- 1Virginia Tech, Forest Resources and Environmental Conservation, Blacksburg, VA, United States
- 2Belle W. Baruch Institute of Coastal Ecology and Forest Science, Clemson University, Georgetown, SC, United States
- 3Forestry and Environmental Conservation Department, Clemson University, Clemson, SC, United States
- 4Virginia Tech, Biological Sciences, Blacksburg, VA, United States
Expanding and restoring forests decreases atmospheric carbon dioxide, a natural solution for helping mitigate climate change. However, forests also have relatively low albedo compared to grass and croplands, which increases the amount of solar energy they absorb into the climate system. An alternative natural climate solution is to replace fossil fuels with bioenergy. Bioenergy crops such as switchgrass have higher albedo than forest ecosystems but absorb less total carbon over their lifetime. To evaluate trade-offs in the mitigation potential by pine and switchgrass ecosystems, we used eddy covariance net ecosystem exchange and albedo observations collected from planted pine forests and switchgrass fields in eastern North America and Canada to compare the net radiative forcing of these two ecosystems over the length of typical pine rotation (30 years). We found that pine had a net positive radiative forcing (warming) of 5.4 ± 2.8 Wm−2 when albedo and carbon were combined together (30 year mean). However the assumptions regarding the fate of harvested carbon had an important effect on the net radiative forcing. When we assumed all switchgrass carbon was emitted to the atmosphere while the harvested pine carbon was prevented from entering the atmosphere, the 30-year mean net radiative forcing reversed direction (−3.6 ± 2.8 Wm−2). Overall, while the pine ecosystem absorbed more carbon than the switchgrass, the difference in albedo was large enough to result in similar climate mitigation potential at the 30-year horizon between the two systems, whereby the direction and magnitude of radiative forcing depends on the fate of harvested carbon.
1. Introduction
Establishing and protecting forests contributes to natural climate solutions (NCS) by maintaining carbon stored in forest biomass and removing carbon dioxide (CO2) from the atmosphere through growth (Pacala, 2004; Bastin et al., 2019; Hemes et al., 2021). However, forests have a lower albedo than alternative land-cover types, such as agriculture or grasslands, which increases the amount of radiation absorbed at the surface (Jackson et al., 2008; Anderson et al., 2011). Depending on the location, the lower albedo associated with a forested land-cover can offset the climate benefit provided by sequestered carbon (Betts, 2000; Bala et al., 2007; Bonan, 2008). This is especially pronounced at high latitudes where there is a large discrepancy in albedo between a tall, dark forest and a short crop or grassland covered by snow from the late fall through early spring. In temperate latitudes, the albedo difference is less pronounced because snow cover is less frequent (Betts, 2000). As a result, the albedo influence on the radiative energy balance can be similar to the carbon influence (O'Halloran et al., 2012). Land-cover change can also indirectly influence climate by altering evapotransipration and cloud cover (Bala et al., 2007; Ahlswede and Thomas, 2017). Simulated changes in cloud fields linked to land cover change can manifest at great distances downwind in the atmosphere, and are highly model-dependent, making attribution a challenge (Devaraju et al., 2018). Albedo radiative forcing exhibits a global climate sensitivity similar to carbon dioxide (Davin and de Noblet-Ducoudré, 2010). Therefore, to estimate the climate benefits of land-cover and land-management decisions in the temperate region, it is critical to quantify the combined impact of the change in carbon and albedo on global climate.
An alternative NCS to establishing forests for carbon absorption and storage is replacing fossil fuel energy sources with a dedicated bioenergy crop. The above-ground biomass of a bioenergy crop is burned after harvesting for energy and, if below-ground biomass increases carbon accumulated in the soil, there is a net removal of CO2 from the atmosphere (Anderson-Teixeira and DeLucia, 2011). Crops and grasses, including perennial bioenergy crops such as Miscanthus x giganteus and switchgrass (Panicum virgatum), also have higher albedo values than forests (Bonan, 2008; Miller et al., 2016; Abraha et al., 2021). The direct effect of the higher albedo of perennial bioenergy crops on the atmospheric radiation balance can be large. One study found it to be six times larger than the offset of fossil fuel use (Georgescu et al., 2011). However, it is unknown how the net climate benefits of forests, with high productivity and potential for long-term carbon storage in wood, compare with high-albedo bioenergy crops.
Using observations of net carbon fluxes and albedo from eddy covariance (EC) sites, we compare the influence of managed pine stands to managed bioenergy crop fields on global climate using the radiative forcing metric (Hansen et al., 1997). We focus on comparing a pine plantation with a switchgrass field because they are managed ecosystems with overlapping natural ranges. In the Southeastern United States (SEUS), pine plantations are most commonly planted with loblolly pine, a wide-spread fast-growing native species that can be used for timber or bioenergy (Wear and Greis, 2013). A typical rotation length for a southern pine plantation is between 25 and 35 years (Stanturf et al., 2003; Fox et al., 2007; Wear and Greis, 2013). Switchgrass is a native, C4, perennial grass species that has emerged as a promising bioenergy feedstock with high expected yields for the SEUS (McLaughlin and Adams Kszos, 2005; Parrish and Fike, 2005; Wullschleger et al., 2010). Both species are reported to perform well on marginal lands and can even be inter-cropped (Albaugh et al., 2014). Unlike a pine plantation that is harvested at the end of the rotation with possible thinning at intermediate ages, switchgrass is typically harvested at least once per year. Therefore, including the entire cycle of establishment and harvest for both systems is critical for a robust comparison of radiative forcing.
Here we ask, (1) which ecosystem (pine plantation or bioenergy switchgrass crop) has a lower radiative forcing over a typical pine rotation and (2) how do assumptions about the fate of harvested carbon influence the conclusions? To address these questions, we combined new observations from central Virginia with published data from EC sites in the Eastern U.S. and Canada to model the relationship between age since establishment, net carbon storage (defined using net ecosystem exchange; NEE), and albedo. The age vs. albedo and NEE relationships were used with an established method for calculating relative forcing (Kirschbaum et al., 2011) over a 30-year time horizon that includes one complete harvest rotation of a southern pine plantation (a typical rotation length for the region) and annual harvests of switchgrass.
2. Methods and Materials
We describe our new eddy-covariance site and data processing, the synthesis of data from existing eddy-covariance sites, the development of the NEE and albedo age curves over a full rotation for the pine plantation and switchgrass fields, and the modeling of radiative forcing. Throughout, we use the atmospheric sign convention for NEE, whereby negative NEE corresponds to transfer of carbon from the atmosphere into the ecosystem.
2.1. New Eddy-Covariance Measurements
2.1.1. Site Description
This study uses data collected from three co-located eddy covariance towers located in Amherst county in central Virginia. The climate of this region is humid subtropical (Köppen climate classification Cfa), with warm humid summers and cool mild winters. The first tower (AmeriFlux designation: US-SB1, Pine hereafter) was installed over a mixed age loblolly pine plantation and began measurements May 6th, 2015. The pine tower is located at 37.57342 N, −79.08482 W, elevation 242 m, with a mixture of Clifford loam and Clifford clay loam soils in the fetch. The second tower (US-SB2, Grass hereafter) was installed over a switchgrass field that was harvested annually for bioenergy and began measurements March 26th, 2016 (Ahlswede et al., 2022). The grass tower is located at 37.5605 N, −79.0884 W, elevation 240 m, with a mixture of Clifford clay loam and wintergreen clay loam soils in the fetch. The third tower (US-SB3, Clearcut hereafter) was installed over a clear-cut loblolly pine stand that was harvested and removed in late 2017; measurements began March 28th, 2018. The clearcut tower is located at 37.5753 N, −79.0872 W, elevation 242 m, with Clifford loam soils in the fetch. These three towers were within 1.6 km of each other, ensuring that each system share weather conditions and soil characteristics. Mean annual temperature and precipitation for Amherst county between 1990 and 2020 is 13.1 °C, 1,210 mm (Vose et al., 2014).
2.1.2. Measurement of Carbon and Albedo
Each site was equipped to measure meteorological data and an eddy covariance system to measure CO2 and water fluxes. Each tower measured incoming and outgoing shortwave radiation with a CNR4 four-way net radiometer (Model CNR4, Kipp and Zonen). The CNR4 of the pine tower was 26 m above ground level, 4–6 m above the upper canopy (20–22 m in height). At the clearcut and grass towers, instruments were mounted on a tripod tower, with the CNR4 at a height of 4 m and extended away from the tower base to avoid non-vegetation in the instrument's field of view. Meteorological sensors were sampled every one second and 1 min averages were stored on a data logger (Model XLite 9210, Sutron).
Each tower was equipped with an eddy covariance (EC) system that was used to measure the flux of CO2. Gas concentrations were measured with a closed-path infrared gas analyzer (LI-7200, Licor) at the pine and grass towers at 26 and 4 m, respectively. The clearcut tower was equipped with an open-path infrared gas analyzer (LI-7500, Licor) 4 m above ground level. Each tower had a 3D sonic anemometer (WindMaster, Gill Instruments) co-located with the gas analyzer based on manufacturer recommendations. Data were collected continuously at 10 Hz and averaged to 30 min time intervals using EddyPro version 6.2.2 (LI-COR Inc., 2019). We implemented the EddyPro default double-rotation method to account for tilt in the anemometer as the terrain surrounding the towers is not complex, a block average detrending method, and compensation for density fluctuations. Quality checks were performed according to (Foken et al., 2004). Raw data were screened for spikes, amplitude resolution, drop-outs, absolute limits, skewness, and kurtosis (Vickers and Mahrt, 1997). Footprint estimation was performed according to Kljun et al. (2004). Because this footprint model is dependent on canopy height, we supplied a dynamic height file to the EddyPro software that describes the rapidly changing height of the switchgrass. Height was determined by visually inspecting PhenoCam images (Seyednasrollah et al., 2019), using a vertical PVC pipe in the field, marked every 10 cm from the ground up to 2 m. We did not include an angle of attack correction, as the available correction includes an artificial inflation of the vertical wind measurement (LI-COR Inc., 2019). The anemometer at the pine tower is subject to the w-boost issue that artificially inflating fluxes by approximately 20%. We used the approach in Billesbach et al. (2019a) to correct the raw data for the inflation.
We applied a friction velocity filtering routine to remove data with inadequate turbulence. We determined the friction velocity threshold by examining the relationship between friction velocity and net ecosystem exchange (NEE) fluxes as in Papale et al. (2006) using the REddyProc R-package (version 1.2) (Wutzler et al., 2018) for all three towers independently and filtered out data that fell below this threshold. We also filtered out observations that came from a direction or distance that did not represent the specific ecosystem.
In order to obtain annual and seasonal totals of NEE, we filled the gaps resulting from measurement interruptions or filtering with modeled values using the REddyProc R-package (version 1.2) marginal distribution sampling algorithm (Reichstein et al., 2005; Wutzler et al., 2018), a widely-used approach. This method fills data gaps by leveraging the co-variation of fluxes with meteorological variables and the temporal auto-correlation with time of day.
Albedo (α) at the three sites was calculated as the ratio of mid-day (11:00–13:00) outgoing (So) and incoming (Si) shortwave radiation and aggregated to the monthly time step.
2.2. Modeling Carbon and Albedo
To create a model describing how albedo and NEE changes with age for both ecosystem types we combined the Virginia site observations with published and publicly available EC and albedo data of pine and switchgrass ecosystems. Annual NEE values for southern pine stands were gathered from the literature, i.e., Bracho et al. (2012) and Bracho et al. (2018). Annual NEE values were available for switchgrass in the eastern United States and southern Canada from Skinner and Adler (2010); Zeri et al. (2011); Abraha et al. (2016) and Eichelmann et al. (2016). Annual albedo values for pine were obtained from this study and publicly available data on the Ameriflux repository from Oishi et al. (2018); Noormets et al. (2022); Noormets (2022) and calculated using Equation (1). Albedo values for grass were obtained from Billesbach et al. (2019b); Miller et al. (2016) and this study. We determined the age of the annual albedo and NEE values from reported ages where given, and by taking the difference between reported year of observation and year of establishment. We restricted our analysis to only include measurements in the first 30 years since establishment, a realistic harvesting age for a loblolly pine stand (Fox et al., 2007).
First, we modeled the relationship between NEE and age in both systems using a three parameter Michelis-Menten equation that included an intercept.
where C is the NEE of the ecosystem, ac is the intercept, bc is the saturation value, and hc is the age at half saturation. Superscripts p and g reference ecosystem (p = pine, g = grass). Second, we modeled albedo with a three parameter asymptotic relationship for pine ecosystems, where aα is the intercept, bα is the asymptote, and bα is proportional to the rate of increase or decrease.
Finally, albedo was modeled as a constant for the switchgrass ecosystem,
Each model included normally distributed random error (ϵ) with a mean of zero and independently estimated standard deviation for each model.
All four models were fit using a Bayesian framework with non-informative priors. The rjags package (Plummer, 2019) was used to generate the joint posterior distributions of the parameters. We ran three chains for 10,000 iterations with a 1,000 iterative burn-in and evaluated convergence using the Gelman, Rubin, and Brooks convergence diagnostic (Gelman and Rubin, 1992). Parameter estimates, 90% credible interval and the coefficient of determination of each model can be found in Supplementary Table 1. To generate posterior predictions, we generated 5,000 samples from the joint posterior distribution of the parameters, calculated the NEE and albedo annually at each age using each sample, and added randomly distributed noise based using the σ parameter associated with each model. However, radiative forcing from an albedo change is ideally calculated monthly (Bright et al., 2015). To generate monthly predictions of albedo, we calculated the average observed deviation of each month from the annual mean and applied this bias to the annual predictions described above. Each set of 5,000-sample time series for NEE and albedo was used to calculate the 95% predictive intervals (thus including both parameter uncertainty and residual error represented by ϵ) and used in the radiative forcing calculations described below.
2.3. Radiative Forcing
2.3.1. Albedo
We calculated radiative forcing using the approach described in Kirschbaum et al. (2011).
The radiative forcing from albedo first required calculating the change in albedo at the monthly scale:
where αp is the albedo of the pine stand, and αg is the albedo of the grass field, i is the year, and m is the month.
The annual radiative forcing from a change in albedo was calculated as:
where K(m) is the monthly change in shortwave absorption resulting from a 100% change in albedo. We extracted K(m) for the Virginia site from a gridded global radiative kernel product (Bright and O'Halloran, 2019). This product is a monthly assessment of the maximum radiative forcing resulting from a change in surface albedo, derived from remote sensing observations and serves as an alternative to radiative transfer models in global climate models. The kernel has been applied in other studies to calculate the albedo radiative forcing generated by, e.g., hydro-power reservoirs, bioenergy plantations, and boreal vegetation shifts under climate change (Sieber et al., 2020; Webb et al., 2021; Wohlfahrt et al., 2021).
2.3.2. Carbon
The radiative forcing of a carbon change from one square meter of ground was calculated using:
where ΔC(i) is the change in carbon balance between the pine and grass systems for year i, “21.3414” is the weight in grams of 1 ppm of carbon calculated from first principles, “5113 (m−2)” is the surface area of the earth, 400 ppm is used as a baseline CO2 concentration, “5.35” (Wm−2) is conversion factor that converts from CO2 ppm units to radiative forcing (Betts, 2000; Kirschbaum et al., 2011; O'Halloran et al., 2012). We reduced ΔC(i) by a factor of 2, roughly estimating the amount of released carbon that remains in the atmosphere annually (Cavallaro et al., 2018).
2.4. Sensitivity to Fate of Harvest
In managed ecosystems, harvested biomass represents a horizontal movement of carbon not observed by the eddy covariance systems. Depending on the magnitude, the loss of carbon via harvesting may entirely offset the gains of carbon from photosynthesis. To place logical bounds on how the fate of the harvested carbon influences the difference in climate benefits, we used four scenarios to test the assumptions about the fate of harvested carbon. Scenario A assumed that none of the harvested carbon from either ecosystem is emitted to the atmosphere. Scenario B assumed that all of the harvested pine carbon is emitted to the atmosphere, but none of the harvested switchgrass carbon is emitted. Scenario C assumed that all of the harvested switchgrass carbon is emitted to the atmosphere, but none of the harvested pine carbon is emitted. Scenario D assumed that all of the harvested carbon from both ecosystems is emitted to the atmosphere. By analyzing the bounds, it allows a realistic harvest (i.e., only a fraction of the pine is immediately burned, etc.) between our four scenarios.
The scenarios required estimates for harvested carbon from each ecosystem. Yields for both pine and switchgrass are highly variable in time and space (Parrish and Fike, 2005; Fox et al., 2007; Wear and Greis, 2013). Therefore we assume that yields cannot be predicted from NEE and instead used average expected yields for both ecosystems from literature values. For switchgrass, we used an average reported yield of 870 gm−2yr−1 (Wullschleger et al., 2010), assumed a carbon content of 42% (Eichelmann et al., 2016), which converts to 365.4 gC m−2 yr−1. We applied this harvest value to each non-establishment year of our simulated switchgrass field (excludes year 1,11, and 21 where the field is reestablished) resulting in 9,855 gC m−2 total harvested carbon. In the pine ecosystem of we assumed dry yields of 897 gm−2 yr−1 as reported in Stanturf et al. (2003), assumed a 50% carbon content (Chapin et al., 2011), which converts to 448.3 gC m−2 yr−1. This value was applied for each year of the simulated pine stand, except for the pre-establishment year (excludes year 1) for a total of 12,992 gC m−2 of harvested carbon. Together, these two values result in a 105 gC m−2 yr−1 difference in average annual yields between the pine and switchgrass.
3. Results
3.1. Observed Albedo and NEE at New Virginia Site
At the Virginia three-tower site, the mean annual NEE in the 27–30 year old loblolly pine plantation over the 4-years of measurements was −896 gC m−2 yr−1 with a range of −659 to −1,014 gC m−2 yr−1 (Table 1). The mean annual NEE in the 3–7 year old Switchgrass field was −79 gC m−2 yr−1 for the 5-years of measurement. The switchgrass included three years with positive NEE, where the switchgrass field was a source of carbon to the atmosphere. The single year of measurement at the clearcut site (representing a 0 aged pine plantation) was a carbon source, releasing 658 gC m−2 yr−1 to the atmosphere (Table 1).
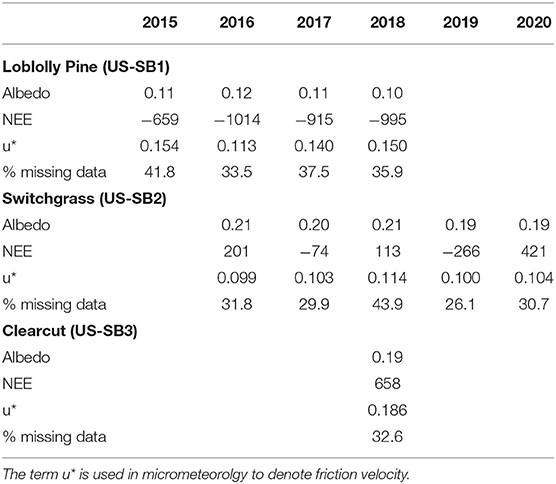
Table 1. Average annual net ecosystem exchange (NEE; gC m−2 yr−1), albedo (unit-less), friction velocity threshold (u*; s/m), and percent missing data for the pine, grass, and clearcut eddy covariance towers at Sweet Briar College.
The pine plantation had lower albedo than both grass and clearcut. The average albedo at the pine stand was 0.1 darker than the grass and clearcut (Table 1).
3.2. Models of Albedo and NEE Through Ecosystem Development
Combining the annual NEE measurements from our Virginia pine plantation with published values revealed a clear saturating relationship with stand age. The pine plantations released carbon for the first 5 years before becoming a carbon sink as the stand continues to mature to a typical rotation length of 30-years. Based on the modeled relationship, the pine plantations initially released 1041 ± 268 gC m−2 yr−1 to the atmosphere. Productivity increased quickly and the pine plantation was neutral by year 5 (Figure 1A). Productivity continued to increase each year but the rate of increase slowed over time. In year 30, pine NEE reaches it's minimum at −910 ± 237 gC m−2 yr−1.
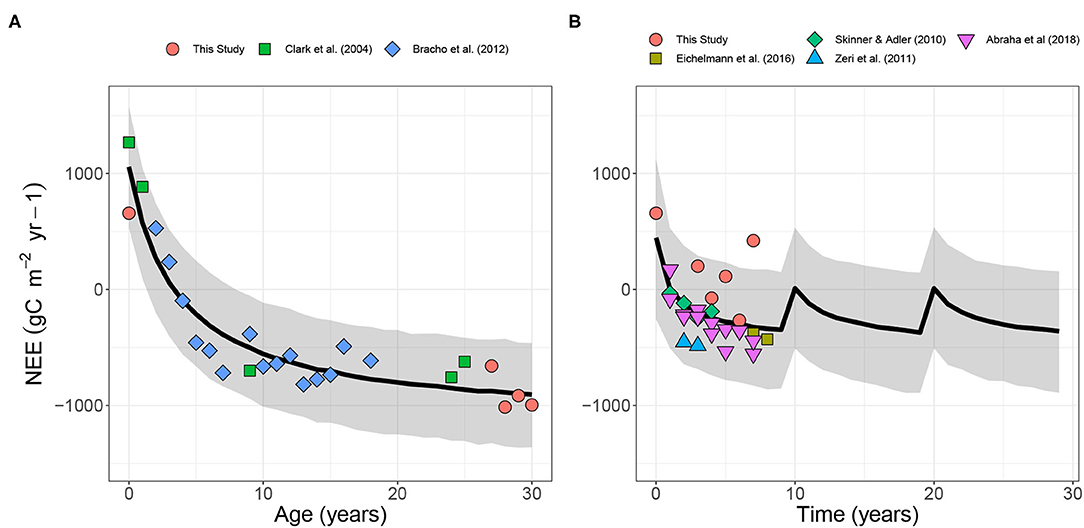
Figure 1. Annual net ecosystem exchange (NEE) for (A) Loblolly pine and (B) Switchgrass over a 30-year and 10-year rotation, respectively. Points represent annual NEE reported from this study and published values using eddy-covariance measurements. The black line represents the mean for the modeled fit. The gray shaded region represent the 95% predictive interval. Data are from this study and (Clark et al., 2004; Skinner and Adler, 2010; Zeri et al., 2011; Bracho et al., 2012; Eichelmann et al., 2016; Abraha et al., 2018) the way this is displayed implies that the data are from the cited paper.
In contrast to the pine plantation, the modeled switchgrass field quickly became a net sink of CO2 after establishment (Figure 1B). One key outlier was the Virginia site where switchgrass was a source in multiple years following establishment (Ahlswede et al., 2022). Minimum NEE was only −355 ± 263 gC m−2 yr−1.
For the purpose of modeling radiative forcing, we extended the switchgrass NEE model to age 10 and then repeated three times to provide a 30-year cycle that can be compared to the pine ecosystem's 30-year rotation. From the NEE models (Eq: 2), the pine plantation accumulated 16242 ± 1899 gC m−2 over 30-years, while the simulated switchgrass field accumulated 6671 ± 1962 gC m−2.
Similar to NEE in the pine plantation, albedo showed a clear saturating relationship with age. The age 0 stand (i.e., the year following the harvest) had an albedo of 0.17 ± 0.008 (Figure 2A). As the canopy closed, albedo declined quickly and reached half saturation at year 5. By year 20, albedo was nearly constant through age 30 at a value of 0.11 ± 0.007.
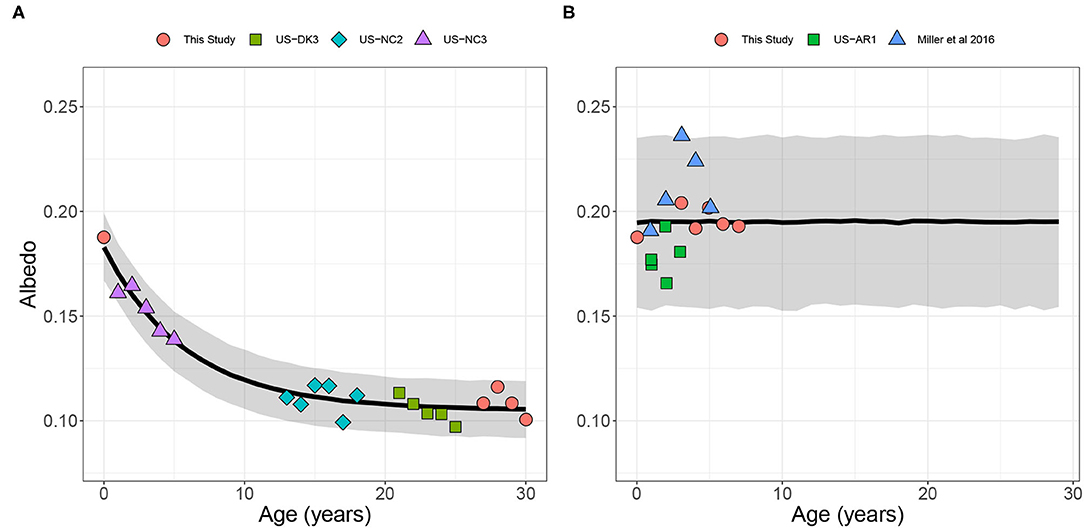
Figure 2. Albedo for (A) loblolly pine and (B) switchgrass over a 30-year and 10-year rotation, respectively. Points represent annual average albedo calculated from our site and publicly available data through Ameriflux. The black line represents the mean for the modeled fit. The gray shaded region represent the 95% predictive interval. Data are from this study and (Miller et al., 2016; Oishi et al., 2018; Billesbach et al., 2019b; Noormets et al., 2022; Noormets, 2022) the way this is displayed implies that the data are from the cited paper.
Switchgrass albedo within site showed no distinct age relationship (Figure 2B). Furthermore, the albedo was similar to the clearcut site with limited vegetation, indicating that the albedo of a newly established switchgrass field with limited vegetation would likely have similar albedo to a more mature switchgrass field. Therefore, we modeled the switchgrass field as constant through age with a mean of 0.19 ± 0.02. The wide predictive intervals reflect the variability of albedo between sites, likely due to differences in soil properties.
3.3. Radiative Forcing
Using the modeled albedo and NEE, we calculated the annual radiative forcing difference between a pine plantation and a switchgrass field. In our analysis, positive numbers indicate that the pine plantation increases energy at the top of the atmosphere. At the ecosystem scale (not including harvested carbon), the initial losses of carbon from pine plantation and the rapid growth of the switchgrass field resulted in a positive radiative forcing from the carbon balance (ΔFc) for the first 13 years, peaking in year 6 at 3.7 ± 1.8 Wm−2 (Figure 3). The ΔFc became negative in year 14 and continued to decline to −15.7 ± 4.9 Wm−2 at the end of the 30 year period.
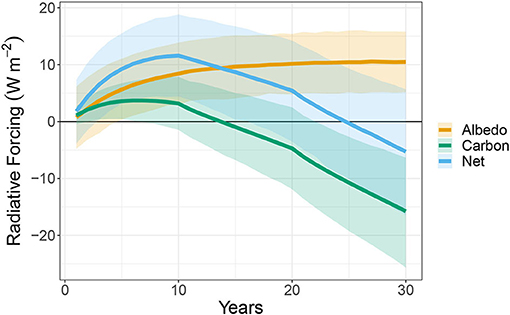
Figure 3. Modeled annual radiative forcing created by planting a pine forest relative to a switchgrass field in terms of carbon balance, albedo, and their sum. Positive values indicate that loblolly pine has a warming effect when compared to switchgrass while negative values indicate that pine has a cooling effect when compared to switchgrass. The ribbon indicates the 95% predictive interval. Scenario A is used for this estimation, where all carbon from both ecosystems is stored.
The modeled pine plantation was darker than the modeled switchgrass at all ages. As a result, the radiative forcing from a change in albedo (ΔFα) was always positive over the 30-year rotation. However, this difference was minimal in ages 0 through 5 as the higher albedo clearcut transitioned to a closed canopy forest (Figure 2). ΔFα in the first year was 0.7 ± 2.8 Wm−2 and grew to a maximum in year 30 of 10.5 ± 2.7 Wm−2 (Figure 3).
The total radiative forcing (ΔFnet) was increasingly positive early in the times series, but this effect reduced as carbon accumulated in the pine plantation (Figure 3). The ΔFnet in year 1 was 1.8 ± 2.9 Wm−2, and reached the maximum in year 10 at 11.6 ± 3.6 Wm−2. In year 25, ΔFα and ΔFc offset each other, resulting in ΔFnet centered at −0.3 ± 4.9 Wm−2. The average annual radiative forcing (ΔFnet) over the 30-year period was positive 5.3 ± 2.8 Wm−2yr−1. Based on the propagation of uncertainty derived from the Bayesian analysis, the probability of the radiative forcing being greater than 0 Wm−2 was 97.2%. This indicates that, assuming all harvested carbon not measured by the EC systems was stored (Scenario A), the negative forcing from NEP in the mature pine stand was not large enough to compensate for the loss of carbon at younger ages and the lower albedo of the pine forest (Figure 4A).
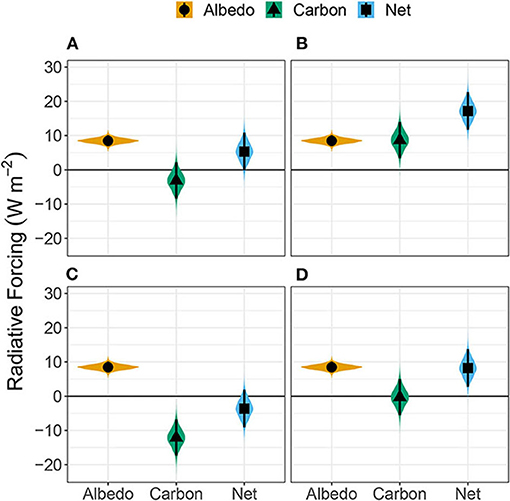
Figure 4. Radiative forcing (RF) from a change in land-cover over a 30 year period where; (A) No harvested carbon returns to the atmosphere, (B) only the pine harvest returns to the atmosphere, (C) only the grass harvest returns to the atmosphere, and (D) where harvested carbon from both systems enters the atmosphere. Here, positive values indicate that the pine system has a warming effect on climate compared to switchgrass, and negative values mean that the pine system has a cooling effect. Points represent the mean values, lines represent the 95% predictive interval, and the violin plots show the shape of the underlying distribution.
3.4. Sensitivity of Radiative Forcing to Fate of Harvest
We examined the sensitivity of ΔFc and ΔFnet to assumptions about the fate of the harvested carbon by assuming two extremes—either all harvested carbon (using literature derived estimates as described in the methods) returns to the atmosphere during the 30-year analysis period (complete combustion) or none of the harvested carbon returns to the atmosphere (complete storage). Scenario A where both systems are treated as complete storage, is reported above. In Scenario B, we assumed that all of the harvested pine carbon returned to the atmosphere while the switchgrass carbon was stored. This scenario resulted in a large positive ΔFc of 8.7 ± 2.7 Wm−2 and ΔFnet of 17.2 ± 2.8 Wm−2 (Figure 4B). In Scenario C, we assumed that none of the pine carbon returned to the atmosphere, and all of the switchgrass carbon returned over the 30-year analysis period. This scenario resulted in lower ΔFc, -12.1 ± 2.7 Wm−2 (Figure 4C). As a result the ΔFnet reversed direction (−3.6 ± 2.8Wm−2) but the probability of ΔFnet being greater than zero was 10.2%. Finally, in Scenario D, we assumed that all harvested carbon from both systems was returned to the atmosphere during the 30-year period. In this scenario, the literature-derived average annual yields were very similar for the pine plantation (448 gC m−2 yr−1), and switchgrass (365 gC m−2 yr−1). Therefore the offsetting yields lead to similar results to Scenario A (Figure 4). The average ΔFc was −0.3 ± 2.7 Wm−2 and the average ΔFnet was 8.2 ± 2.8 Wm−2. The probability of ΔFnet being greater than zero in this scenario was 99.8%.
4. Discussion
We compared the climate mitigation potential of two ecosystems in the mid-latitudes of the eastern U.S using the radiative forcing metric. First, we found that the radiative forcing from albedo and carbon associated with the establishment of a pine plantation rather than a switchgrass field were on the same order of magnitude and that the pine had a slightly net positive (warming) radiative forcing when averaged over the 30-year analysis time horizon. This finding is the result of site-level observations of NEE and albedo from eddy-covariance studies across eastern North America, including observations from a newly established cluster of towers with co-located pine, switchgrass, and clear-cut systems. Therefore, (1) our results are not specific to a single location, (2) our results include the temporal dynamics of NEE and albedo following the establishment of the managed ecosystem, and (3) the carbon radiative forcing includes both the gains of carbon through photosynthesis and the loss of carbon through autotrophic and heterotrophic respiration.
Second, we found that the fate of harvested carbon influenced the direction the overall climate forcing, whereby the slight positive radiative forcing when only considering NEE and albedo measurement either increased in magnitude or changed sign when considering harvest fate. Specifically, we examined the end members for the potential fate of harvested carbon, where we assumed that all of the carbon from either loblolly pine or switchgrass was released back into the atmosphere or held in storage over the 30-year analysis horizon. The combined 30-year mean radiative forcing was negative (i.e., net cooling by the pine plantation) in only one of the scenarios, where switchgrass harvested carbon was returned to the atmosphere (e.g., bioenergy combustion) and pine harvested carbon was stored (e.g., in wood structures) (Figure 4C). Within that scenario 10.2% of the realizations had a net positive ΔFnet, resulting from uncertainty in the NEP and albedo relationships. Overall, this highlights the importance of managing the fate of harvested carbon after it is removed from the field. In addition, it illustrates the strength of the radiative forcing from a change in albedo, even in lower latitude ecosystems. Future work can (1) refine the literature values used for harvested carbon to evaluate whether management to increase productivity and harvested carbon can alter the balance between (ΔFc) and (ΔFα), (2) evaluate how the offset of fossil fuel combustion through the use of harvested carbon as bioenergy influences the net radiative forcing outcome and (3) investigate how ecosystems can be managed for albedo in addition to carbon climate benefits.
Our results may be sensitive to some assumptions embedded in the construction of the 30-year albedo and NEE time series. First, our study assumes that the switchgrass field had a 10-year rotation before it was replanted, resulting in three 10-year rotations to compare with a 30-year pine rotation. Ideally, we would explore the implications of longer periods between switchgrass plantings, as switchgrass can remain productive for over 20 years (Parrish and Fike, 2005). However, we could not find any published studies examining the carbon balance and albedo of switchgrass fields older than 8 years. Future work should prioritize observations of switchgrass fields over 10 years of age. Second, we assumed that the starting point for each time series was the pine clear cut. This starting point did not have a strong influence on the albedo dynamics of the switchgrass since the switchgrass and clearcut albedo values were similar. However, including the clearcut as the initial starting point for the switchgrass does add a loss of carbon that may not occur if using an alternative starting point, such as a cropland. We do not expect this to change our results substantially because the same alternative starting point would be also applied to the pine ecosystem in the comparative analysis. Finally, to build the albedo and NEE models we used data from other sites that did not have co-located measurements like the Virginia site. This is was necessary to generate NEE and albedo models across the full rotation of both systems as it would be challenging to build the models using only the Virginia site. However, since the pine ecosystem observations at the Virginia site fell along the NEE curve but the switchgrass was below (thus absorbed less carbon than the other sites), an analysis that only used data from the Virginia site would shift the net radiative forcing in the negative direction (favoring pine), but provide a less general result.
Our findings differ from those reported by two other studies that use similar approaches for calculating radiative forcing of pine forests in temperate latitudes. Each of these studies found that ΔFc exceeded ΔFα with a combined negative radiative forcing by pine ecosystems (Betts, 2000; Kirschbaum et al., 2011). There are four primary causes of the differences. First, our study used a productive bioenergy grass ecosystem with carbon accumulation as the baseline rather than a carbon-neutral baseline as used in Betts (2000) and Kirschbaum et al. (2011). As a result the difference in carbon accumulation at the end of our analysis was only 70 tC ha−1 compared to 200 and 170 tC ha−1 in Betts (2000) and Kirschbaum et al. (2011), respectively. Second, our study used time-integrated NEE flux measurements from eddy-covariance sites across eastern North America to determine the (ΔFc) rather than observations of biomass change from a single site (Kirschbaum et al., 2011) or literature values of biomass and soil carbon (Betts, 2000). By using NEE to quantify carbon storage, our study also includes the loss of carbon through heterotrophic respiration. Consequently, our carbon storage rates were lower for the pine than switchgrass ecosystems early in succession, a difference that would be absent from an analysis that focused only on vegetation carbon. Third, the albedo contrast between the pine and grass systems was larger in our study (9%) compared to Betts (2000) (5%) and Kirschbaum et al. (2011) (7%). This creates a larger radiative forcing from albedo, particularly when compared to Betts (2000). Finally, each study differs in the time horizon of analysis. However, our 30-year horizon was less than the 50-year in Betts (2000) but longer than the 20-year horizon used in Kirschbaum et al. (2011), indicating that horizon is not the primary cause of the difference. Overall, the difference in carbon accumulations in both the pine and grass ecosystems is likely the most critical difference between our work and previous work.
Our analysis does not account for the indirect effects of land cover change such as a changes in evapotranspiration and biogenic volatile organic compounds (BVOC) emissions. Previous work using climate models has found that the indirect effect of evapotranspiration, via changes in planetary albedo from altered cloudiness, can offset the direct effects of a change in albedo (Bala et al., 2007; Spracklen et al., 2008; Pongratz et al., 2011; Ahlswede and Thomas, 2017). However, at the Virginia site, we found evapotranspiration was similar between the pine and switchgrass field, so changes in atmospheric water content and cloudiness should be minimal (Ahlswede, 2021). However, this result may not apply to all locations where decisions to establish pine or switchgrass occur, especially those with greater water stress. Therefore, additional work is necessary to examine the potential importance of evapotranspiration differences as a driver of indirect climate mitigation.
Furthermore, forests emit more BVOCs than crops and grasses, directly and indirectly influencing radiative forcing (Guenther et al., 2012; Unger, 2014). Forests in the SEUS are known for releasing large amounts of BVOCs, creating, for example, the famous Blue Ridge haze. The combination of BVOCs and anthropogenic oxidants in the SEUS creates high levels of secondary organic aerosol during the summer, which has a detectable cooling effect on the region (Goldstein et al., 2009). Since loblolly pine is a strong source (Stroud, 2005; Hu et al., 2015), and switchgrass a weaker source (Graus et al., 2013) of BVOCs, including the aerosol effect would potentially alter our findings by decreasing radiative forcing of the pine forest. Recent analyses in boreal forests found that biogenic aerosols contribute toward the net cooling effects of forest when weighed with carbon and albedo (Arvesen et al., 2018; Kalliokoski et al., 2020). Thus future analyses in the SEUS that weigh the net climate effects of land cover change may be more accurate if the influence of biogenic aerosols is included.
Decisions to use land-based climate solutions involve trade-offs when establishing different ecosystems for mitigation potential. Our study is a specific example that quantifies the trade-off between two managed ecosystem types in the eastern U.S. However, our conclusions likely apply to other pine plantation vs. cropland/grassland comparisons because the switchgrass albedo is typical of similar ecosystem types and our representation of uncertainty in both the NEE and albedo for the switchgrass was large enough to include variation across a wide region. Our results may not extend to a decision to establish a deciduous forest that has a higher average albedo than a pine plantation. Furthermore, forests and timber production have other indirect benefits that we did not account for here such as the substitution of timber-based building materials for emission-intensive products (O'Halloran and Bright, 2017; Kalliokoski et al., 2020). These indirect climate and ecosystem services may take higher precedence, given the small differences in the direct climate mitigation potential between the two ecosystems illustrated here. In conclusion, while the pine ecosystem absorbs more carbon than switchgrass, the difference in albedo was large enough to result in similar climate mitigation potential at the 30-year horizon between the two systems, whereby the direction and magnitude of radiative forcing depends on the fate of harvested carbon. This conclusion is a critical consideration if the expansion of either pine plantations or switchgrass is a component of a regional land-based climate mitigation strategy.
Data Availability Statement
The original contributions presented in the study are included in the article/Supplementary Material, further inquiries can be directed to the corresponding author/s.
Author Contributions
BA, TO'H, and RT conceived the analysis, conducted the measurements, and edited the article. BA performed the analyses and wrote the first draft of the article. All authors contributed to the article and approved the submitted version.
Funding
This work was supported by the USDA National Institute of Food and Agriculture (2017-68002-26612 and 2015-67003-23485) and McIntire Stennis project 1025966. We also received support from the Global Change Center and Fralin Life Sciences Institute at Virginia Tech and Clemson University Experiment Station.
Conflict of Interest
The authors declare that the research was conducted in the absence of any commercial or financial relationships that could be construed as a potential conflict of interest.
Publisher's Note
All claims expressed in this article are solely those of the authors and do not necessarily represent those of their affiliated organizations, or those of the publisher, the editors and the reviewers. Any product that may be evaluated in this article, or claim that may be made by its manufacturer, is not guaranteed or endorsed by the publisher.
Acknowledgments
The authors thank Sweet Briar College for hosting the towers, especially Linda Fink, Lisa Powell, Rich Meyer, and David Hawkins for support. We also thank SBC students Verena Joerger, Emily Dallas, Shannon Skeffington, Chelsea McKinney, Natalie Jones, and Annika Kuleba for conducting field work. We thank FDC Enterprises for sharing switchgrass harvest data and Virginia Forestry and Wildlife Group for cooperating with pine forest management. We thank James Le Moine, Michael Kline, and Joshua Rady for technical support.
Supplementary Material
The Supplementary Material for this article can be found online at: https://www.frontiersin.org/articles/10.3389/ffgc.2022.774067/full#supplementary-material
References
Abraha, M., Chen, J., Hamilton, S. K., Sciusco, P., Lei, C., Shirkey, G., et al. (2021). Albedo-induced global warming impact of Conservation Reserve Program grasslands converted to annual and perennial bioenergy crops. Environ. Res. Lett. 16, 084059. doi: 10.1088/1748-9326/ac1815
Abraha, M., Gelfand, I., Hamilton, S. K., Shao, C., Su, Y.-J., Robertson, G. P., et al. (2016). Ecosystem water-use efficiency of annual corn and perennial grasslands: contributions from land-use history and species composition. Ecosystems 19, 1001–1012. doi: 10.1007/s10021-016-9981-2
Abraha, M., Hamilton, S. K., Chen, J., and Robertson, G. P. (2018). Ecosystem carbon exchange on conversion of Conservation Reserve Program grasslands to annual and perennial cropping systems. Agric. Forest Meteorol. 253–254:151–160. doi: 10.1016/j.agrformet.2018.02.016
Ahlswede, B., and Thomas, R. Q. (2017). Community earth system model simulations reveal the relative importance of afforestation and forest management to surface temperature in eastern North America. Forests 8, 499. doi: 10.3390/f8120499
Ahlswede, B. J. (2021). Evaluating the influence of establishing pine forests and switchgrass fields on local and global climate (dissertation). Virginia Tech, Blacksburg, VA, United States.
Ahlswede, B. J., O'Halloran, T. L., Forsythe, J. D., and Thomas, R. Q. (2022). A minimally managed switchgrass ecosystem in a humid subtropical climate is a source of carbon to the atmosphere. GCB Bioenergy 14, 24–36. doi: 10.1111/gcbb.12897
Albaugh, J. M., Domec, J.-C., Maier, C. A., Sucre, E. B., Leggett, Z. H., and King, J. S. (2014). Gas exchange and stand-level estimates of water use and gross primary productivity in an experimental pine and switchgrass intercrop forestry system on the Lower Coastal Plain of North Carolina, U.S.A. Agric. Forest Meteorol. 192–193, 27–40. doi: 10.1016/j.agrformet.2014.02.013
Anderson, R. G., Canadell, J. G., Randerson, J. T., Jackson, R. B., Hungate, B. A., Baldocchi, D. D., et al. (2011). Biophysical considerations in forestry for climate protection. Front. Ecol. Environ. 9, 174–182. doi: 10.1890/090179
Anderson-Teixeira, K. J., and DeLucia, E. H. (2011). The greenhouse gas value of ecosystems. Global Change Biol. 17, 425–438. doi: 10.1111/j.1365-2486.2010.02220.x
Arvesen, A., Cherubini, F., del Alamo Serrano, G., Astrup, R., Becidan, M., Belbo, H., et al. (2018). Cooling aerosols and changes in albedo counteract warming from CO2 and black carbon from forest bioenergy in Norway. Sci. Rep. 8, 3299. doi: 10.1038/s41598-018-21559-8
Bala, G., Caldeira, K., Wickett, M., Phillips, T. J., Lobell, D. B., Delire, C., et al. (2007). Combined climate and carbon-cycle effects of large-scale deforestation. Proc. Natl. Acad. Sci. U.S.A. 104, 9911–9911. doi: 10.1073/pnas.0704096104
Bastin, J.-F., Finegold, Y., Garcia, C., Mollicone, D., Rezende, M., Routh, D., et al. (2019). The global tree restoration potential. Science 365, 76–79. doi: 10.1126/science.aax0848
Betts, R. A. (2000). Offset of the potential carbon sink from boreal forestation by decreases in surface albedo. Nature 408, 187–190. doi: 10.1038/35041545
Billesbach, D., Chan, S., Cook, D., Papale, D., Bracho-Garrillo, R., Verfallie, J., et al. (2019a). Effects of the Gill-Solent WindMaster-Pro “w-boost” firmware bug on Eddy covariance fluxes and some simple recovery strategies. Agric. Forest Meteorol. 265, 145–151. doi: 10.1016/j.agrformet.2018.11.010
Billesbach, D., Bradford, J., and Torn, M. (2019b). AmeriFlux BASE US-AR1 ARM USDA UNL OSU Woodward Switchgrass 1, Ver. 3-5. AmeriFlux AMP. doi: 10.17190/AMF/1246137
Bonan, G. B. (2008). Forests and climate change: Forcings, feedbacks, and the climate benefits of forests. Science 320, 1444–1449. doi: 10.1126/science.1155121
Bracho, R., Starr, G., Gholz, H. L., Martin, T. A., Cropper, W. P., and Loescher, H. W. (2012). Controls on carbon dynamics by ecosystem structure and climate for southeastern U.S. slash pine plantations. Ecol. Monogr. 82, 101–128. doi: 10.1890/11-0587.1
Bracho, R., Vogel, J. G., Will, R. E., Noormets, A., Samuelson, L. J., Jokela, E. J., et al. (2018). Carbon accumulation in loblolly pine plantations is increased by fertilization across a soil moisture availability gradient. Forest Ecol. Manage. 424, 39–52. doi: 10.1016/j.foreco.2018.04.029
Bright, R. M., and O'Halloran, T. L. (2019). Developing a monthly radiative kernel for surface albedo change from satellite climatologies of Earth's shortwave radiation budget: CACK v1.0. Geosci. Model Dev. 12, 3975–3990. doi: 10.5194/gmd-12-3975-2019
Bright, R. M., Zhao, K., Jackson, R. B., and Cherubini, F. (2015). Quantifying surface albedo and other direct biogeophysical climate forcings of forestry activities. Global Change Biol. 21, 3246–3266. doi: 10.1111/gcb.12951
Cavallaro, N., Shrestha, G., Birdsey, R., Mayes, M. A., Najjar, R. G., Reed, S. C., et al. (2018). Second State of the Carbon Cycle Report. Technical report, U.S. Global Change Research Program. doi: 10.7930/Soccr2.2018
Chapin, F. S., Matson, P. A., and Vitousek, P. M. (2011). Principles of Terrestrial Ecosystem Ecology. New York, NY: Springer. doi: 10.1007/978-1-4419-9504-9
Clark, K. L., Gholz, H. L., and Castro, M. S. (2004). Carbon dynamics along a chronosequence of slash pine plantations in north Florida. Ecol. Appl. 14, 1154–1171. doi: 10.1890/02-5391
Davin, E. L., and de Noblet-Ducoudré, N. (2010). Climatic impact of global-scale deforestation: radiative versus nonradiative processes. J. Clim. 23, 97–112. doi: 10.1175/2009JCLI3102.1
Devaraju, N., de Noblet-Ducoudre, N., Quesada, B., and Bala, G. (2018). Quantifying the relative importance of direct and indirect biophysical effects of deforestation on surface temperature and teleconnections. J. Clim. 31, 3811–3829. doi: 10.1175/JCLI-D-17-0563.1
Eichelmann, E., Wagner-Riddle, C., Warland, J., Deen, B., and Voroney, P. (2016). Carbon dioxide exchange dynamics over a mature switchgrass stand. GCB Bioenergy 8, 428–442. doi: 10.1111/gcbb.12259
Foken, T., Gaockede, M., Mauder, M., Mahrt, L., Amiro, B., and Munger, W. (2004). “Post-field data quality control,” in Handbook of Micrometeorology (Dodrecht: Springer), 181–208. doi: 10.1007/1-4020-2265-4_9
Fox, T. R., Jokela, E. J., and Allen, H. L. (2007). The development of pine plantation silviculture in the southern United States. J. Forest. 105, 337–347. doi: 10.1093/jof/105.7.337
Gelman, A., and Rubin, D. B. (1992). Inference from iterative simulation using multiple sequences. Stat. Sci. 7, 457–472. doi: 10.1214/ss/1177011136
Georgescu, M., Lobell, D. B., and Field, C. B. (2011). Direct climate effects of perennial bioenergy crops in the United States. Proc. Natl. Acad. Sci. U.S.A. 108, 4307–4312. doi: 10.1073/pnas.1008779108
Goldstein, A. H., Koven, C. D., Heald, C. L., and Fung, I. Y. (2009). Biogenic carbon and anthropogenic pollutants combine to form a cooling haze over the southeastern United States. Proc. Natl. Acad. Sci. U.S.A. 106, 8835–8840. doi: 10.1073/pnas.0904128106
Graus, M., Eller, A. S., Fall, R., Yuan, B., Qian, Y., Westra, P., et al. (2013). Biosphere-atmosphere exchange of volatile organic compounds over C4 biofuel crops. Atmos. Environ. 66, 161–168. doi: 10.1016/j.atmosenv.2011.12.042
Guenther, A., Jiang, X., Heald, C. L., Sakulyanontvittaya, T., Duhl, T., Emmons, L., et al. (2012). The Model of Emissions of Gases and Aerosols from Nature version 2.1 (MEGAN2. 1): an extended and updated framework for modeling biogenic emissions. Geosci. Model Dev. 5, 1471–1492. doi: 10.5194/gmd-5-1471-2012
Hansen, J., Sato, M., and Ruedy, R. (1997). Radiative forcing and climate response. J. Geophys. Res. Atmos. 102, 6831–6864. doi: 10.1029/96JD03436
Hemes, K. S., Runkle, B. R. K., Novick, K. A., Baldocchi, D. D., and Field, C. B. (2021). An ecosystem-scale flux measurement strategy to assess natural climate solutions. Environ. Sci. Technol. 55, 3494–3504. doi: 10.1021/acs.est.0c06421
Hu, X.-M., Fuentes, J. D., Toohey, D., and Wang, D. (2015). Chemical processing within and above a loblolly pine forest in North Carolina, USA. J. Atmos. Chem. 72, 235–259. doi: 10.1007/s10874-013-9276-3
Jackson, R. B., Randerson, J. T., Canadell, J. G., Anderson, R. G., Avissar, R., Baldocchi, D. D., et al. (2008). Protecting climate with forests. Environ. Res. Lett. 3, 044006–044006. doi: 10.1088/1748-9326/3/4/044006
Kalliokoski, T., Back, J., Boy, M., Kulmala, M., Kuusinen, N., Makela, A., et al. (2020). Mitigation impact of different harvest scenarios of Finnish forests that account for albedo, aerosols, and trade-offs of carbon sequestration and avoided emissions. Front. Forests Global Change 3, 562044. doi: 10.3389/ffgc.2020.562044
Kirschbaum, M. U. F., Whitehead, D., Dean, S. M., Beets, P. N., Shepherd, J. D., and Ausseil, A. G. E. (2011). Implications of albedo changes following afforestation on the benefits of forests as carbon sinks. Biogeosciences 8, 3687–3696. doi: 10.5194/bg-8-3687-2011
Kljun, N., Calanca, P., Rotach, M. W., and Schmid, H. P. (2004). A simple parameterisation for flux footprint predictions. Boundary Layer Meteorol. 112, 503–523. doi: 10.1023/B:BOUN.0000030653.71031.96
McLaughlin, S. B., and Adams Kszos, L. (2005). Development of switchgrass (Panicum virgatum) as a bioenergy feedstock in the United States. Biomass Bioenergy 28, 515–535. doi: 10.1016/j.biombioe.2004.05.006
Miller, J. N., VanLoocke, A., Gomez-Casanovas, N., and Bernacchi, C. J. (2016). Candidate perennial bioenergy grasses have a higher albedo than annual row crops. GCB Bioenergy 8, 818–825. doi: 10.1111/gcbb.12291
Noormets, A. (2022). AmeriFlux BASE US-NC3 NC_Clearcut#3, Ver. 4-5. AmeriFlux AMP. doi: 10.17190/AMF/1419506
Noormets, A., Mitra, B., Sun, G., Miao, G., King, J., Minick, K., et al. (2022). AmeriFlux BASE US-NC2 NC_Loblolly Plantation, Ver. 10-5. AmeriFlux AMP. doi: 10.17190/AMF/1246083
O'Halloran, T. L., and Bright, R. M. (2017). More diverse benefits from timber versus dedicated bioenergy plantations for terrestrial carbon dioxide removal. Environ. Res. Lett. 12, 021001. doi: 10.1088/1748-9326/aa54ec
O'Halloran, T. L., Law, B. E., Goulden, M. L., Wang, Z., Barr, J. G., Schaaf, C., et al. (2012). Radiative forcing of natural forest disturbances. Global Change Biol. 18, 555–565. doi: 10.1111/j.1365-2486.2011.02577.x
Oishi, C., Novick, K., and Stoy, P. (2018). AmeriFlux BASE US-Dk3 Duke Forest - loblolly pine, Ver. 4-5. AmeriFlux AMP. doi: 10.17190/AMF/1246048
Pacala, S. (2004). Stabilization wedges: solving the climate problem for the next 50 years with current technologies. Science 305, 968–972. doi: 10.1126/science.1100103
Papale, D., Reichstein, M., Aubinet, M., Canfora, E., Bernhofer, C., Kutsch, W., et al. (2006). Towards a standardized processing of Net Ecosystem Exchange measured with eddy covariance technique: algorithms and uncertainty estimation. Biogeosciences 3, 571–583. doi: 10.5194/bg-3-571-2006
Parrish, D. J., and Fike, J. H. (2005). The biology and agronomy of switchgrass for biofuels. Crit. Rev. Plant Sci. 24, 423–459. doi: 10.1080/07352680500316433
Pongratz, J., Reick, C. H., Raddatz, T., Caldeira, K., and Claussen, M. (2011). Past land use decisions have increased mitigation potential of reforestation. Geophys. Res. Lett. 38, 1–5. doi: 10.1029/2011GL047848
Reichstein, M., Kotterer, T., Andron, O., Ciais, P., Schulze, E.-D., Cramer, W., et al. (2005). Temperature sensitivity of decomposition in relation to soil organic matter pools: critique and outlook. Biogeosciences 2, 317–321. doi: 10.5194/bg-2-317-2005
Seyednasrollah, B., Young, A., Hufkens, K., Milliman, T., Friedl, M., Frolking, S., et al. (2019). PhenoCam Dataset v2.0: Vegetation Phenology from Digital Camera Imagery 2000–2018.
Sieber, P., Ericsson, N., Hammar, T., and Hansson, P. (2020). Including albedo in time-dependent LCA of bioenergy. GCB Bioenergy 12, 410–425. doi: 10.1111/gcbb.12682
Skinner, R. H., and Adler, P. R. (2010). Carbon dioxide and water fluxes from switchgrass managed for bioenergy production. Agric. Ecosyst. Environ. 138, 257–264. doi: 10.1016/j.agee.2010.05.008
Spracklen, D. V., Bonn, B., and Carslaw, K. S. (2008). Boreal forests, aerosols and the impacts on clouds and climate. Philos. Trans. R. Soc. A Math. Phys. Eng. Sci. 366, 4613–4626. doi: 10.1098/rsta.2008.0201
Stanturf, J. A., Kellison, R. C., Broerman, F., and Jones, S. B. (2003). Productivity of southern pine plantations: where are we and how did we get here? J. Forest. 101, 26–31. doi: 10.1093/jof/101.3.26
Stroud, C. (2005). Role of canopy-scale photochemistry in modifying biogenic-atmosphere exchange of reactive terpene species: results from the CELTIC field study. J. Geophys. Res. 110, D17303. doi: 10.1029/2005JD005775
Unger, N. (2014). Human land-use-driven reduction of forest volatiles cools global climate. Nat. Clim. Change 4, 907–910. doi: 10.1038/nclimate2347
Vickers, D., and Mahrt, L. (1997). Quality control and flux sampling problems for tower and aircraft data. J. Atmos. Ocean. Technol. 14, 512–526. doi: 10.1175/1520-0426(1997)014<0512:QCAFSP>2.0.CO;2
Vose, R. S., Applequist, S., Squires, M., Durre, I., Menne, M. J., Williams, C. N., et al. (2014). NOAA Monthly U.S. Climate Divisional Database (NClimDiv). NOAA National Climatic Data Center. doi: 10.7289/V5M32STR
Wear, D. N., and Greis, J. G. (2013). The Southern Forest Futures Project: Technical Report. Technical Report SRS-GTR-178, U.S. Department of Agriculture, Forest Service, Southern Research Station, Asheville, NC. doi: 10.2737/SRS-GTR-178
Webb, E. E., Loranty, M. M., and Lichstein, J. W. (2021). Surface water, vegetation, and fire as drivers of the terrestrial Arctic-boreal albedo feedback. Environ. Res. Lett. 16, 084046. doi: 10.1088/1748-9326/ac14ea
Wohlfahrt, G., Tomelleri, E., and Hammerle, A. (2021). The albedo-climate penalty of hydropower reservoirs. Nat. Energy 6, 372–377. doi: 10.1038/s41560-021-00784-y
Wullschleger, S. D., Davis, E. B., Borsuk, M. E., Gunderson, C. A., and Lynd, L. R. (2010). Biomass production in switchgrass across the united states: database description and determinants of yield. Agron. J. 102, 1158–1168. doi: 10.2134/agronj2010.0087
Wutzler, T., Lucas-Moffat, A., Migliavacca, M., Knauer, J., Sickel, K., Aigut, L., et al. (2018). Basic and extensible post-processing of eddy covariance flux data with REddyProc. Biogeosciences 15, 5015–5030. doi: 10.5194/bg-15-5015-2018
Keywords: loblolly pine, switchgrass, radiative forcing, natural climate solutions, land cover change
Citation: Ahlswede BJ, O'Halloran TL and Thomas RQ (2022) Combined Carbon and Albedo Climate Forcing From Pine and Switchgrass Grown for Bioenergy. Front. For. Glob. Change 5:774067. doi: 10.3389/ffgc.2022.774067
Received: 10 September 2021; Accepted: 22 March 2022;
Published: 13 May 2022.
Edited by:
Elke Eichelmann, University College Dublin, IrelandReviewed by:
Jiangong Liu, Seoul National University, South KoreaXue-Hai Fei, Guizhou University, China
Copyright © 2022 Ahlswede, O'Halloran and Thomas. This is an open-access article distributed under the terms of the Creative Commons Attribution License (CC BY). The use, distribution or reproduction in other forums is permitted, provided the original author(s) and the copyright owner(s) are credited and that the original publication in this journal is cited, in accordance with accepted academic practice. No use, distribution or reproduction is permitted which does not comply with these terms.
*Correspondence: Benjamin J. Ahlswede, YnN3ZWRlQHZ0LmVkdQ==