- 1Departamento de Biología Molecular, Universidad de Cantabria, Santander, Spain
- 2Facultad de Ingeniería Química, Universidad Central del Ecuador, Quito, Ecuador
- 3Instituto de Investigación en Zoonosis (CIZ), Universidad Central del Ecuador, Quito, Ecuador
- 4Institute of Integrative Systems Biology (I2Sysbio), University of Valencia and Consejo Superior de Investigaciones Científicas (CSIC), Valencia, Spain
- 5Department of Horticulture and Crop Science, The Ohio State University, Columbus, OH, United States
- 6Instituto Nacional de Biodiversidad, Quito, Ecuador
- 7Grupo de Investigación en Biodiversidad, Medio Ambiente y Salud (BIOMAS), Universidad de Las Américas, Quito, Ecuador
- 8Instituto de Biomedicina y Biotecnología de Cantabria, CSIC – Universidad de Cantabria, Santander, Spain
- 9Facultad de Medicina Veterinaria y Zootecnia, Universidad Central del Ecuador, Quito, Ecuador
Our study is a pioneering exploration of the microbiome in the soil of the Sumaco stratovolcano and an assessment of the effects of an elevational gradient and related physicochemical soil parameters on richness and community structure. The Sumaco, as an isolated Amazonian stratovolcano, may be among one of the least studied ecosystems in Ecuador and perhaps the Amazon region. Universal patterns remain unresolved or available information inconclusive to establish a supported consensus on general governing processes by which elevation and its associated environmental gradients may determine the microbial richness and community structure. We tested a recent proposal on how microbial diversity responds to montane gradients, placing a central role in soils as potentially independent of altitude along an elevational gradient. Correlations and effects among soil physicochemical parameters and altitude were contrasted against richness and community structure through quantitative ecology. The most informative physicochemical parameter in our assessment of bacterial community structure was neither pH nor altitude, but sulfur, which was mostly independent of the other tested parameters. We established a positive effect of richness by parameters associated with metallic cations such as Mn2+, and CEC, which were negatively correlated to altitude and pH. The possible relation between the significant role of sulfur on bacterial community structure with the unique geological origin of the Sumaco stratovolcano should be examined in the context of specialized sulfur metabolisms and additional information on community structure and environmental constraints. Our study establishes an initial baseline for further explorations of microbial diversity in this unexplored tropical stratovolcano.
Introduction
Amazonian soils have been described as a vast source of novel bacteria (Bruce et al., 2010). Despite widespread and incessant deforestation in favor of agricultural lands, there are yet relatively few studies in the Amazon region that have quantified microbial diversity and linked it to ecological roles and services, especially when compared to other groups of living organisms such as plants or animals (Ranjan et al., 2015). Soil health and structure, flux of nutrients, and symbiotic relationships with plants are all dependent on the microbial community present in the ecosystems (Fonseca et al., 2018). Soil biogeochemistry is intimately connected to bacterial communities and modifications to the original forest cover inexorably alter both the available nutrients and the bacterial communities present in the affected area (Merloti et al., 2019). Forest soils in the Amazon region are being largely affected by anthropogenic activities and disturbances that are associated with an expanding agricultural frontier, which also affects bacterial diversity and the presence of functional microbial genes related to soil biogeochemistry (Melo et al., 2021). The transformation of forests into pastures or agricultural lands in the Amazon region increases pH, bacterial richness, and alpha diversity; it also alters community structure with yet unknown consequences for the functions and services of ecosystems (de Carvalho et al., 2016). Increased soil methane emissions and reduced carbon sinks are expected as Amazon forests are replaced by pastures (Kroeger et al., 2021) or warm-up along altitudinal gradients to highland environments, accelerating bacterial carbon metabolism (Looby and Martin, 2020). Consequences of deforestation are wide-ranging and with unknown or poorly understood effects on human health, as land conversion in the Amazon could also promote an increased frequency of antibiotic resistance genes in soil bacteria (Lemos et al., 2021).
Pristine forests in the Amazon region provide opportunities to study untouched microbiotas and how environmental parameters, such as those along an altitudinal gradient, can cause shifts in microbial diversity (de Carvalho et al., 2016). Information on the diversity profile of healthy soils, from pristine forests in the Amazon region, provides a component of value to ecosystems and a source of comparison for degraded environments (de Carvalho et al., 2016; Merloti et al., 2019). Thus, the study of microbiome profiles in pristine environments and changes in response to environmental gradients can provide information to establish downstream applications, such as bioremediation, estimates on the effects of climate change in bacterial diversity and soil nutrient enrichment, or other associated services from bacterial biodiversity (de Carvalho et al., 2016; Looby and Martin, 2020).
The Sumaco volcano remained for many centuries a legend of the Amazon region, briefly mentioned by explorers, naturalists, and geographers (Colony and Sinclair, 1928), until it was officially reported by Sinclair and Wasson (1923), with an estimate of its elevation and geographical location. The Sumaco is the result of the back-arc magmatism of Ecuador that formed a chain of three Amazonian volcanoes, set off to the east of the main volcanic axis of the Andean cordillera, with Pan de Azúcar and Reventador being the other two (Hoffer et al., 2008; Puerini, 2009; Garrison et al., 2018). Sumaco is an active stratovolcano that raises from the Amazon basin at 300 m above sea level (m.a.s.l.) to its peak at 3732 m.a.s.l., and it is dominated by a conic structure with a basal radius of 12 km (Puerini, 2009). A heavily forested and active volcano, with the latest eruption estimated between 1933 and 1958, it is part of the Gran Sumaco Biological Reserve and Sumaco Napo-Galeras National Park (Lozano et al., 2020). Because of its unique geological origin, over 100 km to the east of the Andean arc front, the Sumaco presents unusual geochemical characteristics, with distinct alkaline lavas that are strongly saturated by silica (i.e., high alkalinity basanite to tephriphonolite), and that distinguishes it from all other active volcanoes in Ecuador (Rosenbaum et al., 2018). Presenting frequent heavy rains and challenging accessibility, it has been poorly studied in comparison to other areas of the Amazon region, with only four publications related to animal or plant diversity (Lozano et al., 2020). Its remoteness and inaccessibility have also contributed to preserving its forest. Our study was a pioneering exploration of the microbiome in the soil of the Sumaco and an assessment of the effects of an elevational gradient, and related physicochemical soil parameters, on richness and community structure. As a pristine environment, unmodified by human intervention, our study was also a valuable first record of the natural state of the bacterial community in the Sumaco. Here we used the concepts defined by Stevens (2009), where richness represents the count of the number of taxa in a sample and community structure is the set of taxa in a site or sample that includes a measure of abundance.
Ever since von Humboldt and Bondpland (1805) proposed that climate along elevational gradients in the tropical Andes was responsible for how plant communities changed, environmental gradients have been essential in the development of ecological and evolutionary theories that suggest the presence of general principles that regulate biological diversity (Peay et al., 2017). It is currently accepted that patterns of species richness along elevational gradients in mountains vary accordingly to taxa and do not conform to a single all-encompassing model (Looby and Martin, 2020). Elevational gradients in the tropics are considered natural laboratories for ecosystem ecology and global change research, which contain contrasting changes in climate and environment over relatively small scales and without potential confounding effects by geographical latitude (Malhi et al., 2010). A synthesis and meta-analysis on 235 soil communities across 20 studies on the effects of elevation, temperature, and soil pH gradients found no consensus among diversity patterns in soil microbial communities (Hendershot et al., 2017). Other studies have either found significant effects of elevational gradients for bacteria (Corneo et al., 2013) and fungi (Looby et al., 2016) or no evidence of elevation being a relevant factor, but instead geological aspect or some component of soil biochemistry such as pH (Fierer et al., 2011; Wu et al., 2017). Soil microbes have been expected to respond similarly to macroscopic organisms; however, evidence on how microorganisms respond to altitudinal gradients remains inconclusive. The latest synthesis across Fungi, Bacteria, and Archea found that nearly one-third of the 77 studies analyzed across the world for montane gradients reported a decline in total microbial diversity with increasing elevation, with the other two thirds showing a range of results that included either no consistent trend, an increase, mid-elevation peak, or other trends (Looby and Martin, 2020). More specifically, when elevation increased along mountain gradients, Bacteria showed a marked lack of patterns in comparison to Fungi in this latter meta-analysis study.
Along an elevational gradient, from the Amazon to the Andes, community structure and increased dissimilarity in soil bacteria responded to elevation, with a decline in richness at higher altitudes (Nottingham et al., 2018). Such changes were correlated with similar patterns in fungi and plants and strongly associated with temperature (Nottingham et al., 2018). The diversity gradient observed by Nottingham et al. (2018) was strongly correlated with microbial traits associated with slow-growing oligotrophic taxa at higher elevations. On the contrary, and on a more complex venue where temperature was not considered a significant factor, Fungi, Archea, and Bacteria showed strong responses to an elevational and precipitation gradient in Hawai’i (50–1000 m, 280–3280 mm/yr), but with different and unique linear (Fungi and Archea) or unimodal (Bacteria) trends (Peay et al., 2017). Bacterial diversity and community structure were predicted mostly by variation in elevation at Mt. Hallasan in Jeju Island, South Korea, suggesting that climate and vegetation cover type, rather than soil texture or geochemistry, were the determining factors (Singh et al., 2014). These studies must be also compared with the proposal for the dominant role of soil over every other environmental parameter in the most recent review and meta-analysis by Looby and Martin (2020).
These studies, with different approaches to measuring diversity and under a varied set of geographical and environmental conditions, hint toward several modes by which elevation or its associated environmental parameters may be influencing richness and community structure in microorganisms. However, universal patterns remain unresolved or available information inconclusive to establish a supported consensus on general governing processes by which elevation and its associated environmental gradients may determine the microbial richness and community structure. Microbes respond to environmental parameters that are inherently complex and highly heterogeneous at small scales and have diverse ecologies, most of them unknown; thus, microbial responses to environmental gradients are expected to be also heterogeneous (Peay et al., 2017).
By considering that there is no definitive consensus on how bacterial and microbial communities respond along elevational gradients, our survey on the Sumaco volcano adds additional sources of evidence on the role of soil chemistry on richness, diversity, and community structure along an elevational gradient. Amidst so many varying results in previous studies, we took a conservative stance and followed the most recent synthesis by Looby and Martin (2020). We hypothesized that bacterial communities would respond most strongly to soil pH, regardless of varying elevation along the sampled altitudinal gradient (H1). A null hypothesis is also possible to establish for no effect of the measured physicochemical parameters on the structure of bacterial communities (H0). Thus, we tested the most recent proposal on how microbial diversity responds to montane gradients, placing a central role in soils as potentially independent of other abiotic factors along an elevational gradient (Looby and Martin, 2020).
Materials and Methods
Sample Collection
Samples were collected between 22 and 24 July 2016, during a 3-day survey toward the summit of the Sumaco volcano and spanned an elevation gradient between 1584 and 3809 m.a.s.l.; thus, covering a range of vegetation transitions that included tropical, subtropical and paramo ecosystems (Lozano et al., 2020). The climbing route extended over 20 km from the town of “Pacto Sumaco” to the summit (Figure 1). After reaching the summit on the second day, we descended during the third and proceeded to collect a total of nine soil samples (1 kg each). A shovel or ice axe was used to dig into the sampling point at an approximate depth of 10–25 cm below the surface, removing roots and rocks. Soil samples were taken in duplicate with a shovel previously washed and disinfected with 70% alcohol. Each duplicate was used for sequencing and physical-chemical characterization, respectively. Each soil sample was a unique observation along the altitudinal gradient, such that each one was characterized by physicochemical measurements. Samples were labeled as “SUM##” and numbered. The physicochemical properties of each sample were measured at the Center for Integral Analytical Solutions (CENTROCESAL Cía. Ltda., Ecuador. Accreditation No. SAE LEN 12-001) and consisted of the following parameters: electrical conductivity (EC), organic matter content (Org), humidity (Hum), cation exchange capacity (CEC), phosphorus (P), nitrogen (N), calcium (Ca2+), magnesium (Mg2+), manganese (Mn2+), sulfur (S), potassium (K+), iron (Fe), and sodium (Na+). These parameters were obtained according to the procedures described in Baird et al. (2017). pH was evaluated in the laboratory with a pH meter (Mettler Toledo SevenGo, Millipore, Columbus, OH, United States). Sample coordinates, elevation, and values for the physicochemical parameters are included in Supplementary Table 1.
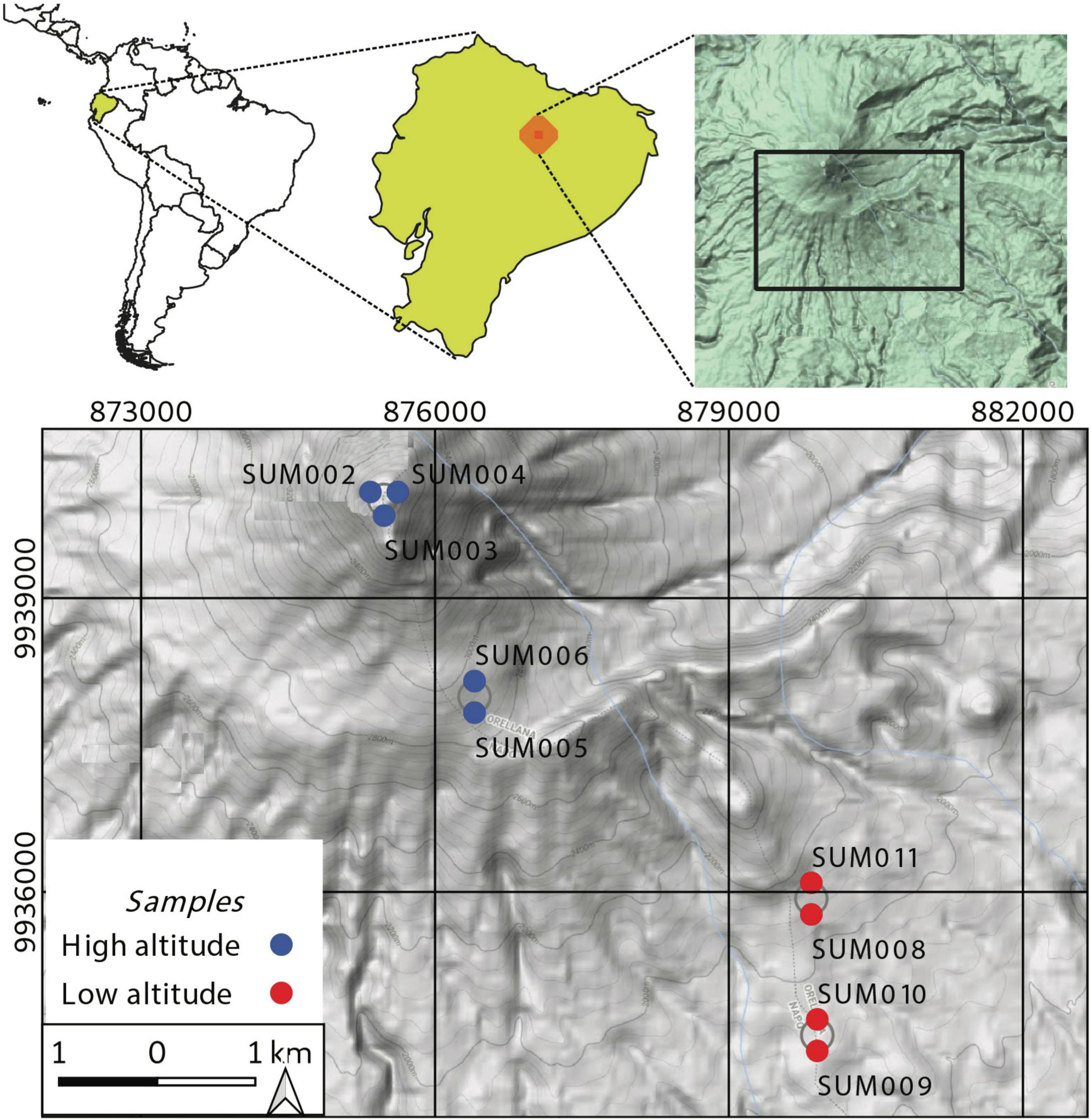
Figure 1. Sampling locations along an elevational gradient in the Sumaco Volcano. Locations that were close together are represented as points in a circle. Samples have been classified into two altitudinal categories, above (blue) and below 2900 m.a.s.l. (red).
DNA Extraction, 16S rRNA Gene Library Preparation, and Sequencing
Metagenomic DNA extraction was performed with the PowerSoil DNA Isolation kit (Cat. Nos. 12888-50, MO BIO Laboratories, Inc.) and according to the manufacturer’s instructions. DNA quality and concentration were quantified spectrophotometrically with a NanoDrop 2000 (Thermo Fisher Scientific). The total extracted genomic DNA is currently stored at −80°C in the collection of the Ecuadorian Microbiome Project (EcuMP) at the Institute of Research on Zoonoses (CIZ) of Central University of Ecuador. A partial region of 500 bp including the hypervariable regions V3 and V4 of the 16S rRNA genes was amplified with custom primers based on previous work (Klindworth et al., 2013). 16s rRNA libraries of 300 bp paired-end fragments were obtained by synthesis sequencing technology on an Illumina MiSeq platform (San Diego, CA, United States).
Sequence Processing and Taxonomic Analysis
Bacterial phylogenetic clades were estimated with Mothur v.1.43.0 (Schloss et al., 2009) and by following the MiSeq Standard Operational Procedure (Kozich et al., 2013). Forward and reverse reads were assembled into contigs and followed by filtering and processing of the resulting sequences. In brief, sequences with a minimum overlap of 20 bp, which included a length between 580 and 348 bp were retained. Sequences with ambiguities or homopolymers longer than 14 bp were removed from the analysis. The filtered sequences were deduplicated and aligned against the V3–V4 region of the SILVA v132 reference small subunit rRNA gene alignment database (Quast et al., 2013). Those sequences that did not span the full alignment were filtered out by optimizing the start and end positions using a 95% criterion. The resulting alignments were further processed by eliminating columns that contained gaps or dot characters, and the sequences were deduplicated for a second time. Denoising was performed by preclustering sequences with less than one difference per 100 bp, and chimeras were removed using Mothur’s implementation of the VSEARCH algorithm (Rognes et al., 2016). Sequences were classified by the Wang method (Wang et al., 2007), with the naive Bayesian classifier against the SILVA v132 reference taxonomy database and using a 70% bootstrap threshold. Sequences belonging to Chloroplast, Mitochondria, and Eukarya were removed. The optiClust algorithm (Westcott and Schloss, 2017) was applied on the resulting sequences for estimating clusters and OTUs at 99% identity. Consensus classifications and representative sequences were determined for each OTU based on the most abundant sequence within each cluster. After processing the data in Mothur, OTUs were further filtered. We only retained OTUs that belonged to the Bacteria kingdom. Moreover, OTUs not classified at the phylum level and singletons were filtered out, with the assistance of the phyloseq package (McMurdie and Holmes, 2013) in R (R Core Team, 2020). Bacterial community structure was explored at various taxonomic levels.
Multivariate Analysis
Alpha diversity measures (including Richness, Shannon and Simpson indexes) were calculated with the estimate_richness() function of the phyloseq package at the genus level. Diversity rarefaction curves were graphed with a step size of 600 counts with the ggrare() function of the ranacapa package (Kandlikar et al., 2018). The effect of altitude on the Simpson index was tested with a simple linear regression. Samples were grouped according to their altitude, below or above 2900 m.a.s.l., this classification facilitated contrasts among the sampled communities and represented a large altitudinal gap in the sampled communities of at least 700 m.
The nine available samples represented an effective eight degrees of freedom for multivariate ordination models. This limited quantitative models on community structure and Richness to a maximum of seven environmental parameters which we will refer to, in the context of the multivariate analysis, as independent environmental constraints. The selection of an appropriate set of environmental constraints consisted of several steps which started at a correlogram assisted by a hierarchical clustering based on the unweighted pair group method with arithmetic mean (UPGMA). This correlogram was estimated with the corrplot package (Wei and Simko, 2017) and provided an appraisal of collinearity and groups of variables based on Pearson correlation distances. The goal was to choose environmental parameters that minimized redundancy but kept physiochemically distinct sets of explanatory variables (Peay et al., 2017). The observed patterns in the correlogram provided an initial set of variables for further exploration of quantitative ecology.
The community structure matrix consisted of the observed abundances at the genus level for the eight most-abundant phyla since they represented over 90% of the relative abundance throughout taxa. A canonical correspondence analysis (CCA) provided the framework to estimate the effects of environmental constraints on bacterial community structure. The most informative variables within the selected six-constraint model were chosen through a forward and reverse stepwise selection procedure based on the Akaike Information Criterion (AIC), with the ordistep algorithm as implemented in Vegan (Oksanen et al., 2020). A complementary PERMANOVA by the adonis algorithm (permutation ANOVA using distance matrices) provided a significance assessment of the model and estimated the marginal effect of the environmental constraints (Oksanen et al., 2020).
A measure of the importance or strength of environmental constraints for bacterial richness was estimated by the linear effects of the six selected explanatory variables on total richness (Peay et al., 2017). The contribution to the R2 of the model by each environmental constraint was estimated as the average through all possible orderings of entry into the model by each one of the previously selected six environmental constraints. This was possible through the “lmg” algorithm implemented in the relaimpo package (Grömping, 2006). The residual effect of each selected environmental constraint on total richness was estimated by partial-regression plots for the assessed six-constraint linear model, as implemented in the avPlots algorithm of the CAR package (Fox and Weisberg, 2019). In addition, the community structure of the 25 most abundant taxa at the family level were explored with the ComplexHeatmap package (Gu et al., 2016).
Differential Abundance Analysis
To discover genera that were significantly different across the altitudinal gradient, a differential genus detection analysis based on the negative binomial distribution (Gamma-Poisson) was performed with the DESeq2 package (Love et al., 2014). The dataset included all observed genera. The phyloseq object was exported to a DESeq2 object for further preprocessing. With the transformed DESeq2 object, the size factors were calculated based on the median rate method (Anders and Huber, 2010). The abundances in the DESeq2 object were subjected to variance stabilizing transformation by using the estimated size factors. The comparison between the high and low altitude group was carried out to determine statistically significant differences in bacterial genera. To reduce the false discovery rate, corrected p-values were obtained with the Benjamini–Hochberg method (Benjamini et al., 1995). To improve the visualization of the results, unclassified taxa at the genus level were filtered. Finally, all genera with an FDR < 0.05 and an | log2fc| > 0.6 were classified as significant.
Results
A total of 300934 high-quality reads were obtained with an average of 33437 and a standard deviation of 5905.89 reads per sample. Rarefaction curves suggested adequate sample size and approached the asymptote with an average of 90% of reads (Figure 2A). No definitive pattern was discernable for the effect of altitude on richness in the rarefaction curves. The pattern provided by an abundance barplot on the most abundant phyla and families (Figures 2D,E) showed this was consistent with the spatial distribution of samples in the CCA (Figure 3). Of the nine samples, the most distinct patterns in abundance were those of SUM002 (3783 m.a.s.l.), SUM005 (2998.4 m.a.s.l.), and SUM006 (2990 m.a.s.l.), which were high-altitude samples with distinct community structures. SUM002 is characteristic due to its large proportion of Pseudomonadaceae and Pseudomonas. Within low altitude samples (<2900 m.a.s.l.), abundance patterns showed consistency and small variation relative to those at higher altitudes (>2900 m.a.s.l.) (Figures 2A,B, 3). Remarkable families include Ktedonobacteraceae (Chloroflexi, Ktedonobacteria), an actinomycete-like group that has been previously reported as an abundant family present in aerosol for the Amazon region (Souza et al., 2021) and known only from two described species Ktedonobacter racemifer (Cavaletti et al., 2006) and Dictyobacter aurantiacus (Yabe et al., 2017; Figures 2, 3). The former belongs to an undescribed location in Italy and the latter was obtained from Mount Salak stratovolcano in West Java, Indonesia at a rice paddy field, at 600 m.a.s.l. Members of the Ktedonobacterales have been found associated with geothermal soils and geothermally heated biofilms (Yabe et al., 2017). Present in all samples “soil group” WD2101 was a yet undescribed family (Planctomycetes, Tepidisphaerales) and an “enigmatic planctomycete group” that has been detected in terrestrial habitats, particularly at boreal peatlands of European North Russia, where it has been considered as one the most abundant and characteristic groups in Sphagnum-dominated bogs (Dedysh et al., 2021; Figure 2E). WD2101 has been previously reported for the Amazon region as an abundant group found in native soils and teak plantations (de Gannes et al., 2016). There was a notable presence throughout all samples of two groups of unknown families belonging to the class Acidobacteriia. These two groups suggest gaps in knowledge on the diversity present at the Sumaco, especially as an unexplored environment for microorganisms.
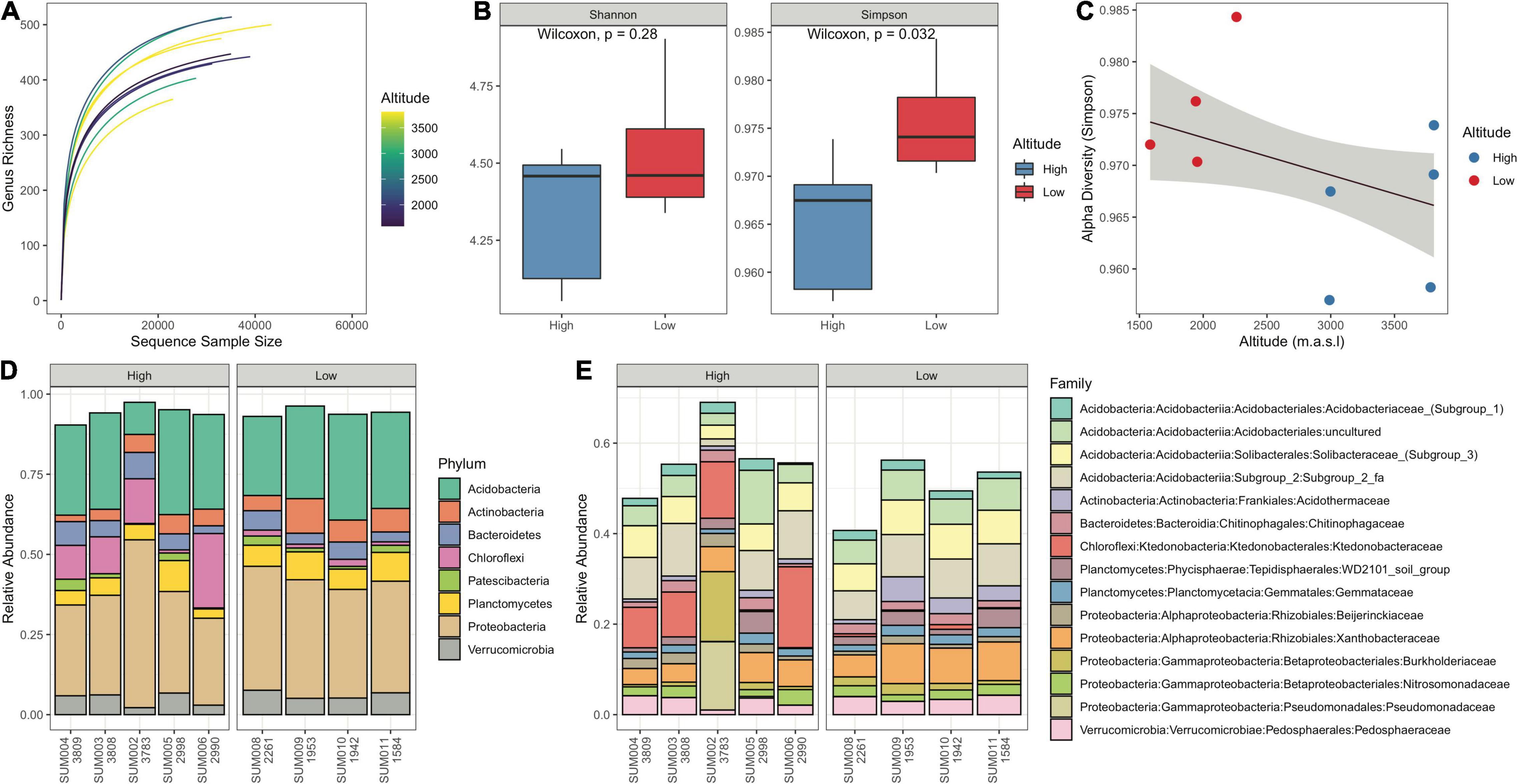
Figure 2. Diversity analysis for soil samples. (A) Rarefaction curves for soil samples. The color palette refers to the altitude at which the sample was collected, warmer colors represent higher altitudes. (B) Boxplot of Shannon and Simpson alpha diversity indexes for soil samples, with their corresponding significance values for a Wilcoxon contrast test. (C) Robust linear regression with the Simpson index as the predicted value and altitude as the predictor, it includes a 95% confidence interval around the regression estimate. (D) Stacked bar plots contrasting high and low samples for the eight most abundant Phyla. Values under each sample name are the corresponding altitudes. (E) Stacked bar plots contrasting high and low samples for the 15 most abundant families in the samples.
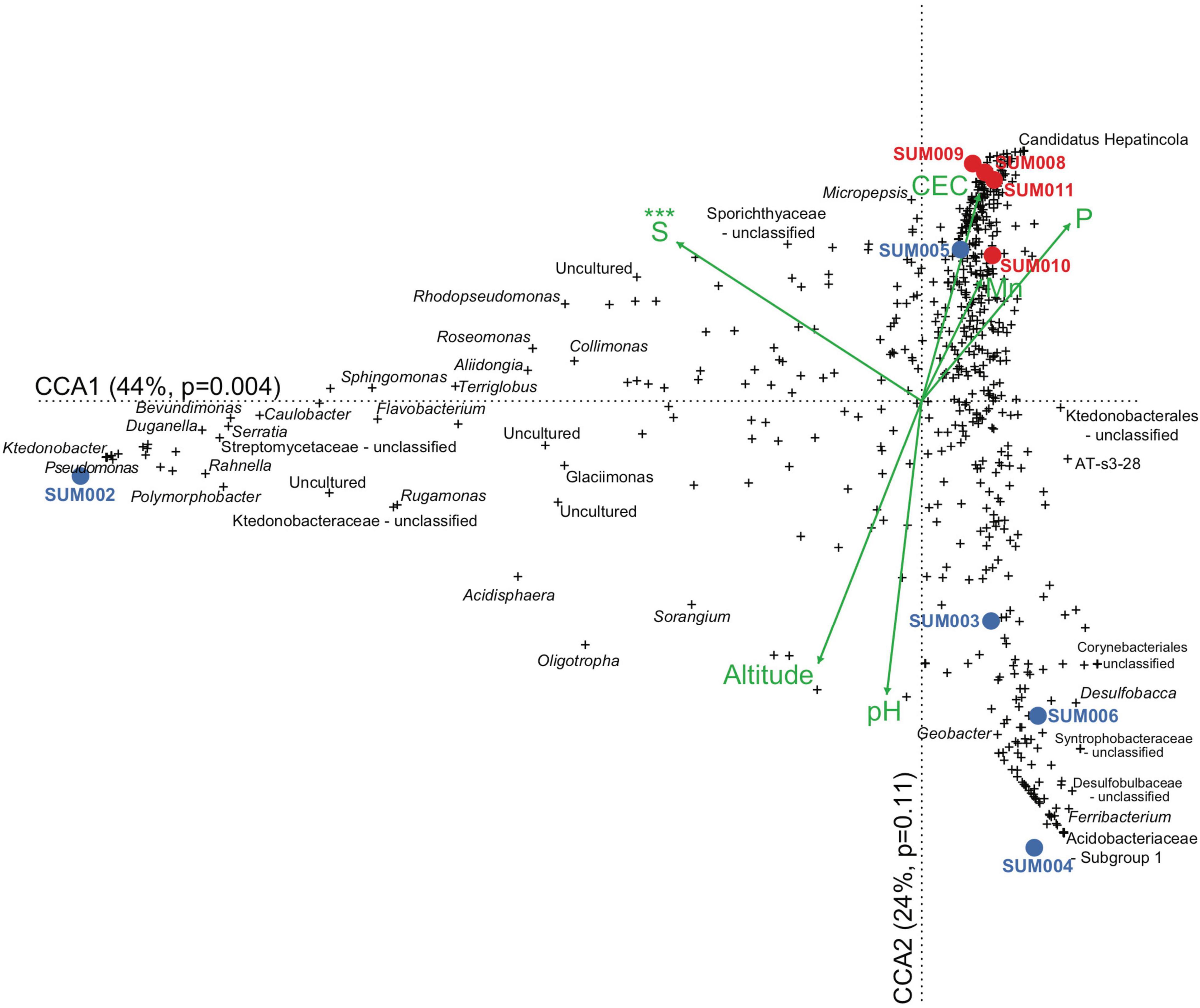
Figure 3. Graphical depiction of the CCA analysis. The first two canonical axis represent 68% of the total explained variance. Environmental constraints are shown as green arrows. Blue and red dots represent high and low-altitude soil samples, respectively. Crosses represent the genera from the selected 8 most abundant phyla that represented >90% of the relative abundances. Only the most salient genera have been annotated, as the available space precludes including the names for every sample. The three asterisks on top of sulfur, represent its significance test for the marginal effects of each environmental variable.
Shannon and Simpson diversity indexes for both high (>2900 m.a.s.l.) and low (<2900 m.a.s.l.) altitudinal groups showed discernable differences. A one-sided Wilcoxon signed-rank test for differences between high and low altitudinal groups resulted in statistically significant differences for the Simpson index only (P = 0.032) (Figure 2B). A first assessment of the inferred effect of altitude on diversity was provided by a simple linear regression (Figure 2C), with an inverse relationship. A Wald test for multiple coefficients (robust F-test) indicated no relationship between alpha diversity and altitude (F = 0.64; P = 0.45).
A correlogram, accompanied by clustering, provided a quantitative criterion for the selection of variables that accounted for the observed community structure and abundance. Thus, the clustering resulted in five groups of variables, which were similar in their correlation patterns. Within each of the five groups suggested by the clustering analysis, we choose a single variable, which represented the one with the strongest correlation to richness and the smallest value for significance within several iterations in explorative models for the CCA. The exception was for the selection of both altitude and pH, as we considered that both parameters were of special relevance to test the hypothesis of our study (Figure 4).
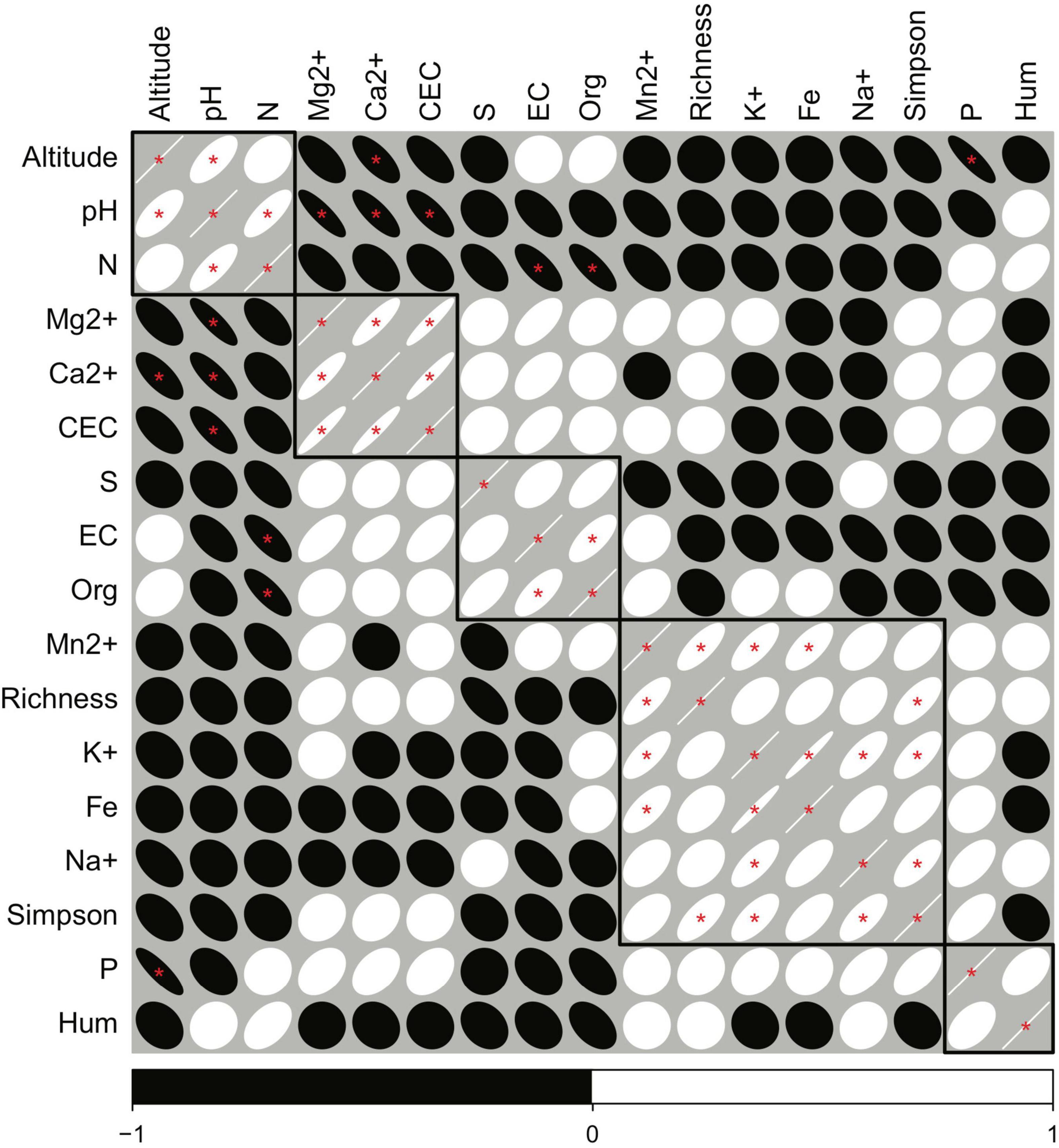
Figure 4. Correlogram of the environmental constraints. Black squares show the selected groups after a clustering analysis on the Pearson correlation distances. Red asterisks show significant correlations at P < 0.05. White ovals are positive correlations and black ovals negative correlations. The strength of the correlation is represented by oval narrowness. Richness and the Simpson diversity index are also included as references for variable selection.
The chosen six variables, considered as environmental constraints, showed little or no collinearity as informed by the variance inflation factor (altitude = 10.54, pH = 6.83, P = 6.04, S = 1.46, Mn2+ = 2.41, CEC = 3.18). The relative larger values for pH and altitude seemed to reflect the correlation between these two. The results of the stepwise selection procedure, for establishing the contribution of each environmental constraint to the R2 of the CCA model, resulted in the selection of two constraints S (AIC = 93.44, F = 3.45, df = 1, P = 0.01) and altitude (AIC = 90.34, F = 4.58, df = 1, P = 0.01). These two environmental constraints were the minimum necessary to explain the variability observed in the community structure as interpreted by the CCA. The results of the PERMANOVA on the full six-constrain CCA model showed significance (R2 = 0.83, F = 1.59, df = 6, P = 0.03), and the assessment of the marginal effect of environmental constraints resulted in only S as significant (R2 = 0.17, F = 1.96, df = 1, P = 0.04). The relative importance of each constraint to explain richness, as measured as the percentage of total R2 in a linear regression model, resulted in Mn2+ as the largest marginal effect (R2 = 41%), with the rest of constraints having smaller contributions (S = 28%, pH = 11%, P = 9%, altitude = 8%, CEC = 2%).
Once the effect of other variables in the linear model had been partialed out, the effect on richness could be depicted as in Figure 5. Mn2+ and P were positively correlated with richness, with the first having the strongest effect on the model. Negative effects on richness were estimated for S and pH, with the first doubling the second in terms of R2. CEC had a limited positive effect on richness. Surprisingly, once the effect of other constraints was controlled for, altitude had a positive effect on richness (Figure 5), which does not fully concord with the initial proposal depicted in Figure 2C of a regression of altitude on the Simpson index.
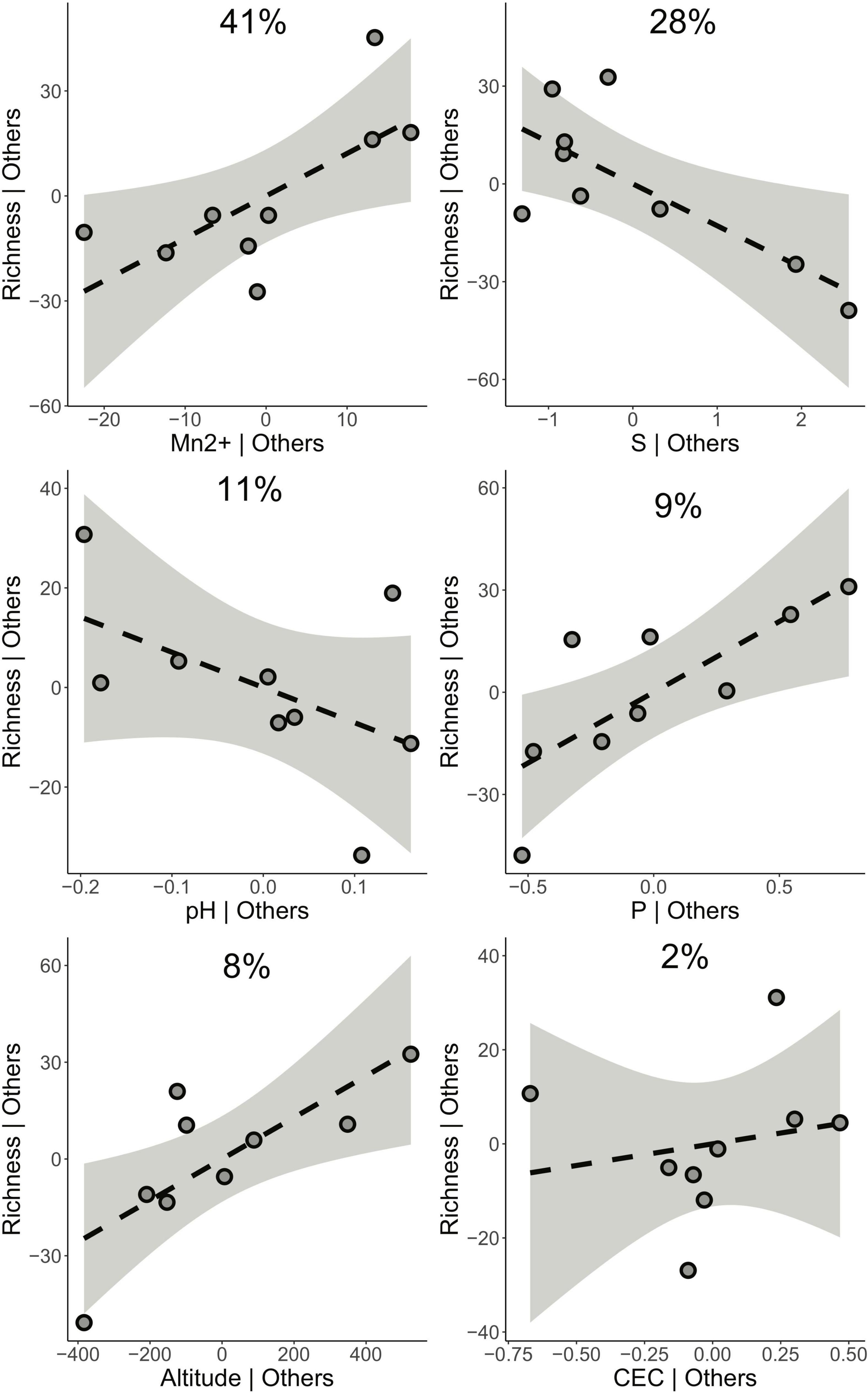
Figure 5. The six environmental constraints that have been considered drivers of richness in the Sumaco Volcano. The scatterplots represent the partial correlation of each environmental constraint on richness, after controlling for the effect of the other five constraints. Percentages at each tile represent the average relative contribution to the R2 of the constraint in the linear model, after considering all possible linear combinations of entry into the model.
The depiction of the CCA model (Figure 3) showed a strong presence of Pseudomonas and Ktedonobacter in sample SUM002. Genus Candidatus Hepatincola is particularly abundant in low-altitude samples SUM008, SUM009, and SUM011. The largest number of genera remain clustered in quadrant I of the cartesian plane, which is the direction of the positive vectors for Mn2+, P, and CEC. These three constraints had positive correlations with richness as previously shown in the assessed linear models. The largest vectors correspond to the three significant marginal effects of the model (altitude, pH, and S). S, which is the only constraint that extends toward the negative range of the first canonical component (CCA1), defines sample SUM002. pH and altitude are opposite to Mn2+, CEC, and P. Genera Geobacter and Ferribacterium are strongly associated with large pH and altitude, as are, both abundant in samples SUM003 (3808.3 m.a.s.l.), SUM004 (3808.2 m.a.s.l.), and SUM006 (2990 m.a.s.l.). Soil samples have been classified in high (blue color) and low-altitude (red color) and the overall distances between both groups of samples are large. The distance between high and low-altitude samples is more evident in a three-dimensional representation (Supplementary Video 1), which includes the third canonical component (CCA3). Overall, the representation provided by the CCA in Figure 3 shows: (1) a strong and unique correlation of S with a particular aspect of the bacterial community, which is not correlated to other environmental constraints and which is the only one to show significance in marginal effects of terms in the CCA model (X2 = 0.29, F = 5.50, df = 1, P = 0.005), (2) a strong and opposite effect of altitude and pH with inorganic nutrients and cations represented by CEC, Mn2+, and P, (3) the presence of numerous unknown taxa and unclassified genera, and (4) larger heterogeneity in high-altitude samples when compared to low-altitude samples, suggesting that at high altitude the microbiome is more heterogeneous than at low altitudes.
The abundance and distribution of the 25 most abundant families, across the altitudinal gradient, provided a broad perspective on different patterns, which we describe next. Samples were grouped by the similarities and differences of their microbiome community structure, with close correspondence to either high or low-altitude group classification, except for SUM005 (i.e., the sample-wide cluster on top of the heatmap), which is placed within the cluster of low-altitude samples. The family-wide cluster (the vertical cluster on the left of the heatmap), divided families into three clusters, with cluster 3 having most of the families with more abundance relative to those grouped in cluster 1 and 2; however, the latter had uniquely superabundant families. Notable taxa-wise differences in the heatmap correspond to Ktedonobacteraceae with larger abundance at higher altitudes and Nitrosomonadaceae, which, in sharp contrast to other samples, is absent in SUM002 (Figure 6).
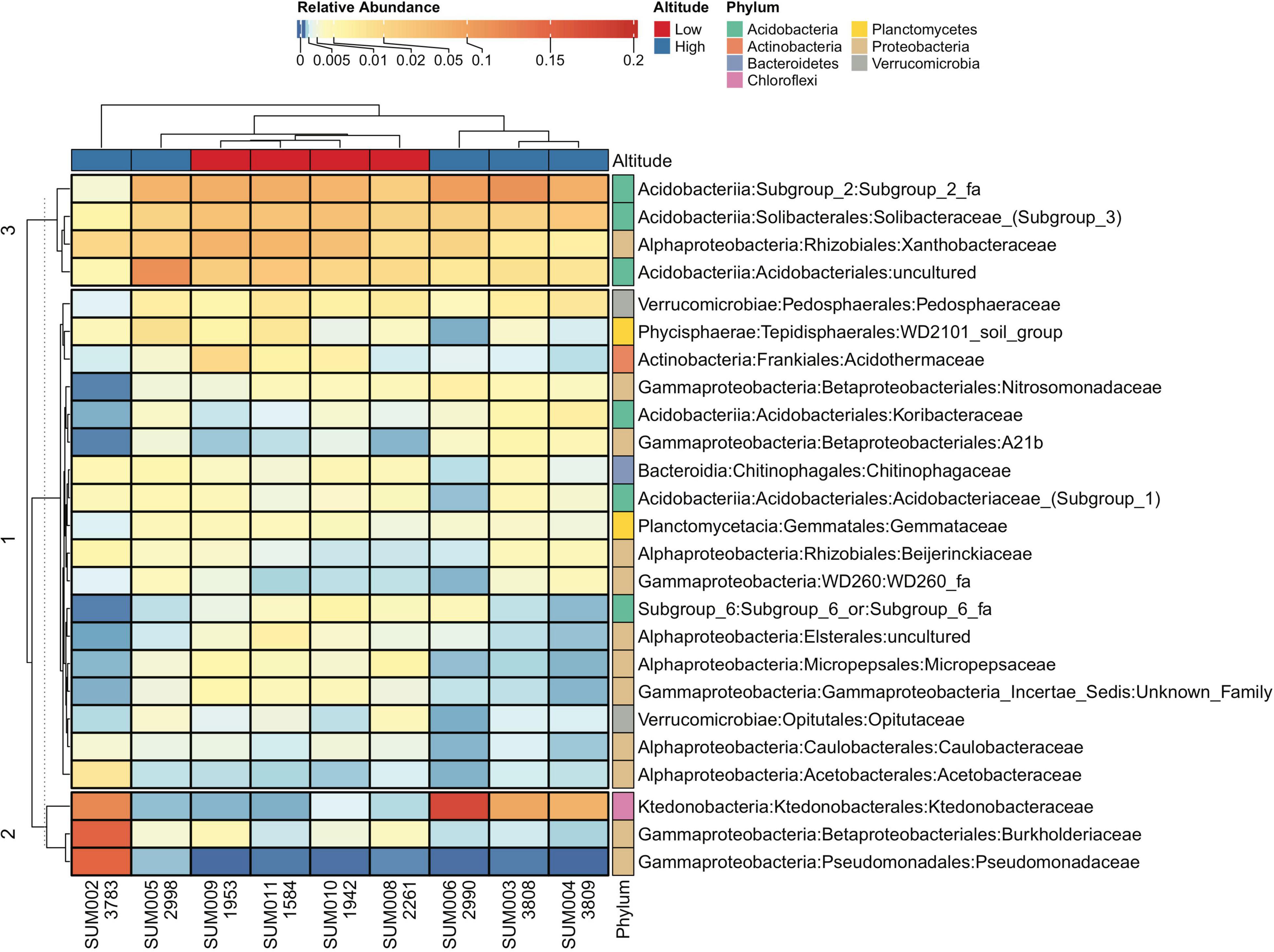
Figure 6. Heatmap of the bacterial soil community in soil samples for the 25 most abundant families. Samples have been classified as low (red) or high (blue) according to altitude. Except for sample SUM005, there is a sharp differentiation in community structure between high and low altitude samples. Values for altitude are included below each sample name.
The −log10 p-value was displayed against log2 fold change (low altitude/high altitude) to depict a volcano plot and evaluate genera that met the criteria of significance over the p < 0.05 threshold and | log2fc| > 0.6 (Figure 7). On the one hand, genera Dyella, Xylophilus, Haliangium, Rubrivivax and OLB12 had a significant and positive fold-change, with increased abundance at low altitudes. On the other hand, Rhodoblastus, Syntrophobacter, Geobacter, Candidatus, Nitrotoga, Geothrix, Paludibaculum, Rhizobacter, Desulfovibrio, Pseudolabrys, Rhodovastum, Acidisphaera, Sideroxydans, and Collimonas had a significant and negative fold-change, with increased abundance at high altitudes (which also corresponds to the distribution of genera in Figure 3). In synthesis, significant positive-fold or significant negative-fold changes set a strong contrast between the former two groups of bacterial genera in terms of their altitudinal niches.
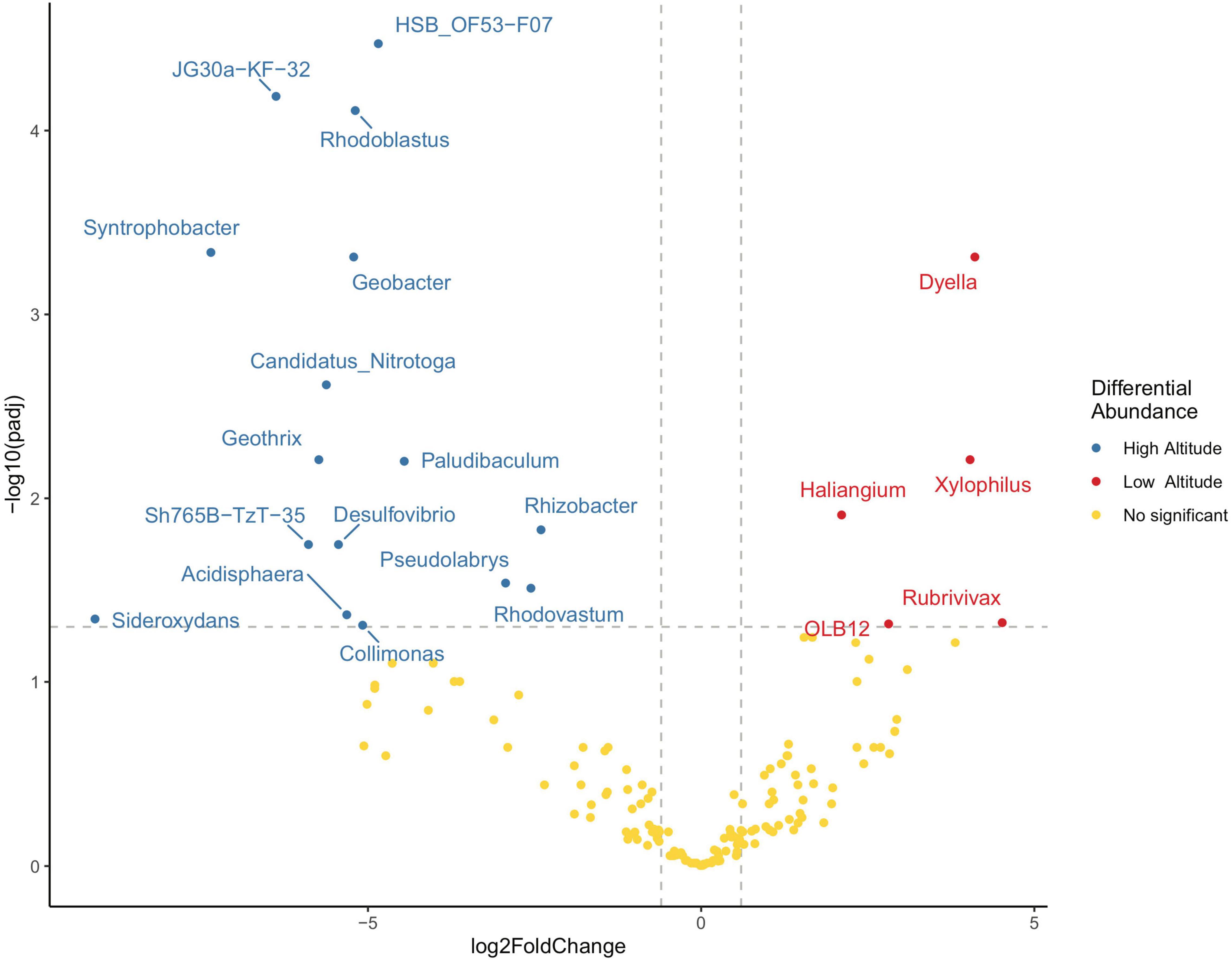
Figure 7. Volcano plot of the differential genera analysis for soil samples. The cutoff to minimize the false discovery rate was set at padj < 0.05 and | log2fc| > 0.6. Samples to the left are significantly more abundant at higher elevations, to the right at lower elevations. To improve the visualization of the results, unclassified taxa at the genus level were filtered.
Discussion and Conclusion
The present is a pioneering study of the microbiome in an isolated and poorly understood Amazonian volcano. It is the first to provide insights into the effects of an elevational gradient and associated physicochemical parameters for the Amazon region of Ecuador. The bacterial community profile, quantified along this unique volcanic elevational gradient, represents a snapshot of the pristine state of this forest and the foundations for further explorations of potential sources for biodiscovery. Our overarching hypothesis was to test if altitude contributed less, as a microbiome shaping variable, than characteristics inherent to soils such as pH. The latter, we believed, as part of the chemistry of soils, may influence the structure of the bacterial community more strongly and independently from abiotic factors such as elevation.
A first and univariate assessment of altitude on alpha-diversity was provided by a non-parametric contrast and a graphic overview of variability (a boxplot) among samples, where both diversity indexes (Shannon and Simpson) showed larger values at low altitudes, with significance on the Simpson index. It was evident that low altitude samples were more diverse than high-altitude samples, which concords with previous studies in other biomes such as the Himalayan desert (Kumar et al., 2019) and mountain grassland in China (Liu et al., 2017). As we have previously discussed, there are diverse patterns by which bacteria may respond to altitudinal gradients and do not fit a linear trend. Some reported trends for diversity along elevation have been fitted to a second-degree curve with correlation coefficients below 0.6 (Liu et al., 2016) or a hump-back curve (Han et al., 2018). The variety of estimated results for the effect of elevation may have to do with the inherent scale at which the microbiome operates (i.e., micrometers) and the presence of heterogeneous microenvironments along elevational gradients. For example, the hump-back curve observed in mount Fuji was attributed to heterogeneous perturbation gradients and the volcanic ash soil surface from mid-elevation upward (Singh et al., 2012). In the case of our study, elevation did show differences or an effect on richness, community structure, and diversity. However, these differences were not strongly supported or included samples that did not fully conform to a sharp distinction in the altitudinal gradient or the two altitudinal groups we proposed.
According to our quantitative assessment through a CCA, S was essential for unique features of the bacterial community. It was also the only one with statistical significance on a test for the marginal effects in the model (i.e., the effect of each environmental constraint on community structure when controlled for other constraints), it was mostly uncorrelated with other selected physicochemical parameters related to a single soil sample and associated to a few genera and groups. The CCA model also determined the significant contribution of altitude in explaining variation in the community. The latter two environmental parameters were important for separating bacterial communities in low and high-altitude groups and were significantly and positively correlated. The CCA model determined no significant effects on community structure for the remaining four physicochemical parameters pH, Mn2+, P, and CEC.
However, the linear model on richness determined that the largest contribution to the model was for Mn2+ with almost half of the explained R2, followed in order of importance by S, pH, P, altitude, and CEC. The partial effects of each one of the six physicochemical parameters on richness suggested that metallic cations (represented by Mn2+, and CEC) correlated positively with richness, while pH and S were negatively correlated. Richness and altitude had an inverse relationship when controlling for the effect of the physicochemical parameters. This last relationship had nevertheless a small contribution to the linear model (relative R2 = 8%).
We believe that our approach in using both a CCA on the overall community structure of bacteria (at the genus level) and an assessment of the linear effects on richness (at the genus level) provided complementary information that, for the most part, can be corroborated in the depiction of the CCA space (Figure 3). On the one hand, the largest concentration of taxa occurred in the direction of the positive variation of Mn2+, CEC, and P, which is quadrant I of the CCA space. On the other, S was an essential constraint to explain the structure of the community in terms of the presence of extreme taxa. We also determined strong and discernable differences in community structure and physicochemical profile between samples classified as low or high-altitude, where altitude and pH were inversely correlated with the concentration of nutrients as represented by Mn2+, CEC, and P.
The significant role of S in the soil of the Sumaco to determine the structure of bacterial communities may be related to the exceptional geological origin of this volcano, as part of the rear-arc of the Andes in Ecuador and with unique sulfate-saturated lava composition (Garrison et al., 2018). This is an incipient supposition that will require future analyses centered on the biogeochemistry of S and other related sulfur compounds related to the functional role of bacterial communities at Sumaco. Studies related to sulfur bacterial metabolism have mainly focused on the role it has in the bioremediation of heavy metals (Chen et al., 2019; Sun et al., 2019), but there are no comprehensive studies in the context of quantitative ecology.
We also found less variation in community structure for low-altitude samples, these were clumped in a relatively small area of the estimated space by the CCA, in sharp contrast to the large dispersion of high-altitude samples. The observed heterogeneity in community structure by high-altitude samples may be related to more chemically complex and diverse soils, in closer proximity to the volcanic crater, where recent geological events may have created larger physicochemical variability among differing soil patches. Sample SUM005, classified as part of the high-altitude group, clustered with low altitude samples in terms of its community structure and showed close distances to low-altitude samples in the CCA. This may not be unexpected due to the high heterogeneity observed in high-altitude samples and since sample SUM005 was closer to the low altitude threshold (<2900 m.a.s.l.).
Sharp differences in the abundance of specific genera, specialized to either high or low-altitude, were also possible to determine. For those which a formal genus has been identified, Dyella, Xylophilus, and Haliangium stand out as specialized to low altitude in contrast to Rhodoblastus, Geobacter, Rhizobacter, Pseudolabrys, and Rhodovastum which are specialized to high altitudes. Geobacter is of interest as it is known as an Fe(III) reductor (Caccavo et al., 1994). This functionality has been applied to different purposes (Shi et al., 2019); for example, to hydrocarbon-contaminated soils (Wang et al., 2019), electricity production (Bond and Lovley, 2003), and electrobiosynthesis (Gregory et al., 2004; Strycharz et al., 2008). Our study provides a baseline for future functional surveys to discover new species in Geobacter, particularly since these may be uniquely associated with high-altitude environments in Sumaco, near the volcanic crater.
In conclusion, the most relevant physicochemical parameter in our assessment of bacterial community structure was neither pH nor altitude, but S. The former two environmental parameters were strongly correlated, resulting in lower elevation soils having higher values for pH. These three environmental parameters (i.e., S, altitude, and pH) were the minimum necessary to explain community structure. However, an assessment of richness showed that the role of metallic ions was essential for higher numbers of taxa (i.e., richness). Recalling the hypothesis proposed in the “Introduction” section, we showed the importance of “partialing out” the effects of multiple correlated variables that may have specific roles in defining community structure and richness. Thus, even if altitude and pH are intimately correlated in the studied ecosystem at Sumaco, it is S, as an environmental parameter in soil, that seems to play a fundamental role in community structure. Yet, a complex ecosystem will depend on multiple environmental parameters, acting in complex networks of interactions, as we have hinted in our analysis.
Additional biotic and abiotic parameters may shed further insights on the ecological mechanisms determining bacterial communities in pristine environments, such as the effect of seasonality or daily cycles in temperature, rainfall (i.e., soil water content), and solar radiation. It is known that bacterial communities are constrained by the role of bacteria-consuming protozoa (Matz and Kjelleberg, 2005; Chan et al., 2021). Grazing by protozoans on soil bacteria favors transient cycles of abundance (Clarholm, 1981) and inputs of N and other nutrients into higher trophic levels of the ecosystem (Clarholm, 2002; Geisen et al., 2017). Understanding the broad interactions between populations of protozoa and bacteria, within an environmental envelope of soil physicochemical parameters and other environmental variables (e.g., solar radiation), will require a broader metagenomic approach and a longitudinal follow up through measures of time (e.g., days or season).
Our study sets the diversity profile for further and more detailed explorations of the features, potentials, and value of the bacteria occurring along the environmental gradient of the Sumaco, as a unique and seldom explored tropical stratovolcano. The study of the soil bacterial community of extreme environments, as the Sumaco volcano, will further contribute to the Ecuadorian Microbiome Project (EcuMP), which is an ambitious long-term initiative to research the bacterial diversity of Ecuador (Díaz et al., 2021).
Data Availability Statement
The studied sequences are available at NCBI with the BioProject accession number PRJNA744540. The scripts used for sequencing processing and analysis can be found at GitLab (http://gitlab.com/ec.microbiome.proj/sumaco-microbiome-year-1).
Author Contributions
MD: sample collection, laboratory experiments, data analysis, and writing initial draft. CQ-M: bioinformatic and data analysis. ER: sample collection and laboratory experiments. PC: sample collection. VA, WD, PM-L, and SP-E: bioinformatic analysis. FS: writing-review and editing. PJ-V: sample collection, data analysis, and writing-review and editing. CAM: sample collection, writing-review and editing, and grant administration. All authors contributed to the article and approved the submitted version.
Funding
This work was supported by The World Academy of Sciences through the TWAS Research Grants Programme, under Grant 16-172 RG/BIO/LA_I and La Cooperátion Belge au Développement through the program ARES-UCE and the project ARES-07-15K. PM-L was supported by a Research Initiation Grant from the University of Valencia.
Conflict of Interest
The authors declare that the research was conducted in the absence of any commercial or financial relationships that could be construed as a potential conflict of interest.
Publisher’s Note
All claims expressed in this article are solely those of the authors and do not necessarily represent those of their affiliated organizations, or those of the publisher, the editors and the reviewers. Any product that may be evaluated in this article, or claim that may be made by its manufacturer, is not guaranteed or endorsed by the publisher.
Acknowledgments
This work was an initiative of the Ecuadorian Microbiome Project (EcuMP). Our special recognition to the Pacto Sumaco community who provided us with guidance and care. This work was supported by The World Academy of Sciences (TWAS) and Belgium Academy of Research and Higher Education (ARES). We extend our gratitude to both funding institutions. The Ecuadorian Microbiome Project would not have been possible without the support of Central University of Ecuador. The Institute for Integrative Systems Biology (I2SysBio) at University of Valencia provided valuable assistance to this research.
Supplementary Material
The Supplementary Material for this article can be found online at: https://www.frontiersin.org/articles/10.3389/ffgc.2022.738568/full#supplementary-material
References
Anders, S., and Huber, W. (2010). Differential expression analysis for sequence count data. Genome Biol. 11:R106. doi: 10.1186/gb-2010-11-10-r106
Baird, R., Eaton, A., and Rice, E. (2017). Standard Methods for the Examination of Water and Wastewater, 23rd Edn. Washington, D.C: American Public Health Association.
Benjamini, Y., Hochberg, Y., and Benjamini Yoav, H. Y. (1995). Controlling the false discovery rate: a practical and powerfull approach to multiple testing. J. R. Stat. Soc. Ser. B 57, 289–300. doi: 10.2307/2346101
Bond, D., and Lovley, D. (2003). Electricity production by geobacter sulfurreducens attached to electrodes. Appl. Environ. Microbiol. 69, 1548–1555. doi: 10.1128/AEM.69.3.1548
Bruce, T., Martinez, I. B., Maia Neto, O., Vicente, A. C. P., Kruger, R. H., and Thompson, F. L. (2010). Bacterial community diversity in the brazilian atlantic forest soils. Microb. Ecol. 60, 840–849. doi: 10.1007/s00248-010-9750-9752
Caccavo, F., Lonergan, D. J., Lovley, D. R., Davis, M., Stolz, J. F., and Mcinerneyl, M. J. (1994). Oxidizing dissimilatory metal-reducing microorganism. Appl. Environ. Microbiol. 60, 3752–3759. doi: 10.1128/aem.60.10.3752-3759.1994
Cavaletti, L., Monciardini, P., Bamonte, R., Schumann, P., Rohde, M., Sosio, M., et al. (2006). New lineage of filamentous, spore-forming, gram-positive bacteria from soil. Appl. Environ. Microbiol. 72, 4360–4369. doi: 10.1128/AEM.00132-136
Chan, S. H., Ismail, M. H., Tan, C. H., Rice, S. A., and McDougald, D. (2021). Microbial predation accelerates granulation and modulates microbial community composition. BMC Microbiol. 21:91. doi: 10.1186/s12866-021-02156-2158
Chen, C., Li, L., Huang, K., Zhang, J., Xie, W.-Y., Lu, Y., et al. (2019). Sulfate-reducing bacteria and methanogens are involved in arsenic methylation and demethylation in paddy soils. ISME J. 13, 2523–2535. doi: 10.1038/s41396-019-0451-457
Clarholm, M. (1981). Protozoan grazing of bacteria in soil-impact and importance. Microb. Ecol. 7, 343–350. doi: 10.1007/BF02341429
Clarholm, M. (2002). Bacteria and protozoa as integral components of the forest ecosystem - their role in creating a naturally varied soil fertility. Antonie Van Leeuwenhoek 81, 309–318. doi: 10.1023/A:1020543424098
Colony, R. J., and Sinclair, J. H. (1928). The lavas of the volcano sumaco, Eastern ecuador, South America. Am. J. Sci. s5-16, 299–312. doi: 10.2475/ajs.s5-16.94.299
Corneo, P. E., Pellegrini, A., Cappellin, L., Roncador, M., Chierici, M., Gessler, C., et al. (2013). Microbial community structure in vineyard soils across altitudinal gradients and in different seasons. FEMS Microbiol. Ecol. 84, 588–602. doi: 10.1111/1574-6941.12087
de Carvalho, T. S., Jesus, E., da, C., Barlow, J., Gardner, T. A., Soares, I. C., et al. (2016). Land use intensification in the humid tropics increased both alpha and beta diversity of soil bacteria. Ecology 97, 2760–2771. doi: 10.1002/ecy.1513
de Gannes, V., Bekele, I., Dipchansingh, D., Wuddivira, M. N., De Cairies, S., Boman, M., et al. (2016). Microbial community structure and gunction of soil following ecosystem conversion from native forests to teak plantation forests. Front. Microbiol. 7:1976. doi: 10.3389/fmicb.2016.01976
Dedysh, S. N., Beletsky, A. V., Ivanova, A. A., Kulichevskaya, I. S., Suzina, N. E., Philippov, D. A., et al. (2021). Wide distribution of Phycisphaera-like planctomycetes from WD2101 soil group in peatlands and genome analysis of the first cultivated representative. Environ. Microbiol. 23, 1510–1526. doi: 10.1111/1462-2920.15360
Díaz, M., Jarrín-V, P., Simarro, R., Castillejo, P., Tenea, G. N., and Molina, C. A. (2021). The ecuadorian microbiome project: a plea to strengthen microbial genomic research. Neotrop. Biodivers. 7, 223–237. doi: 10.1080/23766808.2021.1938900
Fierer, N., McCain, C. M., Meir, P., Zimmermann, M., Rapp, J. M., Silman, M. R., et al. (2011). Microbes do not follow the elevational diversity patterns of plants and animals. Ecology 92, 797–804. doi: 10.1890/10-1170.1
Fonseca, J. P., Hoffmann, L., Cabral, B. C. A., Dias, V. H. G., Miranda, M. R., de Azevedo Martins, A. C., et al. (2018). Contrasting the microbiomes from forest rhizosphere and deeper bulk soil from an Amazon rainforest reserve. Gene 642, 389–397. doi: 10.1016/j.gene.2017.11.039
Fox, J., and Weisberg, S. (2019). An R Companion to Applied Regression, 3rd Edn. Thousand Oaks, CA: SAGE Publicatios, Inc.
Garrison, J. M., Sims, K. W. W., Yogodzinski, G. M., Escobar, R. D., Scott, S., Mothes, P., et al. (2018). Shallow-level differentiation of phonolitic lavas from Sumaco Volcano, Ecuador. Contrib. Mineral. Petrol. 173:19. doi: 10.1007/s00410-017-1431-1434
Geisen, S., Mitchell, E., Wilkinson, D., Adl, S., Bonkowski, M., Brown, M., et al. (2017). Soil protistology rebooted: 30 fundamental questions to start with. Soil Biol. Biochem. 111, 94–103. doi: 10.1016/j.soilbio.2017.04.001
Gregory, K. B., Bond, D. R., and Lovley, D. R. (2004). Graphite electrodes as electron donors for anaerobic respiration. Environ. Microbiol. 6, 596–604. doi: 10.1111/j.1462-2920.2004.00593.x
Grömping, U. (2006). Relative importance for linear regression in R: the package relaimpo. J. Stat. Softw. 17, 1–27. doi: 10.18637/jss.v017.i01
Gu, Z., Eils, R., and Schlesner, M. (2016). Complex heatmaps reveal patterns and correlations in multidimensional genomic data. Bioinformatics 32, 2847–2849. doi: 10.1093/bioinformatics/btw313
Han, D., Wang, N., Sun, X., Hu, Y., and Feng, F. (2018). Biogeographical distribution of bacterial communities in Changbai Mountain, Northeast China. Microbiologyopen 7, 1–9. doi: 10.1002/mbo3.529
Hendershot, J. N., Read, Q. D., Henning, J. A., Sanders, N. J., and Classen, A. T. (2017). Consistently inconsistent drivers of microbial diversity and abundance at macroecological scales. Ecology 98, 1757–1763. doi: 10.1002/ecy.1829
Hoffer, G., Eissen, J. P., Beate, B., Bourdon, E., Fornari, M., and Cotten, J. (2008). Geochemical and petrological constraints on rear-arc magma genesis processes in Ecuador: the Puyo cones and Mera lavas volcanic formations. J. Volcanol. Geotherm. Res. 176, 107–118. doi: 10.1016/j.jvolgeores.2008.05.023
Kandlikar, G. S., Gold, Z. J., Cowen, M. C., Meyer, R. S., Freise, A. C., Kraft, N. J. B., et al. (2018). ranacapa: an R package and Shiny web app to explore environmental DNA data with exploratory statistics and interactive visualizations. F1000Res 7:1734. doi: 10.12688/f1000research.16680.1
Klindworth, A., Pruesse, E., Schweer, T., Peplies, J., Quast, C., Horn, M., et al. (2013). Evaluation of general 16S ribosomal RNA gene PCR primers for classical and next-generation sequencing-based diversity studies. Nucleic Acids Res. 41:e1. doi: 10.1093/nar/gks808
Kozich, J. J., Westcott, S. L., Baxter, N. T., Highlander, S. K., and Schloss, P. D. (2013). Development of a dual-index sequencing strategy and curation pipeline for analyzing amplicon sequence data on the miseq illumina sequencing platform. Appl. Environ. Microbiol. 79, 5112–5120. doi: 10.1128/AEM.01043-1013
Kroeger, M. E., Meredith, L. K., Meyer, K. M., Webster, K. D., de Camargo, P. B., de Souza, L. F., et al. (2021). Rainforest-to-pasture conversion stimulates soil methanogenesis across the Brazilian Amazon. ISME J. 15, 658–672. doi: 10.1038/s41396-020-00804-x
Kumar, S., Suyal, D. C., Yadav, A., Shouche, Y., and Goel, R. (2019). Microbial diversity and soil physiochemical characteristic of higher altitude. PLoS One 14:e0213844. doi: 10.1371/journal.pone.0213844
Lemos, L. N., Pedrinho, A., Vasconcelos, A. T. R., de, Tsai, S. M., and Mendes, L. W. (2021). Amazon deforestation enriches antibiotic resistance genes. Soil Biol. Biochem. 153:108110. doi: 10.1016/j.soilbio.2020.108110
Liu, D., Wu, X., Shi, S., Liu, H., and Liu, G. (2016). A hollow bacterial diversity pattern with elevation in Wolong Nature Reserve, Western Sichuan Plateau. J. Soils Sediments 16, 2365–2374. doi: 10.1007/s11368-016-1422-1425
Liu, X., Cong, J., Lu, H., Xue, Y., Wang, X., Li, D., et al. (2017). Community structure and elevational distribution pattern of soil Actinobacteria in alpine grasslands. Acta Ecol. Sin. 37, 213–218. doi: 10.1016/j.chnaes.2017.02.010
Looby, C. I., and Martin, P. H. (2020). Diversity and function of soil microbes on montane gradients: the state of knowledge in a changing world. FEMS Microbiol. Ecol. 96:fiaa122. doi: 10.1093/femsec/fiaa122
Looby, C. I., Maltz, M. R., and Treseder, K. K. (2016). Belowground responses to elevation in a changing cloud forest. Ecol. Evol. 6, 1996–2009. doi: 10.1002/ece3.2025
Love, M. I., Huber, W., and Anders, S. (2014). Moderated estimation of fold change and dispersion for RNA-seq data with DESeq2. Genome Biol. 15, 1–21. doi: 10.1186/s13059-014-0550-558
Lozano, P., Cabrera, O., Peyre, G., Cleef, A., and Toulkeridis, T. (2020). Plant diversity and composition changes along an altitudinal gradient in the isolated volcano sumaco in the ecuadorian amazon. Diversity 12:229. doi: 10.3390/D12060229
Malhi, Y., Silman, M., Salinas, N., Bush, M., Meir, P., and Saatchi, S. (2010). Introduction: elevation gradients in the tropics: laboratories for ecosystem ecology and global change research. Glob. Chang. Biol. 16, 3171–3175. doi: 10.1111/j.1365-2486.2010.02323.x
Matz, C., and Kjelleberg, S. (2005). Off the hook – how bacteria survive protozoan grazing. Trends Microbiol. 13, 302–307. doi: 10.1016/j.tim.2005.05.009
McMurdie, P. J., and Holmes, S. (2013). Phyloseq: an R package for reproducible interactive analysis and graphics of microbiome census data. PLoS One 8:e61217. doi: 10.1371/journal.pone.0061217
Melo, V. F., Barros, L. S., Silva, M. C. S., Veloso, T. G. R., Senwo, Z. N., Matos, K. S., et al. (2021). Soil bacterial diversities and response to deforestation, land use and burning in North Amazon, Brazil. Appl. Soil Ecol. 158:103775. doi: 10.1016/j.apsoil.2020.103775
Merloti, L. F., Mendes, L. W., Pedrinho, A., de Souza, L. F., Ferrari, B. M., and Tsai, S. M. (2019). Forest-to-agriculture conversion in Amazon drives soil microbial communities and N-cycle. Soil Biol. Biochem. 137:107567. doi: 10.1016/j.soilbio.2019.107567
Nottingham, A. T., Fierer, N., Turner, B. L., Whitaker, J., Ostle, N. J., McNamara, N. P., et al. (2018). Microbes follow Humboldt: temperature drives plant and soil microbial diversity patterns from the Amazon to the Andes. Ecology 99, 2455–2466. doi: 10.1002/ecy.2482
Oksanen, J., Blanchet, G., Friendly, M., Kindt, R., Legendre, P., McGlinn, D., et al. (2020). Vegan: Community Ecology Package. Available online at: https://cran.r-project.org/package=vegan (accessed June 15, 2021).
Peay, K., Sperber, C., Cardarelli, E., Toju, H., Francis, C., Chadwick, O., et al. (2017). Convergence and contrast in the community structure of Bacteria, Fungi and Archaea along a tropical elevation-climate gradient. FEMS Microbiol. Ecol. 93, 1–12. doi: 10.1093/femsec/fix045
Puerini, M. (2009). Origin and evolution of the back arc magmatism of Ecuador (Northern volcanic zone, Andes): El Reventador and Sumaco active volcanoes. Plinius 35, 164–172.
Quast, C., Pruesse, E., Yilmaz, P., Gerken, J., Schweer, T., Yarza, P., et al. (2013). The SILVA ribosomal RNA gene database project: improved data processing and web-based tools. Nucleic Acids Res. 41, D590–D596. doi: 10.1093/nar/gks1219
R Core Team (2020). R: a Language and Environment for Statistical Computing. Vienna: R Foundation for Statistical Computing.
Ranjan, K., Paula, F. S., Mueller, R. C., Jesus, E., da, C., Cenciani, K., et al. (2015). Forest-to-pasture conversion increases the diversity of the phylum Verrucomicrobia in Amazon rainforest soils. Front. Microbiol. 6:779. doi: 10.3389/fmicb.2015.00779
Rognes, T., Flouri, T., Nichols, B., Quince, C., and Mahé, F. (2016). VSEARCH: a versatile open source tool for metagenomics. PeerJ 2016, 1–22. doi: 10.7717/peerj.2584
Rosenbaum, G., Sandiford, M., Caulfield, J., and Garrison, J. M. (2018). A trapdoor mechanism for slab tearing and melt generation in the northern Andes. Geology 47, 23–26. doi: 10.1130/G45429.1
Schloss, P. D., Westcott, S. L., Ryabin, T., Hall, J. R., Hartmann, M., Hollister, E. B., et al. (2009). Introducing mothur: open-source, platform-independent, community-supported software for describing and comparing microbial communities. Appl. Environ. Microbiol. 75, 7537–7541. doi: 10.1128/AEM.01541-1549
Shi, M. M., Jiang, Y. G., and Shi, L. (2019). Electromicrobiology and biotechnological applications of the exoelectrogens Geobacter and Shewanella spp. Sci. China Technol. Sci. 62, 1670–1678. doi: 10.1007/s11431-019-9509-9508
Sinclair, J., and Wasson, T. (1923). Explorations in Eastern Ecuador. Geogr. Rev. 13, 190–210. doi: 10.2307/208447
Singh, D., Lee-Cruz, L., Kim, W.-S., Kerfahi, D., Chun, J., and Adams, J. (2014). Strong elevational trends in soil bacterial community composition on Mt. Halla, South Korea. Soil Biol. Biochem. 68, 140–149. doi: 10.1016/j.soilbio.2013.09.027
Singh, D., Takahashi, K., Kim, M., Chun, J., and Adams, J. M. (2012). A Hump-Backed trend in bacterial diversity with elevation on mount Fuji, Japan. Microb. Ecol. 63, 429–437. doi: 10.1007/s00248-011-9900-9901
Souza, F. F. C., Mathai, P. P., Pauliquevis, T., Balsanelli, E., Pedrosa, F. O., Souza, E. M., et al. (2021). Influence of seasonality on the aerosol microbiome of the Amazon rainforest. Sci. Total Environ. 760:144092. doi: 10.1016/j.scitotenv.2020.144092
Stevens, M. H. H. (2009). “Community composition and diversity,” in A Primer of Ecology with R, ed. M. H. H. Stevens (New York, NY: Springer-Verlag New York), 285–333. doi: 10.1007/978-0-387-89882-7_10
Strycharz, S. M., Woodard, T. L., Johnson, J. P., Nevin, K. P., Sanford, R. A., Löffler, F. E., et al. (2008). Graphite electrode as a sole electron donor for reductive dechlorination of tetrachlorethene by Geobacter lovleyi. Appl. Environ. Microbiol. 74, 5943–5947. doi: 10.1128/AEM.00961-968
Sun, Z., Pang, B., Xi, J., and Hu, H.-Y. (2019). Screening and characterization of mixotrophic sulfide oxidizing bacteria for odorous surface water bioremediation. Bioresour. Technol. 290:121721. doi: 10.1016/j.biortech.2019.121721
von Humboldt, A., and Bondpland, A. (1805). Essai sur la Géographie des Plantes. Paris: Kessinger Publishing, LLC.
Wang, H., Lu, L., Mao, D., Huang, Z., Cui, Y., Jin, S., et al. (2019). Dominance of electroactive microbiomes in bioelectrochemical remediation of hydrocarbon-contaminated soils with different textures. Chemosphere 235, 776–784. doi: 10.1016/j.chemosphere.2019.06.229
Wang, Q., Garrity, G. M., Tiedje, J. M., and Cole, J. R. (2007). Naive Bayesian classifier for rapid assignment of rRNA sequences into the new bacterial taxonomy. Appl. Environ. Microbiol. 73, 5261–5267. doi: 10.1128/AEM.00062-67
Wei, T., and Simko, V. (2017). R Package “corrplot”: Visualization of a Correlation Matrix. Available online at: https://github.com/taiyun/corrplot (accessed June 15, 2021).
Westcott, S. L., and Schloss, P. D. (2017). OptiClust, an improved method for assigning amplicon-based sequence data to Operational Taxonomic Units. mSphere 2:e00073-17. doi: 10.1128/mSphereDirect.00073-17
Wu, J., Anderson, B. J., Buckley, H. L., Lewis, G., and Lear, G. (2017). Aspect has a greater impact on alpine soil bacterial community structure than elevation. FEMS Microbiol. Ecol. 93:fix032. doi: 10.1093/femsec/fix032
Yabe, S., Sakai, Y., Abe, K., Yokota, A., Také, A., Matsumoto, A., et al. (2017). Dictyobacter aurantiacus gen. nov., sp. nov., a member of the family Ktedonobacteraceae, isolated from soil, and emended description of the genus Thermosporothrix. Int. J. Syst. Evol. Microbiol. 67, 2615–2621. doi: 10.1099/ijsem.0.001985
Keywords: soil microbiome, microbial diversity, altitudinal gradient, volcano, Amazon region, environmental constraints
Citation: Díaz M, Quiroz-Moreno C, Jarrín-V P, Piquer-Esteban S, Monfort-Lanzas P, Rivadeneira E, Castillejo P, Arnau V, Díaz W, Sangari FJ and Molina CA (2022) Soil Bacterial Community Along an Altitudinal Gradient in the Sumaco, a Stratovolcano in the Amazon Region. Front. For. Glob. Change 5:738568. doi: 10.3389/ffgc.2022.738568
Received: 09 July 2021; Accepted: 28 February 2022;
Published: 24 March 2022.
Edited by:
Matthias Ros, Unidad Oaxaca, Centro Interdisciplinario de Investigación para el Desarrollo Integral Regional, Instituto Politécnico Nacional, MexicoReviewed by:
Alan Feest, University of Bristol, United KingdomBenito Mendoza, National University of Chimborazo, Ecuador
Copyright © 2022 Díaz, Quiroz-Moreno, Jarrín-V, Piquer-Esteban, Monfort-Lanzas, Rivadeneira, Castillejo, Arnau, Díaz, Sangari and Molina. This is an open-access article distributed under the terms of the Creative Commons Attribution License (CC BY). The use, distribution or reproduction in other forums is permitted, provided the original author(s) and the copyright owner(s) are credited and that the original publication in this journal is cited, in accordance with accepted academic practice. No use, distribution or reproduction is permitted which does not comply with these terms.
*Correspondence: C. Alfonso Molina, Y2Ftb2xpbmFAdWNlLmVkdS5lYw==