- 1Resource Analysis, Forest Resources and Management, Swiss Federal Institute for Forest, Snow and Landscape Research (WSL), Birmensdorf, Switzerland
- 2Forest Division, Federal Office for the Environment, Bern, Switzerland
Introduction: Among terrestrial ecosystems, forests represent large carbon stocks threatened by changing climatic conditions, deforestation, overexploitation, and forest degradation. Close to nature forestry may help forests to continue to acting as carbon sinks by promoting their resilience against disturbances. The EU decided to carry out carbon accounting of emissions and removals from managed forests under the Paris Agreement (PA) by using a projected Forest Reference Level (FRL) based on the continuation of recent management practices.
Methods: We developed four conceptual scenarios that could build the Swiss Forest Reference Level and performed simulations over 50 years using Swiss National Forest Inventory (NFI) data and the empirical forest model MASSIMO. To improve MASSIMO, we further developed a new tree species-specific model for small scale mortality that accounts for the Swiss NFI design. Then, using projected biomass and mortality from MASSIMO, carbon budgets of mineral soil, litter, and dead wood were estimated using the Yasso07 model.
Results: The U-shaped mortality model performed well (AUC 0.7). Small as well as large trees had the highest mortality probabilities, reflecting both young trees dying due to self-thinning and old trees from age, pests or abiotic influences. All scenarios matched their given harvesting and growing stock targets, whereby the share of broadleaves increased in all regions of Switzerland. This resulted in decreasing biomass growth, possibly due to a species shift from typically fast growing and more shade tolerant conifers to broadleaves. The CO2-balance of the conceptual scenarios ranged from 1.06 to −3.3 Mt CO2 a–1 under Increased Harvesting and Recent Management Practices (RMP), respectively. Rotation periods are shortened under Increased Harvesting, which is an important climate adaptive management strategy, but forests were predicted to become a net carbon source. In contrast, RMP resulted in similar harvesting amounts and forests as carbon sinks, as reported in the past. Further, the RMP scenario does not involve political assumptions and reflects the idea of the CMP approach used by the EU member states, which makes it comparable to other countries. Therefore, we propose the scenario RMP as a suitable and ideal candidate for the Swiss FRL.
Introduction
Among terrestrial ecosystems, peatlands and forests constitute the main carbon stocks. However, due to changing climatic conditions, peatlands are predicted to shift from a sink to a carbon source (Loisel et al., 2021). In addition, forests may become a source due to changing climate, deforestation, overexploitation and forest degradation (Friedlingstein et al., 2019). On the other hand, close to nature forestry and climate smart forestry may help forests to continue acting as carbon sinks by promoting their resilience against disturbances (Brang et al., 2014; Gusti et al., 2020). Further, forest management can make a significant contribution to reducing CO2-emissions. Specifically, fossil fuels can be substituted by wood (energetic substitution) and the use of long-lived harvested wood products (HWP) can be increased at the expense of more energy-intensive materials and construction methods (material substitution; Werner et al., 2010; Braun et al., 2016). However, substitution effects are currently not considered in the reporting and accounting of the Land Use, Land-Use Change and Forestry (LULUCF) sector under the United Nations Framework Convention on Climate Change (UNFCCC) and associated agreements such as the Kyoto Protocol.
The complexity of LULUCF sector has been reason for discussions on accounting rules (e.g., Grassi et al., 2018). In the first commitment period of the Kyoto Protocol 2008–2012, the carbon accounting procedure for forests under Forest management was based on a voluntary gross-net approach taking country-specific caps into consideration (UNFCCC, 1998). A gross-net or absolute approach in this context means that countries do not include gains and losses of carbon from the LULUCF sector in their base year emissions (gross) but account for net emissions and removals from LULUCF during the commitment period (net). With this gross-net accounting approach, most managed European forests acted as carbon sinks due to legacy effects of past management and environmental changes (Nabuurs et al., 2003; Bellassen et al., 2010). In the second commitment period (CP2) of the Kyoto Protocol 2013–2020, accounting for Forest management – including harvested wood products (HWP) – became mandatory and the gross-net accounting changed to a relative or net-net accounting: only the difference between the recent forest balance and a (projected) Forest Management Reference Level (FMRL) is accounted for. The purpose of this “relative accounting with a reference level” in CP2 is to ensure that emissions or removals that occur purely from age-legacy-effects or because of effects of historical management or past natural disturbances are not accounted for Iversen et al. (2014).
With the Paris Agreement (PA), accounting rules became more flexible and in order to achieve “a balance between anthropogenic emissions by sources and removals by sinks of greenhouse gases in the second half of this century” individual countries could design their specific accounting rules based on a set of existing IPCC reporting documents (UNFCCC, 2015; Nabuurs et al., 2018). However, the PA urges its Parties to preserve and enhance existing carbon sinks including forests, integrating the “LULUCF” sector for the first time to international climate mitigation targets (Forsell et al., 2019). Moreover, given the concerns about the need to factoring out the effects of historic forest development as a result of past forest management, the UNFCCC laid out principles on situations where countries must “account” for the impact of mitigation actions (Art. 4.13 of the Paris Agreement). In response to these principles, the EU compiled rules and conditions to account for carbon credits under the LULUCF-regulation 2018/8411. Therefore, EU member states have to account their emissions and removals of carbon dioxide in managed forest land against a projected forest reference level (FRL), similar to the accounting of forest management under CP2 (Grassi et al., 2018). This FRL, however, must be defined more strictly and “transparently and in consideration of the business-as-usual procedures” based on the period 2000–2009 (Forsell et al., 2018). This should avoid the inclusion of increased carbon sink effects (considered as credits) due to age development of trees and forest that can be expected without any additional efforts from the forest sector (Nabuurs et al., 2018). The main difference between the FMRL in CP2 of the Kyoto Protocol and the FRL is that the FMRL projections of forest development included assumptions on future effects of policies agreed on until 2009 such as the development of harvesting levels, whereas in the FRL projections assumptions of future impacts of policies are not considered (Nabuurs et al., 2018). Since the responsibility for defining FRLs lies with the EU member states (Valade et al., 2017), member states have developed their own systems to simulate recent management practices, based on national forest inventories (NFI) and forest development models (e.g., Barreiro et al., 2016; Pilli et al., 2017; Korosuo et al., 2021).
Similar to EU member states, Switzerland decided in 2015 to account for managed forest on a net-net basis by using a reference level that includes living and dead biomass and HWP, while it excludes large-scale disturbances in forest land (see Switzerland’s “intended nationally determined contribution” INDC)2. To do so, Switzerland uses data from its NFI in combination with the empirical forest development model MASSIMO (Stadelmann et al., 2019; Stadelmann, 2020) to project living biomass. Then, using MASSIMO biomass predictions, the C-cycling model Yasso07 (Tuomi et al., 2009, 2011) is applied for estimating the carbon budget of mineral soil, litter and dead wood (Didion et al., 2014). The Swiss FRL is finally calculated as the mean annual projected CO2-balance of managed forest land over the period 2021–2030 by using a specific scenario.
The aim of this study is to assess how different forest management scenarios, built on the existing guidelines under UNFCCC and IPCC methodologies and consistent with the Swiss forest policy, may affect the projected mean CO2-balances 2021–2030. To do so, we developed four conceptual scenarios and a new model for small scale (i.e., single-tree) mortality. In this article, we addressed the following questions: (i) How can we formulate small scale mortality in an unbiased manner? (ii) How suitable are the four conceptual forest management scenarios developed based on recent forest data, forest management and forest policy objectives to represent the Swiss FRL? and (iii) How do the scenarios differ in their effect on the combined estimate of the accounting of the Swiss CO2 balance under the Paris Agreement?
Materials and Methods
Swiss National Forest Inventory Data
Forests cover roughly one third of Switzerland’s area, with higher shares in the Jura and on the Southern Alps but lower shares on the Plateau and in the Alps (Abegg et al., 2020; Figure 1)3. Since 1983, the Swiss NFI has repeatedly measured state and change of these forests using a systematic 1.4 × 1.4 km grid of more than 6,000 permanent plots (Fischer and Traub, 2019). Permanent plots consist of two concentric circles of 200 and 500 m2, in which trees are sampled if their diameter at breast height (1.3 m above the ground—DBH) exceeds the caliper threshold of 12 cm and 36 cm, respectively. In this study, we used data of the inventory campaigns NFI2 (1993/95), NFI3 (2004/06) and NFI4 (2009/17) for the fitting of the mortality model. However, for modeling the CO2-budgets, we only used data up to NFI3 (i.e., until 2006) in order to be in line with the KP-supplement [“not use data later than 2009”; (IPCC, 2014)] and to align with the specific requirements of the EU LULUCF-regulation 2018/841 [reference period 2000–2009; (Forsell et al., 2018). However, within this 10 years reference period, only one full inventory campaign, namely NFI3 (2004–2006) was conducted in Switzerland. Thus, to derive changes in forest resources we needed to use additional data of the second inventory campaign NFI2 (1993–1995)]. For fitting of the empirical process models and for the RMP scenario, we further included data of the first inventory in order to achieve more robust models.
MASSIMO
All simulations of the living biomass presented in this study were performed with MASSIMO (Stadelmann et al., 2019; Stadelmann, 2020), implemented in Java SE 8. MASSIMO is individual-tree growth simulator that runs on the grid of the Swiss NFI allowing the projection of growth, ingrowth (i.e., tree recruitment), mortality and management of trees in 10-year time steps, representatively. The basal area increment (BAI) model is specific to tree species groups and depends on stand basal area, basal area of trees larger than the subject tree, site index, elevation, stand age, and dominant DBH. That growth model further accounts for a growth release (i.e., increased growth) following management interventions (Thürig et al., 2005a). Models on ingrowth and non-growth above the caliper thresholds of 12 cm and 36 cm, respectively, are climate sensitive (Zell et al., 2019). For the simulation of ingrowth and in Yasso07, we applied a relatively warm and dry A1B scenario (CH2011, 2011; Remund et al., 2016). MASSIMO differentiates between large-scale wind-throw induced mortality and individual-tree mortality. Since the last MASSIMO release (Stadelmann et al., 2019; Stadelmann, 2020), the individual tree mortality module has been updated to better account for stand conditions and tree species (cf. section “Mortality Module”). Forest management treatments (thinning and shelterwood cutting) can be adjusted to approximate either user-defined growing stock or harvesting targets at the level of 14 economic regions (for details cf. Stadelmann et al., 2019). While thinning is applied in all forests (except forest reserves), shelterwood cutting is only applied in even-aged high elevation forests outside of the protection forest areas (i.e., forests protecting against rockfall and avalanches). In protection forests, thinning retains stand basal area in a range defined by given recommendations (Dorren et al., 2015).
All processes were parameterized using data from the Swiss NFI (Abegg et al., 2020) ensuring accurate representation of forest dynamics in Switzerland. Model simulations are initialized with country-specific data for comparability between model results and observations and between simulated scenarios. MASSIMO and its processes have been presented beforehand (Thürig et al., 2005a, b, 2019; Stadelmann et al., 2019; Stadelmann, 2020). Since tree recruitment strongly influences forest development, a new climate sensitive ingrowth model was implemented to make MASSIMO able to cover large gradients of stand and climate conditions (Zell et al., 2019). However, the sub-models for growth and mortality are not climate-sensitive yet, as they are still in development. MASSIMO has been applied at regional (Temperli et al., 2017) and national scale (Stadelmann et al., 2016; Temperli et al., 2020).
Mortality Module
Two inventory intervals were used to model tree-mortality: NFI2 to NFI3 and NFI3 to NFI4. For each interval, tree- and stand-level information were extracted either at the first inventory (NFI2 for the NFI2-NFI3 interval and NFI3 for the NFI3-NFI4 interval), the second inventory (NFI3 for the NFI2-NFI3 interval; NFI4 for the NFI3-NFI4 interval), or both. For each inventory interval, only trees that were alive at the first inventory were included in the analyses. The status of trees at the second inventory was defined as dead or alive. The inclusion probability of each tree was extracted at the first inventory. It is defined based on the DBH of the tree as well as on whether the plot could be entirely measured. Tree species were classified into 12 groups based on the most represented tree species or genera in Switzerland: spruce (Picea abies), fir (Abies alba), larch (Larix sp.), pine (Pinus sylvestris, Pinus nigra, Pinus strobus, Pinus mugo arborea), stone pine (Pinus cembra), beech (Fagus sylvatica), oak (Quercus sp.), ash (Fraxinus sp.), maple (Acer sp.), and chestnut (Castanea sativa); others were classified as other conifers or other broadleaves. The diameter at breast height (DBH, 1.3 m above ground) was extracted from the first inventory. Finally, stand basal area (in m2 ha–1) was used as a plot-level variable of the first inventory. The normalized number of vegetation years between each inventory (number of vegetation years minus 10) and the production region of each plot were also noted.
The tree mortality analyses were performed using R version 3.5.1 (R Core Team, 2018). Models were built using the survey R package (Lumley, 2020) that is able to account for complex survey samples. The svydesign function was used to specify the data design including plot number as the cluster id and trees’ inclusion probabilities as sampling weights. Binomial regression models were then fitted with the svyglm function using tree status at the second inventory (dead/alive) as the response variable and DBH (second-degree polynomial term), species group, production region, stand basal area, and normalized vegetation years as explanatory variables. An interaction between DBH and species group was also added to account for size differences between species. The goodness of fit of the model could be assessed using AUC.
Yasso07
Yasso07 (Tuomi et al., 2009, 2011) is a model of C cycling in mineral soil, litter, and dead wood. The model was developed for general application with low parameter and input requirements. For estimating stocks of organic C in mineral soil up to a depth of ca. 100 cm and the temporal dynamics of the C stocks, Yasso07 requires information on C inputs from dead organic matter components (i.e., litter and dead wood) and climate (temperature, temperature amplitude, and precipitation). Decomposition of the different components of C inputs is modeled based on their chemical composition, size of woody parts and climate (Tuomi et al., 2009, 2011). Decomposition rates of C that is either insoluble (N), soluble in ethanol (E), in water (W), or in acid (A), and flow rates of C between these four compounds, to a more stable humus compartment (H) and out of the soil were derived from a global data set (Tuomi et al., 2009, 2011). Parameters defining C decomposition rates and C cycling between carbon compartments are obtained probabilistically using Markov Chain Monte Carlo sampling and are fitted based on data from litterbag studies (Tuomi et al., 2009) and woody litter decomposition experiments (Tuomi et al., 2011). The approach allows calculating the maximum a posteriori point estimate and confidence sets for the model parameter vector, which consists of a unique combination of 24 correlated parameters. At the time of this study, three independent parameter sets, i.e., vectors of 24 parameters have been developed and published (Tuomi et al., 2009, 2011; Rantakari et al., 2012).
Didion et al. (2014) examined the validity of the Yasso07 model in Swiss forests based on data on observed decomposition of (i) foliage and fine root litter from sites along a climatic and elevation gradient and (ii) of dead trees from plots of the Swiss National Forest Inventory. The study analyzed, among other, the accuracy of Yasso07 for reproducing observed C decomposition in litter and dead wood in Swiss forests. The best agreement of Yasso07 results with observed C decomposition was obtained with the parameter presented in Rantakari et al. (2012), which was obtained by restricting the global dataset on dead wood, litter, and soil decomposition set (Tuomi et al., 2009, 2011) to European sites. The Yasso07 model is used to obtain estimates of C stocks in dead wood and litter and of C stock changes in dead wood, litter, and mineral soil for reporting in Switzerland’s annual National Inventory Reports to the UNFCCC. Austria, Finland and Norway also apply the Yasso07 model for their reports to the UNFCCC. Yasso07 simulations for this study are based on the Yasso07 Fortran source code of the Yasso07 release 1.0.14.
Testing Four Conceptual Scenarios for the Swiss Reference Level for Forest Land
Together with representatives of the Swiss Federal Office for the Environment (FOEN) we developed four conceptual scenarios to be evaluated for establishing a reference level for Forest Land and ran them from NFI3 (2004/06) for 5 time-steps of 10 years (Table 1). Thus, the simulations started with the NFI3 conditions with a growing stock of 360 m3 ha–1 which corresponds to a living biomass stock of 121 TC ha–1 (data per NFI production region given in Supplementary Table 2). The “Forest Policy (FPOL)” scenario, however, ran for 30 years only as scenario assumptions could not be met in longer simulations. Thereby, we ran each scenario with 30 replicates to account for stochastic differences between each run. Except for the “Recent Management Practices (RMP)” scenario, we simulated all scenarios with standard management algorithms and a scenario approximation routine to fulfill given growing stock or harvesting, respectively, per economic region (for details on the scenario adaption routine, cf. Stadelmann et al., 2019). The RMP scenario did not use this routine nor the standard management algorithms, as it has been developed to be self-approximating via its single-tree selection model (Stadelmann et al., in prep).
As default settings in MASSIMO, we defined heavy storms to occur every 15 years as it has been observed in the past (Pfister, 1999; Thürig et al., 2005b) and 80% of storm damaged timber was salvage logged to avoid bark beetle outbreaks (cf. Stadelmann et al., 2013). However, in the RMP scenario we shortened the storm interval to 10 years as observed between NFI1 and NFI3. This is in line with Usbeck et al. (2010) that found increasing storm damages over time. Further, we activated protection forest management as presented in Stadelmann (2020) and respected existing forest reserves.
The amount of mortality simulated with the new mortality module agrees well with the empirically measured mortality (Abegg et al., 2020)5. However, to meet given targets of the background mortality defined for Swiss forest under management under the Kyoto Protocol (for details on the estimation of background mortality cf. FOEN, 2016), the empirical mortality had to be increased slightly for FPOL, “Increased Harvesting (IH),” and “Retain Growing Stock (RGS)” by additionally simulating harvesting losses (i.e., we attributed part of the harvested tree compartments to remain in the forest as harvesting losses). The background mortality, estimated as 16.39%, is defined as a share on all losses (harvesting of wood + mortality) between the years 1990 and 2009 (cf. FOEN, 2016) that include two heavy storm events – Vivian/Wiebke in 1990 and Lothar in 1999 – both followed by large scale bark beetle outbreaks. While the storm damages by Lothar were treated as outlier, the mean of all other damages build the background mortality of 16.39%, which must be reached as input in Yasso07. Besides simulated mortality, stumps and roots of harvested trees entered this pool. But additional deadwood was needed to reach the given target for all scenarios but RMP. By assumptions on harvesting losses, we could ensure that exactly the predefined amount of background mortality is used as input in the model Yasso07. Harvesting losses differed for conifers and broadleaves as well as for stem, thick (>7 cm in diameter) and small (<7 cm in diameter) branches (Table 1).
The initial state of the dead wood, litter, and mineral soil pools in 2006 (Supplementary Table 3) was taken from Yasso07 simulations for reporting in the Swiss Greenhouse Gas Inventory report 2020 (NIR; FOEN, 2020) with annual litter and dead wood data derived from measured NFI data. The plausibility of simulated C stocks and stock changes in these pools is thus continuously evaluated (see section 3.1 and 3.2 in Didion, 2019 for the data submitted to NIR 2020). In this study, the simulated development of those pools is driven by annual input of dead wood (incl. stemwood and large branchwood that are not removed from the plot and all coarse-root and stump biomass) and litter (incl. fine woody litter from small branchwood and non-woody litter like foliage and fine roots) derived from simulated MASSIMO results for each NFI plot following the methodology presented in Didion and Zell (2019). The Yasso07 simulations until 2006 were driven by temperature and precipitation data extracted from the gridded data prepared by MeteoSwiss (2016a, b). From 2007, we used simulations from MASSIMO to derive data on growth and mortality, as well as litter and dead wood remaining in the forest as inputs for the Yasso07 simulation. To account for model uncertainty, the Yasso07 simulations were based on 10 randomly selected simulated initial states for 2006 (out of 100 replicates prepared for the reporting to the NIR, cf. Didion and Thürig, 2019) and 10 randomly selected MASSIMO replicates to obtain the time series of dead wood and litter production starting 2007.
Projected CO2-Balance
In the context of climate reporting, Switzerland announced in its “Nationally determined contribution” (NDC)6 to account for its forest sector on a net-net basis, i.e., relative accounting against a FRL. The FRL is a projected mean CO2- balance of all forest pools for the compliance period 2021–2030 based on a conceptual scenario. The conceptual scenarios for the Swiss FRL were evaluated by calculating the total CO2-balances per scenario (Table 2). To this end, we averaged simulation results of the decades 2017–2026 and 2027–2036 by weighted means for the compliance period 2021–2030 for all modeled carbon pools of the Swiss forest sector. The CO2-balance is the sum of the carbon stock changes in living biomass, soil carbon, litter, dead wood and “harvested wood products (HWP)” and emissions from controlled fires. HWP calculations followed the methodology described in FOEN (2021a) and based on the methodology internationally agreed on and applied during the second commitment period of the Kyoto Protocol (IPCC, 2014).
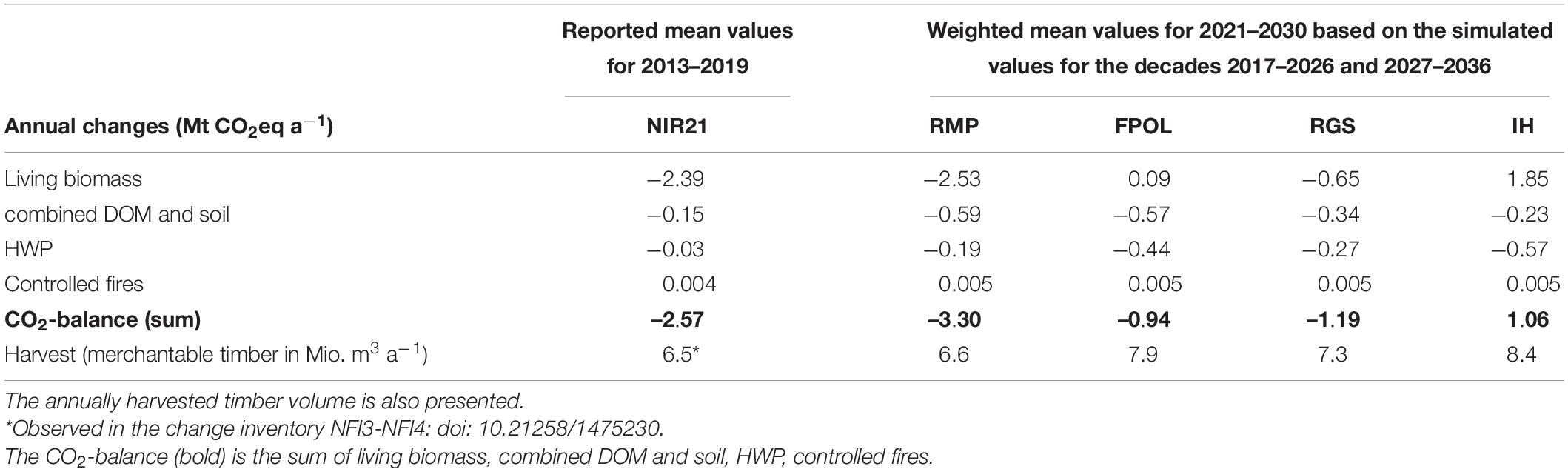
Table 2. Mean CO2-balances of the Swiss Forest sector [i.e., managed forest land including Harvested Wood Products (HWP)] as reported in the Swiss National Inventory Report 2021 for the period 2013–2019 (NIR21) and as calculated for 2021–2030 from the projections of the conceptual scenarios RMP, FPOL, RGS, and IH.
Results
Mortality Model
The mortality model fitted a positive relationship between tree mortality probability and stand basal area, used as a proxy for competition. The relationship to DBH had a U-shaped form for most species groups, where small and large trees had a larger mortality probability than average-sized trees (Figure 2). The model performed well with an AUC of 0.70. A table on model estimates and predictions is given in the Supplementary Table 1.
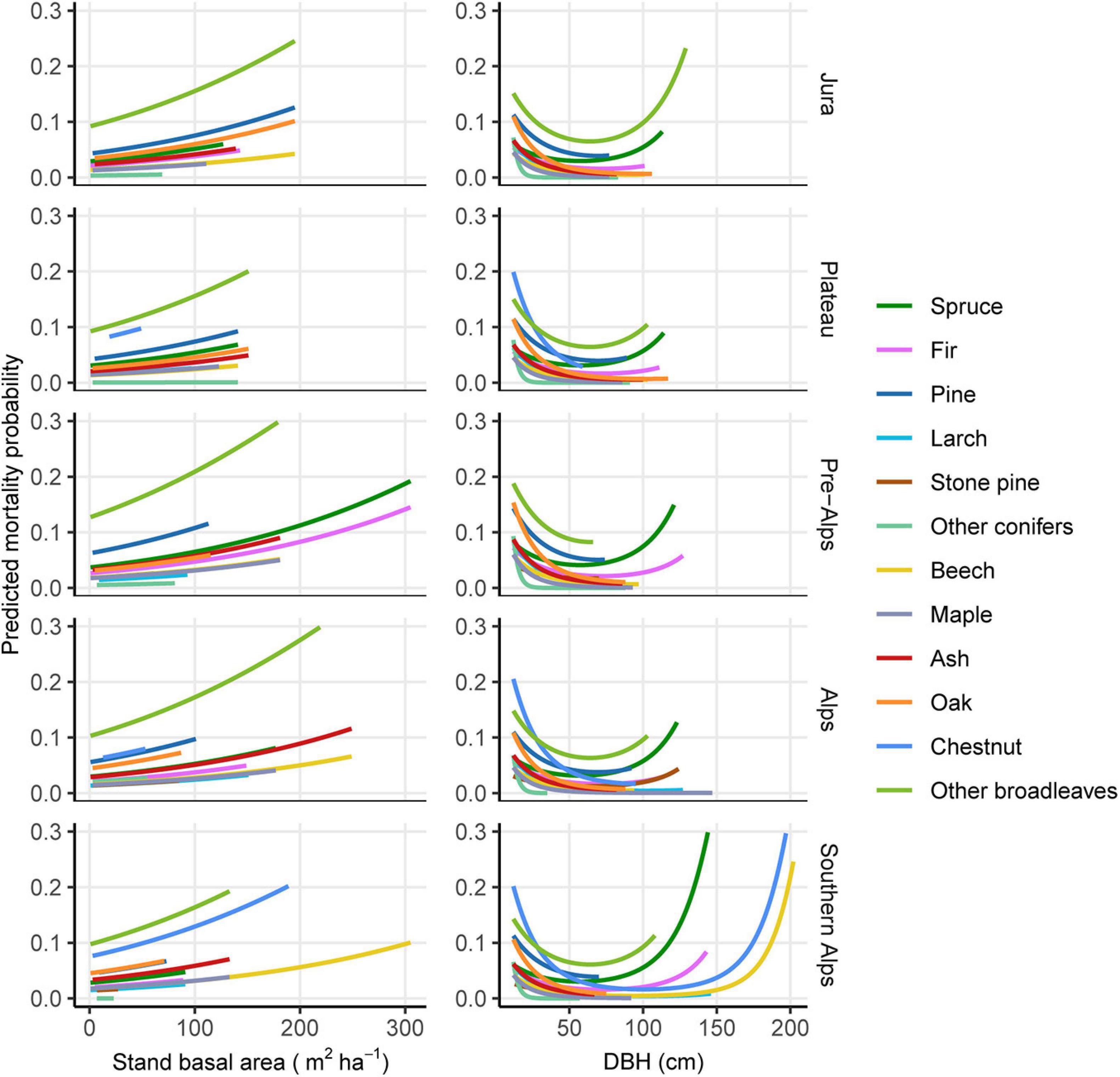
Figure 2. Predicted mortality probabilities per tree species group along gradients of stand basal area and DBH per production region of Switzerland. Other variables were kept constant at their mean species-specific values.
Scenario Simulations
All scenarios (but RMP that has no defined target) matched their defined harvesting and growing stock targets (Supplementary Figure 1), whereby the shares of broadleaves increased in all production regions and for all scenarios. Consequently, we found the same pattern for living biomass (Figure 3). RMP resulted in increasing biomass for broadleaves, but also for conifers in the Alps and the Southern Alps, where harvesting is lower than growth. In these regions, FPOL followed the same pattern as RMP, while the other regions – that have better harvesting infrastructure – did not. On the Plateau, where harvesting is similar to IH, biomass and growing stock strongly decreased. With IH, biomass and growing stock also decreased in all other regions except for the southern Alps that currently has relatively low growing stocks (Supplementary Figure 1), due to legacy effects for past coppice forest management (i.e., chestnut coppice forests). IH is the only scenario that showed similar trends for conifers and broadleaves in all regions. While RGS resulted in constant growing stocks, biomass slightly increased with mounting shares of broadleaves but the biomass of conifers slightly decreased.
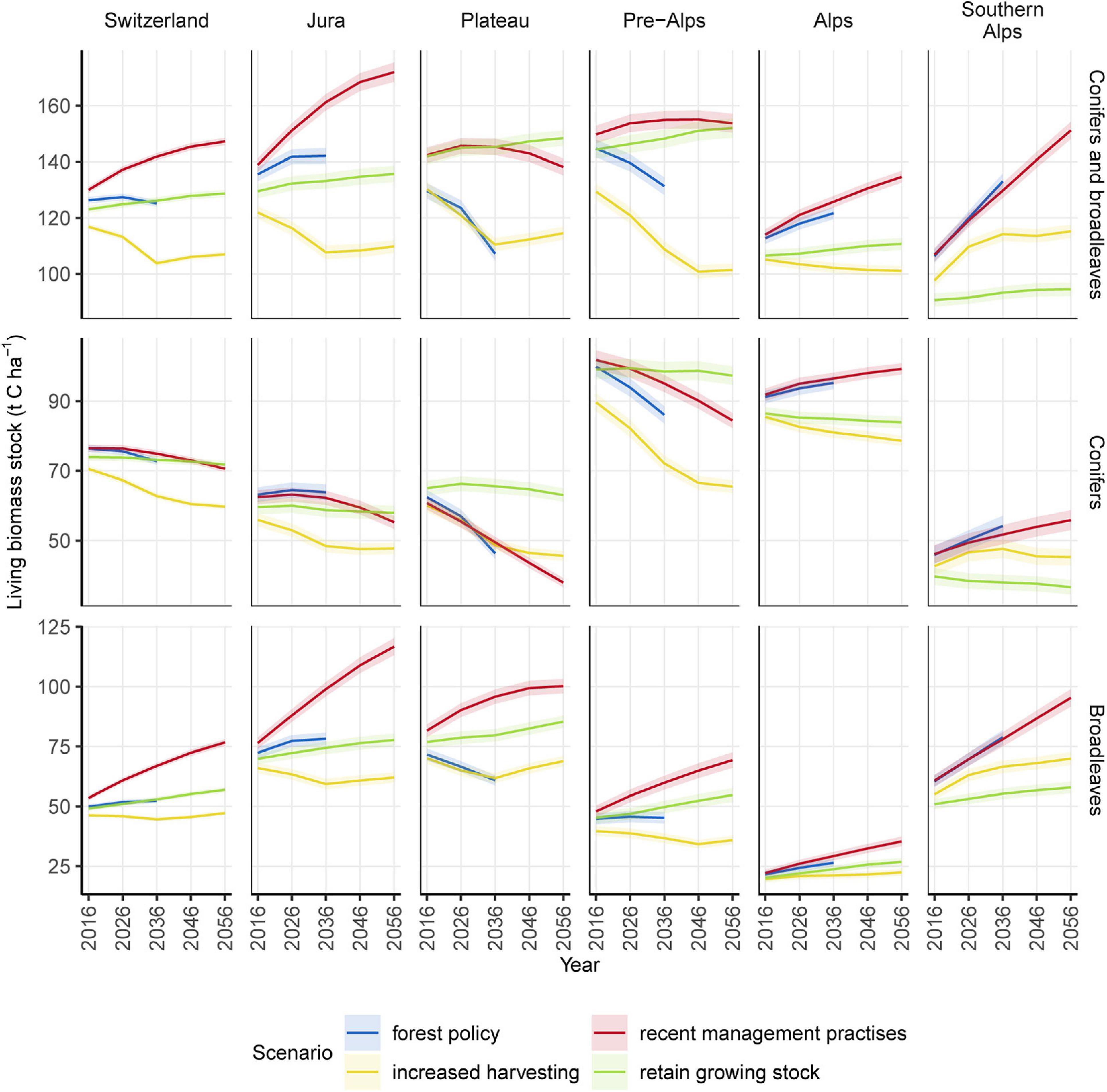
Figure 3. Switzerland’s living biomass stock (above and below ground) for all Switzerland and its production regions. Results of conifers (middle row) and broadleaves (lower row) sum up to the total biomass stock in the upper row. Simulation results include mean (thick line) and Standard Error of 30 replicates (shaded area). The lines between each end of a time-step (e.g., between 2026 and 2036) are linearly interpolated.
Gross growth of biomass decreased for all scenarios and production regions for total timber, as well as conifers and partly also for broadleaves (Figure 4A and Supplementary Figure 2), with decreases being most pronounced in IH that resulted in lowest biomass stocks. Similarly, total losses of biomass were highest for IH (Figure 4B). These trends were related to the given scenario targets. For RMP, however, we found a steep increase in losses of broadleaves biomass, mainly in Jura and on the Plateau (cf. Supplementary Figure 3).
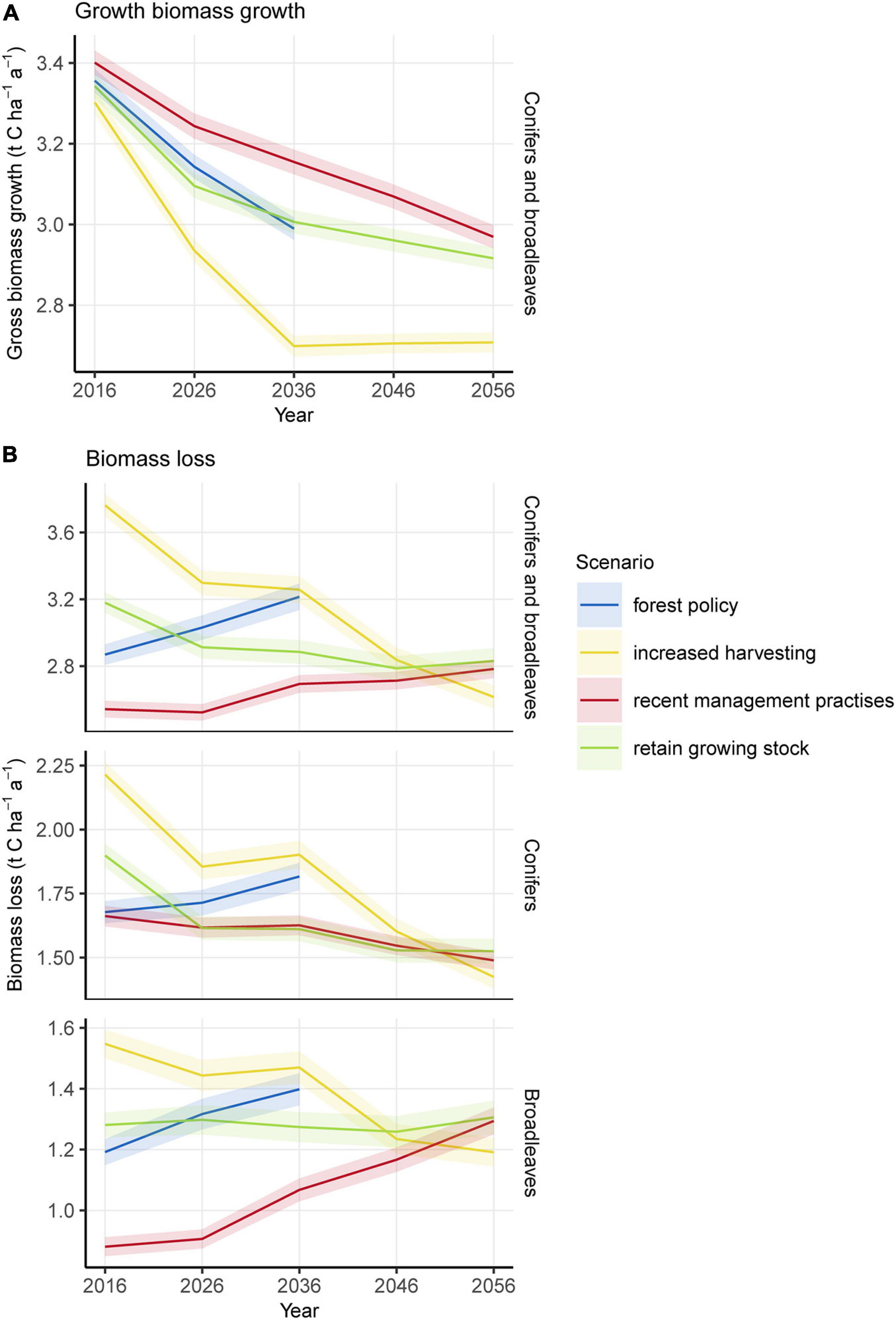
Figure 4. (A) Gross biomass growth including ingrowth (above and below ground). (B) Losses (harvesting and mortality) in biomass for conifers and broadleaves. More details are presented in Supplementary Figures 2, 3. Simulations results include mean (thick line) and Standard Error of 30 replicates (shaded). The lines between each end of a time-step (e.g., between 2026 and 2036) are linearly interpolated.
Yasso07 Simulations
To assess the relative impact of the different scenarios on the changes of carbon stocks in DOM (dead organic matter, i.e., the sum of litter, dead wood) and mineral soil pools, simulated C stocks over time are presented relative to the RMP scenario (Figure 5). Because the initial state of the DOM and soil C pools were based on litter and dead wood input data derived from NFI measurements (cf. section “Testing Four Conceptual Scenarios for the Swiss Reference Level for Forest Land”), the change in inputs for the Yasso07 model from observed NFI data to predicted MASSIMO data was primarily responsible for the dynamics of DOM and soil carbon stocks in the first decades of the modeling. Thereby, the application of a relative level of mortality (16.39% background mortality) led to more pronounced absolute mortality rates, especially for scenarios with increased harvesting rates compared to RMP. But mortality of stemwood also increased for RMP, since we simulated shorter time intervals (i.e., 10 instead of 15 years) between heavy storm events. Carbon stocks in DOM and soil changed inversely to living biomass stocks, as 16.39% of the total losses (harvest and mortality of biomass) entered the pool of litter and dead wood, and, in the longer term, mineral soil. At the national level, living biomass continuously increased under RMP, while this increase was less pronounced for FPOL and RGS and decreased for IH until 2036 (Figure 3). This contributed to the increase in DOM and soil carbon stocks for all scenarios compared to RMP (Figure 5) for the first 15–30 years, with regional differences. DOM and soil carbon stocks then decreased compared to RMP for the remaining simulation period. The only region with a different trend from the national level was the Plateau, where the increase of carbon stocks in these pools compared to RMP was higher for all scenarios, but decreased between 2036 and 2046 for IH, ending with similar DOM and soil carbon stocks at the end of the simulation period. For the other regions, non-living carbon developed similarly to the national scale.
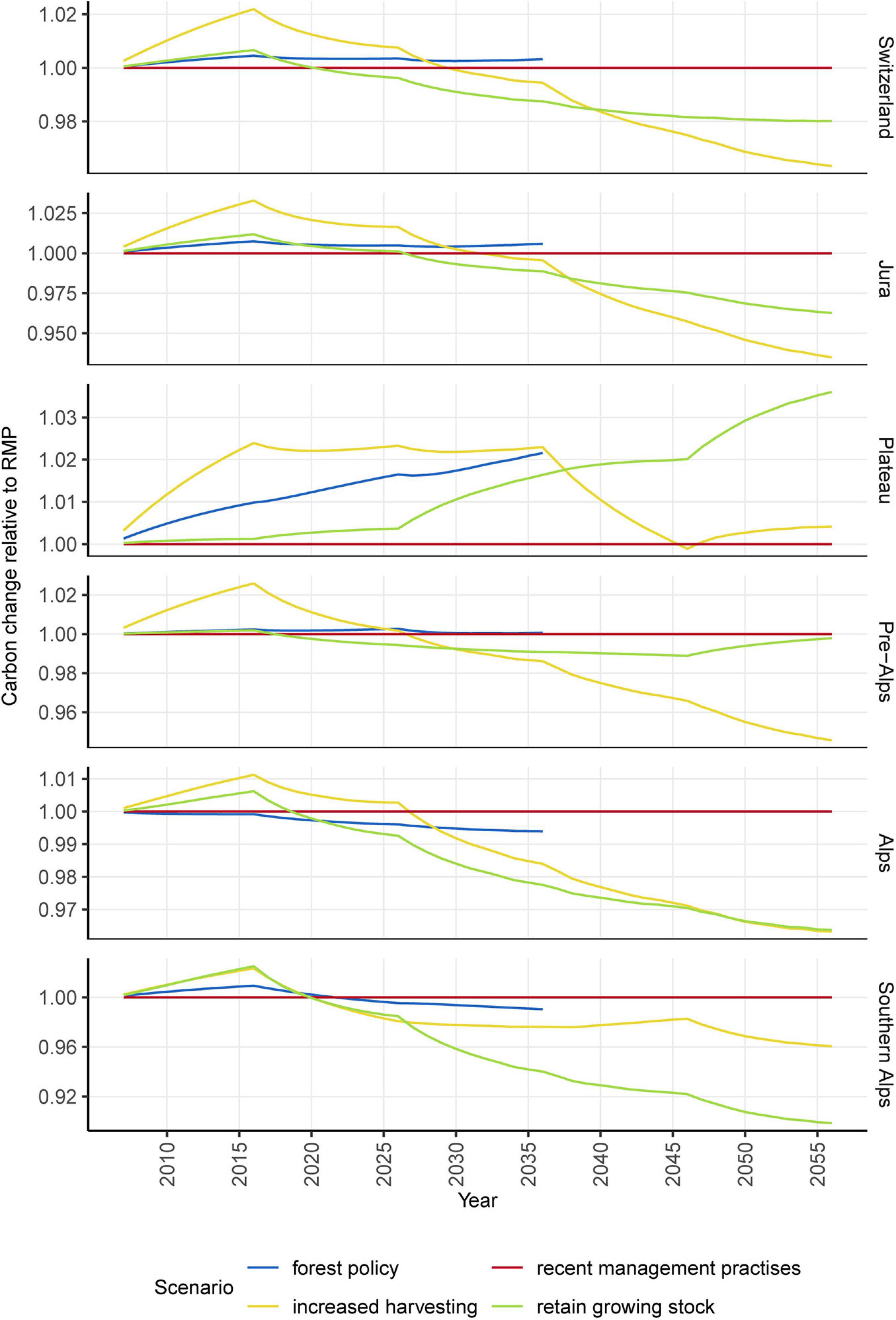
Figure 5. Carbon stock relative to the recent management practices (RMP) scenario. Values above 1 indicate higher combined dead organic matter (DOM) and soil carbon stocks than in RMP and vice versa.
Mean CO2-Budget 2021–2030
Similarly to the observed CO2-budget of managed forest land during the period 2013–2019 reported by the Switzerland’s Greenhouse Gas Inventory (NIR 2021: FOEN, 2021b; Thürig et al., 2021), the scenarios RMP, RGS, and FPOL resulted in a CO2-sink for the next compliance period 2021–2030, while IH resulted in a CO2-source. The highest sink occurred with RMP, resulting in harvesting amounts similar to those reported over the period 2013–2019 (NIR21). Harvesting rates obtained with the scenarios FPOL, RGS, and IH are remarkably higher than those of RMP. With increasing harvesting rates, the total CO2-balances declined or even turned into a net source (IH).
Discussion
The new mortality model performed reasonably well, not only when assessing the goodness-of-fit of the model directly, but also within the modeling framework MASSIMO where growth and losses were within the range of NFI observations (Thürig et al., 2019). Under RMP, where future management (harvesting amounts) remains within the range of past and recent management, we did not need to simulate additional harvesting losses to reach a given background level of mortality as defined by FOEN (2016) in accordance with the accounting rules given in the second commitment period of the Kyoto Protocol. This background level of mortality includes mortality of single trees and large scale disturbances (see section “Testing Four Conceptual Scenarios for the Swiss Reference Level for Forest Land”) that have been observed between 1990 and 2010. Since the background mortality is defined in relation to the total losses (harvest + mortality) any scenario with increased harvesting inevitably results in increased absolute mortality. Thus, to reach the 16.39% background mortality predefined by FOEN (2016), we needed to simulate harvesting losses for the scenarios IH, FPOL, and RGS that account for stumps, thick and small branches. In RMP, where harvesting is similar to recent observations, the empiric mortality is well predicted. Thus, the new species-specific mortality model allows minimization of additional artificial mortality (i.e., harvesting losses). Moreover, it plausibly reflected the effects of site and stand conditions. Increasing basal area, for example, led to a well-known density-dependent mortality (Larson et al., 2015). The U-shaped mortality response to tree size reflects both young trees dying due to self-thinning (e.g., Westoby, 1984) as well as old trees dying for various reasons (age, pests, or abiotic influences). However, regional or national increases in harvesting intensity, as represented by the IH scenario, reduces stand density and the number of large trees, resulting in turn in decreasing mortality rates (Stadelmann et al., 2016; Temperli et al., 2017).
In all scenarios, the up to 2056 simulated share of broadleaved trees increased, while the gross growth of volume and biomass decreased. This can result from a shift in tree species from fast growing and typically more shade-tolerant conifers to broadleaves, a pattern that has been observed since the first NFI, and which is expected to increase due to climate warming. Broadleaves typically need more stand space, resulting in lower potential stem densities. Further, all scenarios except RMP resulted in an increase of harvesting that led in the short-term to a rapid decrease in growth. The species shift from conifers to broadleaves was most pronounced in RMP, with the consequence that harvesting and therefore biomass losses (i.e., harvest and mortality) of broadleaves increased steeply. However, if harvesting costs decrease and the demand for wood fuel increases (c.f. Thees et al., 2020), the removal of thick branches – especially from broadleaves – will need to be intensified. This would result in lower harvesting losses that would, when summed up with background mortality, not reach the predefined 16.39% threshold. Consequently, conceptual scenarios could be further developed to include a varying demand in wood fuel resulting in varying harvesting losses and varying background mortality.
Our scenarios strongly influenced the amount of harvesting losses needed to reach the predefined background mortality and volume of branchwood that entered the Yasso07-pools for carbon change simulations. Indeed, scenarios other than RMP were based on higher harvesting rates, which resulted in an increase in the combined DOM and soil carbon stock. Intensifying management led to a decrease in stem density compared to RMP and therewith, litter volumes from living trees decreased. Since climate is an important driver of processes such as forest regeneration, growth and mortality, as well as decomposition of DOM, it would be preferable to use several climate scenarios to obtain a corresponding range of carbon sequestration trajectories. However, since so far, only the regeneration module in MASSIMO is climate sensitive, we decided to apply only one climate scenario for the simulation of ingrowth and Yasso07, assuming that growth and mortality are not affected. Compared to current climate, the use of this scenario likely resulted in an increased C sink of the DOM and soil pools due to more optimal conditions (i.e., warmer temperatures with still sufficient precipitation in the majority of Swiss forests) lead to increased tree growth but also to a faster decomposition of the DOM. Similar forest growth models applied in Europe are not climate sensitive (Barreiro et al., 2016; Vauhkonen et al., 2019) with the assumption that on relatively short time scales of 50 years management effects are more important than climate ones (Perin et al., 2021). For future improvements of MASSIMO, however, the effects of climate change on forest development should be accounted for, as they are becoming increasingly apparent (e.g., Schelhaas et al., 2015; Reyer et al., 2017; Seidl et al., 2017; Rohner et al., 2021). Consequently, including climate-sensitivity into increment and mortality models will be inevitable to allow for analyses of scenarios assuming changing climatic conditions (Matala et al., 2005; Kindermann, 2010; Rohner et al., 2018; Schelhaas et al., 2018).
Swiss forests are predicted to become a net source of carbon under scenarios with fast reductions in growing stock in the short-term (e.g., FPOL and IH), while they may lead to increasing gross growth in the long-term (Stadelmann et al., 2016). These growing stock reductions are obtained by shortening the rotation periods, which is a climate adaptive management strategy (Brang et al., 2014). Additionally, increased harvesting allows for material and energetic substitution effects that contribute in mitigating climate change (Mehr et al., 2018; Churkina et al., 2020; Jonsson et al., 2021). However, according to reporting requirements under the international climate agreements such as the Kyoto Protocol and the Paris Agreement, substitution effects of the forestry sector cannot be directly accounted for, although they indirectly are through the resulting lower emissions in other sectors such as energy. Nevertheless, these substitution effects have a considerable impact on the CO2-balance of forest management including harvested wood products (Werner et al., 2010). Substitution effects might even overcome harvesting-induced carbon emissions in some cases (Braun et al., 2016).
The four presented scenarios were evaluated for their plausibility to represent the Swiss FRL for the accounting of emissions and removals on managed forest land under the Paris Agreement. While RGS is a good base scenario to assess changes in growth and losses, it provides no path-breaking information, nor a direct reference to a realistic future development of Swiss forests. Thus, it does not suit as Swiss reference level. While RMP is fully empiric (i.e., the single-tree harvesting model depends on observed management only), IH, RGS and FPOL are based on transparent and reproducible management assumptions such as thinning frequency and intensity and rotation periods. FPOL includes harvesting targets of the Swiss Forest Policy, which envisages a distinct increase in harvesting rates in order to obtain stable, resilient, multifunctional and climate-adapted forests in the future. However, in this scenario a strong priority setting of harvesting costs was implemented, i.e., forests with very high harvesting costs were not harvested. Therefore, we simulated harvesting mainly on the Plateau in the Jura and at the pre-Alps where harvesting infrastructure is better than in the Alps and the Southern Alps, resulting in a decrease of available timber that prevented simulating the scenario for more than 30 years. In fact, current harvesting rates are significantly lower than simulated in FPOL and without a substantial increase in timber prices, this scenario is not realistic in the near future. This limitation also emerges for the IH scenario, where harvesting is even higher than in FPOL but more evenly distributed across Switzerland. Consequently, IH and FPOL both include policy assumptions that lead to an overestimation of short-term harvesting. These policies could seem arbitrary and unrealistic, and overestimate carbon emissions in the reference level. Consequently, choosing these scenarios to be used for a Swiss reference level would clearly result in unwarranted credits and thus jeopardize the environmental integrity of these scenarios.
Recent Management Practices, eventually, is the most impartial scenario as it is empirically based on country-specific NFI observations. We consider RMP as the most environmentally sound scenario, since only CO2-effects directly related to changes in management practices are accounted for. Additionally, the EU has chosen to base its accounting of managed forest land on a FRL relying on a scenario called “continuation of forest management practices (as documented in the period 2000–2009) (CMP),” on which RMP is conceptually based. Choosing RMP therefore makes the Swiss results comparable to those of neighboring countries. In December 2020, Switzerland submitted its carbon-emission reduction pledge under the PA (cf. Switzerland’s full NDC)7 and announced to account for managed forest land on a net-net basis, i.e., relative accounting against an FRL with an underlying scenario excluding assumptions on the impact of future forest policies. Since the Swiss RMP is empirically driven from NFI data covering the period 1983–2006, this approach is very similar (but not identical, since years outside 2000–2009 are used) to the approach applied by the EU. To conclude, the RMP scenario for Switzerland is reliably based on observed data, comparable but not identical to the EU approach and results in plausible estimates, making it the ideal candidate for the Swiss FRL.
Data Availability Statement
The original contributions presented in the study are included in the article/Supplementary Material, further inquiries can be directed to the corresponding author/s.
Author Contributions
GS developed the conceptual scenarios to be tested for the Swiss Reference Level in collaboration with NR and ET, ran all simulations with MASSIMO and Yasso07, and led the writing of the manuscript with contributions by all other authors. JP developed the new mortality module together with GS and ET. NR calculated the CO2-balances. MD supported all work related to Yasso07. All authors contributed to the article and approved the submitted version.
Funding
This study was performed with the support of Swiss Federal Office for the Environment.
Conflict of Interest
The authors declare that the research was conducted in the absence of any commercial or financial relationships that could be construed as a potential conflict of interest.
Publisher’s Note
All claims expressed in this article are solely those of the authors and do not necessarily represent those of their affiliated organizations, or those of the publisher, the editors and the reviewers. Any product that may be evaluated in this article, or claim that may be made by its manufacturer, is not guaranteed or endorsed by the publisher.
Acknowledgments
We would like to thank Barbara Schneider for her efforts in programming MASSIMO, as well as Sandro Bishof and Rolf Meile for their support on underlying data models and software engineering. We would also like to thank Beat Rihm (Meteotest) for the calculation of HWP and acknowledge the Swiss NFI team for completing fieldwork and data management.
Supplementary Material
The Supplementary Material for this article can be found online at: https://www.frontiersin.org/articles/10.3389/ffgc.2021.685574/full#supplementary-material
Footnotes
- ^ Avl. Online at: https://ec.europa.eu/clima/policies/forests/lulucf_en
- ^ Avl. Online at: https://tinyurl.com/SwissINDC2015
- ^ NFI result table No. 877654 avl. online at: https://doi.org/10.21258/1687553
- ^ http://code.google.com/p/yasso07ui/
- ^ NFI result tables No. 890644 (NFI3 avl. Online at: https://doi.org/10.21258/1698041) and 890645 (NFI4 avl. Online at: https://doi.org/10.21258/1717224)
- ^ Avl. Online at: https://tinyurl.com/SwissNDC
- ^ Avl. Online at: https://tinyurl.com/SwissFullNDC2020
References
Abegg, M., Brändli, U.-B., Cioldi, F., Fischer, C., Herold, A., Meile, R., et al. (2020). Swiss National Forest Inventory. Birmensdorf: Swiss Federal Institute for Forest, Snow and Landscape Research WSL.
Barreiro, S., Schelhaas, M.-J., Kändler, G., Antón-Fernández, C., Colin, A., Bontemps, J.-D., et al. (2016). Overview of methods and tools for evaluating future woody biomass availability in European countries. Ann. For. Sci. 73, 823–837. doi: 10.1007/s13595-016-0564-3
Bellassen, V., Le Maire, G., Dhôte, J. F., Ciais, P., and Viovy, N. (2010). Modelling forest management within a global vegetation model—Part 1: model structure and general behaviour. Ecol. Modell. 221, 2458–2474. doi: 10.1016/j.ecolmodel.2010.07.008
Brang, P., Spathelf, P., Larsen, J. B., Bauhus, J., Boncčìna, A., Chauvin, C., et al. (2014). Suitability of close-to-nature silviculture for adapting temperate European forests to climate change. Forestry 87, 492–503. doi: 10.1093/forestry/cpu018
Braun, M., Fritz, D., Weiss, P., Braschel, N., Büchsenmeister, R., Freudenschuß, A., et al. (2016). A holistic assessment of greenhouse gas dynamics from forests to the effects of wood products use in Austria. Carbon Manage. 7, 271–283. doi: 10.1080/17583004.2016.1230990
Churkina, G., Organschi, A., Reyer, C. P. O., Ruff, A., Vinke, K., Liu, Z., et al. (2020). Buildings as a global carbon sink. Nat. Sustain. 3, 269–276. doi: 10.1038/s41893-019-0462-4
Didion, M. (2019). Data on soil Carbon Stock Change, Carbon Stock and Stock Change in Surface Litter and in Coarse Dead Wood Prepared for the Swiss NIR 2020 (GHGI 1990–2018). Birmensdorf: Swiss Federal Institute for Forest, Snow and Landscape Research WSL.
Didion, M., Frey, B., Rogiers, N., and Thürig, E. (2014). Validating tree litter decomposition in the Yasso07 carbon model. Ecol. Model. 291, 58–68. doi: 10.1016/j.ecolmodel.2014.07.028
Didion, M., and Thürig, E. (2019). Data on soil carbon stock change, carbon stock and stock change in surface litter and in coarse dead wood prepared for the Swiss NIR 2020 (GHGI 1990–2018)”. Birmensdorf: Swiss Federal Institute for Forest, Snow and Landscape Research WSL.
Didion, M., and Zell, J. (2019). “Model of carbon cycling in dead organic matter and Soil (Yasso07),” in Swiss National Forest Inventory – Methods and Models of the Fourth Assessment. Managing Forest Ecosystems, Vol. 35, eds C. Fischer and B. Traub (Cham: Springer), 281–284. doi: 10.1007/978-3-030-19293-8_18
Dorren, L., Berger, F., Frehner, M., Huber, M., Kühne, K., Métral, R., et al. (2015). The new NaiS target profile for rockfall | [Das neue NaiS-Anforderungsprofil Steinschlag]. Schweiz. Z. Forstwesen 166, 16–23. doi: 10.3188/szf.2015.0016
Fischer, C., and Traub, B. (2019). Swiss National Forest Inventory – Methods and Models of the Fourth Assessment. Cham: Springer. doi: 10.1007/978-3-030-19293-8
FOEN (2013). Forest Policy 2020. Visions, Objectives and Measures for the Sustainable Management of Forests in Switzerland. Bern: Federal Office for the Environment FOEN.
FOEN (2016). Switzerland’s Second Initial Reportunder the Kyoto Protocol. Report to facilitate the calculation of the assigned amount pursuant to Article3, paragraphs7bis, 8 and 8bis, of the Kyoto Protocol for the Second Commitment Period 2013–2020. (Bern: Federal Office for the Environment FOEN).
FOEN (2020). Switzerland’s Greenhouse Gas Inventory 1990–2018. National Inventory Report including reporting elements under the Kyoto Protocol. Submission of April 2020 under the United Nations Framework Convention on Climate Change and under the Kyoto Protocol. Bern: Federal Office for the Environment FOEN.
FOEN (2021a). Harvested Wood Products (HWP) in the Swiss National Inventory Report 2021 (GHG inventory 1990-2019). Description of Time Series, Modelling Methodology and Results Used for Accounting HWP Under the Kyoto Protocol. Bern: Federal Office for the Environment FOEN.
FOEN (2021b). Switzerland’s Greenhouse Gas Inventory 1990–2019: National Inventory Report, CRF-tables. Submission of April 2021 Under the United Nations Framework Convention on Climate Change and Under the Kyoto Protocol. Bern: Federal Office for the Environment FOEN.
Forsell, N., Korosuo, A., Federici, S., Gusti, M., Rincón-Cristóbal, J.-J., Rüter, S., et al. (2018). Guidance on Developing and Reporting Forest Reference Levels in Accordance with Regulation (EU) 2018/841. Available online at: https://ec.europa.eu/clima/policies/forests/lulucf_en (accessed March 12, 2021).
Forsell, N., Korosuo, A., Gusti, M., Rüter, S., Havlik, P., and Obersteiner, M. (2019). Impact of modelling choices on setting the reference levels for the EU forest carbon sinks: How do different assumptions affect the country-specific forest reference levels? Carbon Balance Manage. 14:10. doi: 10.1186/s13021-019-0125-9
Friedlingstein, P., Jones, M. W., O’sullivan, M., Andrew, R. M., Hauck, J., Peters, G. P., et al. (2019). Global Carbon Budget 2019. Earth Syst. Sci. Data 11, 1783–1838. doi: 10.5194/essd-11-1783-2019
Grassi, G., Pilli, R., House, J., Federici, S., and Kurz, W. A. (2018). Science-based approach for credible accounting of mitigation in managed forests. Carbon Balance Manage. 13:8. doi: 10.1186/s13021-018-0096-2
Gusti, M., Di Fulvio, F., Biber, P., Korosuo, A., and Forsell, N. (2020). The effect of alternative forest management models on the forest harvest and emissions as compared to the forest reference level. Forests 11:794. doi: 10.3390/f11080794
IPCC (2014). Revised Supplementary Methods and Good Practice Guidance Arising from the Kyoto Protocol (KP Supplement). Intergovernmental Panel on Climate Change, eds T. Hiraishi, T. Krug, K. Tanabe, N. Srivastava, J. Baasansuren, M. Fukuda, et al. (Cham: IPCC).
Jonsson, R., Rinaldi, F., Pilli, R., Fiorese, G., Hurmekoski, E., Cazzaniga, N., et al. (2021). Boosting the EU forest-based bioeconomy: market, climate, and employment impacts. Technol. Forecast. Soc. Change 163:120478. doi: 10.1016/j.techfore.2020.120478
Kindermann, G. (2010). A climate sensitive refining of the basal area increment model in PrognAus | [Eine klimasensitive Weiterentwicklung des Kreisflächenzuwachsmodells aus PrognAus]. Austrian J. For. Sci. 147–178. doi: 10.1038/npre.2011.6301.1
Korosuo, A., Vizzarri, M., Pilli, R., Fiorese, G., Colditz, R., Abad Viñas, R., et al. (2021). Forest Reference Levels under Regulation (EU) 2018/841 for the Period 2021-2025. Luxembourg: Publications Office of the European Union.
Larson, A. J., Lutz, J. A., Donato, D. C., Freund, J. A., Swanson, M. E., Hillerislambers, J., et al. (2015). Spatial aspects of tree mortality strongly differ between young and old-growth forests. Ecology 96, 2855–2861. doi: 10.1890/15-0628.1
Loisel, J., Gallego-Sala, A. V., Amesbury, M. J., Magnan, G., Anshari, G., Beilman, D. W., et al. (2021). Expert assessment of future vulnerability of the global peatland carbon sink. Nat. Clim. Change 11, 70–77. doi: 10.1038/s41558-020-00944-0
Lumley, T. (2020). "“survey: analysis of complex survey samples.” R package version 4.0. Available online at: https://cran.r-project.org/web/packages/survey/citation.html (accessed March 1, 2021).
Matala, J., Ojansuu, R., Peltola, H., Sievänen, R., and Kellomäki, S. (2005). Introducing effects of temperature and CO2 elevation on tree growth into a statistical growth and yield model. Ecol. Model. 181, 173–190. doi: 10.1016/j.ecolmodel.2004.06.030
Mehr, J., Vadenbo, C., Steubing, B., and Hellweg, S. (2018). Environmentally optimal wood use in Switzerland—Investigating the relevance of material cascades. Resour. Conserv. Recycl. 131, 181–191. doi: 10.1016/j.resconrec.2017.12.026
MeteoSwiss (2016a). Monthly and Yearly Mean Temperature: TabsM and TabsY. Zürich: Federal Office of Meteorology and Climatology MeteoSwiss.
MeteoSwiss (2016b). Monthly and Yearly Precipitation: RhiresM and RhiresY. Zürich: Federal Office of Meteorology and Climatology MeteoSwiss.
Nabuurs, G.-J., Arets, E. J. M. M., and Schelhaas, M.-J. (2018). Understanding the implications of the EU-LULUCF regulation for the wood supply from EU forests to the EU. Carbon Balance Manage. 13:18. doi: 10.1186/s13021-018-0107-3
Nabuurs, G. J., Schelhaas, M. J., Mohren, G. M. J., and Field, C. B. (2003). Temporal evolution of the European forest sector carbon sink from 1950 to 1999. Glob. Change Biol. 9, 152–160. doi: 10.1046/j.1365-2486.2003.00570.x
Perin, J., Pitchugin, M., Hébert, J., Brostaux, Y., Lejeune, P., and Ligot, G. (2021). SIMREG, a tree-level distance-independent model to simulate forest dynamics and management from national forest inventory (NFI) data. Ecol. Model. 440:109382. doi: 10.1016/j.ecolmodel.2020.109382
Pfister, C. (1999). Wetternachhersage. 500 Jahre Klimavariationen und Naturkatastrophen (1496-1995). Bern: Verlag P.
Pilli, R., Grassi, G., Kurz, W. A., Fiorese, G., and Cescatti, A. (2017). The European forest sector: past and future carbon budget and fluxes under different management scenarios. Biogeosciences 14, 2387–2405. doi: 10.5194/bg-14-2387-2017
R Core Team (2018). R: A Language and Environment for Statistical Computing. Vienna: R Foundation for Statistical Computing.
Rantakari, M., Lehtonen, A., Linkosalo, T., Tuomi, M., Tamminen, P., Heikkinen, J., et al. (2012). The Yasso07 soil carbon model – Testing against repeated soil carbon inventory. For. Ecol. Manage. 286, 137–147. doi: 10.1016/j.foreco.2012.08.041
Remund, J., Von Arx, G., Gallien, L., Rebetez, M., Huber, B., and Zimmermann, N. E. (2016). “Klimawandel in der Schweiz - Entwicklung waldrelevanter Klimagrössen,” in Wald im Klimawandel. Grundlagen für Adaptationsstrategien, eds A. R. Pluess, S. Augustin, and P. Brang (Ittigen: Bundesamt für Umwelt BAFU), 23–37.
Reyer, C., Bathgate, S., Blennow, K., Borges, J. G., Bugmann, H., Delzon, S., et al. (2017). Are forest disturbances amplifying or canceling out climate change-induced productivity changes in European forests? Environ. Res. Lett. 12:034027. doi: 10.1088/1748-9326/aa5ef1
Rohner, B., Kumar, S., Liechti, K., Gessler, A., and Ferretti, M. (2021). Tree vitality indicators revealed a rapid response of beech forests to the 2018 drought. Ecol. Indic. 120:106903. doi: 10.1016/j.ecolind.2020.106903
Rohner, B., Waldner, P., Lischke, H., Ferretti, M., and Thürig, E. (2018). Predicting individual-tree growth of central European tree species as a function of site, stand, management, nutrient, and climate effects. Eur. J. For. Res. 137, 29–44. doi: 10.1007/s10342-017-1087-7
Schelhaas, M.-J., Hengeveld, G. M., Heidema, N., Thürig, E., Rohner, B., Vacchiano, G., et al. (2018). Species-specific, pan-European diameter increment models based on data of 2.3 million trees. For. Ecosyst. 5:21. doi: 10.1186/s40663-018-0133-3
Schelhaas, M.-J., Nabuurs, G.-J., Hengeveld, G., Reyer, C., Hanewinkel, M., Zimmermann, N. E., et al. (2015). Alternative forest management strategies to account for climate change-induced productivity and species suitability changes in Europe. Reg. Environ. Change 15, 1581–1594. doi: 10.1007/s10113-015-0788-z
Seidl, R., Thom, D., Kautz, M., Martin-Benito, D., Peltoniemi, M., Vacchiano, G., et al. (2017). Forest disturbances under climate change. Nat. Clim. Change 7, 395–402. doi: 10.1038/nclimate3303
Stadelmann, G. (2020). Quantification of forest biomass and timber harvesting potential in Switzerland | [Quantifizierung der Waldbiomasse und des Holznutzungspotenzials in der Schweiz]. Schweiz. Z. Forstwesen 171, 124–132. doi: 10.3188/szf.2020.0124
Stadelmann, G., Bugmann, H., Meier, F., Wermelinger, B., and Bigler, C. (2013). Effects of salvage logging and sanitation felling on bark beetle (Ips typographus L.) infestations. For. Ecol. Manage. 305, 273–281. doi: 10.1016/j.foreco.2013.06.003
Stadelmann, G., Herold, A., Didion, M., Vidondo, B., Gómez, A., and Thürig, E. (2016). Timber-harvesting potential of Swiss forests: simulation of management scenarios | [Holzerntepotenzial im Schweizer Wald: simulation von Bewirtschaftungsszenarien]. Schweiz. Z. Forstwesen 167, 152–161. doi: 10.3188/szf.2016.0152
Stadelmann, G., Temperli, C., Rohner, B., Didion, M., Herold, A., Rösler, E., et al. (2019). Presenting MASSIMO: a management scenario simulation model to project growth, harvests and carbon dynamics of Swiss forests. Forests 10:94. doi: 10.3390/f10020094
Stadelmann, G., Zell, J., Rogiers, N., and Thürig, E. (in prep). Simulation of a Forest Reference Level under current management practices – a Swiss case study for the Greenhouse Gas Inventory. Environ. Sci. Policy.
Temperli, C., Blattert, C., Stadelmann, G., Brändli, U.-B., and Thürig, E. (2020). Trade-offs between ecosystem service provision and the predisposition to disturbances: a NFI-based scenario analysis. For. Ecosyst. 7:27. doi: 10.1186/s40663-020-00236-1
Temperli, C., Stadelmann, G., Thürig, E., and Brang, P. (2017). Silvicultural strategies for increased timber harvesting in a Central European mountain landscape. Eur. J. For. Res. 136, 493–509. doi: 10.1007/s10342-017-1048-1
Thees, O., Erni, M., Lemm, R., Stadelmann, G., and Zenner, E. K. (2020). Future potentials of sustainable wood fuel from forests in Switzerland. Biomass Bioenergy 141:105647. doi: 10.1016/j.biombioe.2020.105647
Thürig, E., Kaufmann, E., Frisullo, R., and Bugmann, H. (2005a). Evaluation of the growth function of an empirical forest scenario model. For. Ecol. Manage. 204, 53–68. doi: 10.1016/j.foreco.2004.07.070
Thürig, E., Palosuo, T., Bucher, J., and Kaufmann, E. (2005b). The impact of windthrow on carbon sequestration in Switzerland: a model-based assessment. For. Ecol. Manage. 210, 337–350. doi: 10.1016/j.foreco.2005.02.030
Thürig, E., Stadelmann, G., and Didion, M. (2019). Estimating Carbon Stock Changes in Living and Dead Biomass and Soil for the Technical Correction of Switzerland’s Forest Management Reference Level – Modeling Methodology and Results. Birmensdorf: Swiss Federal Institute for Forest, Snow and Landscape Research WSL.
Thürig, E., Stadelmann, G., and Didion, M. (2021). Estimating Carbon Stock Changes in Living and Dead Biomass and Soil for the Technical Correction of Switzerland’s Forest Management Reference Level for the Swiss NIR 2021 (1990–2019) – Modeling Methodology and Results”. Available online at: https://www.bafu.admin.ch/bafu/en/home/topics/climate/state/data/climate-reporting/references.html (accessed July 15, 2021).
Tuomi, M., Laiho, R., Repo, A., and Liski, J. (2011). Wood decomposition model for boreal forests. Ecol. Model. 222, 709–718. doi: 10.1016/j.ecolmodel.2010.10.025
Tuomi, M., Thum, T., Järvinen, H., Fronzek, S., Berg, B., Harmon, M., et al. (2009). Leaf litter decomposition—Estimates of global variability based on Yasso07 model. Ecol. Model. 220, 3362–3371. doi: 10.1016/j.ecolmodel.2009.05.016
UNFCCC (2015). Adoption of the Paris Agreement (No. FCCC/CP/2015/L.9/Rev.1:). Conference of the Parties. Paris: UNFCCC.
Usbeck, T., Wohlgemuth, T., Dobbertin, M., Pfister, C., Burgi, A., and Rebetez, M. (2010). Increasing storm damage to forests in Switzerland from 1858 to 2007. Agric. For. Meteorol. 150, 47–55. doi: 10.1016/j.agrformet.2009.08.010
Valade, A., Bellassen, V., Magand, C., and Luyssaert, S. (2017). Sustaining the sequestration efficiency of the European forest sector. For. Ecol. Manage. 405, 44–55. doi: 10.1016/j.foreco.2017.09.009
Vauhkonen, J., Berger, A., Gschwantner, T., Schadauer, K., Lejeune, P., Perin, J., et al. (2019). Harmonised projections of future forest resources in Europe. Ann. For. Sci. 76:79. doi: 10.1007/s13595-019-0872-5
Werner, F., Taverna, R., Hofer, P., Thürig, E., and Kaufmann, E. (2010). National and global greenhouse gas dynamics of different forest management and wood use scenarios: a model-based assessment. Environ. Sci. Policy 13, 72–85. doi: 10.1016/j.envsci.2009.10.004
Westoby, M. (1984). “The Self-Thinning Rule,” in Advances in Ecological Research, eds A. Macfadyen and E. D. Ford (Cambridge, MA: Academic Press), 167–225.
Keywords: forest reference level, FRL, forest model, MASSIMO, CMP, Yasso07, carbon, Paris Agreement
Citation: Stadelmann G, Portier J, Didion M, Rogiers N and Thürig E (2021) From Paris to Switzerland: Four Pathways to a Forest Reference Level. Front. For. Glob. Change 4:685574. doi: 10.3389/ffgc.2021.685574
Received: 25 March 2021; Accepted: 09 September 2021;
Published: 29 September 2021.
Edited by:
Giorgio Vacchiano, University of Milan, ItalyReviewed by:
Matteo Vizzarri, European Commission, ItalyRobert Jandl, Federal Research and Training Centre for Forests, Natural Hazards and Landscape (BFW), Austria
Copyright © 2021 Stadelmann, Portier, Didion, Rogiers and Thürig. This is an open-access article distributed under the terms of the Creative Commons Attribution License (CC BY). The use, distribution or reproduction in other forums is permitted, provided the original author(s) and the copyright owner(s) are credited and that the original publication in this journal is cited, in accordance with accepted academic practice. No use, distribution or reproduction is permitted which does not comply with these terms.
*Correspondence: Golo Stadelmann, golo.stadelmann@wsl.ch