- 1U.S. Geological Survey, Alaska Science Center, University Drive, Anchorage, AK, United States
- 2Missoula Fire Sciences Laboratory, USDA Forest Service Rocky Mountain Research Station, Missoula, MT, United States
Complex, reciprocal interactions among climate, disturbance, and vegetation dramatically alter spatial landscape patterns and influence ecosystem dynamics. As climate and disturbance regimes shift, historical analogs and past empirical studies may not be entirely appropriate as templates for future management. The need for a better understanding of the potential impacts of climate changes on ecosystems is reaching a new level of urgency, especially in highly perturbed or vulnerable ecological systems. Simulation models are extremely useful tools for guiding management decisions in an era of rapid change, thus providing potential solutions for wicked problems in land management—those that are difficult to solve and inherently resistant to easily definable solutions. We identify three experimental approaches for landscape modeling that address management challenges in the context of uncertain climate futures and complex ecological interactions: (1) an historical comparative approach, (2) a future comparative approach, and (3) threshold detection. We provide examples of each approach from previously published studies of simulated climate, disturbance, and landscape dynamics in forested landscapes of the western United States, modeled with the FireBGCv2 ecosystem process model. Cumulatively, model outcomes indicate that typical land management strategies will likely not be sufficient to counteract the impacts of rapid climate change and altered disturbance regimes that threaten the stability of ecosystems. Without implementation of new, adaptive management strategies, future landscapes are very likely to be different than historical or contemporary ones, with significant and sometimes persistent changes triggered by interactions of climate and wildfire.
Introduction
Globally, climate changes have altered the timing, extent, frequency, and severity of wildfires (Westerling et al., 2006; Krawchuk et al., 2009; van Mantgem et al., 2013; Abatzoglou et al., 2018). Wildfire disturbance often occurs against a backdrop of more gradual changes resulting from shifting climate patterns (Hamann and Wang, 2006; Danby and Hik, 2007; Kelly and Goulden, 2008; Case and Lawler, 2017). In systems in which both biological and physical elements are simultaneously or serially perturbed, highly visible, rapidly occurring, and persistent changes in landscape composition and structure can occur. Interacting stressors of climate and uncharacteristic fire disturbance can trigger abrupt changes in ecosystems, including emergence of novel species assemblages, local extinctions, major shifts in forest composition, reduced biodiversity, and loss of ecosystem resilience (Root et al., 2003; Johnstone et al., 2010; Brown et al., 2015; Abatzoglou and Williams, 2016; Franklin et al., 2016; Loehman et al., 2018; Stevens-Rumann et al., 2018).
Highly perturbed ecological systems may exhibit complex, emergent behavior and non-linear feedbacks that produce novel and unanticipated landscape responses (Temperli et al., 2013; Buma, 2015; Coop et al., 2016). These may include profound shifts in successional dynamics, species composition, and loss of landscape carbon (Goetz et al., 2007; Johnstone et al., 2010; Brown and Johnstone, 2012; Thom et al., 2017). Climate changes, fire, and plant species or communities can interact in a complex of synergistic and antagonistic effects that amplify the negative impacts of natural disturbance on ecosystems (Figure 1). For example, in ecosystems with sufficient fuels to carry fire, warmer, drier climates are expected to increase fuel aridity, flammability, and fire activity (Gergel et al., 2017; McKenzie and Littell, 2017). The resulting larger extent of wildfire area burned and higher severity of wildfires can increase the proportion of the landscape in the early stages of post-fire recovery (Falk et al., 2019). If the post-fire bioclimatic environment is unfavorable for seedling establishment (e.g., with severe drought), forests may transition to alternative states such as shrub- or grasslands (Guiterman et al., 2018; Davis et al., 2019). Thus, interactions of altered climate and changing fire regimes impact vegetation regeneration, community structure and composition, and the amount, type, and flammability of fuels (Abatzoglou and Williams, 2016; Coop et al., 2016). Climate influence on wildfire regimes and ecosystems occurs in the context of other human impacts; for example, in the southwestern U.S., forests and fire regimes have been altered by more than 100 years of livestock grazing, logging, and fire exclusion, leading to high risk of severe fire associated with increased surface fuel loads and reduced structural and spatial heterogeneity of vegetation, especially in dry conifer forests with frequent-fire regimes (Covington and Moore, 1994; Allen et al., 2002; Allen, 2007; Reynolds et al., 2013). However, increased fire activity in fire-adapted but low-productivity ecosystems can be self-limiting, as fire-consumption of fuels can limit occurrence, extent, and effects of subsequent fires (Collins et al., 2007; Parks et al., 2015).
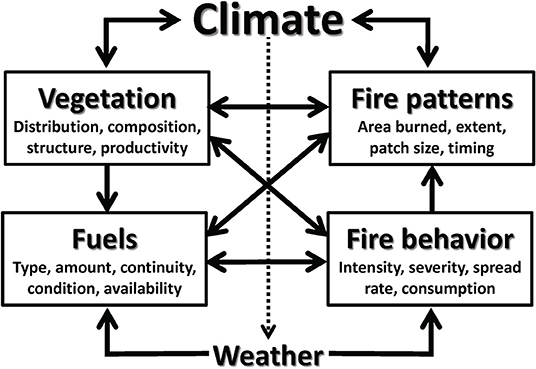
Figure 1. In fire-prone ecosystems, landscape vegetation patterns are determined by reciprocal interactions with fire and interactions within and among species and the surrounding environment. Climate and weather, vegetation, and fire interact in a complex of synergistic and antagonistic effects that amplify the impacts of disturbance on ecosystems. Climate influences landscape vegetation patterns and fuel characteristics at long time scales, whereas weather is short-term driver of fuel flammability and fire behavior. Globally, positive feedbacks may exist between climate warming, fire activity, carbon loss, and future climate change. This combination of scale dependent and spatially overlapping processes produces complex spatial patterns that are a central challenge in natural resource planning. Accounting for this complexity may require new approaches such as process-based simulation models that integrate multiple feedbacks, simultaneous interactions, and anthropogenic influences.
Forecasting ecological futures and evaluating potential impacts of human activities is a critical but challenging task in land management. Development of effective management strategies in the context of changing climate and wildfire regimes is a central challenge in natural resource planning, and may require new, agile approaches (Lawler, 2009; Falk, 2013). The rapid rate of contemporary climate change is exceeding the range of natural climate variability and accelerating the rate at which habitats are degraded and species are lost (Overpeck et al., 2003; Thomas et al., 2004; Hannah et al., 2005; Allen et al., 2015). From local to global scales, these changes co-occur with anthropogenic ecosystem disruptions including landscape fragmentation and urbanization, pollution, grazing, deforestation, non-native species invasions, and a new and unique “human pyrome,” or global expression of a fire regime (Vitousek, 1997; Millar et al., 2007; Archibald et al., 2013; Alencar et al., 2015; Blackhall et al., 2015). Whereas historically land management professionals used results from empirical studies coupled with their own expertise to plan and evaluate management activities, climate and fire futures—and ecological responses—may have few analogs in the past (Whitlock et al., 2003; Marlon et al., 2009). As a result, past empirical studies and the accrued wisdom of the last century may not completely inform management strategies for tomorrow's landscapes (Gustafson, 2013; Keane et al., 2015). Although continental-scale climate changes (e.g., increasing mean temperature) can be modeled with a high degree of accuracy and consistency, climate predictions at regional to local scales (scales that are relevant for land management) are more uncertain (Xie et al., 2015). This is particularly true for precipitation change, which is highly variable spatially in sign and amplitude. For these reasons, land management problems are “wicked” problems—those that are difficult to solve because of incomplete or variable information and are inherently resistant to clear definitions and easily identifiable, predefined solutions (Rittel and Webber, 1973; DeFries and Nagendra, 2017).
Simulation models—predictive relationships representing natural phenomena that are used for the purposes of exploration, scenario-building, projection, prediction, and forecasting (Reinhardt and Dickinson, 2010; Perera et al., 2015a)—are extremely effective tools for guiding management decisions in an era of rapid change (Cuddington et al., 2013). Models can be used to extrapolate limited empirical data over larger areas and longer time spans to provide greater spatiotemporal scope for management decisions (Keane, 2012), visualize the effects of alternative management strategies (Turner et al., 1995), explicitly incorporate ecological feedbacks, simulate interactions among various elements of the modeled system (e.g., climate, weather, biota, disturbances), and project emergent ecosystem responses as a result of changing conditions (Loehman et al., 2018). In the past, ecological modeling for resource management was limited by sufficient ecological knowledge necessary to build models, the lack of computer resources to run the models, and limited technical expertise to execute models (McKenzie et al., 2014; Keane et al., 2015). Today, there are numerous spatial and non-spatial ecological models that can be used to explore effects of management actions (Keane et al., 2004; He, 2008). Moreover, many of today's modelers incorporate climate into models' design, enabling them to be used for future climate change forecasts (Canelles et al., 2019; Gupta and Sharma, 2019).
Box (1979) observed that no real-world system can be exactly represented by a model, suggesting that we ask not whether any model is “true,” but rather whether it is illuminating and useful. Landscape and ecosystem models range in complexity from simple conceptual models such as state-and-transition models that simulate vegetation dynamics using discrete successional pathways (Wimberly, 2002; Tipton et al., 2018) to complex biogeochemical models that explain vegetation processes and related energy and matter exchanges between vegetation, soil, and the atmosphere (Keane et al., 2011; Dong et al., 2019). Simple models are easier to use and interpret but have a limited set of output variables that are often highly dependent on input parameters (Jørgensen and Bendoricchio, 2001). Complex models require abundant training, greater computing resources, longer simulation times, and more data to implement, but provide greater exploratory power and an extensive array of output variables (Grant and Swannack, 2011). Complex models also provide the ability to explicitly simulate emergent and dynamic processes (Lucash et al., 2018). There are tradeoffs between model complexity and practical utility for any particular problem, and a model's structure should be consistent with both the question(s) asked and the assessments being made by researchers and managers (Jackson et al., 2000). Recent advances in complex simulation modeling include a shift toward mechanistic models that are based on understanding and quantifying ecological processes, the integration of complex feedbacks and non-stationary behavior due to stochastic dynamics and changes in climate, and incorporation of disturbance interactions and anthropogenic influences (Perera et al., 2015b).
Experimental Approaches for Landscape Modeling
We identify three experimental approaches for landscape modeling that address the wicked management challenges resulting from uncertain climate futures and complex ecological interactions: historical comparative, future comparative, and threshold detection approaches. An historical comparative approach compares contemporary or projected future conditions to the range and variation of historical conditions (“historical range and variation,” or HRV). Historical, baseline conditions are generally defined as the period prior to European settlement, often corresponding to the availability of tree-ring or other long-term ecological records (Millar et al., 2007), although increasing recognition of the extent and importance of earlier human-caused landscape transformations (Bowman et al., 2011; Barak et al., 2016; Liebmann et al., 2016; Roos et al., 2018) warrants extending the HRV envelope to earlier periods in time. Historical conditions have been used extensively and successfully as references, benchmarks, or targets in ecosystem management (Hessburg et al., 1999; Keane et al., 2007; Dickinson, 2014), and as resilience metrics for evaluating ecosystems or landscapes to inform potential strategies and tactics (Keane et al., 2018). HRV assumes that variations of historical characteristics represent the broad envelope of responses possible for a resilient ecosystem under natural perturbations of climate, competitive stress, disturbances, and other stressors (Keane et al., 2018), and that potential responses to changing conditions can be represented by past responses to ecological conditions (Millar et al., 2007; Veblen et al., 2009). Simulation models are ideal tools for historical comparative approaches because comprehensive quantification of landscape HRV demands temporally deep, spatially explicit historical data, which are otherwise rarely available and difficult to obtain (Humphries and Bourgeron, 2001; Dickinson, 2014). Simulation modeling can also define the range and variability of future conditions (“future range and variation,” or FRV), and identify possible target areas for management in the overlap between HRV and FRV (Hansen et al., 2014; Keane et al., 2019).
In a future comparative approach, multiple scenarios (“futures”) are simulated over decades or centuries and results are used to evaluate ecosystem responses to perturbations and assess impacts of management (Figure 2). Future comparative experiments typically use fully factorial statistical designs where each major factor (e.g., climate change, management approach) has several implementation levels (e.g., different climate model projections and management prescriptions or treatment intensities), combinations of factors and levels are individual scenarios, and response variables are statistically compared across scenarios (Holsinger et al., 2014; Clark et al., 2017; Loehman et al., 2018). Including multiple interpretations of future climate accounts for some of the inherent uncertainty and variation in climate projections that result from emissions-scenario uncertainty, model-response uncertainty, and natural variability (Lugato and Berti, 2008; Deser et al., 2012). Future comparative modeling creates a robust, risk-free decision space wherein managers can explore consequences of potential adaptation strategies within the context of plausible climate futures (Peterson et al., 2003; Moss et al., 2010).
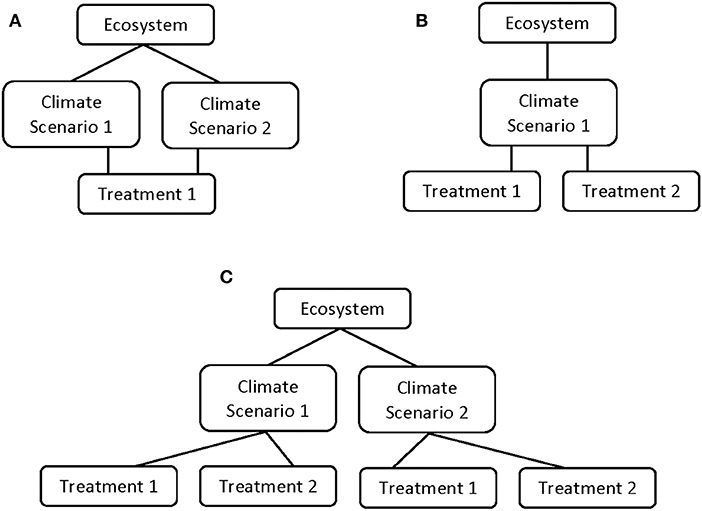
Figure 2. The future comparative modeling approach evaluates ecosystem responses under a range of climate futures and management strategies. Scenario comparisons can include impacts of several climates and a single management strategy (A), different management strategies within a single modeled climate (B), or factorial comparisons of several climate futures and management strategies (C). Adapted from Friggens et al. (2019).
The threshold detection approach identifies critical thresholds of climate or disturbance that induce rapid and persistent transformations of ecological systems (e.g., loss of resilience) (Holling, 1973). In many cases, ecological attributes show minimal change until a critical environmental threshold is reached (Qian et al., 2003). Process-based simulation modeling is deemed one of the only methods available to generate the spatially and temporally extensive data streams necessary to detect disturbance thresholds, and explicitly represent the important cross-scale process interactions that drive ecological tipping points (Reyer et al., 2015; Keane et al., 2019). For example, in climate-sensitive and fire-prone ecosystems, water limitations and warming temperatures can radically alter ecosystems and trigger wildfires that are uncharacteristically severe or frequent, capable of abruptly reorganizing vegetation and fuel patterns and setting the stage for future, novel fire regimes (Drever et al., 2006; Allen et al., 2010, 2015; Turner, 2010). Mechanistically linking incremental climate changes or degree of fire severity or frequency of burning to specific ecological outcomes using observational data alone is difficult, as these data may not explain which aspect of disturbance drives ecosystem responses, and future climates (Kreyling et al., 2014). Threshold shifts can be detected using a gradient design with multiple, finely incremented factor levels (e.g., degree or amount of change in one or more climate variables) spanning the range of possible values for the factor. Threshold detection is an important aspect of ecological risk assessment and environmental management intended to prevent severe social, economic and environmental impacts that occur when biophysical thresholds are crossed (Kelly et al., 2015).
Modeling Complex Climate, Wildfire, and Vegetation Interactions
Here, we illustrate historical comparative, future comparative, and threshold detection modeling approaches using simulations of climate change, vegetation, and disturbance interactions in forested landscapes across the western United States. These were all produced using the FireBGCv2 landscape-scale, ecosystem-fire process model, a platform ideal for informing land management in an era of rapid and uncharted environmental change, as it provides insights that would not arise from simpler, non-spatial, and empirical models (Bestelmeyer et al., 2011; Scheller, 2018). As described in Keane et al. (2011), the model operates across hierarchical spatial scales from landscape, stand, plot, and species to individual trees with attributes such as species, age, height, diameter at breast height (DBH), and leaf area. Modeled climate, wildland fire, and landscape vegetation are dynamically and reciprocally linked; long-term records of daily temperature, precipitation, and radiation influence fuel production and moisture, which determine landscape ignition potential, fire frequency and size, and fire behavior. Climate and weather influence the productivity and mortality rates of individual plant species—and thus stand composition and structure—with feedbacks to the fire regime via fuel type, fuel amount, and fuel arrangement. Fire regimes in turn affect vegetation species' regeneration, composition, successional trajectories, and productivity directly through fire-caused mortality and successional patterns, and indirectly through influence on availability of light, water, and other necessary resources. During initialization, FireBGCv2 standardizes input site-specific mean fire return intervals (MFRI) with the Keetch Byram Drought Index (KBDI, Keetch and Byram, 1968), computed from the input weather records. Climate changes and impacts on fire and vegetation can be simulated in the model via daily weather streams that represent future climates, either acquired directly from downscaled climate models or calculated as adjustments to observed daily weather. Fire ignition probabilities are computed each year for each simulation landscape pixel based on the degree of departure of each modeling year's weather and the standardized KDBI-MFRI distribution. Once a fire ignition has occurred, its spread is quasi-mechanistically simulated along gradients of slope and wind. Spread is halted if a fire encounters a pixel with a lower fine fuel loading than the user-specified threshold for fire spread, or fuels that are too moist to sustain fire. Otherwise, fires spread until they reach a stochastically determined fire size computed from the current year's weather and a user-defined mean fire size parameter. Ultimately, fire spread ends when a fire reaches the edge of the simulation landscape or a pixel with insufficient fine fuel to carry fire, or when it has met the stochastically computed fire size. In FireBGCv2 climate changes influence fire patterns along a number of pathways. Fire frequency increases with increasing KBDI, warmer and drier weather lowers fuel moistures and increases the probability of spreading fires, and changes in the amount of type of fuel on the simulation landscape changes the spatial arrangement and behavioral characteristics of fires. Individual tree mortality occurs as the result of wildfire damage, hydrologic stress, crowding, light reduction, and random mortality. Fire-caused tree mortality is modeled as a function of bark thickness (a user-defined, species-specific parameter) and scorch height, and can be used to assess fire severity where the degree of crown scorch and cambial kill depends on fire intensity and duration. Thermal limits are defined for each species in the model (GDD, base 3°C) and temperatures outside of these limits affect trees through a reduction in the annual growth increment and eventual mortality. Tree regeneration is driven by soil moisture, litter depth, and climate-influenced cone crop production.
Historical Comparative Simulation Modeling
Keane et al. (2018) provided a comparative, historical reference for contemporary and future ecological states, where substantial departures of FRV from HRV indicated loss of resilience. Response variables—vegetation composition and structure, tree basal area, coarse and fine woody debris, outflow, net primary productivity, and area burned—were derived for the East Fork of the Bitterroot River watershed, a 128,000-ha landscape in the interior northern Rocky Mountains, USA, for an historical time period not influenced by land management or fire suppression, and three future scenarios that combined future climate [CRM-C5 RCP8.5 (+ 5.5°C, 95% baseline precipitation)] with varying levels of fire suppression (0%, FRV1; 50%, FRV2; 98%, FRV3). These were compared in a multivariate framework (principal components analysis, PCA) that indicated that the East Fork landscape departed from its HRV benchmark under all levels of fire suppression enacted within a future climate (Figure 3). Zones of overlap among the three future scenarios and HRV were smaller with increasing fire suppression levels, suggesting that suppression is limited in its ability to ameliorate undesired wildfire impacts given the potentiating effects of warmer, drier climates on fire frequency and severity. Results from Keane et al. (2018) are consistent with recent publications on the effectiveness of fire management activities under changing climates; in particular, indications that treatments may be less effective in systems where future fire patterns are influenced more by climate than by fuels (Littell et al., 2009), and recommendations for adaptive management approaches that include increased use of prescribed fire, much reduced fire suppression, and recognition of the limited ability of fuel treatments to alter regional fire patterns (Schoennagel et al., 2017).
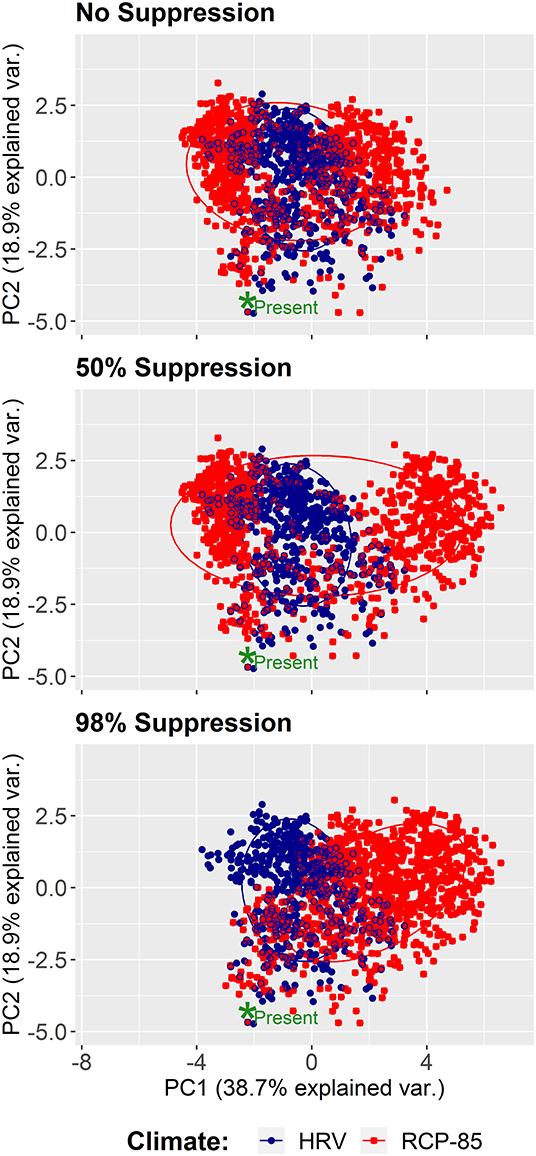
Figure 3. Principal components analysis (PCA) of multivariate model responses for the East Fork of the Bitterroot River watershed, Montana, USA, for an historical time period (HRV, blue dots) and three future scenarios that combined future climate (CRM-C5 RCP8.5) with three levels of fire suppression (0%, 50%, 98%, red dots). Red or blue circles contain 68% of the variation in the spread of the points for the three scenarios; the green asterisk represents the present state of the landscape in multivariate space. Overlapping HRV and FRV zones suggest less departure from historical conditions and greater landscape resilience; increasing distance between HRV and FRV occurred with higher fire suppression levels, suggesting that suppression may not be an effective management strategy under changing climates. Adapted from Keane et al. (2018).
Loehman (2016) modeled changes in fire occurrence in a Ponderosa pine-dominated landscape in the Jemez Mountains of north-central New Mexico in response to prehistoric human activities of fuelwood gathering and tree harvest—activities that disrupted landscape fuel continuity, reduced surface and canopy fuel loads, and altered fire occurrence. In simulations with relatively small, spatially concentrated populations (<500 people, ca. 1200-1325 AD) human impact on landscape fire occurrence occurred mainly within the area of occupation, but as populations increased (>5,000 people, ca. 1350-1525 AD) and expanded, human activities reduced fire occurrence in outlying, unoccupied areas (Figure 4)—consistent with contemporary observations of decreased fire frequency in upper elevation, western U.S. forests attributed to fire suppression and disrupted fire spread from lower elevations (Margolis and Balmat, 2009). This study and a related, growing body of research highlights the significant role of anthropogenic burning in some landscapes, even in environments with abundant natural ignitions (Guyette et al., 2002; Liebmann et al., 2016; Roos et al., 2019). In these places, contemporary ecological patterns and processes that are thought to be natural may in fact be highly influenced by past human land use legacies.
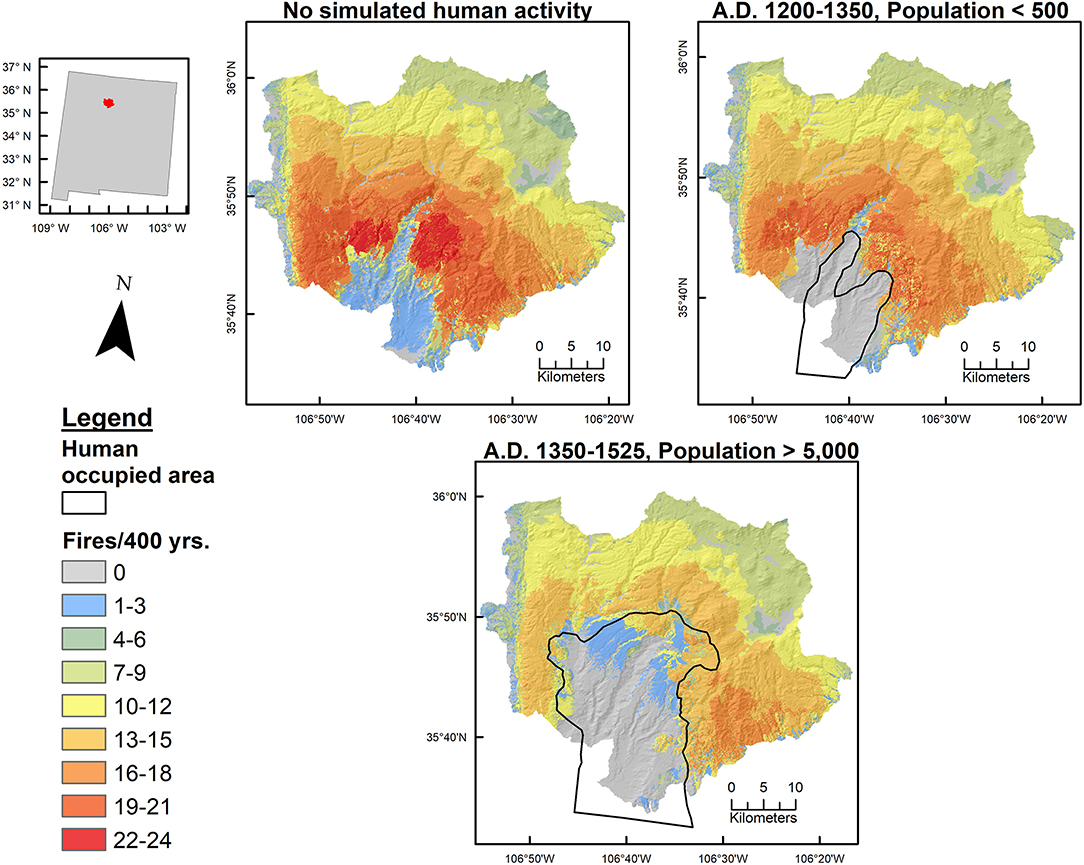
Figure 4. Fire occurrence (cumulative fires/400 years) simulated for two historical time periods in the Jemez Mountains, New Mexico, U.S. As compared with a scenario in which prehistoric human activities were not included, modeled surface and canopy fuelwood gathering and tree harvest reduced fire occurrence proportional to population size (< 500 people or > 5,000 people) and extent of activity area (indicated by black polygons). Changes to fire regimes occurred because of human-influenced changes in the amount, type, and arrangement of fuels, against a backdrop of climate variability. Adapted from Loehman (2016).
Future Comparative Simulation Modeling
Future comparative FireBGCv2 modeling studies address ecosystem impacts of climate change (Clark et al., 2017) or climate change and management activities (Loehman et al., 2018) on fire-prone ecosystems. In Yellowstone National Park, Wyoming, U.S modeled warmer future climates, especially >2°C, increased the amount of fire on the landscape, resulting in decreased forest cover and a change in species composition from lodgepole pine- to Douglas-fir-dominated stands (Figure 5) (Clark et al., 2017). Species conversion was attributed to complex mechanisms related to increased fire activity and greater fire and drought tolerance of Douglas-fir as compared with lodgepole pine. This future pathway was not identified in other simulations of climate and fire impacts within the region (Smithwick et al., 2011; Westerling et al., 2011), because the models used did not incorporate complex and long-term dynamics and feedbacks of climate, vegetation, and wildfire.
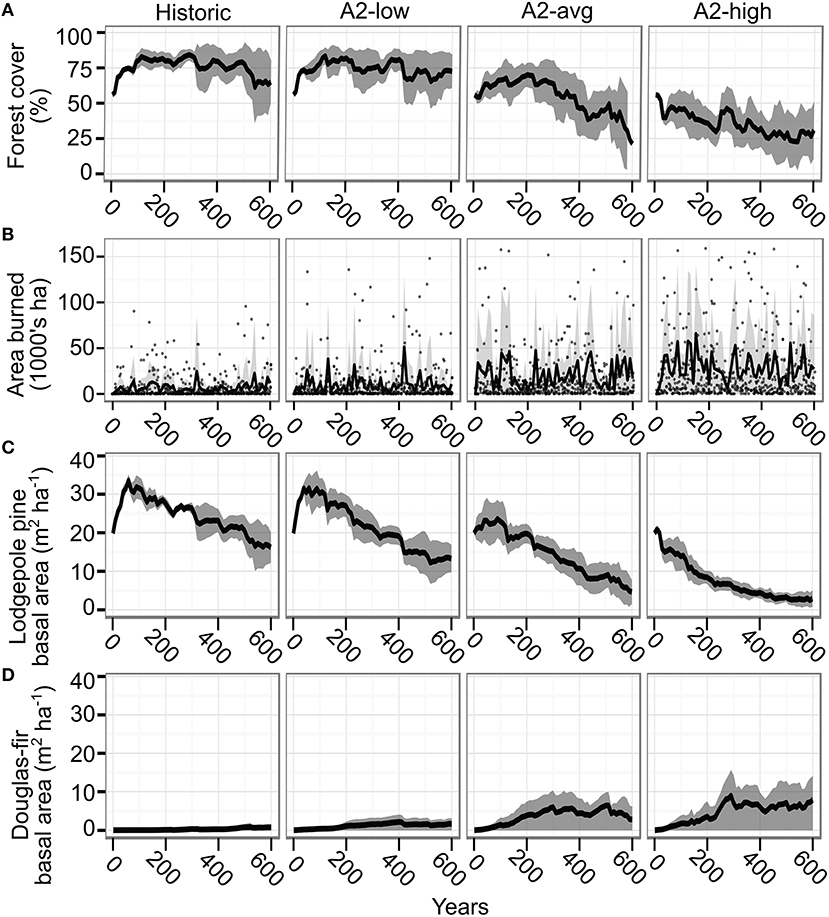
Figure 5. Time series of simulation landscape variables: forest cover (A), area burned (B), lodgepole pine basal area (C), and Douglas-fir basal area (D). Black lines represent means of replicate model simulations, gray-shaded areas represent ±1 standard deviation, and black dots in (B) represent total area burned for a given year. Warmer future climates, especially >2°C, increased the amount of fire on the landscape, resulting in decreased forest cover and a change in dominant species composition from lodgepole pine- to Douglas-fir-dominated stands. Adapted from Clark et al. (2017).
Loehman et al. (2018) tracked simulated changes in forest basal area in response to climate and management activities in a fire-adapted and fire-prone forested landscape in the Jemez Mountains of north-central New Mexico, U.S. Basal area is an indicator of forest cover and stand structure that reflects both climate conditions that influence tree growth and survival and fire effects on tree mortality and stand establishment. Management activities, developed from local prescriptions and burn plans, included thinning and prescribed fire treatments implemented annually in dry forest stands of the simulation area. “Business as usual” (BAU) management activities corresponded to a 66-years treatment rotation, and future management was modeled as a three-fold (3xBAU, 22-years treatment rotation) or 6-fold (6× BAU, 11-years treatment rotation) annual increase in BAU, or a fire suppression treatment (90% suppression level). Among three modeled climate scenarios—Contemporary, Warm-Dry (CCSM4 RCP 4.5), and Hot-Arid (HadGEM2ES RCP 8.5)—basal area declined substantially over the Hot-Arid as compared with Contemporary or Warm-Dry climate (Figure 6). Loss of basal area throughout the 100-year climate period was attributed to tree mortality, regeneration failure, and compositional and structural shifts to shrublands and early successional forests caused by wildfires, climate stress, and changes in the distribution of bioclimatic space suitable for plant growth. Although fuel treatments have been shown to be highly effective at reducing potential fire severity at stand scales (Pollet and Omi, 2002; Wimberly et al., 2009), thinning/prescribed burning treatments at BAU or intensified application rates were not sufficient to offset impacts of warming climate on forests at a landscape scale.
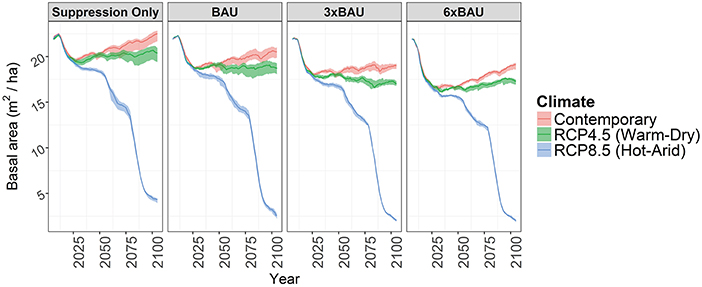
Figure 6. Basal area (m2/ha) of ponderosa pine and dry mixed conifer sites for a Ponderosa pine-dominated landscape in the Jemez Mountains, New Mexico, U.S. Scenarios are factorial combinations of management (“business as usual,” BAU; three-fold (3×BAU) or six-fold (6×BAU) annual increase in BAU; 90% fire suppression) and climate (Contemporary; Warm-Dry; Hot-Arid). Shaded regions show median (darker line) and 25th and 75th percentiles (lighter shading) among model replicates for each scenario. With Hot-Arid climate, basal area decreased early in the simulation period ca. AD 2025, and by AD 2100 was about 10 percent of its starting amount. Basal area was maintained with Warm-Dry climate and any of the four management factors. Adapted from Loehman et al. (2018).
Detection of Critical Thresholds
Keane and Loehman (2012) systematically varied climate drivers to detect climatic thresholds related to changes in landscape vegetation and fire regimes. Modeling scenarios were 42 combinations of temperature factors (ranging from 1 to 6 degrees Celsius (°C) in 1-degree increments) and precipitation factors (ranging from 70 to 130 percent in 10-percent increments) used to modify long-term, daily baseline instrumental weather. This approach, commonly referred to as the “delta” method, retains the inherent variability in observed climate data (has a high level of climate realism) but only accounts for changes to the mean climate signal (Ekström et al., 2015). Climate shifts spanned the range of climate model projections for Yellowstone National Park, Wyoming, U.S., but provided a finer gradient of temperature and precipitation change than climate models based on discrete emissions scenarios. Simulation detected several climatic tipping points beyond which landscape patterns and fire regimes were significantly and persistently different from reference conditions. These included substantial decreased forest cover caused by warmer, drier climate conditions, with a buffering effect of precipitation for moderate warming levels of 3 degrees or less (Figure 7), as well as an increase in annual burned area increased with increasing temperature.
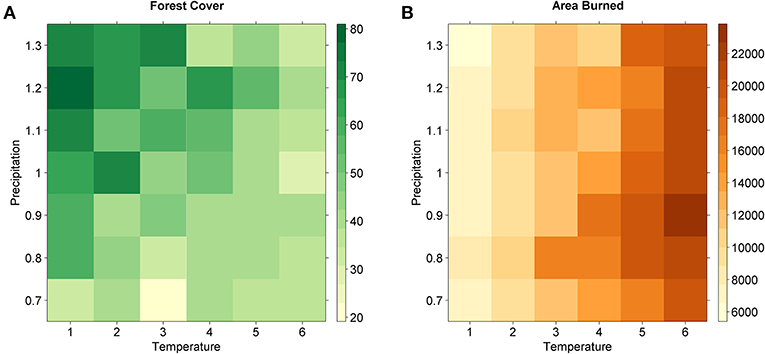
Figure 7. Forest cover (A, percent of simulation area) and area burned (B, ha) simulated for Yellowstone National Park, Wyoming, U.S., for scenarios combining warming of 1–6 degrees Celsius (°C) and precipitation change of 70–130%. Forest cover and area burned responses for each scenario are means across simulation periods and replicates. Adapted from Keane and Loehman (2012).
Discussion
Climate changes are widely recognized as the largest threat to biodiversity, species survival, and ecosystem integrity across most of Earth's biomes (Hulme, 2005; Thuiller et al., 2008; Maclean and Wilson, 2011), challenging historical interpretations, foundational assumptions, and attribution of ecological and evolutionary change. Approaches that consider the full ensemble of processes and feedbacks in biological systems and their intersection with human land-use legacies and policy are necessary to address the fundamental challenges of 21st century land management—anticipating risk, fostering resilience, and acting within the context of uncertainty (Carpenter et al., 2009; Seidl, 2014). The need for a better understanding of the potential impacts of climate changes on ecosystems is reaching new levels of urgency. A common finding among recent papers evaluating the effectiveness of fire management and forest restoration activities in the western U.S. under changing climates is the limited ability of current strategies to ameliorate undesired wildfire impacts in many ecological systems (Stephens et al., 2012; Svenning and Sandel, 2013; Schoennagel et al., 2017). As a path forward, Stephens et al. (2013) suggested that new strategies to mitigate and adapt to increased fire are needed to sustain fire-prone forest landscapes (e.g., promote resilience) including the restoration of historical stand conditions in high frequency, low-to-moderate severity fire regimes, while allowing for shifts away from historical forest structure and composition in forests with low-frequency, high-severity fire regimes. As observed by Svenning and Sandel (2013), facilitating the adaptation of forests to changing climate and fire regimes may ultimately create more resilient systems as vegetation communities come into equilibrium with climate. Schoennagel et al. (2017) indicated the importance of adaptive management approaches that include increased use of prescribed fire, much reduced fire suppression, and recognition of the limited ability of fuel treatments to alter regional fire patterns.
Simulation modeling provides useful guidance for managers in the context of rapid and unanticipated landscape changes. Three modeling approaches are particularly applicable for land management: historical comparisons that increase our understanding of the dynamic nature of landscapes and provide a frame of reference for assessing contemporary patterns and processes (Swetnam et al., 1999); future comparative modeling that enables risk-free exploration of management impacts within the context of plausible climate futures (Peterson et al., 2003; Moss et al., 2010); and threshold detection that identifies critical disturbance thresholds that lead to loss of ecosystem stability. Model outcomes can be used to game multiple scenarios and gain critical insight on the range of magnitudes and direction of possible future changes (Millar et al., 2007), define the critical array of multiple and interacting links that define a complex system (Game et al., 2014), and encourage action to address looming management challenges in systems characterized by overwhelming complexity (DeFries and Nagendra, 2017).
Although the simulation modeling projects described here were developed for different objectives and geographies, they indicate a consistent set of outcomes across a diversity of landscapes and ecosystems within the western U.S. First, future landscapes are likely to be different than historical or contemporary landscapes. Shifts in vegetation and fire regimes were associated with nearly all simulated levels of climate change, but in particular for scenarios with both increased warming and increased drying. Such changes are consistent with projections of future climate in the western United States, which is expected to warm by ~2–4°C during the 21st century, with associated increased frequency and persistence of drought conditions (Diffenbaugh et al., 2005; Christensen et al., 2007). Second, interactions of climate and wildfire are likely to cause more rapidly occurring and persistent changes in landscapes than climate change alone. As noted by Flannigan et al. (2000; p. 227), “The almost instantaneous response of the fire regime to changes in climate has the potential to overshadow importance of direct effects of global warming on species distribution, migration, substitution and extinction…fire is a catalyst for vegetation change.” Third, current land management strategies are likely not sufficient to counteract the impacts of rapid climate change and altered disturbance regimes that threaten the stability of ecosystems (Falk et al., 2007).
Simulation modeling is a dynamic field, challenged by ecological complexities and emerging, non-analog system drivers and responses. At the center of future modeling research is a need for ongoing empirical studies that provide comprehensive calibration data and parameters that reflect emerging environments. Models developed using empirical data representative of historical conditions become less robust under climate change, because species dynamics—for example, seedling establishment rates after wildfire—are different in novel, non-equilibrium environments (Scheller, 2018). The balance of data needs vs. model advancement reflects an imperative for collaboration between field ecologists, who provide data and equations, and modelers, who must then integrate that knowledge to provide descriptions of phenomena at different spatial and temporal scales. It is critical that extensive field programs be intimately integrated with simulation efforts to ensure sufficient parameter and validation data are measured for model applications. Temporally deep, spatially explicit databases created from extensive field measurements are needed to quantify input parameters, describe initial conditions, and provide a reference for model testing and validation, especially as landscape fire models are ported across large geographic areas and to new ecosystems (Cary et al., 2006). For example, Hessl et al. (2004) compiled a number of ecophysiological parameters for use in mechanistic ecosystem models, which has increased parameter standardization and decreased the time modelers spend on parameterization. New sampling methods and techniques for collecting data are needed to ensure that essential variables are measured at the proper scales; once collected, data should be stored in standardized, accessible databases so that they are easily accessible for complex modeling tasks. Comparative modeling studies using such standardized data sets can identify key uncertainties and areas for model improvement and increase our understanding of the key processes and parameters affecting diverse ecosystems (Cary et al., 2006; French et al., 2011).
Data Availability Statement
The datasets generated for this study are available on request to the corresponding author.
Author Contributions
RL, RK, and LH conceived of and designed the simulation experiments described herein. RL and LH performed the experiments, analyzed the data, and produced summary data and graphics. RL and RK wrote the paper. Any use of trade, firm, or product names is for descriptive purposes only and does not imply endorsement by the U.S. Government.
Funding
Funding for the FireBGCv2 modeling projects described here was provided by the Joint Fire Science Program (Projects 16-3-01-14, 15-1-03-26, and 09-3-01-17) and the National Science Foundation Coupled Natural and Humans Systems Program (project 1114898).
Conflict of Interest
The authors declare that the research was conducted in the absence of any commercial or financial relationships that could be construed as a potential conflict of interest.
References
Abatzoglou, J. T., and Williams, A. P. (2016). Impact of anthropogenic climate change on wildfire across western US forests. Proc. Natl. Acad. Sci. U.S.A. 113, 11770–11775. doi: 10.1073/pnas.1607171113
Abatzoglou, J. T., Williams, A. P., Boschetti, L., Zubkova, M., and Kolden, C. A. (2018). Global patterns of interannual climate–fire relationships. Global Change Biol. 24, 5164–5175. doi: 10.1111/gcb.14405
Alencar, A. A., Brando, P. M., Asner, G. P., and Putz, F. E. (2015). Landscape fragmentation, severe drought, and the new Amazon forest fire regime. Ecol. Appl. 25, 1493–1505. doi: 10.1890/14-1528.1
Allen, C. D. (2007). Interactions across spatial scales among forest dieback, fire, and erosion in northern New Mexico landscapes. Ecosystems 10, 797–808.
Allen, C. D., Breshears, D. D., and McDowell, N. G. (2015). On underestimation of global vulnerability to tree mortality and forest die-off from hotter drought in the Anthropocene. Ecosphere 6, 1–55. doi: 10.1890/ES15-00203.1
Allen, C. D., Macalady, A. K., Chenchouni, H., Bachelet, D., McDowell, N., Vennetier, M., et al. (2010). A global overview of drought and heat-induced tree mortality reveals emerging climate change risks for forests. Forest Ecol. Manag. 259, 660–684. doi: 10.1016/j.foreco.2009.09.001
Allen, C. D., Savage, M., Falk, D. A., Suckling, K. F., Swetnam, T. W., Schulke, T., et al. (2002). Ecological restoration of southwestern ponderosa pine ecosystems: a broad perspective. Ecol. Appl. 12, 1418–1433.
Archibald, S., Lehmann, C. E., Gómez-Dans, J. L., and Bradstock, R. A. (2013). Defining pyromes and global syndromes of fire regimes. Proc. Natl. Acad. Sci. U.S.A. 110, 6442–6447. doi: 10.1073/pnas.1211466110
Barak, R. S., Hipp, A. L., Cavender-Bares, J., Pearse, W. D., Hotchkiss, S. C., Lynch, E. A., et al. (2016). Taking the long view: Integrating recorded, archeological, paleoecological, and evolutionary data into ecological restoration. Int. J. Plant Sci. 177, 90–102. doi: 10.1086/683394
Bestelmeyer, B. T., Goolsby, D. P., and Archer, S. R. (2011). Spatial perspectives in state-and-transition models: a missing link to land management? J. Appl. Ecol. 48, 746–757. doi: 10.1111/j.1365-2664.2011.01982.x
Blackhall, M., Veblen, T. T., and Raffaele, E. (2015). Recent fire and cattle herbivory enhance plant-level fuel flammability in shrublands. J. Veg. Sci. 26, 123–133. doi: 10.1111/jvs.12216
Bowman, D. M., Balch, J., Artaxo, P., Bond, W. J., Cochrane, M. A., D'antonio, C. M., et al. (2011). The human dimension of fire regimes on Earth. J. Biogeogr. 38, 2223–2236. doi: 10.1111/j.1365-2699.2011.02595.x
Box, G. E. (1979). “Robustness in the strategy of scientific model building,” in Robustness in Statistics eds R. L. Launer and G. N. Wilkinson (New York, NY: Academic Press), 201–236. doi: 10.1016/B978-0-12-438150-6.50018-2
Brown, C. D., and Johnstone, J. F. (2012). Once burned, twice shy: repeat fires reduce seed availability and alter substrate constraints on Picea mariana regeneration. Forest Ecol. Manag. 266, 34–41. doi: 10.1016/j.foreco.2011.11.006
Brown, C. D., Liu, J., Yan, G., and Johnstone, J. F. (2015). Disentangling legacy effects from environmental filters of postfire assembly of boreal tree assemblages. Ecology 96, 3023–3032. doi: 10.1890/14-2302.1
Buma, B. (2015). Disturbance interactions: characterization, prediction, and the potential for cascading effects. Ecosphere 6, 1–15. doi: 10.1890/ES15-00058.1
Canelles, Q., Aquilué, N., Duane, A., and Brotons, L. (2019). From stand to landscape: modelling post-fire regeneration and species growth. Ecol. Model. 404, 103–111. doi: 10.1016/j.ecolmodel.2019.05.001
Carpenter, S. R., Mooney, H. A., Agard, J., Capistrano, D., DeFries, R. S., Díaz, S., et al. (2009). Science for managing ecosystem services: beyond the millennium ecosystem assessment. Proc. Natl. Acad. Sci. U.S.A. 106, 1305–1312. doi: 10.1073/pnas.0808772106
Cary, G. J., Keane, R. E., Gardner, R. H., Lavorel, S., Flannigan, M. D., Davies, I. D., et al. (2006). Comparison of the sensitivity of landscape-fire-succession models to variation in terrain, fuel pattern, climate and weather. Landsc. Ecol. 21, 121–137. doi: 10.1007/s10980-005-7302-9
Case, M. J., and Lawler, J. J. (2017). Integrating mechanistic and empirical model projections to assess climate impacts on tree species distributions in northwestern North America. Global Change Biol. 23, 2005–2015. doi: 10.1111/gcb.13570
Christensen, J. H., Hewitson, B., Busuioc, A., Chen, A., Gao, X., Held, I., et al. (2007). “Regional climate projections,” in Climate Change 2007: The Physical Science Basis. Contribution of Working Group I to the Fourth Assessment Report of the Intergovernmental Panel on Climate Change, eds S. Solomon, D. Qin, M. Manning, Z. Chen, M. Marquis, K. B. Averyt, et al. (Cambridge; New York, NY: Cambridge University Press), 848–940.
Clark, J. A., Loehman, R. A., and Keane, R. E. (2017). Climate changes and wildfire alter vegetation of Yellowstone National Park, but forest cover persists. Ecosphere 8:e01636. doi: 10.1002/ecs2.1636
Collins, B. M., Kelly, M., van Wagtendonk, J. W., and Stephens, S. L. (2007). Spatial patterns of large natural fires in Sierra Nevada wilderness areas. Landsc. Ecol. 22, 545–557. doi: 10.1007/s10980-006-9047-5
Coop, J. D., Parks, S. A., McClernan, S. R., and Holsinger, L. M. (2016). Influences of prior wildfires on vegetation response to subsequent fire in a reburned southwestern landscape. Ecol. Appl. 26, 346–354. doi: 10.1890/15-0775
Covington, W. W., and Moore, M. M. (1994). Southwestern ponderosa forest structure: changes since Euro-American settlement. J. Forest. 92, 39–47.
Cuddington, K., Fortin, M. J., Gerber, L. R., Hastings, A., Liebhold, A., O'Connor, M., et al. (2013). Process-based models are required to manage ecological systems in a changing world. Ecosphere 4, 1–12. doi: 10.1890/ES12-00178.1
Danby, R. K., and Hik, D. S. (2007). Variability, contingency and rapid change in recent subarctic alpine tree line dynamics. J. Ecol. 95, 352–363. doi: 10.1111/j.1365-2745.2006.01200.x
Davis, K. T., Dobrowski, S. Z., Higuera, P. E., Holden, Z. A., Veblen, T. T., Rother, M. T., et al. (2019). Wildfires and climate change push low-elevation forests across a critical climate threshold for tree regeneration. Proc. Natl. Acad. Sci. U.S.A. 116, 6193–6198. doi: 10.1073/pnas.1815107116
DeFries, R., and Nagendra, H. (2017). Ecosystem management as a wicked problem. Science 356, 265–270. doi: 10.1126/science.aal1950
Deser, C., Knutti, R., Solomon, S., and Phillips, A. S. (2012). Communication of the role of natural variability in future North American climate. Nat. Clim. Change 2:775. doi: 10.1038/nclimate1562
Dickinson, Y. (2014). Landscape restoration of a forest with a historically mixed-severity fire regime: what was the historical landscape pattern of forest and openings? Forest Ecol. Manag. 331, 264–271. doi: 10.1016/j.foreco.2014.08.018
Diffenbaugh, N. S., Pal, J. S., Trapp, R. J., and Giorgi, F. (2005). Fine-scale processes regulate the response of extreme events to global climate change. Proc. Natl. Acad. Sci. U.S.A. 102, 15774–15778. doi: 10.1073/pnas.0506042102
Dong, Z., Driscoll, C. T., Johnson, S. L., Campbell, J. L., Pourmokhtarian, A., Stoner, A. M., et al. (2019). Projections of water, carbon, and nitrogen dynamics under future climate change in an old-growth Douglas-fir forest in the western Cascade Range using a biogeochemical model. Sci. Total Environ. 656, 608–624. doi: 10.1016/j.scitotenv.2018.11.377
Drever, C. R., Peterson, G., Messier, C., Bergeron, Y., and Flannigan, M. (2006). Can forest management based on natural disturbances maintain ecological resilience? Can. J. For. Res. 36, 2285–2299. doi: 10.1139/x06-132
Ekström, M., Grose, M. R., and Whetton, P. H. (2015). An appraisal of downscaling methods used in climate change research. Wiley Interdiscipl. Rev. Clim. Change 6, 301–319. doi: 10.1002/wcc.339
Falk, D. A. (2013). “Are Madrean ecosystems approaching tipping points? Anticipating interactions of landscape disturbance and climate change,” in Merging Science and Management in a Rapidly Changing World: Biodiversity and Management of the Madrean Archipelago III and 7th Conference on Research and Resource Management in the Southwestern Deserts; 2012 May 1-5; Tucson, AZ. Proceedings. RMRS-P-67, Vol. 67, eds G. J. Gottfried, P. F. Ffolliott, B. S. Gebow, L. G. Eskew, and L. C. Collins (Fort Collins, CO: US Department of Agriculture, Forest Service, Rocky Mountain Research Station), 40–47.
Falk, D. A., Miller, C., McKenzie, D., and Black, A. E. (2007). Cross-scale analysis of fire regimes. Ecosystems 10, 809–823. doi: 10.1007/s10021-007-9070-7
Falk, D. A., Watts, A. C., and Thode, A. E. (2019). Scaling ecological resilience. Front. Ecol. Evol. 7:275. doi: 10.3389/fevo.2019.00275
Flannigan, M., Stocks, B., and Wotton B. (2000). Climate change and forest fires. Sci. Total Environ. 262, 221–229. doi: 10.1016/s0048-9697(00)00524-6
Franklin, J., Serra-Diaz, J. M., Syphard, A. D., and Regan, H. M. (2016). Global change and terrestrial plant community dynamics. Proc. Natl. Acad. Sci. U.S.A. 113, 3725–3734. doi: 10.1073/pnas.1519911113
French, N. H., de Groot, W. J., Jenkins, L. K., Rogers, B. M., Alvarado, E., Amiro, B., et al. (2011). Model comparisons for estimating carbon emissions from North American wildland fire. J. Geophys. Res. Biogeosci. 116:G00K05. doi: 10.1029/2010JG001469
Friggens, M., Loehman, R., Thode, A., Flatley, W., Evans, A., Bunn, W., et al. (2019). User Guide to the FireCLIME Vulnerability Assessment (VA) Tool: A Rapid and Flexible System for Assessing Ecosystem Vulnerability to Climate-Fire Interactions. Gen. Tech. Rep. RMRS-GTR-395. Fort Collins, CO: U.S. Department of Agriculture, Forest Service, Rocky Mountain Research Station, 42.
Game, E. T., Meijaard, E., Sheil, D., and McDonald-Madden, E. (2014). Conservation in a wicked complex world; challenges and solutions. Conserv. Lett. 7, 271–277. doi: 10.1111/conl.12050
Gergel, D. R., Nijssen, B., Abatzoglou, J. T., Lettenmaier, D. P., and Stumbaugh, M. R. (2017). Effects of climate change on snowpack and fire potential in the western USA. Clim. Change 141, 287–299. doi: 10.1007/s10584-017-1899-y
Goetz, S., Mack, M., Gurney, K., Randerson, J., and Houghton, R. (2007). Ecosystem responses to recent climate change and fire disturbance at northern high latitudes: observations and model results contrasting northern Eurasia and North America. Environ. Res. Lett. 2:045031. doi: 10.1088/1748-9326/2/4/045031
Grant, W. E., and Swannack, T. M. (2011). Ecological Modeling: A Common-Sense Approach to Theory and Practice. New York: John Wiley & Sons.
Guiterman, C. H., Margolis, E. Q., Allen, C. D., Falk, D. A., and Swetnam, T. W. (2018). Long-term persistence and fire resilience of oak shrubfields in dry conifer forests of northern New Mexico. Ecosystems 21, 943–959. doi: 10.1007/s10021-017-0192-2
Gupta, R., and Sharma, L. K. (2019). The process-based forest growth model 3-PG for use in forest management: a review. Ecol. Model. 397, 55–73. doi: 10.1016/j.ecolmodel.2019.01.007
Gustafson, E. (2013). When relationships estimated in the past cannot be used to predict the future: using mechanistic models to predict landscape ecological dynamics in a changing world. Landsc. Ecol. 28, 1429–1437. doi: 10.1007/s10980-013-9927-4
Guyette, R. P., Muzika, R. M., and Dey, D. C. (2002). Dynamics of an anthropogenic fire regime. Ecosystems 5, 472–486. doi: 10.1007/s10021-002-0115-7
Hamann, A., and Wang, T. (2006). Potential effects of climate change on ecosystem and tree species distribution in British Columbia. Ecology 87, 2773–2786. doi: 10.1890/0012-9658(2006)87[2773:PEOCCO]2.0.CO;2
Hannah, L., Lovejoy, T. E. and Schneider, S. H. (2005). “Biodiversity and climate change in context,” in Climate Change and Biodiversity, eds T. E. Lovejoy and L. Hannah (New Haven, CT: Yale University Press,), 3–14.
Hansen, A. J., Piekielek, N., Davis, C., Haas, J., Theobald, D. M., Gross, J. E., et al. (2014). Exposure of U.S. National Parks to land use and climate change 1900–2100. Ecol. Appl. 24, 484–502. doi: 10.1890/13-0905.1
He, H. S. (2008). Forest landscape models, definition, characterization, and classification. Forest Ecol. Manag. 254, 484–498. doi: 10.1016/j.foreco.2007.08.022
Hessburg, P. F., Smith, B. G., and Salter, R. B. (1999). Detecting change in forest spatial patterns from reference conditions. Ecol. Appl. 9, 1232–1252. doi: 10.1890/1051-0761(1999)009[1232:DCIFSP]2.0.CO;2
Hessl, A. E., Milesi, C., White, M. A., Peterson, D. L., and Keane, R. E. (2004). Ecophysiological Parameters for Pacific Northwest trees. Gen. Tech. Rep. PNW-GTR-618. Portland, OR: US Department of Agriculture, Forest Service, Pacific Northwest Research Station. doi: 10.2737/PNW-GTR-618
Holling, C. S. (1973). Resilience and stability of ecological systems. Annu. Rev. Ecol. System. 4, 1–23. doi: 10.1146/annurev.es.04.110173.000245
Holsinger, L., Keane, R. E., Isaak, D. J., Eby, L., and Young, M. K. (2014). Relative effects of climate change and wildfires on stream temperatures: a simulation modeling approach in a Rocky Mountain watershed. Clim. Change 124, 191–206. doi: 10.1007/s10584-014-1092-5
Hulme, P. E. (2005). Adapting to climate change: is there scope for ecological management in the face of a global threat? J. Appl. Ecol. 42, 784–794. doi: 10.1111/j.1365-2664.2005.01082.x
Humphries, H. C., and Bourgeron, P. S. (2001). “Methods for determining historical range of variability,” in A Guidebook for Integrated Ecological Assessments, eds M. E. Jensen and P. S. Bourgeron (New York, NY: Springer-Verlag), 273–291. doi: 10.1007/978-1-4419-8620-7_20
Jackson, L. J., Trebitz, A. S., and Cottingham, K. L. (2000). An introduction to the practice of ecological modeling. BioScience 50, 694–706. doi: 10.1641/0006-3568(2000)050[0694:AITTPO]2.0.CO;2
Johnstone, J. F., Hollingsworth, T. N., Chapin, F. S., I. I. I., and Mack, M. C. (2010). Changes in fire regime break the legacy lock on successional trajectories in Alaskan boreal forest. Global Change Biol. 16, 1281–1295. doi: 10.1111/j.1365-2486.2009.02051.x
Jørgensen, S. E., and Bendoricchio, G. (2001). Fundamentals of Ecological Modelling, Vol. 21. Oxford, UK: Elsevier.
Keane, R. E. (2012). “Creating historical range of variation (HRV) time series using landscape modeling: overview and issues,” in Historical Environmental Variation in Conservation and Natural Resource Management, 1st Edn. eds J. A. Wiens, G. D. Hayward, H. D. Safford, and C. M. Giffen (John Wiley and Sons, Ltd.) doi: 10.1002/9781118329726.ch8
Keane, R. E., Cary, G., Davies, I. D., Flannigan, M. D., Gardner, R. H., Lavorel, S., et al. (2004). A classification of landscape fire succession models: spatially explicit models of fire and vegetation dynamic. Ecol. Model. 256, 3–27. doi: 10.1016/j.ecolmodel.2004.03.015
Keane, R. E., Gray, K., Davis, B., Holsinger, L. M., and Loehman, R. (2019). Evaluating ecological resilience across wildfire suppression levels under climate and fuel treatment scenarios using landscape simulation modelling. Int. J. Wildland Fire 28, 533–549. doi: 10.1071/WF19015
Keane, R. E., Loehman, R. A., and Holsinger, L. M. (2011). The FireBGCv2 Landscape Fire and Succession Model: A Research Simulation Platform for Exploring Fire and Vegetation Dynamics. General Technical Report RMRS-GTR-255, U.S. Department of Agriculture, Forest Service, Rocky Mountain Research Station, Fort Collins, CO. doi: 10.2737/RMRS-GTR-255
Keane, R. E., and Loehman, R. A. (2012). Estimating Critical Climate-Driven Thresholds in Landscape Dynamics Using Spatial Simulation Modeling: Climate Change Tipping Points in Fire Management. Final Report, Joint Fire Sciences Program, JFSP project: 09-3-01-17, Boise, ID.
Keane, R. E., Loehman, R. A., Holsinger, L. M., Falk, D. A., Higuera, P., Hood, S. M., et al. (2018). Use of landscape simulation modeling to quantify resilience for ecological applications. Ecosphere 9:e02414. doi: 10.1002/ecs2.2414
Keane, R. E., McKenzie, D., Falk, D. A., Smithwick, E. A. H., Miller, C., and Kellogg, L.-K. B. (2015). Representing climate, disturbance, and vegetation interactions in landscape models. Ecol. Model. 309–310, 33–47. doi: 10.1016/j.ecolmodel.2015.04.009
Keane, R. E., Rollins, M., and Zhu, Z. L. (2007). Using simulated historical time series to prioritize fuel treatments on landscapes across the United States: the LANDFIRE prototype project. Ecol. Model. 204, 485–502. doi: 10.1016/j.ecolmodel.2007.02.005
Keetch, J. J., and Byram, G. M. (1968). A Drought Index for Forest Fire Control. Research Paper SE-38. Asheville, NC: US Department of Agriculture, Forest Service, Southeastern Forest Experiment Station, 35–38.
Kelly, A. E., and Goulden, M. L. (2008). Rapid shifts in plant distribution with recent climate change. Proc. Natl. Acad. Sci. U.S.A. 105, 11823–11826. doi: 10.1073/pnas.0802891105
Kelly, R. P., Erickson, A. L., Mease, L. A., Battista, W., Kittinger, J. N., and Fujita, R. (2015). Embracing thresholds for better environmental management. Philos. Trans. R. Soc. B Biol. Sci. 370:20130276. doi: 10.1098/rstb.2013.0276
Krawchuk, M. A., Moritz, M. A., Parisien, M. A., Van Dorn, J., and Hayhoe, K. (2009). Global pyrogeography: the current and future distribution of wildfire. PLoS ONE 4:e5102. doi: 10.1371/journal.pone.0005102
Kreyling, J., Jentsch, A., and Beier, C. (2014). Beyond realism in climate change experiments: gradient approaches identify thresholds and tipping points. Ecol. Lett. 17, 125–e1. doi: 10.1111/ele.12193
Lawler, J. J. (2009). Climate change adaptation strategies for resource management and conservation planning. Ann. N. Y. Acad. Sci. 1162, 79–98. doi: 10.1111/j.1749-6632.2009.04147.x
Liebmann, M. J., Farella, J., Roos, C. I., Stack, A., Martini, S., and Swetnam, T. W. (2016). Native American depopulation, reforestation, and fire regimes in the Southwest United States, 1492–1900 CE. Proc. Natl. Acad. Sci. U.S.A. 113, E696–E704. doi: 10.1073/pnas.1521744113
Littell, J. S., McKenzie, D., Peterson, D. L., and Westerling, A. L. (2009). Climate and wildfire area burned in western US ecoprovinces, 1916–2003. Ecol. Appl. 19, 1003–1021. doi: 10.1890/07-1183.1
Loehman, R., Flatley, W., Holsinger, L., and Thode, A. (2018). Can land management buffer impacts of climate changes and altered fire regimes on ecosystems of the southwestern United States? Forests 9:192. doi: 10.3390/f9040192
Loehman, R. A. (2016). Modeling ancient land use and resilient forests in the Jemez Mountains. Archaeol. Southwest Magaz. 30:23.
Lucash, M. S., Scheller, R. M., Sturtevant, B. R., Gustafson, E. J., Kretchun, A. M., and Foster, J. R. (2018). More than the sum of its parts: how disturbance interactions shape forest dynamics under climate change. Ecosphere 9:e02293. doi: 10.1002/ecs2.2293
Lugato, E., and Berti, A. (2008). Potential carbon sequestration in a cultivated soil under different climate change scenarios: a modelling approach for evaluating promising management practices in north-east Italy. Agric. Ecosyst. Environ. 128, 97–103. doi: 10.1016/j.agee.2008.05.005
Maclean, I. M., and Wilson, R. J. (2011). Recent ecological responses to climate change support predictions of high extinction risk. Proc. Natl. Acad. Sci. U.S.A. 108, 12337–12342. doi: 10.1073/pnas.1017352108
Margolis, E. Q., and Balmat, J. (2009). Fire history and fire–climate relationships along a fire regime gradient in the Santa Fe Municipal Watershed, NM, USA. Forest Ecol. Manage. 258, 2416–2430. doi: 10.1016/j.foreco.2009.08.019
Marlon, J. R., Bartlein, P. J., Walsh, M. K., Harrison, S. P., Brown, K. J., Edwards, M. E., et al. (2009). Wildfire responses to abrupt climate change in North America. Proc. Natl. Acad. Sci. U.S.A 106, 2519–24. doi: 10.1073/pnas.0808212106
McKenzie, D., and Littell, J. S. (2017). Climate change and the eco-hydrology of fire: will area burned increase in a warming western USA? Ecol. Appl. 27, 26–36. doi: 10.1002/eap.1420
McKenzie, D., Shankar, U., Keane, R. E., Stavros, E. N., Heilman, W. E., Fox, D. G., et al. Riebau., (2014). Smoke consequences of new wildfire regimes driven by climate change. Earth Fut. 2, 35–59. doi: 10.1002/2013EF000180
Millar, C. I., Stephenson, N. L., and Stephens, S. L. (2007). Climate change and forests of the future: Managing in the face of uncertainty. Ecol. Appl. 17, 2145–2151. doi: 10.1890/06-1715.1
Moss, R. H., Edmonds, J. A., Hibbard, K. A., Manning, M. R., Rose, S. K., and van Vuuren, D. P. (2010). The next generation of scenarios for climate change research and assessment. Nature 463:747–756. doi: 10.1038/nature08823
Overpeck, J., Whitlock, C., and Huntley, B. (2003). “Terrestrial biosphere dynamics in the climate system: past and future,” in Paleoclimate, Global Change and the Future, eds K. D. Alverson, R. S. Bradley and T. Pedersen, (Berlin; Heidelberg; New York: Springer-Verlag) 81–103. doi: 10.1007/978-3-642-55828-3_5
Parks, S. A., Holsinger, L. M., Miller, C., and Nelson, C. R. (2015). Wildland fire as a self-regulating mechanism: the role of previous burns and weather in limiting fire progression. Ecol. Appl. 25, 1478–1492. doi: 10.1890/14-1430.1
Perera, A. H., Sturtevant, B. R., and Buse, L. J. (eds.). (2015a). “Simulation modeling of forest landscape disturbances: an overview,” in Simulation Modeling of Forest Landscape Disturbances (Cham: Springer), 1–15. doi: 10.1007/978-3-319-19809-5_1
Perera, A. H., Sturtevant, B. R., and Buse, L. J. (eds.). (2015b). “Simulation modeling of forest landscape disturbances: where do we go from here?” in Simulation Modeling of Forest Landscape Disturbances (Cham: Springer), 287–311. doi: 10.1007/978-3-319-19809-5_11
Peterson, G. D., Cumming, G. S., and Carpenter, S. R. (2003). Scenario planning: a tool for conservation in an uncertain world. Conserv. Biol. 17, 358–366. doi: 10.1046/j.1523-1739.2003.01491.x
Pollet, J., and Omi, P. N. (2002). Effect of thinning and prescribed burning on crown fire severity in ponderosa pine forests. Int. J. Wildland Fire 11, 1–10. doi: 10.1071/WF01045
Qian, S. S., King, R. S., and Richardson, C. J. (2003). Two statistical methods for the detection of environmental thresholds. Ecol. Model. 166, 87–97. doi: 10.1016/S0304-3800(03)00097-8
Reinhardt, E. D., and Dickinson, M. B. (2010). First-order fire effects models for land management: overview and issues. Fire Ecol. 6:131. doi: 10.4996/fireecology.0601131
Reyer, C. P., Brouwers, N., Rammig, A., Brook, B. W., Epila, J., Grant, R. F., et al. (2015). Forest resilience and tipping points at different spatio-temporal scales: approaches and challenges. J. Ecol. 103, 5–15. doi: 10.1111/1365-2745.12337
Reynolds, R. T., Meador, A. J. S., Youtz, J. A., Nicolet, T., Matonis, M. S., Jackson, P. L., et al. (2013). Restoring Composition and Structure in Southwestern Frequent-Fire Forests General Technical Report RMRS-GTR-310. Fort Collins, CO: USDA Forest Service, Rocky Mountain Research Station.
Rittel, H. W. J., and Webber, M. M. (1973). Dilemmas in a general theory of planning. Policy Sci. 4, 155–169. doi: 10.1007/BF01405730
Roos, C. I., Williamson, G. J., and Bowman, D. M. (2019). Is anthropogenic pyrodiversity invisible in paleofire records? Fire. 2, 42.
Roos, C. I., Zedeño, M. N., Hollenback, K. L., and Erlick, M. M. (2018). Indigenous impacts on North American Great Plains fire regimes of the past millennium. Proc. Natl. Acad. Sci. U.S.A. 115, 8143–8148. doi: 10.1073/pnas.1805259115
Root, T. L., Price, J. T., Hall, K. R., Schneider, S. H., Rosenzweig, C., and Pounds, J. A. (2003). Fingerprints of global warming on wild animals and plants. Nature 421, 57–60. doi: 10.1038/nature01333
Scheller, R. M. (2018). The challenges of forest modeling given climate change. Landscape Ecol. 33, 1481–1488. doi: 10.1007/s10980-018-0689-x
Schoennagel, T., Balch, J. K., Brenkert-Smith, H., Dennison, P. E., Harvey, B. J., Krawchuk, M. A., et al. (2017). Adapt to more wildfire in western North American forests as climate changes. Proc. Natl. Acad. Sci. U.S.A. 114, 4582–4590. doi: 10.1073/pnas.1617464114
Seidl, R. (2014). The shape of ecosystem management to come: anticipating risks and fostering resilience. Bioscience. 64(12), 1159–1169.
Smithwick, E. A., Westerling, A. L., Turner, M. G., Romme, W. H., and Ryan, M. G. (2011). Climate, Fire and Carbon: Tipping Points and Landscape Vulnerability in the Greater Yellowstone Ecosystem. Boise, ID: Final Report, Joint Fire Sciences Program, JFSP project: 01–47.
Stephens, S. L., Agee, J. K., Fule, P. Z., North, M. P., Romme, W. H., Swetnam, T. W., et al. (2013). Managing forests and fire in changing climates. Science 342, 41–42. doi: 10.1126/science.1240294
Stephens, S. L., McIver, J. D., Boerner, R. E., Fettig, C. J., Fontaine, J. B., Hartsough, B. R., et al. (2012). The effects of forest fuel-reduction treatments in the United States. BioScience 62, 549–560. doi: 10.1525/bio.2012.62.6.6
Stevens-Rumann, C. S., Kemp, K. B., Higuera, P. E., Harvey, B. J., Rother, M. T., Donato, D. C., et al. (2018). Evidence for declining forest resilience to wildfires under climate change. Ecol. Lett. 21, 243–252. doi: 10.1111/ele.12889
Svenning, J. C., and Sandel, B. (2013). Disequilibrium vegetation dynamics under future climate change. Am. J. Botany 100, 1266–1286. doi: 10.3732/ajb.1200469
Swetnam, T. W., Allen, C. D., and Betancourt, J. L. (1999). Applied historical ecology: using the past to manage for the future. Ecol. Appl. 9, 1189–1206 doi: 10.1890/1051-0761(1999)009[1189:AHEUTP]2.0.CO;2
Temperli, C., Bugmann, H., and Elkin, C. (2013). Cross-scale interactions among bark beetles, climate change, and wind disturbances: a landscape modeling approach. Ecol. Monograph. 83, 383–402. doi: 10.1890/12-1503.1
Thom, D., Rammer, W., and Seidl, R. (2017). Disturbances catalyze the adaptation of forest ecosystems to changing climate conditions. Global Change Biol. 23, 269–282. doi: 10.1111/gcb.13506
Thomas, C. D., Cameron, A., Green, R. E., Bakkenes, M., Beaumont, L. J., Collingham, Y. C., et al. (2004). Extinction risk from climate change. Nature 427, 145–148. doi: 10.1038/nature02121
Thuiller, W., Albert, C., Araujo, M. B., Berry, P. M., Cabeza, M., Guisan, A., et al. (2008). Predicting global change impacts on plant species' distributions: future challenges. Persp. Plant Ecol. Evol. Syst. 9, 137–152. doi: 10.1016/j.ppees.2007.09.004
Tipton, C. Y., Ocheltree, T. W., Mueller, K. E., Turk, P., and Fernández-Giménez, M. E. (2018). Revision of a state-and-transition model to include descriptions of state functional attributes. Ecosphere 9:e02201. doi: 10.1002/ecs2.2201
Turner, M. G. (2010). Disturbance and landscape dynamics in a changing world 1. Ecology 91, 2833–2849. doi: 10.1890/10-0097.1
Turner, M. G., Arthaud, G. J., Engstrom, R. T., Hejl, S. J., Liu, J., Loeb, S., et al. (1995). Usefulness of spatially explicit population models in land management. Ecol. Appl. 5, 12–16. doi: 10.2307/1942046
van Mantgem, P. J., Nesmith, J. C., Keifer, M., Knapp, E. E., Flint, A., and Flint, L. (2013). Climatic stress increases forest fire severity across the western United States. Ecol. Lett. 16, 1151–1156 doi: 10.1111/ele.12151
Veblen, T. T., Kitzberger, T., Raffaele, E., Mermoz, M., González, M. E., Sibold, J. S., et al. (2009). The historical range of variability of fires in the Andean–Patagonian Nothofagus forest region. Int. J. Wildland Fire 17, 724–741. doi: 10.1071/WF07152
Vitousek, P. M., Mooney, H. A., Lubchenco, J., and Melillo, J. M. (1997). Human domination of Earth's ecosystems. Science. 277(5325), 494–499.
Westerling, A. L., Hidalgo, H. G., Cayan, D. R., and Swetnam, T. W. (2006). Warming and earlier spring increase western US forest wildfire activity. Science 313, 940–943. doi: 10.1126/science.1128834
Westerling, A. L., Turner, M. G., Smithwick, E. A., Romme, W. H., and Ryan, M. G. (2011). Continued warming could transform Greater Yellowstone fire regimes by mid-21st century. Proc. Natl. Acad. Sci. U.S.A. 108, 13165–13170. doi: 10.1073/pnas.1110199108
Whitlock, C., Shafer, S. L., and Marlon, J. (2003). The role of climate and vegetation change in shaping past and future fire regimes in the northwestern US and the implications for ecosystem management. Forest Ecol. Manage. 178, 5–21. doi: 10.1016/S0378-1127(03)00051-3
Wimberly, M. C. (2002). Spatial simulation of historical landscape patterns in coastal forests of the Pacific Northwest. Can. J. Forest Res. 32, 1316–1328. doi: 10.1139/x02-054
Wimberly, M. C., Cochrane, M. A., Baer, A. D., and Pabst, K. (2009). Assessing fuel treatment effectiveness using satellite imagery and spatial statistics. Ecol. Appl. 19, 1377–1384. doi: 10.1890/08-1685.1
Keywords: HRV, FRV, landscape modeling, ecosystem management, climate change, land management, landscape ecology, historical ecology
Citation: Loehman RA, Keane RE and Holsinger LM (2020) Simulation Modeling of Complex Climate, Wildfire, and Vegetation Dynamics to Address Wicked Problems in Land Management. Front. For. Glob. Change 3:3. doi: 10.3389/ffgc.2020.00003
Received: 28 October 2019; Accepted: 09 January 2020;
Published: 29 January 2020.
Edited by:
Dominique Bachelet, Oregon State University, United StatesReviewed by:
Brendan Morris Rogers, Woods Hole Research Center, United StatesOlga Viedma Sillero, University of Castilla La Mancha, Spain
Copyright © 2020 Loehman, Keane and Holsinger. This is an open-access article distributed under the terms of the Creative Commons Attribution License (CC BY). The use, distribution or reproduction in other forums is permitted, provided the original author(s) and the copyright owner(s) are credited and that the original publication in this journal is cited, in accordance with accepted academic practice. No use, distribution or reproduction is permitted which does not comply with these terms.
*Correspondence: Rachel A. Loehman, cmxvZWhtYW5AdXNncy5nb3Y=