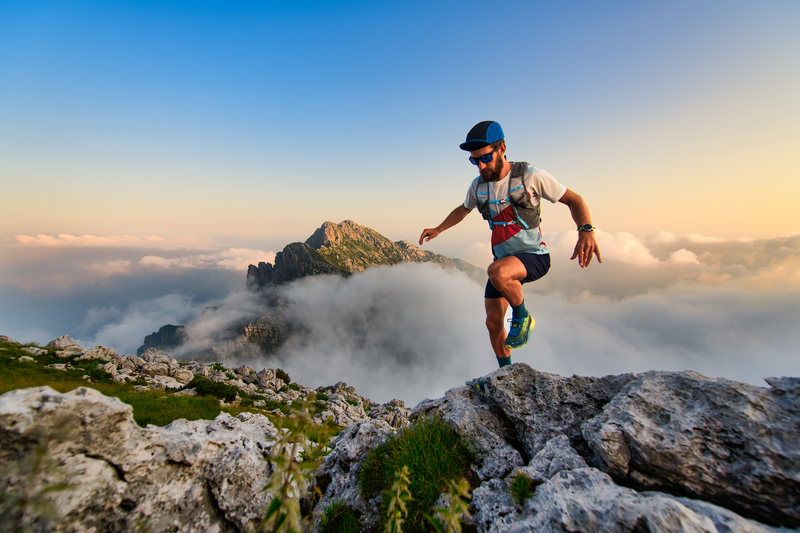
94% of researchers rate our articles as excellent or good
Learn more about the work of our research integrity team to safeguard the quality of each article we publish.
Find out more
ORIGINAL RESEARCH article
Front. For. Glob. Change , 25 February 2022
Sec. Pests, Pathogens and Invasions
Volume 4 - 2021 | https://doi.org/10.3389/ffgc.2021.756678
This article is part of the Research Topic Forest Pathology in Changing Climate View all 10 articles
A growing body of evidence suggests that climate change is altering the epidemiology of many forest diseases. Nothophaeocryptopus gaeumannii (Rhode) Petrak, an ascomycete native to the Pacific Northwest and the causal agent of the Swiss needle cast (SNC) disease of Douglas-fir [Pseudotsuga menziesii (Mirbel) Franco], is no exception. In the past few decades, changing climatic conditions have coincided with periodic epidemics of SNC in coastal forests and plantations from Southwestern British Columbia (B.C.) to Southwestern Oregon, wherein an increase in the colonization of needles by N. gaeumanii causes carbon starvation, premature needle shedding and a decline in growth. Two major sympatric genetic lineages of N. gaeumannii have been identified in the coastal Pacific Northwest. Past research on these lineages suggests they have different environmental tolerance ranges and may be responsible for some variability in disease severity. In this study, we examined the complex dynamics between biologically pertinent short- and long-term climatic and environmental factors, phylogenetic lineages of N. gaeumannii and the severity patterns of the SNC disease. Firstly, using an ensemble species distribution modeling approach using genetic lineage presences as model inputs, we predicted the probability of occurrence of each lineage throughout the native range of Douglas-fir in the present as well as in 2050 under the “business as usual” (RCP8.5) emissions scenario. Subsequently, we combined these model outputs with short-term climatic and topographic variables and colonization index measurements from monitoring networks across the SNC epidemic area to infer the impacts of climate change on the SNC epidemic. Our results suggest that the current environmental tolerance range of lineage 1 exceeds that of lineage 2, and we expect lineage 1 to expand inland in Washington and Oregon, while we expect lineage 2 will remain relatively constrained to its current range with some slight increases in suitability, particularly in coastal Washington and Oregon. We also found that disease colonization index is associated with the climatic suitability of lineage 1, and that the suitability of the different lineages could impact the vertical patterns of colonization within the crown. We conclude that unabated climate change could cause the SNC epidemic to intensify.
Anthropogenic climate change threatens the health and resilience of forest trees and their ecosystems through a variety of direct and indirect effects (Shaw and Osborne, 2011; Sturrock et al., 2011; Desprez-Loustau et al., 2016). Conifers are believed to be particularly vulnerable to a warming climate (McDowell et al., 2016). Among these effects is the rising risk from biotic disturbance agents such as forest diseases (Drew Harvell et al., 2002; Sturrock et al., 2011). Fungal disease agents, due to their relatively short lifespans, are able to evolve adaptive traits more rapidly in response to environmental changes than their perennial hosts. Consequently, unprecedented epidemics can occur when favorable environmental conditions persist and exacerbate the effects of climate change on forest health, potentially resulting in severe ecological and economic impacts (Desprez-Loustau et al., 2016; Hessenauer et al., 2021).
One noteworthy example is Nothophaeocryptopus gaeumannii (Rhode) Petrak, an endophytic fungal associate of Douglas-fir [Pseudotsuga menziesii (Mirbel) Franco], an ecologically, economically and culturally important conifer native to the Pacific Northwest Coast in the United States and Canada. Though N. gaeumannii was previously only considered pathogenic in Douglas-fir’s planted range, it has emerged as a threat in the tree’s native range in western North America in recent decades (Hansen et al., 2000). The fungus is the causal agent of Swiss needle cast (SNC), a disease that results in premature defoliation of Douglas-fir, jeopardizing its health and productivity (Gaumann, 1928; Boyce, 1940; Hood and Kershaw, 1975; Maguire et al., 2002; Lavender and Hermann, 2014). Like most forest foliar pathogens, the presence and abundance of N. gaeumannii is linked to short and long-term climate patterns (Manter et al., 2003, 2005; Stone et al., 2007; Mildrexler et al., 2019). As outbreaks of SNC have become unprecedentedly frequent and severe, there have been considerable efforts to understand the factors driving its distribution and severity patterns (Shaw et al., 2021).
The emergence of SNC is inextricably tied to the reproductive cycle of N. gaeumannii, which in turn depends on local environmental conditions and the life cycle of its host. The fungus produces sexual fruiting bodies called pseudothecia that erupt through the stomata of Douglas-fir needles. With the right environmental conditions, these structures can increase in abundance and subsequently inhibit gas exchange and cause needle chlorosis and premature senescence, ultimately reducing vertical and radial growth by over 50% in the most severely affected stands (Manter et al., 2000; Maguire et al., 2011). The specific conditions leading to proliferation of N. gaeumannii are understood to be in large part linked to temperature and moisture fluctuations at relevant times in the life cycle of the fungus (Stone et al., 2008a). Mild fall and winter temperatures, concurrent with epiphytic and endophytic hyphae growth in Douglas-fir needles, are conducive to fungal development (Manter et al., 2005; Bennett and Stone, 2019). Wet conditions in the spring and summer, coincident with Douglas-fir bud burst and N. gaeumannii spore dispersal, increase spore-needle adherence and rainsplash dispersal, favoring N. gaeumannii abundance. Additionally, topographical features such as elevation, slope aspect and shading that moderate microclimate have also been identified as important predictors of disease severity (Rosso and Hansen, 2003; Manter et al., 2005; Lee et al., 2017). While the extent and severity of epidemics has varied over the last decades, coastal forests of Oregon and Washington states largely planted with young Douglas-fir have been the epicenter of SNC outbreaks. Hence, silvicultural factors such as tree age and species diversity, along with the Pacific Northwest coastal characteristics such as fog and oceanic climate are considered conducive to increased severity (Ritóková et al., 2016, 2021; Shaw et al., 2021).
Recent explorations into the phylogeography of N. gaeumannii in the Pacific Northwest suggest that the current crisis is not solely attributable to climatic changes (Winton et al., 2006; Bennett and Stone, 2016, 2019). The population structure and genetics of N. gaeumannii have also been investigated as a source of variability in disease severity. Two major non-interbreeding phylogenetic lineages of N. gaeumannii (named lineages 1 and 2) have been identified in the Pacific Northwest, and though they are sympatric, their spatial distributions suggest that they may be adapted to different environmental conditions and climates (Bennett and Stone, 2016, 2019). Whereas lineage 1 has been detected across the native range of Douglas-fir as well as in exotic plantations from Europe to New Zealand, lineage 2 has been detected almost exclusively in coastal areas west of the Coast mountains of Washington and Oregon in addition to a small number of sites in New Zealand (Bennett and Stone, 2016; Bennett et al., 2019). Prior observations have indicated that regions where both lineages are detected are generally more severely infected (Winton et al., 2006; Bennett and Stone, 2016), but thus far, the role of the lineages in disease patterns remains unclear (Bennett and Stone, 2019).
In this paper, we report the use of genetic lineage distribution modeling (LDM) to explore the epidemiology of SNC at different scales. Presence-only species distribution models (SDM), which predict the probability of occurrence of a species based on georeferenced presence points, true absence or pseudo-absence points and environmental predictors, are becoming essential tools in conservation biology and disease epidemiology, including for assessing the ecological and epidemiological implications of climate change (Thuiller et al., 2005; Purse and Golding, 2015; Liu et al., 2020). In the context of a changing climate and increasingly accessible genomic tools, the integration of evolutionary information such as genetic clusters into SDMs can provide more accurate and informative predictions (Hoffmann and Sgrò, 2011; Gotelli and Stanton-Geddes, 2015; Desprez-Loustau et al., 2016; Nadeau and Urban, 2019). We believe that incorporating lineage-level distribution modeling with fine-scale severity data at monitoring sites may answer some important questions about the genetic and climatic characteristics underlying SNC epidemiology. The main questions we sought to address are: (i) What is the relationship between the climatic determinants of SNC and the distribution of lineages 1 and 2?; (ii) How will the potential distributions of the lineages change from the current climate to a future time period (2035–2065) under the “business as usual” climate scenario (RCP8.5)?; and (iii) can lineage climatic suitability be used to predict the average SNC severity at a site and to explain differences in within-tree severity patterns?
The study area chosen for the training and testing of our LDMs is the Marine West Coast Forests EPA Level I ecoregion, which covers the area of monitoring and provides a relatively ecologically homogeneous area for pseudo-absence selection (Figure 1A). The area of interest onto which we extrapolated our predictions is the entire Douglas-fir native range, which encompasses both the coastal (P. menziesii var. menziesii) and interior (P. menziesii var. glauca) varieties of Douglas-fir (Little, 1971) (Figure 1A).
Figure 1. (A) Douglas-fir native range in the Pacific Northwest (Little, 1971). The darker green shaded area is the Marine West Coast Forest EPA Level I Ecoregion, which is the study area used to train and test our lineage distribution modelings (LDMs). The lighter green shaded area is the rest of the Douglas-fir native range, the Area of Interest onto which we extrapolate our LDM predictions. (B) 62 presence and 9 absence points of lineage 1 of Nothophaeocryptopus gaeumannii across 71 sampled locations. (C) 42 presence and 29 absence points of lineage 2 of N. gaeumannii across 71 sampled locations.
Presence and absence points for the two phylogenetic lineages of N. gaeumannii in the PNW (Supplementary Data Sheet S1) were obtained from two sources: (i) lineage proportion data published by Bennett and Stone (2019) obtained using multilocus genotypes of a collection of isolates and (ii) lineage presence detection from samples of pseudothecia via real-time PCR using the methods described in Supplementary Protocol S1. The lineage identification from pseudothecia via real-time PCR used an established false positive signal to determine true presence with 99.5% accuracy if the targeted lineage constituted at least 5% of the pseudothecia in the sample using a statistical approach based on Geng et al. (1983). Therefore, to establish an equivalency in presence detection between these two sources of points, a lineage was considered absent in Bennett and Stone’s (2019) collection of isolates wherever the proportion of the lineage was below 5%. The compilation of points from these two sources produced a dataset of 62 presence points and 9 absence points of lineage 1 (Figure 1B), and 42 presence points and 29 absence points of lineage 2 (Figure 1C). In order to reduce the spatial clustering of the data, the points were rarefied to a 5 km resolution using the SDMtoolbox 2.0 python package (Brown et al., 2017).
Nine pseudo-absence datasets were generated using several pseudo-absence selection methods and numbers of pseudo-absences to collectively maximize performance for all the selected algorithms while reducing the bias from each individual pseudo-absence generation method. These were (i) surface range envelope with the quantile value set at 0.025, (ii) a minimum buffer of 20 km between an absence and the nearest presence, and (iii) randomly selected points masked by an exhaustive collection of N. gaeumannii presence points from past published research and aerial surveys in Oregon, Washington and British Columbia (Supplementary Data Sheet S2). For each of the pseudo-absence methods, three datasets were generated containing different numbers of points. These were (i) the same number of pseudo-absence points as presence points (ii) one hundred pseudo-absence points, and (iii) one thousand pseudo-absence points. In total, this led to nine pseudo-absence datasets. The prevalence was set at 0.5, weighting the total presences and total absences and pseudo-absences equally. These methods are based on our interpretation of the results and recommendations of Barbet-Massin et al. (2012) and Liu et al. (2019).
The environmental variables incorporated in this analysis were average degree days above 5°C (DD5), Summer Heat Moisture Index (SHM) and annual Relative Humidity (RH) between 1980 and 2010 (henceforth the ‘‘current’’ climate), as well as distance from the nearest coastline (km).1 DD5, SHM and RH were selected from a set of 28 bioclimatic variables produced by ClimateNA for different time periods (Wang et al., 2016). The variables were narrowed down based on their contribution to aforementioned temperature and wetness conditions conducive to N. gaeumannii proliferation, and then filtered based on their multicollinearity; only variables with a VIF below 0.5 were maintained. A short description of each variable and its rationale for inclusion in the LDM analysis is described in Table 1.
Rasters for future climate conditions were downloaded from ClimateNA for the period 2035-2065 (midpoint = 2050) under an ensemble of 13 Atmosphere-Ocean General Circulation Models (ACCESS-ESM1-5, BCC-CSM2-MR, CNRM-ESM2-1, CanESM5, EC-Earth3, GFDL-ESM4, GISS-E2-1-G, INM-CM5-0, IPSL-CM6A-LR, MIROC6, MPI-ESM1-2-HR, MRI-ESM2-0 and UKESM1-0-LL) of the Representative Concentration Pathway 8.5 climate change scenario (henceforth the “RCP8.5 2050 scenario”). This scenario, also known as the “business as usual” scenario, is intended to indicate the worst case scenario of climate change in the absence of mitigation policies, reflecting an increase in radiative forcing of 8.5 Watts/m2 by the end of twenty-first century relative to pre-industrial levels (Moss et al., 2010). To provide an overview of how the values of the climate variables are forecast to change under the RCP8.5 2050 scenario, we extracted values from the climate variable rasters at the 71 lineage sampling locations and graphed box plots and probability densities comparing their current and future values.
We used the SDM framework of the biomod2 R package (Thuiller et al., 2009). Four algorithms, Generalized Linear Models (GLM), Multiple Adaptive Regression Splines (MARS), Breiman and Cutler’s Random Forests (RF) and Maximum Entropy (MAXENT) were used to construct an ensemble model for each lineage. This set of algorithms was selected to maximize both predictive performance and interpretability while minimizing bias by combining traditional regression methods with more flexible and computationally intensive machine learning algorithms. Three cross validation runs with a training:testing data split of 80:20 were computed for each pseudo-absence dataset. A total of 108 models were developed (nine pseudo-absence sets x four algorithms x three cross-validation runs) to predict the potential distributions of lineage 1 and lineage 2 in the study area.
Models were evaluated by examining the True Skill Statistic (TSS) scores, which are calculated by taking the sum of the True Positive Rate (sensitivity) and True Negative Rate (specificity) minus 1, for each combination of the algorithm, pseudo-absence set, and cross-validation run. To examine the relationship between N. gaeumanii lineage occurrence and the explanatory variables used in this analysis, variable importance values were computed as explained in Thuiller et al. (2009). In our study, this was calculated using three permutations (i.e., random rearrangements of data) of each variable and via comparison of the correlations between the predictions from the re-arranged data versus the unshuffled data. Influential variables will have higher variable importance values (range: 0–1). Finally, to understand the relationships between the variables and the occurrence probability of the lineages, we graphed response plots for each variable developed based on the GLM models with a TSS score of 0.7 or above.
Ensemble modeling, where predictions from several different modeling techniques are combined, is applied to reduce bias and improve performance of SDMs (Araújo et al., 2005; Marmion et al., 2009). The predictions from the different modeling techniques were “ensembled” based on the average probabilities from high performing models (TSS > 0.7 for lineage 1 and TSS > 0.75 for lineage 2). Predictions from the ensembles were then projected to the entire range of Douglas-fir in the Pacific Northwest for current (1980–2010) and future conditions (2035–2065) under the RCP8.5 2050 scenario. Interpreting the probabilities of occurrence from this analysis as a measure of climatic suitability, we sought to explore associations between these outputs and disease severity.
Fine-scale severity data from across the epidemic zone sampled between 2015 and 2019 were acquired from researchers of monitoring networks in Washington, Oregon and B.C. The response variable used to signify N. gaeumannii abundance and therefore disease severity is the Colonization Index (CI), a heuristic approximation of the probability percentage that a given stoma is occluded by N. gaeumannii pseudothecia. This was assessed on 2-year old needles from the top, middle and bottom crown sections of trees. The number of trees and crown sections sampled per site varied in the different networks; only the upper crown was sampled in Washington, and mostly 5 trees were assessed in B.C. and 10 trees in Washington and Oregon. The CI (%) was obtained by multiplying the incidence (proportion of needles exhibiting occluded stomata) by the severity (proportion of pseudothecia occluded on the base, middle and tip of needles) on a subset of needles. The number of needles in these subsets also varied between the networks, with ten to 50 needles used for incidence assessment, and five to ten needles used for severity assessment.
To investigate the influence of lineage suitability on site-level average CI while controlling for sampling heterogeneity, local topography and short-term climate patterns, we utilized a binomial logistic regression with a logit link, incorporating only sites where data from all three crown sections were available (131 sites in total from Oregon and B.C.) (Supplementary Data Sheet S3). To account for sampling heterogeneity across the monitoring sites, we implemented a weighting system of observations with weights assigned as a function of the amount of sampling done at the site. To account for the effect of fine-scale topography and the degree of shading on a site, we chose to include hillshade pixel depth into the analysis, which is an indication of the level of shading at the site; high pixel depth signifies lighter color and hence a more illuminated site, while low pixel depth means a darker color and therefore a more shaded site. This variable was obtained by applying the hillshade function in QGIS 3.10.9 to a digital elevation model from ClimateNA with the azimuth set at 315° (NW). Additionally, the natural log of the average precipitation as snow in January (PAS01) from the two years preceding needle sampling was used to control for short-term winter conditions.
Model selection and inference in this study drew on an information theoretic approach proposed by Burnham and Anderson (2002) and implemented in the AICcmodavg package in R (Mazerolle, 2017). We built a global model along with a set of plausible nested candidate models (Table 2). Variables were scaled and centered on the mean prior to modeling. We assessed the evidence for each model based on the second-order Akaike Information Criterion for small sample sizes (AICc); the evidence ratios obtained are an indication of the number of times a given model is more parsimonious than a lower-ranked model (Burnham and Anderson, 2002; Mazerolle, 2017). Since more than one model carried a consequential proportion of the AICc weight, we employed multi-model averaging to compute model-averaged parameters and measures of uncertainty for the explanatory variables, with shrinkage applied based on the AICc weight of the different candidate models as suggested by Calin-Jageman and Cumming (2019). In accordance with Mazerolle (2017), model fit was evaluated based on the global model, which was designed as follows:
where is the odds ratio that a given stomata on a given needle is occluded versus unoccluded by pseudothecia at the ith site. The weight of each observation is an estimation of the number of needles measured for pseudothecia occlusion at the site, calculated by taking the total of the product of the number of needles measured for prevalence and severity at the site. For instance, at a site where all three crown sections of ten trees are sampled, and at every crown section ten needles are used for severity estimation and 50 needles for prevalence estimation, the weight is equal to 3 × 10 × (10 × 50) = 15000. The weights range from 2,450 to 18,150. The intercept, β0 is the value of the CI when the other variables are held at 0 (i.e., at their mean values). β1 is the coefficient for the effect of PAS01 on CI, X1i is the millimeters of PAS01 at the ith site. β2 is the coefficient for the effect of hillshade on CI, X2i is the hillshade pixel depth at the ith site. β3 is the coefficient for the effect of lineage 1 probability of occurrence on CI, X3i is the probability of occurrence of lineage 1 at the ith site. β4 is the coefficient for the effect of lineage 2 probability of occurrence on CI, X4i is the probability of occurrence of lineage 2 at the ith site. The probability of occurrence values for lineage 1 (X3i) and lineage 2 (X4i) for each site were extracted from the ensemble model-based lineage potential distribution maps for the current (1980-2010) time period.
Table 2. (A) Akaike’s Information Criterion adjusted for small sample sizes (AICc) weight and AICc score of the highest ranked (AICc weight above 0) generalized linear models associating colonization index (CI) values with lineage suitability, climate and topography and (B) estimates of the mean and standard deviations of the response (CI probability) and explanatory variables [ln(PAS01), hillshade, L1 suitability, L2 suitability].
Finally, we explored correlations between the two lineage suitability outcomes and average CI in the different crown sections using correlation plots and Pearson’s correlation tests based on data from all the SNC monitoring networks (a total of 211 sites in Oregon, Washington and B.C.).
DD5 and SHM are the two most important predictors among the explanatory variables for both lineage 1 and lineage 2, though the importance of DD5 appears to be higher for lineage 1, while SHM is more important for lineage 2 (Figure 2A). Distance to coast and RH are also much more important predictors for presence of lineage 2 than for lineage 1 (Figure 2A). According to the averaged predictions of the best performing GLMs, the optimal conditions for presence differ significantly between the lineages. Lineage 1 has a much broader range of suitability for distances further from the coast and for hotter and drier summers than lineage 2 (Figure 2B). The tolerance range of lineage 1 for both low and high annual average RH values exceeds that of lineage 2 (Figure 2B). In contrast, lineage 2 has a slightly broader tolerance in terms of higher DD5 (a greater accumulation of daily degrees experienced above 5°C) (Figure 2B).
Figure 2. (A) Importance of explanatory variables for lineage (blue bars) 1 and lineage 2 (red bars) of Nothophaeocryptopus gaeumannii for the ensemble models. Error bars indicate the maximum and minimum importance values calculated by permuting each variable three times and comparing the correlation between predictions when the variable is randomized versus not randomized. (B) Response plots of explanatory variables for the generalized linear models included in the ensembles of lineage 1 (blue lines and light blue shading) and lineage 2 (red lines and light red shading). Lines indicate the mean probability of occurrence prediction of the models. Shading indicates the standard error of the mean probability of occurrence estimates of the models.
Evaluation scores for lineage 1 were generally lower than for lineage 2, which may be a reflection of its broader environmental tolerance throughout the study area (Figure 3). According to their TSS scores, the regression methods discriminated between presences and absences better for lineage 2 than for lineage 1 (L1 GLM = 0.72, L1 MARS = 0.7, L2 GLM = 0.6, L2 MARS = 0.64), while random forests performed similarly for both (L1 RF = 0.66, L2 RF = 0.65). Maxent had the weakest discrimination capacity for both lineages (L1 MAXENT.Phillips = 0.5, L2 MAXENT.Phillips = 0.61) (Figure 3). However, the TSS scores, which range from -1 (no points classified correctly) to 1 (all points classified correctly) were 0.74 and 0.78 for lineages 1 and 2 ensemble models, respectively (Figure 3). Such TSS values indicate “good” to “very good” model predictions (Préau et al., 2019).
Figure 3. Mean True Skill Statistic (TSS) scores of the individual and ensemble models for lineage 1 (blue bars) and lineage 2 (red bars) of Nothophaeocryptopus gaeumannii. Error bars represent the standard error around the mean TSS score. TSS scores range from -1 (none of the presences or absences classified correctly) to 1 (all presences and absences classified correctly).
At the lineage presence points included in the LDMs, the climate variables are forecast to change between “current” climate conditions and those in the 2050 RCP8.5 scenario. The most remarkable change is DD5, which will increase across its entire range of values, with its mean increasing from 1996 to 2700 degree days (Figure 4A). The mean SHM will increase from 53 to 73, indicating hotter and drier summers overall (Figure 4B). The range of RH will remain relatively unchanged, with the mean decreasing from 71 to 69% (Figure 4C).
Figure 4. Boxplots of median, interquartile range and outliers, with probability distributions of (A) degree days above 5°C, (B) summer heat moisture index and (C) relative humidity (%) at the 71 sampling sites used in the LDMs. The left side violin plot represents the current (1980–2010) distribution of values, while the right side violin plot represents distribution of values in 2035–2065 under the RCP8.5 emissions scenario (RCP8.5 2050).
These changes are reflected in the patterns of probability of occurrence forecast for the two lineages, which can be interpreted as a measure of climatic suitability. Under the current climate, the coastal zone west of the Cascade and Coast ranges extending from the Lower Mainland of B.C. to central Oregon is highly suitable for both lineages. However, the areas of very high suitability for lineage 1 extend further inland into the B.C. Fraser valley and northeast out to the eastern coast of Vancouver Island, as well as inland to the western slopes of the Oregon Cascades (Figure 5A). Lineage 1 also has relatively higher suitability values throughout the interior Douglas-fir range than lineage 2, particularly in the interior (Figures 5A vs. C). In contrast, the areas of high suitability for lineage 2 under current conditions are constrained to a narrow strip of land along the coast of the study area along with a pocket on the eastern coast of Vancouver Island. However, its range of high suitability values extends further South than that of lineage 1, to the Southern limit of our study area (Figure 5C).
Figure 5. Maps of climatic suitability of lineages 1 and 2 of Nothophaeocryptopus gaeumannii in the Pacific Northwest Douglas-fir range (A) Lineage 1 climatic suitability map under current (1980–2010) climate; (B) Change in lineage 1 climatic suitability under the RCP8.5 2050 climate; (C) Lineage 2 climatic suitability map under current (1980–2010) climate; (D) Change in lineage 2 climatic suitability under the RCP8.5 2050 climate.
Under the climatic conditions forecast by the RCP8.5 2050 scenario, changes in the climatic suitability of lineage 1 are predicted to increase by a probability of up to 0.1 in large swathes of the Douglas-fir range (Figure 5B). The highest increase in suitability is predicted east of the coast in Washington and Oregon; for example, the Willamette Valley and Western slopes of the Oregon and Washington Cascades should become significantly more suitable areas for lineage 1 (Figure 5B). There are some areas where the suitability of lineage 1 is forecast to decrease; Vancouver Island, the Olympic Peninsula and Puget Sound (Figure 5B).
Under the RCP8.5 scenario, lineage 2 is likely to remain similarly geographically limited, with areas in the interior of the Douglas-fir range becoming generally even less suitable to Lineage 2 (Figure 5D). Some increases in suitability are forecast in Southeastern Vancouver Island and the Puget Sound (Figure 5D).
Out of our set of plausible candidate models for predicting CI, two models carried the highest proportion of the AICc weight. These were (i) the one including ln(PAS01), hillshade and lineage 1 suitability and the global model, carrying 74% and 25%, respectively (Table 2). Using model-averaging, we are able to obtain parameter estimates and their uncertainties as measured by standard error for each of our explanatory variables. These are 0.03 (±0.01) for lineage 1, 0.0 for lineage 2, –0.03 (±0.01) for ln(PAS01), and 0.01 (±0.01) for hillshade (Table 2). This means that when ln(PAS01) and hillshade are held constant, the odds of a stomata being occluded by a pseudothecium are multiplied by 1.03 (e0.03) with a 1 unit increase in lineage 1 suitability. In effect, this indicates a positive association between lineage 1 climatic suitability and CI, even when short-term winter conditions and local topography are taken into account. This association is supported by the evidence ratios between the models; the candidate model including lineage 1 suitability along with the log of PAS01 and hillshade was 93.966 times more parsimonious than the one including only the log of PAS01 and hillshade. We found no such association with lineage 2 climatic suitability. In terms of the effects of short-term winter climate patterns and topography, ln(PAS01) of the previous two years has a negative association with CI, while hillshade pixel value has a positive association with CI, implying that higher CI should be found where there is lower precipitation in January and on less shaded sites.
The logistic regression model fit of our global model was excellent, with a Hosmer Lemeshow test p-value of 0.99 and no concerning patterns in the residuals once a log-transformation was applied to PAS01 (Supplementary Figure S1).
Significant positive correlations were observed between lineage 1 climatic suitability and CI in the middle (r = 0.17, P = 0.04) and lower (r = 0.29, P = 0.0004) crown sections (Figure 6A). A weak positive correlation was observed between lineage 2 climatic suitability and the upper crown section CI (r = 0.14, P = 0.05). Lineage 2 climatic suitability and the CI values in the lower crown section were negatively correlated (r = –0.39, P < 0.0001) (Figure 6B).
Figure 6. Correlation plots between Nothophaeocryptopus gaeumannii lineage suitability and colonization index (CI) in different crown sections (A) Relationship between lineage 1 suitability and CI in the upper, middle and lower sections of the tree; (B) Relationship between lineage 2 suitability and CI in the upper, middle and lower sections of the tree.
Our results indicate that the two genetic lineages described for N. gaeumannii have overlapping environmental distributions but different environmental tolerance ranges. Indeed, while there are areas where one lineage completely supplants the other, they are also often present in the same site, sometimes in very close proximity; in fact, both lineages have been identified on a single needle (Bennett and Stone, 2019). Our research adds support to a number of previous findings relating to N. gaeumannii and its lineages, while providing further insight into the niche optima of the lineages in terms of a few key variables.
Overall, both lineages 1 and 2 (and therefore the whole N. gaeumannii species in the Pacific Northwest) prefer coastal climate and warm temperatures, and favor mild and wet conditions, as opposed to hot and dry conditions, in the spring and summer, suggesting that geographic areas under these conditions should be more susceptible to SNC epidemics. This agrees with the conclusions of a wide range of other studies into the climatic determinants of SNC distribution and severity (Manter et al., 2005; Lee et al., 2017; Shaw et al., 2021).
The difference in niche in terms of DD5 corresponds with the dominance of lineage 2 and absence of lineage 1 at survey sites in southernmost Oregon sites. Similarly, the much broader tolerance for higher SHM and continentality of lineage 1 manifests in its complete supplantation of lineage 2 in hotter and drier conditions farther from the coast, a phenomenon also remarked by Bennett and Stone (2019). These differences in tolerance may be explained by the population structure of the lineages. N. gaeumannii is a homothallic species which appears to reproduce predominantly via selfing with occasional outcrossing events (Bennett and Stone, 2019). According to multilocus genotypes analyzed by Bennett and Stone (2016, 2019) lineage 1 has a higher genotypic diversity, genotypic richness and genetic diversity than lineage 2, which is considered relatively clonal. The diversity of genotypes in lineage 1 and the different phenotypes they can produce could be an explanation for its broad environmental tolerance. Conversely, the lack of genotypic diversity in lineage 2 may explain its limited environmental range. The phenotypes produced by these different genetic clusters may also hold insights into relationships between severity and lineage climatic suitability evoked by our CI modeling.
The results of our severity modeling demonstrates the utility of LDMs for predicting forest disease severity, while also highlighting the importance of using both short-term winter climate along with topography data in improving prediction of colonization levels of N. gaeumannii when using down-scaled climate data.
While precipitation as snow was a strong predictor of CI, the inclusion of hillshade in models helped to explain the values of outliers such as several highly infected sites in the Chilliwack area of B.C. and the Tillamook area of Oregon, as well as relatively uninfected sites in the Florence and Coos Bay areas of Oregon that were not explained by PAS01 or lineage suitability. This is in agreement with previous research that noted the importance of shading, aspect and elevation in SNC epidemics (Rosso and Hansen, 2003; Lee et al., 2017). Our results also suggest that the distribution and suitability of the lineages may assist in predicting disease severity along with short-term climate.
Differences in pathogenicity between genetic lineages is not uncommon in forest pathogens. Some of the most notable examples include the lineages of Phytophthora ramorum Werres, De Cock, and Man in’t Veld (Elliott et al., 2011) and Ophiostoma ulmi (Buisman) Melin and Nannf. (Hessenauer et al., 2020). In the case of SNC for which infection severity is dependent on abundance of pseudothecia, differences in pathogenicity between lineages should arise as the result of competitive advantages such as improved dispersal ability, higher growth rate, or an adaptation to the local environment. Initially, the clonal population structure of lineage 2 and the concentration of the most severe outbreaks in areas of Washington and Oregon where this lineage was abundant led to the proposition that this lineage had a competitive advantage over lineage 1 and is part of the etiology of the PNW’s SNC epidemic (Winton et al., 2006; Bennett et al., 2019). Yet, lineage 2 is conspicuously absent from the B.C. Lower Mainland’s most severely infected stands, while several sites in Southern Oregon almost exclusively populated with lineage 2 have little to no symptoms of SNC according to aerial surveys (Bennett and Stone, 2019). Indeed, though they do not allow us to infer causality, our results indicate a slight association between climatic suitability of lineage 1 and the site average levels of colonization by N. gaeumannii, even after accounting for short-term climatic and topographic effects. In contrast, our model averaging revealed no such association with lineage 2 climatic suitability. According to ecological niche theory, the climatic suitability values generated by our LDMs should approximate the local abundance of each lineage (Maguire, 1973; Guisan et al., 2017). As CI is a reflection of pathogen abundance, its association with lineage 1 suitability and lack thereof with lineage 2 suitability may be interpreted as evidence for a competitive advantage in favor of lineage 1. Our findings are supported by preliminary results from phenotyping experiments, which showed that isolates of lineage 1 most common on the coast have a higher phenotypic plasticity and grow faster than those of lineage 2 in three different temperature conditions and three drought conditions (Yin et al., 2020). Our findings also concur with those from Bennett and Stone (2019), who tested the hypothesis of higher pathogenicity of lineage 2, but found no association between the relative proportion of lineage 2 and SNC symptoms when distance from the coast was held constant.
The significant association between CI and lineage suitability, as well as the high evidence ratios of models that incorporated lineage 1 suitability over those that didn’t, provides evidence that LDMs can be useful in estimating the severity patterns of the epidemic. This not only helps us to make inferences to greater spatial scales, but also to much smaller scales such as within the tree. Differences in the relationships between CI and lineage suitability in the upper, middle and lower crown sections reveal some intriguing possibilities such as within-tree niche partitioning between the lineages, and may help to explain the differing patterns of vertical colonization in the monitoring networks. The occupation of the same host by different species or reproductively isolated lineages as is the case with N. gaeumannii poses a problem in ecology as it violates the competitive exclusion principle (Hardin, 1960). However, a possible answer to this conundrum has been found in fine-scale spatial or temporal niche partitioning between cryptic species or non-interbreeding subpopulations. Evidence of this in forest pathogens includes the complex of fungal species causing oak powdery mildew in Europe and fungal symbionts of the mountain pine beetle in western North-America (Feau et al., 2012; Hamelin et al., 2016; Ojeda Alayon et al., 2017). The correlation between lineage 1 suitability and lower and middle crown section CI and the weak correlation between lineage 2 suitability and the upper crown section CI suggests that the lineages may prefer different crown sections - lineage 1 the lower and middle sections, and lineage 2 the upper section. This would be consistent with the different colonization levels found in B.C., where lineage 1 is most prominent and the lower sections are most severely infected, as opposed to Oregon where the upper section of the crown is most infected in highly diseased sites and lineage 2 is more common (Shaw et al., 2014; Bennett and Stone, 2019).
It is important to note that although CI is the primary mechanistic sign of SNC, it is not always correlated with the ultimately damaging symptom of premature defoliation (Manter et al., 2003; Montwé et al., 2021). The host dynamics of this disease remain obscure; indeed, studies on silvicultural practices (Filip et al., 2000; El-Hajj et al., 2004; Lan et al., 2019a), as well as host age (Lan et al., 2019b), tree provenance and family (Johnson, 2002; Montwé et al., 2021) have reported different and even contradictory results on relationships of these factors with symptom severity and disease tolerance and resistance. Crucially, the interacting effect of climate change on both the host and pathogen is an important knowledge gap which should be addressed in further research. In addition, the importance of different climate variables on disease severity seems to vary spatially, according to differences in site biogeoclimatic characteristics (Stone et al., 2008a; Lee et al., 2013). Hitherto, distance from the coast has been widely used in SNC modeling as a proxy for this variability in site characteristics as it applies well to the relatively linear coastline of Oregon where the disease has been most studied, but the parameter is not as useful in Washington and Southwestern B.C, which have more complex coastlines. Modeling work such as ours on the full extent of the epidemic requires replacement of this proxy variable with more widely applicable variables such as ecosystem classification zones. The lack of compatibility between ecosystem classification systems across the national boundaries of the Pacific Northwest is a barrier to this effort. While many important challenges for understanding and managing the SNC epidemic remain, the combined use of genetic information along with climate data at different timescales and topographic data provides some useful insight that could aid forest management decision-making in the context of climate change.
The results of our study suggest that it is not lineage 2 but rather lineage 1 that may be the driver of disease severity currently in the coastal Pacific Northwest. Though it can be problematic to extrapolate results beyond the spatial and temporal scope of an observational dataset, we do so under the fundamental assumption that species conserve their niches (Peterson et al., 1999; Liu et al., 2020). This allows us to make some informed predictions about the trajectory of the SNC epidemic to the rest of the Douglas-fir range under the RCP8.5 2050 scenario. The increase in lineage 1 suitability observed in our predictions in this scenario indicate that the frequency of SNC epidemics may increase in coming years, particularly extending into the Willamette valley and Western slopes of the Cascades, which until now have been spared of the disease’s impact (Ritóková et al., 2016; Shaw et al., 2021). The expansion to new suitable locations reflects the current tolerance of lineage 1 for a wide range of climate conditions. Considering the relatively high genetic diversity of this lineage, it is consistent with the general idea that a more diverse subpopulation of a fungus will have more chance to adapt successfully to a changing environment than a clonal subpopulation (Drenth et al., 2019). When taken together with our severity modeling results, the outputs of our lineage 1 LDMs can be interpreted as indicators of future disease severity, assuming that warmer climates will also lead to an increase in the frequency of optimal short-term winter conditions such as low PAS01. As such, the increase in the overall suitability values across much of the Douglas-fir range in the RCP8.5 2050 scenario suggests that the severity of epidemics can be expected to intensify throughout much of the lineage 1 distribution if greenhouse gas emissions continue unabated in the coming decades.
In contrast, the extent and suitability levels of lineage 2 are predicted to remain relatively similar according to our LDMs, with increases in probability over 0.1 in Northern Washington and Northeastern Vancouver Island. We believe this may be due to increases in suitability resulting from favorable DD5 being offset by rising SHM to which lineage 2 has a very limited tolerance (Figure 4). However, it is important to mention a number of caveats to this result. Firstly, clonal populations such as that of lineage 2 tend to expand spatially as they do not need to compete against other genotypes and are usually indicative of a highly successful individual, but they are more vulnerable to collapse due to their lack of adaptability; this population structure is epidemiologically important but difficult to factor into modeling. It is also noteworthy that various studies in the epidemic zone show a historical trend toward increasing spring and summer precipitation in the epidemic zone (Stone et al., 2008b; Mildrexler et al., 2019; Montwé et al., 2021), as opposed to the drying trend forecast in our RCP8.5 scenario. This and fluctuations in short-term weather patterns introduce additional uncertainties in our future predictions under the RCP8.5 emission scenario, which call for caution in interpreting our maps. Future risk assessments will immensely benefit from rangewide determinations of SNC genetic lineages and accurate estimations of climate and host dynamics in this region.
Climate-driven SDMs have traditionally been used to assess habitat suitability for a given species including forest pathogens (Pandit et al., 2020; Pedlar et al., 2020). We are unaware of studies in forest pathology that have used genetic clusters as inputs into SDM, and outputs of these models for assessing the severity of forest pests or diseases. Our study addresses this knowledge gap by including results from predictive maps of the climatic suitability of the two N. gaeumannii lineages (lineage 1 and 2) into severity modeling. By considering the whole epidemic area this study provides the most geographically extensive investigation of the short and long-term climate dynamics of SNC. We believe that future research should focus on the phenotyping of the N. gaeumannii lineages, including the coastal and interior variants of lineage 1, as well as other evolutionary differences between the lineages, such as dispersal ability. This could be used to fine-tune our SDMs using mechanistic models which are known to perform better with native pathogens in their realized niches. Our results indicate that there is a sound theoretical and empirical underpinning for using LDMs as tools to not only predict the current and future climatic suitability of the SNC lineages, but also to attempt to identify high disease severity risk areas under current and future climate conditions.
The original contributions presented in the study are included in the article/Supplementary Material, further inquiries can be directed to the corresponding author.
NH-S conceptualized the research with help from KRS and RCH, did labwork for lineage qPCR identification, completed the data analysis, and wrote the manuscript. KRS contributed to conceptualization, co-supervision, and writing (review and editing). XY contributed by developing the methods used for lineage identification by qPCR in this research and by helping NH-S with labwork and data collection. NF contributed by supervising the development of the methods used for lineage identification by qPCR and writing (review and editing). SZ contributed to sample and data acquisition. DO and GR contributed to sample acquisition. CC contributed to data acquisition. RCH contributed to conceptualization. All authors contributed to the article and approved the submitted version.
This research was financially supported by the CoAdapTree Project (241REF), with funding from Genome Canada, Genome British Columbia, Genome Alberta, and Genome Québec (a full list of sponsors is available at https://coadaptree.forestry.ubc.ca/sponsors/). The Pest Risk Management Programme of Natural Resources Canada also provided funding for this project.
The authors declare that the research was conducted in the absence of any commercial or financial relationships that could be construed as a potential conflict of interest.
All claims expressed in this article are solely those of the authors and do not necessarily represent those of their affiliated organizations, or those of the publisher, the editors and the reviewers. Any product that may be evaluated in this article, or claim that may be made by its manufacturer, is not guaranteed or endorsed by the publisher.
We would like to thank the many researchers and assistants who helped provide us with samples and severity data from the different monitoring networks. From the B.C. monitoring network, Lucy Stad, Ann Wong, Jack Sweeten, B A Blackwell and Associates and Kerley and Associates Forestry Consulting. From the Oregon and Washington network, the members of the Swiss Needle Cast Cooperative, particularly Doug Mainwaring and Andrew Bluhm, plus John Browning and Anna Leon. From the Washington monitoring network, Rachel Brooks. We also owe a debt of gratitude to the student lab assistants involved in the quantification of disease severity, including Berni Van der Meer, Alanna Love and Yifan Yuan. Finally, we would like to thank Isabelle Giguère for her essential logistical work in receiving samples from the different networks and providing lab training and overall support throughout the completion of this project.
The Supplementary Material for this article can be found online at: https://www.frontiersin.org/articles/10.3389/ffgc.2021.756678/full#supplementary-material
Supplementary Figure S1 | Assumptions of logistic regression tested on our global model. (A) Parametric bootstrap of the deviance simulated on 5000 binomial models. (B) Kolmogorov-Smirnov tests for model adjustment and comparisons of standardized residuals with ranked model predictions to test for outliers and model fit, based on 250 simulations of binomial models. (C) Various plots to check for residual heterogeneity (residuals vs fitted), normality (QQ-plot), and outliers (Scale-location, residuals vs leverage), as well as to check for patterns in residuals for the different predictors.
Supplementary Data Sheet S1 | Data sheet of SNC presence points collected by Chantal Coté and Naomie Herpin-Saunier. Chantal Coté compiled a collection of points from published articles and online repositories of species occurrences (e.g. GBIF), while Naomie generated additional points by converting shapefiles of areas showing SNC symptoms from aerial surveys by the Swiss Needle Cast Cooperative, Washington Department of Natural Resources and British Columbia Ministry of Forests, Lands, Natural Resource Operations and Rural Development into points. All of these points were combined and rarefied to a 5 km distance between points. These were used as a mask for pseudo-absence data selection in the building of the SDM datasets.
Supplementary Data Sheet S2 | Data sheet of lineages 1 and 2 presence and absence points.
Supplementary Data Sheet S3 | Data sheet of colonization index measurements at Swiss needle cast monitoring sites used for severity modelling.
Supplementary Protocol S1 | Real-time PCR protocol used for lineage presence detection at monitoring sites using multiple pseudothecia per site.
Araújo, M. B., Whittaker, R. J., Ladle, R. J., and Erhard, M. (2005). Reducing uncertainty in projections of extinction risk from climate change. Glob. Ecol. Biogeogr. 14, 529–538.
Barbet-Massin, M., Jiguet, F., Albert, C. H., and Thuiller, W. (2012). Selecting pseudo-absences for species distribution models: how, where and how many? Methods Ecol. Evol. 3, 327–338.
Bennett, P. I., Hood, I. A., and Stone, J. K. (2019). The genetic structure of populations of the Douglas-Fir Swiss needle cast fungus Nothophaeocryptopus gaeumannii in New Zealand. Phytopathology 109, 446–455. doi: 10.1094/PHYTO-06-18-0195-R
Bennett, P. I., and Stone, J. K. (2016). Assessments of population structure, diversity, and phylogeography of the Swiss needle cast fungus (Phaeocryptopus gaeumannii) in the U.S. Pacif. Northwest. For. Trees Livelihoods 7:14.
Bennett, P. I., and Stone, J. K. (2019). Environmental variables associated with Nothophaeocryptopus gaeumannii population structure and Swiss needle cast severity in Western Oregon and Washington. Ecol. Evol. 9, 11379–11394. doi: 10.1002/ece3.5639
Beyer, M., Verreet, J.-A., and Ragab, W. S. M. (2005). Effect of relative humidity on germination of ascospores and macroconidia of Gibberella zeae and deoxynivalenol production. Int. J. Food Microbiol. 98, 233–240. doi: 10.1016/j.ijfoodmicro.2004.07.005
Boyce, J. S. (1940). A needle cast of douglas-fir associated with Adelopus gaeumannii. Phytopathol 30, 649–659.
Brown, J. L., Bennett, J. R., and French, C. M. (2017). SDMtoolbox 2.0: the next generation Python-based GIS toolkit for landscape genetic, biogeographic and species distribution model analyses. PeerJ 5:e4095. doi: 10.7717/peerj.4095
Burnham, K. P., and Anderson, D. R. (Eds.) (2002). Model Selection and Multimodel Inference: A Practical Information–Theoretic Approach. New York, NY: Springer.
Calin-Jageman, R. J., and Cumming, G. (2019). The New statistics for better science: ask how much, how uncertain, and what else is known. Am. Stat. 73(Supp 1), 271–280. doi: 10.1080/00031305.2018.1518266
Capitano, B. R. (1999). The Infection and Colonization of Douglas-fir Needles by the Swiss Needle Cast Pathogen, Phaeocryptopus gaeumannii (Rhode) Petrak (MS Thesis). Corvallis, OR: Oregon State University.
Desprez-Loustau, M.-L., Aguayo, J., Dutech, C., Hayden, K. J., Husson, C., Jakushkin, B., et al. (2016). An evolutionary ecology perspective to address forest pathology challenges of today and tomorrow. Ann. For. Sci. 73, 45–67. doi: 10.1007/s13595-015-0487-4
Drenth, A., McTaggart, A. R., and Wingfield, B. D. (2019). Fungal clones win the battle, but recombination wins the war. IMA Fungus 10:18.
Drew Harvell, C., Mitchell, C. E., Ward, J. R., Altizer, S., Dobson, A. P., Ostfeld, R. S., et al. (2002). Climate warming and disease risks for terrestrial and marine Biota. Science 296, 2158–2162. doi: 10.1126/science.1063699
El-Hajj, Z., Kavanagh, K., Rose, C., and Kanaan-Atallah, Z. (2004). Nitrogen and carbon dynamics of a foliar biotrophic fungal parasite in fertilized Douglas-fir. New Phytol. 163, 139–147. doi: 10.1111/j.1469-8137.2004.01102.x
Elliott, M., Sumampong, G., Varga, A., Shamoun, S. F., James, D., Masri, S., et al. (2011). Phenotypic differences among three clonal lineages of Phytophthora ramorum. For. Pathol. 41, 7–14. doi: 10.1111/j.1439-0329.2009.00627.x
Feau, N., Lauron-Moreau, A., Piou, D., Marçais, B., Dutech, C., and Desprez-Loustau, M.-L. (2012). Niche partitioning of the genetic lineages of the oak powdery mildew complex. Fungal Ecol. 5, 154–162. doi: 10.1016/j.funeco.2011.12.003
Filip, G. M., Kanaskie, A., Kavanagh, K. L., Johnson, G., Johnson, R., and Maguire, D. A. (2000). Silviculture and Swiss Needle Cast: Research and Recommendations. Corvallis, OR: Forest Research Laboratory, Oregon State University.
Gaumann. (1928). Eine neue krankheit der douglastanne. Zeitschrift Fur Pflanzenkrankheiten Pflanzenschutz 38, 70–78.
Geng, S., Campbell, R. N., Carter, M., and Hills, F. J. (1983). Quality control programs for seedborne pathogens. Plant Dis. 67, 236–242. doi: 10.1094/pd-67-236
Gotelli, N. J., and Stanton-Geddes, J. (2015). Climate change, genetic markers and species distribution modelling. J. Biogeogr. 42, 1577–1585. doi: 10.1111/jbi.12562
Guisan, A., Thuiller, W., and Zimmermann, N. E. (2017). Habitat Suitability and Distribution Models: With Applications in R. Cambridge: Cambridge University Press.
Hamelin, F. M., Bisson, A., Desprez-Loustau, M.-L., Fabre, F., and Mailleret, L. (2016). Temporal niche differentiation of parasites sharing the same plant host: oak powdery mildew as a case study. Ecosphere 7:e01517.
Hansen, E. M., Stone, J. K., Capitano, B. R., Rosso, P., Sutton, W., Winton, L., et al. (2000). Incidence and impact of Swiss needle cast in forest plantations of Douglas-fir in Coastal Oregon. Plant Dis. 84, 773–778. doi: 10.1094/PDIS.2000.84.7.773
Hessenauer, P., Feau, N., Gill, U., Schwessinger, B., Brar, G. S., and Hamelin, R. C. (2021). Evolution and adaptation of forest and crop pathogens in the Anthropocene. Phytopathology 111, 49–67. doi: 10.1094/PHYTO-08-20-0358-FI
Hessenauer, P., Fijarczyk, A., Martin, H., Prunier, J., Charron, G., Chapuis, J., et al. (2020). Hybridization and introgression drive genome evolution of Dutch elm disease pathogens. Nat. Ecol. Evol. 4, 626–638. doi: 10.1038/s41559-020-1133-6
Hoffmann, A. A., and Sgrò, C. M. (2011). Climate change and evolutionary adaptation. Nature 470, 479–485.
Hood, I. A., and Kershaw, J. D. (1975). Distribution and Infection Period of Phaeocryptopus gaeumannii in New Zealand. Available online at: https://www.scionresearch.com/__data/assets/pdf_file/0006/58848/NZJFS5 1975HOOD201_208.pdf (accessed October 30, 1974).
Johnson, G. R. (2002). Genetic variation in tolerance of Douglas-fir to Swiss needle cast as assessed by symptom expression. Silvae Genet. 51, 80–88.
Lan, Y.-H., Shaw, D. C., Ritóková, G., and Hatten, J. A. (2019a). Associations between Swiss needle cast severity and foliar nutrients in young-growth Douglas-fir in Coastal Western Oregon and Southwest Washington, USA. For. Sci. 65, 537–542. doi: 10.1093/forsci/fxz022
Lan, Y. H., Shaw, D. C., Beedlow, P. A., Lee, E. H., and Waschmann, R. S. (2019b). Severity of Swiss needle cast in young and mature Douglas-fir forests in western Oregon, USA. For. Ecol. Manag. 442, 79–95. doi: 10.1016/j.foreco.2019.03.063
Lavender, D. P., and Hermann, R. K. (2014). The Genus Pseudotsuga. Available online at: https://www.for.gov.bc.ca/ftp/rsi/external/!publish/Dry%20Fir%20Committee/Literature%20Review/Baseline%20Literature/Lavender_Hermann_2014_Douglasfir.pdf (accessed June 25, 2021).
Lee, E. H., Beedlow, P. A., Waschmann, R. S., Burdick, C. A., and Shaw, D. C. (2013). Tree-ring analysis of the fungal disease Swiss needle cast in western Oregon coastal forests. Can. J. For. Res. 43, 677–690. doi: 10.1139/cjfr-2013-0062
Lee, E. H., Beedlow, P. A., Waschmann, R. S., Tingey, D. T., Cline, S., Bollman, M., et al. (2017). Regional patterns of increasing Swiss needle cast impacts on Douglas-fir growth with warming temperatures. Ecol. Evol. 7, 11167–11196. doi: 10.1002/ece3.3573
Little, E. L. (1971). Atlas of United States trees. Volume 1. Conifers and Important Hardwoods. Miscellaneous Publication 1146. Washington, DC: U.S. Department of Agriculture, Forest Service, 320.
Liu, C., Newell, G., and White, M. (2019). The effect of sample size on the accuracy of species distribution models: considering both presences and pseudo-absences or background sites. Ecography 42, 535–548. doi: 10.1111/ecog.03188
Liu, C., Wolter, C., Xian, W., and Jeschke, J. M. (2020). Most invasive species largely conserve their climatic niche. Proc. Natl. Acad. Sci. U.S.A. 117, 23643–23651. doi: 10.1073/pnas.2004289117
Maguire, B. (1973). Niche response structure and the analytical potentials of its relationship to the habitat. Am. Natural. 107, 213–246.
Maguire, D. A., Kanaskie, A., and Voelker, W. (2002). Growth of young Douglas-fir plantations across a gradient in Swiss needle cast severity. West. J. Appl. For. 17, 86–95. doi: 10.1093/wjaf/17.2.86
Maguire, D. A., Mainwaring, D. B., and Kanaskie, A. (2011). Ten-year growth and mortality in young Douglas-fir stands experiencing a range in Swiss needle cast severity. Can. J. For. Res. 41, 2064–2076. doi: 10.1139/x11-114
Manter, D. K., Bond, B. J., Kavanagh, K. L., Rosso, P. H., and Filip, G. M. (2000). Pseudothecia of Swiss needle cast fungus, Phaeocryptopus gaeumannii, physically block stomata of Douglas fir, reducing CO2 assimilation. New Phytol. 148, 481–491. doi: 10.1046/j.1469-8137.2000.00779.x
Manter, D. K., Reeser, P. W., and Stone, J. K. (2005). A climate-based model for predicting geographic variation in swiss needle cast severity in the Oregon Coast range. Phytopathology 95, 1256–1265. doi: 10.1094/PHYTO-95-1256
Manter, D. K., Winton, L. M., Filip, G. M., and Stone, J. K. (2003). Assessment of Swiss needle cast disease: temporal and spatial investigations of fungal colonization and symptom severity. Phytopathologische Zeitschrift. J. Phytopathol. 151, 344–351. doi: 10.1046/j.1439-0434.2003.00730.x
Marmion, M., Parviainen, M., Luoto, M., Heikkinen, R. K., and Thuiller, W. (2009). Evaluation of consensus methods in predictive species distribution modelling. Divers. Distrib. 15, 59–69. doi: 10.1111/j.1472-4642.2008.00491.x
Mazerolle, M. J. (2017). Package “AICcmodavg.” R Package. 281. Available online at: https://cran.uib.no/web/packages/AICcmodavg/AICcmodavg.pdf (accessed August 21, 2020).
McDowell, N. G., Williams, A. P., Xu, C., Pockman, W. T., Dickman, L. T., Sevanto, S., et al. (2016). Multi-scale predictions of massive conifer mortality due to chronic temperature rise. Nat. Clim. Chang. 6, 295–300. doi: 10.1038/nclimate2873
Michaels, E., and Chastagner, G. A. (1984). Seasonal availability of Phaeocryptopus gaeumannii ascospores and conditions that influence their release. Plant Dis. 68, 942–944. doi: 10.1094/pd-69-942
Mildrexler, D. J., Shaw, D. C., and Cohen, W. B. (2019). Short-term climate trends and the Swiss needle cast epidemic in Oregon’s public and private coastal forestlands. For. Ecol. Manag. 432, 501–513. doi: 10.1016/j.foreco.2018.09.025
Mildrexler, D. J., Yang, Z., Cohen, W. B., and Bell, D. M. (2016). A forest vulnerability index based on drought and high temperatures. Remote Sens. Environ. 173, 314–325.
Montwé, D., Elder, B., Socha, P., Wyatt, J., Noshad, D., Feau, N., et al. (2021). Swiss needle cast tolerance in British Columbia’s coastal Douglas-fir breeding population. Forestry 94, 193–203. doi: 10.1093/forestry/cpaa024
Moss, R. H., Edmonds, J. A., Hibbard, K. A., Manning, M. R., Rose, S. K., van Vuuren, D. P., et al. (2010). The next generation of scenarios for climate change research and assessment. Nature 463, 747–756. doi: 10.1038/nature08823
Nadeau, C. P., and Urban, M. C. (2019). Eco-evolution on the edge during climate change. Ecography 42, 1280–1297. doi: 10.1086/704780
Ojeda Alayon, D. I., Tsui, C. K. M., Feau, N., Capron, A., Dhillon, B., Zhang, Y., et al. (2017). Genetic and genomic evidence of niche partitioning and adaptive radiation in mountain pine beetle fungal symbionts. Mol. Ecol. 26, 2077–2091. doi: 10.1111/mec.14074
Pandit, K., Smith, J., Quesada, T., Villari, C., and Johnson, D. J. (2020). Association of recent incidence of foliar disease in pine species in the Southeastern United States with Tree and Climate Variables. Forests 11:1155. doi: 10.3390/f11111155
Pedlar, J. H., McKenney, D. W., Hope, E., Reed, S., and Sweeney, J. (2020). Assessing the climate suitability and potential economic impacts of Oak wilt in Canada. Sci. Rep. 10:19391. doi: 10.1038/s41598-020-75549-w
Peterson, A. T., Soberón, J., and Sánchez-Cordero, V. (1999). Conservatism of ecological niches in evolutionary time. Science 285, 1265–1267. doi: 10.1126/science.285.5431.1265
Préau, C., Isselin-Nondedeu, F., Sellier, Y., Bertrand, R., and Grandjean, F. (2019). Predicting suitable habitats of four range margin amphibians under climate and land-use changes in southwestern France. Reg. Environ. Chang. 19, 27–38. doi: 10.1007/s10113-018-1381-z
Purse, B. V., and Golding, N. (2015). Tracking the distribution and impacts of diseases with biological records and distribution modelling. Biol. J. Linn. Soc. 115, 664–677. doi: 10.1186/s12868-016-0283-6
Ritóková, G., Mainwaring, D. B., Shaw, D. C., and Lan, Y.-H. (2021). Douglas-fir foliage retention dynamics across a gradient of Swiss needle cast in coastal Oregon and Washington. Can. J. For. Res. 51, 573–582. doi: 10.1139/cjfr-2020-0318
Ritóková, G., Shaw, D. C., Filip, G., Kanaskie, A., and Norlander, D. (2016). Swiss needle cast in western oregon douglas-fir plantations: 20-year monitoring results. For. Trees Livelihoods 7:155.
Rosso, P. H., and Hansen, E. M. (2003). Predicting swiss needle cast disease distribution and severity in young douglas-fir plantations in coastal Oregon. Phytopathology 93, 790–798. doi: 10.1094/PHYTO.2003.93.7.790
Shaw, D. C., Ritóková, G., Lan, Y.-H., Mainwaring, D. B., Russo, A., Comeleo, R., et al. (2021). Persistence of the Swiss needle cast outbreak in Oregon Coastal Douglas-fir and new insights from research and monitoring. J. For. 119, 407–421.
Shaw, D. C., Woolley, T., and Kanaskie, A. (2014). Vertical foliage retention in Douglas-fFir across environmental gradients of the Western Oregon Coast range influenced by Swiss needle cast. Northwest Sci. 88, 23–32. doi: 10.3955/046.088.0105
Shaw, M. W., and Osborne, T. M. (2011). Geographic distribution of plant pathogens in response to climate change. Plant Pathol. 60, 31–43. doi: 10.1111/j.1365-3059.2010.02407.x
Stone, J. K., Capitano, B. R., and Kerrigan, J. L. (2008a). The histopathology of Phaeocryptopus gaeumannii on Douglas-fir needles. Mycologia 100, 431–444. doi: 10.3852/07-170r1
Stone, J. K., Coop, L. B., and Manter, D. K. (2008b). Predicting effects of climate change on Swiss needle cast disease severity in Pacific Northwest forests. Can. J. Plant Pathol. 30, 169–176.
Stone, J. K., Hood, I. A., Watt, M. S., and Kerrigan, J. L. (2007). Distribution of Swiss needle cast in New Zealand in relation to winter temperature. Australas. Plant Pathol. APP 36, 445–454.
Sturrock, R. N., Frankel, S. J., Brown, A. V., Hennon, P. E., Kliejunas, J. T., Lewis, K. J., et al. (2011). Climate change and forest diseases. Plant Pathol. 60, 133–149. doi: 10.1016/j.actatropica.2021.106123
Thuiller, W., Lafourcade, B., Engler, R., and Araújo, M. B. (2009). BIOMOD - a platform for ensemble forecasting of species distributions. Ecography 32, 369–373. doi: 10.1111/j.1600-0587.2008.05742.x
Thuiller, W., Lavorel, S., Araújo, M. B., Sykes, M. T., and Colin Prentice, I. (2005). Climate change threats to plant diversity in Europe. Proc. Natl. Acad. Sci. U.S.A. 102, 8245–8250. doi: 10.1073/pnas.0409902102
Wang, T., Hamann, A., Spittlehouse, D., and Carroll, C. (2016). Locally downscaled and spatially customizable climate data for historical and future periods for North America. PLoS One 11:e0156720. doi: 10.1371/journal.pone.0156720
Watts, A., Meinzer, F., and Saffell, B. J. (2014). Fingerprints of a Forest Fungus: Swiss Needle Cast, Carbon Isotopes, Carbohydrates, and Growth in Douglas-fir. Science Findings 167. Portland, OR: US Department of Agriculture, Forest Service, Pacific Northwest Research Station, 167.
Weiskittel, A. R., Crookston, N. L., and Rehfeldt, G. E. (2012). Projected future suitable habitat and productivity of Douglas-fir in western North America. Schweiz. Z. For. 163, 70–78.
Wicklow, D. T., and Zak, J. C. (1979). Ascospore germination of carbonicolous ascomycetes in fungistatic soils: an ecological interpretation. Mycologia 71, 238–242.
Wilhelmi, N. P., Shaw, D. C., Harrington, C. A., St. Clair, J. B., and Ganio, L. M. (2017). Climate of seed source affects susceptibility of coastal Douglas-fir to foliage diseases. Ecosphere 8:e02011.
Winton, L. M., Hansen, E. M., and Stone, J. K. (2006). Population structure suggests reproductively isolated lineages of Phaeocryptopus gaeumannii. Mycologia 98, 781–791. doi: 10.3852/mycologia.98.5.781
Yin, X., Feau, N., Tanney, J. B., and Hamelin, R. C. (2020). Mining herbarium samples to study adaptation to climate change in a tree pathogen. [Conference Presentation Abstract]. British Columbia regional meeting, 2020/Réunion régionale de la Colombie-Britannique, 2020. Can. J. Plant Pathol. 43, 332–338. doi: 10.1080/07060661.2021.1889804
Keywords: climate change, epidemiology, Douglas-fir, Swiss needle cast, pathogen genetics
Citation: Herpin-Saunier NYH, Sambaraju KR, Yin X, Feau N, Zeglen S, Ritokova G, Omdal D, Côté C and Hamelin RC (2022) Genetic Lineage Distribution Modeling to Predict Epidemics of a Conifer Disease. Front. For. Glob. Change 4:756678. doi: 10.3389/ffgc.2021.756678
Received: 10 August 2021; Accepted: 23 December 2021;
Published: 25 February 2022.
Edited by:
Ari Mikko Hietala, Norwegian Institute of Bioeconomy Research (NIBIO), NorwayReviewed by:
Juha Honkaniemi, Natural Resources Institute Finland (Luke), FinlandCopyright © 2022 Herpin-Saunier, Sambaraju, Yin, Feau, Zeglen, Ritokova, Omdal, Côté and Hamelin. This is an open-access article distributed under the terms of the Creative Commons Attribution License (CC BY). The use, distribution or reproduction in other forums is permitted, provided the original author(s) and the copyright owner(s) are credited and that the original publication in this journal is cited, in accordance with accepted academic practice. No use, distribution or reproduction is permitted which does not comply with these terms.
*Correspondence: Naomie Y. H. Herpin-Saunier, bmFvbWllLmhlcnBpbi1zYXVuaWVyLjFAdWxhdmFsLmNh
Disclaimer: All claims expressed in this article are solely those of the authors and do not necessarily represent those of their affiliated organizations, or those of the publisher, the editors and the reviewers. Any product that may be evaluated in this article or claim that may be made by its manufacturer is not guaranteed or endorsed by the publisher.
Research integrity at Frontiers
Learn more about the work of our research integrity team to safeguard the quality of each article we publish.