- 1Bren School of Environmental Science and Management, University of California, Santa Barbara, Santa Barbara, CA, United States
- 2School of Interdisciplinary Arts and Sciences Division of Sciences and Mathematics, University of Washington Tacoma, Tacoma, WA, United States
- 3Bren School of Environmental Science and Management, University of California Cooperative Extension, Agriculture and Natural Resources Division, Modesto, CA, United States
Fuel treatments are a key forest management practice used to reduce fire severity, increase water yield, and mitigate drought vulnerability. Climate change exacerbates the need for fuel treatments, with larger and more frequent wildfires, increasing water demand, and more severe drought. The effects of fuel treatments can be inconsistent and uncertain and can be altered by a variety of factors including the type of treatment, the biophysical features of the landscape, and climate. Variation in fuel treatment effects can occur even within forest stands and small watershed management units. Quantifying the likely magnitude of variation in treatment effects and identifying the dominant controls on those effects is needed to support fuel treatment planning directed at achieving specific fire, water, and forest health goals. This research aims to quantify and better understand how local differences in treatment, landscape features, and climate alter those fuel treatment effects. We address these questions using a mechanistic coupled ecohydrologic model—the Regional Hydro-Ecological Simulation System (RHESSys). We ran 13,500 scenarios covering a range of fuel treatment, biophysical, and climate conditions, for the Southern Sierra Nevada of California. Across fuel treatment type, biophysical, and climate parameters, we find nontrivial variation in fuel treatment effects on stand carbon, net primary productivity, evapotranspiration, and fire-related canopy structure variables. Response variable estimates range substantially, from increases (1–48%) to decreases (−13 to −175%) compared to untreated scenarios. The relative importance of parameters differs by response variable; however, fuel treatment method and intensity, plant accessible water storage capacity (PAWSC), and vegetation type consistently demonstrate a large influence across response variables. These parameters interact to produce non-linear effects. Results show that projections of fuel treatment effects based on singular mean parameter values (such as mean PAWSC) provide a limited picture of potential responses. Our findings emphasize the need for a more complete perspective when assessing expected fuel treatment outcomes, both in their effects and in the interacting biophysical and climatic parameters that drive them. This research also serves as a demonstration of methodology to assess the likely variation in potential effects of fuel treatments for a given planning unit.
Introduction
Informed forest and vegetation management is progressively more important as both severe drought and wildfire activity are predicted to increase in the Western US (Moritz et al., 2012; Clark et al., 2016). In many Mediterranean fire-prone ecosystems drought is already shaping stand-scale dynamics, shifting habitats, and altering the severity and frequency of disturbances including fire and insects (Clark et al., 2016). Recent droughts, like the 2012-2015 California event and subsequent water stress and mortality (Asner et al., 2016), highlight the magnitude of potential impacts of droughts on forest structure and water resources. At the same time, increasing fire severity in many of these regions has led to unprecedented social and economic costs (Moritz et al., 2014). Given these ecologic and socio-economic costs, fuel treatments are increasingly proposed as a way to reduce risks associated with both droughts and fires. Fuel treatments modify forest structure typically by removing understory and small diameter trees, either through mechanical harvest or controlled burns (Agee and Skinner, 2005). Fuel treatments have a variety of purposes, from timber harvest-oriented practices to increase productivity, to the restoration of historic forest structures and associated habitat. Key among these purposes is the role that fuel treatments can play in reducing wildfire severity (Hessburg et al., 2016; Barros et al., 2019) and mitigating drought impacts on vegetation (Tague et al., 2019). We need to understand more broadly how those treatments are altering our landscapes and affecting resources we care about, both directly and indirectly.
Heterogeneity in forest species and stand structures, along with different goals and available resources for forest management, leads to a wide range of actions that fall under the broad category of fuel treatments. Mechanical thinning is frequently used to reduce fire severity and limit canopy fires by reducing surface fuels, increasing the height to live canopy, and decreasing the density of the canopy (Agee and Skinner, 2005; Evans et al., 2011). Prescribed fire is often paired with mechanical thinning, and in this same context aims to increase forest resilience through reductions in surface fuels and scorching (killing) lower branches of trees, increasing the height to live canopy (Evans et al., 2011; Fernandes, 2015). The size and placement of fuel treatments, however, varies. Treatments, particularly thinning, are expensive and are typically focused on areas where fire threatens residences and communities, or where abnormally high severity fire is expected (Wibbenmeyer et al., 2016; Anderson et al., 2018). Fuel treatments can be effective in reducing fire severity or altering fire regimes, but effectiveness varies with forest type and treatment implementation. Treatments can also have adverse and unintended effects (Omi and Martinson, 2002; Agee and Skinner, 2005; Safford et al., 2012). For instance—the stems removed during thinning, called slash, if left on the forest floor can result in greater surface fuels which then increase fire intensity (Stephens et al., 2012). The long-term efficacy and effects of treatments are linked to regrowth and the presence or absence of new and competing species, leading to uncertainty in the net effects on fire severity (Moritz et al., 2014). The uncertainty in these long-term effects, combined with the (often large) expense of each treatment, make long-term planning for, and prediction of, the effectiveness of fuel treatments for reducing fire severity challenging.
In addition to reducing fire severity, fuel treatments, specifically forest density reductions through thinning, have been used to increase forest productivity and growth as part of silviculture, and more recently, as a forest management tool to reduce drought vulnerability and forest mortality (Spittlehouse and Stewart, 2003; McDowell et al., 2007; Cabon et al., 2018). While there is general agreement on the short-term effectiveness of treatments to reduce drought vulnerability and forest mortality, there is still noteworthy uncertainty in the long-term net effects of treatments. In fact, there is potential for post-treatment scenarios to instead increase vulnerability to future drought (Clark et al., 2016; Tague et al., 2019). Typically, density reduction increases the productivity of remaining trees, and reduces overall water stress, largely by a reduction in tree-scale competition for water (Clark et al., 2016; Sohn et al., 2016). However, in semi-arid regions, increases to productivity may be diminished during dry periods (Sohn et al., 2016). Increased leaf-to-sapwood area ratios and type conversion can also both lead to greater drought vulnerability (Clark et al., 2016). Treatment effects on productivity are further affected by the access of remaining trees to shared subsurface storage and changes to the tree scale radiation environment (Tague et al., 2019; Tsamir et al., 2019). Density reductions both directly and indirectly affect carbon sequestration, and while the short-term effect is straightforward, long term sequestration depends on post-disturbance regrowth (North et al., 2009). The interactions between treatments and forest health are also expected to evolve with climate change, making a priori predictions of treatment success uncertain (Allen et al., 2010).
Fuel treatments can also be used to alter water yield (surface and subsurface water leaving an area). Though paired catchment clear cutting studies show consistent increases in water yield; thinning, particularly in Mediterranean forests, shows variability in the magnitude and direction of effects on water yield (Hewlett and Hibbert, 1967; Brown et al., 2005; Saksa et al., 2017). Thinning effects on water yield are dependent on a range of factors, including regrowth, access to storage, species changes, and the resulting forest structure, and there remains persistent debate on the dominant controls on the forest cover-water yield relationship (Brown et al., 2005; Ellison et al., 2012; Filoso et al., 2017; Tsamir et al., 2019; Kirchner et al., 2020, Tague and Moritz, 2019).
The wide range of covarying factors that both affect and are affected by fuel treatments combine to make predicting the net effects of a given treatment difficult. Much of this difficulty is associated with the multiple sources of variation including fuel treatment options, species characteristics, landscape, topographic position, climate, and the possible interactions among them. Understanding and ultimately predicting the total impacts of fuel treatments requires considering the interplay between these factors and thinning objectives, such as carbon sequestration, fire management, forest health. Models are key tools that can be used to explore variable interactions and identify particularly important sources of variation even within the same watershed (Fatichi et al., 2016). By identifying which factors matter and when, these tools provide uncertainty bounds on expected outcomes and can guide strategic fuel treatment placement.
Here we use a mechanistic coupled ecohydrologic model to explore the range of fuel treatment scenarios through time and across biophysical sources of variation. We focus on a mid-elevation forest stand within the California Sierra as a representative example of a region where fuel treatments are both likely to occur and may be focused on multiple benefits (Gould, 2019a,b). In the context of existing uncertainty around the effects of treatments, the goals of this work are twofold:
1. To characterize the expected distribution of fuel treatment effects on key response variables (covering the domains of forests, water, and fire), across likely variability in biophysical contexts that would occur within a management unit (e.g., a forest stand within a particular bioclimatic region).
2. To understand how variability in fuel treatment effects is explained by different biophysical, climatic, or fuel treatment parameters. We demonstrate a novel approach, combining modeling and statistical methods, to understand this parameter-driven variability in fuel treatment effects.
In our analysis, we highlight fuel treatment effects on fire severity, carbon sequestration, water yield and forest productivity and examine whether estimates of these effects are similar to commonly held assumptions of treatment outcomes. We typically expect that over short to medium time periods (5–30 years) fuel treatments:
H1. Reduce fire severity – fuel treatments remove fuel and alter canopy structure, limiting the ability of fire to reach the canopy and thus reducing risk of high severity fires (Agee and Skinner, 2005).
H2. Reduce carbon sequestration – fuel treatments are a direct removal of carbon from the landscape, and so lead to lower carbon sequestration, in the short term and in the absence of future fires (North et al., 2009).
H3. Increase water yield – removal of vegetation directly reduces total transpiration. Though more soil is exposed, increasing ET, those increases are typically smaller than decreases to transpiration, and so water yield (or streamflow) is expected to increase overall (Brown et al., 2005).
H4. Increase productivity – remaining vegetation after a fuel treatment will tend to have less competition and greater access to resources (light, water, nutrients) following a treatment, increasing net primary productivity (Clark et al., 2016; Cabon et al., 2018).
Through sensitivity analysis, we assess how biophysical and treatment variation within a given watershed impact these expected outcomes. While the goal of precise prediction of the total long-term effects of fuel treatments on a specific landscape is still in the future, this work demonstrates a watershed scale approach for mapping the fuel treatment-ecohydrologic parameter space. Our approach can be leveraged to assess fuel treatment effects not only at the stand to watershed scale, but regionally. Moreover, understanding the linkages between biophysical parameters and fuel treatment effects can serve to inform future modeling and forest management in similar watersheds.
Methods
Model Framework
We use the Regional Hydro-Ecological Simulation System (RHESSys) to simulate the effects of thinning (RHESSys 7.1.1). RHESSys captures the relevant range of processes, at scales that support analysis of the hydrologic and vegetation carbon cycling impacts of density reduction. RHESSys is a process-based ecohydrologic model, which in addition to traditional hydrologic modeling, dynamically models plant growth, carbon, and nitrogen cycling, and has successfully been applied to simulate the effects of thinning and climate change impacts on forest growth, carbon cycling, and hydrologic fluxes (Tague et al., 2009; Grant et al., 2013; Saksa et al., 2017; Tague and Moritz, 2019, Tsamir et al., 2019). In particular, Saksa et al. (2017) demonstrated the use of RHESSys to estimate post-thinning water fluxes and vegetation responses. The model has also been used to estimate hydrologic impacts of the restoration of natural fire regimes, including the removal of understory vegetation in Yosemite National Park (Boisramé et al., 2019). RHESSys has recently been coupled with fire spread and fire effects models and coupled model evaluation shows the model can capture spatial and temporal variation in fire regimes (e.g., variation in fire return interval) (Kennedy et al., 2017) and expected relationships in pre- and post-fire forest structure (Bart et al., 2020). Previous work has also evaluated the ability of RHESSys to capture hydrologic and carbon cycling in semi-arid mountain systems (Garcia et al., 2016; Son et al., 2016).
RHESSys accounts for both understory and overstory vegetation. Vegetation ecophysiology parameters can be adjusted to simulate a different plant species. These parameters are set via the RHESSys parameter database (https://github.com/RHESSys/ParamDB), literature derived values, previous RHESSys implementations, or a combination of these methods. Precipitation, wind, and radiation are attenuated through overstory and then understory canopies. All vegetation grows stems, leaves, and roots dynamically. Downwelling radiation is adjusted by topography following MT-CLIM (Running et al., 1987) and landscape scale topographic shading through horizon angles. Radiation interactions with the ecosystem are modeled separately for direct and diffuse radiation, as radiation is attenuated through the canopy. Leaf scale fluxes differentiate between sunlit and shaded leaves. Gross photosynthesis is estimated using the Farquhar Photosynthesis model (Farquhar et al., 1980), which is driven primarily by the availability of light, water, and nitrogen, as well as growth and maintenance respiration models adapted from Ryan (1991). Net photosynthesis is allocated using the method from Dickinson et al. (1998) as also described in Garcia et al. (2016), and carbon and nitrogen both cycle vertically and can transfer laterally. Water input to RHESSys is driven by precipitation, and the model features vertical and horizonal water fluxes, both above and below-ground. Above-ground there is canopy, litter, and soil evaporation and transpiration [using Penman-Monteith (Monteith, 1965)], as well as overland flow (either Hortonian or saturation) and infiltration. Snow accumulation and melt, and the impact of forest shading on these processes is also simulated. Below-ground water (and nutrient) stores are separated into the root zone, which is dynamically defined by the depth of vegetation roots, the unsaturated zone, and the saturated zone. A groundwater store can also be used both as a sink from the saturated zone and contribution to the stream, and water fluxes occur vertically between these below-ground stores as well as laterally, driven by elevation gradients derived from above ground elevation.
A stochastic fire spread module has been recently added to RHESSys (Kennedy et al., 2017). In the module, spread is iteratively tested against a spread probability that is calculated from the litter load, relative deficit (1-ET/PET), topographic slope, and wind direction relative to the direction of spread. RHESSys also calculates fire effects on forest stand and litter variables for those burned cells (Bart et al., 2020) by using the spread probability as a proxy for surface fire intensity. This, in combination with biomass and the relative heights of the understory and overstory, is used to calculate fire-related changes to the surface, understory, and overstory carbon stores. We use a subset of this functionality for our purposes, not running the full fire spread and effects models but instead components derived from them, which is detailed more in section fuel treatment scenarios.
Previously in RHESSys the patch was the smallest modeling unit both spatially and with respect to nutrient and water routing. Here, we include the use of a new “multiscale routing” method (Burke and Tague, 2019; Tsamir et al., 2019). This approach creates a “patch family” as the smallest spatially explicit model unit and use “aspatial patches” within the patch family to account for within patch heterogeneity (e.g., areas within a spatial stand that comprise thinned, open areas, and remaining trees) without requiring very fine scale (meter) spatially explicit representation that would require computational complexity beyond currently available tools. In this context, the aspatial patch is then the smallest modeling unit for vertical water, energy, and nutrient dynamics. In previous RHESSys applications, RHESSys used only hillslope routing, routing subsurface water between spatially explicit model units (patch families) based only on topography. Within patch family routing or “local” routing occurs not because of topography but rather root access, and at scales smaller than are typically modeled. Crucially for the purposes of this work, we have added RHESSys functionality to capture finer scale density reduction impacts on water availability and growth. These advances account for between vegetation (aspatial patch) exchanges (among gaps, thinned, and unthinned vegetated areas) as well as shading by neighboring trees within a stand (patch family). Thus, RHESSys now supports “multiscale routing” with two scales of water (and nutrient) routing: a) routing due to topography between patch families within a hillslope or watershed and b) a new “local routing” that allows exchanges between aspatial patches and their associated vegetation types, that are typically at scales too small (<30-meter) to characterize as spatially explicit units within a watershed scale model such as RHESSys. Sensitivity of ecophysiological fluxes to the addition of multiscale routing methods is demonstrated by Tsamir et al. and presented by Burke and Tague (2019).
Previous work has shown that this “local” routing between gaps, thinned areas and remaining trees can have a substantial impact on post disturbance (fire or density reduction) hydrology and regrowth (Tague and Moritz, 2019). In this study, local routing (shown conceptually in Figure 1), moves water between patches, with the water content of each patch approaching the mean of the patch family, mediated by the sharing coefficient. Water in the rooting zone and unsaturated zone is transferred among aspatial patches in each patch family. A sharing coefficient is defined to modulate the transfer of water between patches. When gaining water, only water up to field capacity is available to the root zone, with excess going to the unsaturated zone. When losing water, only water down to the wilting point is available from the root zone, with the remainder coming from the unsaturated zone. Sharing coefficients will vary primarily with species (which controls root spread and distribution) and gap size distributions (determined by the preexisting forest structure, thinning method, and thinning intensity; Schenk and Jackson, 2002; Clark et al., 2016). Nitrate and dissolved organic carbon (DOC) are transferred along with water following existing approaches in RHESSys for linking water and nutrient transport.
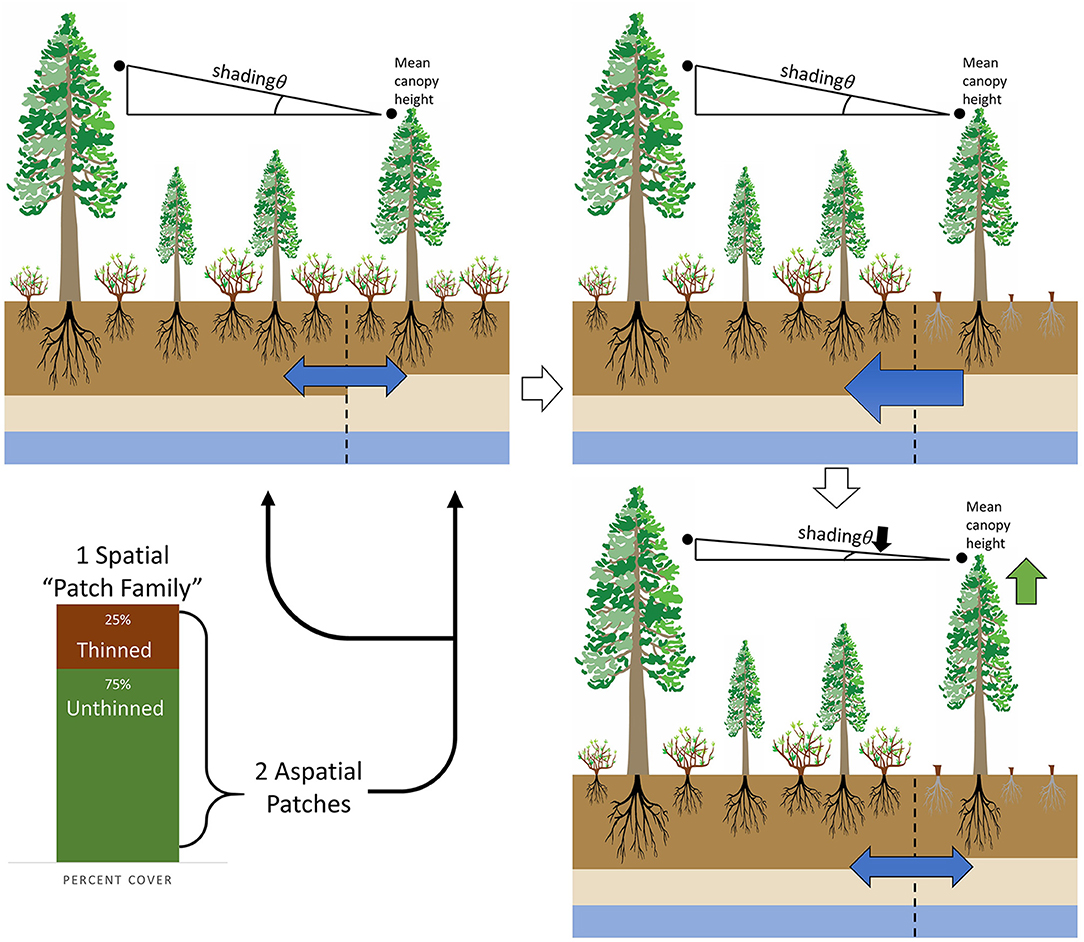
Figure 1. Conceptual model of the multiscale routing method, including the local routing of subsurface storage and shading that occurs between co-located aspatial patches. Shown are examples of pre-treatment, post-treatment, and post-regrowth dynamics, and possible associated changes in subsurface storage and shading.
Shading within the patch family is also accounted for as a part of multi-scale routing. Though the multi-scale routing method does not model individual trees explicitly, by modeling thinned and unthinned areas separately, we approximate the effects of shading between neighboring thinned, unthinned and open area patches. Shading is modified by an adjustment to the east/west horizon, which is used to determine total daily incoming shortwave radiation, based on the relative height of the patch compared to the patch family. Shading is adjusted if the shading angle is greater than the existing horizon angle. Note that for each patch, vertical shading or attenuation of radiation through vertical canopy layers remains as in earlier versions of RHESSys (Tague and Band, 2004). Figure 1 shows our implementation of shading and how it evolves with changing conifer height.
Site
Our study site is a typical mid-elevation conifer forest in the Southern California Sierra, an area that has been previously identified as a high priority area for fuel treatment (Thompson et al., 2016). For model set up and parameterization we use data from the Kings River Experimental Watersheds (KREW) and the Southern Sierra Nevada Critical Zone Observatory (CZO). Higher elevations at this site maintain a seasonal snowpack but transition to rain dominated at lower elevations (Son et al., 2016). Vegetation cover is mainly mixed-conifer forest, consisting of white fir (Abies concolor), ponderosa pine (Pinus ponderosa), Jeffery pine (Pinus jeffreyi), California black oak (Quercus kelloggii), sugar pine (Pinus lambertiana), and incense cedar (Calocedrus), that transition to sclerophyll shrubs [greenleaf manzanita (Arctostaphylos patula), mountain whitehorn (Ceanothus cordulatus)] at lower elevations (Bart et al., 2016; Safeeq and Hunsaker, 2016). Soils are coarse sand and sandy loam (Gerle-Cagwin) with high infiltration capacities, and relatively deep storage (Bales et al., 2011). For this study, we build on previous watershed scale RHESSys simulations at this site (Bart et al., 2016; Son et al., 2016). Here we sample forest stand characteristics by selecting from aspect, elevation, subsurface water storage capacity, and vegetation types within the watershed. For our model scenarios, described in more detail in section scenarios, we use data from a local meteorology station (Grant Grove, National Climate Data Center Station, Lat: 36.73603°N, Lon: 118.96122°W, elevation 2,005 m). Historic records (1943–2015) for this station have a mean annual temperature of 8°C and mean annual precipitation of 1,037 mm.
Scenarios
Model simulation scenarios were designed to cover a reasonable range of possible physical conditions and fuel treatment types for mid-elevations in the Southern Sierra Nevada. A synopsis of these scenarios is included in Table 1. Given the high computational cost of simultaneous parameter variation with continuous sampling of the parameter space, we use a factorial approach and choose 2-3 end member parameter values encompassing high, medium, and low ranges, that define the expected extremes and, in some cases, mid points for each parameter. All simulations are done for a single location (patch family).
Biophysical Parameters and Climate Scenarios
Three vegetation covers were simulated: shrub, conifer overstory with a shrub understory, and a 50/50 mix of uncovered shrub and conifer over shrub (also referred to subsequently as shrub, conifer+shrub, and conifer+shrub/shrub). For aspect we used north and south. For plant (root) accessible subsurface water storage capacity (PAWSC, included at “low,” “medium,” and “high” intervals), we used parameters from Tague and Moritz (2019). These parameters span the range of PAWSC for vegetated locations in mid-elevation Sierras. We note that “high” PAWSC is greater than typical soil depth for this site, and acknowledge that plants often access water well below organic soil depths (Klos et al., 2018). We use root sharing coefficients of {0, 0.25, 0.5, 0.75, 1}, where 0 indicates no root sharing (all aspatial patches are isolated) and 1 indicates complete sharing by all vegetation. Climate in each scenario is varied in two ways: the aridity and the presence or lack of climate warming. “Aridity” is defined by the subset of the observed climate record at Grant Grove station over which the simulation is run, with “wet,” “variable,” and “dry” periods being the maximum, median, and minimum of 30-years moving averages of annual precipitation. The “wet” period is (water years) 1953–1983 (1,103 mm mean annual precipitation), “variable” is 1942–1972 (1,057 mm), and the “dry” period is 1985–2015 (967 mm). Though there is overlap in these periods, importantly the wet and dry periods are mutually exclusive, and the dry period captures the recent Californian droughts which is of particular interest here. Climate warming is included through a uniform shift in the observed climate record, increasing temperature by 2°C, and increasing CO2 to 450 ppm. Climate warming is applied to the wet, dry, and variable periods to extend the range of climate conditions (e.g., to include the possibility of warmer droughts). We acknowledge that future climate may include a wider range of conditions (such as longer duration or more frequent droughts). However, climate model estimates of precipitation change for this region remain uncertain (Hayhoe et al., 2018). To limit computational and model complexity we focus on our simple set of scenarios that have a high likelihood of occurring in the short-term (next decade).
Model estimates require initial conditions that may vary with the biophysical parameters listed above. To account for this, spin-up to initial conditions was done separately for each vegetation, PAWSC, root sharing coefficient, and aspect, as each of these factors could alter the long-term soil nutrient and above ground biomass supported by the plot. Each instance was initialized with known soil nutrient values for the mid-elevation Southern Sierra site, and then each was run for an additional 140 years (looping the observed climate record) to further initialize the soil nutrients and allow vegetation to grow and reach maturity. Our analysis focuses on mature forest/shrubs, assuming no recent fires as these are likely to be the conditions targeted by fuel treatments.
Fuel Treatment Scenarios
Fuel treatment scenarios were selected to explore the range of possible thinning methods, intensities, and frequencies, while being limited and guided based on reasonable real-world (financial and physical) constraints on area treated and treatment frequency (Calkin and Gebert, 2006; North et al., 2015). Three main categories of treatment were selected: understory thinning (paired with prescribed fire), overstory thinning, and prescribed fire alone. In RHESSys, fuel removal is implemented as removal of a combination of litter and vegetation understory or overstory carbon and nitrogen stores (including stores in leaf, stems, and roots). RHESSys does not currently track individual stems, thus all thinning scenarios remove a given percentage of litter, overstory and/or understory pools, based on the type and intensity of thinning. Understory thinning is meant to approximate a thinning from below strategy, though we limit fuels removed to only the shrub understory. All understory treatments were coupled with a lagged (by 1 month) prescribed fire. Understory thinning was simulated in RHESSys through removal of both carbon and nitrogen from the shrub understory. Prescribed fire following thinning removes litter carbon and nitrogen stores. Overstory thinning is meant to approximate a selection thinning strategy and is limited to removal of overstory vegetation carbon and nitrogen pools. Overstory thinning was combined with two slash (vegetation removed during thinning) management scenarios. One where slash remains and becomes part of litter pools (potentially increasing future fire spread and severity) and a second where slash is removed. Prescribed fire, both when it follows an understory thinning and when used alone, is simulated by removal of both litter and coarse woody debris.
Understory and overstory treatments were performed at three intensities, implemented in RHESSys through application of the treatment (e.g., removal of vegetation) at fractional area coverages of 0.1, 0.25, and 0.4. For example, a 0.1 intensity understory treatment removes all understory carbon and nitrogen for an aspatial patch with 10% coverage, which for the encompassing patch family, translates to removal of 10% of the total understory (and a smaller reduction in total stand carbon). A treatment of only prescribed fire was also run where 100% of litter and coarse woody debris pools were removed for all aspatial patches. For scenarios with only shrub vegetation cover, where there is no understory, we omit the overstory thinning scenarios (as the single shrub canopy “overstory” is already thinned equivalently by the understory thinning scenarios).
Each of the treatment method and intensity combinations was run at three different temporal frequencies over the 30-years simulation. All treatment scenarios start with a treatment at the simulation start. We then have three different temporal treatment frequencies over the 30-years simulations: no further treatments, treatments every 5 years, and treatments every 10 years. Each of these treatment scenarios were repeated for all combinations of biophysical parameters. A no treatment scenario was also run for each biophysical scenario. A total of 31 treatment scenarios, and 540 biophysical and climatic scenarios were run yielding a total of 13,500 scenarios (with incompatible vegetation type + treatment method scenarios removed). All scenarios were run at a daily timestep for 30 years. For each scenario we output three key biophysical variables: stand carbon, net primary productivity (NPP), and evapotranspiration (ET), and three fire-related variables: fire spread probability (FSP), shrub fuel height (shrub only scenarios), and conifer canopy fuel gap (conifer+shrub scenarios). The three biophysical variables broadly serve as metrics for key functions in the domains included in Figure 2. Stand carbon is included as a means of tracking carbon sequestration, NPP is used as a metric of forest health and is further useful as a measure of drought resilience, and ET shows direct effects on the water balance and indirect effects of treatments on water yield.
The fire-related variables: FSP, shrub fuel height, and conifer canopy fuel gap, are indicators of how fire regimes might vary across scenarios and parameters. FSP denotes the likelihood that a location would burn, given ignition (or fire in a neighboring patch), and is broadly an indicator of surface fire occurrence and fire spread. This metric however does not reflect the fire severity or the impact of a fire on stand structure and biomass. We note that for the single patch family implementation used here (without neighboring patch families), we cannot run the full RHESSys-Fire model (Kennedy et al., 2017; Bart et al., 2020) directly. RHESSys, however, does provide fire-related outputs at the patch scale, from which we calculate the metrics included here. Shrub fuel height and conifer canopy fuel gap are direct indicators of stand structure/biomass, and indirectly serve as proxies for potential fire severity. In the shrub only case we use mean annual maximum shrub height (over the simulation period), as it is indicative of available fuels. In conifer+shrub scenarios we use the difference in understory and overstory fuel heights. We use the canopy height gap here as an indicator of the likelihood that ladder fuels (understory shrubs) would facilitate a crown fire if fire were to spread into this patch. The mixed 50/50 vegetation runs (conifer overstory with shrub understory combined with uncovered shrub alone) were excluded in these analyses as the severity metrics are not comparable. Together the six variables, stand carbon, ET, NPP, FSP, shrub fuel height, and conifer canopy fuel gap, span the range of domains encompassed in Figure 2.
Analysis
The number and breadth of simulation outputs presents a challenge in analyzing the simulation results. Each scenario produces a time series of responses to the fuel treatments, that reflects the impact of daily to inter-annual variation in meteorological forcing. Figure 3 highlights an example of this, illustrating the roles of fuel treatment timing, vegetation regrowth, and seasonally driven trends in stand carbon. There are complex interactions that arise from the layered effects of baseline seasonal trends (in stand carbon) and post-treatment regrowth—Figure 3 shows just one example of this that illustrates differences between treatments and the baseline “no treatment” case at a monthly time scale. Though these finer-time scale regrowth dynamics certainly merit greater investigation, this work is focused on a broader synthetic perspective. Our goal is to assess the differential role of biophysical and climatic parameters and treatment scenarios on the long-term aggregate effects of fuel treatments. For all response variables, our analyses look at changes in treated scenarios relative to otherwise equivalent untreated scenarios, computed as the percent change of the simulation-long (30-years) annual averages, between each treated scenario and untreated equivalent scenario. Because we average over the 30-years simulation, we provide a longer-term perspective of fuel treatment effects, with less emphasis on the ephemeral and more immediate fuel treatment responses.
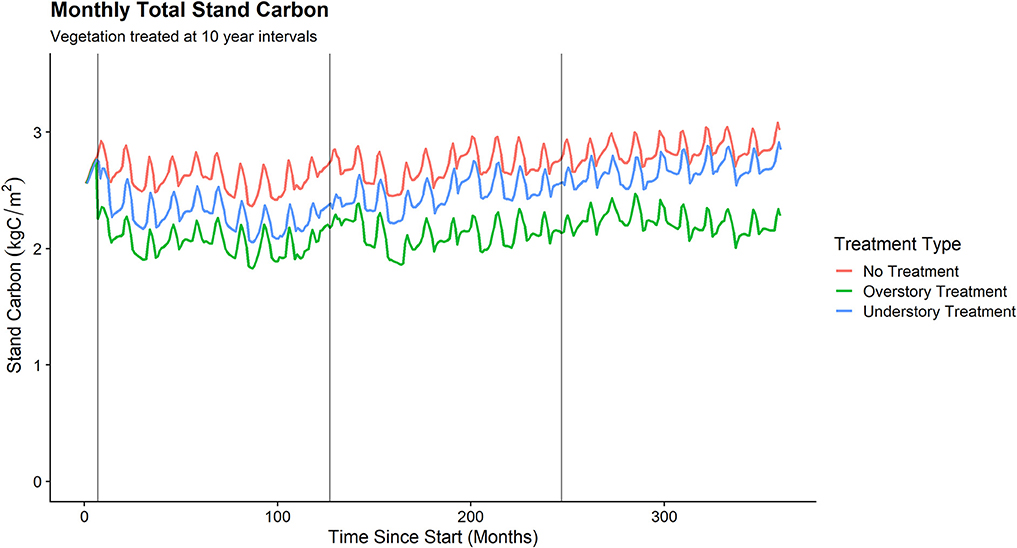
Figure 3. Monthly stand carbon for two treatment scenarios (40% understory removal with following prescribed fire and 40% overstory removal) and a no treatment scenario, performed on conifer overstory with shrub understory, implemented every 10 years (vertical lines), with otherwise identical biophysical and climatic parameters (“wet” aridity, no climate warming, “low” PAWSC, 0.5 root sharing coefficient, North aspect).
As the goal of this research is both to characterize the broader scope of outcomes, while also interrogating specific parameter interactions, we include analyses to facilitate both goals. Histograms are used to capture the range and distribution of fuel treatment effects on each response variable. To illustrate parameter interactions, we also use a series of boxplots, showing response variable distributions subset by parameters. Showing all possible parameter interactions in this way is not feasible, thus we select several particularly salient examples. We also use Random Forests [with the R packages RandomForest and randomForestExplainer; (Liaw and Wiener, 2002; Paluszynska et al., 2019)] to identify the relative importance of biophysical and climatic parameters in predicting the treatment effects. Random forests use a bootstrap of the regression tree combined with random sampling of predictors at each node in the tree. We generated the random forests each with 500 trees (bootstrap runs) and with local importance set to TRUE. We use minimum depth to rank the parameters by importance. The depth in a tree indicates the order in which a parameter is selected. A smaller value for depth indicates higher importance, with typical low values (for our purposes) of ~1, and high values >3.
Results
The 13,500 scenarios produced by the varied input parameters result in noteworthy range and variability in effects on forests (stand carbon and NPP), water (ET), and fire (FSP, shrub fuel height, and conifer canopy fuel gap). The distribution of effect sizes of the biophysical and fire variables of interest, across expected variability in biophysical, climatic, and fuel treatment parameters, is shown in Figure 4. Effect sizes highlight the long-term mean changes in each response variable to a fuel treatment, relative to untreated equivalents. Distributions shown for each response variable are grouped (colored) only by treatment type, and thus results for each treatment type include variation in not only biophysical parameters but also fuel treatment intensities and timing. All four of the expected fuel treatment outcomes (H1–H4) are confirmed to varying degrees by means of simulation distributions, although for NPP mean is not significantly different from 0 (no change). Fire severity (as indicated by shrub fuel height and conifer canopy fuel gap) is reduced, carbon sequestration goes down, and water yield increases. However, for all effects there is substantial variation in the magnitude, and for some scenarios, direction of the outcomes. Most treatment effect distributions are roughly normally distributed, although some variables including ET, shrub fuel height, and conifer canopy fuel gap (Figures 4C,E,F) show left tailed skews. The result of this is that, despite fuel treatment effects broadly conforming to expected outcomes (H1–H4), some subset of scenarios will diverge from those expectations. Stand carbon and ET (Figures 4A,C) adhere to expected treatment effects (H2, H3) in most cases, with only 23.4 and 22.4% of scenarios showing increases in stand carbon and ET respectively, and those increasing scenarios are weighted toward 0% change. NPP features a large range of treatment effects (-150–50%), with 42% of scenarios leading to decreases, departing from expected treatment effects (H4). FSP has a narrow range, spanning only−13–8%, which is an expected outcome given that fuel treatments are not typically expected to have a strong effect on fire spread rates. Potential fire severity, on the other hand, is expected to be affected by fuel treatments. Shrub fuel height and conifer canopy fuel gap show a substantial range of outcomes,−62–1% for shrubs, and−170–48% for conifer. Treatment effects on shrub fuel height consistently align with expected reductions in fire severity (H1) whereas changes in conifer canopy fuel gap are strongly dependent on treatment type with overstory treatments leading to increases in potential fire severity, diverging from expected effects.
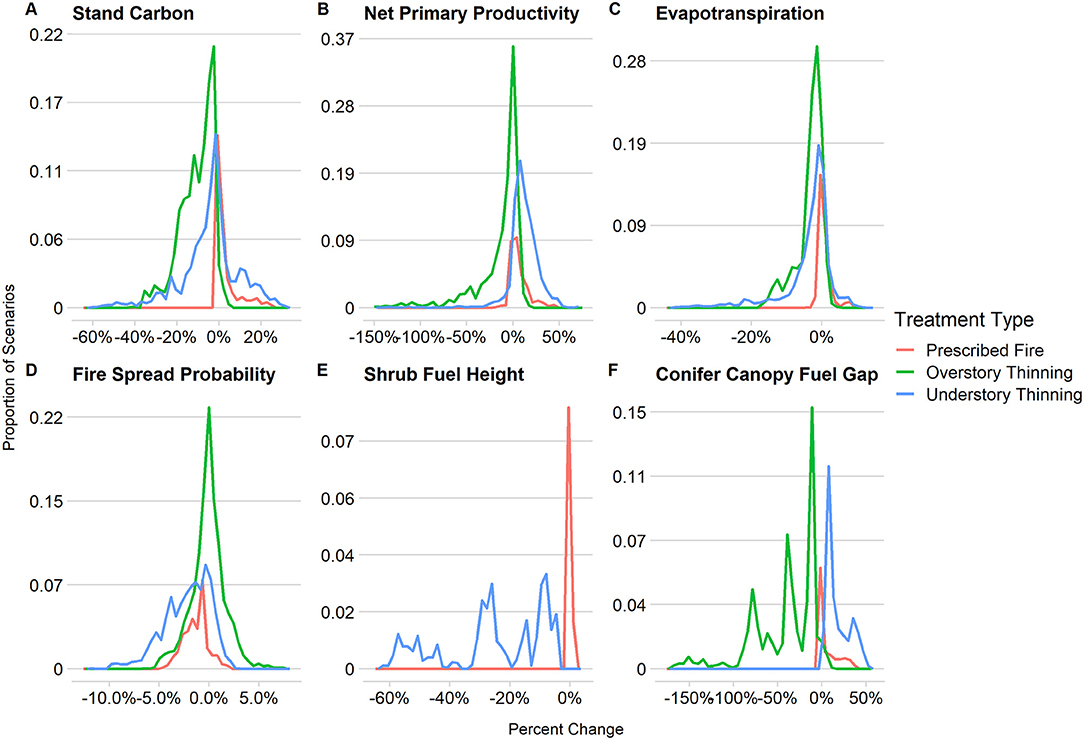
Figure 4. Histograms of fuel treatment effect sizes, in percent change of simulation long (30-years) means relative to untreated equivalent scenarios, for stand carbon (A), net primary productivity (B), evapotranspiration (C), fire spread probability (D), shrub fuel height (E), and conifer canopy fuel gap (F). Colored by treatment type.
Interactions between fuel treatment and biophysical parameters, and the subsequent impact on fuel treatment effects, are of specific interest in this research. Interactions between treatment type and PAWSC alter fuel treatment effects on NPP, ET, conifer canopy fuel gap, and fire spread probability (subset for only conifer+shrub vegetation scenarios; Figure 5). Treatments, of all types, performed on high PAWSC, largely lead to increasing NPP (Figure 5A), In contrast, in low PAWSC, overstory thinning produces substantial decreases in NPP (median of−24%), while understory thinning and prescribed fire both have a positive median change of 4%. These varied treatment effects show that for some sites with lower PAWSC (shallow soils), NPP declines may occur and are more likely, while for other sites with high PAWSC, differences in treatment can lead to substantially larger or smaller increases. Treatment effects on ET (Figure 5B), by comparison to NPP, tend to be smaller and have less variation, both across PAWSC and treatment type. At medium and low PAWSC, thinning leads to expected reductions in ET, while at high PAWSC and for all prescribed fire scenarios ET increases, deviating from expectations (H3). Conifer canopy fuel gap (Figure 5C) shows a more notable difference in treatment effects across treatment type as opposed to PAWSC. Overstory treatment effects on conifer canopy fuel gap are nearly all negative (median−32-−38%), indicating increasing fire severity contrary to expected reductions (H1), while understory treatments and prescribed fire have more moderate, and typically positive effects on conifer canopy fuel gap (median ~ 0–32%). Fire spread probability (Figure 5D) has much smaller magnitude of effects overall than any of the other responses, and shows increasingly negative changes with lower PAWSC, though across all treatments and PAWSC, median changes still only range from 0% (prescribed fire on high PAWSC) to−3% (understory thinning on low PAWSC).
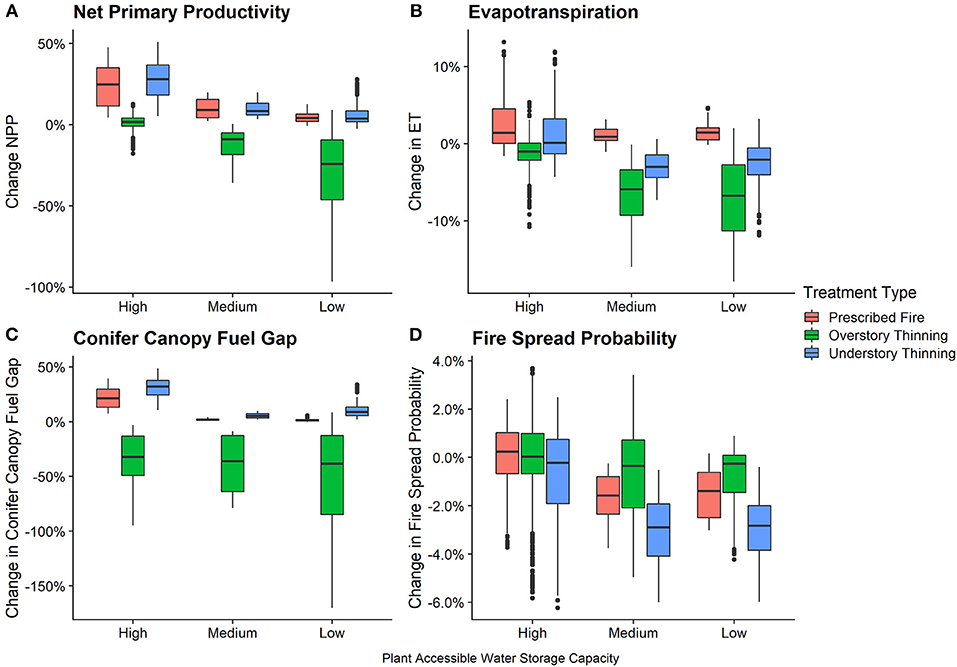
Figure 5. Boxplots of percent change of simulation long (30-years) means relative to untreated equivalent scenarios, for net primary productivity (A), evapotranspiration (B), conifer canopy fuel gap (C), and fire spread probability (D), for only conifer+shrub scenarios, subdivided by PAWSC on the x-axis and colored by treatment type. Upper and lower hinges indicate the 1st and 3rd quartiles (25 and 75th percentiles), and whiskers indicate the greatest/smallest value within 1.5 times the inter-quartile range.
For a subset of parameters, assessed across treatment type, treatment effects on conifer canopy fuel gap vary consistently with fuel treatment type, and inconsistently with the other varied parameters (Figure 6). Across all parameters, fuel treatment effects on conifer canopy fuel gap are split, with consistent negligible to moderate increases from understory treatments and prescribed fire, and reductions from overstory treatments. Though treatment type is the strongest determinant of whether treatment effects will lead to expected reductions in potential fire severity (through increases in conifer canopy fuel gap), the other varied parameters alter the magnitude of those changes. Climate warming (Figure 6A) and aridity (Figure 6B) lead to marginal differences in conifer canopy fuel gap. Increased warming and dry aridity scenarios reduce variability of understory treatments and prescribed fire, though median effects are consistent regardless warming at 9 and 2%, respectively (for both parameters). Treatment intensity (Figure 6C) results in progressively larger changes in conifer canopy fuel gap with greater treatment intensities. For intensities of 0.1–0.4, overstory treatments lead to reductions of −12 to −77%, while understory treatments produce the expected increases (H1) from 5 to 11% (prescribed fire does not have an associated intensity). Treatment interval (Figure 6D) mirrors treatment intensity somewhat, though with greater variability and smaller median shifts. The shortest treatment interval (most frequent) leads to the largest magnitude changes in conifer canopy fuel gap, increases coming from understory treatments and prescribed fire, and reductions from overstory treatments.
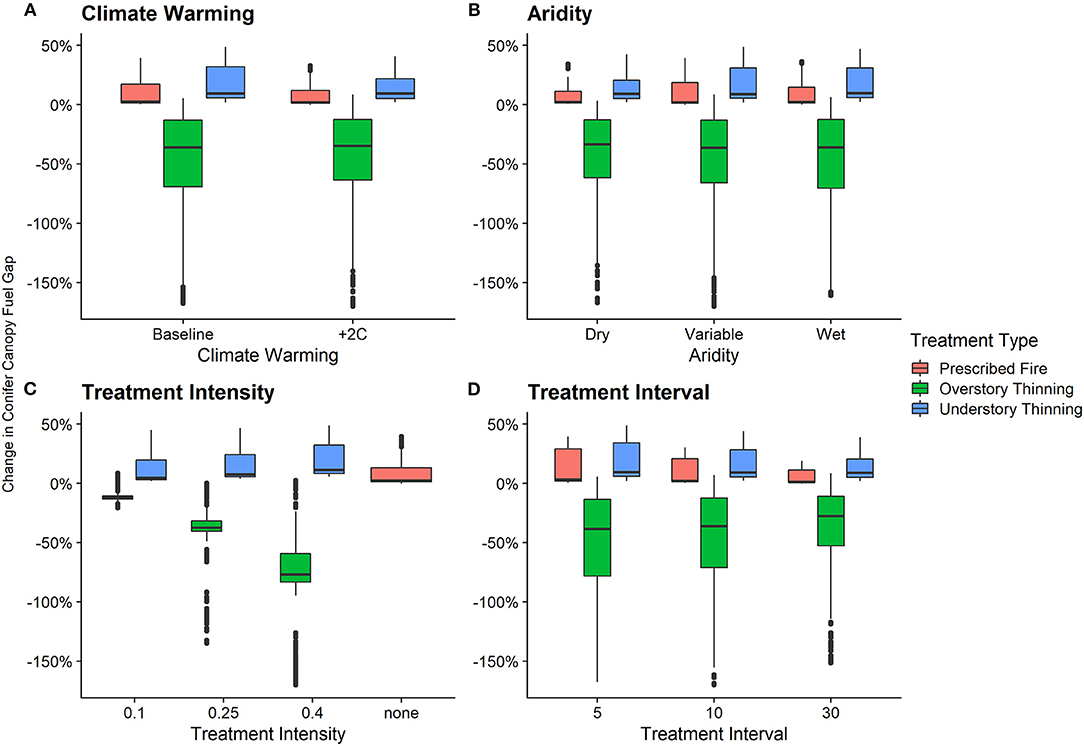
Figure 6. Boxplots of percent change of simulation long (30-years) mean conifer canopy fuel gap, relative to untreated equivalent scenarios, for only conifer+shrub scenarios, subdivided by climate warming (A), aridity (B), treatment intensity (C), and treatment interval (D) on the x-axes and colored by treatment type. Upper and lower hinges indicate the 1st and 3rd quartiles (25 and 75th percentiles), and whiskers indicate the greatest/smallest value within 1.5 times the inter-quartile range.
To summarize the influences of all parameters, accounting for their potential interactions we use random forests. Minimum depth distributions, generated from the random forest decision trees for stand carbon, NPP, ET, FSP, shrub fuel height, and conifer canopy fuel gap are shown in Figure 7. Climate, treatment scenarios and biophysical parameters (collectively “parameters”) are ordered by mean minimal depth. In all cases the predicted metric is the difference between the treated and untreated paired simulation. The rank order of simulation parameters differs across effects—a parameter is ranked higher (has a lower mean minimum depth) when it has a greater ability to reduce variability in subsets of the variable of interest, with the mean value indicating the mean decision tree level at which that occurs. However, lower ranked parameters may still contribute to explaining variability in effect size, particularly if there are a substantial number of trees (cases) where this parameter is ranked highly (ex. minimal depth < = 3). This variable importance occurs for all parameters to some degree apart from aspect.
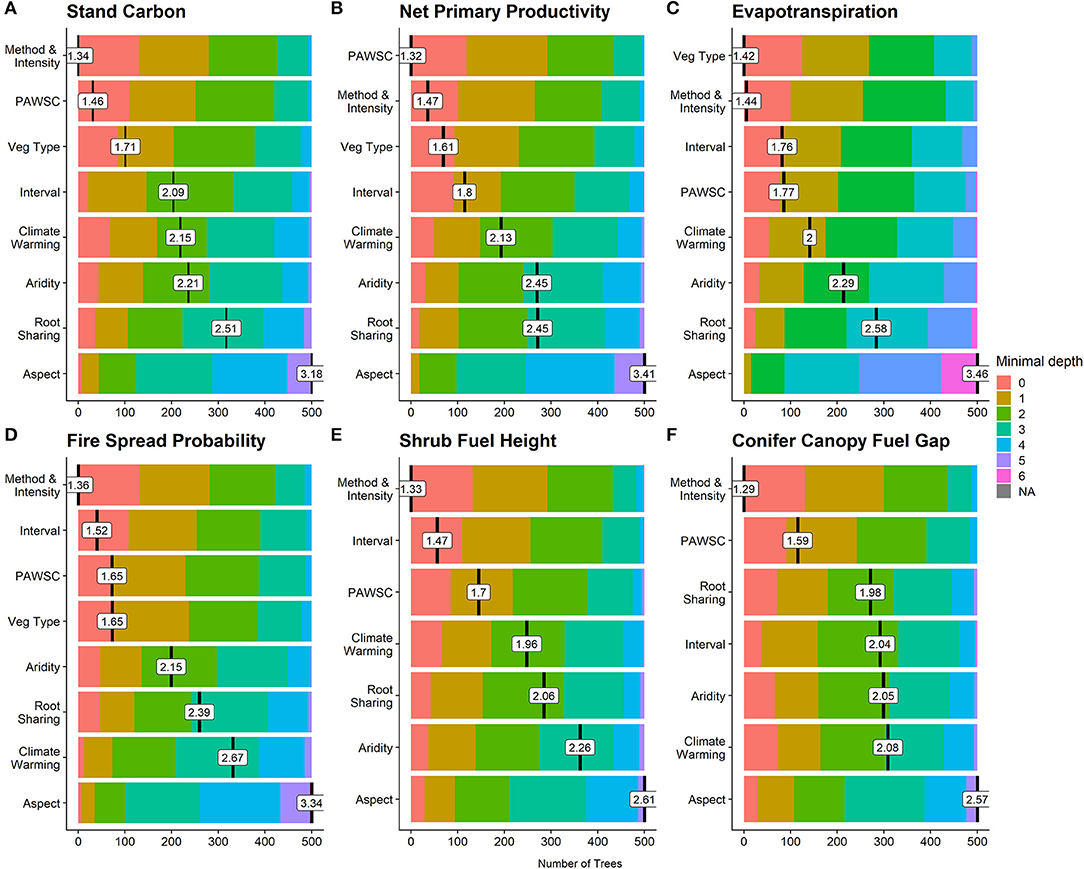
Figure 7. Means and distributions of minimal depths for the random forest decision trees of stand carbon (A), net primary productivity (B), evapotranspiration (C), fire spread probability (D), shrub fuel height (E), and conifer canopy fuel gap (F). Minimal depth indicates, for each random forest, the first decision tree node that a given parameter best grouped (minimized variance) for the output variable.
Fuel treatment method and intensity rank either first or second for all response variables while treatment interval shows more variation in its contribution to treatment effects and tends to rank lower, ranging from second to fourth. Nonetheless fuel treatment interval is a higher-order control, often ranking higher than biophysical or climate parameters. The most consistent parameter across variables, and least influential is aspect, ranking last for all parameters and with a particularly high mean minimal depth of 3.1–3.4. Both PAWSC and vegetation type are moderately important with a consistently high degree of influence. PAWSC matches or exceeds the mean minimal depth of the treatment parameters for stand carbon and NPP effects.
Aridity and climate warming tend to rank relatively low but still contribute to variation in effect. For stand carbon (Figure 7A) these climate parameters have influence that is nearly equal to that of treatment interval. Climate warming, compared to aridity, has a slightly more pronounced effect on NPP and ET (Figures 7B,C), and has less influence in the case of FSP (Figure 7D), but both the ranking and magnitude of the mean minimal depths (~2–2.7) of climate warming and aridity are very similar. The root sharing coefficient, which determines fine-scale within-stand interaction, ranks low, second to last in general, but both the mean minimal depth values (2.39–2.58) and the distributions of minimal depth are similar to that of climate parameters.
Minimum depths of shrub fuel height (Figure 7E) and conifer canopy fuel gap (Figure 7F) feature fewer parameters due to already being subset by vegetation type. The mean minimal depth values and distributions for shrub fuel height follow both the form and general order of the mean minimal depths and distributions of the other response variables. The minimal depth distributions for conifer canopy fuel gap have a somewhat different form, with four parameters grouped tightly at mean minimum depths of 1.98–2.08. Root sharing coefficient also stands out in the conifer case, ranking 3rd with a mean minimal depth of 1.98 (ranked 5th at 2.06 for shrub fuel height), indicating a greater influence of this parameter on the effect of thinning on conifer canopy fuel gap, relative to the role of root sharing coefficient for the other response variables.
Discussion
This analysis has improved our understanding of the effects of fuel treatments across a range of biophysical and climate settings with varied fuel treatment practices. Through the simulations and subsequent analysis done here we provide insight toward two goals: (1) understanding the scope and magnitude of expected fuel treatments effects on forests, water, and fire for a mid-elevation Southern Sierra site and (2) understanding how fuel treatments, biophysical parameters, and climate interact and serve to explain responses in fuel treatment effects on forests, water, and fire.
Distribution of Fuel Treatment Effects on Water, Carbon, and Fire
The distributions of fuel treatment effect sizes characterize the range of outcomes across expected biophysical conditions and varying treatments at the Southern Sierra site (Figure 4). While simulations reflect results for a particular site, these distributions have broader use in a few main ways: (1) By varying topographic and climate parameter sets used in our simulations, results are likely to be representative of much of the Southern Sierra Nevada region. Thus, these results can support regional-scale questions and goals or be upscaled into multi-region analyses. (2) The distributions of effect sizes serve as a starting point, highlighting potential sources of variation in fuel treatment effects that should be explored by more focused simulations for watershed-specific fuel treatment impact assessments. (3) Our approach demonstrates a method that could be readily applied in other locations.
Our sensitivity analysis found non-trivial differences in fuel treatment impacts on mean annual stand carbon, NPP, ET, FSP, shrub fuel height, and conifer canopy fuel gap across fuel treatment type, biophysical, and climate parameters. This is evident both through the varying parameter relationships, such as effects on NPP resulting from varied fuel treatment type and PAWSC (Figure 5A), or effects on conifer canopy fuel gap across fuel treatment type and treatment intensity (Figure 6C), and the differences in parameter influence across response variables shown via the random forest analysis (Figure 7). These parameter relationships are complex, context dependent, and vary by response variable, but together they emphasize that fuel treatment effects are likely to be highly variable even within the same watershed. Variation is not only in magnitude, but often also in direction with some conditions leading to increases and others decreases in the response variable of interest. We find key instances where fuel treatment effects deviate from expected outcomes (H1–H4), such as increases in carbon sequestration or reductions in water yield. This variation across fuel treatment practices, biophysical conditions, and climate parameters (that could all occur within the same management unit) underline the need for a more comprehensive understanding of the factors affecting fuel treatment effectiveness. Results here can extend to regional planning to meet forest management goals; attempting to balance key regional priorities like fire severity reduction and carbon sequestration will require accounting for the likely variation in fuel treatment effects.
Our results serve as a first-order approximation of possible outcomes resulting from a fuel treatment, as well as distributions indicating likely outcomes. Stand carbon (Figure 4A) and ET (Figure 4C; showing changes in water yield), are noteworthy here. Both response variables have relatively few scenarios resulting in increases (percent change > 0%), which is indicative both of how often treatments lead to increases in water yield (reduce ET) and the challenge in increasing carbon sequestration through fuel treatments. These results are generally consistent with our expectations (H2, H3) from other modeling and field-based studies. While these results suggest that fuel treatments alone will generally lead to a decline in sequestered carbon, other studies have shown that if fuel treatments effectively reduce fire severity, this could lead to a long term net gain in carbon storage in the Sierra (Liang et al., 2018). In this study, where wildfire is not explicitly included, the scenarios that do show modest increases in carbon (up to 30%), reflect cases where thinning effectively stimulates growth of remaining vegetation (potentially by reducing competition for water or reducing understory shading). These cases are particularly noteworthy given the baseline assumption of decreasing sequestration (H2). While large scale biomass removal generally leads to increases in streamflow due to declines in transpiration (Brown et al., 2005), the smaller biomass removal associated with thinning is often compensated for by increases in evaporation, and transpiration of remaining trees (Saksa et al., 2017; Tague and Moritz, 2019). We find similar outcomes in this study where some scenarios have a net decrease in water availability (a net increase in ET), diverging from the typically expected water yield increases (H3). The magnitude of changes resulting from treatment are modest—a positive skew from 0% up to 14% increase in ET. For both stand carbon and ET, understanding the limited, but still present, scenarios that depart from typically expected outcomes (H2 and H3), will be key to forest management planning, but also useful as a basis for further, more focused modeling and analysis.
In considering the distribution of fuel treatment effects on fire related variables we see a dichotomy between the small range of effects on FSP (Figure 4D) and the more noteworthy range of effects on shrub fuel height and conifer canopy fuel gap (Figures 4E,F). The difference between the fire metrics shown in Figure 4 underscores the often-small magnitude of effects a fuel treatment is likely to have on fire spread. However, treatments do produce a large range of effects on fire severity, shown in our study particularly when considering the conifer canopy fuel gap, which broadly aligns with expected treatment effects (H1). It should be noted that despite generating metrics assessing potential fire spread and severity, we do not run these simulations dynamically with fires affecting the landscape. Our results emphasize that fuel treatments mostly contribute to reducing potential fire severity, rather than fire spread. We note, however, that our spread indicator does not consider active fire suppression and it is likely that the fire suppression will be more effective at reducing spread when fires are less extreme. Our results also highlight that reductions in potential fire severity also differ both with biophysical/climatic conditions and the type of fuel treatment. Critically, even when only considering understory treatment followed by prescribed fire, a treatment option supported by the literature in regards to its efficacy in reducing fire severity (Agee and Skinner, 2005), there is still a nontrivial range of effects, with many at or near 0% change. This range of effects is in contrast with the (often assumed) expectation of consistent treatment effects on fire severity (H1), and in turn emphasizes the challenge simply in consistently altering fire severity through fuel treatments. Though more specificity and detail on a fuel treatment scenario may lead to greater certainty on the efficacy of that treatment, the baseline assumption should account for this distribution of outcomes, or at the very least should emphasize the uncertainty inherent in these estimates.
Parameter Interactions
For all types of fuel treatment responses - carbon, water, and fire—our results demonstrate substantial interactions among biophysical, climatic, and fuel treatment parameters. Even when only viewing the influence of two parameters on fuel treatment effects (Figure 5), we find that treatment type and PAWSC can interact to produce varied effects across both dimensions. When comparing high and low PAWSC, changes in NPP (Figure 5A) are divergent across treatment type. ET (Figure 5B) and conifer canopy fuel gap (Figure 5C) show similar trends, though it is both the median effect as well as variability that varies across treatment type and PAWSC. This variability arising from parameter interactions is not present for all response variables—fire spread probability (Figure 5D) varies little across PAWSC. Similarly, not all parameters interact and lead to variation in effects. Conifer canopy fuel gap (Figure 6) responds similarly across some parameter combinations and shows varying or diverging trends across others. Both climate warming and aridity (Figures 6A,B), subset by treatment type, show small median impacts on conifer canopy fuel gap, with the primary response being small effects on variability. Treatment intensity and interval (Figures 6C,D), on the other hand, show much less consistency, with conifer canopy fuel gap changing in median effect and variability across both parameters. A critical repercussion of the variable responses we demonstrate is that a treatment strategy, or expected outcome of a treatment (e.g., H1–H4), assessed solely across a single parameter, may miss key trends in how that treatment will more broadly affect forests, water, and fire.
When we look at the effects of all parameters simultaneously using the Regression Trees (Figure 7), we find that most of the parameters play a nontrivial role in explaining response variability. Some parameters, however, do appear to be consistently more important—treatment method and intensity, for example, more strongly control trends in treatment effects as compared to aspect. The high ranking of fuel treatment parameters (treatment method and intensity and treatment interval) is encouraging, suggesting that these actions (and changes in them) are likely to have an impact across a range of site and climate conditions. Nonetheless PAWSC and vegetation type also consistently rank high. Collectively this pattern underscores the importance of biophysical setting and its interaction with treatment strategies in determining how a treatment affects forests, water, and fire. Based on this, PAWSC and vegetation type should be considered in fuel treatment selection. This is not always actionable from a management perspective, as often specific locations in the wildland urban interface necessitate treatment to mitigate high severity fire risk—but in modeling or planning possible treatments with a degree of flexibility, the cost-benefit of where to treat should consider PAWSC and vegetation type with weight similar to the type of fuel treatment itself. This is particularly true of treatments aimed at a broader range of forest and water-related goals—key among them are drought mitigation efforts like reduction in forest mortality or increasing water yield, while still aiming to reduce fire severity.
Climate is a less dominant control on fuel treatment effects as compared to the treatment method and intensity, treatment interval, vegetation type, and PAWSC. Though there is a consistent difference in rank order between the climate parameters (climate warming and aridity) and the above four parameters, the margin can be small, as with treatment effects on stand carbon (Figure 7A) or conifer canopy fuel gap (Figure 7F). Our results indicate that while climate is not a clear primary control on the outcome of a fuel treatment, neither can we ignore it given the often-marginal difference from other, higher ranked, parameters. As focus on fuel treatments used for climate change mitigation increases, the need for inclusion of climate in analyses of fuel treatment effects will also increase. This work serves to contextualize that inclusion of climate as a control on fuel treatments; in more expansive analyses, or those simulating long-term projections, climate (both climate warming and aridity) is a reasonable or even necessary control to include and vary, with the opposite being true in narrower, or shorter term analyses. The role of climate here is also likely underestimated as we simulate climate warming only with a 2°C increase in temperature and our aridity scenarios do not account for the expected increased variability of precipitation (Hayhoe et al., 2018).
Our results are consistent with other research that has considered factors like treatment method, storage capacity, vegetation type, and climate as variables that can influence treatment responses (Finney et al., 2007; Hurteau et al., 2014). Tree-scale interactions between neighboring vegetation, specifically lateral transfers of water and shading, are not typically considered. In this study, the root sharing coefficient reflects variation in tree scale interactions. While the root sharing coefficient is not the dominant factor influencing fuel treatment effects, it is consistently comparable to the climate parameters, and has a particularly large influence on conifer canopy fuel gap. Our research underscores the importance of tree-scale lateral root access in facilitating emergent differences in vegetation heights. While more work is needed to fully understand tree-scale water transfers due to lateral root access, and how this varies with species and canopy structure, the role of tree-scale lateral transfers shown here is noteworthy. Finally, we note that aspect demonstrates a consistently weaker influence on all fuel treatment effects. Inevitably there will be specific cases in which aspect has a more noteworthy influence on treatment effects, but it nonetheless would be the first parameter to exclude when narrowing the scope of analysis.
Model Limitations and Future Work
Though our research makes meaningful strides to better characterize fuel treatments and fuel treatment effects, both through the incorporation of tree-scale lateral transfers, as well as other recent advances to RHESSys, our modeling approach (like any) remains an imperfect approximation of reality. Some limitations include the use of indicators of fire severity rather than natively including fires within the model, and the absences of lateral subsurface water inputs (see Methods). These are not limitations of RHESSys but rather are constraints due to modeling a single “patch family” rather than a hillslope. Focusing on a single patch allowed us to fully explore a complex parameter space. Practical computing would limit this exploration for a full watershed implementation, but future work will investigate watershed scale behaviors for parameter scenarios selected from this study. In this study we did not account for heterogeneity in vegetation size classes nor species differences.
The relationships between scenarios and treatment effects in this research are based on assumptions and limitations specific to our mid-elevation Southern Sierra Nevada site. Despite this, little of the model or scenario parameterization is truly exclusive to our site. Parameter sets were selected specifically to be regionally representative. The results found here are then useful across regions where vegetation, climate, and PAWSC are comparable—Southern Sierra Nevada mid-elevation regions. Beyond the broader application of the results of this work, the methodology developed here, both the modeling methods (RHESSys and multiscale routing) and the general architecture of the scenarios, has merit for use elsewhere. Interest in fuel treatments for fire severity reduction, improved drought resilience, increased water yield, and myriad other purposes is not unique to the Southern Sierras. The methods demonstrated here can be replicated in other regions to build improved understanding of global effects of fuel treatments, which continues to be a key yet challenging goal (Evaristo and McDonnell, 2019; Kirchner et al., 2020). The methods shown in this work also present an opportunity for synthesis with empirical data on fuel treatment effects, and can serve as a foundational step, to preface either more focused modeling work, or to inform the planning of field work. Replication of this work is already planned across a series of sites in the Western United Sates, but with climate-driven increases to fire activity projected for many regions of the world (Moritz et al., 2012), additional locations merit further investigation of fuel treatment effects.
Conclusions
Interactions between biophysical setting, climate, and fuel treatments are complex and have non-linear effects on forests, water, and fire. As fuel treatments receive more interest, and more often with goals beyond fire severity reduction, it becomes increasingly important to understand and ultimately quantify the range and distribution of likely effects that a treatment may have. This presents a challenging task for modelers and field scientists alike given the intersecting scientific domains and complex interconnected processes. Our research works to address this problem and provide a blueprint for how to robustly identify both the range of expected treatment effects and which factors have the greatest influence on those treatment effects. Across our range of scenarios, we highlight cases where treatment effects deviate from expectations, such as instances of increasing carbon sequestration or decreasing water yields. Even when treatment effects conform to expected direction of change (e.g., increasing water yields), results show substantial variation in the magnitude of effects even within the same watershed. For our mid-elevation Southern Sierra site, fuel treatment parameters (i.e., treatment method and intensity, and treatment interval) along with biophysical parameters (i.e., vegetation type and PAWSC), are important controls on fuel treatment effects. Climate and root sharing coefficient are of lesser, albeit variable importance across fuel treatment effects, while aspect stands out with particularly little influence on fuel treatment effects for this site. Arising from these analyses, we underscore the difficulty in estimating fuel treatment effects over narrow ranges of biophysical and fuel treatment parameters, and the need for greater variation across the parameter space, particularly as treatments are used with multiple goals in mind concerning forests, water, and fire. This approach allows for more focused analyses to further interrogate, at finer spatial and temporal scales, how fuel treatments affect our natural environment.
Data Availability Statement
Datasets generated from this study can be found on HydroShare: Burke, W. (2020). Big Creek Patch Scale RHESSys Simulations, HydroShare, http://www.hydroshare.org/resource/2f41fc04cb8645d0938097af5c57f98e. Code used to generate those datasets and perform analysis can be found on Github: https://github.com/wburke24/BigCreekPatchScale. Specific version/commit of the model used – RHESSys 7.1.1 (3/2/20, commit: 01e391f, full SHA: 01e391f56f0f63e6cf3ff3ac4b21122ed6bfce7b) can be found on Github: https://github.com/RHESSys/RHESSys/tree/01e391f56f0f63e6cf3ff3ac4b21122ed6bfce7b.
Author Contributions
WB and CT conceived of initial research and lead research design which was contributed to by MK and MM. WB conducted the modeling and data analysis, lead figure design, and manuscript writing. All authors contributed to figure design and manuscript writing.
Funding
This research was supported by funding from National Science Foundation [Hazard SEES program (Grant number: 094878394)]: Land Management Strategies for Confronting Risks and Consequences of Wildfire (EAR-1520847).
Conflict of Interest
The authors declare that the research was conducted in the absence of any commercial or financial relationships that could be construed as a potential conflict of interest.
Acknowledgments
The authors thank Janet Choate for contributions to figures, and both the Tague lab and SERI-Fire team for their feedback and contributions.
References
Agee, J. K., and Skinner, C. N. (2005). Basic principles of forest fuel reduction treatments. For. Ecol. Manage. 211, 83–96. doi: 10.1016/j.foreco.2005.01.034
Allen, C. D., Macalady, A. K., Chenchouni, H., Bachelet, D., McDowell, N., Vennetier, M., et al. (2010). A global overview of drought and heat-induced tree mortality reveals emerging climate change risks for forests. For. Ecol. Manage. 259, 660–684. doi: 10.1016/j.foreco.2009.09.001
Anderson, S. E., Bart, R. R., Kennedy, M. C., MacDonald, A. J., Moritz, M. A., Plantinga, A. J., et al. (2018). The dangers of disaster-driven responses to climate change. Nat. Clim. Chang. 8, 651–653. doi: 10.1038/s41558-018-0208-8
Asner, G. P., Brodrick, P. G., Anderson, C. B., Vaughn, N., Knapp, D. E., and Martin, R. E. (2016). Progressive forest canopy water loss during the 2012–2015 California drought. Proc. Natl. Acad. Sci. U. S. A. 113, E249–E255. doi: 10.1073/pnas.1523397113
Bales, R. C., Hopmans, J. W., O'Geen, A. T., Meadows, M., Hartsough, P. C., Kirchner, P., et al. (2011). Soil moisture response to snowmelt and rainfall in a Sierra Nevada mixed-conifer forest. Vadose Zone J. 10, 786–799. doi: 10.2136/vzj2011.0001
Barros, A. M., Ager, A. A., Day, M. A., and Palaiologou, P. (2019). Improving long-term fuel treatment effectiveness in the National Forest System through quantitative prioritization. Forest Ecol. Manag. 433, 514–527. doi: 10.1016/J.FORECO.2018.10.041
Bart, R. R., Kennedy, M. C., Tague, C. L., and McKenzie, D. (2020). Integrating fire effects on vegetation carbon cycling within an ecohydrologic model. Ecol. Modell. 416:108880. doi: 10.1016/j.ecolmodel.2019.108880
Bart, R. R., Tague, C. L., and Moritz, M. A. (2016). Effect of tree-to-shrub type conversion in lower montane forests of the sierra nevada (USA) on streamflow. PLoS ONE 11:e0161805. doi: 10.1371/journal.pone.0161805
Boisramé, G. F., Thompson, S. E., Tague, C., and Stephens, S. L. (2019). Restoring a natural fire regime alters the water balance of a sierra nevada catchment. Water Resour. Res. 55, 5751–5769. doi: 10.1029/2018WR024098
Brown, A. E., Zhang, L., McMahon, T. A., Western, A. W., and Vertessy, R. A. (2005). A review of paired catchment studies for determining changes in water yield resulting from alterations in vegetation. J. Hydrol. 310, 28–61. doi: 10.1016/j.jhydrol.2004.12.010
Burke, W., and Tague, N. (2019). “Multiscale routing-integrating the tree-scale effects of disturbance into a watershed ecohydrologic model,” in AGU Fall Meeting (San Francisco, CA). Available online at: https://agu.confex.com/agu/fm19/meetingapp.cgi/Paper/510712
Cabon, A., Mouillot, F., Lempereur, M., Ourcival, J.-M., Simioni, G., and Limousin, J.-M. (2018). Thinning increases tree growth by delaying drought-induced growth cessation in a mediterranean evergreen oak coppice. For. Ecol. Manage. 409, 333–342. doi: 10.1016/j.foreco.2017.11.030
Calkin, D., and Gebert, K. (2006). Modeling fuel treatment costs on forest service lands in the western United States. Western J. Appl. For. 21, 217–221. doi: 10.1093/wjaf/21.4.217
Clark, J. S., Iverson, L., Woodall, C. W., Allen, C. D., Bell, D. M., Bragg, D. C., et al. (2016). The impacts of increasing drought on forest dynamics, structure, and biodiversity in the United States. Glob. Change Biol. 22, 2329–2352. doi: 10.1111/gcb.13160
Dickinson, R. E., Shaikh, M., Bryant, R., and Graumlich, L. (1998). Interactive canopies for a climate model. J. Clim. 11, 2823–2836. doi: 10.1175/1520-0442(1998)011<2823:ICFACM>2.0.CO;2
Ellison, D., Futter, M. N., and Bishop, K. (2012). On the forest cover–water yield debate: from demand- to supply-side thinking. Glob. Chang. Biol. 18, 806–820. doi: 10.1111/j.1365-2486.2011.02589.x
Evans, A. M., Everett, R. G., Stephens, S. L., and Youlz, J. A. (2011). Comprehensive fuels treatment practices guide for mixed conifer forests: california, central and southern rockies, and the Southwest. JFSP Synth. Rep. 12:113. Available online at: https://digitalcommons.unl.edu/jfspsynthesis/12/
Evaristo, J., and McDonnell, J. J. (2019). Global analysis of streamflow response to forest management. Nature 570, 455–461. doi: 10.1038/s41586-019-1306-0
Farquhar, G. D., von Caemmerer, S., and Berry, J. A. (1980). A biochemical model of photosynthetic CO2 assimilation in leaves of C 3 species. Planta 149, 78–90. doi: 10.1007/BF00386231
Fatichi, S., Vivoni, E. R., Ogden, F. L., Ivanov, V. Y., Mirus, B., Gochis, D., et al. (2016). An overview of current applications, challenges, and future trends in distributed process-based models in hydrology. J. Hydrol. 537, 45–60. doi: 10.1016/j.jhydrol.2016.03.026
Fernandes, P. M. (2015). Empirical support for the use of prescribed burning as a fuel treatment. Curr. For. Rep. 1, 118–127. doi: 10.1007/s40725-015-0010-z
Filoso, S., Bezerra, M. O., Weiss, K. C. B., and Palmer, M. A. (2017). Impacts of forest restoration on water yield: a systematic review. PLoS ONE 12:e0183210. doi: 10.1371/journal.pone.0183210
Finney, M. A., Seli, R. C., McHugh, C. W., Ager, A. A., Bahro, B., and Agee, J. K. (2007). Simulation of long-term landscape-level fuel treatment effects on large wildfires. Int. J. Wildl. Fire 16, 712–727. doi: 10.1071/WF06064
Garcia, E. S., Tague, C. L., and Choate, J. S. (2016). Uncertainty in carbon allocation strategy and ecophysiological parameterization influences on carbon and streamflow estimates for two western US forested watersheds. Ecol. Modell. 342, 19–33. doi: 10.1016/j.ecolmodel.2016.09.021
Gould, D. (2019a). Revised Draft Land Management Plan for the Sequoia National Forest USDA. Available online at: https://www.fs.usda.gov/nfs/11558/www/nepa/3403_FSPLT3_4658766.pdf (accessed July 29, 2020).
Gould, D. (2019b). Revised Draft Land Management Plan for the Sierra National Forest. USDA. Available online at: https://www.fs.usda.gov/nfs/11558/www/nepa/3403_FSPLT3_4658767.pdf (accessed July 29, 2020).
Grant, G. E., Tague, C. L., and Allen, C. D. (2013). Watering the forest for the trees: an emerging priority for managing water in forest landscapes. Front. Ecol. Environ. 11, 314–321. doi: 10.1890/120209
Hayhoe, K., Wuebbles, D. J., Easterling, D. R., Fahey, D. W., Doherty, S., Kossin, J., et al. (2018). “2018: our changing climate,” in Impacts, Risks, and Adaptation in the United States: Fourth National Climate Assessment, Volume II, eds D. R. Reidmiller, C. W. Avery, D. R. Easterling, K. E. Kunkel, K. L. M. Lewis, T. K. Maycock, and B. C. Stewart (Washington, DC: U.S. Global Change Research Program), 72–144. doi: 10.7930/NCA4.2018.CH2
Hessburg, P. F., Spies, T. A., Perry, D. A., Skinner, C. N., Taylor, A. H., Brown, P. M., et al. (2016). Tamm review: management of mixed-severity fire regime forests in Oregon, Washington, and Northern California. For. Ecol. Manage. 366, 221–250. doi: 10.1016/j.foreco.2016.01.034
Hewlett, J. D., and Hibbert, A. R. (1967). Factors affecting the response of small watersheds to precipitation in humid areas. For. Hydrol. 1, 275–290.
Hurteau, M. D., Robards, T. A., Stevens, D., Saah, D., North, M., and Koch, G. W. (2014). Modeling climate and fuel reduction impacts on mixed-conifer forest carbon stocks in the Sierra Nevada, California. For. Ecol. Manage. 315, 30–42. doi: 10.1016/j.foreco.2013.12.012
Kennedy, M. C., McKenzie, D., Tague, C., and Dugger, A. L. (2017). Balancing uncertainty and complexity to incorporate fire spread in an eco-hydrological model. Int. J. Wildl. Fire 26, 706–718. doi: 10.1071/WF16169
Kirchner, J. W., Berghuijs, W. R., Allen, S. T., Hrachowitz, M., Hut, R., and Rizzo, D. M. (2020). Streamflow response to forest management. Nature 578, E12–E15. doi: 10.1038/s41586-020-1940-6
Klos, P. Z., Goulden, M. L., Riebe, C. S., Tague, C. L., O'Geen, A. T., Flinchum, B. A., et al. (2018). Subsurface plant-accessible water in mountain ecosystems with a mediterranean climate. Wiley Interdiscip. Rev. 5:e1277. doi: 10.1002/wat2.1277
Liang, S., Hurteau, M. D., and Westerling, A. L. (2018). Large-scale restoration increases carbon stability under projected climate and wildfire regimes. Front. Ecol. Environ. 16, 207–212. doi: 10.1002/fee.1791
McDowell, N. G., Adams, H. D., Bailey, J. D., and Kolb, T. E. (2007). The role of stand density on growth efficiency, leaf area index, and resin flow in southwestern ponderosa pine forests. Can. J. For. Res. 37, 343–355. doi: 10.1139/X06-233
Moritz, M. A., Batllori, E., Bradstock, R. A., Gill, A. M., Handmer, J., Hessburg, P. F., et al. (2014). Learning to coexist with wildfire. Nature 515, 58–66. doi: 10.1038/nature13946
Moritz, M. A., Parisien, M.-A., Batllori, E., Krawchuk, M. A., Dorn, J. V., Ganz, D. J., et al. (2012). Climate change and disruptions to global fire activity. Ecosphere 3:art49. doi: 10.1890/ES11-00345.1
North, M., Brough, A., Long, J., Collins, B., Bowden, P., Yasuda, D., et al. (2015). Constraints on mechanized treatment significantly limit mechanical fuels reduction extent in the Sierra Nevada. J. For. 113, 40–48. doi: 10.5849/jof.14-058
North, M., Hurteau, M., and Innes, J. (2009). Fire suppression and fuels treatment effects on mixed-conifer carbon stocks and emissions. Ecol. Appl. 19, 1385–1396. doi: 10.1890/08-1173.1
Omi, P. N., and Martinson, E. J. (2002). Effects of fuels treatment on wildfire severity. Fort Collins: Colorado State University: Joint Fire Science Program Governing Board, Western Forest Fire Research Center. Available online at: https://www.ntc.blm.gov/krc/uploads/399/Effects%20of%20Fuels%20Treatment%20on%20Wildfire%20Severity.pdf (accessed April 4, 2020).
Paluszynska, A., Biecek, P., and Jiang, Y. (2019). randomForestExplainer: Explaining and Visualizing Random Forests in Terms of Variable Importance. Available online at: https://CRAN.R-project.org/package=randomForestExplainer (accessed May 17, 2020).
Running, S. W., Nemani, R. R., and Hungerford, R. D. (1987). Extrapolation of synoptic meteorological data in mountainous terrain and its use for simulating forest evapotranspiration and photosynthesis. Can. J. For. Res. 17, 472–483. doi: 10.1139/x87-081
Ryan, M. G. (1991). Effects of climate change on plant respiration. Ecol. Appl. 1, 157–167. doi: 10.2307/1941808
Safeeq, M., and Hunsaker, C. T. (2016). Characterizing runoff and water yield for headwater catchments in the southern sierra nevada. J. Am. Water Resour. Assoc. 52, 1327–1346. doi: 10.1111/1752-1688.12457
Safford, H. D., Stevens, J. T., Merriam, K., Meyer, M. D., and Latimer, A. M. (2012). Fuel treatment effectiveness in California yellow pine and mixed conifer forests. For. Ecol. Manage. 274, 17–28. doi: 10.1016/j.foreco.2012.02.013
Saksa, P. C., Conklin, M. H., Battles, J. J., Tague, C. L., and Bales, R. C. (2017). Forest thinning impacts on the water balance of S ierra N evada mixed-conifer headwater basins. Water Resour. Res. 53, 5364–5381. doi: 10.1002/2016WR019240
Schenk, H. J., and Jackson, R. B. (2002). Rooting depths, lateral root spreads and below-ground/above-ground allometries of plants in water-limited ecosystems. J. Ecol. 90, 480–494. doi: 10.1046/j.1365-2745.2002.00682.x
Sohn, J. A., Saha, S., and Bauhus, J. (2016). Potential of forest thinning to mitigate drought stress: a meta-analysis. For. Ecol. Manage. 380, 261–273. doi: 10.1016/j.foreco.2016.07.046
Son, K., Tague, C., and Hunsaker, C. (2016). Effects of model spatial resolution on ecohydrologic predictions and their sensitivity to inter-annual climate variability. Water 8:321. doi: 10.3390/w8080321
Spittlehouse, D. L., and Stewart, R. B. (2003). Adaptation to climate change in forest management. Adapt. Clim. Change 4:12. Available online at: https://jem-online.org/index.php/jem/article/download/254/173.pdf
Stephens, S. L., McIver, J. D., Boerner, R. E., Fettig, C. J., Fontaine, J. B., Hartsough, B. R., et al. (2012). The effects of forest fuel-reduction treatments in the United States. Bioscience 62, 549–560. doi: 10.1525/bio.2012.62.6.6
Tague, C., Seaby, L., and Hope, A. (2009). Modeling the eco-hydrologic response of a mediterranean type ecosystem to the combined impacts of projected climate change and altered fire frequencies. Clim. Change 93, 137–155. doi: 10.1007/s10584-008-9497-7
Tague, C. L., and Band, L. E. (2004). RHESSys: regional hydro-ecologic simulation system—an object-oriented approach to spatially distributed modeling of carbon, water, and nutrient cycling. Earth Interact. 8, 1–42. doi: 10.1175/1087-3562(2004)8<1:RRHSSO>2.0.CO;2
Tague, C. L., Moritz, M., and Hanan, E. (2019). The changing water cycle: the eco-hydrologic impacts of forest density reduction in mediterranean (seasonally dry) regions. Wiley Interdiscip. Rev. 6:e1350. doi: 10.1002/wat2.1350
Tague, C. L., and Moritz, M. A. (2019). Plant accessible water storage capacity and tree-scale root interactions determine how forest density reductions alter forest water use and productivity. Front. For. Glob. Change 2:36. doi: 10.3389/ffgc.2019.00036
Thompson, M. P., Bowden, P., Brough, A., Scott, J. H., Gilbertson-Day, J., Taylor, A., et al. (2016). Application of wildfire risk assessment results to wildfire response planning in the southern Sierra Nevada, California, USA. Forests 7:64. doi: 10.3390/f7030064
Tsamir, M., Gottlieb, S., Preisler, Y., Rotenberg, E., Tatarinov, F., Yakir, D., et al. (2019). Stand density effects on carbon and water fluxes in a semi-arid forest, from leaf to stand-scale. For. Ecol. Manage. 453:117573. doi: 10.1016/j.foreco.2019.117573
Wibbenmeyer, M., Anderson, S., and Plantinga, A. J. (2016). Risk Salience, Public Pressure, and Agency Action: Wildfire and the Management of Public Lands. Mimeo. Available online at: https://www.fs.usda.gov/Internet/FSE_DOCUMENTS/fseprd640156.pdf (accessed October 25, 2018).
Keywords: climate, forest management, modeling, ecohydrology, fuel treatment
Citation: Burke WD, Tague C, Kennedy MC and Moritz MA (2021) Understanding How Fuel Treatments Interact With Climate and Biophysical Setting to Affect Fire, Water, and Forest Health: A Process-Based Modeling Approach. Front. For. Glob. Change 3:591162. doi: 10.3389/ffgc.2020.591162
Received: 03 August 2020; Accepted: 30 November 2020;
Published: 05 January 2021.
Edited by:
David Findlay Scott, University of British Columbia Okanagan, CanadaReviewed by:
Camille Susan Stevens-Rumann, Colorado State University, United StatesJohn T. Van Stan, Georgia Southern University, United States
Copyright © 2021 Burke, Tague, Kennedy and Moritz. This is an open-access article distributed under the terms of the Creative Commons Attribution License (CC BY). The use, distribution or reproduction in other forums is permitted, provided the original author(s) and the copyright owner(s) are credited and that the original publication in this journal is cited, in accordance with accepted academic practice. No use, distribution or reproduction is permitted which does not comply with these terms.
*Correspondence: William D. Burke, wburke@ucsb.edu