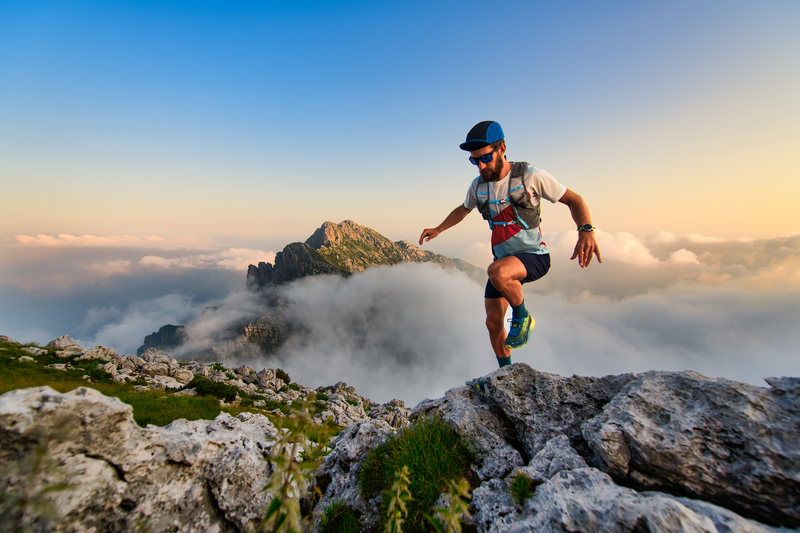
95% of researchers rate our articles as excellent or good
Learn more about the work of our research integrity team to safeguard the quality of each article we publish.
Find out more
ORIGINAL RESEARCH article
Front. For. Glob. Change , 05 December 2022
Sec. Forest Hydrology
Volume 5 - 2022 | https://doi.org/10.3389/ffgc.2022.1051210
This article is part of the Research Topic Natural Disturbance, Forest Management, and Ecosystem Functioning View all 4 articles
Forests have the largest terrestrial nutrient pools. The loss of soil carbon and nitrogen in forests under ongoing climate warming is subject to severe environmental degradation. To mitigate the negative effects of global warming on soil carbon and nitrogen in forest, it is important to obtain a better understanding of how elevated temperature and altered precipitation variability impact soil nutrient dynamics. To explore such interactions, we coupled an eco-hydrological model (Multi-Layer Canopy model, MLCan) with a biogeochemical model and applied the combined model to Pinus densiflora forest in Gwangneung Experimental Forest, South Korea, from 2004 to 2020. Our results showed that there was a time lag of 4 years to trigger soil organic carbon losses under the elevated temperature of +1.11°C during 2014–2020 compared to 2010–2013. A temperature rise over a prolonged period increased microbial biomass and activity, stimulating soil organic carbon decomposition. The combination of soil nitrate accumulation and exceptional but expected delay in heavy precipitation seasons of 2 months led to nitrate leaching four times higher than the average at 1 m depth in 2010. Reduced evapotranspiration and heavy precipitation during early fall caused intense subsurface water flux, resulting in a great increase in the risk of nitrate leaching. Our results highlight that the impacts of global warming on soil carbon decompositions has a time lag of 4 years and changes in precipitation characteristics will lead to excessive nitrate loss in P. densiflora forests under climate change.
Climate crisis is no longer a threat in the future. We are now facing the consequences of increased greenhouse gas emissions accumulated over the past decades. Global mean temperature has risen by 0.2°C per decade since the late 1970s (Allen et al., 2019) and by more than 1°C since the late 1800s (IPCC, 2013). A recent IPCC report (IPCC, 2021) notes that the rates of climate change, such as increase in atmospheric temperature and CO2 concentration, are higher than values that are projected a decade ago. In the past, scientists expected that when the global mean temperature exceeded 5°C above the mean of the pre-industrial period, natural systems would cross a tipping point, resulting in an irreversible shift. However, more recent studies have alerted that the shift is expected to occur when the global mean temperature exceeds 1.5°C above the pre-industrial mean (Cai et al., 2016; Lenton et al., 2020). In other words, we are close to or maybe even already in the midst of the irreversible transition period. Afforestation and reforestation are the dominant management practices to mitigate climate change (Lewis et al., 2019; Rohatyn et al., 2022). Reforestation of ~1,700 Mha would lead to an additional carbon sequestration of 205.7 billion tons (Bastin et al., 2019). However, there have been debates on whether soil organic carbon in forests will decrease or increase under the global warming (Kirschbaum, 2006; Bao et al., 2016; Kong et al., 2022).
Several studies have found a strong negative correlation between soil organic carbon and temperature (e.g., Follett et al., 2012; Pries et al., 2017; Yan et al., 2017; Li et al., 2020). An increase in temperature leads to stimulated microbial activity that increases soil organic matter decomposition. The rise in atmospheric temperature has an imperative role in determining soil carbon dynamics and vice versa (Zhao et al., 2017; Hartley et al., 2021). Since more carbon is stored as soil organic carbon than the sum of carbon in atmosphere and terrestrial vegetation which accounts for ~40% of terrestrial carbon (Jackson et al., 2017), soil carbon governs the global carbon cycle that influences the undergoing rapid human-driven climate change (Xu et al., 2018; Harris et al., 2021). However, other studies have shown that mean annual temperature have a positive effect on soil carbon accumulation in forests by increasing photosynthesis and thus litterfall and root death (Rodeghiero and Cescatti, 2005; Dusenge et al., 2019; Wang and Huang, 2020). Soil properties and climatic zones also influence these dynamics (Wang and Huang, 2020; Hu et al., 2021).
The trends of precipitation have also changed over the last decades with spatial, temporal, and periodic variations mainly due to the ongoing climate change (Pendergrass, 2018; Tabari et al., 2019; Tabari, 2020). Changes in the intensity of extreme precipitation and the variability of seasonal precipitation have become more pronounced than ever (Dourte et al., 2015; Tabari, 2020; Li et al., 2021). In particular, a rapid shift from low to abundant precipitation (known as, “precipitation whiplash”) has been observed globally, including the United States, East Asia, and Europe (Loecke et al., 2017; Coffey et al., 2018; Swain et al., 2018; Collins et al., 2019; Dai et al., 2022). In South Korea, heavy precipitation occurred during the summers of 2010 (2,044 mm yr−1) and 2020 (1,650 mm yr−1), which are considerably large compared to the previous years in 2009 (1,560 mm yr−1) and 2019 (890 mm yr−1). Note that the annual precipitation in 2020 is almost double the amount in 2019.
Nitrate is highly mobile in soil due to its negative charge and high solubility. An anticipated increase in the volume of precipitation caused by the ongoing climate change will increase soil nitrogen loss, leading to severe degradation in water quality (Loecke et al., 2017; Kruger et al., 2021). In particular, drought-to-flood transitions result in transporting accumulated nitrate during drought periods to adjacent water bodies (Loecke et al., 2017). A long-term experimental study (Rupp et al., 2021) also highlighted that the amount of precipitation alone could considerably increase the nitrogen loss by increased seepage fluxes. However, drought-to-flood transitions and increased precipitation do not necessarily result in increased soil nitrogen leaching (e.g., Gu and Riley, 2010; Hess et al., 2020). Soil texture and management also influence these nitrogen dynamics.
The goals of this study are (i) to explore the effects of warming on soil carbon and (ii) to examine the impacts of precipitation variability on soil nitrogen from 2004 to 2020. We used a mechanistic and process-based biogeochemical model (Woo and Kumar, 2019) coupled with an eco-hydrological model (Muti-Layer Canopy model, MLCan) for plant-water-energy dynamics. This coupled model has been validated using soil temperature, litterfall, root death, soil carbon, mineralization, and nitrification observed at Gwangneung Experimental Forest, South Korea. We applied the model to explore soil water, carbon, and nitrogen dynamics in response to the changes in temperature and precipitation observed at the experimental forest during the study period.
We explored soil carbon and nitrogen dynamics at Gwangneung Experimental Forest, South Korea (37°47′01″ N, 127°10′37″ E, 420 m above sea level). There are several tree species including Pinus densiflora, Carpinus laxiflora, and Quercus serrata. We studied soil carbon and nitrogen dynamics under P. densiflor, which is the most dominant tree species in the experimental forest and across South Korea, occupying nearly 23% of the entire forested areas in the country (Yoon et al., 2015; Woo and Do, 2021). This site has been protected from human influence since its designation as an experimental site since 1912, as a Long-Term Ecological Research, and Korean Flux Monitoring Network's Past, Present, and Future (KoFlux) since 2002 to explore and characterize forest ecosystem dynamics (Yoon et al., 2015).
The study site experienced strong variations in both temperature and precipitation during the study period. Mean temperature between 2004 and 2020 was 12.96°C with mean monthly temperature ranging between −2.06°C (January) and 26.50°C (August). The mean annual precipitation was 1,380 mm with a standard deviation of 78 mm. The soil type is silt loam with a bulk density of 1.02 g cm−3 and pH of 4.56 obtained from a previous experimental study of the site (Yoon et al., 2015). Based on the soil type, we assumed a saturated hydraulic conductivity of 0.02 mm, s−1, a saturated soil moisture of 0.45 m3 m−3, and residual soil moisture of 0.02 m3 m−3 (Oleson et al., 2013).
The coupled ecohydrological and biogeochemical model requires hourly weather forcings, such as precipitation, temperature, humidity, wind speed, solar radiation, and leaf area index. Since weather data were not available for the study site, a record of meteorological variables observed at an adjacent site (~30 km away) was used alternatively. The meteorological record for 2004–2020 (17 years) was obtained from Korea Meteorological Administration (https://data.kma.go.kr). For a leaf area index, we linearly interpolated 8-day leaf area index data collected from Moderate Resolution Imaging Spectroradiometer (Myneni et al., 2015), to hourly time series. Soil temperature, litterfall, root death, soil carbon, mineralization, and nitrification were measured at the study site at different time periods from 2004 to 2009 (Lee et al., 2009; Yoon et al., 2015; Yun and Chun, 2018), which were used for model validation to increase confidence of the coupled model in exploring carbon and nitrogen dynamics under the ongoing climate change.
We coupled an ecohydrological model with a biogeochemical model to explore the impacts of increased temperature and altered precipitation on soil nutrient dynamics (Figure 1) The multi-Layer Canopy model (MLCan) was used to estimate ecohydrological processes, such as soil moisture and temperature, evapotranspiration, CO2 fixation, and energy balance. MLCan is a process-based model that solves biophysical, physiological, and hydrological dynamics in an hourly interval by discretizing canopy and soil into multiple vertical layers (Drewry et al., 2010a,b). Each canopy layer has a unique photosynthesis process in sunlit and shaded leaves under microenvironment conditions. This model has been widely used to enhance the understanding over a gradient of contrasting ecosystems, including forest (Quijano et al., 2012, 2013; Quijano and Kumar, 2015; Richardson and Kumar, 2020), semiarid (Lee et al., 2018, 2021), agricultural (Drewry et al., 2010a,b, 2014; Le et al., 2011), and green roof (William et al., 2016) settings. In this paper, we briefly describe soil moisture, plant water uptake, and soil temperature dynamics, but not in details. A comprehensive description of MLCan model can be found in previous studies (Drewry et al., 2010a; Quijano et al., 2013; Quijano and Kumar, 2015).
Figure 1. A schematic diagram showing a coupled MLCan (left) and biogeochemical (right) model for the first soil layer. Multiple canopy and soil layers were implemented. Layers not shown in the right panel follow the first layer approach except for litterfall and surface nitrogen transport which only occur in the first soil layer. Precipitation, temperature, solar radiation, humidity, and wind speed were used as forcings. Soil moisture, temperature, subsurface water flux, and water uptake estimated in MLCan, directly and indirectly, influence soil organic carbon and nitrogen, and inorganic nitrogen dynamics. More details about this model including equations and parameters can be found in previous studies (Drewry et al., 2010a,b; Woo et al., 2014; Woo and Kumar, 2019).
Soil moisture and plant water uptake play important roles in soil carbon and nitrogen dynamics. Soil moisture is a key factor affecting soil microbial biomass and activity, mineralization, nitrification, and inorganic nitrogen transport. The soil depth of 5 m was used with 12 layers using a negative exponential profile depth function (Amenu and Kumar, 2008) where central nodes were located at 0.01, 0.05, 0.10, 0.18, 0.29, 0.44, 0.67, 0.98, 1.44, 2.09, 3.02, and 4.35 m below the surface. MLCan adopts a coupled root water uptake and Richards' equations by solving the soil and root water potentials for each soil layer (Quijano et al., 2012):
where θ is soil moisture [-]; t is time [T]; z is soil depth [L]; Ks is unsaturated soil hydraulic conductivity [LT−1]; ψs is soil water potential [L]; is the unit-upward vector; qe is a source and sink term [T−1], such as infiltration and bottom boundary condition (free flow); Kr is radial root conductivity [T−1]; ψr is root water potential [L]. This model solves hydraulic redistribution that leads to homogeneous soil water moisture over the columns of soil layers. In the model, transpiration is estimated using (i) root and leaf water potentials and (ii) resistance to flow through the plant (Drewry et al., 2010a), and is used as an upper boundary condition.
Soil temperature drives soil microbial activity and biomass, influencing soil carbon and nitrogen dynamics. MLCan uses a heat diffusion equation:
where Ts is soil temperature [K]; KT is thermal diffusivity [L2T−1], which is calculated as a function of soil moisture. Ground heat flux at the soil surface is used to estimate subsurface temperature by solving surface energy balance that accounts for latent, sensible, and ground heat flux and soil longwave emission. MLCan uses temperature gradient between the first soil layer (Ts,1) and atmosphere near the soil surface (Ta,1) to determine ground heat flux (G):
where dz0 is a thickness of the first soil layer.
We coupled MLCan with a biogeochemical model (Woo et al., 2014; Woo and Kumar, 2016, 2019; Roque-Malo et al., 2022) that followed the main structure of a carbon and nitrogen model developed by previous studies (D'Odorico et al., 2003; Porporato et al., 2003). A salient feature of this model is its mechanistic expressions of microbe-meditated carbon and nitrogen dynamics in the soil based on mass balance equations. Three soil carbon pools are considered, fast (or litter Cl), slow (or humus Ch), and microbial (Cb) pools (Figure 1). The carbon and nitrogen (CN) ratios of fast and slow pools varied during a simulation depending on weather, soil type, and litter inputs. A fixed CN ratio of microbial biomass (11.5) is used to account for soil carbon and nitrogen available to support and sustain soil microbial growths. When microbial nitrogen demands are not satisfied at the fixed CN ratio, soil microbial biomass is reduced based on the nitrogen availability and thus in microbe-meditated processes, such as mineralization, nitrification, and denitrification. Here, we briefly describe key carbon and nitrogen equations but not in full detail. A detailed description of the model can be found in previous studies (D'Odorico et al., 2003; Porporato et al., 2003; Woo et al., 2014; Woo and Kumar, 2016). Soil organic carbon pools are written as:
and
where Cl, Cb, and Ch are soil carbon concentration in the fast, slow, and microbial pools, respectively [ML−2]; Ii is litterfall [ML−2T−1]; Ir is root death [ML−2T−1]; kb is microbial death rate [T−1]; rh and rr are the fractions of humificated substrate and CO2 respiration, respectively, during litter decomposition [-]; Ranges of rh and rr are defined as following: 0 ≦ rr ≦ 1-rh. Kl and Kh are the decomposition rates of the fast and the slow carbon pools [T−1], which are given as:
and
where kl and kh are the weighted averages of decomposition rates for different compounds in the fast and slow carbon pools, respectively [T−1]. ϕ is a non-dimensional factor [-] that describes a nitrogen-limiting condition for a microbial growth. fθ and fT are non-dimensional factors [-] accounting for the effects of soil moisture and temperature, respectively, on decompositions. For simplicity of the model, microbial biomass and activity are assumed to have a linear relationship between them.
The model considers mineralization and microbial uptake to link soil inorganic nitrogen with soil organic matter (Figure 1, right panel). The positively charged ion of ammonium is attached to the negatively charged clay and retains ammonium against leaching. To account for this phenomenon, mobile and immobile ammonium are considered using dynamic volumetric fractions (D'Odorico et al., 2003; Woo and Kumar, 2016). The diffusion and dispersion terms are not considered in this study and thus inorganic nitrogen transport is restricted to advection. The mass balance of nitrate, and mobile and immobile ammonium are modeled, respectively as:
and
where the superscripts of − and + represent processes relevant to nitrate and ammonium respectively; N−, , and are nitrate, mobile ammonium, and immobile ammonium [ML−2], respectively; , , and represent the ratios of the volumetric fraction of nitrate, mobile ammonium, and immobile ammonium [-]; , , and are used to convert given volumes. Oi and Om are nitrification from mobile and immobile ammonium [ML−2T−1]; W− and W+ are atmospheric nitrate and ammonium deposition, respectively [ML−2T−1]; P− and P+ are (active, passive, and remobilized) plant nitrate and ammonium uptake, respectively [ML−2T−1]; L−, , and are microbial nitrate and mobile and immobile ammonium uptake, respectively [ML−2T−1]; Q is N2O production by nitrification [ML−2T−1]; J is denitrification [ML−2T−1]; is water flux between soil layers [LT−1]; M is mineralization [ML−2T−1]; Om and Oi are nitrifications estimated from mobile and immobile ammonium, respectively [ML−2T−1]; V is volatilization [ML−2T−1]; R is nitrogen exchange between mobile and immobile ammonium [ML−2T−1]. Through nitrification and ammonium mobility exchange, inorganic nitrogen dynamics are interconnected.
We used a spin-up period of 50 years before 2004 to minimize the influences of initial conditions of soil and canopy. Since weather forcings were not available during the period, we used a weather generator (Fatichi et al., 2010) to provide a series of precipitation, temperature, humidity, wind, and solar radiation with parameters estimated based on the observed weather record from 2004 to 2020. The generated hourly time series of synthetic metrological variables are statistically identical to the ones that are observed. Key variables are shown in Figures 2A–C. Soil and P. densiflora parameters used in this study were obtained from previous experimental and numerical studies (Table 1). The litterfall was estimated using leaf area index, leaf life span, and specific carbon leaf area index, following a formulation described in a previous study (Quijano et al., 2013). We assumed that the rate of root death had a linear relationship with litterfall (Ir = krIi, where kr is a constant representing litterfall-to-root death). Weather forcings were used as an upper canopy boundary condition while the free flow was used as a bottom soil boundary condition. To explore the impacts of drought-to-flood transition on nitrogen loss (Section 3.3), we conducted additional numerical experiments that were presented in Table 2. Model forcings, as well as measured and simulated variables used in this study are presented in Supplementary Table 1.
Figure 2. Forcings and initial conditions implemented in the simulation. Observed (A) hourly precipitation, (B) cumulative hourly precipitation, and (C) temperature in 2020 (black) overlaid on the ensemble of generated 50-year weather variables (gray). The nonlinear dynamics in (D) soil organic carbon (SOC), (E) organic carbon pools, and (F) inorganic nitrogen pools during the 50-year spin-up period before 2004 to reach a statistical equilibrium state for initial conditions.
Table 2. Numerical scenarios used in this study to explore the impacts of drought-to-flood transition on nitrogen loss in Section 3.3.
To capture abrupt shifts in time-dependent variables (Section 3.2), we used a Bayesian change-point detection model (Zhao et al., 2019). This model employs ensemble learning that uses the combination of multiple models through the Bayesian model average to provide the probabilities of change-point occurrences in a given time series. In addition, we evaluated the Pearson correlation coefficient to measure a linear correlation between two variables.
To explore the interactions between an abrupt precipitation event due to ongoing climate change and subsequent effects on nitrate losses, we estimated the Standardized Precipitation Index (SPI, McKee et al., 1993). A precipitation shift index (PSI) was also estimated to explore a shift of precipitation toward the winter (most precipitation occurs in July). Similar to a weather whiplash index developed by Loecke et al. (2017), PSI is calculated as the normalization of (i) the total precipitation during August and September of each year subtracted by (ii) the total precipitation during 10 months before August of each year, divided by the sum of them [(i) and (ii)] based on observed precipitation from 1908 to 2020.
The quasi-steady state of soil carbon and nitrogen was obtained from the 50-year spin-up simulation as an initial condition for 2004 (Figures 2D–F) because there were no experimental data at the beginning of the study period. Canopy and soil energy, water, temperature, carbon, and nitrogen modeled at the end of this spin-up period were used to initialize simulations for which results were analyzed below. Over the spin-up period, soil organic carbon and inorganic nitrogen have reached a statistical equilibrium state.
Once the model was set in equilibrium, we simulated the hydro-biogeochemical dynamics from 2004 to 2020 and compared modeled and observed soil temperature, litterfall, root death, soil organic carbon, mineralization, and nitrification. Note that the comparison periods were not consistent over 2014–2015 due to limited availability in observed data. The model accurately captured the seasonal variations of observed soil temperature to a depth of 0.15 m from 2004 to 2015 (Figures 3A,B, Yun and Chun, 2018). The coefficient of determination (R2) and root mean square error (RMSE) were 0.89 and 3.03°C, respectively. We compared observed litterfall averaged from 2007 to 2008 and from 2007 to 2009 (Lee et al., 2009) and root death averaged from 2007 to 2009 (Noh et al., 2017) with modeled values (Figures 3C,D). Since the only available observed data for litterfall and root death from previous experimental studies were mean values over multiple years, we validated the simulated results with the measurements averaged over multiple years. We found a strong agreement between modeled and observed carbon inputs (RMSE = 12.38 gC m−2 yr−1). Modeled soil organic carbon was similar to the measured value in 2007 to a depth of 0.3 m (Figure 3D, Lee et al., 2009). The model estimated soil organic carbon of 4,795 gC m−2 that was within the range of observed values. For inorganic nitrogen, we compared modeled and observed rates of mineralization and nitrification from March 2008 to April 2009 (Figures 3E,F, Yoon et al., 2015). The modeled mineralization and nitrification during the simulated period generally fell within the ranges of the observed values. Overall, modeled results seemed to coincide well with observed values, not only in a particular year but also over different observation periods, providing confidence in using the coupled model to explore the influence of ongoing climate change on soil nutrient dynamics.
Figure 3. Comparisons of modeled and observed (A,B) soil temperature to a depth of 0.15 m from 2004 to 2015 (Yun and Chun, 2018), (C) carbon inputs from 2007 to 2009 (Lee et al., 2009; Noh et al., 2017), (D) soil organic carbon to a depth of 0.3 m in 2007 (Lee et al., 2009), and (E) mineralization and (F) nitrification to a depth of 0.15 m from 2008 to 2009 (Yoon et al., 2015). The black line in (B) represents the trend line. Gray dashed lines in (B,C) represent a 1:1 relationship. The boxplot in (D) shows the distribution of observed soil organic carbon. The bar plots in (E,F) represent the observed ranges of mineralization and nitrification, respectively.
To explore the impacts of ongoing temperature rise on soil organic carbon, we first explored temperature variations from 2004 to 2020 (Figure 4A). We noted the extended period of years with higher than normal temperatures in recent years. We found two change-point (in 2010 and 2014) where pronounced temperature changes were detected during the study period (Figure 4B). Annual mean temperature was 13.37°C during 2014–2020, whereas the mean was 12.26°C during 2010–2013. In particular, differences in the mean annual minimum and maximum temperatures between the two periods were 2.56 and 2.08°C, respectively. The annual mean temperature during 2014–2020 was 0.69°C warmer than that over the previous 10 years (2004–2013). That is, the study site experienced prolonged periods of temperature up-shift in the recent years.
Figure 4. The impacts of temperature rise on soil organic carbon and microbial biomass. (A) Observed annual mean temperature and (B) its probabilities of trend changepoints, (C) modeled soil organic carbon, (D) its probabilities of trend changepoints, (E) modeled soil microbial biomass, and (F) its probabilities of trend changepoints during the study period. The shaded gray areas in (C,E) indicate the 20th and 80th percentiles that represent their variations in a given year. Red in (A) and blue (B,D,F) dotted lines represent linear trend lines and changepoints, respectively.
We found a decreasing trend in modeled soil organic carbon over the study period with the rate of −1.50 gC m−2 yr−1 (Figure 4C). In particular, the decrease in soil organic carbon became pronounced a few years after the elevated temperature. Trend changepoints of soil organic carbon were identified in 2008 and 2017 (Figure 4D). Soil microbial biomass acted as a key regulator of soil organic carbon decomposition due to microbial metabolism. The elevated temperature stimulated the growth and activity of soil microbes (Figure 4E). However, relationship between soil carbon and microorganisms did not show linear dynamics. We found that a year when soil carbon decreased (2017) was not synchronized with the year when microorganisms increased (2015, Figures 4D,F).
To further explore time-dependent temperature effects on soil organic carbon, temperature was averaged over different time windows: 1–10 years (Figure 5A). Among them, a change in soil organic carbon was the most significantly associated with 4-years average temperature with a linear trend of −4 gC m−2 °C−1 (P < 0.02). We noted that a prolonged period of warming accelerated the microbial decomposition of soil organic carbon (Figure 5B). The accumulated long-term warming drove the divergent growth trends of soil microbes, shifting soil organic carbon from a quasi-steady state to another. In other words, accumulated warming has been and will have a considerable influence on the growths of soil microbes, leading to an increase in soil carbon decomposition rate in the coming years.
Figure 5. Relationships between soil organic carbon, microbial biomass, and atmospheric temperature. (A) 1:1 plot for 4-year average annual temperature (x-axis) and changes in annual soil organic carbon (y-axis). (B) The relationship between annual average soil organic carbon (x-axis), soil microbial biomass (y-axis), and temperature (color bar). Soil organic carbon and microbial biomass were analyzed to a depth of 1 m.
The annual precipitation ranged from 792 to 2,044 mm with a mean and standard deviation of 1,380 and 377 mm, respectively, during the study period (Figure 6A). There was an insignificant relationship between interannual precipitation variations and soil ammonium (P > 0.05, Figure 6B). This irrelevance is mainly due to positively charged soil ammonium ion that is bonded to the negatively charged soil. On the other hand, the model predicted a considerable decrease in soil nitrate in 2010 due to a dramatic increase in nitrate leaching caused by high subsurface water flux (hereafter referred to as the 2010 N event) at a depth of 1 m (Figures 6C–F). Nitrate leaching in 2010 was more than four times higher than the average during the study period. Mean annual precipitation from 2004 to 2009 (6 years) was 1,445 mm while that in 2010 was 2,044 mm. The combination of intense precipitation and high nitrate concentration resulted in extreme nitrate losses in 2010. However, an abrupt change in annual precipitation (1,650 mm in 2020; mean annual precipitation of 891 mm during 2014–2019, over 6 years before 2020) combined with high nitrate concentration (13% higher than average) in 2020 did not lead to excess nitrate loss (hereafter referred to as the 2020 N event).
Figure 6. The impacts of abrupt precipitation changes on soil inorganic nitrogen dynamics. (A) Observed annual precipitation, and modeled (B) soil ammonium, (C) soil nitrate, (D) soil nitrate profile, (E) subsurface water flux, and (F) soil nitrate leaching at a depth of 1 m. Soil inorganic nitrogen dynamics were analyzed to a depth of 1 m. The shaded gray areas in (B,C,E) indicate the 20th and 80th percentiles that represent their variations in a given year. Red in (A) represents linear trend lines. Blue dotted lines represent 2010 and 2020, respectively, for visualization purposes.
For the case of the 2010 N event, we found an annual SPI of 0.56 (near normal) in 2009 followed by an annual SPI of 1.63 (severely wet) in 2010 (Figure 7A). The annual SPI estimated from 2004 to 2009 did not get below −1.0 (moderate drought). On the other hand, for the 2020 N event, an annual SPI of −1.40 (moderate drought) in 2019 was followed by 0.77 (near normal) in 2020. Under P2020useP×1.3 scenario, an annual SPI of 1.64 (severely wet) was modeled. However, the model showed that nitrate loss slightly increased from 0.010 (under baseline scenario) to 0.013 gN m−2 d−1 at a depth of 1 m (Figure 7B). The 2010 and 2020 N events modeled in this study are not explained by widely accepted findings of the impacts of drought-to-flood transition on the nitrogen loss.
Figure 7. Analysis of precipitation to explore excessive the 2010 N event. (A) The probability density function of yearly standardized precipitation index. (B) Soil nitrate leaching under baseline, a scenario of 2020 precipitation multiplied by 1.3 (P2020useP×1.3, red), a scenario of replacing 2020 precipitation with 2010 precipitation (P2020useP2010, blue), and a scenario of P2020useP2010 with substituting soil carbon and nitrate concentrations on Jan 1, 2010, for those on Jan 1, 2020 (P2020usePN2010, green). Dynamics associated with 2010 and 2020 were in dotted black and gray lines. (C) Monthly precipitation in 2010 was overlaid on monthly mean precipitation with associated standard deviations (error bars).
A question remains on identifying the key factors that drive excessive nitrate loss. To answer this question, additional numerical experiments were conducted. Under P2020useP2010 scenario, nitrate leaching at a depth of 1 m increased by 191% compared to the baseline (Figure 7B). Nitrate leaching further increased by 284% compared to the baseline under P2020usePN2010 scenario (Figure 7B). That is, the combination of accumulated nitrate in the soil and a unique precipitation event created excessive nitrate loss.
What is then a unique feature in the 2010 precipitation? We explored monthly precipitation characteristics during the study period (Figure 7C). The study site generally experiences the wettest month in July based on the observed precipitation records, which accounts for ~33% of the annual precipitation. However, the monthly distribution of the 2010 precipitation was exceptionally unique, receiving 599 and 672 mm in August and September, respectively. These amounts were two- and four-times greater than the monthly mean precipitation during the study period, respectively. Reduced evapotranspiration and heavy precipitation during late summer and early fall increased subsurface water flow, thus resulting in excessive nitrate loss. These results highlight that abnormally heavy precipitation during August and September combined with accumulated soil nitrate are important factors, rather than drought-to-flood transition, for excessive nitrate loss at the study site.
How frequently does precipitation shift toward winter occur? To address the question, we quantified a precipitation shift index (PSI, Figure 8). Analogous to SPI, PSI values >2 were used to indicate abnormal wetness during early fall. Between 1909 and 2020, 6 years experienced abnormal precipitation shift toward winter, where two-thirds of the shifts occurred during the last 30 years (from 1991 to 2020). Therefore, more precipitation shift is expected to be observed in the future.
Figure 8. To explore monthly precipitation shifts toward winter, we estimated (A) the precipitation shift index from 1909 to 2020 and (B) the frequency histogram of the precipitation shift index. The blue dotted line in (A) represents the precipitation shift index of 2. The black dotted and gray lines in (B) are for precipitation in 2010 and 2020, respectively.
The main purpose of this study was to assess the impacts of ongoing climate change on soil carbon and nitrogen dynamics. Using a coupled ecohydrological and biogeochemical model, we characterized (i) the influence of elevated temperature in recent years on soil organic carbon and (ii) the impacts of monthly precipitation characteristics on soil nitrate leaching in a mature P. densiflora forest. The model validation was carried out using available data (such as soil temperature, litterfall, root death, soil organic carbon, mineralization, and nitrification) measured at the study site in different periods between 2004 and 2015. Our results showed that elevated temperature led to decreases in soil organic carbon with a time lag of 4 years. The prolonged period of temperature rise increased the microbial biomass and activity over time, thus stimulating soil organic carbon decomposition. In addition, we noted that a combination of abnormal but expected extreme precipitation in August and September and accumulated soil nitrate resulted in excessive nitrate loss.
Changes in atmospheric temperature could, directly and indirectly, influence the fate of soil organic carbon by regulating soil microbial growth and activity, litterfall, root death, and soil moisture (e.g., Qi et al., 2016; Yan et al., 2017; Hartley et al., 2021). Several studies have explored the effects of climate warming on soil organic carbon in forests over several decades (Kasischeke et al., 1995; Melillo et al., 2011; Zhao et al., 2021; Nottingham et al., 2022). However, there is still no scientific consensus on whether soil organic carbon decreased or increased in forests under climate warming due to the contradictory results under different experimental and numerical conditions (Kirschbaum, 2006; Bao et al., 2016; Kong et al., 2022). Climate warming is found to simulate more rapid soil organic carbon loss since warming has a greater impact of soil respiration compared to photosynthesis (e.g., Qian et al., 2010; Nottingham et al., 2022) while other studies report results either that are opposite or show insignificant effects of warming on soil carbon decomposition (e.g., Melillo et al., 2011; Hartley et al., 2012). Among these contradictory results, an experimental study (Ofiti et al., 2021) found that elevated temperature over 4.5 years reduced root biomass and accelerated soil organic matter decomposition. Analogous to that study, our results showed that the prolonged period of elevated temperature stimulated soil microbial biomass, leading to consequent decrease in soil organic carbon 4 years after the onset of temperature elevation. Demonstrating the connection between long-term elevated temperature and soil carbon loss leads us to infer that climate change stimulates soil carbon loss in a P. densiflora forest.
Using the modeling approach, we characterized the impacts of precipitation whiplash on nitrate loss. In this study, we defined precipitation whiplash that caused an excessive nitrogen loss as the occurrence of abnormally high precipitation in late summer and early fall (August and September) at the study site. The combination of precipitation whiplash and relatively high soil nitrate concentration due to prolonged dry periods resulted in extreme nitrate leaching. A precipitation whiplash event occurred during the study period over 17 years. However, there has been already substantial evidence of changes in precipitation characteristics due to global warming. For example, California, United States, forecasts more frequent occurrences of extremely dry and wet seasons in the twenty-first century (Swain et al., 2018). An increase in frequency and intensity of extreme events has also been observed over East Asia during the past decades partially due to a shift of high-frequency tropical cyclones toward the north (Lee et al., 2021; Ren et al., 2021). A global study projected a permanent shift of heavy precipitation events over a few days to months at the end of the twenty-first century (Marelle et al., 2018). In addition, it has been widely observed that the variation and intensity of precipitation are one of the most important factors governing nitrate leaching (e.g., Di and Cameron, 2002; Jabloun et al., 2015; He et al., 2018). Nitrogen in groundwater, especially in an unconfined aquifer, is a direct response to nitrogen leaching from the vadose zone (Hansen et al., 2017; Kuchta et al., 2020). Petrovic (1990) found that there is a great potential for nitrate leaching under increased precipitation in the late fall due to reduced evapotranspiration and decreased microbial activity. These findings support the results of this study that precipitation shift toward winter will result in a high risk of nitrate leaching.
This study considered only a single tree, P. densiflora, and used one coupled model to explore the impacts of ongoing climate change on soil carbon and nitrogen dynamics. To extend our results in future analyses, we recommend that the long-term effects of climate change, various trees, and parameter uncertainties be examined further to assess the impacts of warming and altered precipitation characteristics on forest soil carbon and nitrogen dynamics. However, there is no doubt that the maintenance of forest health and vitality is a key aspect of climate change mitigation. Forests are important carbon and nitrogen pools that serve as a safety net under climate change. Our study showed that the persistence of elevated temperature and abnormally heavy precipitation during late summer and early fall could lead to severe environmental consequences. Scientists have argued that multiple tipping points could be triggered if global warming exceeds 1.5°C above pre-industrial levels (Cai et al., 2016; Lenton et al., 2020). Under such conditions, it will not be surprising if forest ecosystems do not function properly as we have expected and observed. Therefore, in light of the findings obtained from this study, we conclude that climate change has been already influencing carbon and nitrogen dynamics in P. densiflora forests.
The original contributions presented in the study are included in the article/Supplementary material, further inquiries can be directed to the corresponding author/s.
DW and YS: conceptualization, formal analysis, and writing—review and editing. DW: writing—original draft preparation. All authors have read and agreed to the published version of the manuscript.
This research was supported by the Bisa Research Grant of Keimyung University in 2020.
The authors declare that the research was conducted in the absence of any commercial or financial relationships that could be construed as a potential conflict of interest.
All claims expressed in this article are solely those of the authors and do not necessarily represent those of their affiliated organizations, or those of the publisher, the editors and the reviewers. Any product that may be evaluated in this article, or claim that may be made by its manufacturer, is not guaranteed or endorsed by the publisher.
The Supplementary Material for this article can be found online at: https://www.frontiersin.org/articles/10.3389/ffgc.2022.1051210/full#supplementary-material
Achat, D. L., Pousse, N., Nicolas, M., and Augusto, L. (2018). Nutrient remobilization in tree foliage as affected by soil nutrients and leaf life span. Ecol. Monogr. 88, 408–428. doi: 10.1002/ecm.1300
Allen, M., de Coninck, H., Dube, O. P., OveHoegh-Guldberg, Jacob, D., Jiang, K., et al. (2019). Technical Summary: Global Warming of 1.5°C. An IPCC Special Report on the Impacts of Global Warming of 1.5°C Above Pre-Industrial Levels and Related Global Greenhouse Gas Emission Pathways, in the Context of Strengthening the Global Response to the Threat of Climate Change, Sustainable Development, and Efforts to Eradicate Poverty. Technical Report, The Intergovernmental Panel on Climate Change.
Amenu, G. G., and Kumar, P. (2008). A model for hydraulic redistribution incorporating coupled soil-root moisture transport. Hydrol. Earth Syst. Sci. 12, 55–74. doi: 10.5194/hess-12-55-2008
Bao, X., Zhu, X., Chang, X., Wang, S., Xu, B., Luo, C., et al. (2016). Effects of soil temperature and moisture on soil respiration on the Tibetan Plateau. PLoS ONE 11:e0165212. doi: 10.1371/journal.pone.0165212
Bastin, J.-F., Finegold, Y., Garcia, C., Mollicone, D., Rezende, M., Routh, D., et al. (2019). The global tree restoration potential. Science 365, 76–79. doi: 10.1126/science.aax0848
Cai, Y., Lenton, T. M., and Lontzek, T. S. (2016). Risk of multiple interacting tipping points should encourage rapid co2 emission reduction. Nat. Clim. Change 6, 520–525. doi: 10.1038/nclimate2964
Carsel, R. F., and Parrish, R. S. (1988). Developing joint probability distributions of soil water retention characteristics. Water Resour. Res. 24, 755–769. doi: 10.1029/WR024i005p00755
Coffey, R., Paul, M. J., Stamp, J., Hamilton, A., and Johnson, T. (2018). A review of water quality responses to air temperature and precipitation changes 2: nutrients, algal blooms, sediment, pathogens. J. Am. Water Resour. Assoc. 55, 844–868. doi: 10.1111/1752-1688.12711
Collins, S. M., Yuan, S., Tan, P. N., Oliver, S. K., Lapierre, J. F., Cheruvelil, K. S., et al. (2019). Winter precipitation and summer temperature predict lake water quality at macroscales. Water Resour. Res. 55, 2708–2721. doi: 10.1029/2018WR023088
Cousins, I. T., Mackay, D., and Jones, K. C. (1999.). Measuring and modelling the vertical distribution of semi-volatile organic compounds in soils. II: Model development. Chemosphere 39, 2519–2534. doi: 10.1016/S0045-6535(99)00165-4
Dai, L., Cheng, T. F., and Lu, M. (2022). Anthropogenic warming disrupts intraseasonal monsoon stages and brings dry-get-wetter climate in future East Asia. NPJ Clim. Atmos. Sci. 5:11. doi: 10.1038/s41612-022-00235-9
Di, H. J., and Cameron, K. C. (2002). Nitrate leaching in temperate agroecosystems: sources, factors and mitigating strategies. Nutr. Cycl. Agroecosyst. 64, 237–256. doi: 10.1023/A:1021471531188
D'Odorico, P., Laio, F., Porporato, A., and Rodriguez-Iturbe, I. (2003). Hydrologic controls on soil carbon and nitrogen cycles. II. A case study. Adv. Water Resour. 26, 59–70. doi: 10.1016/S0309-1708(02)00095-7
Dourte, D. R., Fraisse, C. W., and Wendy-LinBartelsb (2015). Exploring changes in rainfall intensity and seasonal variability in the Southeastern U.S.: stakeholder engagement, observations, and adaptation. Clim. Risk Manage. 7, 11–19. doi: 10.1016/j.crm.2015.02.001
Drewry, D. T., Kumar, P., Long, S., Bernacchi, C., Liang, X.-Z., and Sivapalan, M. (2010a). Ecohydrological responses of dense canopies to environmental variability: 1. Interplay between vertical structure and photosynthetic pathway. J. Geophys. Res. 115:G04002. doi: 10.1029/2010JG001340
Drewry, D. T., Kumar, P., Long, S., Bernacchi, C., Liang, X.-Z., and Sivapalan, M. (2010b). Ecohydrological responses of dense canopies to environmental variability: 2. Role of acclimation under elevated CO2. J. Geophys. Res. 115:G04023. doi: 10.1029/2010JG001341
Drewry, D. T., Kumar, P., and Long, S. P. (2014). Simultaneous improvement in productivity, water use, and albedo through crop structural modification. Glob. Change Biol. 20, 1955–1967. doi: 10.1111/gcb.12567
Dusenge, M. E., Duarte, A. G., and Way, D. A. (2019). Plant carbon metabolism and climate change: elevated co2 and temperature impacts on photosynthesis, photorespiration and respiration. New Phytol. 221, 32–49. doi: 10.1111/nph.15283
Fatichi, S., Ivanov, V. Y., and Caporali, E. (2010). Simulation of future climate scenarios with a weather generator. Adv. Water Resour. 34, 448–467. doi: 10.1016/j.advwatres.2010.12.013
Follett, R. F., Stewart, C. E., Pruessner, E. G, and Kimble, J. M. (2012). Effects of climate change on soil carbon and nitrogen storage in the US Great Plains. J. Soil Water Conserv. 67, 331–342. doi: 10.2489/jswc.67.5.331
Gu, C., and Riley, W. J. (2010). Combined effects of short term rainfall patterns and soil texture on soil nitrogen cycling - a modeling analysis. J. Contam. Hydrol. 112, 141–154. doi: 10.1016/j.jconhyd.2009.12.003
Han, Q., Kawasaki, T., Nakano, T., and Chiba, Y. (2008). Leaf-age effects on seasonal variability in photosynthetic parameters and its relationships with leaf mass per area and leaf nitrogen concentration within a Pinus densiflora crown. Tree Physiol. 28, 551–558. doi: 10.1093/treephys/28.4.551
Hansen, B., Thorling, L., Schullehner, J., Termansen, M., and Dalgaard, T. (2017). Groundwater nitrate response to sustainable nitrogen management. Sci. Rep. 7:8566. doi: 10.1038/s41598-017-07147-2
Harris, N. L., Gibbs, D. A., Baccini, A., Birdsey, R. A., de Bruin, S., Farina, M., et al. (2021). Global maps of twenty-first century forest carbon fluxes. Nat. Clim. Chang. 11, 234–240. doi: 10.1038/s41558-020-00976-6
Hartley, I. P., Garnett, M. H., Sommerkorn, M., Hopkins, D. W., Fletcher, B. J., Sloan, V. L., et al. (2012). A potential loss of carbon associated with greater plant growth in the european arctic. Nat. Clim. Change 2, 874–879. doi: 10.1038/nclimate1575
Hartley, I. P., Hill, T. C., Chadburn, S. E., and Hugelius, G. (2021). Temperature effects on carbon storage are controlled by soil stabilisation capacities. Nat. Commun. 12:6713. doi: 10.1038/s41467-021-27101-1
Hauer, R. J. (2008). Forever Not Green–Fall Leaf Drop of Conifers. Technical Report, AREA E-Newsletter. Arboricultural Research and Education Academy.
He, W., Yang, J. Y., Qian, B., Drury, C. F., Hoogenboom, G., He, P., et al. (2018). Climate change impacts on crop yield, soil water balance and nitrate leaching in the semiarid and humid regions of Canada. PLoS ONE 13:e0207370. doi: 10.1371/journal.pone.0207370
Hess, L. J. T., Hinckley, E.-L. S., Robertson, G. P., and Matson, P. A. (2020). Rainfall intensification increases nitrate leaching from tilled but not no-till cropping systems in the U.S. Midwest. Agric. Ecosyst. Environ. 290:106747. doi: 10.1016/j.agee.2019.106747
Hillel, D. (1998). Environmental Soil Physics: Fundamentals, Applications, and Environmental Considerations. Waltham, MA: Academic Press.
Hu, P., Zhang, W., Chen, H., Li, D., Zhao, Y., Zhao, J., et al. (2021). Soil carbon accumulation with increasing temperature under both managed and natural vegetation restoration in calcareous soils. Sci. Tot. Environ. 767:145298. doi: 10.1016/j.scitotenv.2021.145298
IPCC (2013). Summary for Policymakers. Climate Change 2013: The Physical Science Basis. Contribution of Working Group I to the Fifth Assessment Report of the Intergovernmental Panel on Climate Change. Cambridge, UK; New York, NY: Cambridge University Press.
IPCC (2021). Climate Change 2021: The Physical Science Basis. Contribution of Working Group I to the SixthAssessment Report of the Intergovernmental Panel on Climate Change. Cambridge, UK; New York, NY: Cambridge University Press.
Jabloun, M., Schelde, K., Tao, F., and Olesen, J. E. (2015). Effect of temperature and precipitation on nitrate leaching from organic cereal cropping systems in denmark. Eur. J. Agron. 62, 55–64. doi: 10.1016/j.eja.2014.09.007
Jackson, R. B., Lajtha, K., Crow, S. E., Hugelius, G., Kramer, M. G., and Pineiro, G. (2017). The ecology of soil carbon: pools, vulnerabilities, and biotic and abiotic controls. Annu. Rev. Ecol. Evol. Syst. 48, 419–445. doi: 10.1146/annurev-ecolsys-112414-054234
Kasischeke, E. S., Christensen, N. L. Jr, and Stocks, B. J. (1995). Fire, global warming, and the carbon balance of boreal forests. Ecol. Appl. 5, 437–451.
Kimm, H., and Ryu, Y. (2015). Seasonal variations in photosynthetic parameters and leaf area index in an urban park. Urban For. Urban Green. 14, 1059–1067. doi: 10.1016/j.ufug.2015.10.003
Kirschbaum, M. U. F. (2006). The temperature dependence of organic-matter decomposition–still a topic of debat. Soil Biol. Biochem. 38, 2510–2518. doi: 10.1016/j.soilbio.2006.01.030
Kong, J., He, Z., Chen, L., Zhang, S., Yang, R., and Du, J. (2022). Elevational variability in and controls on the temperature sensitivity of soil organic matter decomposition in alpine forests. Ecosphere 13:e4010. doi: 10.1002/ecs2.4010
Kruger, M., Potthast, K., Michalzik, B., Tischer, A., Kusel, K., Deckner, F. F. K., et al. (2021). Drought and rewetting events enhance nitrate leaching and seepage-mediated translocation of microbes from beech forest soils. Soil Biol. Biochem. 154:108153. doi: 10.1016/j.soilbio.2021.108153
Kuchta, S., Neilsen, D., Forge, T., Zebarth, B. J., and Nichol, C. (2020). Nitrogen, irrigation, and alley management effects on nitrate leaching from raspberry. Vadose Zone J. 19:e20054. doi: 10.1002/vzj2.20054
Le, P. V. V., Kumar, P., and Drewry, D. T. (2011). Implications for the hydrologic cycle under climate change due to the expansion of bioenergy crops in the midwestern united states. Proc. Natl. Acad. Sci. U.S.A. 108, 15085–15090. doi: 10.1073/pnas.1107177108
Lee, A.-R., Noh, N.-J., Cho, Y.-S., Lee, W.-K., and Son, Y.-W. (2009). Estimating the soil carbon stocks for a pinus densiflora forest using the soil carbon model, yasso. J. Ecol. Environ. 32, 47–53. doi: 10.5141/JEFB.2009.32.1.047
Lee, E., Kumar, P., Barron-Gafford, G. A., Hendryx, S. M., Sanchez-Canete, E. P., Minor, R. L., et al. (2018). Impact of hydraulic redistribution on multispecies vegetation water use in a semiarid savanna ecosystem: an experimental and modeling synthesis. Water Resour. Res. 54, 4009–4027. doi: 10.1029/2017WR021006
Lee, E., Kumar, P., Knowles, J. F., Minor, R. L., Tran, N., Barron-Gafford, G. A., et al. (2021). Convergent hydraulic redistribution and groundwater access supported facilitative dependency between trees and grasses in a semi-arid environment. Water Resour. Res. 57:e2020WR028103. doi: 10.1029/2020WR028103
Lee, S. J., Han, S., Yoon, T. K., Han, S. H., Jung, Y., Yun, S. J., et al. (2013). Growth and physiological characteristics of pinus densiflora seedlings in response to open-field experimental warming using the infrared lamp. J. Korean Soc. For. Sci. 102, 522–529. doi: 10.14578/jkfs.2013.102.4.522
Lenton, T. M., Rockstrom, J., Gaffney, O., Rahmstorf, S., Richardson, K., Steffen, W., et al. (2020). Climate tipping points—too risky to bet against. Nature 575, 592–595. doi: 10.1038/d41586-019-03595-0
Lewis, S. L., Wheeler, C. E., Mitchard, E. T. A., and Koch, A. (2019). Restoring natural forests is the best way to remove atmospheric carbon. Nature 568, 25–28. doi: 10.1038/d41586-019-01026-8
Li, J., Pei, J., Pendall, E., Reich, P. B., Noh, N. J., Li, B., et al. (2020). Rising temperature may trigger deep soil carbon loss across forest ecosystems. Adv. Sci. 7:2001242. doi: 10.1002/advs.202001242
Li, Y., Bai, J., You, Z., Hou, J., and Li, W. (2021). Future changes in the intensity and frequency of precipitation extremes over China in a warmer world: Insight from a large ensemble. PLoS ONE 16:e0252133. doi: 10.1371/journal.pone.0252133
Loecke, T. D., Burgin, A. J., Riveros-Iregui, D. A., Ward, A. S., Thomas, S. A., Davis, C. A., et al. (2017). Weather whiplash in agricultural regions drives deterioration of water quality. Biogeochemistry 113, 7–15. doi: 10.1007/s10533-017-0315-z
Marelle, L., Myhre, G., Hodnebrog, O., Sillmann, J., and Samset, B. H. (2018). The changing seasonality of extreme daily precipitation. Geophys. Res. Lett. 45, 11352–11360. doi: 10.1029/2018GL079567
McKee, T. B., Doesken, N. J., and Kleist, J. (1993). “The relationship of drought frequency and duration to time scales,” in Proceedings of the Eighth Conference on Applied Climatology, American Meteorological Society (Anaheim, CA), 179–184.
Melillo, J. M., Sarah Butler, J. J., Mohan, J., Steudler, P., Lux, H., Burrows, E., et al. (2011). Soil warming, carbon–nitrogen interactions, and forest carbon budgets. Proc. Natl. Acad. Sci. U.S.A. 108, 9508–9512. doi: 10.1073/pnas.1018189108
Myneni, R., Knyazikhin, Y., and Park, T. (2015). MCD15A2H MODIS/Terra+Aqua Leaf Area Index/FPAR 8-day L4 Global 500m SIN Grid V006. NASA EOSDIS Land Processes DAAC. doi: 10.5067/MODIS/MCD15A2H.006
Noh, N. J., Yoon, T. K., Kim, R.-H., Bolton, N. W., Kim, C., and Son, Y. (2017). Carbon and nitrogen accumulation and decomposition from coarse woody debris in a naturally regenerated Korean red pine (Pinus densiflora s. et z.) forest. Forests 8:214. doi: 10.3390/f8060214
Nottingham, A. T., Gloor, E., Baath, E., and Meir, P. (2022). Soil carbon and microbes in the warming tropics. Funct. Ecol. 36, 1338–1354. doi: 10.1111/1365-2435.14050
Ofiti, N. O. E., Zosso, C. U., Soong, J. L., Solly, E. F., Torn, M. S., Wiesenberg, G. L. B., et al. (2021). Warming promotes loss of subsoil carbon through accelerated degradation of plant-derived organic matter. Soil Biol. Biochem. 156:108185. doi: 10.1016/j.soilbio.2021.108185
Oleson, K., Lawrence, D. M., Bonan, G. B., Drewniak, B., Huang, M., Koven, C. D., et al. (2013). Technical Description of Version 4.5 of the Community Land Model (CLM). Boulder, CO doi: 10.5065/D6RR1W7M
Pendergrass, A. G. (2018). What precipitation is extreme? Science 360, 1072–1073. doi: 10.1126/science.aat187
Petrovic, A. M. (1990). The fate of nitrogenous fertilizers applied to turfgass. J. Environ. Qual. 19, 1–14. doi: 10.2134/jeq1990.00472425001900010001x
Porporato, A., D'Odorico, P., Laio, F., and Rodriguez-Iturbe, I. (2003). Hydrologic controls on soil carbon and nitrogen cycles. I. Modeling scheme. Adv. Water Resour. 26, 45–58. doi: 10.1016/S0309-1708(02)00094-5
Pries, C. E. H., Castanha, C., Porras, R. C., and Torn, M. S. (2017). The whole-soil carbon flux in response to warming. Science 355, 1420–1423. doi: 10.1126/science.aal1319
Qi, R., Li, J., Lin, Z., Li, Z., Li, Y., Yang, X., et al. (2016). Temperature effects on soil organic carbon, soil labile organic carbon fractions, and soil enzyme activities under long-term fertilization regimes. Appl. Soil Ecol. 102, 36–45. doi: 10.1016/j.apsoil.2016.02.004
Qian, H., Joseph, R., and Zeng, N. (2010). Enhanced terrestrial carbon uptake in the northern high latitudes in the 21st century from the coupled carbon cycle climate model intercomparison project model projections. Glob. Change Biol. 16, 641–656. doi: 10.1111/j.1365-2486.2009.01989.x
Quijano, C. J., and Kumar, P. (2015). Numerical simulations of hydraulic redistribution across climates: the role of the root hydraulic conductivities. Water Resour. Res. 51, 3729–3746. doi: 10.1002/2014WR016509
Quijano, J. C., Kumar, P., and Drewry, D. T. (2013). Passive regulation of soil biogeochemical cycling by root water transport. Water Resour. Res. 49, 227–288. doi: 10.1002/wrcr.20310
Quijano, J. C., Kumar, P., Drewry, D. T., Goldstein, A., and Misson, L. (2012). Competitive and mutualistic dependencies in multispecies vegetation dynamics enabled by hydraulic redistribution. Water Resour. Res. 48:W05518. doi: 10.1029/2011WR011416
Ren, G., Chan, J. C. L., Kubota, H., Zhang, Z., Li, J., Zhang, Y., et al. (2021). Historical and recent change in extreme climate over East Asia. Clim. Change 168:22. doi: 10.1007/s10584-021-03227-5
Richardson, M., and Kumar, P. (2020). Discerning the thermodynamic feasibility of the spontaneous coexistence of multiple functional vegetation groups. Sci. Rep. 10:18321. doi: 10.1038/s41598-020-75050-4
Rodeghiero, M., and Cescatti, A. (2005). Main determinants of forest soil respiration along an elevation/temperature gradient in the Italian Alps. Glob. Change Biol. 11, 1024–1041. doi: 10.1111/j.1365-2486.2005.00963.x
Rohatyn, S., Yakir, D., Rotenberg, E., and Carmel, Y. (2022). Limited climate change mitigation potential through forestation of the vast dryland regions. Science 377, 1436–1439. doi: 10.1126/science.abm9684
Roque-Malo, S., Yan, Q., Woo, D. K., Druhan, J. L., and Kumar, P. (2022). Advances in Biogeochemical Modeling for Intensively Managed Landscapes. Cham: Springer. doi: 10.1007/978-3-030-95921-0_6
Rupp, H., Tauchnitz, N., and Meissner, R. (2021). The effects of soil drying out and rewetting on nitrogen and carbon leaching-results of a long-term lysimeter experiment. Water 13:2601. doi: 10.3390/w13182601
Swain, D. L., Langenbrunner, B., Neelin, J. D., and Hall, A. (2018). Increasing precipitation volatility in twenty-first-century California. Nat. Clim. Change 8, 427–433. doi: 10.1038/s41558-018-0140-y
Tabari, H. (2020). Climate change impact on flood and extreme precipitation increases with water availability. Sci. Rep. 10:13768. doi: 10.1038/s41598-020-70816-2
Tabari, H., Hosseinzadehtalaei, P., AghaKouchak, A., and Willems, P. (2019). Latitudinal heterogeneity and hotspots of uncertainty in projected extreme precipitation. Environ. Res. Lett. 14:124032. doi: 10.1088/1748-9326/ab55fd
Wang, S., and Huang, Y. (2020). Determinants of soil organic carbon sequestration and its contribution to ecosystem carbon sinks of planted forests. Glob. Change Biol. 26, 3163–3173. doi: 10.1111/gcb.15036
William, R., Goodwell, A., Richardson, M., Le, P. V. V., Kumar, P., and Stillwell, A. S. (2016). An environmental cost-benefit analysis of alternative green roofing strategies. Ecol. Eng. 95, 1–9. doi: 10.1016/j.ecoleng.2016.06.091
Woo, D. K., and Do, W. (2021). The role of forests in climate change regarding carbon, nitrogen, and water: a case study of Pinus densiflora. Water 13:3050. doi: 10.3390/w13213050
Woo, D. K., and Kumar, P. (2016). Mean age distribution of inorganic soil-nitrogen. Water Resour. Res 52, 5516–5536. doi: 10.1002/2015WR017799
Woo, D. K., and Kumar, P. (2019). Impacts of subsurface tile drainage on age–concentration dynamics of inorganic nitrogen in soil. Water Resour. Res. 55, 1470–1489. doi: 10.1029/2018WR024139
Woo, D. K., Quijano, J. C., Kumar, P., Chaoka, S., and Bernacchi, C. J. (2014). Threshold dynamics in soil carbon storage for bioenergy crops. Environ. Sci. Technol. 48, 12090–12098. doi: 10.1021/es5023762
Xu, J., Morris, P. J., Liu, J., and Holden, J. (2018). Peatmap: refining estimates of global peatland distribution based on a meta-analysis. CATENA 160, 134–140. doi: 10.1016/j.catena.2017.09.010
Yan, D., Li, J., Pei, J., Cui, J., Nie, M., and Fang, C. (2017). The temperature sensitivity of soil organic carbon decomposition is greater in subsoil than in topsoil during laboratory incubation. Sci. Rep. 7:5181. doi: 10.1038/s41598-017-05293-1
Yoon, T. K., Noh, N. J., Chung, H., Yang, A.-R., and Son, Y. (2015). Soil nitrogen transformations and availability in upland pine and bottomland alder forests. Forests 6, 2941–2958. doi: 10.3390/f6092941
Yun, S. J., and Chun, J. (2018). Long-term ecological research on korean forest ecosystems: the current status and challenges. Ecol. Res. 33, 1289–1302. doi: 10.1007/s11284-018-1645-6
Zhao, C., Liu, B., Piao, S., Wang, X., Lobell, D. B., Huang, Y., et al. (2017). Temperature increase reduces global yields of major crops in four independent estimates. Proc. Natl. Acad. Sci. U.S.A. 114, 9326–9331. doi: 10.1073/pnas.1701762114
Zhao, F., Wu, Y., Hui, J., Sivakumar, B., Meng, X., and Liu, S. (2021). Projected soil organic carbon loss in response to climate warming and soil water content in a loess watershed. Carbon Bal. Manage. 16:24. doi: 10.1186/s13021-021-00187-2
Keywords: climate change, warming, precipitation whiplash, organic carbon, nitrogen leaching, Pinus densiflora
Citation: Woo DK and Seo Y (2022) Effects of elevated temperature and abnormal precipitation on soil carbon and nitrogen dynamics in a Pinus densiflora forest. Front. For. Glob. Change 5:1051210. doi: 10.3389/ffgc.2022.1051210
Received: 22 September 2022; Accepted: 22 November 2022;
Published: 05 December 2022.
Edited by:
Taehee Hwang, Indiana University Bloomington, United StatesReviewed by:
Jianning Ren, University of Nevada, Reno, United StatesCopyright © 2022 Woo and Seo. This is an open-access article distributed under the terms of the Creative Commons Attribution License (CC BY). The use, distribution or reproduction in other forums is permitted, provided the original author(s) and the copyright owner(s) are credited and that the original publication in this journal is cited, in accordance with accepted academic practice. No use, distribution or reproduction is permitted which does not comply with these terms.
*Correspondence: Yongwon Seo, eW9uZ3dvbi5zZW9AeXUuYWMua3I=
Disclaimer: All claims expressed in this article are solely those of the authors and do not necessarily represent those of their affiliated organizations, or those of the publisher, the editors and the reviewers. Any product that may be evaluated in this article or claim that may be made by its manufacturer is not guaranteed or endorsed by the publisher.
Research integrity at Frontiers
Learn more about the work of our research integrity team to safeguard the quality of each article we publish.