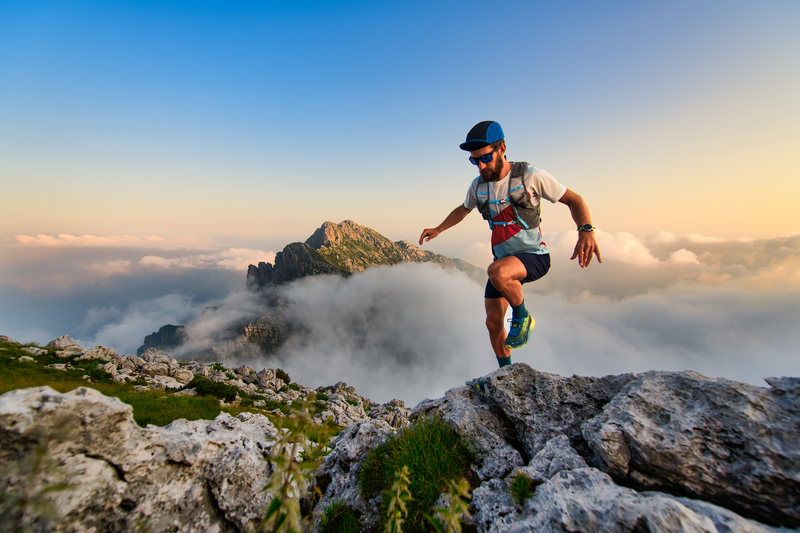
95% of researchers rate our articles as excellent or good
Learn more about the work of our research integrity team to safeguard the quality of each article we publish.
Find out more
HYPOTHESIS AND THEORY article
Front. For. Glob. Change , 24 April 2020
Sec. Tropical Forests
Volume 3 - 2020 | https://doi.org/10.3389/ffgc.2020.00035
This article is part of the Research Topic Land-use Change Impacts on Tropical Ecosystems View all 7 articles
Lianas are woody vines, rooted in the soil, and supported physically by trees. Lianas contribute to forest ecosystem functioning globally, but especially in the tropics and subtropics. However, prolific liana growth following heavy disturbance frequently affects subsequent recovery of forest tree diversity, biomass, structure, and function. Understanding this forest liana dynamic, and its sensitivity to climate and anthropogenic forces, is essential for worldwide forest restoration and climate change mitigation. Here, we synthesise the evidence for both positive and negative effects of lianas on forests and propose a framework that outlines the expected global response of forests to disturbance-induced liana proliferation. Emerging evidence suggests that lianas play a major role in both facilitating and delaying forest recovery following disturbance. At low levels of disturbance and/or where environmental conditions favour tree growth, lianas can facilitate forest recovery by protecting trees from extreme weather, fire, weed invasion and herbivory. However, under conditions where lianas proliferate beyond critical thresholds, positive feedbacks are expected to induce and sustain liana-dominated forest states that can endure for decades or even longer. We conceptualise alternative classes of forest recovery response to disturbance and describe measurement and modelling of liana thresholds. We identify four essential challenges for global change science relating to lianas: (1) incorporation of lianas and sapling stems into forest monitoring and tree stand measurements worldwide; (2) long-term experiments to determine variation in liana-tree competition, and potential drivers across forest successional gradients; (3) identification and prediction of liana thresholds and other alternative forest recovery response classes; and (4) dynamic mechanistic modelling of forest recovery to determine regional and global variation within and among different recovery response classes, in relation to variation in potential drivers, liana feedbacks and their interactions. Addressing these challenges will determine the importance of lianas in shaping regional and global forest composition, recovery and dynamics.
When ecosystems are disturbed beyond threshold levels for sustaining ecological function, they can lose resilience and undergo abrupt reductions in biological attributes and core processes (Scheffer et al., 2001; Newton and Cantarello, 2015). The consequence of exceeding these critical thresholds in forests has global significance, because these ecosystems sustain global biodiversity (Gibson et al., 2011), carbon sequestration (Pan et al., 2011) and national economies (Carrasco et al., 2014). Resilience thresholds in forests may be exceeded in several ways: conversion to treeless or alternative forest states by fire, drought, pests and grazing (Chapin et al., 2004; Malhi et al., 2009; Hirota et al., 2011; Hoffmann et al., 2012) and potentially climate change (Scheffer et al., 2012); reduced stand growth with over-harvesting of timber Bahamondez and Thompson, 2016); increasing biomass with rising CO2 (Higgins and Scheiter, 2012); declining forest cover with shifting agriculture (Magnuszewski et al., 2015); and more positively, increasing biodiversity with plant biomass recovery following disturbance (Lennox et al., 2018). However, identification of thresholds requires extensive spatial and temporal data to diagnose true state changes resulting from exceeding a threshold, versus abrupt changes in state resulting from abrupt changes in environmental influences (Ratajczak et al., 2018) (see glossary, Table 1). Threshold identification continues to be a major challenge for global change science, particularly relating to human forest disturbance (Reyer et al., 2015; Martin et al., 2017), which has led to worldwide reductions in carbon sequestration (Grace et al., 2014) and biodiversity (Barlow et al., 2016).
Different forest attributes vary in their rates of recovery from disturbance. Global satellite-based observations of forest loss have identified an average biomass recovery time of 444 years following disturbance, ranging from <50 years in managed temperate forests to >1,000 years in tropical evergreen forests (Pugh et al., 2019). Tree species richness typically recovers to pre-disturbance levels within just a few years in temperate forests and a few decades in tropical forests (Martin et al., 2013; Meli et al., 2017), varying between 11 and 228 years based on recent neotropical data (median 54 years; Rozendaal et al., 2019). Full species composition has even slower recovery, ranging from <10 to 6,846 years in tropical forests, but often not recovering at all (mean 503–780 years; Cole et al., 2014; Rozendaal et al., 2019). While poorly understood, high temporal variation in forest biomass recovery, at least in part, varies with environmental conditions, particularly water availability (Poorter et al., 2016) and also soil fertility and temperature (Hobbs and Cramer, 2007). Moreover, meta-analysis of 221 landscapes worldwide shows that forest recovery is mostly related to the duration and extent of forest disturbance (Crouzeilles et al., 2016). Palaeoecological records across four tropical regions further show that this disturbance often entirely prevents forest recovery as a result of high spatial extent or temporal frequency (Cole et al., 2014). Data limitations have precluded identification of forest recovery thresholds (i.e., critical levels of disturbance preventing tree canopy regeneration), their spatio-temporal variation and mechanisms leading to them (Reyer et al., 2015).
Consensus is growing that lianas (i.e., woody vines; Table 1) play an important role in driving variation in tropical, and some temperate, forest recovery pathways, with profound implications for forest recovery and the global carbon sink (Phillips et al., 2002; Durán and Gianoli, 2013; Van der Heijden et al., 2013; Durán et al., 2015; Lai et al., 2017). Accordingly, research focussing on lianas has increased exponentially, at a rate exceeding the general increase in ecological research (Figure 1). Lianas grow rapidly in response to increased light levels caused by heavy disturbance in many tropical and subtropical forests (Schnitzer and Bongers, 2011), e.g., from logging or cyclones. Subsequently, multiple empirical observations from across the tropics show that forests with abundant lianas have slow rates of tree growth (and hence also slow forest recovery following disturbance) compared to those with few lianas (Marshall et al., 2017). The presence of two alternative slow vs. fast forest recovery trajectories dependent on liana abundance (Schnitzer et al., 2000; Foster et al., 2008; Tymen et al., 2016; Cazzolla Gatti, 2018) would be consistent with a threshold response (Bestelmeyer et al., 2011), i.e., with recovery only possible below a critical threshold of liana abundance.
Figure 1. Increasing international research focus on lianas, expanding on previous analysis (Schnitzer et al., 2015). Primary y-axis shows the number of papers per year with primary focus on lianas (i.e., title mentions of “liana” or “lianas”; N title; peach-coloured points; exponential r2 = 0.93). Secondary y-axis shows the number of papers per year with more general inclusion of lianas (i.e., title, keywords or abstract mentions; N topic; green points; not exponential). Inset: Percentage of 477,915 ecological publications with “liana” or “lianas” mentioned in the title (polynomial r2 = 0.60). Ecological publications were those categorised as “ecology” by the data source: Thompson Reuters Web of Science Core Collection, 10th April 2019.
Despite the global significance of lianas and stalled forest recovery, to our knowledge forest recovery thresholds have never been examined with respect to changes in liana abundance, cover or biomass (hereafter “liana thresholds”). Understanding forest thresholds will improve our capacity to predict biomass and biodiversity recovery (Norden et al., 2015), particularly in forested regions with intensive and growing human presence (Arroyo-Rodríguez et al., 2017). With global land-use intensification now far exceeding that in previous centuries (Foley et al., 2005), the constraints on forest recovery have increased, forming novel and uncertain interactions with simultaneous changes in climate (Higgins and Scheiter, 2012) and liana abundance (Schnitzer and Bongers, 2011). Systematic identification of liana thresholds against a background of interacting climatic and anthropogenic forces has major utility for predicting forest recovery potential and framing the growing body of research into liana-forest interactions.
Here we aim to stimulate vital research into liana thresholds, using current theory and empirical evidence to conceptualise the global response of forests to disturbance-induced liana proliferation. We begin by summarising the expected variation and scale of liana effects on forest ecology and management. We then introduce a working hypothesis regarding how lianas interact with disturbance to affect forest recovery, and how these interactions vary along environmental gradients. Finally, we determine the methods and measurements required to test the hypothesis and thus determine the presence, consistency and environmental controls on alternative forest recovery responses.
Primary forests are naturally dynamic, with frequent, non-catastrophic disturbances such as treefalls (Thompson, 2011; Ghazoul et al., 2015), where newly formed canopy gaps are soon re-filled by tree growth (Toledo-Aceves and Swaine, 2008; Tymen et al., 2016). While highly variable and data-limited, liana biomass in primary tropical lowland forests has mostly been estimated below 5–10% of total woody biomass (Schnitzer and Bongers, 2011; Van der Heijden et al., 2013). Global analyses show that liana abundance and biomass decline toward temperate ecosystems and high elevation (DeWalt et al., 2015; Fadrique and Homeier, 2016). The rate of liana biomass decline is approximately −0.18 Mg ha−1 per 100 m of elevation in tropical ecosystems, contributing <1% of above-ground woody biomass in upper montane forest (Fadrique and Homeier, 2016). In secondary tropical and temperate forests, lianas are typically more abundant than in primary forests and can be a natural part of succession, typically increasing over 30–70 years post disturbance, before declining (Capers et al., 2005; Letcher and Chazdon, 2009; Ladwig and Meiners, 2010; Letcher, 2015).
In secondary forests, data from Amazonia and Panama show that liana recruitment can increase by up to 5-fold and growth by 15-fold (Benítez-Malvido and Martínez-Ramos, 2003; Barry et al., 2015), and that liana biomass can approach 30% of total woody biomass (Gerwing and Farias, 2000). In extreme cases, lianas may even dominate the woody vegetation following temperate forest disturbance (Fike and Niering, 1999; Royo and Carson, 2006). Accordingly, in fragmented forests, liana abundance, and carbon stocks are positively associated with proximity to forest edge (Ladwig and Meiners, 2015; Magnago et al., 2017; Campbell et al., 2018) and land-use intensity (Jakovac et al., 2015). Liana abundance is also positively correlated with disturbance-induced canopy gaps (Schnitzer et al., 2000, 2015) and negatively correlated with the biomass of large trees on a global scale (Durán and Gianoli, 2013).
With at least 400 million hectares of secondary forest in the humid tropics alone (Asner et al., 2009) and lianas present in forests worldwide (DeWalt et al., 2015; Ladwig and Meiners, 2015), the potential geographic influence of lianas is huge. While liana abundance appears to vary across tropical regions (Box S1), insufficient data preclude comprehensive spatial projection of liana abundance or influence. However, the majority association of lianas with lowland tropical and subtropical forests is well-supported (DeWalt et al., 2015). Approximately 51% of tropical and subtropical forests (1,168 Mha) are moist lowland forests, suitable for lianas, comprising 64% of forest live carbon stocks (188 Pg) in this region, and respectively, 29 and 55% of forests and forest live carbon stocks worldwide (Figure 2). If disturbed forests are managed or naturally regenerate toward closed canopy, published estimates suggest a maximum live woody vegetation carbon stock potential of 217–244 Pg (calculations in Table S1) and hence up to 29–56 Pg carbon restoration potential across all disturbed forests (9–17% of the live global stock). This potential live carbon stock is equivalent to 23–31% of total global forest carbon budget, including live, deadwood, litter and soil carbon (861 ± 66 Pg) (Pan et al., 2011), albeit an upper estimate (Table S1). However, even these coarse estimates, plus occasional high abundance in temperate forests (Fike and Niering, 1999; Ladwig and Meiners, 2015) and at high elevation (Figure S1), show that lianas are associated with an extensive forest area of huge significance for the global economy and carbon budget. Improved estimates could be made through combining emerging liana research (Figure 1) with emerging forest restoration datasets (Bastin et al., 2019; Brancalion et al., 2019).
Figure 2. Expected location and live (above- and below-ground) woody carbon stock, of forest with conditions most favourable for lianas. This expected liana forest cover was derived from the ESA Climate Change Initiative (version v2.0.7; 2015; http://maps.elie.ucl.ac.be/CCI/viewer/index.php), land uses 50 (Tree cover, broadleaved, evergreen, closed to open [>15%]) and 61 (Tree cover, broadleaved, deciduous, closed [>40%]), −40 to +40 degrees latitude and ≤ 1,000 m elevation. Other forests are shown in grey, including land use 62 (Tree cover, broadleaved, deciduous, closed [>15%]), which comprised large areas of dry open woodland, unsuitable for liana proliferation. Carbon estimates sourced from Ruesch and Gibbs (2008).
During the past 50 years, data from the Neotropics show that lianas have increased across various scales (Schnitzer and Bongers, 2011), including the proportion of trees colonised by lianas (Ingwell et al., 2010; Toledo-Aceves, 2015), and abundance, biomass and flower/litter production of lianas relative to trees (Phillips et al., 2002; Wright et al., 2004; Wright and Osvaldo, 2006). Across all causes of mortality in moist tropical forests besides direct human influence, liana abundance (together with fire frequency) has the greatest rate of increase, exceeding changes in drought, climate change and biotic agents (McDowell et al., 2018). Lianas are expected to increase further globally under ongoing disturbance and climate warming, because these conditions are expected to favour liana physiological adaptations (Schnitzer and Bongers, 2011). Therefore, quantifying liana influence on forest ecosystem resilience is fundamental to predicting the structure and function of forests under global environmental change (Verbeeck and Kearsley, 2016).
From their various negative associations with trees, lianas have long been considered structural parasites, dependent on host trees for support (Putz, 1984b; Stevens, 1987; Ewers F. W. et al., 2015; Muller-Landau and Pacala, 2020). Multiple physical and physiological adaptations can allow disturbance-favouring liana species to proliferate (Figure 3), competing intensely with trees for light, nutrients, and water (Goriely and Neukirch, 2006). Lianas subsequently affect recovery of forest diversity, biomass, carbon uptake, structure, and function (Box 1). However, liana-tree interactions are highly variable and empirical evidence is often lacking to distinguish parasitism from resource-based competition (Stewart and Schnitzer, 2017). Some of the reported negative impacts of lianas on trees may be confounded by abiotic conditions favouring lianas that also covary with disturbance, e.g., high temperatures, humidity and exposure to wind (Magnago et al., 2017). Data showing negative liana influence are also biased toward neotropical lowlands, often with simple and inconsistent metrics (mostly diameter growth and rarely biomass) and timeframes mostly <5 years, with few data on their impacts on biodiversity, nutrient cycles, and productivity (Marshall et al., 2017). These negative influences therefore require greater understanding and consideration together with their essential contributions to forest functions (Box 1).
Figure 3. Liana proliferation forming “blankets”, “towers” and “cones” over secondary forest across the tropics, including (A) Tanzania [30–40 years post-logging], (B) Amazon Basin [repeated riverside disturbance], (C) Malaysia [15–20 years post logging], and (D) Australia [repeated cyclone damage], are fastened tight by various forms of armature and stem adaptations, such as (E) simple spines or thorns; (F) prickles on bosses; (G) paired recurved spines; (H) 3-branched thorns; (I) hooked spines; (J) tendrils. Photographs by ARM, except for B (with permission from Stefan Schnitzer) and C (MP).
Box 1. Liana adaptation and ecology.
Liana adaptation and ecology. Lianas are woody vines (Table 1) occurring in various plant families that usually also include trees and shrubs. Lianas have various growth guilds, including main stem twiners, branch twiners, tendril climbers, root climbers, and scramblers (Putz, 1984b). Liana growth forms are an adaptation to varying forest conditions (Ladwig and Meiners, 2015; Campbell et al., 2018), indicating that lianas also vary in their ecological functions. Multiple physical and physiological adaptations allow lianas to compete with trees, while also facilitating forest ecosystem function and thus, also conditions suitable for host trees, on which they depend.
(A) COMPETITION WITH TREES
Lianas are best known for growing over the top of trees, aided by an array of adaptations for climbing and clasping (Figure 3). Liana leaves are also lighter (Kazda and Salzer, 2000) and have greater area and biomass per unit stem size compared to trees (Putz, 1983). Hence lianas have more exposure to sunlight and presumably lower photosynthetic costs, while also exerting physical pressure on trees. Lianas also grow more rapidly than trees because less energy is required to develop structural support (Pérez-Salicrup et al., 2001). This rapid growth allows lianas to colonise canopy gaps quickly, further facilitated by increased seed rain, seedlings and side shoots compared to trees (Toledo-Aceves, 2015). Liana physical advantages also extend belowground, potentially including deeper, more extensive root systems and faster capillary flow than trees (Ewers et al., 1991; Restom and Nepstad, 2001). However, recent data from Costa Rica suggest that liana roots extend no deeper than trees (Smith-Martin et al., 2019). Further liana advantages among some species also include increased tolerance to herbivores (Ashton and Lerdau, 2008) and release of allelopathic chemicals (Ladwig et al., 2012). These various competitive advantages have collectively resulted in negative effects on tree growth, recruitment, fecundity, mortality (Schnitzer and Bongers, 2002; Parren and Bongers, 2005; Phillips et al., 2005; Ladwig and Meiners, 2009; Van der Heijden and Phillips, 2009) and wood density (Berenguer et al., 2018), with the most comprehensive data from Panama showing that trees with lianas have reduced population growth by 1.4% (Visser et al., 2017) and net annual carbon uptake by 76% (Van der Heijden et al., 2015). Lianas also disrupt water and nutrient availability through alteration of forest structure and through horizontal redistribution and transpiration during seasonal droughts (Schnitzer and Bongers, 2011). Because they connect adjacent trees, lianas also significantly increase canopy disturbance from treefalls (Vidal et al., 1997).
(B) BENEFITS TO FORESTS
Lianas comprise ~10% of tropical plant biodiversity (up to 44%) and on average around one fifth to one quarter of stem density in tropical forests (Gentry, 1992; Schnitzer and Bongers, 2002) and contribute ~15% of net primary productivity (Van der Heijden et al., 2013). Lianas also comprise up to ~10% of temperate woody plant floras, but with huge variation and very little understanding regarding productivity (Ladwig and Meiners, 2015). Liana leaf turnover is also typically higher than trees, contributing 16–40% of leaf litter in moist tropical and subtropical forests, making significant contribution to soil fertility and the carbon cycle (Tang et al., 2012). Lianas also provide animal food and movement pathways (Lambert and Halsey, 2015) and liana-dominated forest gaps are important for maintaining biodiversity (Schnitzer and Carson, 2001). Accordingly, lianas may enhance recovery from disturbance, including soil (Tang et al., 2012), seed diversity (César et al., 2017) and fauna (Ødegaard, 2000; Arroyo-Rodríguez et al., 2015), and may provide protection from fire (Uhl et al., 1988), lightning (Yanoviak, 2013), wind (Putz, 1984b), cyclones (Unwin et al., 1986), weeds (Goosem and Tucker, 2013) and herbivory (Odell et al., 2019). We collectively refer to these protective influences of lianas as their “bandage effect”. Growth on top of trees gives lianas abundant, prolonged access to the canopy of structurally-impacted forests. And yet while this secondary state has high light levels and temperature, beneath the lianas the forest floor remains shaded, humid, and maintains a healthy seed or seedling stock, thus preserving the forest ecosystem on which both lianas and trees depend. The bandage effect is consistent with recently discovered thermal buffering of forest understorey following logging (Senior et al., 2018), very feasibly resulting from rapid shading by lianas and other secondary vegetation. The resulting decelerated tree growth and hence prolonged understorey buffering may also give forests necessary time to develop structural and community complexity, that would be lost if lianas were outcompeted or cut too quickly.
Many lianas do not have significant adverse effects on their host trees, and indeed in some cases can facilitate forest recovery following disturbance (Box 1). Many lianas help to protect forests from extreme weather, fire, weed invasion, and herbivory (Campbell et al., 2015) resulting in a “bandage effect” that allows tree seedlings to survive and grow where they might otherwise die (Box 1). Large lianas are also an indicator of healthy primary forest in tropical lowlands (Clark, 1996), where liana tangles can also be crucial for threatened understorey animals (Michel et al., 2015). Some liana-dominated habitats are even protected as threatened ecosystems (Webb, 1959). For the purposes of our discussion, these non-harmful lianas are important to distinguish from liana functional types that have adverse effects on their host trees. From numerous cases of increased tree mortality and other adverse effects (Box 1), and regular liana-induced mortality at our own study sites (unpublished data), we consider these “harmful” lianas to be structural parasitoids (Table 1), hereafter shortened to “parasitoids”.
Understanding forest resilience through detection, description and prediction of thresholds assists identification of techniques and critical intervention points for forest management (Thompson, 2011; Cerullo and Edwards, 2019) and progress toward international forest restoration targets, e.g., the Bonn Challenge. For example, the forestry technique of liana cutting has shown promise for restoring secondary forests (César et al., 2016; Box S2). Selective hand-cutting of liana stems in tropical forests has improved soil moisture, tree growth, recruitment, survival and fruiting (Estrada-Villegas and Schnitzer, 2018). However, liana removal may have undesirable side effects by removal of the positive liana contributions to ecosystem function (previous section; Box 1). Accordingly, variation in liana ecology, abundance and function within the forest ecosystem requires consideration during management (Piovesan et al., 2018). Therefore, besides removal of exotics, liana cutting is only appropriate for excessively abundant, parasitoid species in heavily disturbed areas, where they are most likely to arrest succession (Schnitzer and Carson, 2001; César et al., 2016). Recent mixed success of liana cutting for improving tree growth and survival in Malaysia also suggests inconsistent applicability, including reduced effect during drought (O'Brien et al., 2019). Research is urgently needed to evaluate the broader applicability of liana cutting to avoid its misuse in forest management, and to determine its potential for recovering the global carbon sink.
We introduce a Liana Threshold Hypothesis, proposing that beyond critical thresholds of disturbance, lianas can impair forest resilience and slow or arrest forest recovery, varying according to climate and other biotic and abiotic conditions. Our hypothesis asserts that the impact of disturbance on forest recovery is proportional to disturbance-induced changes in liana competitive success over trees (hereafter “liana dominance”; Table 1) through direct impact on trees and stimulated liana growth. This aligns with previous speculation that lianas can be central to disturbance-induced “feedback” mechanisms (Jakovac et al., 2015; Table 1). We propose that these liana feedbacks play a significant and fundamental role in global forest dynamics. Our hypothesis is not limited to tropical and subtropical climates, because lianas also influence community dynamics in all temperate regions (Ladwig and Meiners, 2015), sometimes affecting tree growth (Ladwig and Meiners, 2009) and even proliferating and stalling forest recovery where disturbance and climate conditions align (Fike and Niering, 1999; Royo and Carson, 2006).
Forest succession, e.g., secondary–primary forest transition, is highly variable (Arroyo-Rodríguez et al., 2017). We position our threshold hypothesis within a broad classification of forest recovery from disturbance as rapid, slow, or arrested relative to baseline rates (Ghazoul and Chazdon, 2017). We conceptualise linear, threshold and hysteretic forest ecological responses to environmental change, described for other ecosystems (Bestelmeyer et al., 2011; Ratajczak et al., 2018) and propose five potential forest recovery response classes (Figure 4).
Figure 4. Hypothesised forest recovery response classes following disturbance-induced liana growth (see section above, Five Forest Recovery Response Classes). We visualise forest responses using (A) response curves, based on indicators of forest state, i.e., measurements of forest condition/function, and (B) stability diagrams, showing conceptual variation in “basins of attraction” (see glossary, Table 1). Column I (solid lines)—Threshold Response Class: This hypothesises that the forest response after heavy disturbance and under high competitive success of lianas relative to trees (liana dominance) is abrupt and non-linear and gravitates toward a persistent secondary state indicating arrested succession. Column II (solid lines)— Pseudo-threshold Response Class: This hypothesises that the forest response to disturbance and liana dominance follows a smooth non-linear trend and results in an unstable secondary state indicating positive but delayed recovery. Column III—Linear Response Class: This hypothesises that the forest response to disturbance and liana dominance is straight-linear and results in a highly unstable secondary state, indicating steady recovery. Columns I and II also illustrate alternative, more resistant, Hysteretic and Pseudo-hysteretic Response Classes, which hypothesise that the response of forest state indicators during disturbance and increasing liana dominance may follow threshold or pseudo-threshold trajectories, respectively, but after disturbance has ceased (dashed lines) they may follow more resistant response pathways with more persistent secondary states. Green and red balls, respectively indicate the mean expected primary and secondary traits in forest condition/function. Black shading = high variability in primary forest, resulting from natural dynamism (Ghazoul et al., 2015) and forest indicator variation (Gower et al., 1996; Sheil and Burslem, 2003). Figures are developed from established classes of ecological response to environmental change (Andersen et al., 2009; Bestelmeyer et al., 2011).
Observed responses are categorised under a Threshold Response Class when indicators of post-disturbance forest state, i.e., the condition or function of a forest, follow an abruptly non-linear relationship with liana dominance (Figure 4). In this first response class, recovery is arrested beyond a critical threshold. Once correctly diagnosed (see section below, Identifying Liana Thresholds), the presence of this threshold would infer that lianas are acting as “positive feedbacks”, i.e., following disturbance, they are preventing net tree growth and maintaining the secondary forest state. While liana positive feedback thresholds between primary and secondary forest remain untested, they can be indirectly inferred from repeated observations of enhanced tree growth without lianas at multiple tropical locations (Marshall et al., 2017). Further inference can be made from persistence/expansion of low-stature liana dominated patches, with tree growth stalled for years or decades, in both tropical and temperate forests (Fike and Niering, 1999; Schnitzer et al., 2000; Royo and Carson, 2006; Foster et al., 2008; Tymen et al., 2016).
Many tropical and temperate forests show steady recovery of tree biomass and/or biodiversity following total or near-total removal of trees (Martin et al., 2013; Poorter et al., 2016; Meli et al., 2017). This steady recovery can be irrespective of concurrent liana growth in the first 10-20+ years (Letcher and Chazdon, 2009; Lai et al., 2017). Such observations would be classified as a Linear Response Class (Figure 4) and yet appears contrary to consistently reduced tree growth with liana colonisation (Marshall et al., 2017). A possible mechanism is that forests recovering from complete tree clearance, i.e., deforestation (as opposed to disturbance; Table 1), would initially lack host tree structural support essential for liana growth, hence precluding the positive liana feedbacks central to a threshold response. Accordingly, limited lowland neotropical forest data show that ratios of liana to tree biomass and basal area remain below 2% for the first 2-4 years of forest succession from abandoned land, before increasing (Feldpausch et al., 2005; Barry et al., 2015; Lai et al., 2017).
Many recovering forests do not begin to show obvious recovery of trees until several years after abandonment (Ladwig and Meiners, 2010; Poorter et al., 2016). Forest recovery from heavy disturbance (and liana proliferation) may be delayed and then begin slowly, initially undetectable from a persistent secondary state (Ghazoul and Chazdon, 2017). We define this third recovery response into a Pseudo-threshold Response Class, in which a threshold exists, but with an unstable secondary state (Figure 4), where forest tree recovery is both nursed and hampered by other regenerating vegetation. Negative feedbacks from liana bandage effects would exceed positive feedbacks from liana parasitoid effects, resulting in net tree biomass gain. Accordingly, while tropical forest tree growth is consistently higher without lianas, where lianas are present, tree growth is marginally positive (28 studies) more often than zero or negative (3 studies) (Marshall et al., 2017).
Finally, long-lasting or frequent disturbance might also stimulate a Hysteretic Response Class (Figure 4), where forest recovery would require a relatively greater push (e.g., through restoration management) that exceeds the initial disturbance. Hysteretic responses are well-established for forest-savanna-grassland transitions caused by drought (Van Nes et al., 2014). Similarly, hysteresis might also occur under a Pseudo-threshold Response Class (Pseudo-hysteretic Response Class; Figure 4). Disturbances under this fifth response class would induce stronger positive liana feedbacks than a pseudo-hysteretic response, while still outweighed by negative feedbacks from liana bandage effects, resulting in even slower, but still positive, recovery.
Observed variation in forest recovery rates provides compelling evidence of variable impacts of disturbance and liana proliferation. We expect that variation in liana dominance along environmental gradients will modify forest recovery pathways, affecting trade-offs between positive and negative influences of lianas on forest ecosystems (e.g., bandage and parasitoid effects). We suggest that positive feedbacks between liana growth and disturbance, and hence non-linear (threshold or hysteretic) forest recovery, most likely occur under biotic and abiotic conditions favouring liana growth relative to tree growth, assuming that these conditions do not exclude host trees entirely. This expected variation is illustrated in the graphical representation of our Liana Threshold Hypothesis in Figure 5.
Figure 5. Liana Threshold Hypothesis, proposing that post-disturbance forest states are dependent on human, biotic, and abiotic drivers (possible examples for moist forest to the left) that are expected to influence positive liana feedbacks. The hypothesis is illustrated using stability diagrams (A–E) and their associated forest state indicator response curves (insets) as described in Figure 4. Following heavy disturbance at sites where drivers favour trees over lianas, a Linear Response Class is expected (A), where the resulting post-disturbance state is unstable, and hence forest recovery can gradually proceed unimpeded. However, the post-disturbance state is expected to become progressively more stable as drivers tend toward favouring lianas over trees, from (B) Pseudo-threshold, to (C) Pseudo-hysteretic, to (D) Threshold, to (E) Hysteretic Response Classes.
Current understanding of conditions that regulate liana dominance is limited, with more data needed from understudied tropical regions (Schnitzer et al., 2016). Available data on liana growth and geographic range suggest that high liana abundance and competitive vigour (and hence also non-linear forest recovery pathways) are most feasible in moist forests (Durán et al., 2015) under high seasonality and long dry season length (DeWalt et al., 2015; Schnitzer, 2018) and local scale disturbance-induced increases in water stress and light (Capers et al., 2005; Dupuy and Chazdon, 2006; Jirka et al., 2007; Schnitzer and Bongers, 2011; Magnago et al., 2017). In addition, liana growth and/or abundance correlate positively with elevated CO2 (Granados and Körner, 2002), windspeed (Magnago et al., 2017) and probably also mammal herbivory (Omeja et al., 2014; Luskin et al., 2019) and fire (Gerwing, 2001) although findings are inconsistent (Wright et al., 2007; Balch et al., 2011). Recent data, whilst limited (Fadrique and Homeier, 2016), show a positive influence of soil fertility on liana growth relative to trees (Lai et al., 2017).
Where conditions are not favourable to liana proliferation, liana impact on forest recovery would be minimized, with forest recovery more likely tending toward a pseudo-threshold or linear response (Figure 5). Liana growth and/or abundance correlate negatively with increasing mean annual rainfall (DeWalt et al., 2015), frost (Jiménez-Castillo and Lusk, 2013) and successional stage (Capers et al., 2005), the latter more likely driven by canopy closure (Ingwell et al., 2010). Lianas also decrease in abundance with increasing latitude, drought (DeWalt et al., 2015), elevation (Fadrique and Homeier, 2016) (Figure S1), and with distance inside forest edge (Schnitzer and Bongers, 2011; Campbell et al., 2018). Liana cutting experiments also suggest reduced liana vigour at high elevation (Chapman and Chapman, 1997), presumably because of cool, aseasonal and wet climates, and also in small clearings that lack full daylight (Toledo-Aceves and Swaine, 2008; Van der Heijden and Phillips, 2009). Under these conditions, liana removal stimulated little or no improvement in tree growth.
Climatic and biogeographic variation in liana and tree species community composition further affects liana vigour and tree vulnerability, and hence also variation in forest recovery. While liana species vary in their climbing strategies and tendency to proliferate and affect trees (Box 1), tree species vary in their vulnerability to liana proliferation and competition (Toledo-Aceves, 2015; Muller-Landau and Visser, 2019). Fast-growing, pioneer tree species have evolved physical adaptations to shed lianas (Putz, 1984a) and have been shown to respond more positively to liana removal than shade-tolerant species (Schnitzer and Carson, 2010). Primary forest species also endure high liana loads through adaptations to cope with mechanical stress and low light, water and nutrients, prompting speculation that perceived greater liana tolerance among pioneer species may be incorrect (Visser et al., 2017; Muller-Landau and Visser, 2019). However, this contrasting observation was derived from data exclusively from undisturbed forest, where pioneer species are at a competitive disadvantage.
Lianas predominate over other disturbance-favouring plant forms in moist tropical lowland secondary forests, which have greater carbon density and land area than any other forest type (Pan et al., 2013). No other plant form impacts trees from understorey to canopy. However, the variability in liana influence on forest recovery responses also depends on interactions with disturbance-induced feedbacks from other forms of secondary vegetation. For example, the disturbance-favouring niche can be occupied by ferns (Kessler, 2010), bamboo (Griscom and Ashton, 2006), shrubs (Jiménez-Castillo et al., 2007), or grasses (Van Nes et al., 2014), particularly at high elevations and latitudes, or in degraded forests. These plant forms can be common and widespread, e.g., bracken fern (Pteridium aquilinum) is found on every continent except Antarctica and inhibits forest succession through soil deterioration and shading, and by facilitating seed predation (Ssali et al., 2018, 2019). Grass is widely implicated in thresholds between forest and savanna through fuelling wildfires (Van Nes et al., 2014). Positive feedbacks from bamboo may even rival those from lianas in some lowland tropical forests, supplementing or supplanting liana effects on woody regrowth (Griscom and Ashton, 2006; Carneiro et al., 2017). Finally, non-native species are also pervasive invaders in disturbed forests, including hundreds of liana species (Harris and Gallagher, 2011), where their impact on tree growth can far exceed that of native secondary vegetation (Fike and Niering, 1999).
Our hypothesis predicts that forest recovery varies between alternative response classes, supported by mounting evidence (see section above, Five Forest Recovery Response Classes). Alternatively, forests may simply vary in their rate of recovery, with variable disturbance and liana dominance (under the influence of biotic and abiotic conditions) solely leading to variation in the slope of forest recovery response curves. In the absence of a threshold under this alternative hypothesis, forest recovery response curves would be approximately linear (or at least non-abrupt).
Adapting an existing framework (Bestelmeyer et al., 2011), we propose three data elements that will be central to characterising recovery dynamics of disturbed forest systems in the context of liana thresholds (Figure 6): (1) the biological response; (2) drivers; and (3) response mechanisms (Table 1). The first central component, the biological response, comprises temporal changes in important biological features, e.g., forest structural/species reorganisation. The biological response is measured using indicator variables, which for liana thresholds would include indicators of forest state (Figures 4, 5; Biological Response section, below).
Figure 6. Three data elements for identifying and describing ecological thresholds in forests (drivers, response mechanisms, and biological response; bold italic type), are expected to form a dynamic causal loop system, maintained by liana feedbacks, under scenarios of pervasive forest decline (peach arrows), recovery (green arrows), and stasis (white arrows).
The biological response depends on variation in one or more drivers, i.e., factors that cause ecosystem change. These drivers may directly affect the biological response, or indirectly, e.g., through their effect on lianas. The drivers may be environmental, i.e., the ultimate abiotic and biotic (including human) influences, or contextual, i.e., less predictable site- or time- specific proximate influences on the environmental biological response relationships, including topography, land-use history and human/biological interactions. “Pulses” or “triggers” can also occur, i.e., abrupt events or changes in one or more drivers that directly cause a biological response. The primary driver of liana proliferation is increasing light transmittance resulting from declining tree canopy cover following heavy forest disturbance (see section above, Variation Between Forest Recovery Response Classes; Figures 5, 6). Conversely, decreasing light in secondary forests, e.g., resulting from targeted management or naturally closing forest canopies, drives liana reduction and forest recovery (Capers et al., 2005).
The third data element, the response mechanisms, encompasses interactions between the drivers and positive feedbacks inherent to a threshold, e.g., accelerated liana growth relative to trees (or vice versa), stalling (or stimulating) recovery. This means that while the biological response and drivers may be measured directly (next section) and thresholds (and other response classes) may be inferred directly from these measurements (see Identifying Liana Thresholds, below), response mechanisms require experimental and modelling approaches (see Identifying Response Mechanisms, below).
Adequate representation of all data elements is required to understand the interactions that shape the forest recovery response (response curves, Figure 4). Where feasible, direct measurement of data elements will provide greater accuracy than coarse or interpolated data. Data elements must be sampled over sufficient time, often decades, to assess the full transition between secondary and primary forest states (see Identifying Liana Thresholds, below) and the underlying response mechanisms (see Identifying Response Mechanisms, below). Chronosequences might provide a more realistic alternative for identifying response classes over much shorter time frames (Lennox et al., 2018). Regardless, field sampling must employ a sufficiently orthogonal experimental design, i.e., sampling across gradients of all expected major drivers.
Multiple forest state indicators can be used to build a composite picture of the biological response to liana feedbacks, either individually or through multivariate techniques (Eason et al., 2016). First, above-ground net primary production (ANPP), the most commonly used measure of forest productivity, is calculated from highly comprehensive repeat forest stand measurements, as the sum of biomass increment plus losses from mortality, herbivory, leaching, and volatilization (Clark et al., 2001). More practical proxies for ANPP include rates of litterfall for short life span tissues and basal area or diameter increments for long life span tissues (Kloeppel et al., 2007). The latter increments are widely used to measure forest recovery and degradation (Ghazoul and Chazdon, 2017), sometimes combined with stem recruitment, minus mortality (net biomass gain) (Marshall et al., 2017). Given the positive relationship between productivity and biodiversity (Liang et al., 2016), our hypothesis applies equally to tree productivity, forest indicator plant and animal species/communities, and to variation in biodiversity-productivity relationships. While ANPP showed no relationship to liana infestation at a site in French Guiana, biomass turnover was much faster in liana-infested forest than in and primary forest (Tymen et al., 2016). However, both net biomass and net basal area gains have been observed following liana removal from three neotropical and African sites (Marshall et al., 2017). Tree species richness has also responded positively to liana removal (Schnitzer and Carson, 2010), however more data are required for late-successional species (César et al., 2016), which have responded inconsistently (Martínez-Izquierdo et al., 2016; Visser et al., 2017).
The natural dynamism of primary forests leads to high variability in species richness and biomass, and these forests often have lower biomass accumulation and species richness than forests of earlier successional stages (Gower et al., 1996; Sheil and Burslem, 2003) (Figure 4). Hence forest state indicators may also include species composition (e.g., functional groups), age/canopy structure, soil nutrients, and seed stocks (Ghazoul et al., 2015), with the latter two particularly important for representing degradation and ecological memory (Ghazoul and Chazdon, 2017). Metrics to capture the complexity of succession and degradation may also include functional processes, e.g., fecundity or phenology (seed/fruit/flower production), wood density, species turnover and biotic interactions (Rapport, 2007; Ghazoul et al., 2015; Ghazoul and Chazdon, 2017; Berenguer et al., 2018). In addition, both soil and forest canopy functioning are emerging as essential variables for understanding global climate change science, particularly soil carbon and moisture, leaf area index and fraction of absorbed photosynthetically active radiation (Global Climate Observing System, 2016).
Our hypothesis is mostly applicable to biological responses associated with seedlings and saplings, because secondary forests have few large trees (Swanson et al., 2011) and lianas have shown a more consistent influence on the growth and architecture of fast-growing, pioneer species (Putz, 1984a; Duncan and Chapman, 2003; Schnitzer and Carson, 2010; Visser et al., 2017) (although see section above, Variation Between Forest Recovery Response Classes). Therefore, liana thresholds are most likely for small trees establishing themselves in direct sunlight. Biological response data from tree and liana stems below 10 cm diameter (and small vs. large stem size class structure) are thus very important and yet the majority of forest recovery research has focussed on stems 10 cm diameter and larger (Berenguer et al., 2018). The influence of lianas on this biomass and biodiversity recruitment has seldom been addressed, but limited tropical data suggest that tree biomass recruitment (Marshall et al., 2017) and wood density (Berenguer et al., 2018) are higher without lianas. Investigating succession and structural/spatial complexity in early-successional forests is also vital for understanding and restoring their biodiversity legacy (Swanson et al., 2011).
Informed selection and measurement of drivers will help to explain response mechanisms and their variation, and to reduce inherent noise associated with thresholds. These drivers will need to represent the major biotic and abiotic influences on liana abundance, size, and vigour. Besides established measures of water availability (e.g., precipitation, annual moisture index, potential evapotranspiration, and seasonality), water deficit is emerging as an important predictor of forest functioning (Tng et al., 2018) and structure (Pfeifer et al., 2018). Moreover, within the moist forests suitable for lianas, disturbance is the primary driver of liana proliferation and competitive advantage over trees (see section above, Variation Between Forest Recovery Response Classes; Figure 5), hence the most crucial driver measurements will be rates and/or quantities of tree-cutting/wind damage, and/or directly proportional changes in canopy openness or light levels. Other expected drivers include temperature, soil nutrients, herbivores, fire and community variation in tree and liana species and functional groups (see section above, Variation Between Forest Recovery Response Classes; Figure 5).
Forest tree abundance and size also positively affect the abundance of lianas, e.g., empirical models of tree height structure accurately predicted liana coverage in West Africa (combined with other vines and weeds) (Cazzolla Gatti, 2018). Accordingly, tree size is the major predictor of global forest dynamics (West et al., 2009). Thus biological response (forest state) indicators may influence one another. In particular, “fast” indicators, e.g., recruitment, that are directly related to succession and forest recovery, are dependent on “slow” indicators, e.g., soil organic matter, which control the impact of drivers (Walker et al., 2012). Hence potential influences on liana competitive success also need to be identified among the forest state indicators, albeit not defined as drivers in the strict sense (Table 1). These two-way interactions between the biological response and drivers, and also between lianas and trees, further emphasise the circularity of forest dynamics (with interdependency between parasitoids and hosts, and drivers and feedbacks pushing simultaneously toward and away from recovery) and the importance of experimentation and modelling for determining directionality.
In order to determine the presence of positive liana feedbacks in the response mechanism (see Identifying Response Mechanisms, below), liana dominance must be measured, e.g., the ratio of liana biomass (or stem counts) to trees (L:T) across space or time (Phillips et al., 2002; Jones et al., 2017; Lai et al., 2017; Magnago et al., 2017). Measuring and counting lianas is not straightforward, because climbing plants have both horizontal and vertical growth, through or over other plants, often with multiple stems (Schnitzer et al., 2008). For this reason, liana abundance and size are considerable knowledge gaps in global forest surveys. Of the 1.2 million documented forest inventory plots across the world, <1% have liana records (Liang et al., 2016; Steidinger et al., 2019). Estimating liana biomass is therefore problematic and currently relies on formulae derived from just a few hundred, primarily neotropical samples (Schnitzer et al., 2006; Miao et al., 2016). Most studies have instead used liana diameter or basal area to quantify growth (Marshall et al., 2017) and stem number to quantify abundance (Durán and Gianoli, 2013).
Liana assessment has traditionally required direct measurement by hand, but the immense challenge of conducting fieldwork in liana thickets and the imprecision of allometric equations may be alleviated using terrestrial laser scanning (LiDAR) to estimate 3-D structure beneath the thick mat of vines (Sánchez-Azofeifa et al., 2017; Moorthy et al., 2018). Subsequently, rapidly developing algorithms can facilitate extraction of tree and liana structural attributes from the complex point clouds of LiDAR data (Moorthy et al., 2019). These can then be used directly to parameterise vegetation models or to validate other methods.
The L:T-biological response relationship may become circular and some statistical patterns will be inevitable (including non-linearity; Magnago et al., 2017), potentially confounding threshold measurement. This is because forest biological response (forest state) indicators will often comprise tree biomass, abundance and/or productivity (see Biological Response, above), which are the core components that determine L:T. L:T will also not fully represent liana competitive success over trees, because even small lianas can reach the forest canopy and their competitive effect per unit biomass is greater than for trees (Tobin et al., 2012). A less circular measure of liana competition is the crown occupancy index (COI) (Clark and Clark, 1990). However, COI only measures canopy competition, thus ignoring important below-ground (root) competition (Van der Heijden et al., 2010). Furthermore, COI varies with (1) seasonal leaf loss, because many lianas are deciduous, and (2) the emergence and disappearance of annual vines, particularly in secondary forests where these annuals reach the upper branches of most trees, superficially resembling lianas. COI is also typically measured on a 5-point ordinal scale and consequently has limited potential for continuous scaling required for measuring thresholds. Proportion liana coverage of the forest canopy (load; Muller-Landau and Visser, 2019), tree stems (prevalence; Campbell et al., 2018; Visser et al., 2018) or vegetation plots (Cazzolla Gatti, 2018) may be more versatile than COI, potentially capitalising on emerging unmanned aerial vehicle technology (Waite et al., 2019). Importantly, many of the ground-based measures do not correlate (Cox et al., 2019) and biases still remain in the aerial approaches (Sánchez-Azofeifa et al., 2017). Therefore, multiple measures may be required alongside tree measurements, e.g., to produce a composite index of liana dominance.
While subsequent data elements can be sampled by careful stratification, measurement of liana dominance may require cutting trials to adjust L:T ratios to ensure sufficient spread for determining the effect of variation on forest recovery. While experimental manipulations are often difficult in ecological systems (Magnuszewski et al., 2015), procedures for liana cutting trials are well-established (Estrada-Villegas and Schnitzer, 2018). Inclusion of controls with no manipulation (before-after control-impact; Stewart-Oaten et al., 1986) across important driver gradients, will ensure that outcomes have arisen from liana competition rather than other positive feedbacks and drivers. While this approach is largely standard, the challenge will be to cut lianas at varying intensities, rather than the standard, dichotomous, cut versus uncut approach.
Description of forest response mechanisms will also require measurement of potential alternative positive feedbacks, so that liana feedbacks can be isolated. For instance, measurements or controls may be needed within the same forest stands as liana measurements, to determine relative abundance of other forms of secondary vegetation and disturbance-induced changes in microclimate, soil health, and leaf herbivory. Similarly, opposing feedbacks from parasitoid or bandage tendencies between different liana species or growth guilds may again be inferred from the direction and magnitude of biological response over time, chronosequences, or experimental manipulation. Therefore, identification of liana species and growth guilds in any ground-sourced forest inventories would permit assessment of their relative roles in forest recovery responses and hence also their relative importance for management.
The importance of measurement or experimental control of potential positive feedbacks also applies to animal community or abundance changes following forest disturbance, which are often overlooked in forest dynamics, despite their functional importance (Wright et al., 2007). Disturbance can lead to shifts in forest resilience in response to feedbacks from invertebrate declines (Ewers R. M. et al., 2015). The consequences of invertebrate declines for lianas are poorly understood, but subsequently altered litter decomposition, seed and invertebrate predation, and pollination, will have uncertain consequences for liana-tree competition and forest recovery. Similarly, disturbance to forest canopies is often accompanied by other forms of potential disturbance feedbacks, including defaunation from hunting that removes a functional component vital for recovery, further impairing seed predation and dispersal (Wright et al., 2007). Conversely, in the absence of hunting, canopy disturbance may instigate feedbacks from large animals, e.g., savanna elephants and pigs, that appear to maintain open canopy forest suitable for lianas (Marshall et al., 2012; Omeja et al., 2014; Luskin et al., 2019).
Liana thresholds cannot be determined by a single statistical analysis. The principle challenge for identifying thresholds is to determine true (state) thresholds from false (driver) thresholds. These driver thresholds are where non-linear biological responses have arisen from abrupt changes in one or more drivers, rather than from positive feedbacks (Van Nes et al., 2016). Therefore, the emphasis of liana threshold detection will be to identify a non-linearity in the response curve, i.e., the relationship between liana dominance measurements and forest state indicators (Figure 7C). Additionally, to rule out a driver threshold, liana dominance should increase approximately linearly (Figure 7B) or at least not abruptly. Concurrently, the change in forest state indicators should be abruptly non-linear (Figure 7A). The direction of these temporal relationships would then be expected to reverse under a scenario of forest recovery (Figures 7A,B).
Figure 7. Theoretical application of five established figures for diagnosing thresholds (Bestelmeyer et al., 2011) to distinguish between alternative forest response classes under liana proliferation following heavy disturbance. Peach and green shading, respectively, show phases of disturbance and recovery (A,B,D), or primary and secondary forest (E). While an abrupt change in the “biological response”, i.e., forest state indicators, over time (A-I) might be indicative of a Threshold Response Class, this would have to be driven by a non-abrupt increase (or experimentally manipulated variation) in liana dominance (B-I) to indicate a true threshold. Consequently, the response curve (C-I, from Figure 4) should then show an abrupt non-linear relationship. This assumes that other drivers remain constant over the same time period. Further verification of a threshold could be identified from increased variance in forest state indicators during and immediately prior to the threshold in (A-I, D-I) and from a bimodal frequency of forest state indicators across the study landscape (E-I). In the absence of a threshold, liana dominance and forest state indicators would be expected to covary under a Linear Response Class (C-II, from Figure 4), and hence show similar trends over time (A-II and B-II), or would show no relationship at all if lianas are not driving forest state change (not illustrated). Similarly, in the absence of a threshold, forest state indicators would be expected to show no temporal change in variance (D-II) and a unimodal frequency distribution (E-II). Black dashed lines further indicate expected trajectories under a Hysteretic Response Class. Red dashed lines indicate a false (driver) threshold whereby an abrupt change in forest state indicators (A-II) has resulted from an abrupt change in liana dominance (B-II). For simplicity, expected variation in state indicators is not shown, plus disturbance and recovery phases are shown as equal in duration, but this is highly unlikely.
Threshold identification requires “breakpoint” (or “change-point”) techniques to detect abrupt changes in both drivers and the biological response (Andersen et al., 2009; Bestelmeyer et al., 2011). The greatest variance would be expected on either side of any threshold along the recovery trajectory over time (Figure 7D). Thus, the breakpoint method determines temporal (or spatial) variation in residual sums of squares (e.g., F statistics) to identify the maximum ratio of variances in consecutive years using inferential methods (Quandt, 1958; Andersen et al., 2009). Analysis of such time-series typically requires autocorrelation adjustments to account for time-lags (Dutilleul et al., 1993) and detrending adjustments to decouple short- and long-term temporal trends (Bestelmeyer et al., 2011).
Using similar breakpoint approaches, an increase in variance before a threshold is reached has potential for predicting forest ecosystem collapse (Carpenter and Brock, 2006; Scheffer et al., 2009). Increasing variance can be a result of two phenomena that may occur close to thresholds: (1) critical slowing down, i.e., slow recovery from disturbance; and/or (2) flickering, i.e., biological response indicators switching back-and-forth between two states (Scheffer et al., 2001). Therefore, monitoring of forest state indicator variance in the early stages of disturbance and liana increase may allow prediction of imminent loss of resilience. However, both modelling and empirical approaches have shown that variance changes are not sufficiently consistent for either threshold prediction or retrospective diagnosis (Moore, 2018), hence the response curve and breakpoint approaches are likely to be more reliable.
Assuming that a threshold has been detected, continued analysis after forest disturbance has ceased or across a chronosequence of forest recovery (Figure 7C, and recovery phases of Figures 7A,B), could then determine whether a hysteretic, pseudo-threshold, or pseudo-hysteretic response has occurred (Figure 4). From this analysis, a Hysteretic Response Class would be indicated by reduced forest state indicator values relative to liana dominance, slower recovery of these indicators over time, and potentially also increased variance compared to the disturbance phase (Bestelmeyer et al., 2011) (Figures 7A–D). Further temporal (or chronosequence) data from secondary forests will be required to distinguish persistent secondary states from subtle recovery under our proposed pseudo-threshold and pseudo-hysteretic responses. A Pseudo-threshold Response Class would be indicated by immediate but very slow recovery of forest state indicators, initially undetectable, but with rapid recovery occurring once the threshold level of liana dominance is reached as identified in the disturbance phase. A Pseudo-hysteretic Response Class would mirror the pseudo-threshold response, but with reduced forest state indicator values relative to liana dominance and slower recovery of these indicators over time. Pseudo-threshold and pseudo-hysteretic responses are not illustrated in Figure 7, as their distinction from threshold and hysteretic responses would be very subtle.
Infrequent occurrence of forest stands with state indicator values intermediate between those of primary forest and liana dominated secondary forest can be used as further evidence of a liana-induced threshold response (Figure 7E). This expectation arises from the steep gradient at the threshold value in the driver- (or liana-) forest state indicator relationship, showing that transition between secondary and primary forest states is rapid. Therefore, any forest ecosystem recovery following two alternative trajectories is consistent with a threshold response (Bestelmeyer et al., 2011). Breakpoint analysis or statistical testing of unimodality (Hartigan and Hartigan, 1985) could identify weak (threshold) or pronounced (hysteretic) maxima (i.e., bimodality) in the frequency distribution of the forest state indicators (Figure 7E). However, previously proposed (fast/stalled) liana-driven successional pathways (Schnitzer et al., 2000; Cazzolla Gatti et al., 2015; Tymen et al., 2016), have been derived exclusively from dichotomous study designs (open vs. closed canopy forest; high vs. low liana abundance; cut vs. uncut lianas) and hence frequency distributions have so far not been assessed. A dichotomous approach to liana cutting experiments would also be expected to yield a false driver threshold, regardless of the recovery response class, in that tree biomass (and other forest state indicators) would be expected to recover abruptly in response to the abrupt removal of lianas.
Assessment of liana thresholds would be complicated where disturbance and subsequent liana proliferation has been rapid, e.g., through clear-felling of trees. This is because there would not be sufficient spread of driver, liana dominance or forest state indicator data to model the shape of the required relationships, nor to determine whether the response has resulted from a driver threshold. In this case, data would subsequently be needed in the recovery phase to determine whether a non-linear relationship appears. Alternatively, a complete lack of recovery would indicate a threshold that has resulted from a pulse driver (see section above, Selecting and Quantifying Data Elements) (Bestelmeyer et al., 2011; Ratajczak et al., 2018). However, a lack of recovery following liana decline seems unlikely, given the very consistent tendency of forests to recover when lianas are absent (Marshall et al., 2017). Therefore, we would only expect a complete lack of recovery where forest has been completely degraded (Table 1) and thus the essential properties for forest recovery have been removed, i.e., not a result of lianas per se, and not within the scope of this paper.
While liana thresholds (and other response classes) may be identifiable using the methods in the previous section, the final challenge will be to diagnose how they have arisen, testing for the effects of multiple, interacting drivers and feedbacks. The complex interactions that comprise the response mechanisms are the least understood data element of forest thresholds (Reyer et al., 2015). Threshold relationships between liana dominance and forest state indicators do not necessarily indicate positive feedbacks or causation. Forest state indicator responses may not be immediate, and evidence from biological responses in other ecosystems suggests that they will be affected by mean, variance, frequency and duration of change in drivers (Ratajczak et al., 2018) and interactions between them. High measurement error and multiple driver and feedback interactions can even mute the biological response such that variance changes are undetectable (Scheffer et al., 2009; Bestelmeyer et al., 2011).
In simple cases, the response mechanism may be determined by additional analysis to demonstrate that potential confounding drivers are unrelated to the liana dominance—forest state indicator relationship, thus demonstrating cause-effect. For example, following observation of a threshold relationship between liana abundance and tree productivity, a study might conduct a secondary analysis to show that tree productivity is consistently positive for stems not structurally parasitised by lianas at the same locations, thereby excluding environmental bias from climatic and topographic drivers. In addition to controlling for environmental bias, sampling and analyses can also be implemented to control for liana dominance, through controlled liana cutting to ensure sufficient variation in liana abundance (see Positive Feedbacks, above). However, where there are multiple drivers, large-scale experiments will be crucial for understanding and projecting forest ecosystem responses to change (Fayle et al., 2015), including thresholds and hysteresis (Scheffer and Carpenter, 2003; Carpenter and Brock, 2006; Ratajczak et al., 2018) and liana proliferation (Piovesan et al., 2018).
Multivariate analyses, with covariates or random effects to control for environmental and spatial biases, can be used together with data from field experiments to investigate thresholds under a more complete range of environments (Scheffer and Carpenter, 2003; Medlyn et al., 2015; Ratajczak et al., 2018). A range of regression and mixed-effects techniques were used to demonstrate the relative influence of lianas and soil properties on tree growth and biomass dynamics in Panama (Lai et al., 2017). However, while these unidirectional approaches to modelling thresholds can describe the basic relationships, conclusions are vastly improved through combined use with more complex empirical models (Moore, 2018).
Liana-forest dynamics are complex in that: (1) both lianas and trees are influenced by multiple drivers and feedbacks; and (2) lianas and trees influence one another. Further evidence suggests that liana-tree interactions can also be nested, in that species interactions increase from lianas to their hosts (Sfair et al., 2010). These are multivariate, multi-directional, interactions, best understood through a complex systems approach. Forest recovery response classes and underlying response mechanisms could be unravelled using mechanistic models, i.e., models that assume that complex systems can be explained from their component parts, and can be parameterised and validated using field observation and experimental data (Scheffer et al., 2001). The challenge will be to identify all mechanistic components (biological response, drivers, feedbacks) that interact to produce the real-life dynamic forest system of disturbance, stability and recovery (Figure 6).
A dynamic model of land conversion vs. forest recovery, using literature-derived data on agricultural productivity and fallow recovery from multiple regions, showed that forest recovery can operate around Threshold or Hysteretic Response Classes with two stable states dependent on agricultural pressure and land-use history (Magnuszewski et al., 2015). A West African forest dynamic model further found two basins of attraction (i.e., alternative stable states) determined by canopy structure influence on weed and vine cover (Cazzolla Gatti, 2018). Both the multi-region and West African studies were pioneering in that they demonstrated a threshold between degraded and recovering forest. However, these models were limited in their inclusion of drivers (one included soil quality alone; Magnuszewski et al., 2015) and biological responses [only including landcover change (Magnuszewski et al., 2015), tree height structure and species composition (Cazzolla Gatti, 2018)] and also did not include direct focus or measurement of lianas or other potential feedbacks.
Mechanistic, individual-based dynamic vegetation models (DVMs) of stem increments, productivity and species composition have consistently predicted non-linearities between forest dynamics and competition for light (Purves and Pacala, 2008). However, while individual-based forest growth models have existed for decades, lianas have rarely been explicitly included (Verbeeck and Kearsley, 2016; Muller-Landau and Pacala, 2020). A review of 15 DVMs, none including explicit information on lianas, showed that prediction of tree mortality at stand, landscape and global scales remains highly variable under climate change (Bugmann et al., 2019). Potential for parameterising DVMs to include lianas is improving with emerging inventory data and model accuracy, the two principal challenges for modelling resilience (Purves and Pacala, 2008; Andersen et al., 2009; Scheffer et al., 2009), alongside growing knowledge regarding drivers and liana water use (McDowell et al., 2018). Recent data advances led to reparameterization of a global DVM for two neotropical forests, showing that lianas significantly affect carbon sink strength in secondary forest, while accounting for physiology, biochemistry, structure and functional diversity (di Porcia e Brugnera et al., 2019). With data from more sites in secondary forests, subsequent dynamic model extensions could then identify variation in forest recovery potential (and response classes) vs. drivers, liana dominance, management and climate change scenarios, and hence also vs. parasitoid or bandage effects of different liana species, growth forms, or communities.
Further inspiration for the next stage in understanding liana-forest dynamics can be drawn from emerging research on Barro Colorado Island (BCI), which has applied a complex systems approach inspired by disease ecology and host-parasite dynamics. Here, three stages of research using mechanistic, density-dependent models have shown empirically how (a) tree and liana density-dependent growth, and (b) species variation in shade-tolerance, can combine to determine liana growth and colonisation of tree hosts, subsequent tree growth, reproduction and mortality (Visser et al., 2017, 2018) and total impact of lianas on the per capita tree growth rate (Muller-Landau and Visser, 2019). These models have therefore developed improved understanding of both biological responses (liana burden on net tree growth, carbon, reproduction and mortality) and drivers (light, through comparison of species shade tolerance) but fall short of incorporating the ultimate drivers and full range of biological responses. Beyond BCI, structural equation modelling based on 145 plots from across the tropics has demonstrated that liana influence on above-ground carbon is dependent on climate and stand variables (Durán et al., 2015). However, neither the BCI or pantropical approaches place the forest response to lianas within a resilience framework to test for thresholds, due to their focus on primary forest rather than secondary -primary transitions.
While recent advances have taken fundamental steps in understanding liana-forest dynamics, the challenge remains to better understand environmental variation and moreover, implications for the stability of forest states (Muller-Landau and Pacala, 2020) and ultimately recovery responses to forest disturbance. Alongside expanding field data on both lianas and trees and existing mechanistic and dynamic approaches, emerging modelling techniques provide unprecedented opportunities for improved understanding of response mechanisms in complex forest systems. Mutualistic network analysis provides an opportunity to identify thresholds of habitat disturbance that may lead to the collapse of entire species communities (Fortuna and Bascompte, 2006). Recent developments in Bayesian network modelling are also providing useful tools for analysis of environmental problems, assessing dynamic systems using an acyclical method to make probabilistic assessment of management outcomes (Barton et al., 2012), with clear applicability to liana management for improved forest resilience. Alternatively, the complexity of forest dynamics and thresholds can be simplified though dimension reduction (Jiang et al., 2018), thus focussing conclusions toward the core mutualistic, bipartite relationship between lianas and trees.
The available evidence suggests that forests often lack resilience to liana proliferation following heavy or frequent disturbance. However, forests appear highly variable in their response to lianas and there is currently no direct evidence of a liana threshold, nor the propensity for bandage or parasitoid effects of lianas on forest recovery. Disturbance drivers often lead to secondary states where interactions with other drivers favour liana competition over trees, leading to stalled or arrested recovery. More optimistically, restoration management and natural forest succession under pseudo-thresholds and liana bandage effects are expected to stimulate unimpeded trajectories of recovery and carbon sequestration.
We recommend that future investigations require four levels of attention across forest succession and environmental gradients: (1) incorporation of lianas and both tree and liana saplings into ground-sourced forest inventories worldwide, enabling studies on global geospatial patterns and potential drivers of liana distributions, biodiversity and recruitment relative to trees; (2) long-term experiments to investigate primary-secondary forest state changes and subsequent temporal changes in drivers, lianas and forest state indicators, facilitated by chronosequence data and liana cutting trials; (3) determination of the presence of liana thresholds and other classes of forest recovery response, through assessment of forest state indicator responses to disturbance and liana dominance over time; and (4) determination of the nature of the liana-forest dynamic relationship, including regional and global variation within and between recovery response classes, using dynamic mechanistic modelling incorporating variation in drivers, feedbacks and their interactions. These four data-demanding tasks will determine the current (and potential future) importance of liana feedbacks relative to human, biotic and abiotic drivers and to alternative feedbacks, especially from other plant forms. Thus, imminent crashes might be predicted before they cause long-term damage to ecosystem health. Regardless of these future scientific outcomes, it is already clear that lianas influence millions of hectares of forest, which comprise tens to hundreds of petagrams of stored carbon. Determination of liana influence on forest composition, recovery and dynamics is vital for understanding fundamental ecological relationships that underpin global change and sustainable development agendas.
The datasets generated for this study are available on request to the corresponding author.
The article was conceived and written by AM, with conceptual input from MP, PP and RC and editorial input from MP, PP, RC, HS, MC, OP, RG, RM, JL, JH and YM. All figures were prepared by AM, except for Figure 2, which was prepared by PP.
This work was completed under Australian Research Council funding to AM (Future Fellowship, FT170100279).
The authors declare that the research was conducted in the absence of any commercial or financial relationships that could be construed as a potential conflict of interest.
The reviewer EV declared a past co-authorship with the authors YM and OP to the handling Editor.
Thanks to Ted Feldpausch and four reviewers for comments that helped to improve the draft manuscript. Thanks to Stefan Schnitzer for further useful comments and for permission to use the photograph in Figure 3B. We also thank all those involved in development of the FoRCE experiment and the Reforest Africa field team, who have all helped us to develop the concepts presented here. We thank all the regional managers, experts and landowners for enthusiastic discussions in our search for Australian experimental sites. We thank TAWIRI, COSTECH, TANAPA and the Tanzania Forest Services Agency, for assistance in conducting fieldwork in Tanzania.
The Supplementary Material for this article can be found online at: https://www.frontiersin.org/articles/10.3389/ffgc.2020.00035/full#supplementary-material
Andersen, T., Carstensen, J., Hernandez-Garcia, E., and Duarte, C. M. (2009). Ecological thresholds and regime shifts: approaches to identification. Trends Ecol. Evol. 24, 49–57. doi: 10.1016/j.tree.2008.07.014
Arroyo-Rodríguez, V., Asensio, N., Dunn, J. C., Cristóbal-Azkarate, J., and Gonzalez-Zamora, A. (2015). Use of lianas by primates: more than a food source,” in Ecology of Lianas, eds S. A. Schnitzer, F. Bongers, R. J. Burnham, and F. E. Putz (Chichester: John Wiley & Sons, Ltd.), 407–426. doi: 10.1002/9781118392409.ch27
Arroyo-Rodríguez, V., Melo, F. P., Martínez-Ramos, M., Bongers, F., Chazdon, R. L., Meave, J. A., et al. (2017). Multiple successional pathways in human-modified tropical landscapes: new insights from forest succession, forest fragmentation and landscape ecology research. Biol. Rev. 92, 326–340. doi: 10.1111/brv.12231
Ashton, I. W., and Lerdau, M. T. (2008). Tolerance to herbivory, and not resistance, may explain differential success of invasive, naturalized, and native North American temperate vines. Divers. Distrib. 14, 169–178. doi: 10.1111/j.1472-4642.2007.00425.x
Asner, G. P., Rudel, T. K., Aide, T. M., Defries, R., and Emerson, R. (2009). A contemporary assessment of change in humid tropical forests. Conserv. Biol. 23, 1386–1395. doi: 10.1111/j.1523-1739.2009.01333.x
Bahamondez, C., and Thompson, I. D. (2016). Determining forest degradation, ecosystem state and resilience using a standard stand stocking measurement diagram: theory into practice. Forestry. 89, 290–300. doi: 10.1093/forestry/cpv052
Balch, J. K., Nepstad, D. C., Curran, L. M., Brando, P. M., Portela, O., Guilherme, P., et al. (2011). Size, species, and fire behavior predict tree and liana mortality from experimental burns in the Brazilian Amazon. For. Ecol. Manage. 261, 68–77. doi: 10.1016/j.foreco.2010.09.029
Barlow, J., Lennox, G. D., Ferreira, J., Berenguer, E., Lees, A. C., Mac Nally, R., et al. (2016). Anthropogenic disturbance in tropical forests can double biodiversity loss from deforestation. Nature 535, 144–147. doi: 10.1038/nature18326
Barry, K. E., Schnitzer, S. A., van Breugel, M., and Hall, J. S. (2015). Rapid liana colonization along a secondary forest chronosequence. Biotropica 47, 672–680. doi: 10.1111/btp.12259
Barton, D. N., Kuikka, S., Varis, O., Uusitalo, L., Henriksen, H. J., Borsuk, M., et al. (2012). Bayesian networks in environmental and resource management. Integr. Environ. Assess. Manage. 8, 418–429. doi: 10.1002/ieam.1327
Bastin, J. F., Finegold, Y., Garcia, C., Mollicone, D., Rezende, M., Routh, D., et al. (2019). The global tree restoration potential. Science 365, 76–79. doi: 10.1126/science.aax0848
Benítez-Malvido, J., and Martínez-Ramos, M. (2003). Impact of forest fragmentation on understory plant species richness in Amazonia. Conserv. Biol. 17, 389–400. doi: 10.1046/j.1523-1739.2003.01120.x
Berenguer, E., Gardner, T. A., Ferreira, J., Aragão, L. E., Mac Nally, R., Thomson, J. R., et al. (2018). Seeing the woods through the saplings: using wood density to assess the recovery of human-modified Amazonian forests. J. Ecol. 106, 2190–2203. doi: 10.1111/1365-2745.12991
Bestelmeyer, B. T., Ellison, A. M., Fraser, W. R., Gorman, K. B., Holbrook, S. J., Laney, C. M., et al. (2011). Analysis of abrupt transitions in ecological systems. Ecosphere 2, 1–26. doi: 10.1890/ES11-00216.1
Brancalion, P. H., Niamir, A., Broadbent, E., Crouzeilles, R., Barros, F. S., Zambrano, A. M. A., et al. (2019). Global restoration opportunities in tropical rainforest landscapes. Sci. Adv. 5:eaav3223. doi: 10.1126/sciadv.aav3223
Bugmann, H., Seidl, R., Hartig, F., Bohn, F., Bruna, J., Cailleret, M., et al. (2019). Tree mortality submodels drive simulated long-term forest dynamics: assessing 15 models from the stand to global scale. Ecosphere 10:e02616. doi: 10.1002/ecs2.2616
Campbell, M. J., Edwards, W., Magrach, A., Alamgir, M., Porolak, G., Mohandass, D., et al. (2018). Edge disturbance drives liana abundance increase and alteration of liana–host tree interactions in tropical forest fragments. Ecol. Evol. 8, 4237–4251. doi: 10.1002/ece3.3959
Campbell, M. J., Edwards, W., Odell, E., Mohandass, D., and Laurance, W. F. (2015). Can lianas assist in rainforest restoration? Trop. Conserv. Sci. 8, 257–273. doi: 10.1177/194008291500800119
Capers, R. S., Chazdon, R. L., Brenes, A. R., and Alvarado, B. V. (2005). Successional dynamics of woody seedling communities in wet tropical secondary forests. J. Ecol. 93, 1071–1084. doi: 10.1111/j.1365-2745.2005.01050.x
Carneiro, M. S., Campos, C. C. F., and Ramos, F. N. (2017). Liana and bamboo cover threaten shrub populations in Atlantic forest fragments. Aust. J. Bot. 65, 375–383. doi: 10.1071/BT16121
Carpenter, S. R., and Brock, W. A. (2006). Rising variance: a leading indicator of ecological transition. Ecol Lett. 9, 311–318. doi: 10.1111/j.1461-0248.2005.00877.x
Carrasco, L. R., Nghiem, T. P. L., Sunderland, T., and Koh, L. P. (2014). Economic valuation of ecosystem services fails to capture biodiversity value of tropical forests. Biol. Conserv. 178,163–170. doi: 10.1016/j.biocon.2014.08.007
Cazzolla Gatti, R. (2018). Modelling weed and vine disturbance in tropical forests after selective logging and clearcutting. Ecol. Modell. 375, 13–19. doi: 10.1016/j.ecolmodel.2018.01.018
Cazzolla Gatti, R., Castaldi, S., Lindsell, J. A., Coomes, D. A., Marchetti, M., Maesano, M., et al. (2015). The impact of selective logging and clearcutting on forest structure, tree diversity and above-ground biomass of African tropical forests. Ecol. Res. 30, 119–132. doi: 10.1007/s11284-014-1217-3
Cerullo, G. R., and Edwards, D. P. (2019). Actively restoring resilience in selectively logged tropical forests. J. Appl. Ecol. 56, 107–118. doi: 10.1111/1365-2664.13262
César, R. G., Holl, K. D., Girão, V. J., Mello, F. N., Vidal, E., Alves, M. C., et al. (2016). Evaluating climber cutting as a strategy to restore degraded tropical forests. Biol. Conserv. 201, 309–313. doi: 10.1016/j.biocon.2016.07.031
César, R. G., Rother, D. C., and Brancalion, P. H. (2017). Early response of tree seed arrival after liana cutting in a disturbed tropical forest. Trop. Conserv. Sci. 10, 1–7. doi: 10.1177/1940082917723586
Chapin, F. S., Callaghan, T. V., Bergeron, Y., Fukuda, M., Johnstone, J. F., Juday, G., et al. (2004). Global change and the boreal forest: thresholds, shifting states or gradual change? Ambio 33, 361–366. doi: 10.1579/0044-7447-33.6.361
Chapman, C. A., and Chapman, L. J. (1997). Forest regeneration in logged and unlogged forests of Kibale National Park, Uganda. Biotropica 29, 396–412. doi: 10.1111/j.1744-7429.1997.tb00035.x
Chokkalingam, U., and de Jong, W. (2001). Secondary forest: a working definition and typology. Int. For. Rev. 3, 19–26. Available online at: http://www.cifor.org/publications/pdf_files/secondaryforests.pdf
Clark, D. A., Brown, S., Kicklighter, D. W., Chambers, J. Q., Thomlinson, J. R., and Ni, J. (2001). Measuring net primary production in forests: concepts and field methods. Ecol. App. 11, 356–370. doi: 10.1890/1051-0761(2001)011[0356:MNPPIF]2.0.CO;2
Clark, D. B. (1996). Abolishing virginity. J. Trop. Ecol. 12, 735–739. doi: 10.1017/S0266467400009937
Clark, D. B., and Clark, D. A. (1990). Distribution and effects on tree growth of lianas and woody hemi-epiphytes in a Costa Rican tropical wet forest. J. Trop. Ecol. 6, 321–331. doi: 10.1017/S0266467400004570
Cole, L. E., Bhagwat, S. A., and Willis, K. J. (2014). Recovery and resilience of tropical forests after disturbance. Nat. Commun. 5:3906. doi: 10.1038/ncomms4906
Cox, C. J., Edwards, W., Campbell, M. J., Laurance, W. F., and Laurance, S. G. (2019). Liana cover in the canopies of rainforest trees is not predicted by local ground-based measures. Austral Ecol. 44, 759–767. doi: 10.1111/aec.12746
Crouzeilles, R., Curran, M., Ferreira, M. S., Lindenmayer, D. B., Grelle, C. E., and Benayas, J. M. R. (2016). A global meta-analysis on the ecological drivers of forest restoration success. Nat. Commun. 7:11666. doi: 10.1038/ncomms11666
DeWalt, S. J., Schnitzer, S. A., Alves, L. F., Bongers, F., Burnham, R. J., Cai, Z., et al. (2015). “Biogeographical patterns of liana abundance and diversity,” in Ecology of Lianas, eds S. A. Schnitzer, F. Bongers, R. J. Burnham, and F. E. Putz (Chichester, UK: John Wiley & Sons, Ltd.), 131–146. doi: 10.1002/9781118392409
di Porcia e Brugnera, M., Meunier, F., Longo, M., Moorthy, S. M. K., De Deurwaerder, H., et al. (2019). Modelling the impact of liana infestation on the demography and carbon cycle of tropical forests. Glob. Chang. Biol. 25, 3767–3780. doi: 10.1111/gcb.14769
Duncan, R. S., and Chapman, C. A. (2003). Tree–shrub interactions during early secondary forest succession in Uganda. Restor. Ecol. 11, 198–207. doi: 10.1046/j.1526-100X.2003.00153.x
Dupuy, J. M., and Chazdon, R. (2006). Effects of vegetation cover on seedling and sapling dynamics in secondary tropical wet forests in Costa Rica. J. Trop. Ecol. 22, 65–76. doi: 10.1017/S0266467405002890
Durán, S. M., and Gianoli, E. (2013). Carbon stocks in tropical forests decrease with liana density. Biol. Lett. 9:20130301. doi: 10.1098/rsbl.2013.0301
Durán, S. M., Sánchez-Azofeifa, G. A., Rios, R. S., and Gianoli, E. (2015). The relative importance of climate, stand variables and liana abundance for carbon storage in tropical forests. Global Ecol. Biogeogr. 24, 939–949. doi: 10.1111/geb.12304
Dutilleul, P., Clifford, P., Richardson, S., and Hemon, D. (1993). Modifying the t test for assessing the correlation between two spatial processes. Biometrics 49, 305–314. doi: 10.2307/2532625
Eason, T., Garmestani, A. S., Stow, C. A., Rojo, C., Alvarez-Cobelas, M., and Cabezas, H. (2016). Managing for resilience: an information theory-based approach to assessing ecosystems. J. Appl. Ecol. 53, 656–665. doi: 10.1111/1365-2664.12597
Estrada-Villegas, S., and Schnitzer, S. A. (2018). A comprehensive synthesis of liana removal experiments in tropical forests. Biotropica 50, 729–739. doi: 10.1111/btp.12571
Ewers, F. W., Fisher, J. B., and Fichtner, K. (1991). “Water flux and xylem structure in vines,” in The Biology of Vines, eds F. E. Putz, and H. A. Mooney (Cambridge: Cambridge University Press), 127–160. doi: 10.1017/CBO9780511897658.007
Ewers, F. W., Rosell, J. A., and Olson, M. E. (2015). “Lianas as structural parasites,” in Functional and Ecological Xylem Anatomy, ed U. Hacke (Cham: Springer), 163–188. doi: 10.1007/978-3-319-15783-2_6
Ewers, R. M., Boyle, M. J., Gleave, R. A., Plowman, N. S., Benedick, S., Bernard, H., et al. (2015). Logging cuts the functional importance of invertebrates in tropical rainforest. Nat. Commun. 6:6836. doi: 10.1038/ncomms7836
Fadrique, B., and Homeier, J. (2016). Elevation and topography influence community structure, biomass and host tree interactions of lianas in tropical montane forests of southern Ecuador. J. Veg. Sci. 27, 958–968. doi: 10.1111/jvs.12427
Fayle, T. M., Turner, E. C., Basset, Y., Ewers, R. M., Reynolds, G., and Novotny, V. (2015). Whole-ecosystem experimental manipulations of tropical forests. Trends Ecol. Evol. 30, 334–346. doi: 10.1016/j.tree.2015.03.010
Feldpausch, T. R., Riha, S. J., Fernandes, E. C., and Wandelli, E. V. (2005). Development of forest structure and leaf area in secondary forests regenerating on abandoned pastures in Central Amazonia. Earth Interact. 9, 1–22. doi: 10.1175/EI140.1
Fike, J., and Niering, W. A. (1999). Four decades of old field vegetation development and the role of Celastrus orbiculatus in the northeastern United States. J. Veg. Sci. 10, 483–492. doi: 10.2307/3237183
Foley, J. A., DeFries, R., Asner, G. P., Barford, C., Bonan, G., Carpenter, S. R., et al. (2005). Global consequences of land use. Science 309, 570–574. doi: 10.1126/science.1111772
Fortuna, M. A., and Bascompte, J. (2006). Habitat loss and the structure of plant–animal mutualistic networks. Ecol. Lett. 9, 281–286. doi: 10.1111/j.1461-0248.2005.00868.x
Foster, J. R., Townsend, P. A., and Zganjar, C. E. (2008). Spatial and temporal patterns of gap dominance by low-canopy lianas detected using EO-1 hyperion and landsat thematic mapper. Remote Sens. Environ. 112, 2104–2117. doi: 10.1016/j.rse.2007.07.027
Gentry, A. H. (1992). Tropical forest biodiversity: distributional patterns and their conservational significance. Oikos 63, 19–28. doi: 10.2307/3545512
Gerwing, J. J. (2001). Testing liana cutting and controlled burning as silvicultural treatments for a logged forest in the eastern Amazon. J. Appl. Ecol. 38, 1264–1276. doi: 10.1046/j.0021-8901.2001.00677.x
Gerwing, J. J., and Farias, D. L. (2000). Integrating liana abundance and forest stature into an estimate of total aboveground biomass for an eastern Amazonian forest. J. Trop. Ecol. 16, 327–335. doi: 10.1017/S0266467400001437
Ghazoul, J., Burivalova, Z., Garcia-Ulloa, J., and King, L. A. (2015). Conceptualizing forest degradation. Trends Ecol. Evol. 30, 622–632. doi: 10.1016/j.tree.2015.08.001
Ghazoul, J., and Chazdon, R. (2017). Degradation and recovery in changing forest landscapes: a multiscale conceptual framework. Annu. Rev. Environ. Resour. 42, 161–188. doi: 10.1146/annurev-environ-102016-060736
Gibson, L., Lee, T. M., Koh, L. P., Brook, B. W., Gardner, T. A., Barlow, J., et al. (2011). Primary forests are irreplaceable for sustaining tropical biodiversity. Nature 478, 378–381. doi: 10.1038/nature10425
Global Climate Observing System (2016). The Global Observing System for Climate: Implementation Needs. Geneva: World Meteorological Organisation, Technical Report 2016.
Goosem, S. P., and Tucker, N. I. (2013). Repairing the Rainforest. Cairns: Wet Tropics Management Authority.
Goriely, A., and Neukirch, S. (2006). Mechanics of climbing and attachment in twining plants. Phys. Rev. Lett. 97:184302. doi: 10.1103/PhysRevLett.97.184302
Gower, S. T., McMurtrie, R. E., and Murty, D. (1996). Aboveground net primary production decline with stand age: potential causes. Trends Ecol. Evol. 11, 378–382. doi: 10.1016/0169-5347(96)10042-2
Grace, J., Mitchard, E., and Gloor, E. (2014). Perturbations in the carbon budget of the tropics. Glob. Chang. Biol. 20, 3238–3255. doi: 10.1111/gcb.12600
Granados, J., and Körner, C. (2002). In deep shade, elevated CO2 increases the vigor of tropical climbing plants. Glob. Chang. Biol. 8, 1109–1117. doi: 10.1046/j.1365-2486.2002.00533.x
Griscom, B. W., and Ashton, P. M. S. (2006). A self-perpetuating bamboo disturbance cycle in a neotropical forest. J. Trop. Ecol. 22, 587–597. doi: 10.1017/S0266467406003361
Harris, C. J., and Gallagher, R. (2011). “Vines and lianas,” in Encyclopedia of Biological Invasions, eds D. Simberloff, and M. Rejmánek (Oakland, CA: University of California Press), 680–684.
Hartigan, J. A., and Hartigan, P. M. (1985). The dip test of unimodality. Ann. Stat. 13, 70–84. doi: 10.1214/aos/1176346577
Higgins, S. I., and Scheiter, S. (2012). Atmospheric CO2 forces abrupt vegetation shifts locally, but not globally. Nature 488, 209–212. doi: 10.1038/nature11238
Hirota, M., Holmgren, M., van Nes, E. H., and Scheffer, M. (2011). Global resilience of tropical forest and savanna to critical transitions. Science 334, 232–235. doi: 10.1126/science.1210657
Hobbs, R. J., and Cramer, V. A. (2007). “Old field dynamics: regional and local differences, and lessons for ecology and restoration,” in Old Fields: Dynamic and Resoration of Abandoned Farmland, eds V. A. Cramer, and R. J. Hobbs (Washington, DC: Island Press), 309–318.
Hoffmann, W. A., Geiger, E. L., Gotsch, S. G., Rossatto, D. R., Silva, L. C., Lau, O. L., et al. (2012). Ecological thresholds at the savanna-forest boundary: how plant traits, resources and fire govern the distribution of tropical biomes. Ecol. Lett. 15, 759–768. doi: 10.1111/j.1461-0248.2012.01789.x
Ingwell, L. L., Wright, S. J., Becklund, K. K., Hubbell, S. P., and Schnitzer, S. A. (2010). The impact of lianas on 10 years of tree growth and mortality on Barro Colorado Island, Panama. J. Ecol. 98, 879–887. doi: 10.1111/j.1365-2745.2010.01676.x
Jakovac, C. C., Peña-Claros, M., Kuyper, T. W., and Bongers, F. (2015). Loss of secondary -forest resilience by land-use intensification in the Amazon. J. Ecol. 103, 67–77. doi: 10.1111/1365-2745.12298
Jiang, J., Huang, Z. G., Seager, T. P., Lin, W., Grebogi, C., Lai, Y. C., et al. (2018). Predicting tipping points in mutualistic networks through dimension reduction. Proc. Natl. Acad. Sci. U.S.A. 115, E639–E647. doi: 10.1073/pnas.1714958115
Jiménez-Castillo, M., and Lusk, C. H. (2013). Vascular performance of woody plants in a temperate rain forest: lianas suffer higher levels of freeze–thaw embolism than associated trees. Funct. Ecol. 27, 403–412. doi: 10.1111/1365-2435.12045
Jiménez-Castillo, M., Wiser, S. K., and Lusk, C. H. (2007). Elevational parallels of latitudinal variation in the proportion of lianas in woody floras. J. Biogeogr. 34, 163–168. doi: 10.1111/j.1365-2699.2006.01570.x
Jirka, S., McDonald, A. J., Johnson, M. S., Feldpausch, T. R., Couto, E. G., and Riha, S. J. (2007). Relationships between soil hydrology and forest structure and composition in the southern Brazilian Amazon. J. Veg. Sci. 18, 183–194. doi: 10.1111/j.1654-1103.2007.tb02529.x
Jones, I. L., Peres, C. A., Benchimol, M., Bunnefeld, L., and Dent, D. H. (2017). Woody lianas increase in dominance and maintain compositional integrity across an Amazonian dam-induced fragmented landscape. PLoS ONE 12:e0185527. doi: 10.1371/journal.pone.0185527
Kazda, M., and Salzer, A. J. (2000). Leaves of lianas and self-supporting plants differ in mass per unit area and in nitrogen content. Plant Biol. 2, 268–271. doi: 10.1055/s-2000-3701
Kessler, M. (2010). “Biogeography of ferns,” in Fern Ecology, eds K. Mehltreter, L. R. Walker, and J. M. Sharpe (Cambridge: Cambridge University Press), 22–46. doi: 10.1017/CBO9780511844898.003
Kloeppel, B. D., Harmon, M. E., and Fahey, T. J. (2007). “Estimating aboveground net primary productivity in forest-dominated ecosystems,” in Principles and Standards for Measuring Primary Production, eds T. J. Fahey and A. K. Knapp (New York, NY: Oxford University Press), 63–81. doi: 10.1093/acprof:oso/9780195168662.003.0005
Ladwig, L. M., and Meiners, S. J. (2009). Impacts of temperate lianas on tree growth in young deciduous forests. For. Ecol. Manage. 259, 195–200. doi: 10.1016/j.foreco.2009.10.012
Ladwig, L. M., and Meiners, S. J. (2010). Spatiotemporal dynamics of lianas during 50 years of succession to temperate forest. Ecology 91, 671–680. doi: 10.1890/08-1738.1
Ladwig, L. M., and Meiners, S. J. (2015). “The role of lianas in temperate tree communities,” in Ecology of Lianas, eds S. A. Schnitzer, F. Bongers, R. J. Burnham, and F. E. Putz (Chichester: John Wiley & Sons, Ltd.), 188–202. doi: 10.1002/9781118392409.ch15
Ladwig, L. M., Meiners, S. J., Pisula, N. L., and Lang, K. A. (2012). Conditional allelopathic potential of temperate lianas. Plant Ecol. 213, 1927–1935. doi: 10.1007/s11258-012-0087-4
Lai, H. R., Hall, J. S., Turner, B. L., and Breugel, M. (2017). Liana effects on biomass dynamics strengthen during secondary forest succession. Ecology 98, 1062–1070. doi: 10.1002/ecy.1734
Lambert, T. D., and Halsey, M. K. (2015). “Relationship between lianas and arboreal mammals: examining the emmons-gentry hypothesis,” in Ecology of Lianas, eds S. A. Schnitzer, F. Bongers, R. J. Burnham, and F. E. Putz (Chichester: John Wiley & Sons, Ltd.), 398–406. doi: 10.1002/9781118392409.ch26
Lennox, G. D., Gardner, T. A., Thomson, J. R., Ferreira, J., Berenguer, E., Lees, A. C., et al. (2018). SecondS rate or a seconds chance? Assessing biomass and biodiversity recovery in regenerating Amazonian forests. Glob. Change Biol. 24, 5680–5694. doi: 10.1111/gcb.14443
Letcher, S. G. (2015). “Patterns of liana succession in tropical forests,” in Ecology of Lianas, eds S. A. Schnitzer, F. Bongers, R. J. Burnham, and F. E. Putz (Chichester: John Wiley & Sons, Ltd.), 116–130. doi: 10.1002/9781118392409.ch10
Letcher, S. G., and Chazdon, R. L. (2009). Lianas and self-supporting plants during tropical forest succession. For. Ecol. Manage. 257, 2150–2156. doi: 10.1016/j.foreco.2009.02.028
Liang, J., Crowther, T. W., Picard, N., Wiser, S., Zhou, M., Alberti, G., et al. (2016). Positive biodiversity-productivity relationship predominant in global forests. Science 354:aaf8957. doi: 10.1126/science.aaf8957
Luskin, M. S., Ickes, K., Yao, T. L., and Davies, S. J. (2019). Wildlife differentially affect tree and liana regeneration in a tropical forest: an 18-yr study of experimental terrestrial defaunation versus artificially abundant herbivores. J. Appl. Ecol. 56, 1379–1388. doi: 10.1111/1365-2664.13378
Magnago, L. F. S., Magrach, A., Barlow, J., Schaefer, C. E. G. R., Laurance, W. F., Martins, S. V., et al. (2017). Do fragment size and edge effects predict carbon stocks in trees and lianas in tropical forests? Funct. Ecol. 31, 542–552. doi: 10.1111/1365-2435.12752
Magnuszewski, P., Ostasiewicz, K., Chazdon, R., Salk, C., Pajak, M., Sendzimir, J., et al. (2015). Resilience and alternative stable states of tropical forest landscapes under shifting cultivation regimes. PLoS ONE 10:e0137497. doi: 10.1371/journal.pone.0137497
Malhi, Y., Aragão, L. E., Galbraith, D., Huntingford, C., Fisher, R., Zelazowski, P., et al. (2009). Exploring the likelihood and mechanism of a climate-change-induced dieback of the Amazon rainforest. Proc. Natl. Acad. Sci. U.S.A. 106, 20610–20615. doi: 10.1073/pnas.0804619106
Marshall, A. R., Coates, M. A., Archer, J., Kivambe, E., Mnendendo, H., Mtoka, S., et al. (2017). Liana cutting for restoring tropical forests: a rare palaeotropical trial. Afr. J. Ecol. 54, 282–197. doi: 10.1111/aje.12349
Marshall, A. R., Willcock, S., Platts, P. J., Lovett, J. C., Balmford, A., Burgess, N. D., et al. (2012). Measuring and modelling above-ground carbon and tree allometry along a tropical elevation gradient. Biol. Conserv. 154, 20–33. doi: 10.1016/j.biocon.2012.03.017
Martin, P., Newton, A. C., Cantarello, E., and Evans, P. M. (2017). Analysis of ecological thresholds in a temperate forest undergoing dieback. PLoS ONE 12:e0189578. doi: 10.1371/journal.pone.0189578
Martin, P. A., Newton, A. C., and Bullock, J. M. (2013). Carbon pools recover more quickly than plant biodiversity in tropical secondary forests. Proc. Biol. Sci. 280:20132236. doi: 10.1098/rspb.2013.2236
Martínez-Izquierdo, L., García, M. M., Powers, J. S., and Schnitzer, S. A. (2016). Lianas suppress seedling growth and survival of 14 tree species in a Panamanian tropical forest. Ecology 97, 215–224. doi: 10.1890/14-2261.1
McDowell, N., Allen, C. D., Anderson-Teixeira, K., Brando, P., Brienen, R., Chambers, J., et al. (2018). Drivers and mechanisms of tree mortality in moist tropical forests. New Phytol. 219, 851–869. doi: 10.1111/nph.15027
Medlyn, B. E., Zaehle, S., De Kauwe, M. G., Walker, A. P., Dietze, M. C., Hanson, P. J., et al. (2015). Using ecosystem experiments to improve vegetation models. Nat. Clim. Change 5, 528–534. doi: 10.1038/nclimate2621
Meli, P., Holl, K. D., Benayas, J. M. R., Jones, H. P., Jones, P. C., Montoya, D., et al. (2017). A global review of past land use, climate, and active vs. passive restoration effects on forest recovery. PLoS ONE 12:e0171368. doi: 10.1371/journal.pone.0171368
Miao, Z., Koerner, S. E., Medjibe, V. P., and Poulsen, J. R. (2016). Wanted: new allometric equations for large lianas and African lianas. Biotropica 48, 561–564. doi: 10.1111/btp.12353
Michel, N. L., Carson, W. P., and Sherry, T. W. (2015). Do collared peccaries negatively impact understory insectivorous rain forest birds indirectly via lianas and vines? Biotropica 47, 745–757. doi: 10.1111/btp.12261
Moore, J. C. (2018). Predicting tipping points in complex environmental systems. Proc. Natl. Acad. Sci. U.S.A. 115, 635–636. doi: 10.1073/pnas.1721206115
Moorthy, S. M. K., Bao, Y., Calders, K., Schnitzer, S. A., and Verbeeck, H. (2019). Semi-automatic extraction of liana stems from terrestrial LiDAR point clouds of tropical rainforests. ISPRS J. Photogramm. Remote Sens. 154, 114–126. doi: 10.1016/j.isprsjprs.2019.05.011
Moorthy, S. M. K., Calders, K., Di Porcia e Brugnera, M., Schnitzer, S. A., and Verbeeck, H. (2018). Terrestrial laser scanning to detect liana impact on forest structure. Remote Sens. 10:810. doi: 10.3390/rs10060810
Muller-Landau, H. C., and Pacala, S. W. (2020). “What determines the abundance of lianas and vines,” in Unsolved Problems in Ecology, eds A. P. Dobson, R. D. Holt, and D. Tilman. Available online at: www.researchgate.net/publication/322764801 (accessed February 19, 2019).
Muller-Landau, H. C., and Visser, M. D. (2019). How do lianas and vines influence competitive differences and niche differences among tree species? Concepts and a case study in a tropical forest. J. Ecol. 107, 1469–1481. doi: 10.1111/1365-2745.13119
Newton, A. C., and Cantarello, E. (2015). Restoration of forest resilience: an achievable goal? New Forests 46, 645–668. doi: 10.1007/s11056-015-9489-1
Norden, N., Angarita, H. A., Bongers, F., Martínez-Ramos, M., Granzow-de la Cerda, I., van Breugel, M., et al. (2015). Successional dynamics in Neotropical forests are as uncertain as they are predictable. Proc. Natl. Acad. Sci. U.S.A. 112, 8013–8018. doi: 10.1073/pnas.1500403112
O'Brien, M. J., Philipson, C. D., Reynolds, G., Dzulkifli, D., Snaddon, J. L., Ong, R., et al. (2019). Positive effects of liana cutting on seedlings are reduced during El Niño-induced drought. J. Appl. Ecol. 56, 891–901. doi: 10.1111/1365-2664.13335
Ødegaard, F. (2000). The relative importance of trees versus lianas as hosts for phytophagous beetles (Coleoptera) in tropical forests. J. Biogeogr. 27, 283–296. doi: 10.1046/j.1365-2699.2000.00404.x
Odell, E. H., Stork, N. E., and Kitching, R. L. (2019). Lianas as a food resource for herbivorous insects: a comparison with trees. Biol. Rev. 94, 1416–1429. doi: 10.1111/brv.12508
Omeja, P. A., Jacob, A. L., Lawes, M. J., Lwanga, J. S., Rothman, J. M., Tumwesigye, C., et al. (2014). Changes in elephant abundance affect forest composition or regeneration? Biotropica 46, 704–711. doi: 10.1111/btp.12154
Pan, Y., Birdsey, R. A., Fang, J., Houghton, R., Kauppi, P. E., Kurz, W. A., et al. (2011). A large and persistent carbon sink in the world's forests. Science 333, 988–993. doi: 10.1126/science.1201609
Pan, Y., Birdsey, R. A., Phillips, O. L., and Jackson, R. B. (2013). The structure, distribution, and biomass of the world's forests. Annu. Rev. Ecol. Evol. Syst. 44, 593–622. doi: 10.1146/annurev-ecolsys-110512-135914
Parren, M. P. E., and Bongers, F. (2005). “Management of climbers in the forests of West Africa,” in Forest Climbing Plants of West Africa, eds F. Bongers, M. Parren, and D. Traore (Wallingford: CABI Publishing), 217–229. doi: 10.1079/9780851999142.0217
Pérez-Salicrup, D. R., Sork, V. L., and Putz, F. E. (2001). Lianas and trees in a liana forest of Amazonian Bolivia. Biotropica 33, 34–47. doi: 10.1111/j.1744-7429.2001.tb00155.x
Pfeifer, M., Gonsamo, A., Woodgate, W., Cayuela, L., Marshall, A. R., Ledo, A., et al. (2018). Tropical forest canopies and their relationships with climate and disturbance: results from a global dataset of consistent field-based measurements. Forest Ecosystems 5:7. doi: 10.1186/s40663-017-0118-7
Phillips, O. L., Martínez, R. V., Arroyo, L., Baker, T. R., Killeen, T., Lewis, S. L., et al. (2002). Increasing dominance of large lianas in Amazonian forests. Nature 418, 770–774. doi: 10.1038/nature00926
Phillips, O. L., Vásquez Martínez, R., Monteagudo Mendoza, A., Baker, T. R., and Núñez Vargas, P. (2005). Large lianas as hyperdynamic elements of the tropical forest canopy. Ecology 86, 1250–1258. doi: 10.1890/04-1446
Piovesan, P. R. R., Camargo, J. L. C., Burnham, R. J., and Ferraz, I. D. K. (2018). Abundance of liana species in an Amazonian forest of Brazil reflects neither adventitious root nor foliar sprout production. J. Trop. Ecol. 34, 257–267. doi: 10.1017/S0266467418000238
Poorter, L., Bongers, F., Aide, T. M., Zambrano, A. M. A., Balvanera, P., Becknell, J. M., et al. (2016). Biomass resilience of neotropical secondary forests. Nature 530, 211–214. doi: 10.1038/nature16512
Pugh, T. A., Arneth, A., Kautz, M., Poulter, B., and Smith, B. (2019). Important role of forest disturbances in the global biomass turnover and carbon sinks. Nat. Geosci. 12, 730–735. doi: 10.1038/s41561-019-0427-2
Purves, D., and Pacala, S. (2008). Predictive models of forest dynamics. Science 320, 1452–1453. doi: 10.1126/science.1155359
Putz, F. E. (1983). Liana biomass and leaf area of a “tierra firme” forest in the Rio Negro Basin, Venezuela. Biotropica 15, 185–189. doi: 10.2307/2387827
Putz, F. E. (1984b). The natural history of lianas on Barro Colorado Island, Panama. Ecology 65, 1713–1724. doi: 10.2307/1937767
Quandt, R. E. (1958). The estimation of the parameters of a linear regression system obeying two separate regimes. J. Am. Stat. Assoc. 53, 873–880. doi: 10.1080/01621459.1958.10501484
Rapport, D. J. (2007). Sustainability science: an ecohealth perspective. Sustain. Sci. 2, 77–84. doi: 10.1007/s11625-006-0016-3
Ratajczak, Z., Carpenter, S. R., Ives, A. R., Kucharik, C. J., Ramiadantsoa, T., Stegner, M. A., et al. (2018). Abrupt change in ecological systems: inference and diagnosis. Trends Ecol. Evol. 33, 513–526. doi: 10.1016/j.tree.2018.04.013
Restom, T. G., and Nepstad, D. C. (2001). Contribution of vines to the evapotranspiration of a secondary forest in eastern Amazonia. Plant Soil 236, 155–163. doi: 10.1023/A:1012776532147
Reyer, C. P., Brouwers, N., Rammig, A., Brook, B. W., Epila, J., Grant, R. F., et al. (2015). Forest resilience and tipping points at different spatio-temporal scales: approaches and challenges. J. Ecol. 103, 5–15. doi: 10.1111/1365-2745.12337
Royo, A. A., and Carson, W. P. (2006). On the formation of dense understory layers in forests worldwide: consequences and implications for forest dynamics, biodiversity, and succession. Can. J. For. Res. 36, 1345–1362. doi: 10.1139/x06-025
Rozendaal, D. M., Bongers, F., Aide, T. M., Alvarez-Dávila, E., Ascarrunz, N., Balvanera, P., et al. (2019). Biodiversity recovery of Neotropical secondary forests. Sci. Adv. 5:eaau3114. doi: 10.1126/sciadv.aau3114
Ruesch, A., and Gibbs, H. K. (2008). New IPCC, Tier-1 Global Biomass Carbon Map For the Year 2000. Oak Ridge National, Laboratory Oak, Ridge Tennessee. Available online at: http://cdiac.ess-dive.lbl.gov
Sánchez-Azofeifa, G. A., Guzmán-Quesada, J. A., Vega-Araya, M., Campos-Vargas, C., Durán, S. M., D'Souza, N., et al. (2017). Can terrestrial laser scanners (TLSs) and hemispherical photographs predict tropical dry forest succession with liana abundance? Biogeosciences 14, 977–988. doi: 10.5194/bg-14-977-2017
Scheffer, M., Bascompte, J., Brock, W. A., Brovkin, V., Carpenter, S. R., Dakos, V., et al. (2009). Early-warning signals for critical transitions. Nature 461, 53–9. doi: 10.1038/nature08227
Scheffer, M., Carpenter, S., Foley, J. A., Folke, C., and Walker, B. (2001). Catastrophic shifts in ecosystems. Nature 413, 591–596. doi: 10.1038/35098000
Scheffer, M., and Carpenter, S. R. (2003). Catastrophic regime shifts in ecosystems: linking theory to observation. Trends Ecol. Evol. 18, 648–656. doi: 10.1016/j.tree.2003.09.002
Scheffer, M., Hirota, M., Holmgren, M., van Nes, E. H., and Chapin, F. S. (2012). Thresholds for boreal biome transitions. Proc. Natl. Acad. Sci.U.S.A. 109, 21384–21389. doi: 10.1073/pnas.1219844110
Schnitzer, S. A. (2018). Testing ecological theory with lianas. New Phytol. 220, 366–380. doi: 10.1111/nph.15431
Schnitzer, S. A., and Bongers, F. (2002). The ecology of lianas and their role in forests. Trends Ecol. Evol. 17, 223–230. doi: 10.1016/S0169-5347(02)02491-6
Schnitzer, S. A., and Bongers, F. (2011). Increasing liana abundance and biomass in tropical forests: emerging patterns and putative mechanisms. Ecol. Lett. 14, 397–406. doi: 10.1111/j.1461-0248.2011.01590.x
Schnitzer, S. A., and Carson, W. P. (2001). Treefall gaps and the maintenance of species diversity in a tropical forest. Ecology 82, 913–919. doi: 10.1890/0012-9658(2001)082[0913:TGATMO]2.0.CO;2
Schnitzer, S. A., and Carson, W. P. (2010). Lianas suppress tree regeneration and diversity in treefall gaps. Ecol. Lett. 13, 849–857. doi: 10.1111/j.1461-0248.2010.01480.x
Schnitzer, S. A., Dalling, J. W., and Carson, W. P. (2000). The impact of lianas on tree regeneration in tropical forest canopy gaps: evidence for an alternative pathway of gap-phase regeneration. J. Ecol. 88, 655–666. doi: 10.1046/j.1365-2745.2000.00489.x
Schnitzer, S. A., DeWalt, S. J., and Chave, J. (2006). Censusing and measuring lianas: a quantitative comparison of the common methods. Biotropica 38, 581–591. doi: 10.1111/j.1744-7429.2006.00187.x
Schnitzer, S. A., Putz, F. E., Bongers, F., and Kroening, K. (2015). “The past, present and potential future of liana ecology,” in Ecology of Lianas, eds S. A. Schnitzer, F. Bongers, R. J. Burnham, and F. E. Putz (Chichester: John Wiley & Sons, Ltd.), 3–10. doi: 10.1002/9781118392409.ch1
Schnitzer, S. A., Rutishauser, S., and Aguilar, S. (2008). Supplemental protocol for liana censuses. For. Ecol. Manage. 255, 1044–1049. doi: 10.1016/j.foreco.2007.10.012
Schnitzer, S. A., van der Heijden, G. M. F., and Powers, J. S. (2016). Reply to verbeeck and kearsley: addressing the challenges of including lianas in global vegetation models. Proc. Natl. Acad. Sci.U.S.A. 113, E5–E6. doi: 10.1073/pnas.1521823113
Senior, R. A., Hill, J. K., Benedick, S., and Edwards, D. P. (2018). Tropical forests are thermally buffered despite intensive selective logging. Glob. Chang. Biol. 24, 1267–1278. doi: 10.1111/gcb.13914
Sfair, J. C., Rochelle, A. L. C., Rezende, A. A., van Melis, J., de Lara Weiser, V., and Martins, F. R. (2010). Nested liana-tree network in three distinct neotropical vegetation formations. Perspect. Plant Ecol. Evol. Syst. 12, 277–281. doi: 10.1016/j.ppees.2010.09.001
Sheil, D., and Burslem, D. F. R. P. (2003). Disturbing hypotheses in tropical forests. Trends Ecol. Evol. 18, 18–26. doi: 10.1016/S0169-5347(02)00005-8
Smith-Martin, C. M., Xu, X., Medvigy, D., Schnitzer, S. A., and Powers, J. S. (2019). Allometric scaling laws linking biomass and rooting depth vary across ontogeny and functional groups in tropical dry forest lianas and trees. New Phytol. doi: 10.1111/nph.16275
Ssali, F., Moe, S. R., and Sheil, D. (2018). Tree seed rain and seed removal, but not the seed bank, impede forest recovery in bracken (Pteridium aquilinum (L.) Kuhn)-dominated clearings in the African highlands. Ecol. Evol. 8, 4224–4236. doi: 10.1002/ece3.3944
Ssali, F., Moe, S. R., and Sheil, D. (2019). The differential effects of bracken (Pteridium aquilinum (L.) Kuhn) on germination and seedling performance of tree species in the African tropics. Plant Ecol. 220, 41–55. doi: 10.1007/s11258-018-0901-8
Steidinger, B. S., Crowther, T. W., Liang, J., Van Nuland, M. E., Werner, G. D. A., Reich, P. B., et al. (2019). Climatic controls of decomposition drive the global biogeography of forest tree symbioses. Nature 569, 44–408. doi: 10.1038/s41586-019-1128-0
Stevens, G. C. (1987). Lianas as structural parasites: the Bursera simaruba example. Ecology 68, 77–81. doi: 10.2307/1938806
Stewart, T. E., and Schnitzer, S. A. (2017). Blurred lines between competition and parasitism. Biotropica 49, 433–438. doi: 10.1111/btp.12444
Stewart-Oaten, A., Murdoch, W. W., and Parker, K. R. (1986). Environmental impact assessment: “Pseudoreplication” in time? Ecology 67, 929–940. doi: 10.2307/1939815
Swanson, M. E., Franklin, J. F., Beschta, R. L., Crisafulli, C. M., DellaSala, D. A., Hutto, R. L., et al. (2011). The forgotten stage of forest succession: early-successional ecosystems on forest sites. Front. Ecol. Environ. 9:157. doi: 10.1890/090157
Tang, Y., Kitching, R., and Cao, M. (2012). Lianas as structural parasites: a re-evaluation. Chin. Sci. Bull. 57, 307–312. doi: 10.1007/s11434-011-4690-x
Thompson, I. (2011). Biodiversity, ecosystem thresholds, resilience and forest degradation. Unasylva 238, 25–30. Available online at: http://www.fao.org/3/i2560e/i2560e05.pdf
Tng, D. Y., Apgaua, D. M., Ishida, Y. F., Mencuccini, M., Lloyd, J., Laurance, W. F., et al. (2018). Rainforest trees respond to drought by modifying their hydraulic architecture. Ecol. Evol. 8, 12479–12491. 10.1002/ece3.4601 doi: 10.1002/ece3.4601
Tobin, M. F., Wright, A. J., Mangan, S. A., and Schnitzer, S. A. (2012). Lianas have a greater competitive effect than trees of similar biomass on tropical canopy trees. Ecosphere 3, 1–11. doi: 10.1890/ES11-00322.1
Toledo-Aceves, T. (2015). “Above- and belowground competition between lianas and trees,” in Ecology of Lianas, eds S. A. Schnitzer, F. Bongers, R. J. Burnham, and F. E. Putz (Chichester: John Wiley & Sons, Ltd.), 149–163. doi: 10.1002/9781118392409.ch12
Toledo-Aceves, T., and Swaine, M. D. (2008). Above-and below-ground competition between the liana Acacia kamerunensis and tree seedlings in contrasting light environments. Plant Ecol. 196, 233–244. doi: 10.1007/s11258-007-9347-0
Tymen, B., Réjou-Méchain, M., Dalling, J. W., Fauset, S., Feldpausch, T. R., Norden, N., et al. (2016). Evidence for arrested succession in a liana-infested Amazonian forest. J. Ecol. 104, 149–159. doi: 10.1111/1365-2745.12504
Uhl, C., Kauffman, J. B., and Cummings, D. L. (1988). Fire in the venezuelan Amazon 2: environmental conditions necessary for forest fires in the evergreen rainforest of Venezuela. Oikos 53, 176–184. doi: 10.2307/3566060
Unwin, G. L., Applegate, G. B., Stocker, G. C., and Nicholson, D. J. (1986). Initial effects of tropical cyclone ‘Winifred’ on forests in north Queensland. Proc. Ecol. Soci. Aust. 15, 283–296.
Van der Heijden, G. M., Feldpausch, T. R., de la Fuente Herrero, A., van der Velden, N. K., and Phillips, O. L. (2010). Calibrating the liana crown occupancy index in Amazonian forests. For. Ecol. Manage. 260, 549–555. doi: 10.1016/j.foreco.2010.05.011
Van der Heijden, G. M., Powers, J. S., and Schnitzer, S. A. (2015). Lianas reduce carbon accumulation and storage in tropical forests. Proc. Natl. Acad. Sci. U.S.A. 112, 13267–13271. doi: 10.1073/pnas.1504869112
Van der Heijden, G. M., Schnitzer, S. A., Powers, J. S., and Phillips, O. L. (2013). Liana impacts on carbon cycling, storage and sequestration in tropical forests. Biotropica 45, 682–692. doi: 10.1111/btp.12060
Van der Heijden, G. M. F., and Phillips, O. L. (2009). Liana infestation impacts tree growth in a lowland tropical moist forest. Biogeosciences 6, 2217–2226. doi: 10.5194/bg-6-2217-2009
Van Nes, E. H., Arani, B. M., Staal, A., van der Bolt, B., Flores, B. M., Bathiany, S., et al. (2016). What do you mean, ‘Tipping Point’? Trends Ecol. Evol. 31, 902–904. doi: 10.1016/j.tree.2016.09.011
Van Nes, E. H., Hirota, M., Holmgren, M., and Scheffer, M. (2014). Tipping points in tropical tree cover: linking theory to data. Glob. Chang. Biol. 20, 1016–1021. doi: 10.1111/gcb.12398
Verbeeck, H., and Kearsley, E. (2016). The importance of including lianas in global vegetation models. Proc. Natl. Acad. Sci. U.S.A. 113:E4. doi: 10.1073/pnas.1521343113
Vidal, E., Johns, J., Gerwing, J. J., Barreto, P., and Uhl, C. (1997). Vine management for reduced-impact logging in eastern Amazonia. For. Ecol. Manage. 98, 105–114. doi: 10.1016/S0378-1127(97)00051-0
Visser, M. D., Muller-Landau, H. C., Schnitzer, S. A., de Kroon, H., Jongejans, E., and Wright, S. J. (2018). A host–parasite model explains variation in liana infestation among co-occurring tree species. J. Ecol. 106, 2435–2445. doi: 10.1111/1365-2745.12997
Visser, M. D., Schnitzer, S. A., Muller-Landau, H. C., Jongejans, E., Kroon, H., Comita, L. S., et al. (2017). Tree species vary widely in their tolerance for liana infestation: a case study of differential host response to generalist parasites. J. Ecol. 106, 781–794. doi: 10.1111/1365-2745.12815
Waite, C. E., van der Heijden, G. M., Field, R., and Boyd, D. S. (2019). A view from above: unmanned aerial vehicles (UAVs) provide a new tool for assessing liana infestation in tropical forest canopies. J. Appl. Ecol. 56, 902–912. doi: 10.1111/1365-2664.13318
Walker, B. H., Carpenter, S. R., Rockstrom, J., Crépin, A. S., and Peterson, G. D. (2012). Drivers, slow variables, fast variables, shocks, and resilience. Ecol. Soc. 17:30. doi: 10.5751/ES-05063-170330
Webb, L. J. (1959). A physiognomic classification of Australian rain forests. J. Ecol. 47, 551–570. doi: 10.2307/2257290
West, G. B., Enquist, B. J., and Brown, J. H. (2009). A general quantitative theory of forest structure and dynamics. Proc. Natl. Acad. Sci. U.S.A. 106, 7040–7045. doi: 10.1073/pnas.0812294106
Wright, S. J., Calderón, O., Hernandéz, A., and Paton, S. (2004). Are lianas increasing in importance in tropical forests? A 17-year record from Panama. Ecology 85, 484–489. doi: 10.1890/02-0757
Wright, S. J., and Osvaldo, C. (2006). Seasonal, El Niño and longer term changes in flower and seed production in a moist tropical forest. Ecol. Lett. 9, 35–44. doi: 10.1111/j.1461-0248.2005.00851.x
Wright, S. J., Stoner, K. E., Beckman, N., Corlett, R. T., Dirzo, R., Muller-Landau, H. C., et al. (2007). The plight of large animals in tropical forests and the consequences for plant regeneration. Biotropica 39, 289–291. doi: 10.1111/j.1744-7429.2007.00293.x
Keywords: ecosystem health, liana, rainforest, succession, tipping point, tropics, vine, weed
Citation: Marshall AR, Platts PJ, Chazdon RL, Seki H, Campbell MJ, Phillips OL, Gereau RE, Marchant R, Liang J, Herbohn J, Malhi Y and Pfeifer M (2020) Conceptualising the Global Forest Response to Liana Proliferation. Front. For. Glob. Change 3:35. doi: 10.3389/ffgc.2020.00035
Received: 24 May 2019; Accepted: 06 March 2020;
Published: 24 April 2020.
Edited by:
Alice Catherine Hughes, Xishuangbanna Tropical Botanical Garden (CAS), ChinaReviewed by:
Tomas Ferreira Domingues, University of São Paulo, BrazilCopyright © 2020 Marshall, Platts, Chazdon, Seki, Campbell, Phillips, Gereau, Marchant, Liang, Herbohn, Malhi and Pfeifer. This is an open-access article distributed under the terms of the Creative Commons Attribution License (CC BY). The use, distribution or reproduction in other forums is permitted, provided the original author(s) and the copyright owner(s) are credited and that the original publication in this journal is cited, in accordance with accepted academic practice. No use, distribution or reproduction is permitted which does not comply with these terms.
*Correspondence: Andrew R. Marshall, YW1hcnNoYTFAdXNjLmVkdS5hdQ==
Disclaimer: All claims expressed in this article are solely those of the authors and do not necessarily represent those of their affiliated organizations, or those of the publisher, the editors and the reviewers. Any product that may be evaluated in this article or claim that may be made by its manufacturer is not guaranteed or endorsed by the publisher.
Research integrity at Frontiers
Learn more about the work of our research integrity team to safeguard the quality of each article we publish.