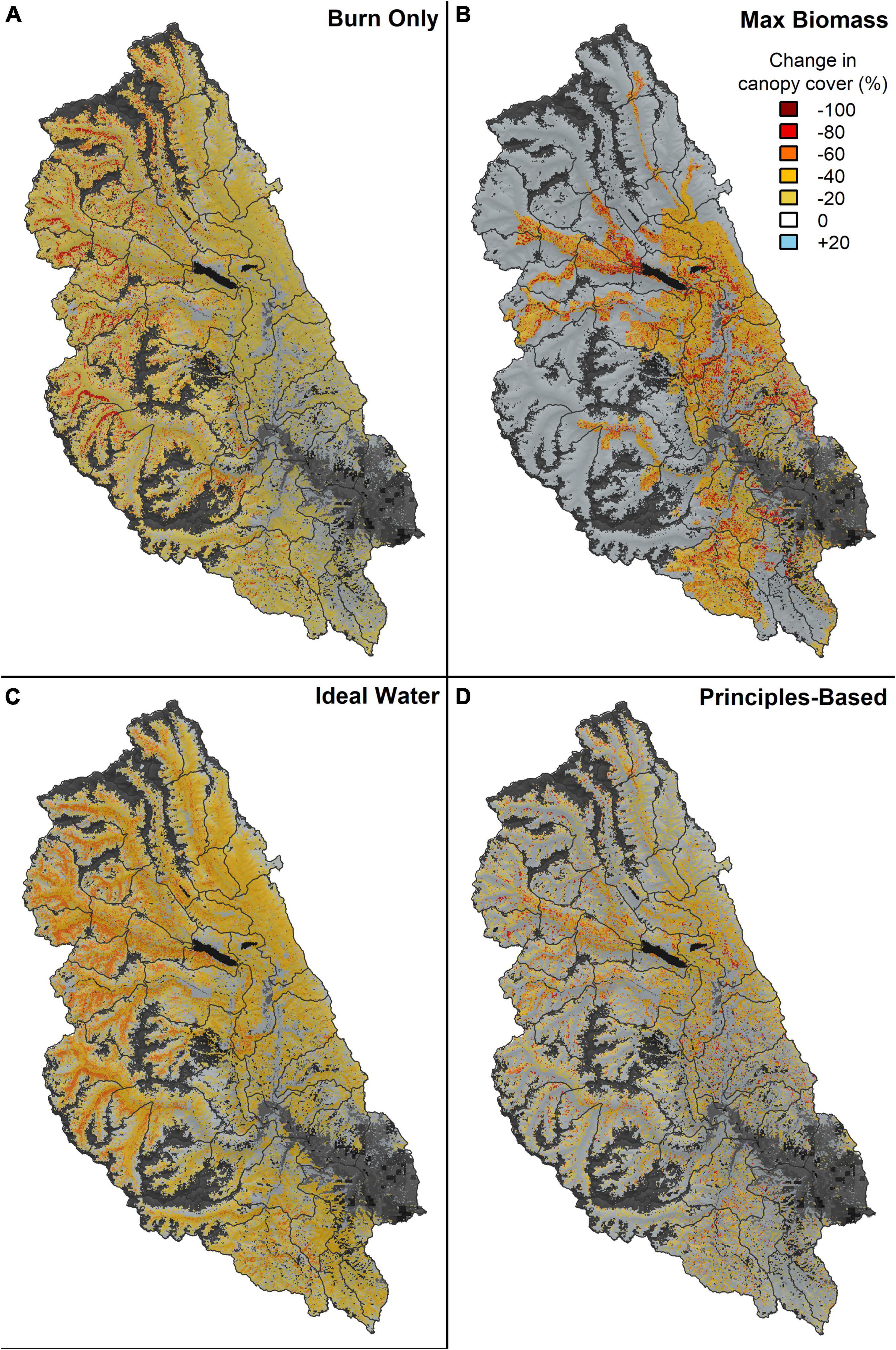
Figure 2. The simulated effects of the four management scenarios on percent canopy cover for the (A) prescribed burn only, (B) max biomass, (C) ideal water, and (D) principles-based scenarios. Dark gray areas represent non-forested area, and black indicates water.
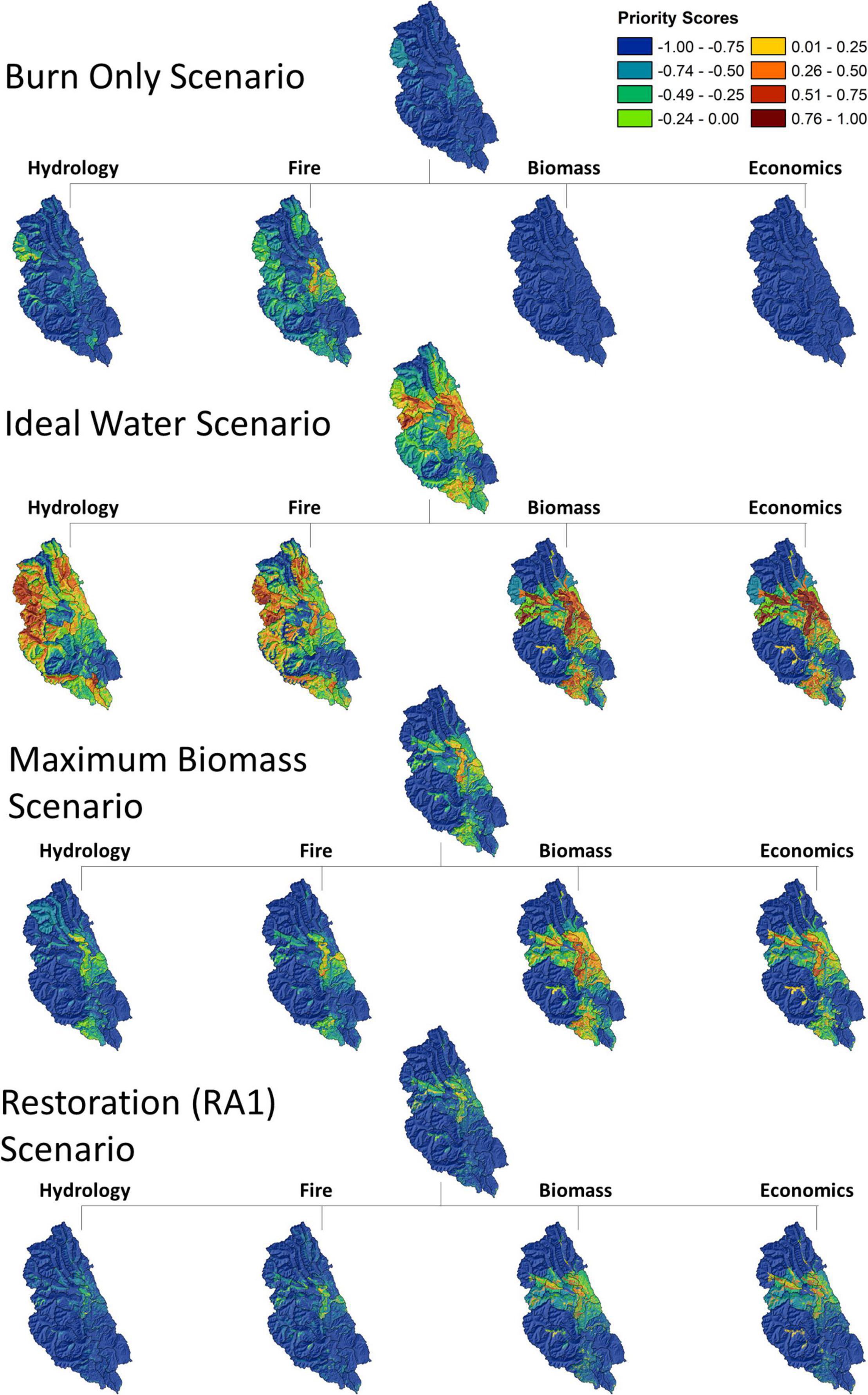
Figure 3. Decision support tool (DST) model results for all four management scenarios. Colors indicate priority values. Warm colors indicate areas with high potential for treatment benefit among each Primary topic area, cool colors indicate lower relative potential.
Figure 4 demonstrates the mapped priority scores resulting from the logic model evaluations for each topic area for the IdealWater scenario. High priority treatment patches for biomass and economics were in the central and eastern portions of the landscape where the treatment produced economic returns associated with biomass removal, while high priority treatment patches for metrics comprising the hydrology and fire topics were found in high-elevation forests where prescribed burning was the primary management tool.
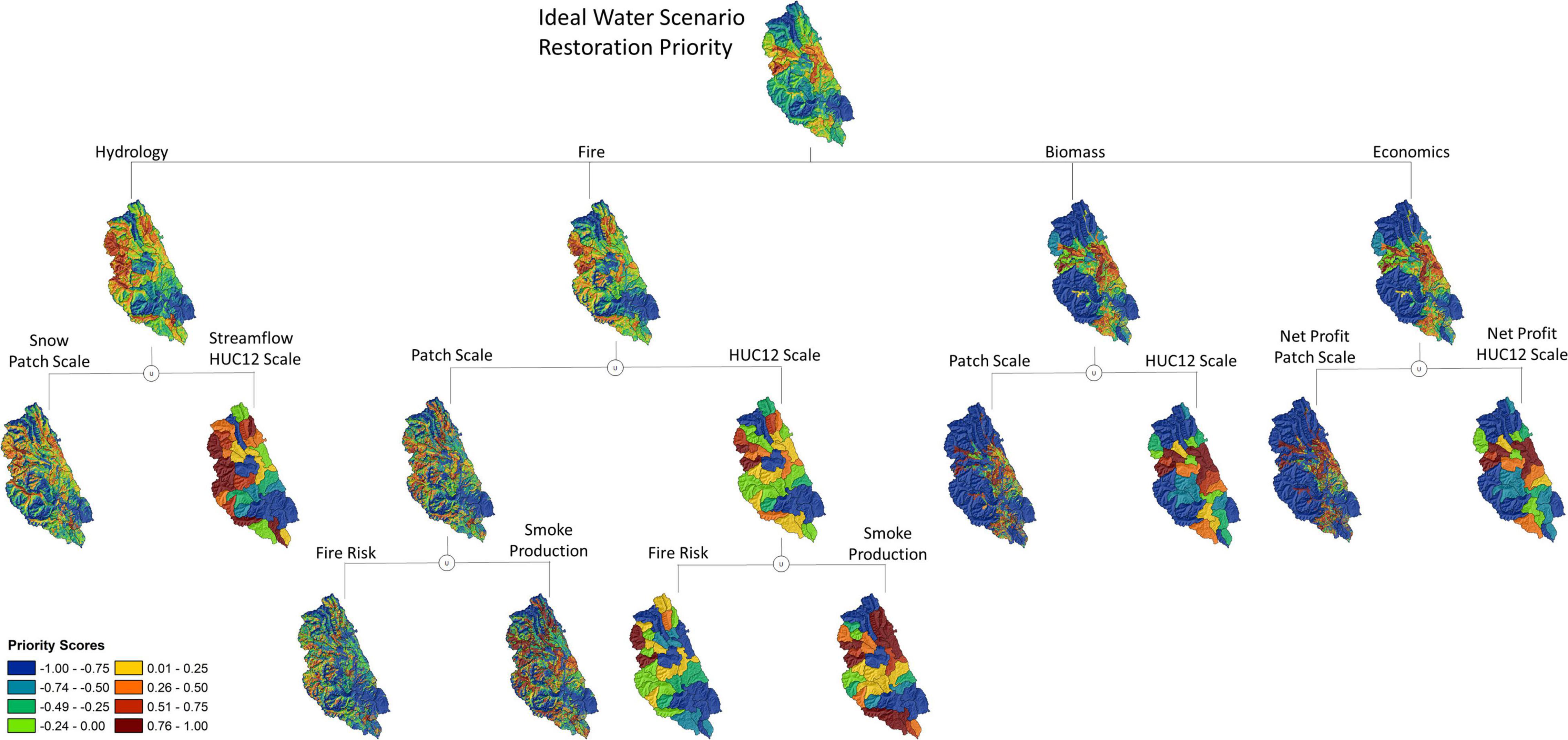
Figure 4. Full DST model results for the IdealWater treatment scenario. Note that the warm colors indicate priority watersheds (HUC-12 scale) and patches (Patch scale) for increasing snow capture, melt out date, and improved flows that are consistent with the land management allocation. Cooler colors indicate lower treatment priority.
The overall priority maps for each scenario (Figure 3) represented a quantitative evaluation of potential synergies and necessary tradeoffs between the Primary topic areas. For example, fire risk reduction and streamflow improvements were greatest in dense forests at mid to high elevations, but these areas were a low priority for the biomass and economics topics because mechanical harvest treatments could not occur in wilderness. This resulted in a neutral priority score in the wilderness, reflecting a necessary tradeoff. The highest overall priority scores were found in actively managed forests that could produce benefits among most topic areas. This is where management will likely lead to synergistic effects on multiple resource benefits and are therefore a high priority for management action.
Tradeoffs and synergies among treatments were apparent in the correlation matrix of Primary topic scores across the treatment scenarios (Figure 5). Strong correlation existed among the hydrology and fire Primary topics for all but the BurnOnly treatment, indicating that most treatments resulted in joint benefits for wildfire hazard and hydrologic outputs. Variation among treatment scenarios existed when examining potential synergies within the biomass and economic Primary topics. For treatments that were generally restricted to areas outside the wilderness (MaxBiomass), biomass production was positively correlated with both fire and hydrology topics. For IdealWater, which allowed for prescribed burning in wilderness, there was a near 0 correlation among biomass and hydrology and among economics and hydrology topics. This indicated that treatment costs were high in upper elevation wilderness, but they also generated the greatest benefits to fire hazard and water yield. Conversely, in low-elevation areas, higher revenues resulted from treatments, but the relative impacts of these treatments on fire hazard and water yield were lower.
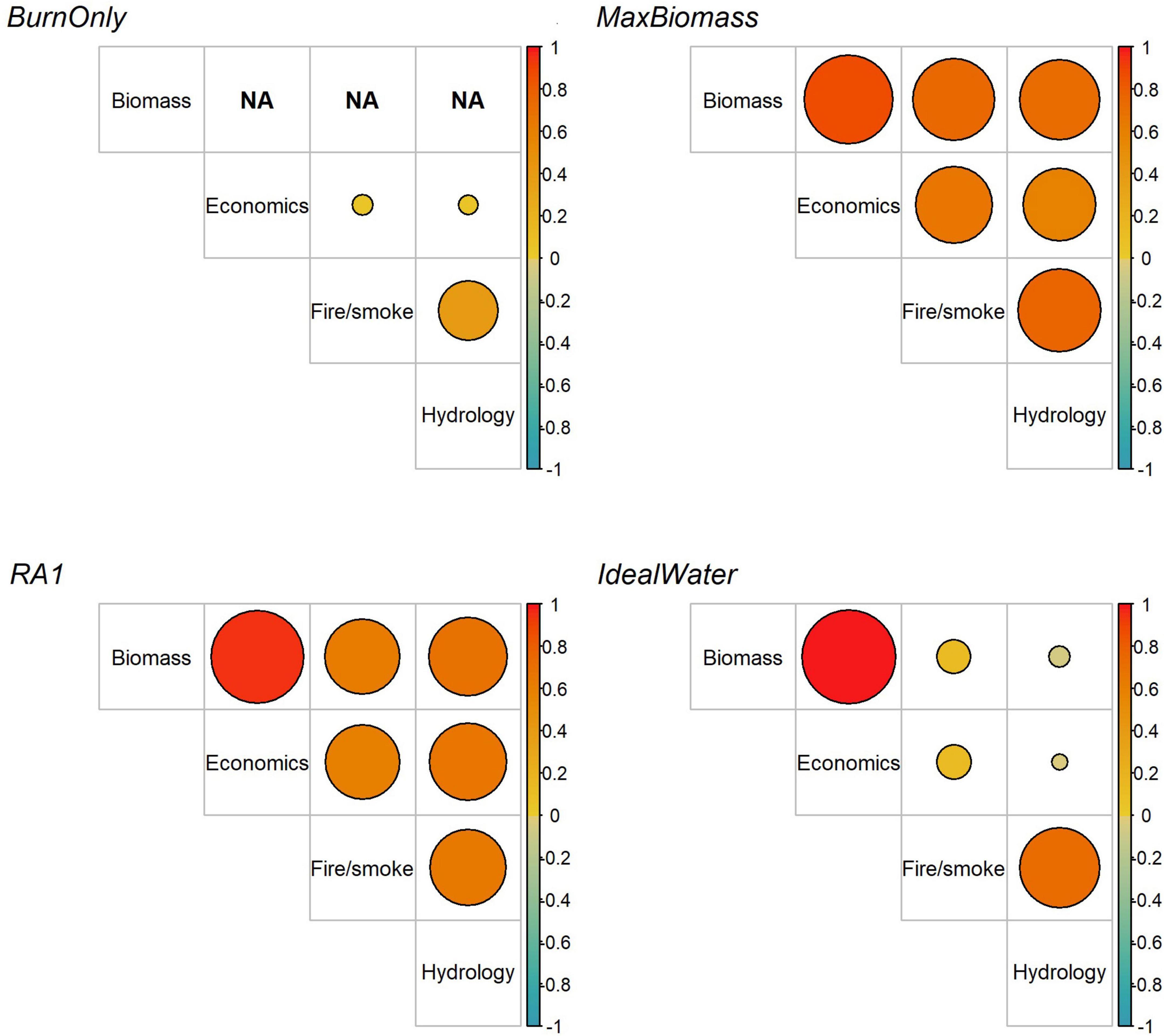
Figure 5. Correlation matrix depicting treatment benefits across resource benefits. Positive relationships indicate a given treatment has a joint positive influence on both resources, while negative numbers indicate a given treatment enhances one benefit at the expense of another. Circle size increases with the absolute value of the correlation coefficient. Analysis was restricted to include only patches where a given treatment was implemented. Main topics are biomass production from harvest (Biomass), economics from harvest (Economics), fire hazard and wildfire smoke reduction (Fire/smoke), and water yield and snowpack retention (Hydrology).
The RA1 scenario represented a realistic application of landscape restoration principles, which resulted in only 36% of the landscape being treated. As such, this scenario exhibited relatively low priority scores overall (Figure 3) in comparison with other scenarios. However, when compared to the No Treatment baseline, in patches where the RA1 treatment was implemented, it led to large reductions in fire hazard (25–93%, depending on metric), reduced potential smoke production from future wildfires (27–33%), a 10% increase in Max SWE and a 3–7% increase in annual flows (Table 2). When the entire Wenatchee catchment was considered (including untreated lands), treatment effects diminished by ∼50% for most metrics, but trends remained the same. Two exceptions were the median flow metrics, which were very similar when considering treated-only versus treated + untreated lands (Table 2).

Table 2. Average percent change in selected patch-level metrics for patches treated in the RA1 restoration scenario.
Discussion
We present the results of a DST applied to a 3,450 km2 landscape in central Washington state, United States. This area is characterized by broad environmental gradients from low-elevation shrublands and ponderosa pine woodlands to high-elevation subalpine forests and meadows, and a social-ecological context comprising WUI, working forests, and wilderness. Ecosystem services are unevenly distributed across this landscape, which drives potential resource benefit tradeoffs and synergies from management. Resilience to wildfire and ongoing climatic warming is a priority, and managers are faced with balancing tradeoffs between competing interests and diverse stakeholder values. Reducing wildfire risk in fire-prone forest types through prescribed burning and mechanical thinning can improve air quality, protect life and property in the WUI, and protect isolated remnant native fish populations from the most harmful effects of large and severe wildfires (Bisson et al., 2003; Rieman et al., 2010; Falke et al., 2015). However, tradeoffs inevitably result as the number of management objectives is compounded. For example, management of northern spotted owl and other threatened or sensitive species may necessitate implementing a late-successional reserve strategy favoring multi-layered canopy structures, but these patches represent high wildfire hazard and an unstable storage of carbon (Hurteau et al., 2016). These forests may serve a critical ecological function in terms of habitat and structural diversity but retaining dense forest conditions will simply forestalls wildfire risk, smoke production, and carbon losses. These divergent priorities are also reflected in the juxtaposition of actively managed and wilderness forests, but this need not be the case as we have shown by application of BurnOnly treatments in the wilderness. Still, competing objectives also exist within actively managed forests. As society places growing pressure on land managers to maintain (or restore) a growing set of ecosystem services, quantitatively evaluating tradeoffs between objectives will become increasingly necessary for strategic and tactical planning.
As the effects of climate change continue to mount, we are increasingly reliant upon forested watersheds to yield high quality water for human communities, endangered fish species, and downstream stakeholders. Yet, research is just beginning to uncover the potential for management to procure water resources across large landscapes (Blöschl et al., 2019). Long-term solutions for improving snow retention and late-season streamflow will require active forest management across private and public lands, and within wilderness areas, to improve hydrologic function while reducing wildfire hazard to protect water yield investments into the future. While fuel reduction and restoration treatments can accomplish wildfire hazard abatement (Prichard et al., 2021; Stephens et al., 2021a), the capacity for management to secure reliable sources of freshwater is more complex (Dickerson-Lange et al., 2021). Lundquist et al. (2013), investigated the relationship between snowpack duration and meteorological variables and found that snow persisted longer in openings than under forested canopies in areas with mean December–February air temperatures > −1°C, but the opposite was true in colder environments. The precise elevation where this shift occurs varies between years and geographies (Dickerson-Lange et al., 2021). Thus, the impacts of treatments on hydrological functioning are nuanced and require sophisticated modeling to provide accurate estimates of treatment effects as the climate continues to change.
Leveraging a mountain landscape hydrology model (DHSVM) designed for complex terrains in our decision support framework allowed us to capture many of the spatial and temporal dynamics that drive snow retention and hydrologic function. Our application revealed potential synergies between hydrologic function and wildfire risk reduction, but achieving these joint benefits was contingent upon treating wilderness where most of the snow resides and where high-severity wildfire hazard exists. Currently, the only way to treat these areas is with prescribed fire or wildland fire use. Our results underscore the importance of actively using fire as a management tool not only to reduce fuels and fire hazard, but to improve streamflow as well. Additional impacts from prescribed burning related to snowpack may exist (e.g., changing albedo) but were not modeled here. For example, black carbon depositions from fires can decrease the albedo of snow-covered landscapes thereby increasing their heat absorption and melting rates (Virkkula et al., 2014). Conversely, removal of overstory canopy may increase albedo overall if snow cover is sustained in the new light environment (Randerson et al., 2006). Wildland fire use is a particularly promising treatment option, as low- to moderate-severity wildfire can restore within-stand spatial pattern complexity and create fine- to meso-scale forest gaps (Furniss et al., 2020), which are an important driver of snow retention and snowmelt dynamics (Lundquist et al., 2013; Sun et al., 2018).
The type and extent of active management that is permitted within wilderness is a contentious issue (Cole, 2001; Miller et al., 2011). Our results suggest that low and moderate-severity fire effects can provide somewhat limited synergies across the ecosystem services modeled here. Joint benefits to the fire and hydrology Primary topics were better realized with MaxBiomass and IdealWater treatments, providing evidence supporting the contributions of mechanical treatments in actively managed forests to multi-resource benefit strategies. However, inclusion of these treatments must consider restrictions on application in wilderness and roadless areas, as well as the potential negative impacts of harvesting practices on terrestrial and riparian ecosystem processes from road building and maintenance, and harvest operations (Forman et al., 2003; Cao et al., 2021).
The lack of benefits realized by the BurnOnly treatment may be due to the conservative fuel moisture conditions we chose for prescribed burning that provided primarily low and moderate-severity fire behavior and effects. Prescribed burning with drier fuels, producing increased amounts of moderate and high-severity fire and therefore greater canopy cover reduction, may have yielded stronger effects. Overall final priority scores were much lower for the BurnOnly scenario given the lack of economic and biomass opportunities assumed under this scenario. It is likely that more spatially complex, mixed-severity burning in wilderness will be assistive as well, as this would increase the extent of early seral ecotypes and overall patchiness of the landscape. This is a testable hypothesis that we intend to pursue in future phases of this work.
Comparison of the outcomes associated with the MaxBiomass and IdealWater scenarios suggests an important difference between spatially homogeneous versus gap-based thinning. The thinning applied in IdealWater was spatially explicit, i.e., variable radius gaps were created within pixels that were equivalent to 1.2 times the canopy height. These gaps directly altered snow interception, shading, and long-wave radiation modeled within DHSVM, which improved streamflow (Supplementary Figure 3). Thus, differences in priority scores between MaxBiomass and IdealWater may be partially attributed to the differences in how each stand was thinned and thinning effects on snow interception and melt dynamics. Capturing spatial heterogeneity in forest management prescriptions can achieve a variety of restoration goals including hydrologic processes (Larson and Churchill, 2012; Stephens et al., 2021a).
Within-patch spatial patterning considerations are rapidly being integrated into tactical forest prescriptions throughout the wUS (Churchill et al., 2013, 2016; Maher et al., 2019), and our results provide another piece of evidence in support of that work. There are, however, other differences between the MaxBiomass and IdealWater scenarios. In IdealWater, we prioritized gap size over other management objectives such as large tree retention. While diameter limits prevent harvesting trees above specific diameter cutoffs to preserve the unique ecological role these structures play (Hessburg et al., 2015; Lutz et al., 2018), these limits are sometimes at odds with other management objectives such as restoring historical spatial patterns, reducing dominance of shade-tolerant and fire-intolerant species, and removing ladder fuels (Hessburg et al., 2022). As shown here, the removal of large trees can, in certain situations, increase the potential for treatments to meet multiple management objectives including improved hydrologic function, reduced wildfire hazard, increased spatial heterogeneity, and increased biomass production.
Management Implications
Management of public forestlands in the wUS is attempting to adapt ecosystems to changing climate and disturbance regimes after 150–170 years of fire exclusion and timber harvest (Hessburg et al., 2016, 2019; Hagmann et al., 2021). The Okanogan-Wenatchee National Forest (OWNF), through its Forest Restoration Strategy, and the Washington State Department of Natural Resources (DNR) via its Twenty Year Forest Health Strategy are implementing landscape-scale forest restoration across north-central Washington with a goal of doubling the restoration footprint over the next decade (Gaines et al., 2012). The current ecological conditions on the OWNF and the restoration-oriented land management mission are common among National Forest landscapes throughout the wUS. Our DST may be directly applicable to these restoration efforts, as it provides a quantitative way to strategically allocate resources for restoration treatments throughout large catchments. Needed for model calibration are applicable meteorological station data to capture variable water years, a recent span of years of continuously instrumented stream gaging data, a representative wall-to wall vegetation coverage to represent current conditions, and the modeling tools discussed here.
By evaluating several disparate management scenarios, our modeling framework can directly define a decision space, evaluate tradeoffs among treatments, and bridge the gap between a strategic (i.e., where on the landscape to apply resources) and tactical models (i.e., what specific prescription to implement). The DST provides a quantitative assessment of treatment priorities based on their level of departure from desired target conditions, and it captures the differential effects of each treatment type on anticipated management outcomes. The result is a unified model to develop spatial management priorities based on treatment need and treatment type based on the benefits associated with various possible prescriptions in a given stand. This provides a quantitative, transparent, and adaptable tool to guide management strategies and treatment tactics at multiple scales.
The multi-scale, spatially explicit design is a key advantage of decision support tools: they allow managers to evaluate the potential contributions of treatment across spatial scales, allowing the DST to be incorporated at multiple stages of the decision-making process and at multiple levels in the organizational hierarchy. The results of our DST do not represent a single solution set; rather, the DST outputs provide empirical support for short-term decision-making and for designing long-term management strategies. For example, HUC-12 priority scores may help guide decadal-scale landscape-wide planning efforts within a National Forest or Ranger District, while the patch-level priority scores can help prioritize key patches and forest stands that can be targeted with a treatment prescription. The multi-scale nature of our DST also facilitates the incorporation of ecological and biophysical processes that are scale dependent. For example, snow-retention is largely determined by aspect at the patch-level, but HUC-12-level streamflow is more strongly influenced by broader-scale elevation gradients (Figure 4). Evaluating treatment effects at multiple scales allowed us to summarize treatment effects at the scale most appropriate to the biophysical process of interest, resulting in a model that is less sensitive to a priori decisions regarding the spatial scale of analysis.
Our DST integrated ecological processes with social priorities in an inherently subjective model. Each priority topic represents some amount of ecological, social, and economic value, but these three types of value need not be weighted equally. We aimed to give relatively even weight to a variety of topics (all unions were 1:1), and the structure of the model was determined a priori for this study. However, the basic DST framework is very well-suited to adaptive management strategies. By evaluating the results of our DST, it becomes clear which individual metrics and priority topic areas are driving the overall results. If management priorities change, or if the resultant model is found to be inconsistent with stakeholder needs, the model can be easily modified to produce priority scores that are proportionate to the evolving landscape of social-ecological values.
Limitations and Future Research
Priority scores do not necessarily indicate the effect size of each treatment. Low priority scores (blue tones in Figures 3, 4) simply indicate that the treatment effect was less than that of other scenarios in a given HUC-10, while high priority scores (red tones in Figures 3, 4) indicate the maximum possible treatment effect within these modeled scenarios. Minimum and maximum treatment effects are set to −1 and 1, respectively, using the DST ramp functions. This can make small differences between scenarios difficult to evaluate. For example, the cost of Rx fire in the BurnOnly scenario was not easily distinguishable from the No Treatment scenario, as the estimated cost of $371/ha was marginal compared to the potential profits available in the IdealWater and MaxBiomass scenarios (Table 1). This nuance is key to interpreting the DST priority maps, as magnitude of potential effect size is an important post hoc consideration that may alter the value one wishes to grant to each branch of the DST. The actual magnitude of treatment effects is reported in Table 2. This could be addressed in future modeling where rather than relying on the distribution of treatment effect sizes across the landscape to determine the logic model cutoffs (e.g., 10th and 90th percentile treatment effect size), these values can be established by the user to indicate a desired treatment outcome for the logic model evaluation.
While we identified treated areas with the potential to produce net benefits to all four Primary topics, an important result revealed by our modeling was the current limitation of the existing vegetation conditions. There has been significant timber harvesting in the Wenatchee subbasin over the past 100 years, with selective harvesting and repeated thinning entries over the century. Consequently, the contemporary landscape represents a low point for available biomass and economic viability. With proper silvicultural management, opportunities for sustainable biomass production and economic returns will likely increase over the next century. This would have a considerable impact on the priority maps produced by the DST, as we anticipate forest regrowth will increase the area available to produce benefits among all four Primary topic areas. This condition is widespread throughout the wUS, where either harvesting or recent fire has touched nearly every acre of actively managed forest land, and where harsh climatic and edaphic conditions limit growth rate. Projecting forest succession into future decades is beyond the scope of the present study, but it will be a primary focus of our future decision support modeling. Based on comparisons of contemporary stocking levels and historical conditions, we estimate that it will take 50–100 years to fully realize the forest growth capacity of this landscape. In that context, this DST represents a snapshot of a broader vision for decision support that can reveal forest growth and development over space and time, within a realistic ongoing disturbance context, while projecting succession and disturbance dynamics and ecosystems service trade-offs and synergies over the coming century.
Modeling future landscape dynamics will also resolve another challenge of the snapshot DST: in reality, treatments cannot be implemented instantaneously. While our results demonstrate the potential for varied management scenarios to have positive impacts on mountain hydrology, streamflow will likely return to a baseline within years to a decade or more post-treatment (Stednick, 1996, 2008). As it is not feasible to treat every stand within a 3,450 km2 landscape over the span of just a few years, the realized effects of treatment would likely be far less than estimated by our DST. In projecting landscape dynamics into the future, we will be able to implement treatments at a realistic pace, represent vegetation regrowth, and our modeled treatment effects will therefore be a more reliable representation of anticipated treatment effects.
Finally, climatic changes were not incorporated into the DST developed in this study, but projected climate warming will have a profound impact on the Wenatchee subbasin over the coming century. Climate will directly affect both hydrology and fire topic areas by altering precipitation amount and form, and through altering fire regimes and high-severity fire risk thresholds. Climate will also have indirect effects on the biomass and economic topics through complex (and potentially divergent) impacts on background tree mortality rates (van Mantgem and Stephenson, 2007), forest regeneration (van Mantgem et al., 2006; Coop et al., 2020), and growth rates (Fang et al., 2014). Incorporating climate, disturbance, and forest succession into a temporally dynamic DST would therefore capture some of these anticipated changes, an approach which would have the potential to provide a quantitative foundation for long-term adaptive management of multi-use, multi-ownership landscapes.
Conclusion
Through our decision support modeling, we show the relative merits of a variety of forest treatments across a complex social-ecological landscape. Treatments varied in their ability to achieve multiple objectives, which included wildfire hazard and smoke emission reductions, improved water yields, biomass production, and economic recovery. Our model accounted for both strategic and tactical decision-making, rendering it particularly useful in assessing opportunities for achieving multiple benefits across the subbasin. We found that constraints on our implementation of management in the wilderness impacted the ability to achieve multiple benefits, given that the BurnOnly treatment provided no direct economic or biomass outputs. MaxBiomass treatment produced high priority scores in actively managed forests but was limited in its overall benefit to the hydrology and fire primary because it was not applied in wilderness. IdealWater produced high priority scores throughout the landscape, but it represented perhaps the least realistic treatment scenario. Finally, the RA1 scenario successfully provided benefits across multiple topic areas while maintaining strong synergies among potential benefits, demonstrating it as a viable landscape restoration strategy for achieving multiple-use management objectives.
Data Availability Statement
The raw data supporting the conclusions of this article will be made available by the authors upon request, without undue reservation.
Author Contributions
NP, PH, RS, and MW contributed to conception and design of the study, and developed the decision support architecture. NP, RS, TF, and ZD organized the database. ZD performed the hydrological modeling. TF performed the FVS treatment analyses. NP performed the decision support modeling. NP, TF, and PH wrote the first draft of the manuscript. RS provided GIS support and developed maps and contributed figures. NP, RS, and TF contributed to figure development. MW, ZD, and RS wrote sections of the manuscript. All authors contributed to manuscript revision, read, and approved the submitted version.
Funding
This research was supported by the U.S. Department of Energy Bioenergy Technologies Office. This project was supported in part by an appointment to the Internship/Research Participation Program at the Pacific Northwest Research Station, U.S. Forest Service, administered by the Oak Ridge Institute for Science and Education (ORISE) through an interagency agreement between the U.S. Department of Energy and U.S. Forest Service.
Conflict of Interest
ML was employed by Resilient Forestry, LLC.
The remaining authors declare that the research was conducted in the absence of any commercial or financial relationships that could be construed as a potential conflict of interest.
Publisher’s Note
All claims expressed in this article are solely those of the authors and do not necessarily represent those of their affiliated organizations, or those of the publisher, the editors and the reviewers. Any product that may be evaluated in this article, or claim that may be made by its manufacturer, is not guaranteed or endorsed by the publisher.
Supplementary Material
The Supplementary Material for this article can be found online at: https://www.frontiersin.org/articles/10.3389/ffgc.2022.805179/full#supplementary-material
Footnotes
- ^ http://www.landfire.gov
- ^ https://www.usgs.gov/core-science-systems/national-geospatial-program/national-map
- ^ https://sourceforge.net/projects/open-fvs/
References
Agee, J. K., and Skinner, C. N. (2005). Basic principles of forest fuel reduction treatments. For. Ecol. Manage. 211, 83–96. doi: 10.1016/j.foreco.2005.01.034
Allen, C. D., Breshears, D. D., and McDowell, N. G. (2015). On underestimation of global vulnerability to tree mortality and forest die-off from hotter drought in the Anthropocene. Ecosphere 6, 1–55. doi: 10.1890/ES15-00203.1
Bisson, P. A., Rieman, B. E., Luce, C., Hessburg, P. F., Lee, D. C., Kershner, J. L., et al. (2003). Fire and aquatic ecosystems of the western USA: current knowledge and key questions. For. Ecol. Manag. 178, 213–229. doi: 10.1016/s0378-1127(03)00063-x
Blöschl, G., Bierkens, M. F., Chambel, A., Cudennec, C., Destouni, G., Fiori, A., et al. (2019). Twenty-three unsolved problems in hydrology (UPH)–a community perspective. Hydrol. Sci. J. 64, 1141–1158.
Cao, L., Elliot, W., and Long, J. W. (2021). Spatial simulation of forest road effects on hydrology and soil erosion after a wildfire. Hydrol. Process. 35:e14139.
Churchill, D. J., Jeronimo, S., Larson, A. J., Fischer, P., Dahlgreen, M. C., and Franklin, J. F. (2016). The ICO Approach to Quantifying and Restoring Forest Spatial Pattern: Implementation Guide. ICO App. 3.
Churchill, D. J., Larson, A. J., Dahlgreen, M. C., Franklin, J. F., Hessburg, P. F., and Lutz, J. A. (2013). Restoring forest resilience: from reference spatial patterns to silvicultural prescriptions and monitoring. For. Ecol. Manag. 291, 442–457. doi: 10.1016/j.foreco.2012.11.007
Cole, D. N. (2001). Management dilemmas that will shape wilderness in the 21st century. J. For. 99, 4–8.
Coop, J. D., Parks, S. A., Stevens-Rumann, C. S., Crausbay, S. D., Higuera, P. E., Hurteau, M. D., et al. (2020). Wildfire-driven forest conversion in western North American landscapes. BioScience 70, 659–673. doi: 10.1093/biosci/biaa061
Crookston, N. L., and Dixon, G. E. (2005). The forest vegetation simulator: a review of its structure, content, and applications. Comput. Electron. Agric. 49, 60–80. doi: 10.1016/j.compag.2005.02.003
Dickerson-Lange, S. E., Vano, J. A., Gersonde, R., and Lundquist, J. D. (2021). Ranking forest effects on snow storage: a decision tool for forest management. Water Resour. Res. 57:e2020WR027926.
Dombeck, M. P., Williams, J. E., and Wood, C. A. (2004). Wildfire policy and public lands: Integrating scientific understanding with social concerns across landscapes. Conserv. Biol. 18, 883–889. doi: 10.1111/j.1523-1739.2004.00491.x
Falke, J. A., Flitcroft, R. L., Dunham, J. B., McNyset, K. M., Hessburg, P. F., and Reeves, G. H. (2015). Climate change and vulnerability of bull trout (Salvelinus confluentus) in a fire-prone landscape. Can. J. Fish. Aquat. Sci. 72, 304–318. doi: 10.1139/cjfas-2014-0098
Fang, J., Kato, T., Guo, Z., Yang, Y., Hu, H., Shen, H., et al. (2014). Evidence for environmentally enhanced forest growth. Proc. Natl. Acad. Sci. 111, 9527–9532. doi: 10.1073/pnas.1402333111
Forman, R. T., Sperling, D., Bissonette, J. A., Clevenger, A. P., Cutshall, C. D., Dale, V. H., et al. (2003). Road Ecology: Science and Solutions. Washington, D.C: Island press.
Furniss, T. J., Das, A., van Mantgem, P., Stephenson, N. L., and Lutz, J. A. (2022). Crowding, climate, and the case for social distancing among trees. Ecol. Appl. 32:e2507. doi: 10.1002/eap.2507
Furniss, T. J., Larson, A. J., Kane, V. R., and Lutz, J. A. (2020). Wildfire and drought moderate the spatial elements of tree mortality. Ecosphere 11:e03214. doi: 10.1002/ecs2.3214
Gaines, W. L., Peterson, D. W., Thomas, C. A., and Harrod, R. J. (2012). Adaptations to Climate Change: Colville and Okanogan-Wenatchee National Forests. Gen. Tech. Rep. PNW-GTR-862. Portland, OR: USDA-FS, Pacific Northwest Research Station, 34.
Goeking, S. A., and Tarboton, D. G. (2020). Forests and water yield: a synthesis of disturbance effects on streamflow and snowpack in western coniferous forests. J. For. 118, 172–192. doi: 10.1093/jofore/fvz069
Hagmann, R. K., Hessburg, P. F., Prichard, S. J., Povak, N. A., Brown, P. M., Fulé, P. Z., et al. (2021). Evidence for widespread changes in the structure, composition, and fire regimes of western North American forests. Ecol. Appl. 31:e02431. doi: 10.1002/eap.2431
Hamlet, A. F., and Lettenmaier, D. P. (1999). Effects of climate change on hydrology and water resources in the Columbia River Basin. J. Am. Water Res. Assoc. 35, 1597–1623. doi: 10.1016/j.scitotenv.2020.141112
Hessburg, P. F., Charnley, S., Gray, A. N., Spies, T. A., Peterson, D. W., Flitcroft, R. L., et al. (2022). Climate and wildfire adaptation of inland Northwest US forests. Front. Ecol. Environ. 20:40–48. doi: 10.1002/fee.2408
Hessburg, P. F., Charnley, S., Wendel, K. L., White, E. M., Singleton, P. H., Peterson, D. W., et al. (2020). The 1994 Eastside Screens Large-tree Harvest Limit: Review of Science Relevant to Forest Planning 25 Years Later. Gen. Tech. Rep. PNW-GTR-990. Portland, OR: US Department of Agriculture, Forest Service, Pacific Northwest Research Station, 114.
Hessburg, P. F., Churchill, D. J., Larson, A. J., Haugo, R. D., Miller, C., Spies, T. A., et al. (2015). Review article, Restoring fire-prone Inland Pacific landscapes: seven core principles. Landsc. Ecol. 30, 1805–1835. doi: 10.1007/s10980-015-0218-0
Hessburg, P. F., Miller, C. L., Parks, S. A., Povak, N. A., Taylor, A. H., Higuera, P. E., et al. (2019). Climate, environment, and disturbance history govern resilience of western North American forests. Front. Ecol. Evol. 7:239. doi: 10.3389/fevo.2019.00239
Hessburg, P. F., Prichard, S. J., Hagmann, R. K., Povak, N. A., and Lake, F. K. (2021). Wildfire and climate change adaptation of western North American forests: a case for intentional management. Ecol. Appl. 31:e02432. doi: 10.1002/eap.2432
Hessburg, P. F., Reynolds, K. M., Salter, R. B., Dickinson, J. D., Gaines, W. L., and Harrod, R. J. (2013). Landscape evaluation for restoration planning on the Okanogan-Wenatchee National Forest USA. Sustainability 5, 805–840. doi: 10.3390/su5030805
Hessburg, P. F., Smith, B. G., Salter, R. B., Ottmar, R. D., and Alvarado, E. (2000). Recent changes (1930s–1990s) in spatial patterns of interior northwest forests, USA. For. Ecol. Manag. 136, 53–83. doi: 10.1016/s0378-1127(99)00263-7
Hessburg, P. F., Spies, T. A., Perry, D. A., Skinner, C. N., Taylor, A. H., Brown, P. M., et al. (2016). Tamm review: management of mixed-severity fire regime forests in Oregon, Washington, and Northern California. For. Ecol. Manag. 366, 221–250. doi: 10.1016/j.foreco.2016.01.034
Hurteau, M. D., Liang, S., Martin, K. L., North, M. P., Koch, G. W., and Hungate, B. A. (2016). Restoring forest structure and process stabilizes forest carbon in wildfire-prone southwestern ponderosa pine forests. Ecol. Appl. 26, 382–391. doi: 10.1890/15-0337
Keane, R. E., Drury, S. A., Karau, E. C., Hessburg, P. F., and Reynolds, K. M. (2010). A method for mapping fire hazard and risk across multiple scales and its application in fire management. Ecol. Model. 221, 2–18. doi: 10.1016/j.ecolmodel.2008.10.022
Larson, A. J., and Churchill, D. (2012). Tree spatial patterns in fire-frequent forests of western North America, including mechanisms of pattern formation and implications for designing fuel reduction and restoration treatments. For. Ecol. Manag. 267, 74–92. doi: 10.1016/j.foreco.2011.11.038
Livneh, B., Bohn, T. J., Pierce, D. W., Munoz-Arriola, F., Nijssen, B., Vose, R., et al. (2015). A spatially comprehensive hydrometeorological data set for Mexico, the U.S., and Southern Canada 1950–2013. Sci. Data 2:150042. doi: 10.1038/sdata.2015.42
Luce, C. H., and Holden, Z. A. (2009). Declining annual streamflow distributions in the Pacific Northwest United States, 1948–2006. Geophys. Res. Lett. 36:L16401.
Lundquist, J. D., Dickerson-Lange, S., Lutz, J. A., and Cristea, N. C. (2013). Lower forest density enhances snow retention in regions with warmer winters: a global framework developed from plot-scale observations and modeling. Water Resour. Res. 49, 6356–6370. doi: 10.1002/wrcr.20504
Lutz, J. A., Furniss, T. J., Johnson, D. J., and Davies, S. J. (2018). Global importance of large-diameter trees. Glob. Ecol. Biogeogr. 27, 849–864. doi: 10.1111/geb.12747
Maher, C. T., Oja, E., Marshall, A., Cunningham, M., Townsend, L., Worley-Hood, G., et al. (2019). Real-time monitoring with a tablet app improves implementation of treatments to enhance forest structural diversity. J. For. 117, 280–292. doi: 10.1093/jofore/fvz003
McGarigal, K., Cushman, S. A., and Ene, E. (2012). FRAGSTATS v4: Spatial Pattern Analysis Program for Categorical and Continuous maps. Computer Software Program Produced by the authors at the University of Massachusetts, Amherst. Available online at: https://fragstats.software.informer.com/4.2/ (accessed April 14, 2022).
Millar, C. I., and Stephenson, N. L. (2015). Temperate forest health in an era of emerging megadisturbance. Science 349, 823–826. doi: 10.1126/science.aaa9933
Millar, C. I., Stephenson, N. L., and Stephens, S. L. (2007). Climate change and forests of the future: managing in the face of uncertainty. Ecol. Appl. 17, 2145–2151. doi: 10.1890/06-1715.1
Miller, C., Abatzoglou, J., Brown, T., and Syphard, A. D. (2011). “Wilderness fire management in a changing environment,” in The Landscape Ecology of Fire, eds D. McKenzie, C. Miller, and D. A. Falk (Dordrecht: Springer), 269–294. doi: 10.1007/978-94-007-0301-8_11
Pierce, D. W., Barnett, T. P., Hidalgo, H. G., Das, T., Bonfils, C., Santer, B. D., et al. (2008). Attribution of declining western US snowpack to human effects. J Clim. 21, 6425–6444. doi: 10.1175/2008jcli2405.1
Prichard, S. J., Hessburg, P. F., Hagmann, R. K., Povak, N. A., Dobrowski, S. Z., Hurteau, M. D., et al. (2021). Adapting western North American forests to climate change and wildfires: ten common questions. Ecol. Appl. 31:e02433. doi: 10.1002/eap.2433
R Core Team. (2021). R: A Language and Environment for Statistical Computing. Vienna: R Foundation for Statistical Computing.
Randerson, J. T., Liu, H., Flanner, M. G., Chambers, S. D., Jin, Y., Hess, P. G., et al. (2006). The impact of boreal forest fire on climate warming. Science 314, 1130–1132. doi: 10.1126/science.1132075
Reinhardt, E., and Crookston, N. L. (2003). The Fire and Fuels Extension to the Forest Vegetation Simulator. Gen. Tech. Rep. RMRS-GTR-116. Ogden, UT: USDA-FS, Rocky Mountain Research Station, 209.
Reynolds, K. M., Hessburg, P. F., and Bourgeron, P. S. (2014). Making Transparent Environmental Management Decisions: Applications of the Ecosystem Management Decision Support System. Berlin: Springer Science & Business Media.
Reynolds, K. M., Hessburg, P. F., Keane, R. E., and Menakis, J. P. (2009). National fuel-treatment budgeting in US federal agencies: Capturing opportunities for transparent decision-making. For. Ecol. Manag. 258, 2373–2381. doi: 10.1016/j.foreco.2009.08.011
Reynolds, K. M., Johnson, K. N., and Gordon, S. N. (2003). The science/policy interface in logic-based evaluation of forest ecosystem sustainability. For. Policy Econ. 5, 433–446. doi: 10.1016/s1389-9341(03)00041-8
Rieman, B. E., Hessburg, P. F., Luce, C., and Dare, M. R. (2010). Wildfire and management of forests and native fishes: conflict or opportunity for convergent solutions? BioScience 60, 460–468. doi: 10.1525/bio.2010.60.6.10
Riley, K. L. I, Grenfell, C., Finney, M. A., and Wiener, J. M. (2021). TreeMap, a tree-level model of conterminous US forests circa 2014 produced by imputation of FIA plot data. Sci. Data 8:11. doi: 10.1038/s41597-020-00782-x
Sahin, V., and Hall, M. J. (1996). The effects of afforestation and deforestation on water yields. J. Hydrol. 178, 293–309. doi: 10.1016/0022-1694(95)02825-0
Saksa, P. C., Conklin, M. H., Battles, J. J., Tague, C. L., and Bales, R. C. (2017). Forest thinning impacts on the water balance of Sierra Nevada mixed-conifer headwater basins. Water Resour. Res. 53, 5364–5381. doi: 10.1002/2016wr019240
Schultz, C. A., Jedd, T., and Beam, R. D. (2012). The Collaborative Forest Landscape Restoration Program: a history and overview of the first projects. J. For. 110, 381–391. doi: 10.5849/jof.11-082
Seaber, P. R., Kapinos, F. P., and Knapp, G. L. (1987). Hydrologic Unit Maps: U.S. Geological Survey Water-Supply Paper 2294, 63 p. Updated Information not From this Source is Enclosed in Square Brackets Below. A Copy of USGS Water-Supply Paper 2294 may be Ordered from USGS Information Services. Virginia: United States Geological Survey.
Seager, R., Ting, M., Li, C., Naik, N., Cook, B., Nakamura, J., et al. (2013). Projections of declining surface-water availability for the southwestern United States. Nat. Clim. Change 3, 482–486. doi: 10.1038/nclimate1787
Shaw, J. D., and Gagnon, A. (2020). Field Note: a new conversion of forest inventory and analysis data for use in the forest vegetation simulator. J. For. 118, 307–312. doi: 10.1093/jofore/fvz050
Stednick, J. D. (1996). Monitoring the effects of timber harvest on annual water yield. J. Hydrol. 176, 79–95. doi: 10.1016/0022-1694(95)02780-7
Stednick, J. D. (2008). “Long-term streamflow changes following timber harvesting,” in Hydrological and Biological Responses to Forest Practices, ed. J. D. Stednick (Berlin: Springer Science & Business Media), 139. doi: 10.1007/978-0-387-69036-0_9
Stephens, S. L., Battaglia, M. A., Churchill, D. J., Collins, B. M., Coppoletta, M., Hoffman, C. M., et al. (2021a). Forest restoration and fuels reduction: convergent or divergent? BioScience 71, 85–101.
Stephens, S. L., Thompson, S., Boisramé, G., Collins, B. M., Ponisio, L. C., Rakhmatulina, E., et al. (2021b). Fire, water, and biodiversity in the Sierra Nevada: a possible triple win. Environ. Res. Commun. 3:081004. doi: 10.1088/2515-7620/ac17e2
Stevens, J. T., Collins, B. M., Long, J. W., North, M. P., Prichard, S. J., Tarnay, L. W., et al. (2016). Evaluating potential trade-offs among fuel treatment strategies in mixed-conifer forests of the Sierra Nevada. Ecosphere 7:e01445.
Sun, N., Wigmosta, M., Zhou, T., Lundquist, J., Dickerson-Lange, S., and Cristea, N. (2018). Evaluating the functionality and streamflow impacts of explicitly modelling forest–snow interactions and canopy gaps in a distributed hydrologic model. Hydrol. Process. 32, 2128–2140. doi: 10.1002/hyp.13150
van Mantgem, P. J., and Stephenson, N. L. (2007). Apparent climatically induced increase of tree mortality rates in a temperate forest. Ecol. Lett. 10, 909–916. doi: 10.1111/j.1461-0248.2007.01080.x
van Mantgem, P. J., Stephenson, N. L., and Keeley, J. E. (2006). Forest reproduction along a climatic gradient in the Sierra Nevada, California. For. Ecol. Manag. 225, 391–399. doi: 10.1016/j.foreco.2006.01.015
Virkkula, A., Levula, J., Pohja, T., Aalto, P. P., Keronen, P., Schobesberger, S., et al. (2014). Prescribed burning of logging slash in the boreal forest of Finland: emissions and effects on meteorological quantities and soil properties. Atmos. Chem. Phys. 14, 4473–4502. doi: 10.5194/acp-14-4473-2014
Wigmosta, M. S., and Burges, S. J. (1997). An adaptive modeling and monitoring approach to describe the hydrologic behavior of small catchments. J. Hydrol. 202, 48–77. doi: 10.1016/s0022-1694(97)00057-7
Wigmosta, M. S., Nijssen, B., and Storck, P. (2002). “The distributed hydrology soil vegetation model,” in Mathematical Models of Small Watershed Hydrology and Applications, Chap. 2, eds V. P. Singh and D. K. Frevert (Littleton, CO: Water Resource Publications), 7–42. doi: 10.1016/j.catena.2016.04.001
Keywords: adaptive management, decision support tools, ecohydrology, water balance, energy balance, mass balance, late-season low flows, climate change adaptation
Citation: Povak NA, Furniss TJ, Hessburg PF, Salter RB, Wigmosta M, Duan Z and LeFevre M (2022) Evaluating Basin-Scale Forest Adaptation Scenarios: Wildfire, Streamflow, Biomass, and Economic Recovery Synergies and Trade-Offs. Front. For. Glob. Change 5:805179. doi: 10.3389/ffgc.2022.805179
Received: 29 October 2021; Accepted: 30 March 2022;
Published: 28 April 2022.
Edited by:
Jose G. Borges, University of Lisbon, PortugalReviewed by:
Gabrielle F. S. Boisrame, Desert Research Institute (DRI), United StatesCristina Vega-Garcia, Universitat de Lleida, Spain
Ola Eriksson, Swedish University of Agricultural Sciences, Sweden
Copyright © 2022 Povak, Furniss, Hessburg, Salter, Wigmosta, Duan and LeFevre. This is an open-access article distributed under the terms of the Creative Commons Attribution License (CC BY). The use, distribution or reproduction in other forums is permitted, provided the original author(s) and the copyright owner(s) are credited and that the original publication in this journal is cited, in accordance with accepted academic practice. No use, distribution or reproduction is permitted which does not comply with these terms.
*Correspondence: Nicholas A. Povak, bmljaG9sYXMucG92YWtAdXNkYS5nb3Y=