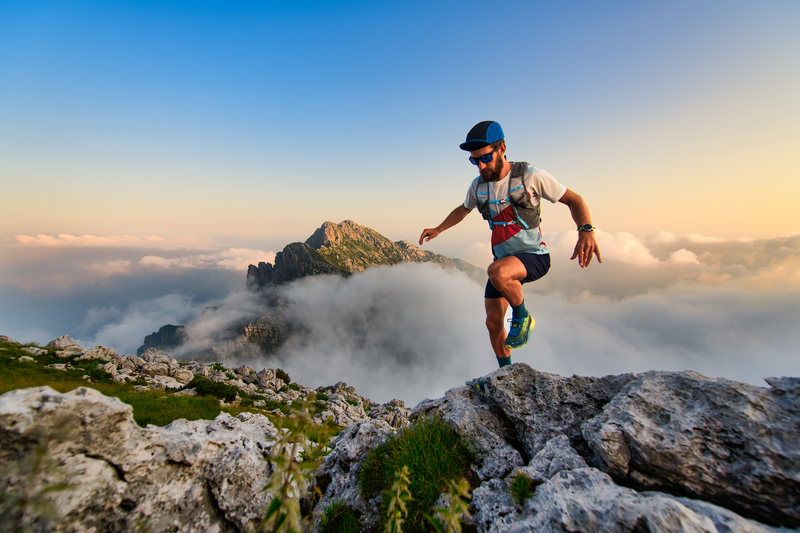
94% of researchers rate our articles as excellent or good
Learn more about the work of our research integrity team to safeguard the quality of each article we publish.
Find out more
ORIGINAL RESEARCH article
Front. For. Glob. Change , 14 February 2022
Sec. Forest Disturbance
Volume 5 - 2022 | https://doi.org/10.3389/ffgc.2022.742157
This article is part of the Research Topic Living with Tropical Storms in a Changing Climate View all 6 articles
Urban Tree Canopy (UTC) greatly enhances the livability of cities by reducing urban heat buildup, mitigating stormwater runoff, and filtering airborne particulates, among other ecological services. These benefits, combined with the relative ease of measuring tree cover from aerial imagery, have led many cities to adopt management strategies based on UTC goals. In this study, we conducted canopy analyses for 300 cities in Florida to assess the impacts of development practices, urban forest ordinances, and hurricanes on tree cover. Within the cities sampled, UTC ranged from 5.9 to 68.7% with a median canopy coverage of 32.3% Our results indicate that the peak gust speeds recorded during past hurricanes events were a significant predictor of canopy coverage (P = 0.001) across the sampled cities. As peak gust speeds increased from 152 km/h (i.e., a lower-intensity Category 1 storm) to 225 km/h (lower-intensity Category 4 and the maximum gusts captured in our data), predicted canopy in developed urban areas decreased by 7.7%. Beyond the impacts of hurricanes and tropical storms, we found that historic landcover and two out of eight urban forest ordinances were significant predictors of existing canopy coverage (P-landcover < 0.001; P-tree preservation ordinance = 0.02, P-heritage tree ordinance = 0.03). Results indicate that some local policies and tree protections can potentially impact urban tree canopy, even in the face of rapid development and periodic natural disturbances.
Urban forests have the potential to provide nature-based solutions for cities to address environmental, health, and social issues (Escobedo et al., 2019; Turner-Skoff and Cavender, 2019). These benefits or ecosystem services include, but are by no means limited to, reducing building energy usage (Ko, 2018), improving human health (Jennings and Johnson Gaither, 2015; Kuo, 2015), providing food (Kowalski and Conway, 2019), and fostering a sense of place (Blicharska and Mikusiński, 2014). Granted, the urban forest can also be a source of disservices such as increasing airborne allergens and damaging property, in addition to being costly to maintain (Fineschi and Loreto, 2020; Roman et al., 2021b). The ability and extent to which the urban forest provides these services and disservices is a function of many interacting biophysical and human legacies and factors (Figure 1; Roman et al., 2018). Extreme weather events such as tropical hurricanes or cyclones can be a source of major disturbances in tropical and coastal cities that have dramatic impacts on the urban forest (Burley et al., 2008). Urban tropical cyclone impacts have been studied at the tree (Klein et al., 2020; Koeser et al., 2020) and plot level (Burley et al., 2008; Thompson et al., 2011; Wiersma et al., 2012; Landry et al., 2021). However, research is needed at larger, city-wide scales to understand the potential interactions between tropical storms (e.g., cyclones, hurricanes, typhoons) and social-cultural factors that have helped shape the urban forest’s structure and composition. Investigating these interactions can help better predict and manage the urban forest’s vulnerabilities and ensure the continuity of its services (Steenberg et al., 2017).
The extent, structure, and composition of the urban forest can be assessed through bottom-up approaches such as on-the-ground tree inventories or through top-down approaches such as evaluating canopy cover from aerial photography or LiDAR (Leff, 2016). Maps of urban tree canopy (UTC) that are hi-resolution (<1 m) and high accuracy (=95%) can provide cost-effective, valuable information about changes in UTC over time and the potential impacts of socioeconomic drivers for very large areas (Locke et al., 2017). UTC data can be used by communities to guide goal setting, planting programs, policies, and management in order to increase the benefits provided by the urban forest (Kimball et al., 2014). For example, Endreny et al. (2017) used UTC to estimate the ecosystem services of the urban forests in 10 megacities across the world. UTC is also often used by governments and other organizations to set goals that drive large scale tree planting initiatives (Young, 2011; Nguyen et al., 2017). Remote sensing data is also a valuable tool for evaluating forest damage caused by tropical cyclones over very large areas (Wang et al., 2010). Granted, aerial UTC assessment approaches are currently limited in their ability to assess age structure and species composition (Leff, 2016) and may not necessarily reflect the condition of trees (Kenney et al., 2011). Nevertheless, this approach can provide extensive spatial and temporal data about the urban forest for researchers and managers.
Biophysical factors create the bioregional context that sets the stage for the urban forest’s composition, extent, and ability to regenerate. They are also a source of disturbances which have both immediate and lasting effects on the urban forest. When comparing between cities in the conterminous United States, climate factors such as mean winter minimum temperature (Jenerette et al., 2016) and potential evapotranspiration (Ossola and Hopton, 2018) can influence tree cover, composition, and structure. Patterns of pre-settlement vegetation (e.g., grasslands vs. forest cover) can influence the extent of the urban forest as well as patterns of regeneration (McBride and Jacobs, 1986; Fahey et al., 2012; Fahey and Casali, 2017). Insect and disease epidemics can drastically change UTC within one to two decades, with three to four decades needed to recover from tree loss (Hauer et al., 2020a). The physical landscape of a city, its terrain and features such as proximity to waterways, can influence patterns of UTC, often in combination with other socio-economic and land use factors (Davies et al., 2008; Lowry et al., 2012; Berland et al., 2015; Fahey and Casali, 2017). In one example of large-scale disturbance, tropical cyclones can have dramatic impacts on the urban forest, though these impacts can vary with other socio-demographic characteristics (Lewis et al., 2017; Van der Sommen et al., 2018; Landry et al., 2021). In the urban environment, biophysical factors are a key driver of UTC, though they clearly cannot be separated from anthropogenic legacies.
In North America, many different social, cultural, and economic variables have been shown to influence the extent of UTC and its changes over time. UTC can reflect population characteristics of a neighborhood, including race, income, and education (Locke et al., 2017; Pham et al., 2017; Gerrish and Watkins, 2018; Watkins and Gerrish, 2018) as well as formal and informal racial segregation (Grove et al., 2018; Locke et al., 2021). The particular effects of social characteristics can interact with other urban features such as housing density or the size of streets and sidewalks (Pham et al., 2017). As mentioned above, in some cases other features of the urban environment such as terrain may exert a stronger place-specific influence on tree cover that obscures socio-demographic patterns (Berland et al., 2015). Large scale economic changes that can lead to the depopulation of cities and the subsequent changes in patterns of vacant land also have the potential to alter vegetation and tree cover, though these plants may be perceived as more nuisance than amenity (Berland et al., 2020). Local policies such as planning and zoning regulations can also influence canopy cover, though the magnitude of these effects depends on the quality and type of regulation (Hilbert et al., 2019; Hauer et al., 2020b). While tree care professionals are recognized as important mediators between the environmental and social factors that influence UTC (Roman et al., 2018), the type of certification and education of these professionals alone may not be a useful predictor of canopy coverage (Hilbert et al., 2019). While many UTC studies have focused on drivers of variability within cities (but see Bigsby et al., 2014; Hilbert et al., 2019; Locke et al., 2021), research is needed to evaluate both anthropogenic and biophysical legacies together in order to understand drivers of UTC across cities, particularly in regions prone to tropical cyclones.
The state of Florida, United States, provides a useful setting for studying the interacting effects of biological and socio-cultural legacies on urban tree cover in the context of extreme weather disturbances such as tropical cyclones (referred to as hurricanes in the eastern US). As of 2010, Florida had some of the largest amounts of urban land in the US and an average of 41.8% UTC (Nowak and Greenfield, 2018). Since 2010, Florida’s population has increased 14% to a total of 21.5 million people in 2019 (U.S. Census Bureau, 2019) and its population is projected to reach approximately 33.8 million residents by 2070 (Carr and Zwick, 2016). If current development trends continue, this population growth would likely lead to increasing urban or developed land cover from 16% in 2015 to 34% of the entire state in 2070 (Carr and Zwick, 2016). Florida also periodically experiences hurricanes and tropical storms. Since 2000, the state has been impacted by 12 hurricanes (Hurricane Research Division, 2020). The state will also likely be subject to more frequent and more intense hurricanes according to high emission climate change models (Balaguru et al., 2016). Understanding large scale drivers of UTC in Florida can provide insight into managing urban forests in the face of extreme weather and continued urban expansion.
Florida is also a valuable case study because many of its municipalities have adopted various types of tree protection ordinances (e.g., Heritage Tree Ordinances, Tree Removal Permit Ordinances, etc.; Koeser et al., 2021) to preserve canopy cover in the face of development. Yet implementation of these ordinances is limited by a state law passed in 2019 (F.S. 163.045 Tree pruning, trimming, or removal on residential property, 2019). This statute prohibits municipalities from applying such ordinances to residential property owners who demonstrate that a tree poses a hazard to life or property based on the assessment of a professional arborist or landscape architect. In this context, it is clearly important to understand the relationship between municipal tree protection ordinances and UTC.
The main objective of this study was to determine what biophysical and anthropogenic factors are associated with UTC in Florida municipalities. As trees are long-lived organisms, this investigation includes historical influences such as pre-settlement land cover, past hurricanes, and development history, as well as the current state of urban forest protections (through local ordinances). Our intent is to provide managers and decision makers with the data and insights needed to make informed management and policy decisions when working toward their urban forest canopy goals.
This study investigated canopy coverage for the 300 largest cities (by population) in Florida (United States). Florida is a peninsular state that is surrounded by the Gulf of Mexico to the west, the Caribbean Sea to the south, and the Atlantic Ocean to the east. The state is predominantly classified as having a humid subtropical climate (Cfa), with the southernmost tip of the state and its keys (islands) being classified as being tropical monsoon, tropical rainforest, or tropical savanna (Am, Af, and Aw, respectively) climates (Beck et al., 2018). Florida has an annual hurricane season that begins June 1 and extends until November 30 (Florida Climate Center, 2021).
In conducting our canopy analysis, we used aerial imagery from the National Agricultural Imagery Program (NAIP; U.S. Department of Agriculture, 2019)—selecting leaf on imagery from 2019 to capture canopy conditions just prior to the passage of a Florida Statute which preempts local oversight of trees found on residential property (The 2021 Florida Statutes, 2021). The spatial resolution of the imagery was 1 m.
A random point sampling method, also known as the “dot method” (Nowak et al., 1996), was conducted to determine canopy coverage for each municipality. Boundary shapefiles for each municipality in the study were obtained from the American Community Survey (ACS; United States Census Bureau, 2015). A geographic information system (ArcGIS v. 10.2.2; ESRI, Redlands, CA, United States) was used to import NAIP aerial imagery and generate random points to evaluate UTC within each city. Each point was interpreted as “no tree,” “tree/shrub,” or “open water” by at least two interpreters per city. A minimum of 2,000, non-“open water” (e.g., lakes, rivers, and retention areas) sampling points were used to determine UTC for each city. To accomplish this objective, 2,500 random points were placed within the city boundaries and were interpreted one by one until the total number of points labeled “no tree” and “tree/shrub” totaled 2,000. Canopy percentage and agreement between interpreters were noted for each municipality.
We derived historic land cover classifications from the natural vegetation map created by Davis (1967). Specifically, we accessed a digitized version of the map through the Florida Geographic Data Library (Table 1) and aggregated land cover types using the categories specified by Volk et al. (2017). While generally limited to description of historic vegetation cover, Davis’s map did delineate the footprint of 11 cities (i.e., Daytona Beach, Jacksonville, Lakeland, Orlando, Miami, Pensacola, St. Augustine, St. Petersburg, Tampa, Vero Beach, and West Palm Beach). For these municipalities (categorized as “urban” in the 1967 map), we used the surrounding land cover for our model predictor.
We accessed hurricane wind field data from the “disasters” repository housed at GeoPlatform.gov. Specifically, we looked at recent hurricanes that made landfall in Florida and were severe enough to become a Federal Emergency Management Agency declared disaster and had wind field data available. Storms included in our analysis were:
• Hurricane Dorian (Geoplatform.gov, 2019)
• Hurricane Michael (Geoplatform.gov, 2018)
• Hurricane Irma (Geoplatform.gov, 2017)
To determine the extent to which cities were impacted by past hurricanes, we overlaid our city boundary layers with the wind field layers noted above. For cities with only one weather station, the maximum recorded wind gust speed for that station was used. For larger cities with more than one station within their boundaries, the average of the recorded peak gusts was calculated prior to analysis. For smaller cities where a weather station was not present, the maximum gust values from the nearest station were used. If a city was hit by more than one hurricane event, we used the peak gust values from the more intense storm in our predictive model (Table 1).
Additionally, we sourced population, median income, population density, and percent of houses built 10, 20, and 30 years ago from the US Census Bureau (Table 1). Housing density was calculated manually based on the area of our city boundaries and the 2018 population projections (U.S. Census Bureau, 2018a).
Finally, as noted in detail in Koeser et al. (2021), we used a multi-mode email survey to communities and a systematic search of online resources to determine which municipal tree protection ordinances were in place within the 300 assessed communities prior to our canopy analysis. The online resources referenced included two municipal code databases1,2 as well as individual municipal websites. The ordinances of interest with regard to their potential impact on canopy coverage are listed in Table 1. We coded each as being present or absent (i.e., yes or no binary) prior to our data analysis.
We analyzed our data using linear regression with percent canopy coverage as the response variable (Table 1). All analyses were conducted in R (R Core Team, 2020). To guide model building, we ran the regsubsets() function from the leaps package (Lumley and Miller, 2017) and plotted (by R2 value) the 20 best subsets of our full set of predictor variables (Table 1; see Hilbert et al., 2019). We then ran a maximal model with all predictor variables using the lm() function (R Core Team, 2020). From this, we employed a one-at-a-time simplification strategy—removing non-significant predictors from our initial model based on P-value and the regsubsets() plot. With each iteration of simplification, we compared changes in overall fit between the original and simplified models using the anova() function in R (Crawley, 2013). Once all of the non-significant terms had been removed in this manner, we retested a few variables that had commonly been associated with high R2 models in our subset plot, as well as potentially meaningful two-way interactions, to see if improvements to model fit could be detected.
In model building we adopted a P-value of 0.05 as our threshold for statistical significance. The underlying assumptions of our linear model were assessed visually using Q-Q plots (i.e., normality of residuals) and residual scatter plots (i.e., homogeneity) to assure that they were not being violated. Additionally, residual plots were used to assess the presence or absence of high-leverage outliers (based on Cook’s distance). Finding none, we adopted the resulting simplified model for our results and discussion.
The cities included in this study sample ranged from Florida’s largest municipality, Jacksonville (population 903,889) to Sneads, a small community of 1798 (Supplementary Table 1). The median population for our sampled cities was 12,678. The cities in our study (combined population of 10,629,924) were home to approximately 50% of the state’s 2018 population. Cities ranged in area from 2,265.3 km2 (Jacksonville) to 0.8 km2 (Virginia Gardens).
Population densities ranged from 8 people per kilometer for Bunnell (a small community with a wildlife refuge within its boundary) to 8,618 people per square kilometer for North Bay Village (a chain of highly developed and largely man-made islands in the Miami-Dade metropolitan area; Figures 2, 3). The median population density for our sampled cities was 814 people per square kilometer (Figures 2, 3). Median household incomes ranged from $17,908 in the city of Opa-locka to $154,415 for the village of Pinecrest (Figure 3). Both municipalities are located in the Miami-Dade metropolitan area within 39 km of each other.
Figure 2. Population density (A), urban tree cover (B), maximum hurricane gust speed (C), and historic landcover (D) among the 300 Florida (United States) municipalities included in this study. Historic landcover is a reprinting of the pre-settlement map published by Volk et al. (2017). Image used with permission.
Figure 3. Distributions for the continuous variables used to model canopy coverage in the 300 largest municipalities in Florida, United States. Includes the response variable, developed urban tree canopy [developed urban tree canopy (UTC)].
The majority (72%) of our cities were built on land that had formerly been wooded (Figure 2). Of these cities, 31% were developed in areas of scrub and sandhill. Another 29% of communities were built in areas that had historically sustained mesic pinelands. A smaller proportion of communities were developed in former upland hardwood forests (5%) or forested wetlands (7%; Figure 2). Of the cities established in non-forested regions, 21% were former uplands and 7% were former wetlands (Figure 2).
Six of the eight tree ordinances evaluated as part of this study were adopted by more than half of the study cities (Table 2). The most commonly adopted tree ordinances in the surveyed cities included Planting requirements—new development (89.3%) and Planting requirements—parking lots (89.3%) followed by Tree preservation ordinance (86.7%) and Ability to fine (84.0%; Table 2). Local licensure was the least commonly adopted ordinance (17.7%; Table 2).
Across the 300 cities sampled in Florida, UTC ranged from 5.9% in Redington Shores to 68.7% in Sanibel, respectively. The median UTC for our sample was 32.3% (Figures 3, 4). Summary statistics for developed UTC (i.e., UTC for the inhabited census blocks in a municipality), which is what we used in our modeling efforts as it excludes forest preserves and wildlife areas that might inflate city-wide canopy levels, were nearly identical to those for straight UTC (Figures 3, 4).
Figure 4. Distribution of municipal percent urban tree canopy (UTC). The vertical solid black line represents the median UTC value. The two dashed lines represent the 25th percentile (left) and 75th percentile (right).
Of the 16 variables initially modeled, we retained five for our final, simplified model (adjusted R2 = 0.230). They included historic land cover (aggregated more simply as forested vs. non-forested after initial exploratory modeling showed this was the main delineation), maximum hurricane gust speed, population density, the presence of a tree preservation ordinance, and the presence of a heritage tree ordinance (Table 3). Of these, increases in population density and increases in hurricane gust wind speeds were associated with decreases in developed UTC (Figure 2 and Table 3). Similarly, non-forested historic land cover and the presence of a tree preservation ordinance were negatively associated with developed UTC (Table 3). Only the presence of a heritage tree ordinance (70% of cities) was positively associated with developed UTC. Median income, housing age, home ownership, and the other six ordinances were not included in the final model given non-significance.
Table 3. Final model and regression results in predicting developed urban tree canopy (UTC) for the 300 most populous municipalities in Florida, United States (adjusted R2 = 0.230).
Our analysis of 300 cities in Florida demonstrated that both natural and anthropogenic legacies can influence UTC at the city scale. Overall, this finding is in line with observations within other U.S. cities such as Toledo, OH (Berland et al., 2015), Philadelphia, PA (Roman et al., 2021a), Chicago, IL (Fahey et al., 2012), and Baltimore, MD (Grove et al., 2018), among others (Roman et al., 2018). This analysis of Florida’s largest cities is an important addition to this body of research because our study included an examination of hurricanes. Considering that climate change is predicted to increase the intensity and intensification of hurricanes and other tropical cyclones (Bhatia et al., 2018), it is imperative to understand how these extreme weather events can influence UTC in regions vulnerable to this type of extreme weather.
Our analysis demonstrated the impact of hurricanes across Florida on the urban forest, though hurricane impacts can be lessened or exacerbated by other factors. Other research in Florida has observed that wind resistance varies among tree species and is also affected by pruning practices (Duryea et al., 2007a,b). Additionally, in Florida larger trees were more likely to fail during a hurricane while neighboring trees and structures appear to offer little protection from high winds (Landry et al., 2021). Escobedo et al. (2009) observed interacting effects between wind speed, tree cover, and urban land cover on debris generation in Florida following the 2004 and 2005 hurricane seasons. Hurricane related tree losses also varied by land use type following Hurricane Ike in Texas, United States (Staudhammer et al., 2011). However, Thompson et al. (2011) found that urban forest structure was a better predictor of hurricane woody debris generation rather than hurricane related variables such as wind speed. Further research is needed to understand the extent to which hurricane associated tree loss is a direct result of hurricane damage vs. tree removals driven by property owner responses to extreme weather. Clearly, hurricane severity can have a significant impact on the urban forest, though understanding the influence of other factors such as pruning and species selection can help to moderate these impacts (Duryea et al., 2007a,b).
The influence of historic vegetation cover on current UTC reflects both the influence of biogeographical constraints on tree growth and the contributions of remnant forest patches. Florida has fairly high average rainfall across the state ranging from 102 to 178 cm annually (Florida Climate Center, 2021) and a distinct dry season (Misra and Mishra, 2016). This dry season and typically sandy soils with low water holding capacity (Kern, 1995) may constrain tree establishment. Indeed, irrigation can improve tree establishment and condition for tree plantings in the state (Koeser et al., 2014; Blair et al., 2019). In environments with low average annual rainfall, UTC can increase in historical non-forest habitats during urbanization as management activities overcome water limitations (McBride and Jacobs, 1986; Nowak, 2012). Given the presence of treed cities in areas that historically were non-forested, it appears management has played a role in shaping the current urban forest of Florida. That said, cities established in former forested areas did have higher UTC percentages (Fig 2). Similar to our findings, the positive association between historical forest habitats and higher UTC suggests remnant forest habitats likely play a key role in contributing to cover as observed in Chicago, Illinois, United States (Fahey et al., 2012). This finding suggests that protecting remnant forests from encroaching development or densification would be an important strategy for maintaining urban tree cover.
The negative relationship between population density and UTC (Figure 2) in Florida at the coarse spatial resolution used in this study was fairly unsurprising and consistent with other research (Bigsby et al., 2014; Locke et al., 2016; Fahey and Casali, 2017). Presumably as a city’s human population grows the extent of infrastructure such as buildings and roads increase, consequently reducing tree canopy. Similarly, increases in impervious cover (Davies et al., 2008) and housing density (Iverson and Cook, 2000; Hilbert et al., 2019) are also associated with decreased urban tree cover. When examining anthropogenic drivers of urban tree cover within cities, socio-demographic factors such as race, income, public policies, and local history may be more useful predictors at finer resolutions (Gerrish and Watkins, 2018; Roman et al., 2018; Watkins and Gerrish, 2018; Locke et al., 2021). However, Nesbitt et al. (2019) observed education level and income were important predictors of tree cover across cities. For regions with expected population increases such as Florida (Carr and Zwick, 2016), special attention should be paid to protecting tree cover as urban populations and development increase.
While municipal ordinances are one method for protecting the urban tree canopy, the contrasting relationships between different types of ordinances and UTC in this study likely suggests that simply having an ordinance is not sufficient for maintaining or increasing canopy cover. Hilbert et al. (2019) observed that across 43 Florida cities, heritage tree ordinances were also associated with greater urban tree cover. This type of ordinance may be particularly effective because they are designed to protect larger trees which contribute to more cover. Or alternatively, communities which already have substantial urban tree cover may adopt such an ordinance to protect what they already have. Landry and Pu (2010) observed properties in Tampa, FL built after the adoption of a tree protection ordinance had higher UTC compared to properties in nearby communities without such an ordinance. By contrast, our analysis of 300 cities in Florida observed a negative association between the adoption of a tree protection ordinance and urban tree cover. This is not to say that tree protections caused a decrease in canopy coverage. Rather, a protective ordinance could have been enacted as a response to a noticeably diminishing UTC. Moreover, it is unclear how much time must elapse between the adoption of any tree related ordinance and the observation of meaningful improvements in UTC. If a community already has a comparatively lower amount of tree cover to begin with, a tree protection ordinance at best would maintain that level of cover rather than producing dramatic increases. Additionally, an ordinance can only be effective if it is enforced. We lacked the data to differentiate between cities that actively enforced their protection ordinances and those lacking the resources or political will to do so.
Support for and knowledge of municipal tree ordinances can be highly variable. In a survey of Canadian communities, Conway and Bang (2014) observed that while residents had generally neutral to favorable attitudes about the urban forest, they expressed less support for particular policies. Knowledge of and support for municipal tree bylaws in the Greater Toronto Area tended to be higher among residents with higher levels of formal education and residents who were born in Canada (Conway and Lue, 2018). Zhang et al. (2007) also observed differences in attitudes toward financing urban tree programs along socio-demographic lines, where individuals with young families and individuals younger than 56 were more likely to feel that financing urban forestry initiatives is the government’s responsibility. In their survey of Florida municipalities after the passage of a bill that limits municipal implementation of tree protection ordinances, Koeser et al. (2021) observed that some municipalities have positive relationships with the public and tree care companies and enjoy support for their programs while other municipalities have more counter-productive relationships with the local tree care industry. The adoption and enforcement of municipal tree ordinances not only directly impacts urban tree cover, but they can also be another mechanism by which a city’s socio-demographic characteristics and culture can influence the urban forest.
Photointerpretation as a method to evaluate urban tree canopy has its limitations (O’Neil-Dunne et al., 2014; Locke et al., 2017), though it is a proven and affordable approach to land classification used in the urban forestry field (Nowak et al., 1996; Walton et al., 2008; Morgan et al., 2010). To assess accuracy, a 10% subset of points for each city was assed by two photo interpreters. Of the 300 cities in the study, 82% had interpreter agreement greater than 90% with agreement for the remaining cities ranging between 80 and 90% (Supplementary Table 1). UTC is only one metric of the condition of the urban forest, consequently this study’s findings cannot offer insight into drivers of other characteristics such as diversity and age structure (Leff, 2016). However, our findings offer interesting avenues to investigate drivers which may affect these other characteristics of the urban forest that are more time and labor intensive to measure.
Moreover, the overall predictive power of our model was low, accounting for only about a quarter of the variability seen in canopy coverage among the communities studied (adjusted R2 = 0.230). While our work does attempt to look at how historic legacies related to landcover and storms can shape the current state of urban forests, we lacked long-term data to assess how management differences over time affected canopy coverage. Moreover, while the state’s dominant urban tree species have largely been spared from the noxious pest and disease outbreaks, several palm species and understory native trees common to wooded remnant forests have not been so lucky. This was not captured in our model.
We tested the effects of 16 biophysical and anthropogenic factors on UTC across 300 cities in Florida, US. Florida provided a valuable test case for this study because it has been affected by many tropical cyclones which can be an important cause of disturbance in coastal urban forests (Burley et al., 2008). Our analysis found both biophysical (historic land cover and hurricane maximum wind gust) and anthropogenic (population density, heritage tree ordinances, and tree protection ordinances) factors explained the most variation in UTC across cities in Florida. Interestingly, while heritage tree ordinances were associated with greater canopy cover, tree protection ordinances were associated with lower canopy cover. The negative correlation between hurricane maximum gust and canopy demonstrates how tropical cyclones can have lasting impacts on the urban forest. This finding has important implications in the context of climate change and potentially increasing tropical cyclone intensity (Balaguru et al., 2016) and emphasizes the importance of considering tropical cyclone impacts in urban forestry planning and management in coastal regions.
The raw data supporting the conclusions of this article will be made available by the authors, without undue reservation.
AK, RH, DH, AA-E, MA, KB, SL, ML, and JM: conceptualization. AK, RH, DH, AA-E, SL, and JM: methodology. AK, DH, and HT: validation and data curation. AK: formal analysis and project administration and visualization. AS and AK: writing–original draft. AK, RH, DH, AA-E, MA, KB, SL, ML, JM, and HT: writing–review and editing. AK and DH: supervision. AK, AA-E, MA, KB, SL, and ML: funding. All authors contributed to the article and approved the submitted version.
Funding for the research was provided by the Florida Forest Service and the University of Florida Center for Land Use Efficiency (CLUE). Matching funds (50%) for the article publishing charges provided by the University of Florida Gulf Coast Research and Education Center.
The authors declare that the research was conducted in the absence of any commercial or financial relationships that could be construed as a potential conflict of interest.
All claims expressed in this article are solely those of the authors and do not necessarily represent those of their affiliated organizations, or those of the publisher, the editors and the reviewers. Any product that may be evaluated in this article, or claim that may be made by its manufacturer, is not guaranteed or endorsed by the publisher.
We thank Saige Middleton and Brooke Anderson for conducting photographic interpretation. We also thank Drew McLean for making additional contributions to this work.
The Supplementary Material for this article can be found online at: https://www.frontiersin.org/articles/10.3389/ffgc.2022.742157/full#supplementary-material
American Legal Publishing Corporation (2018). America’s Codification Professionals. Available online at http://www.amlegal.com/ (accessed November 26, 2018).
Balaguru, K., Judi, D. R., and Leung, L. R. (2016). Future hurricane storm surge risk for the U.S. gulf and Florida coasts based on projections of thermodynamic potential intensity. Clim. Change 138, 99–110.
Beck, H., Zimmermann, N., McVicar, T., Vergopolan, N., Berg, A., and Wood, E. F. (2018). Present and future K ppen-Geiger climate classification maps at 1-km resolution. Sci. Data 5:180214. doi: 10.1038/sdata.2018.214
Berland, A., Locke, D. H., Herrmann, D. L., and Schwarz, K. (2020). Beauty or blight? Abundant vegetation in the presence of disinvestment across residential parcels and neighborhoods in Toledo, OH. Front. Ecol. Evol. 8:566759. doi: 10.3389/fevo.2020.566759
Berland, A., Schwarz, K., Herrmann, D. L., and Hopton, M. E. (2015). How environmental justice patterns are shaped by place: terrain and tree canopy in cincinnati, Ohio, USA. Cities Environ. 8:1.
Bhatia, K., Vecchi, G., Murakami, H., Underwood, S., and Kossin, J. (2018). Projected response of tropical cyclone intensity and intensification in a global climate model. J. Clim. 31, 8281–8303.
Bigsby, K. M., McHale, M. R., and Hess, G. R. (2014). Urban morphology drives the homogenization of tree cover in Baltimore, MD, and Raleigh, NC. Ecosystems 17, 212–227. doi: 10.1007/s10021-013-9718-4
Blair, S. A., Koeser, A. K., Knox, G. W., Roman, L. A., Thetford, M., and Hilbert, D. R. (2019). Health and establishment of highway plantings in Florida. Urban For. Urban Greening 43:126384. doi: 10.1371/journal.pone.0095691
Blicharska, M., and Mikusiński, G. (2014). Incorporating social and cultural significance of large old trees in conservation policy. Conserv. Biol. 28, 1558–1567. doi: 10.1111/cobi.12341
Burley, S., Robinson, S. L., and Lundholm, J. T. (2008). Post-hurricane vegetation recovery in an urban forest. Landsc. Urban Plan. 85, 111–122.
Carr, M., and Zwick, P. D. (2016). Florida 2070 Mapping Florida’s Future - Alternative Patterns of Development in 2070. Technical Report. 1000. Tallahassee, FL: Friends of Florida.
Conway, T. M., and Bang, E. (2014). Willing partners? Residential support for municipal urban forestry policies. Urban For. Urban Greening 13, 234–243. doi: 10.1016/j.ufug.2014.02.003
Conway, T. M., and Lue, A. (2018). Resident knowledge and support for private tree by-laws in the Greater Toronto Area. Arboric. Urban For. 44, 185–200.
Davis, J. H. (1967). General Map of Natural Vegetation of Florida. Gainesville: University of Florida Circular
Davies, R. G., Barbosa, O., Fuller, R. A., Tratalos, J., Burke, N., Lewis, D., et al. (2008). City-wide relationships between green spaces, urban land use and topography. Urban Ecosyst. 11:269.
Duryea, M. L., Kampf, E., and Littell, R. C. (2007a). Hurricanes and the urban forest: I. Arboric. Urban For. 33, 83–97.
Duryea, M. L., Kampf, E., Littell, R. C., and Rodríguez-Pedraza, C. D. (2007b). Hurricanes and the urban forest: II. Effects on tropical and subtropical tree species. Arboric. Urban For. 33, 98–112.
Endreny, T., Santagata, R., Perna, A., Stefano, C. D., Rallo, R. F., and Ulgiati, S. (2017). Implementing and managing urban forests: a much needed conservation strategy to increase ecosystem services and urban wellbeing. Ecol. Modell. 360, 328–335. doi: 10.1016/j.ecolmodel.2017.07.016
Escobedo, F. J., Giannico, V., Jim, C. Y., Sanesi, G., and Lafortezza, R. (2019). Urban forests, ecosystem services, green infrastructure and nature-based solutions: nexus or evolving metaphors? Urban For. Urban Greening 37, 3–12.
Escobedo, F. J., Luley, C. J., Bond, J., Staudhammer, C., and Bartel, C. (2009). Hurricane debris and damage assessment for Florida urban forests. Arboric. Urban For. 35, 100–106.
Fahey, R. T., Bowles, M. L., and Mc Bride, J. L. (2012). Origins of the Chicago Urban Forest: composition and Structure in Relation to Presettlement Vegetation and Modern Land Use. Arboric. Urban For. 38, 181–193. doi: 10.48044/jauf.2012.027
Fahey, R. T., and Casali, M. (2017). Distribution of forest ecosystems over two centuries in a highly urbanized landscape. Landsc. Urban Plan. 164, 13–24. doi: 10.1016/j.landurbplan.2017.03.008
Fineschi, S., and Loreto, F. (2020). A Survey of Multiple Interactions Between Plants and the Urban Environment. Front. For. Global Change 3:30. doi: 10.3389/ffgc.2020.00030
Florida Geographic Data Library (1999). Florida Vegetation Map - 1967. Available online at https://download.fgdl.org/pub/state/vcom67.zip
The 2021 Florida Statutes (2021). Tree Pruning, Trimming, or Removal on Residential Property. Available online at: http://www.leg.state.fl.us/statutes/index.cfm?App_mode=Display_Statute&Search_String=&URL=0100-0199/0163/Sections/0163.045.html
Gerrish, E., and Watkins, S. L. (2018). The relationship between urban forests and income. Landsc. Urban Plan. 170, 293–308. doi: 10.1016/j.landurbplan.2017.09.005
Geoplatform.gov (2017). Disasters Public Files. Available online at: https://disasters.geoplatform.gov/publicdata/NationalDisasters/2017/HurricaneIrma/ (accessed July 12, 2020).
Geoplatform.gov (2018). Disasters Public Files. Available online at: https://disasters.geoplatform.gov/publicdata/NationalDisasters/2018/HurricaneMichael/ (accessed July 12, 2020).
Geoplatform.gov (2019). Disasters Public Files. Available online at: https://disasters.geoplatform.gov/publicdata/NationalDisasters/2019/HurricaneDorian_August2019/ (accessed July 12, 2020).
Grove, M., Ogden, L., Pickett, S., Boone, C., Buckley, G., Locke, D. H., et al. (2018). The Legacy Effect: understanding How Segregation and Environmental Injustice Unfold over Time in Baltimore. Ann. Assoc. Am. Geogr. 108, 524–537.
Hauer, R. J., Hanou, I. S., and Sivyer, D. (2020a). Planning for active management of future invasive pests affecting urban forests. Urban Ecosystems 23, 1005–1022. doi: 10.1007/s11252-020-00976-6
Hauer, R., Koeser, A., Parbs, S., Kringer, J., Krouse, R., Ottman, K., et al. (2020b). Effects of a tree preservation program on tree survival, condition, and growth in Milwaukee, WI, USA. Landsc. Urban Plan. 193:103670. doi: 10.1016/j.landurbplan.2019.103670
Hilbert, D. R., Koeser, A. K., Roman, L. A., Hamilton, K., Landry, S. M., Hauer, R. J., et al. (2019). Development practices and ordinances predict inter-city variation in Florida urban tree canopy coverage. Landsc. Urban Plan. 190:103603. doi: 10.1016/j.landurbplan.2019.103603
Hurricane Research Division (2020). Detailed List of Continental United States Hurricane Impacts/Landfalls 1851-1965, 1983-2019. Available online at: https://www.aoml.noaa.gov/hrd/hurdat/UShurrs_detailed.html (accessed May 7, 2021).
Iverson, L. R., and Cook, E. A. (2000). Urban forest cover of the Chicago region and its relation to household density and income. Urban Ecosyst. 4, 105–124.
Jenerette, G. D., Clarke, L. W., Avolio, M. L., Pataki, D. E., Gillespie, T. W., Pincetl, S., et al. (2016). Climate tolerances and trait choices shape continental patterns of urban tree biodiversity. Glob. Ecol. Biogeogr. 25, 1367–1376.
Jennings, V., and Johnson Gaither, C. (2015). Approaching environmental health disparities and green spaces: an ecosystem services perspective. Int. J. Environ. Res. Public Health 12, 1952–1968. doi: 10.3390/ijerph120201952
Kenney, W. A., van Wassenaer, P. J. E., and Satel, A. L. (2011). Criteria and indicators for strategic urban forest planning and management. Arboric. Urban For. 37, 108–117. doi: 10.48044/jauf.2011.015
Kern, J. S. (1995). Geographic patterns of soil water-holding capacity in the contiguous United States. Soil Sci. Soc. Am. J. 59, 1126–1133. doi: 10.2136/sssaj1995.03615995005900040026x
Kimball, L. L., Wiseman, P. E., Day, S. D., and Munsell, J. F. (2014). Use of urban tree canopy assessments by localities in the chesapeake bay watershed. Cities Environ. 7:9.
Klein, R. W., Koeser, A. K., Kane, B., Landry, S., Shields, H., Lloyd, S., et al. (2020). Evaluating the likelihood of tree failure in Naples, Florida (United States) following Hurricane Irma. Forests 11:485. doi: 10.3390/f11050485
Koeser, A. K., Gilman, E. F., Paz, M., and Harchick, C. (2014). Factors influencing urban tree planting program growth and survival in Florida, United States. Urban For. Urban Greening 13, 655–661.
Koeser, A. K., Hauer, R. J., Downey, E. E., Hilbert, D. R., McLean, D. C., Andreu, M. G., et al. (2021). Municipal response to state legislation limiting local oversight of private urban tree removal in Florida. Land Use Policy 105:105398. doi: 10.1016/j.landusepol.2021.105398
Koeser, A. K., Smiley, E. T., Hauer, R. J., Kane, B., Klein, R. W., Landry, S., et al. (2020). Can professionals gauge likelihood of failure? - Insights from Hurricane Matthew. Urban For. Urban Greening. 52:126701. doi: 10.1016/j.ufug.2020.126701
Kowalski, J. M., and Conway, T. M. (2019). Branching out: the inclusion of urban food trees in Canadian urban forest management plans. Urban For. Urban Greening 45:126142.
Ko, Y. (2018). Trees and vegetation for residential energy conservation: a critical review for evidence-based urban greening in North America. Urban For. Urban Greening 34, 318–335. doi: 10.1016/j.ufug.2018.07.021
Kuo, M. (2015). How might contact with nature promote human health? Front. Psychol. 6:1093. doi: 10.3389/fpsyg.2015.01093
Landry, S. M., Koeser, A. K., Kane, B., Hilbert, D. R., McLean, D. C., Andreu, M., et al. (2021). Urban forest response to Hurricane Irma: the role of landscape characteristics and sociodemographic context. Urban For. Urban Greening 61:127093. doi: 10.1016/j.ufug.2021.127093
Landry, S. M., and Pu, R. (2010). The impact of land development regulation on residential tree cover: an empirical evaluation using high-resolution IKONOS imagery. Landsc. Urban Plan. 94, 94–104. doi: 10.1016/j.landurbplan.2009.08.003
Leff, M. (2016). The Sustainable Urban Forest: A Step-by-Step Approach. Philadelphia: United Sates Department of Agriculture Forest Service.
Lewis, J. A., Zipperer, W. C., Ernstson, H., Bernik, B., Hazen, R., Elmqvist, T., et al. (2017). Socioecological disparities in New Orleans following Hurricane Katrina. Ecosphere 8:e01922.
Locke, D. H., Hall, B., Grove, J. M., Pickett, S. T. A., Ogden, L. A., Aoki, C., et al. (2021). Residential housing segregation and urban tree canopy in 37 US Cities. npj Urban Sustain. 1:15. doi: 10.1038/s42949-021-00022-0
Locke, D. H., Landry, S. M., Morgan Grove, J., and Chowdhury, R. R. (2016). What’s scale got to do with it? J. Urban Ecol. 2:juw006. doi: 10.1093/jue/juw006
Locke, D. H., Romolini, M., Galvin, M., O’Neil-Dunne, J. P. M., and Strauss, E. G. (2017). Tree Canopy Change in Coastal Los Angeles, 2009 - 2014. Cities Environ 10, 2009–2014.
Lowry, J. H. Jr., Baker, M. E., and Ramsey, R. D. (2012). Determinants of urban tree canopy in residential neighborhoods: household characteristics, urban form, and the geophysical landscape. Urban Ecosyst. 15, 247–266. doi: 10.1007/s11252-011-0185-4
Lumley, T., and Miller, A. (2017). Leaps: Regression Subset Selection. Available online at: https://CRAN.Rproject.org/package=leaps
McBride, J. R., and Jacobs, D. F. (1986). Presettlement forest structure as a factor in urban forest development. Urban Ecol. 9, 245–266. doi: 10.1016/0304-4009(86)90003-3
Misra, V., and Mishra, A. (2016). The oceanic influence on the rainy season of Peninsular Florida. J. Geophys. Res. 121, 7691–7709.
Morgan, J. L., Gergel, S. E., and Coops, N. C. (2010). Aerial Photography: a Rapidly Evolving Tool for Ecological Management. Bioscience 60, 47–59. doi: 10.1525/bio.2010.60.1.9
Municode (2018). Municode. Available online at https://www.municode.com/ (accessed November 26, 2018).
Nesbitt, L., Meitner, M. J., Girling, C., Sheppard, S. R. J., and Lu, Y. (2019). Who has access to urban vegetation? Landsc. Urban Plan. 181, 51–79.
Nguyen, V. D., Roman, L. A., Locke, D. H., Mincey, S. K., Sanders, J. R., Smith Fichman, E., et al. (2017). Branching out to residential lands: missions and strategies of five tree distribution programs in the U.S. Urban For. Urban Greening 22, 24–35. doi: 10.1016/j.ufug.2017.01.007
Nowak, D. J. (2012). Contrasting natural regeneration and tree planting in fourteen North American cities. Urban For. Urban Greening 11, 374–382. doi: 10.1016/j.ufug.2012.02.005
Nowak, D. J., and Greenfield, E. J. (2018). US Urban Forest Statistics Values, and Projections. J. For. 116, 164–177. doi: 10.1080/17457300.2018.1467461
Nowak, D. J., Rowntree, R. A., McPherson, E. G., Sisinni, S. M., Kerkmann, E. R., and Stevens, J. C. (1996). Measuring and analyzing urban tree cover. Landsc. Urban Plan. 36, 49–57. doi: 10.1016/s0169-2046(96)00324-6
O’Neil-Dunne, J., MacFaden, S., and Royar, A. (2014). A versatile, production-oriented approach to high-resolution tree-canopy mapping in urban and suburban landscapes using GEOBIA and data fusion. Remote Sens. 6, 12837–12865.
Ossola, A., and Hopton, M. E. (2018). Climate differentiates forest structure across a residential macrosystem. Sci. Total Environ. 639, 1164–1174. doi: 10.1016/j.scitotenv.2018.05.237
Pham, T.-T.-H., Apparicio, P., Landry, S. M., and Lewnard, J. (2017). Disentangling the effects of urban form and socio-demographic context on street tree cover: A multi-level analysis from Montréal. Landsc. Urban Plan. 157, 422–433. doi: 10.1016/j.landurbplan.2016.09.001
R Core Team (2020). R: A Language and Environment for Statistical Computing. Austria: R Foundation for Statistical Computing.
Roman, L. A., Catton, I. J., Greenfield, E. J., Pearsall, H., Eisenman, T. S., and Henning, J. G. (2021a). Linking urban tree cover change and local history in a post-industrial city. Land 10:403.
Roman, L. A., Conway, T. M., Eisenman, T. S., Koeser, A. K., Ordóñez Barona, C., Locke, D. H., et al. (2021b). Beyond “trees are good”: disservices, management costs, and tradeoffs in urban forestry. Ambio 50, 615–630. doi: 10.1007/s13280-020-01396-8
Roman, L. A., Pearsall, H., Eisenman, T. S., Conway, T. M., Fahey, R. T., Landry, S. M., et al. (2018). Human and biophysical legacies shape contemporary urban forests: a literature synthesis. Urban For. Urban Greening 31, 157–168. doi: 10.1016/j.ufug.2018.03.004
Staudhammer, C., Escobedo, F., Lawrence, A., Duryea, M., Smith, P., and Merritt, M. (2011). Rapid Assessment of Change and Hurricane Impacts to Houston’s Urban Forest Structure. Arboric. Urban For. 37, 60–66.
Steenberg, J. W. N., Millward, A. A., Nowak, D. J., and Robinson, P. J. (2017). A conceptual framework of urban forest ecosystem vulnerability. Environ. Rev. 25, 115–126.
Thompson, B. K., Escobedo, F. J., Staudhammer, C. L., Matyas, C. J., and Qiu, Y. (2011). Modeling hurricane-caused urban forest debris in Houston. Texas. Landsc. Urban Plan. 101, 286–297.
Turner-Skoff, J. B., and Cavender, N. (2019). The benefits of trees for livable and sustainable communities. Plants People Planet 1, 323–335. doi: 10.1002/ppp3.39
United States Census Bureau (2015). American Community Survey Data. Hillcrest Heights, MD: United States Census Bureau.
U.S. Census Bureau (2018a). City and Town Population Totals: 2010-2019. Available online at: https://www2.census.gov/programs-surveys/popest/tables/2010-2019/cities/totals/SUB-IP-EST2019-ANNRES-12.Xlsx (accessed July 12, 2021).
U.S. Census Bureau (2018b). Selected Housing Characteristics, 2014–2018. Hillcrest Heights, MD: United States Census Bureau.
U.S. Census Bureau (2019). QuickFacts Florida. Available online at: https://www.census.gov/quickfacts/FL (accessed May 7, 2021).
U.S. Department of Agriculture (2019). National Agriculture Imagery Program. Available online at https://www.fsa.usda.gov/programs-and-services/aerial-photography/imagery-programs/naip-imagery/ (accessed April 1, 2020).
Van der Sommen, F. J., Pearson, D. M., and Boggs, G. S. (2018). Analysis of the interrelationship between houses, trees and damage in a cyclone affected city: can landscape design and planning utilising trees minimise cyclone impact? Int. J. Disast. Risk Re. 28, 701–710. doi: 10.1016/j.ijdrr.2018.01.031
Volk, M. I., Hoctor, T. S., Nettles, B. B., Hilsenbeck, R., Putz, F. E., and Oetting, J. (2017). “Florida Land Use and Land Cover in the Past 100 Years,” in Florida’s Climate: Changes, Variations, & Impacts. eds E. P. Chassignet, J. W. Jones, V. Misra, and J. Obeysekera (Gainesville, FL: Florida Climate Institute).
Walton, J. T., Nowak, D. J., and Greenfield, E. J. (2008). Assessing Urban Forest Canopy Cover Using Airborne or Satellite Imagery. Available online at: https://www.nrs.fs.fed.us/pubs/jrnl/2008/nrs_2008_walton_002.pdf (accessed June 3, 2021).
Wang, W., Qu, J. J., Hao, X., Liu, Y., and Stanturf, J. A. (2010). Post-hurricane forest damage assessment using satellite remote sensing. Agric. For. Meteorol. 150, 122–132. doi: 10.1016/j.agrformet.2009.09.009
Watkins, S. L., and Gerrish, E. (2018). The relationship between urban forests and race: a meta-analysis. J. Environ. Manage. 209, 152–168. doi: 10.1016/j.jenvman.2017.12.021
Wiersma, Y. F., Davis, T. L., Eberendu, E. C., Gidge, I., Jewison, M., Martin, H. C., et al. (2012). Hurricane Igor Impacts at Northern Latitudes: factors Influencing Tree Fall in an Urban Setting. Arboric. Urban For. 38, 92–98.
Young, R. F. (2011). Planting the Living City. J. Am. Plann. Assoc. 77, 368–381. doi: 10.1080/01944363.2011.616996
Keywords: governance, social-ecological system, tropical cyclone, urban forest, urban tree canopy, tropical typhoon
Citation: Salisbury AB, Koeser AK, Hauer RJ, Hilbert DR, Abd-Elrahman AH, Andreu MG, Britt K, Landry SM, Lusk MG, Miesbauer JW and Thorn H (2022) The Legacy of Hurricanes, Historic Land Cover, and Municipal Ordinances on Urban Tree Canopy in Florida (United States). Front. For. Glob. Change 5:742157. doi: 10.3389/ffgc.2022.742157
Received: 15 July 2021; Accepted: 04 January 2022;
Published: 14 February 2022.
Edited by:
Barry Alan Gardiner, Institut Européen De La Forêt Cultivée (IEFC), FranceReviewed by:
Cara Rockwell, Florida International University, United StatesCopyright © 2022 Salisbury, Koeser, Hauer, Hilbert, Abd-Elrahman, Andreu, Britt, Landry, Lusk, Miesbauer and Thorn. This is an open-access article distributed under the terms of the Creative Commons Attribution License (CC BY). The use, distribution or reproduction in other forums is permitted, provided the original author(s) and the copyright owner(s) are credited and that the original publication in this journal is cited, in accordance with accepted academic practice. No use, distribution or reproduction is permitted which does not comply with these terms.
*Correspondence: Andrew K. Koeser, YWtvZXNlckB1ZmwuZWR1
Disclaimer: All claims expressed in this article are solely those of the authors and do not necessarily represent those of their affiliated organizations, or those of the publisher, the editors and the reviewers. Any product that may be evaluated in this article or claim that may be made by its manufacturer is not guaranteed or endorsed by the publisher.
Research integrity at Frontiers
Learn more about the work of our research integrity team to safeguard the quality of each article we publish.