- 1Department of Oceanography and Hydrography, Kenya Marine and Fisheries Research Institute, Mombasa, Kenya
- 2Department of Biological Sciences, University of Embu, Embu, Kenya
- 3Department of Natural Resources and Management, Egerton University, Njoro, Kenya
Mangroves are carbon-rich ecosystems found in tropical and subtropical areas around the world. However, they are threatened by a combination of natural and human-induced factors. When mangroves are lost or degraded, their co-benefits to human society are greatly diminished along with the ecosystem’s ability to sequester carbon. The current study assessed mangrove cover and cover change, as well as measuring carbon stocks and their emissions levels from the mangroves of Lamu County, Kenya. We sampled above-and below-ground carbon pools, including soil organic carbon (SOC), in 191 plots distributed throughout the study area. Lastly, we evaluated the economics of avoiding mangrove deforestation based on the carbon-offset market. The total carbon stock of mangroves in Lamu was estimated at 20 million Mg C, with an average density of 560.22 ± 79.79 Mg C ha–1. Southern swamps recorded significantly higher carbon densities (p < 0.05) than other mangrove management blocks in Lamu. At least 1,739 ha of mangroves in Lamu were lost between 1990 and 2019 due to anthropogenic activities, representing a decline of 60 ha yr–1. Total emissions from loss and degradation of mangroves in Lamu is estimated at 140.1 Mg C ha–1; which translates to 30,840.1 Mg CO2e yr–1. Assuming an offset price of US$10/Mg CO2e, the estimated costs of avoided emissions in Lamu is US$308,401 yr–1 plus other co-benefits such as fishery functions and shoreline protection. Mainstreaming mangroves and associated blue carbon ecosystems into national development and climate change agenda could accelerate Kenya’s achievements of both Sustainable Development Goals (SDGs) and the Paris Agreement.
Introduction
Mangroves and associated coastal wetlands are carbon-rich ecosystems (Donato et al., 2011). Despite occupying only 0.7% of the tropical forest area (Giri et al., 2011), mangroves account for about 3–4% of global carbon sequestration by forests (Bhomia et al., 2016; Alongi, 2020) and 10–15% of total carbon sequestration in the coastal ocean (Alongi, 2014). This is in addition to the support value of mangrove to coastal fisheries (Barbier et al., 2011), shoreline protection (Alongi, 2012, 2014); and the provision of harvestable wood and non-wood resources to millions of people around the world (Siikamäki et al., 2012; Lee et al., 2014; Murdiyarso et al., 2015). Unfortunately, mangroves are being lost globally at an alarming rate of 1–2% by area per year (Duke et al., 2007; Giri et al., 2011), which is significantly higher than the global loss of tropical forests, estimated at 0.5% per year (FAO, 2007; IPCC, 2019). A report by the International Science Policy Platform on Biodiversity and Ecosystem Services (IPBES) predicted catastrophic loss and degradation of ecosystems globally, including mangroves (IPBES, 2019). When mangroves are lost or their areas are converted for other land uses, their co-benefits to human society are greatly diminished along with the ecosystems’ capacity to sequester carbon (Pendleton et al., 2012). Restoration and protection of mangroves is, therefore, recognized as a priority for both climate change mitigation and adaptation; and several countries have identified measures that harness these benefits in the first and second submissions of their Nationally Determined Contributions (NDCs) to the Paris Agreement (Herr and Landis, 2016; Gallo et al., 2017; Lopez, 2021). Inclusions of concrete ocean-based mitigations and adaptations actions allow countries to increase ambitions of realizing their NDCs commitments (Taraska, 2018).
The total area of mangroves in Kenya is estimated at 61,271 ha; 62% of which occur in Lamu and the surrounding Islands (GoK, 2017a). These forests provide goods and services that are of ecological, economic, and environmental value to the people (Kairo et al., 2009; Huxham et al., 2017; Owuor et al., 2019; Hamza et al., 2020). Over-harvesting of mangrove wood products, conversion pressure, pollution effects, and climate change factors contributed to a 40% decline in mangrove cover in Kenya between 1990 and 2010 (GoK, 2017a). Losses and degradation of mangroves have negative effects on fisheries (Huxham et al., 2004), resources sustainability (Dahdouh-Guebas et al., 2000; Kairo et al., 2001; Mohamed et al., 2009), and shoreline stability (Abuodha and Kairo, 2001; Dahdouh-Guebas et al., 2004). In addition, the degradation of mangrove forests changes their roles as carbon sinks to carbon sources (Donato et al., 2011; Kauffman et al., 2020). Estimates indicate that losses and degradation of mangroves can contribute up to 10% of annual greenhouse gas (GHG) emissions from land-use changes; with small mangrove harvesting, that is characteristic of Kenya contributing to emissions of up to 35.7 ± 76.9 Mg C ha–1 yr–1 (Lang’at et al., 2014).
Although Kenya has emissions estimates in its NDCs and emission reductions strategies that include tree planting in the agriculture and forestry sectors (GoK, 2017b), these reforestation and afforestation endeavors rarely included mangrove forests, despite their high carbon sequestration rates and multiple ecosystem services they provide. By not including mangroves and associated blue carbon ecosystems in the NDCs, Kenya may be under- or overestimating its GHG emissions. This gap provided an opportunity of influencing inclusions of blue carbon ecosystems in the updated Kenya’s NDCs; as well as ensuring that coastal wetlands are accounted for in future global stock-take (GoK, 2020). Among ocean climate actions in the updated NDCs, Kenya aims to explore coastal carbon Payment for Ecosystem Services (PES), implement the national mangrove management plan, incorporate nature-based solutions in flood controls, as well as including coastal wetlands in GHG emissions and removals (GoK, 2020).
Estimation of mangrove biomass/carbon is necessary for the development of realistic carbon budgets in coastal wetlands (Jakovac et al., 2020). These involve quantifications of biomass/carbon in above- and below-ground components; and how this is influenced by human and natural factors (Radabaugh et al., 2021; Rasquinha and Mishra, 2021). The objectives of the current study were, therefore, to (i) assess status and conditions of mangroves in Lamu; in terms of cover, forest structure and regeneration potential and (ii) measure vegetation and sediment carbon stocks, as well as estimate carbon at risks of emissions. We assessed a number of biophysical factors related to mangrove forest structure and carbon dynamics with the primary objectives of comparing the results with different mangrove areas in Kenya. We hypothesized that Lamu mangroves are resilient and carbon rich ecosystems comparable to similar productive forests elsewhere in the world. Study findings are expected to inform policy making processes particularly on the use of blue carbon ecosystems as part of nature-based solutions to climate change challenges.
Materials and Methods
Study Area
The study was carried out in Lamu County in Kenya located between latitudes 1° 45′ and 2° 20′ South and longitudes 40° 44′ and 41° 30′ East (Figure 1). The characteristic features of Lamu are the coastal lagoons, multiple small islands, sand dunes, and an expansive seascape that support healthy mangrove stands and other coastal ecosystems (Kairo et al., 2002b). The land comprises geological structures with variations in soil grain sizes, porosity, permeability, compaction, and texture. Specific to mangrove areas, the soils are predominantly unconsolidated collarine, with poor water holding capacity and extreme alkalinity (Boxem et al., 1987). There are no permanent rivers draining into mangroves in Lamu. Freshwater supply is by seasonal streams as well as groundwater aquifers (County Government of Lamu, 2018). Offshore, there is evidence of major rivers draining into the Indian Ocean in the Lamu area which are now separated by fossilized underwater deltaic features (Caswell, 1953).
Lamu is characterized by a hot and humid tropical climate; with an annual rainfall ranging from 500 to 900 mm yr–1 and mean temperature of 27°C. The relative humidity is high throughout the year, reaching 90% during the rainy season; which is ideal for mangrove growth and development (Tomlinson, 1986; Spalding et al., 2010). Monsoon winds strongly influence rainfall seasons along the coast resulting in two rainy seasons. Long rains occur from March to May during the South East Monsoon while the short rains are experienced during the North East Monsoon from October to December (Bosire et al., 2016; Camberlin, 2018). January to March and August to October are usually hot and dry months.
Mangroves in Lamu
Total mangrove area in Lamu is estimated at 37,350 ha; represented by nine species (GoK, 2017a). The dominant mangrove forest types in Lamu are pure and mixed stands of Rhizophora mucronata that account for 38.1% of the total mangrove forest in the area (GoK, 2017a). Other prominent mangrove formations in Lamu are pure stands of Avicennia marina and Ceriops tagal that occupy landwards and mid-zones, respectively.
According to the Kenya’s National Mangrove Ecosystem Management Plan (2017–2027), mangroves in Lamu have been classified into five management blocks, namely; Northern Swamps, Pate Island Swamps, Northern Central Swamps, Southern Swamps, and Mongoni and Dodori Creek Swamps (Figure 1). For ease of reference and comparisons, we have maintained these management boundaries in the current study. Northern Central and Southern Swamps contain 62% of the mangroves in Lamu; much of which falls within the Kiunga–Dodori Marine Protected Area and Biosphere Reserve where removal of wood products is regulated (Kairo et al., 2002b).
Commercial harvesting of mangrove poles is a traditional activity of the Lamu people (Hamza et al., 2020). The harvested poles are traded in urban centers along the coast for building and construction. Clear felling for fuelwood has seriously impacted mangrove forests in some parts of the Southern Swamps at Manda Island; while blank contiguous areas caused by the 1997–98 El-Niño rains in Mongoni and Dodori Creek Swamps have not recovered to-date due to heavy siltation which altered conditions for natural regeneration (GoK, 2017a).
Mapping Mangrove Cover/and Cover Change
Use of remotely sensed data and Geographical Information Systems (GIS) is a common practice in estimating forest cover and cover change; including mangroves (Polidoro et al., 2010; Giri et al., 2011; Kirui et al., 2013; Thomas et al., 2017; Mungai et al., 2019). Mapping of mangroves is challenged by their low spatial extent when compared to terrestrial forests, the cloudy nature associated with coastal areas, as well as problems linked to poor species discrimination (Thomas et al., 2017; Mungai et al., 2019). In the current study, Landsat imagery dating from 1990 were accessed and reviewed for quality and cloud-free scenes. Data was freely acquired from the United States Geological Survey (USGS) Landsat satellites 5, 7, and 8 (path 165, row 61 and 62). This was complemented by SPOT and Sentinel images acquired from the European Space Agency (ESA; Table 1).
Unsupervised classification was performed prior to fieldwork to retrieve different spectral classes for comparison of the best result yielding method. This was followed by intensive field campaigns across the mangroves in Lamu. The Combined Mangrove Recognition Index (CMRI) was used in discriminating mangrove cover from other non-mangrove areas. Normalized Difference Moisture Index (NDMI) was used to generate long-term trends of mangrove gains and losses within the study area. Supervised classification algorithm was then performed to map out different mangrove species formations. Ground control points (GCPs) were collected using a Garmin Global Positioning System (GPS) 76 in the Universal Transverse Mercator (UTM) coordinate system. To minimize errors resulting from GPS accuracy, collected GCPs were ensured to be within a 10 m radius of the same mangrove species formation. The acquired data was converted to top-of-atmosphere spectral reflectance using the Joint Research Center’s IMPACT Toolbox.
Validation of Classification Accuracy
Accuracy assessment and validation of the result of the classification process is key to assess the representativeness of the classified phenomena to the real world in image analysis (FAO, 1994; Giri, 2016). For this study we used ArcGIS 10.6, Google Earth Pro, and part of the ground-truthing data obtained during field campaigns in assessing the classification accuracy. A sample of the generated data from classified satellite imagery was confirmed with the ground-truthed data collected during field campaigns. A confusion matrix was then generated to calculate the producer’s accuracy, user’s accuracy, overall accuracy, from which the Kappa coefficient (KΛ) was assessed. An error matrix was generated using the equation by Bishop et al. (1977) whose applications can be found in; Kamal and Johansen (2017):
Where, KΛ = Kappa Coefficient
r = Number of rows in the matrix
xii = Number of observations in row i and column i (the major diagonal in the confusion matrix)
xi+ and x+i = Marginal totals of row i and column i
N = Total number of observations.
Assessment of Forest Structure
Stratified random sampling design was used for vegetation surveys. Stratification was at two levels: the land-use level that distinguished mangroves from non-mangrove forests; and the tree species level. Mangroves have a clear tonality and texture that makes it easy to discriminate them in satellite images (Ramsey and Jensen, 1996; Díaz and Blackburn, 2003; Howard et al., 2014). In the current study, we assessed the following carbon pools: above-and below-ground vegetation, and soil organic carbon (SOC). These constitute the major carbon pools in mangroves that must be quantified for national emission reporting (IPCC, 2014).
In the field, belt transects were established perpendicular to the waterline. Vegetation sampling was carried out within 400 m2 square quadrats laid along transects. A total number of 191 quadrats were sampled in 64 transects. Within each quadrat, individual trees with a diameter at breast height (dbh) ≥2.5 cm were identified, counted, and their position mapped. Data on species, tree height, and dbh were recorded; from which basal area (m2 ha−1), stocking density (stems ha−1), and stand biomass (Mg ha−1) were estimated. These structural indices were derived by using the blue carbon manual of Howard et al. (2014) as well as protocol for measuring, monitoring and reporting of blue carbon by Kauffman and Donato (2012).
Total Ecosystems Carbon Stocks Assessments
The study focused mostly on above-and below-ground biomass carbon as well as SOC. Litter, deadwood, and understory are important carbon pools for forested ecosystems (IPCC, 2006). However, in mangrove environments, litter is normally consumed by the active benthic community (Lee, 1998) and thus incorporated in the SOC (Andreetta et al., 2014) and some exported through tides and rivers (Kauffman and Donato, 2012). Further, mangroves do not support understory (Janzen, 1985; Corlett, 1986; Snedaker and Lahmann, 1988) as such their overall contribution to above ground biomass carbon is minimal. In developing economies, including Kenya, some of mangrove deadwoods are collected for fuelwood (Dahdouh-Guebas et al., 2000); while the remaining rots and are remineralized into soil carbon pools (Chmura et al., 2003). The IPCC (2014) report on coastal wetlands identifies below-and above-ground vegetation biomass and soil carbon as significant pools that should be included during mangrove carbon accounting.
Measurements of Vegetation Carbon
We aimed at using local equations for estimating biomass carbon of mangroves in Lamu. However, this was not possible, as there are no robust biomass relations that have been developed for principal mangrove species in Kenya. Instead, we used the generalized biomass equations for mangroves using stem diameter as the dependent variable (Komiyama et al., 2005, 2008) and localized species-specific wood densities (Gillerot et al., 2018). The total vegetation biomass was then computed as a sum of below- and aboveground living biomass (Komiyama et al., 2005, 2008):
Where, AGB, above-ground biomass (kg), BGB, below-ground root biomass (kg), ρ, wood density (g cm–3), and D, dbh (cm).
Biomass values were converted to carbon equivalents by multiplying with conversion factors of 0.50 and 0.39 for AGB and BGB, respectively following procedures by Kauffman and Donato (2012).
Soil Carbon
This carbon pool comprised soil organic material to a maximum depth of 100 cm. Soil cores were extracted from the center of the quadrats used for vegetation surveys using a 7.0 cm diameter semi-circular corer. The extracted cores were systematically divided into depth intervals from 0 – 15, 15 – 30, 30 – 50, and 50 – 100 cm (Kauffman and Donato, 2012). Sub-samples of 5.0 cm in length were collected at the midpoint of each depth interval, placed in labeled sample bottles, and transported to the laboratory for determination of dry bulk density, carbon concentration and SOC.
The Loss on Ignition method (LOI) was used to determine SOC. For LOI, oven-dried soil samples were homogenized and placed in pre-weighed aluminum crucibles. The samples were then set in a muffle furnace for combustion at 450°C for 8 h, after which they were cooled in a desiccator and weighed. What was lost during the oxidation represents the soil organic matter (SOM) using the relation:
Where, LOI = Loss on Ignition (Kauffman and Donato, 2012; Howard et al., 2014).
SOM was converted to organic carbon using the relation:
Where, SOM = Soil Organic Matter.
Finally, the soil carbon per sampled depth interval was calculated as:
Where %C was expressed as a whole number.
Total soil carbon was obtained by adding soil carbon masses of all depths (Kauffman and Donato, 2012; Howard et al., 2014).
Data Analysis
Normality test and homogeneity of variance were done using Kolmogrov–Smirnov test and Levene’s test, respectively. Data was further normalized for parametric tests where necessary. Data that met the normality, assumption was further analyzed for any significant differences between and within management blocks using One-way Analysis of Variance (ANOVA). Pearson’s product-moment correlation coefficient was used to determine the relationship between above-and below-ground biomass as well as vegetation carbon and SOC. All data analyses were done using Minitab 17.0 program and p-value was always set at significance level of 0.05.
Results
Mangrove Cover and Cover Change
The current area of mangroves in Lamu has been estimated at 35,678 ha, which is slightly lower than the 37,350 ha used in the National Mangrove Management Plan (GoK, 2017a). Only six of the nine mangrove species described in Kenya (Bosire et al., 2016) were encountered in the current survey. Accuracy levels achieved in mapping overall mangrove coverage was 95% while accuracy achieved in discriminating mangroves by species formation stood at 71.3% registering a Kappa coefficient of 0.6153 (61.5%). In all management blocks, the dominant mangrove formation is Rhizophora mucronata and Avicennia marina forests (Table 2). At least 35% of mangroves in Lamu occur in Northern Central Swamps; followed by Southern Swamps (26.9%), Pate Island Swamps (16.9%), Mongoni and Dodori Creek Swamps (12.8%), and Northern Swamps (8.3%) – Table 2.
Based on the remotely sensed data, mangroves in Lamu have not been static. Overall, a cover loss of 1,739 ha was reported over the 1990–2019 period; translating to a loss of 60 ha yr–1. However, the loss was not uniform within the different study periods; with 2010–2019 experiencing a higher loss (1,029 ha, at a rate of 114 ha yr–1), than the 2000–2010 period (901 ha, at a rate of 90.1 ha yr–1) (Table 3). Hotspots of mangrove loss and degradation were observed close to human settlements and in concession sites at Northern Central, Pate Island, and Southern Swamp forests (Figure 2).
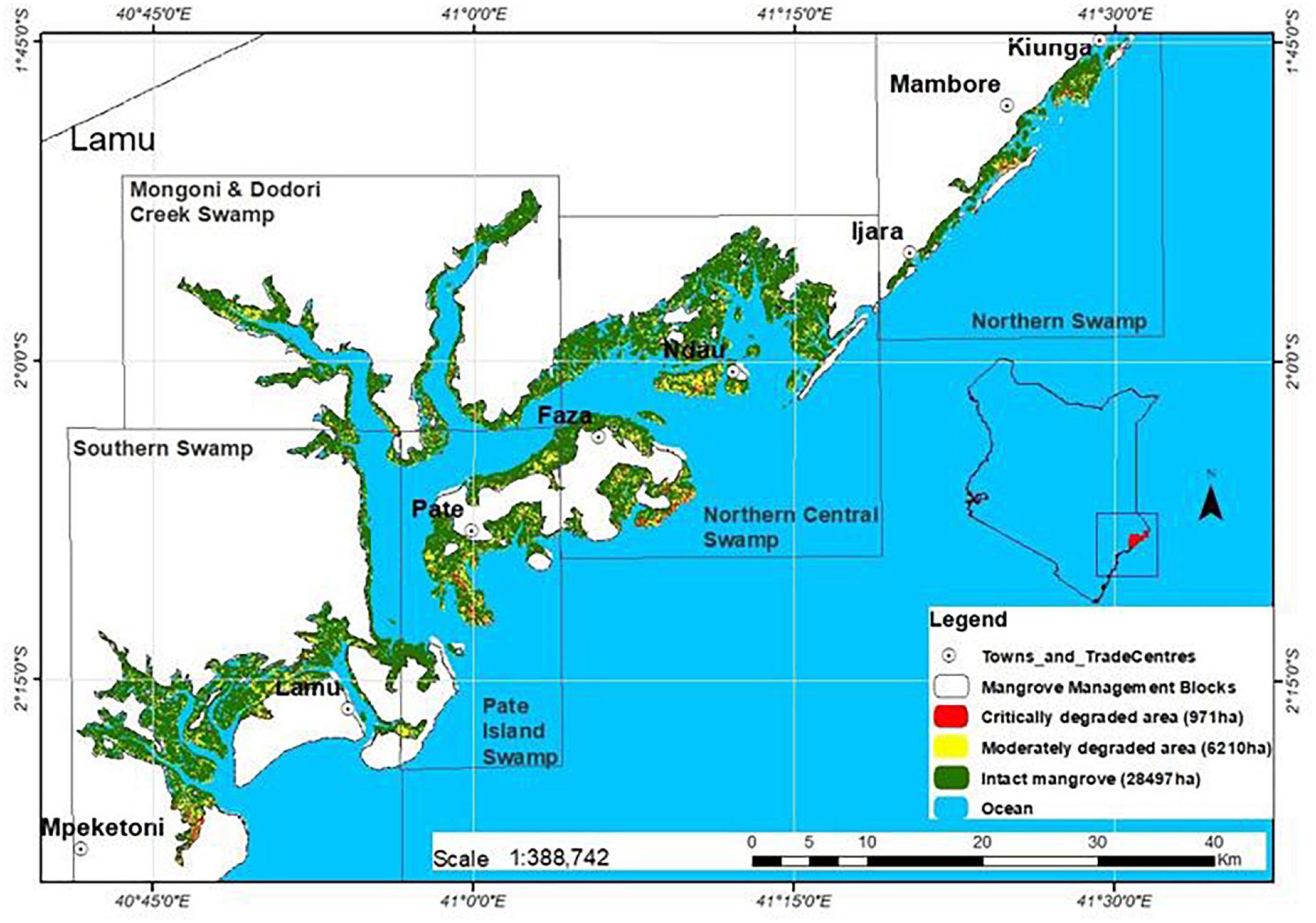
Figure 2. Lamu mangrove cover change in the period 2010–2019. Heighted mangrove loss was witnessed close to human settlement in Pate Island, Northern Central, and Southern swamps.
Further analysis revealed no significant variations in mangrove cover changes in different management blocks over the 1990–2019 periods (T = −1; p > 0.05). Similar trends were observed in Northern Central Swamps, Northern Swamps, and Pate Island swamps whereby mangrove cover increased from the 2000 – 2010 period followed by a decline in the 2010 – 2019 period. On the other hand, mangroves in the Southern Swamps recorded declining trends in the 2000 – 2010 period; and continuously increased in the later period. Unlike other mangrove management blocks, Mongoni and Dodori creek swamps recorded increasing trends throughout the period under investigation (Figure 3).
Stand Density and Biomass
Height-diameter scattergrams of mangroves in Lamu are shown in Figure 4. Across all the management blocks, 50% of the tree diameters and heights were between 3.9 – 10.8 cm and 5.5 – 12 m, respectively. There were significant differences in both diameter and height between the blocks (p < 0.05). Stocking densities of mangroves in Lamu ranged from 1,607 to 3,092 stems ha–1 (mean ± 95% C.I: 2,339 ± 473 stems ha–1). In Northern Central Swamps, where commercial harvesting of mangroves mostly takes place, the mean stocking rate was 2,523 ± 529 stems ha–1 (range: 350 – 7,500 stems ha–1). Stocking densities in all the management blocks decreased with increasing diameter classes (Figure 5). These are typical reversed “J” curves for natural forests with a wide range of size classes and by inference also age classes (Macamo et al., 2018). This pattern was, however, slightly distorted in mangrove harvesting areas of Northern Central Swamps and Southern Swamps forests where Boriti sized poles (dbh range: 11.5–13.9 cm) were significantly reduced (Figures 4B,E).
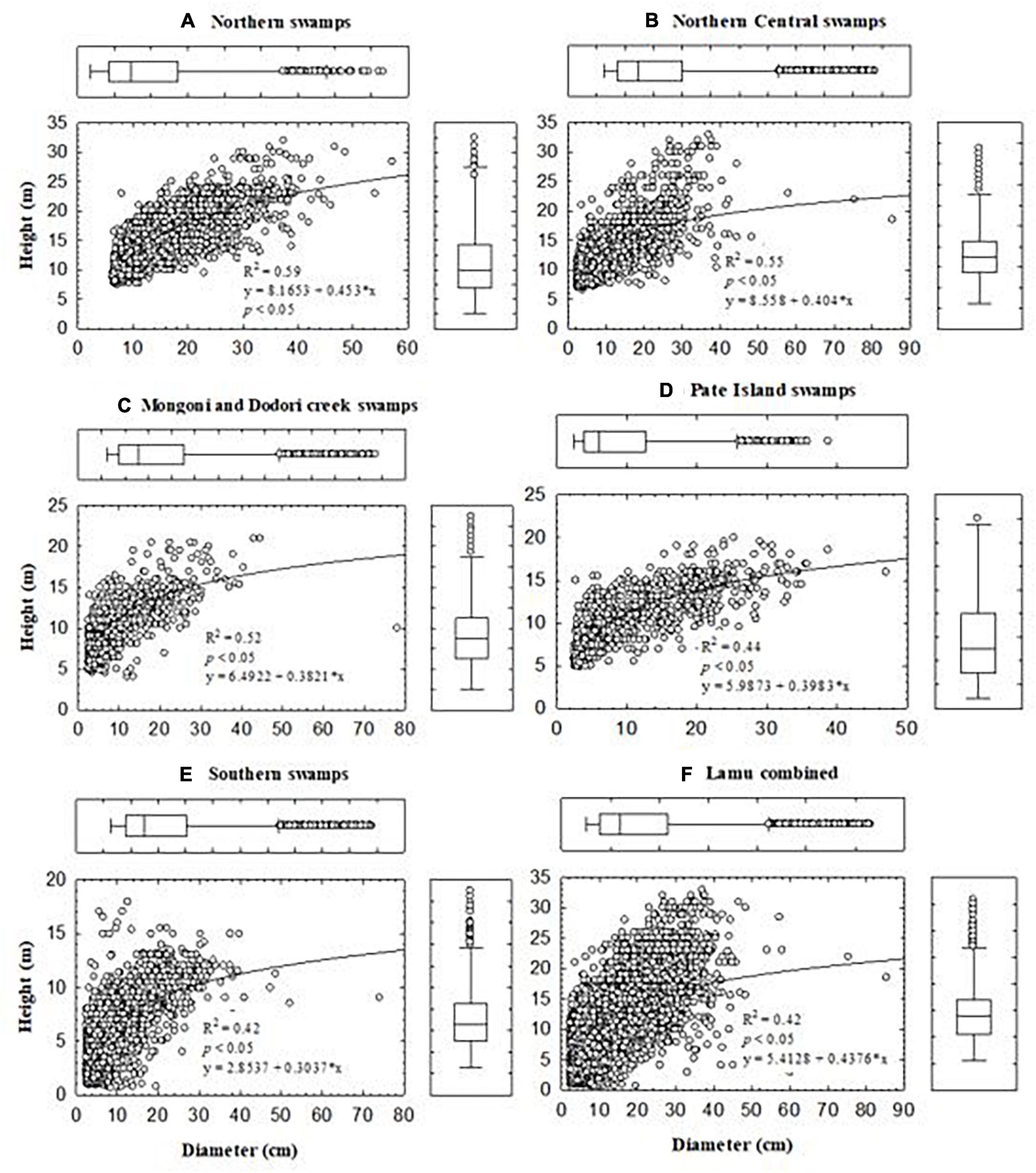
Figure 4. Height – diameter relationships in the five blocks of Lamu mangrove forest (A–E) and the combined ecosystem (F). The box plots display percentile distribution of the DBH and heights in the forest blocks. The extremities of the plot correspond to the maximum and minimum observations in the data set. The ends of the boxes are positioned at the 25 and 75% percentile of the data.
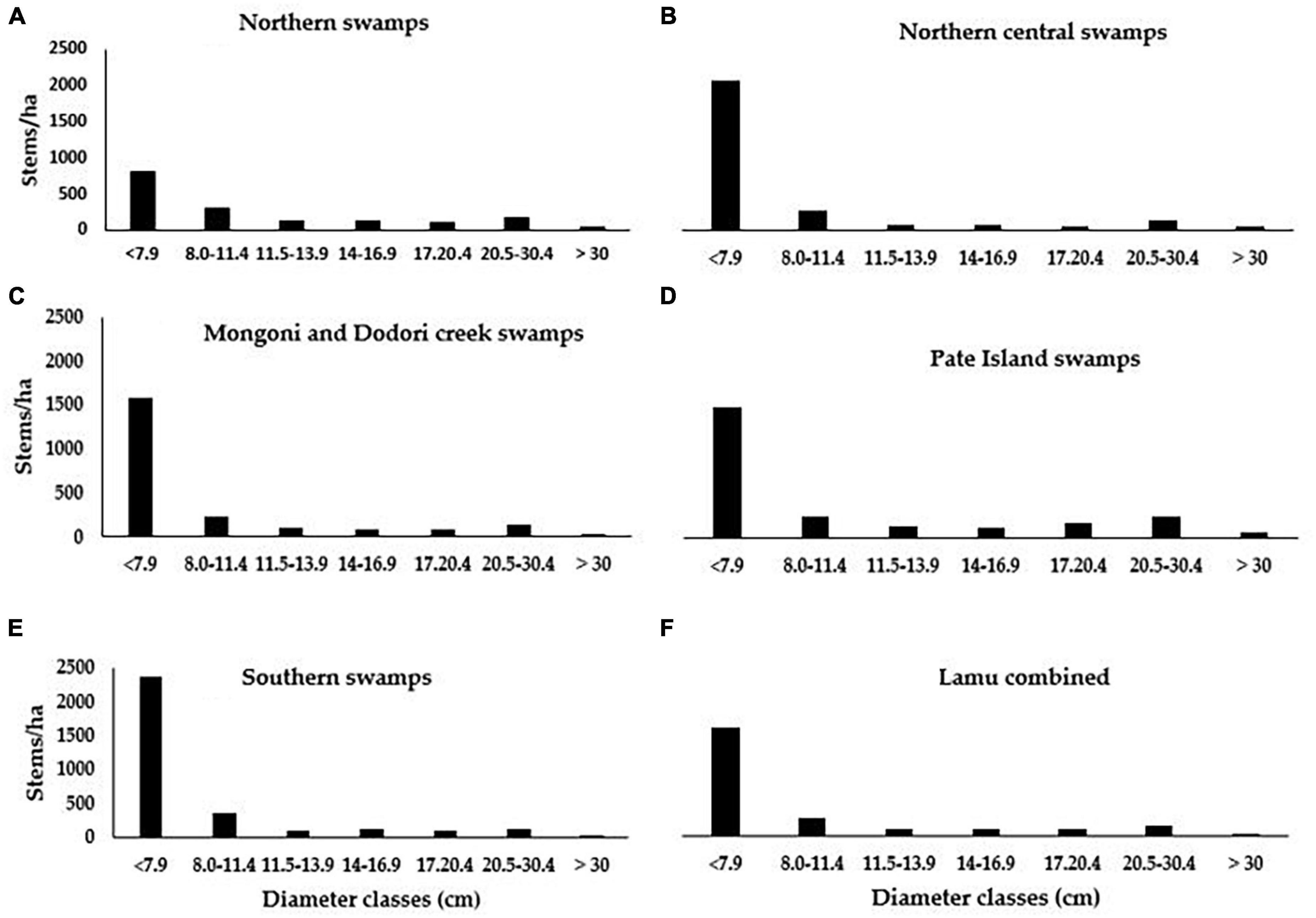
Figure 5. Size class distribution in the blocks of Lamu mangroves (A–E), and the combined ecosystem (F). A typical reversed – J curve was witnessed in all management blocks which is an indicator of non-even aged forests with natural recruitment.
Standing biomass of mangroves in Lamu ranged from 183.22 to 258.87 Mg ha–1 (Mean ± 95% C.I: 255.7 ± 100.61 Mg ha–1). Together with the root biomass, the mean vegetation biomass in mangroves of Lamu was 354.98 ± 137.82 Mg ha–1 (range: 254.63 – 541.98 Mg ha–1) (Table 4). The highest biomass of 541.98 ± 70.59 Mg ha–1 (range: 154.54 – 1,010 Mg ha–1) was recorded in Northern swamps while Mongoni and Dodori creek swamps had the lowest biomass (254.63 ± 60.21 Mg ha–1; range: 28.17– 452.79 Mg ha–1) (Table 4). Mean biomass was significantly different across the five blocks (F = 10.86; p < 0.05). There was a strong positive correlation between the AGB and BGB (r2 = 0.99, p < 0.05).
Total Ecosystem Carbon
Soil carbon in the mangroves of Lamu ranged from 306.8 to 521 Mg C ha–1 (mean ± 95% C.I: 393.66 ± 98.53 Mg C ha–1). There was a significant difference (F = 8.05, p < 0.05) in soil carbon pools between the five mangrove management blocks with Southern Swamps contributing to the difference in means. The other four blocks did not have a significant difference in their means. Southern swamps recorded the highest sediment carbon of 521.0 ± 32.51 Mg C ha–1 (range: 238.62 – 768.72 Mg C ha–1) while Northern swamps recorded the least (306.8 ± 63.78 Mg C ha–1; range: 65.15–560.40 Mg C ha–1) – Figure 6. There was a weak correlation between vegetation and soil carbon pools (r2 = 0.349).
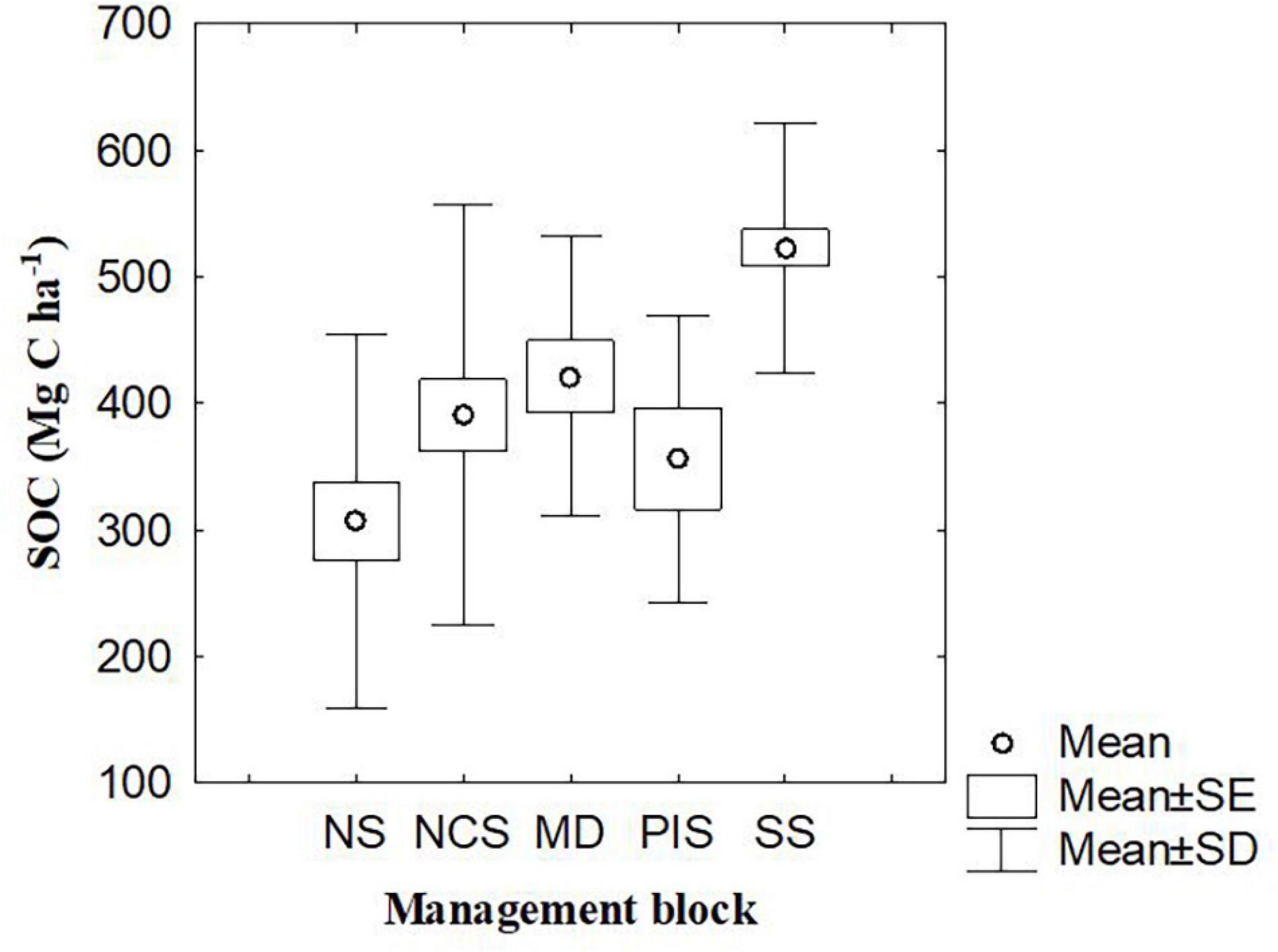
Figure 6. Soil organic carbon (SOC) in different mangrove management blocks of Lamu. MD, Mongoni and Dodori Creek swamps, NS, Northern swamps, NCS, Northern Central swamps, PIS, Pate Island swamps, and SS, Southern swamps.
Total ecosystem carbon (TEC) in mangroves of Lamu was estimated at 20 million Mg C (mean: 560.22 ± 79.79 Mg C ha–1). Of this, 14,031,245.47 Mg C (or 70.3%) constitutes SOC, whereas 4,557,156.65 Mg C (22.8%) and 1,379,139.51 Mg C (6.9%) represents above-and below-ground biomass carbon, respectively (Table 5). The highest mangrove carbon density in Lamu was recorded in Southern swamps (670.73 ± 122.61 Mg C ha–1) while the lowest was in Mongoni and Dodori Creek swamps (513.36 ± 144.31 Mg C ha–1) (Table 5). The mean ecosystemcarbon stocks of mangroves in Lamu were statistically different between the blocks (F = 5.56, p < 0.05).
Discussion
Mangrove Cover and Cover Change
The area of mangroves in Lamu was estimated as 35,678 ha, distributed in five management blocks (Table 2). This is significantly higher than an earlier estimate of 23,500 ha by Kirui et al. (2013), though lower than the 37,350 ha used in the National Mangrove Ecosystem Management Plan (GoK, 2017a). Such differences could mostly be attributed to different methodologies, time and data sources. Kirui et al. (2013) used Landsat imagery while the management plan used high resolution aerial imagery (GoK, 2017a).
The underlying causes of loss and degradation of mangroves in Lamu have been identified as legal and illegal overexploitation of wood products, conversion of mangrove areas for other land uses, and habitat encroachment (Kirui et al., 2013; GoK, 2017a). Climate change has also contributed to the loss of mangroves in Lamu; particularly through siltation (Lang’at and Kairo, 2008; Kairo and Bosire, 2016). During the periods under investigation, gains and losses of mangrove coverage in Lamu were witnessed (Figure 2 and Table 3). Overall, a net loss of 1,739 ha of mangrove cover was experienced from 1990 – 2019; translating to a loss of 60 ha yr–1 (0.16% yr–1). This is similar to the current global loss of mangrove forests, estimated at 0.16% yr–1 (Hamilton and Casey, 2016; Hamilton and Friess, 2018; Alongi, 2020). The current rate is, however, lower than the 0.7% reported by Kirui et al. (2013) for Kenya. Hotspot areas for loss and degradation of mangroves in Lamu were located in Northern Central Swamps at Ndau, Uvondo, and Siyu sites where commercial harvesting of mangrove poles is authorized. Other sites that have suffered similar fates are Yowea and Manda Islands in both Pate Island and Southern Swamps where mangroves are clear-felled for fuelwood [Kairo et al., 2002b; GoK, 2017a].
Structural Attributes
Table 4 summarizes structural attributes for mangroves in Lamu. Only six mangrove species were encountered in Lamu during the present surveys. Earlier studies had encountered eight mangrove species in the Northern and Northern Central Swamps (Kairo et al., 2002b). Hence, the low species count witnessed in the study could mostly be due to sampling intensity. Similar to other mangrove sites in Kenya (e.g., Kairo et al., 2002a,b; Dahdouh-Guebas et al., 2004; Mohamed et al., 2009; Okello et al., 2013) the species Rhizophora mucronata, either alone or in mixed with other species, constitutes the most important forest formation in Lamu.
The standing density of mangroves in Lamu was 2,339 ± 540 stems ha–1 (range: 1,607–3,092 stems ha–1). This is significantly higher than stocking rates recorded in other mangrove areas along the Kenyan coast at Mida (Kairo et al., 2002a), Mombasa (Mohamed et al., 2009), and Tana delta (Bundotich et al., 2009). The stocking density of Mazio and Boriti sized poles (dbh range: 8.0–13.9 cm) was significantly lower (p < 0.05) in Northern Central Swamps, Mongoni and Dodori Creek Swamps, and Southern Swamps possibly due to selective logging to satisfy market demand. These size classes are the most preferred in building and construction industries in the region (Bosire et al., 2016). Selective logging of mangroves has been reported to contribute to low densities of merchantable wood products and reduction in forest quality over time (Kairo et al., 2002a).
Vegetation biomass for mangroves in Lamu ranged from 254.63 to 541.98 Mg ha–1 (Mean ± 95% C.I: 354.98 ± 137.82 Mg ha–1). This is consistent with reported biomass values of productive mangroves elsewhere in Indonesia (Rozainah et al., 2018), Malaysia (Rozainah et al., 2018), Philippines (Thompson et al., 2014), and Dominican Republic (Kauffman et al., 2014); but significantly higher than values reported for degraded systems such as Sofala Bay in Mozambique (Sitoe et al., 2014). Lamu mangroves are amongst the most productive systems in Kenya (Kairo, 2001; Kairo et al., 2002b); and indeed, in the WIO region (Bosire et al., 2012). This attribute has been associated with both geomorphic and oceanographic drivers (Kairo, 2001). Although there are no permanent rivers draining into the mangroves of Lamu, the forcing functions created by the East African Coastal Currents could be involved in pumping freshwaters northward from the Tana River delta to Lamu thus contributing to enhanced marine productivity in the area (United Nations Environmental Programme [UNEP], 1998; ASCLME, 2012; Kamau et al., 2020). High marine productivity in the area may also be as a result of upwelling caused by the interaction of the southerly Somali Coastal Currents with the northward flowing East African Coastal Currents (United Nations Environmental Programme [UNEP], 1998).
Vegetation Carbon
Mean vegetation carbon for mangroves in Lamu was 166.56 Mg C ha–1 (range: 119.46–254.52 Mg C ha–1) (Table 5). This is higher than the 147.5 Mg C ha–1 average global mangrove vegetation carbon (Siikamäki et al., 2012) but lower than the global average of 190.2 Mg C ha–1 (Alongi, 2020). Higher biomass carbon values have been reported on undisturbed mangrove forests of Mozambique [340.6 Mg C ha–1 (Stringer et al., 2015)], West Papua, Indonesia [982 Mg C ha–1 (Murdiyarso et al., 2015), Sumatra, Indonesia [339.7 Mg C ha–1 (Murdiyarso et al., 2015)], and Yap, Micronesia [334.8 Mg C ha–1 (Kauffman et al., 2011)]. Our values, however, are consistent with similar studies in the WIO region in Mahajamba Bay-Madagascar, 88.9 Mg C ha–1 (Jones et al., 2015), Sofala-Mozambique, 53.2 Mg C ha–1 (Sitoe et al., 2014), Zambezi Delta-Mozambique, 211.4 Mg C ha–1 (Stringer et al., 2015), mainland mangroves-Tanzania, 64.7 Mg C ha–1 (Njana et al., 2018), Geza-Tanzania, 103.3 Mg C ha–1 (Alavaisha and Mangora, 2016), and Mtimbwani-Tanzania, 223 Mg C ha–1 (Alavaisha and Mangora, 2016). The observed differences in biomass carbon could be attributed to sample size, species composition, geographical locations, wood density variability, and management regimes (Komiyama et al., 2005; Ren et al., 2010; Donato et al., 2011; Kridiborworn et al., 2012; Alemayehu et al., 2014).
Total Ecosystem Carbon
The average SOC in mangroves of Lamu (mean ± 95% C.I: 393.48 ± 98.58 Mg C ha–1), is within the global range of 37.0 – 2,102.7 Mg C ha–1 (Alongi, 2020); and is comparable to similar studies in the mangroves of Madagascar, 381.02 Mg C ha–1 (Benson et al., 2017), Gabon, 392 Mg C ha–1 (Ajonina et al., 2014) and Brazil, 341 Mg C ha–1 (Kauffman et al., 2018). Soil storage potential of mangroves in Lamu was, however, lower than the global average of 749 Mg C ha–1 (Alongi, 2020) as well as the Indo-Pacific region, 864 Mg C ha–1 (Donato et al., 2011), and Indonesia, 879 Mg C ha–1 (Murdiyarso et al., 2015) mangroves. Such variabilities in carbon storage are expected as mangroves grow in a range of environmental settings experiencing varying energetics (Twilley et al., 2018).
The TEC in mangroves of Lamu is estimated as 20 million Mg C; with a mean of 560.22 ± 64.26 Mg C ha–1 (range: 513.36 – 670.73 Mg C ha–1). This is consistent with the 46.3 – 2,208 Mg C ha–1 global range TEC for mangroves (Alongi, 2020; Kauffman et al., 2020) as well as the IPCC’s default mean of 511 Mg C ha–1 (IPCC, 2014). TEC for Lamu mangroves compares well with regional studies in Geza-Tanzania [414.6 Mg C ha–1 (Alavaisha and Mangora, 2016)] and Madagascar [(454.92 Mg C ha–1 (Benson et al., 2017)]. However, our TEC estimate for mangroves in Lamu is lower than the 664.2 Mg C ha–1 for African mangroves (Alongi, 2020). Equally, TEC value for Lamu is significantly lower than the cenote mangroves of Yucatan Mexico whose soil value alone has been estimated at 2,792 Mg C ha–1, the highest anywhere on earth (Adame et al., 2021).
The deep mangrove soils in Lamu contain 70.3% of the total mangrove organic carbon; while above- and below-ground living biomass contribute 22.8 and 6.9% of the TEC, respectively (Table 5). These values are consistent with global estimates in which 70.65% of mangrove carbon is contained in mangrove soils, 19.57% in above-ground biomass, and 9.78% in below-ground biomass (Hamilton and Friess, 2018).
Carbon Dioxide Emissions
Over the 1990 – 2019 period, mangroves in Lamu decreased from 37,417 to 35,678 ha; a net loss of 1,739 ha (Table 3). Such a change could have activated carbon dioxide (CO2) emissions from the lost vegetation cover as well as from the top 1.0 meter of sediments. IPCC and other studies provide a range of possible fates of “near-surface carbon” upon conversion from 25 to 100% emissions to the atmosphere depending on land use types (Pendleton et al., 2012; IPCC, 2014; Hamilton and Friess, 2018). Using the low end of 25% emissions, potential carbon loss from mangroves in Lamu was calculated as 140.1 Mg C ha–1. To enable comparison with other assessments, the values were expressed in terms of CO2 equivalent, by multiplying C stocks by 3.67, the molecular weight of C in CO2. Therefore, current carbon emissions from mangroves of Lamu is 30,840.1 Mg CO2e yr–1; mainly resulting from deforestation and forest degradation activities (GoK, 2017a). These emissions from mangroves are large, especially when compared to terrestrial ecosystems (GoK, 2017b). Although mangroves occupy only 3% of the forests in Kenya, the total volume of carbon they store is substantial. Globally, C emissions due to land-use change have been estimated to range from 90 to 450 million MgCO2 yr–1 over a global mangrove area of 13.8–15.2 million ha; which translates to 6.55–29.61 MgCO2 ha–1 yr–1 (Murray, 2012; Pendleton et al., 2012).
Economic Costs of Avoided Mangrove Deforestation
It would be unrealistic to think of stopping mangrove exploitation in Kenya, and by extension in other developing countries where mangroves are exploited for wood and non-wood resources. This conclusion is based on the fact that mangroves are lifelines for millions of coastal communities around the world (Dahdouh-Guebas et al., 2020). In Kenya, for instance, at least 70% of the wood requirement of adjacent communities is met by mangrove forests (GoK, 2017a). This is in addition to the people that frequently depend on mangrove areas for fisheries products, traditional medicine as well as leisure (Bosire et al., 2012; Hamza et al., 2020). Preserving mangroves may provide relatively low-cost opportunities to mitigate CO2 emissions; at the same time conserving biodiversity and supporting community livelihood. Payments for ecosystem services (PES) schemes such as Reduced Emissions from avoided Deforestation and forest Degradation (or REDD+) is a potential revenue stream for compensating those involved in mangrove conservation activities. Assuming an offset price of US$10/MgCO2, the estimated cost of avoided emission in Lamu mangroves alone is US$ 308,401 year–1; plus, other co-benefits such as fishery functions and shoreline protection.
Conclusion
There are 35,678 ha of mangroves in Lamu; representing 62% of total mangrove coverage in the country. These forests are threatened by both natural and anthropogenic factors. The present work analyzed status and conditions of mangroves in Lamu county; as well as determining the economics of emission reductions from avoided deforestation. The loss of mangrove in Lamu over the 1990–2019 period contributed to CO2 emissions to the atmosphere amounting to 30,840.1 Mg CO2e yr–1. Assuming an offset price of US$10/Mg CO2 the estimated cost of avoided emission in Lamu is US$308401 yr–1. Although reducing carbon emissions from avoided mangrove deforestation is only one of many benefits from mangroves, their conservation may be warranted by that basis alone. Sustainable mangrove management brings other co-benefits such as biodiversity conservation, shoreline protection, and benefits to fisheries (Bosire et al., 2012; GoK, 2017a), Considering that loss of mangrove may trigger the release of CO2 to the tune of up to three times that of terrestrial forests (Pendleton et al., 2012), efforts through incentivizing their conservation against competing land uses need to be enhanced. Mainstreaming mangroves and associated blue carbon ecosystems into the development and climate change agenda could accelerate Kenya’s achievements of Sustainable Development Goals (SDGs) and the Paris Agreement. Kenya pioneered a carbon incentive scheme involving mangroves forests. Dubbed Mikoko Pamoja, this was perhaps the world’s first community-led mangrove conservation and restoration project funded by carbon credits (Kairo et al., 2009). Revenue generated from the sale of these carbon credits are used to support local development projects as well mangrove reforestation activities. Mikoko Pamoja provides an excellent example of a “triple win” situation in Kenya, with benefits to climate, community and biodiversity conservation (Windham-Myers et al., 2018; Kairo et al., 2019).
Data Availability Statement
The raw data supporting the conclusions of this article will be made available by the authors, without undue reservation.
Author Contributions
AM, MM, and FM helped in conceptualization, methodology, validation, formal analysis, data collection, writing–original draft preparation, and writing–review and editing. JK was involved in conceptualization, methodology, validation, formal analysis, data collection, original–draft preparation, review and editing, visualization, supervision, project administration, and helped in funding acquisition. All authors contributed to the article and approved the submitted version.
Funding
This study was funded by the WWF-Germany and WWF-US, contract number BMZ 68776 granted to KMFRI by WWF-Kenya. Additional financial support was through Pew Charitable Trust’s Contract: 00032875 and The Nature Conservancy’ (TNC)’s Contract No. F104765 – KMFRI MANGROVE-09122019 both awarded to JK. Participation of AM in the project was through Mikoko Project “Conservation and resilience of the mangrove forest of Kenya,” a project funded by the French Ministry of Europe and Foreign Affairs, coordinated by the Kenya Forest Service (KFS), French National Research Institute for Sustainable Development (IRD), and the Center for International Cooperation in Agricultural Research for Development (CIRAD)”.
Conflict of Interest
The authors declare that the research was conducted in the absence of any commercial or financial relationships that could be construed as a potential conflict of interest.
Publisher’s Note
All claims expressed in this article are solely those of the authors and do not necessarily represent those of their affiliated organizations, or those of the publisher, the editors and the reviewers. Any product that may be evaluated in this article, or claim that may be made by its manufacturer, is not guaranteed or endorsed by the publisher.
Acknowledgments
The authors recognize the technical support offered during fieldwork by the following: Judith Okello, Joseph Lang’at, Lilian Mwihaki, Amina Hamza, all of KMFRI; as well as teams from KFS, TNC, and WWF- Kenya.
References
Abuodha, P. A. W., and Kairo, J. G. (2001). Human-induced stresses on mangrove swamps along the Kenyan coast. Hydrobiologia 458, 255–265.
Adame, M. F., Santini, N. S., Torres-Talamante, O., and Rogers, K. (2021). Mangrove sinkholes (cenotes) of the Yucatan Peninsula, a global hotspot of carbon sequestration. Biol. Lett. 17:20210037.
Ajonina, G. N., Kairo, J., Grimsditch, G., Sembres, T., Chuyong, G., and Diyouke, E. (2014). Assessment of Mangrove Carbon Stocks in Cameroon, Gabon, the Republic of Congo (RoC) and the Democratic Republic of Congo (DRC) including their Potential for Reducing Emissions From Deforestation and Forest Degradation (REDD+). In The Land/Ocean interactions in the Coastal Zone of West and Central Africa. Cham: Springer, 177–189.
Alavaisha, E., and Mangora, M. M. (2016). Carbon stocks in the small Estuarine mangroves of Geza and Mtimbwani, Tanga, Tanzania. Int. J. Forest. Res. 2016:2068283. doi: 10.1155/2016/2068283
Alemayehu, F., Richard, O., James, K. M., and Wasonga, O. (2014). Assessment of mangrove covers change and biomass in Mida creek, Kenya. Open J. Forest. 04, 398–413. doi: 10.4236/ojf.2014.44045
Alongi, D. M. (2012). Carbon sequestration in mangrove forests. Carbon Manage. 3, 313–322. doi: 10.4155/cmt.12.20
Alongi, D. M. (2014). Carbon cycling and storage in mangrove forests. Annu. Rev. Mar. Sci. 6, 195–219. doi: 10.1146/annurev-marine-010213-135020
Alongi, D. M. (2020). Global significance of mangrove blue carbon in climate change mitigation. Science 2:57. doi: 10.3390/sci2030057
Andreetta, A., Fusi, M., Cameldi, I., Cimò, F., Carnicelli, S., and Cannicci, S. (2014). Mangrove carbon sink. Do burrowing crabs contribute to sediment carbon storage? Evidence from a Kenyan mangrove system. J. Sea Res. 85, 524–533. doi: 10.1016/j.seares.2013.08.010
ASCLME (2012). National Marine Ecosystem Diagnostic Analysis. Kenya. Contribution to the Agulhas and Somali Current Large Marine Ecosystems Project (supported by UNDP With GEF Grant Financing). Available online at: https://wedocs.unep.org/bitstream/handle/20.500.11822/25890/Kenya_MEDA.pdf?sequence=1&isAllowed=y
Barbier, E. B., Hacker, S. D., Kennedy, C., Koch, E. W., Stier, A. C., and Silliman, B. R. (2011). The value of estuarine and coastal ecosystem services. Ecol. Monogr. 81, 169–193.
Benson, L., Glass, L., Jones, T. G., Ravaoarinorotsihoarana, L., and Rakotomahazo, C. (2017). Mangrove carbon stocks and ecosystem cover dynamics in southwest Madagascar and the implications for local management. Forests 8:190. doi: 10.3390/f8060190
Bhomia, R. K., Kauffman, J. B., and McFadden, T. N. (2016). Ecosystem carbon stocks of mangrove forests along the Pacific and Caribbean coasts of Honduras. Wetlands Ecol. Manage. 24, 187–201. doi: 10.1007/s11273-016-9483-1
Bishop, Y. M. M., Fienberg, S. E., and Holland, P. W. (1977). Book review: discrete multivariate analysis: theory and practice. Appl. Psychol. Meas.t 1, 297–306. doi: 10.1177/014662167700100218
Bosire, J. O., Maina, J., Kairo, J. G., Bandeira, S., Magori, C., Ralison, H., et al. (2012). Vulnerability of mangroves in the WIO region to climate change. VLIZ Special Publ. 57:41.
Bosire, J., Mangora, M., Bandeira, S., Rajkaran, A., Ratsimbazafy, R., Appadoo, C., et al. (2016). Mangroves of the Western Indian Ocean: Status and Management. Zanzibar Town: WIOMSA.
Boxem, H. W., De Meester, T., and Smalin, E. M. (1987). Soils of the Kilifi Area. Reconnaissance Soil Survey Report No. R11. Nairobi: Kenya Soil Survey.
Bundotich, G., Karachi, M., Fondo, E., and Kairo, J. G. (2009). Structural inventory of mangrove forests in Ngomeni. Adv. Coast. Ecol. 20, 111–121.
Camberlin, P. (2018). Climate of Eastern Africa. Climate Science. Oxford: Oxford Research Encyclopedias.
Caswell, P. V. (1953). The Geology of the Mombasa Kwale Area. Coral Survey. Kenya Report No. 24. Nairobi: Ministry of Environment and Natural Resources, 65.
Chmura, G. L., Anisfeld, S. C., Cahoon, D. R., and Lynch, J. C. (2003). Global carbon sequestration in tidal, saline wetland soils. Glob. Biogeochem. Cycles 17:1111.
Corlett, R. T. (1986). The mangrove understory: some additional observations. J. Trop. Ecol. 2, 93–94. doi: 10.1017/s0266467400000651
County Government of Lamu (2018). Lamu County Integrated Development Plan 2018-2022. Lamu: Department of Finance, Strategy & Economic Planning.
Dahdouh-Guebas, F., Ajonina, G. N., Amir, A. A., Andradi-Brown, D. A., Aziz, I., and Balke, T. (2020). Public perceptions of mangrove forests matter for their conservation. Front. Mar. Sci. 7:901.
Dahdouh-Guebas, F., Mathenge, C., Kairo, J. G., and Koedam, N. (2000). Utilization of mangrove wood products around Mida Creek (Kenya) amongst subsistence and commercial users. Econ. Bot. 54, 513–527. doi: 10.1007/BF02866549
Dahdouh-Guebas, F., Van Pottelbergh, I., Kairo, J. G., Cannicci, S., and Koedam, N. (2004). Human-impacted mangroves in Gazi (Kenya): predicting future vegetation based on retrospective remote sensing, social surveys, and tree distribution. Mar. Ecol. Prog. Ser. 272, 77–92. doi: 10.3354/meps272077
Díaz, B. M., and Blackburn, G. A. (2003). Remote sensing of mangrove biophysical properties: evidence from a laboratory simulation of the possible effects of background variation on spectral vegetation indices. Int. J. Remote Sens. 24, 53–73. doi: 10.1080/01431160305012
Donato, D. C., Kauffman, J. B., Murdiyarso, D., Kurnianto, S., Stidham, M., and Kanninen, M. (2011). mangroves among the most carbon-rich forests in the tropics. Nat. Geosci. 4, 293–297. doi: 10.1038/ngeo1123
Duke, N. C., Meynecke, J. O., Dittmann, S., Ellison, A. M., Anger, K., Berger, U., et al. (2007). A world without mangroves? Science 317, 41–42.
Gallo, N. D., Victor, D. G., and Levin, L. A. (2017). Ocean commitments under the Paris Agreement. Nat. Clim. Change 7, 833–838. doi: 10.1038/nclimate3422
Gillerot, L., Vlaminck, E., De Ryck, D. J. R., Mwasaru, D. M., Beeckman, H., and Koedam, N. (2018). Inter- and intraspecific variation in mangrove carbon fraction and wood specific gravity in Gazi Bay, Kenya. Ecosphere 9:e02306. doi: 10.1002/ecs2.2306
Giri, C. (2016). Observation and monitoring of mangrove forests using remote sensing: opportunities and challenges. Remote Sens. 8:783. doi: 10.3390/rs8090783
Giri, C., Ochieng, E., Tieszen, L. L., Zhu, Z., Singh, A., Loveland, T., et al. (2011). Status and distribution of mangrove forests of the world using earth observation satellite data. Glob. Ecol. Biogeogr. 20, 154–159. doi: 10.1111/j.1466-8238.2010.00584.x
GoK (2017b). Nationally Determined Contribution (NDC) Sector Analysis Report: The Evidence Base for Updating Kenya’s National Climate Change Action Plan. Nairobi: Ministry of Environment and Natural Resources.
GoK (2020). Kenya’s Update Nationally Determined Contributions (NDCs). Nairobi: Ministry of Environment and Forestry.
Hamilton, S. E., and Casey, D. (2016). Creation of a high spatio−temporal resolution global database of continuous mangrove forest cover for the 21st century (CGMFC−21). Glob. Ecol. Biogeogr. 25, 729–738. doi: 10.1111/geb.12449
Hamilton, S. E., and Friess, D. A. (2018). Global carbon stocks and potential emissions due to mangrove deforestation from 2000 to 2012. Nat. Clim. Change 8, 240–244. doi: 10.1038/s41558-018-0090-4
Hamza, A. J., Esteves, L. S., Cvitanovic, M., and Kairo, J. (2020). Past and present utilization of mangrove resources in Eastern Africa and drivers of change. J. Coast. Res. 95, 39–44. doi: 10.2112/si95-008.1
Herr, D., and Landis, E. (2016). Coastal Blue Carbon Ecosystems. Opportunities for Nationally Determined Contributions Policy Brief. Gland: IUCN. Available online at: https://www.mangrovealliance.org/wp-content/uploads/2017/08/BC-NDCs_FINAL.pdf
Howard, J., Hoyt, S., Isensee, K., Telszewski, M., and Pidgeon, E. (2014). Coastal Blue Carbon: Methods for Assessing Carbon Stocks and Emissions Factors in Mangroves, Tidal Salt Marshes, and Seagrass Meadows. Arlington, VA: Conservation International, Intergovernmental Oceanographic Commission of UNESCO, International Union for Conservation of Nature, 99–107.
Huxham, M., Dencer-Brown, A., Diele, K., Kathiresan, K., Nagelkerken, I., and Wanjiru, C. (2017). Mangroves and People: Local Ecosystem Services in a Changing Climate. In Mangrove Ecosystems: A Global Biogeographic Perspective. Cham: Springer, 245–274.
Huxham, M., Kimani, E., and Augley, J. (2004). Mangrove fish: a comparison of community structure between forested and cleared habitats. Estuarine Coast. Shelf Sci. 60, 637–647. doi: 10.1016/j.ecss.2004.03.003
IPBES (2019). Global Assessment report on Biodiversity and Ecosystem Services of the Intergovernmental Science-Policy Platform on Biodiversity and Ecosystem Services, eds E. S. Brondizio, J. Settele, S. Díaz, and H. T. Ngo (Bonn: IPBES secretariat), 1148. doi: 10.5281/zenodo.3831673
IPCC (2006). “2006 IPCC guidelines for national greenhouse gas inventories,” in Intergovernmental Panel on Climate Change (IPCC), National Greenhouse Gas Inventories Programme, Institute for Global Environmental Strategies, Hayama, Kanagawa, Japan, eds H. S. Eggleston, L. Buendia, K. Miwa, T. Ngara, and K. Tanabe (Geneva: IPCC).
IPCC (2014). 2013 Supplement to the 2006 IPCC Guidelines for National Greenhouse Gas Inventory, Wetlands, Vol. 2. Geneva: IPCC.
IPCC (2019). Climate Change and Land: An IPCC Special Report on Climate Change, Desertification, land Degradation, Sustainable Land Management, food security, and Greenhouse Gas Fluxes in Terrestrial Ecosystems, eds Shukla, et al. (Geneva: IPCC.).
Jakovac, C. C., Latawiec, A. E., Lacerda, E., Lucas, I. L., Korys, K. A., Iribarrem, A., et al. (2020). Costs and carbon benefits of mangrove conservation and restoration: a global analysis. Ecol. Econ. 176:106758. doi: 10.1016/j.ecolecon.2020.106758
Janzen, D. H. (1985). Mangroves: where’s the understory? J. Trop. Ecol. 1, 89–92. doi: 10.1017/s0266467400000122
Jones, T. G., Ratsimba, H. R., Ravaoarinorotsihoarana, L., Glass, L., Benson, L., Teoh, M., et al. (2015). The dynamics, ecological variability and estimated carbon stocks of mangroves in Mahajamba Bay, Madagascar. J. Mar. Sci. Eng. 3, 793–820. doi: 10.3390/jmse3030793
Kairo, J. G. (2001). Ecology and Restoration of Mangrove Systems in Kenya. Ph.D. dissertation. Brussels: Laboratory of Plant Sciences and Nature Management, Vrije Universiteit Brussel.
Kairo, J. G., and Bosire, J. O. (2016). “Emerging and crosscutting issues,” in Mangroves of the Western Indian Ocean: Status and Management, eds J. O. Bosire, M. M. Mangora, S. Bandeira, A. Rajkaran, R. Ratsimbazafy, C. Appadoo, et al. (Zanzibar: WIOMSA), 136–151.
Kairo, J. G., Bosire, J., Lang’at, J., Kirui, B., and Koedam, N. (2009). Allometry and biomass distribution in replanted mangrove plantations at Gazi Bay, Kenya. Aquatic Conserv. Mar. Freshw. Ecosyst. 19, 63–69. doi: 10.1002/aqc.1046
Kairo, J. G., Dahdouh-Guebas, F., Bosire, J., and Koedam, N. (2001). Restoration and management of mangrove systems - a lesson for and from the East African region. South Afr. J. Bot. 67, 383–389. doi: 10.1016/S0254-6299(15)31153-4
Kairo, J. G., Kivyatu, B., and Koedam, N. (2002b). Application of remote sensing and GIS in the management of mangrove forests within and adjacent to Kiunga Marine Protected Area, Lamu, Kenya. Environ. Dev. Sustain. 4, 153–166. doi: 10.1023/A:1020890711588
Kairo, J. G., Dahdouh-Guebas, F., Gwada, P. O., Ochieng, C., and Koedam, N. (2002a). Regeneration status of mangrove forests in Mida Creek, Kenya: a compromised or secured future? Ambio 31, 562–568. doi: 10.1579/0044-7447-31.7.562
Kairo, J. G., Hamza, A. J., and Wanjiru, C. (2019). “Mikoko Pamoja – a demonstrably effective community-based blue carbon project in Kenya,” in A Blue Carbon Primer: The State of Coastal Wetland Carbon Science, Practice, and Policy, Windham-Myers, eds L. W.-M. Lisamarie, C. Stephen, and G. T. Tiffany (Boca Raton, FL: CRC Press).
Kamal, M., and Johansen, K. (2017). “Explicit area-based accuracy assessment for mangrove tree crown delineation using geographic object-based image analysis (GEOBIA),” in Proceedings of the SPIE Earth Resources and Environmental Remote Sensing/GIS Applications VIII, Vol. 10428 (Bellingham, WA: International Society for Optics and Photonics), 104280I.
Kamau, J., Ngisiange, N., Ochola, O., Kilionzi, J., Kimeli, A., Mahongo, S. B., et al. (2020). Factors influencing spatial patterns in primary productivity in Kenyan territorial waters. Western Indian Ocean J. Mar. Sci. 1, 9–18. doi: 10.4314/wiojms.si2020.1.2
Kauffman, J. B., Adame, M. F., Arifanti, V. B., Schile-Beers, L. M., Bernardino, A. F., Bhomia, R. K., et al. (2020). Total ecosystem carbon stocks of mangroves across broad global environmental and physical gradients. Ecol. Monogr. 90, 1–18. doi: 10.1002/ecm.1405
Kauffman, J. B., and Donato, D. C. (2012). Protocols for the Measurement, Monitoring and Reporting of Structure, Biomass, and Carbon Stocks in Mangrove Forests. Bogor: Cifor, 50.
Kauffman, J. B., Bernardino, A. F., Ferreira, T. O., Giovannoni, L. R., De Gomes, L. E. O., Romero, D. J., et al. (2018). Carbon stocks of mangroves and salt marshes of the Amazon region, Brazil. Biol. Lett. 14:20180208. doi: 10.1098/rsbl.2018.0208
Kauffman, J. B., Heider, C., Cole, T. G., Dwire, K. A., and Donato, D. C. (2011). Ecosystem carbon stocks of micronesian mangrove forests. Wetlands 31, 343–352. doi: 10.1007/s13157-011-0148-9
Kauffman, J. B., Heider, C., Norfolk, J., and Payton, F. (2014). Carbon stocks of intact mangroves and carbon emissions arising from their conversion in the Dominican Republic. Ecol. Appl. 24, 518–527. doi: 10.1890/13-0640.1
Kirui, K. B., Kairo, J. G., Bosire, J., Viergever, K. M., Rudra, S., Huxham, M., et al. (2013). Mapping of mangrove forest land cover change along the Kenya coastline using Landsat imagery. Ocean Coastal Manage. 83, 19–24. doi: 10.1016/j.ocecoaman.2011.12.004
Komiyama, A., Ong, J. E., and Poungparn, S. (2008). Allometry, biomass, and productivity of mangrove forests: a review. Aquatic Bot. 89, 128–137. doi: 10.1016/j.aquabot.2007.12.006
Komiyama, A., Poungparn, S., and Kato, S. (2005). Common allometric equations for estimating the tree weight of mangroves. J. Trop. Ecol. 21, 471–477. doi: 10.1017/S0266467405002476
Kridiborworn, P., Chidthaisong, A., Yuttitham, M., and Tripetchkul, S. (2012). Carbon sequestration by mangrove forest planted specifically for charcoal production in Yeesarn, Samut Songkram. J. Sustain. Energy Environ. 3, 87–92.
Lang’at, J. K. S., Kairo, J. G., Mencuccini, M., Bouillon, S., Skov, M. W., Waldron, S., et al. (2014). Rapid losses of surface elevation following tree girdling and cutting in tropical mangroves. PLoS One 9:e107868. doi: 10.1371/journal.pone.0107868
Lang’at, J. K., and Kairo, J. G. (2008). Conservation and Management of Mangrove Forests in Kenya. Mombasa: Mangrove Reforestation Program, Kenya Marine and Fisheries Research Institute.
Lee, S. Y. (1998). Ecological role of grapsid crabs in mangrove ecosystems: a review. Mar. Freshw. Res. 49, 335–343. doi: 10.1071/mf97179
Lee, S. Y., Primavera, J. H., Dahdouh-Guebas, F., Mckee, K., Bosire, J. O., Cannicci, S., et al. (2014). Ecological role and services of tropical mangrove ecosystems: a reassessment. Glob. Ecol. Biogeogr. 23, 726–743. doi: 10.1111/geb.12155
Lopez, O. (2021). Ocean-Based Climate Solutions in Nationally Determined Contributions. Washington, DC: Ocean Conservancy.
Macamo, C. D. C. F., Adams, J. B., Bandeira, S. O., Mabilana, H. A., and António, V. M. (2018). Spatial dynamics and structure of human disturbed mangrove forests in contrasting coastal communities in Eastern Africa. Wetlands 38, 509–523. doi: 10.1007/s13157-018-0996-7
Mohamed, M. O. S., Neukermans, G., Kairo, J. G., Dahdouh-Guebas, F., and Koedam, N. (2009). Mangrove forests in a peri-urban setting: the case of Mombasa (Kenya). Wetlands Ecol. Manage. 17, 243–255. doi: 10.1007/s11273-008-9104-8
Mungai, F., Kairo, J., Mironga, J., Kirui, B., Mangora, M., and Koedam, N. (2019). Mangrove cover and cover change analysis in the transboundary area of Kenya and Tanzania during 1986–2016. J. Indian Ocean Region 15, 157–176. doi: 10.1080/19480881.2019.1613868
Murdiyarso, D., Purbopuspito, J., Kauffman, J. B., Warren, M. W., Sasmito, S. D., Donato, D. C., et al. (2015). The potential of Indonesian mangrove forests for global climate change mitigation. Nat. Clim. Change 5, 1089–1092. doi: 10.1038/nclimate2734
Murray, B. C. (2012). Economics: mangroves’ hidden value. Nat. Clim. Change 2, 773–774. doi: 10.1038/nclimate1729
Njana, M. A., Zahabu, E., and Malimbwi, R. E. (2018). Carbon stocks and productivity of mangrove forests in Tanzania. Southern Forests 80, 217–232. doi: 10.2989/20702620.2017.1334314
Okello, J. A., Schmitz, N., Kairo, J. G., Beeckman, H., and Koedam, N. (2013). Self-sustenance potential of peri-urban mangroves: a case of Mtwapa creek Kenya. J. Environ. Sci. Water Resour. 2, 277–289.
Owuor, M. A., Mulwa, R., Otieno, P., Icely, J., and Newton, A. (2019). Valuing mangrove biodiversity and ecosystem services: a deliberative choice experiment in Mida Creek, Kenya. Ecosyst. Serv. 40:101040. doi: 10.1016/j.ecoser.2019.101040
Pendleton, L., Donato, D. C., Murray, B. C., Crooks, S., Jenkins, W. A., Sifleet, S., et al. (2012). Estimating global “blue carbon” emissions from conversion and degradation of vegetated coastal ecosystems. PLoS One 7:e43542. doi: 10.1371/journal.pone.0043542
Polidoro, B. A., Carpenter, K. E., Collins, L., Duke, N. C., Ellison, A. M., Ellison, J. C., et al. (2010). The loss of species: mangrove extinction risk and geographic areas of global concern. PLoS One 5:e10095. doi: 10.1371/journal.pone.0010095
Radabaugh, K. R., Dontis, E. E., Chappel, A. R., Russo, C. E., and Moyer, R. P. (2021). Early indicators of stress in mangrove forests with altered hydrology in Tampa Bay, Florida, USA. Estuarine Coast. Shelf Sci. 254:107324. doi: 10.1016/j.ecss.2021.107324
Ramsey, E. W., and Jensen, J. R. (1996). Remote sensing of mangrove wetlands: relating canopy spectra to site-specific data. Photogram. Eng. Remote Sens. 62, 939–948.
Rasquinha, D. N., and Mishra, D. R. (2021). Impact of wood harvesting on mangrove forest structure, composition and biomass dynamics in India. Estuarine Coastal Shelf Sci. 248:106974. doi: 10.1016/j.ecss.2020.106974
Ren, H., Chen, H., Li, Z., and Han, W. (2010). Biomass accumulation and carbon storage of four different aged Sonneratia apetala plantations in Southern China. Plant Soil 327, 279–291. doi: 10.1007/s11104-009-0053-7
Rozainah, M. Z., Nazri, M. N., Sofawi, A. B., Hemati, Z., and Juliana, W. A. (2018). Estimation of carbon pool in soil, above and below ground vegetation at different types of mangrove forests in Peninsular Malaysia. Mar. Pollut. Bull. 137, 237–245. doi: 10.1016/j.marpolbul.2018.10.023
Siikamäki, J., Sanchirico, J. N., and Jardine, S. L. (2012). Global economic potential for reducing carbon dioxide emissions from mangrove loss. Proc. Natl. Acad. Sci. U.S.A. 109, 14369–14374. doi: 10.1073/pnas.1200519109
Sitoe, A. A., Mandlate, L. J. C., and Guedes, B. S. (2014). Biomass and carbon stocks of Sofala Bay mangrove forests. Forests 5, 1967–1981. doi: 10.3390/f5081967
Snedaker, S. C., and Lahmann, E. J. (1988). Mangrove understorey absence: a consequence of evolution? J. Trop. Ecol. 4, 311–314. doi: 10.1017/s0266467400002881
Stringer, C. E., Trettin, C. C., Zarnoch, S. J., and Tang, W. (2015). Carbon stocks of mangroves within the Zambezi River Delta, Mozambique. Forest Ecol. Manage. 354, 139–148. doi: 10.1016/j.foreco.2015.06.027
Taraska, G. (2018). Integrating Ocean and Climate Policy: A Next Step Forward in the Global Climate Effort. 2018. Washington, DC: Center for American Progress.
Thomas, N., Lucas, R., Bunting, P., Hardy, A., Rosenqvist, A., and Simard, M. (2017). Distribution and drivers of global mangrove forest change, 1996-2010. PLoS One 12:e0179302. doi: 10.1371/journal.pone.0179302
Thompson, B. S., Clubbe, C. P., Primavera, J. H., Curnick, D., and Koldewey, H. J. (2014). Locally assessing the economic viability of blue carbon: a case study from Panay Island, the Philippines. Ecosyst. Serv. 8, 128–140. doi: 10.1016/j.ecoser.2014.03.004
Twilley, R. R., Rovai, A. S., and Riul, P. (2018). Coastal morphology explains global blue carbon distributions. Front. Ecol. Environ. 16:503–508. doi: 10.1002/fee.1937
United Nations Environmental Programme [UNEP] (1998). Eastern Africa Atlas of Coastal Resources. UNEP Regional Reports and Studies No. 1. Nairobi: United Nations Environmental Programme, 114.
Keywords: total ecosystem carbon, emission levels, Paris agreement, mangrove cover change, Lamu, Kenya
Citation: Kairo J, Mbatha A, Murithi MM and Mungai F (2021) Total Ecosystem Carbon Stocks of Mangroves in Lamu, Kenya; and Their Potential Contributions to the Climate Change Agenda in the Country. Front. For. Glob. Change 4:709227. doi: 10.3389/ffgc.2021.709227
Received: 13 May 2021; Accepted: 20 September 2021;
Published: 21 October 2021.
Edited by:
Kerry Turner, University of East Anglia, United KingdomReviewed by:
Paula Soares, University of Lisbon, PortugalSilvia Ferrini, University of East Anglia, United Kingdom
Copyright © 2021 Kairo, Mbatha, Murithi and Mungai. This is an open-access article distributed under the terms of the Creative Commons Attribution License (CC BY). The use, distribution or reproduction in other forums is permitted, provided the original author(s) and the copyright owner(s) are credited and that the original publication in this journal is cited, in accordance with accepted academic practice. No use, distribution or reproduction is permitted which does not comply with these terms.
*Correspondence: James Kairo, Z2thaXJvQHlhaG9vLmNvbQ==