- 1Research Institute on Terrestrial Ecosystems, National Research Council, Rome, Italy
- 2National Biodiversity Future Center (NBFC) -National Biodiversity Future Center, Palermo, Italy
- 3Department of Biotechnology and Life Sciences, University of Insubria, Busto Arsizio, Italy
- 4Department of Ecological and Biological Sciences, University of Tuscia, Viterbo, Italy
- 5Servizio Veterinario - Parco Nazionale d’Abruzzo Lazio e Molise, Pescasseroli, Italy
- 6GPG Wildlife Project, Mezzolombardo, Trento, Italy
- 7Norwegian Institute for Nature Research, Trondheim, Norway
- 8Department of Wildlife, Fish and Environmental Studies, Swedish University of Agricultural Sciences, Umeå, Sweden
- 9Department of Biology and Biotechnologies “Charles Darwin”, University of Rome La Sapienza, Rome, Italy
Epigenetic mechanisms such as methylation can influence gene expression and play a crucial role in the adaptation to local environmental conditions, thereby introducing non-genetic variability within species. Here, using a Reduced Representation Bisulfite Sequencing approach (RRBS), we compared the methylation patterns in blood and muscle across three European brown bear populations. Our results clearly demonstrated that, beyond tissue-driven divergences, the methylation patterns of the three populations are significantly distinct. Differentially methylated sites, possibly associated with genomic features involved in development and anatomical differentiation, are widespread across the bear genome. This finding supports previous studies suggesting a role for the alteration of developmental pathways in shaping phenotypic novelties with potential adaptative significance. Our results underscore the importance and the effectiveness of including epigenetic approaches in studying wild non-model organisms. Investigating the epigenome can be especially relevant for endangered populations that have experienced a significant erosion of genomic diversity.
Introduction
The evolutionary trajectory of a species is primarily driven by its genetic diversity. Nevertheless, an increasing body of evidence emphasizes the significant contribution of epigenetic diversity to species evolutionary potential (Baltazar-Soares et al., 2024). Despite the significant implications of epigenetic pattern variations, this aspect at the population level in non-model species remains largely unexplored (Vogt, 2022).
Natural epigenetic variation is increasingly documented in wild populations (Hu and Barrett, 2017; Meröndun et al., 2019). This source of variation is, to some extent, genetically determined and can arise from stochastic epigenetic modifications, independent from the surrounding environment (Feinberg and Irizarry, 2010). Intriguingly, certain emerging epigenetic modifications can assume adaptive significance, thereby contributing to the population’s resilience in dynamic environments, at least in the short term and potentially across longer timescales if transmitted across generations (Feinberg and Irizarry, 2010). The stochastic origin of adaptive epigenetic variation holds particular significance in genetically depauperate populations, including those characterized by small size, isolation, and/or high levels of inbreeding (Verhoeven and Preite, 2014; Leung et al., 2016). Accumulating evidence suggests that comprehensively assessing both genetic and epigenetic structuring across geographical and environmental dimensions can confer unparalleled analytical power in evaluating population-level variations and responses to local adaptation (Meröndun et al., 2019). This approach could establish a functional connection between the genome and the environment (Cavalli and Heard, 2019; Rey et al., 2020), thereby enhancing our understanding of the intricate interplay between genetic and epigenetic factors in the context of evolutionary processes.
Currently, a crucial step involves examining the extent of epigenetic variation in wild populations experiencing different levels of environmental complexity, phenotypic divergence, and genetic structure. In this respect, examining the epigenome provides an additional layer of information for understanding adaptive evolution, allowing insights into regulatory mechanisms such as DNA methylation. These mechanisms influence tissue-specific genetic expression (Lokk et al., 2014; Zhou et al., 2017), as well as the development and maintenance of complex phenotypes (Peaston and Whitelaw, 2006; Venney et al., 2023).
Here we explored whether differences in DNA methylation patterns are potentially associated with population differentiation in non-model wild mammals, such as the brown bear Ursus arctos. This species is widely distributed in the palearctic region but occurs in Europe in scattered populations, some of which are characterized by a very low number of individuals. European populations show marked genotypic similarity (Tumendemberel et al., 2023) but some show differentiated phenotypic characteristics, such as body mass and skull size and shape divergence (Loy et al., 2008; Swenson et al., 2023). Particularly the Apennine brown bear shows enlarged supraorbital apophyses, wider zygomatic arches, and distinct first upper molar shapes. These changes likely result from rapid evolution driven by genetic drift and local selective pressures, potentially linked to dietary shifts favoring vegetation and hard mast, such as beech nuts and acorns (Colangelo et al., 2012). The origin of these phenotypic novelties, that could be interpreted as local adaptations, can be hardly explained exclusively in term of neutral genetic variation, and other mechanisms, such as the alteration of developmental pathways, has also been claimed (Loy et al., 2021).
By using previously collected tissue samples from three European brown bear populations, we examined genome-wide DNA methylation between and within populations using Reduced Representation Bisulfite Sequencing (RRBS). Because the methylome is tissue specific (Lokk et al., 2014), we explored methylation profiles both in blood and muscle samples with the aim to understand if differential methylation signatures could be recognized at the population level in both tissues. Previous studies on muscle tissue have demonstrated how epigenetic structures can uncover subtle levels of differentiation influenced by environmental factors (Meröndun et al., 2019). Similarly, research on blood methylation has highlighted its potential to reflect adaptations to resource availability (Lea et al., 2016) and to capture both the genetic and geographic structure of populations (Vilgalys et al., 2019). Moreover, methylation is known to play a role in the adaptive immune response (Mittelstaedt et al., 2021; Caizergues et al., 2022). Thus, examining methylation patterns in blood could also provide valuable insights into the immune adaptations of wild populations.
We focused our study on three European populations that share a common genomic ancestry (Tumendemberel et al., 2023): the Scandinavian (Norway), the Central Apennine (Italy), and Eastern Alpine (Italy but derived from the Dinaric-Balkan) bear populations. The relict Apennine brown bear population is one of the most endangered in Europe and, even though genetically depauperated (Benazzo et al., 2017), it is still able to express a significant phenotypic variation of possible adaptive significance linked to the exploitation of different trophic resources (Colangelo et al., 2012). Because the analysis of DNA methylation may disclose additional levels of population structuring and elucidate the biological pathways contributing to phenotypic divergence within genetically homogeneous species (Meröndun et al., 2019), that in turn could reflect differences at physiological and metabolic level, the three bear populations included in this study are good candidate to gain initial insights into the role of epigenomic differentiation as a potential source of phenotypic divergence and plasticity.
Material and methods
We analyzed the epigenomic variation among 12 brown bear specimens from three different European populations, two from Italy (Apennines and Alpine), and one from Norway (Scandinavian peninsula). Two different tissues were sampled: blood and skeletal muscle. Blood was collected for Apennine and Alpine populations during previous live-trapping activities carried out by the Parco Nazionale d’Abruzzo Lazio e Molise (Pescasseroli, Italy) and Provincia Autonoma di Trento (Trento, Italy), respectively. Muscle samples were collected for Apennine populations from road killed individuals. Muscle samples from Norway (Scandinavian population) were from legally shot bears. The samples obtained were mainly subadults and includes both males and females (Supplementary Table S1).
DNA extraction and library preparation
The DNA from blood was extracted using a Blood DNA Isolation Mini Kit (Norgen Biotek Corp), whereas DNA from muscle was extracted using a Quick-DNA miniprep Kit (Zymo Research) following companies’ guidelines. Library preparation and sequencing were performed by Genomix4life S.R.L. (Baronissi, Salerno, Italy). Input DNA concentration of each sample was assayed with a Qubit fluorimeter (Life Technologies), and its quality assessed with a TapeStation 4200 (Agilent). Indexed libraries were constructed from 100 ng of purified DNA with an Ovation RRBS Methyl-Seq System according to the manufacturer’s instructions. The current approach utilizes the methylation insensitive restriction enzyme MspI, which recognizes CCGG sites. Individual libraries, whose quality and quantity were assessed with a TapeStation 4200 and Qubit fluorometer, were then pooled equimolarly. The pooled samples were paired-end (2x101bp) sequenced on a Novaseq6000 (Illumina). The 12 methylome raw files were deposited into the NCBI SRA database under the Bioproject PRJNA1104803.
Bioinformatic analyses
Raw reads were demultiplexed and quality was checked with the software Fastqc v0.11.9 (Andrews, 2010). Successively, a first adapter and quality trimming step were performed using TimGalore v0.6.10 (https://github.com/FelixKrueger/TrimGalore) followed by diversity trimming and filtering with NuGEN’s diversity trimming python script (https://github.com/nugentechnologies/NuMetRRBS).
Filtered fastq files were processed using the software Bismark v0.24.1 (Krueger and Andrews, 2011). Initially the fastq files were aligned to the brown bear Ursus arctos genome assembly (UrsArc2.0; NCBI RefSeq code: GCF_023065955.2) using Bowtie2 with parameter –score_min L,0,-0.6. Then the methylation call was performed using default Bismark parameters.
The obtained cytosine coverage files were used to investigate methylation profiles and perform a differential methylation analysis at regional level using the R package methylKit v1.28 (Akalin et al., 2012). We discarded sites with less than 10X and 99.9% of coverage. Then we performed a normalization of the coverage values among samples using a scaling factor derived from differences between median of coverage distributions as implemented in methylKit. Finally, we excluded those CpG sites that have no or little variation among study subjects and are not particularly informative for downstream analyses retaining only those sites with a standard deviation larger than two.
Due to X chromosome dosage compensation between females and males (Cecalev et al., 2024), we filtered out sex chromosomes from the analysis to reduce a potential bias on the inference at a population level. However, because X chromosome may still bring information on differentiation between populations, the methylation pattern in X chromosome was also investigated separately. Initially, sex-filtered (autosomes only) and X chromosome datasets were used to explore the pattern of differentiation between populations and tissues estimating the Pearson correlation coefficient among individuals and performing a Principal Component Analysis (PCA).
Successively we identified Differentially Methylated Regions (DMRs) in autosomes between populations for the muscle (Apennine vs Scandinavia) and for the blood (Apennine vs Alpine).
For DMR analysis the filtering was performed as for site level methylation but retaining sites with at least 3X coverage. Then the DMRs were identified with a non-overlapping sliding window of 1000 bp and retaining DMRs with at least 10 methylated bases.
Differential methylation between Apennine and Alpine bears (for blood) and between Apennine and Scandinavian bears (for muscle) was tested using Chi-square test with overdispersion correction (McCullagh & Nelder method) and P-value adjusted using a Sliding Linear Model (SLIM). DMRs were retained when we found at least 25% methylation difference and a q-value <0.01.
Finally, to get an insight in a genome-wide functional significance of differential methylation we annotated DMRs using the distance from the nearest transcription start site (TSS) calculated using the R package genomation v1.34 (Akalin et al., 2015) and the brown bear genome (NCBI annotation code GCF_023065955.2-RS_2003_06). For each contrast we estimated the percentage of DMRs in promoters, introns, intergenic regions. Then, we used the identified genomic features (nearest genes) to perform an enrichment analysis looking for most significant Gene Ontology (GO) terms using the R package gprofiler2 v0.2.3 (Kolberg et al., 2020) and the GO annotation from polar bear (Ursus maritimus). The g:SCS (Set Counts and Sizes) method was used for multiple testing correction (Reimand et al., 2007).
Results
The mapping efficiency for each sample to the reference was higher than 90%, with approximately 60% of uniquely mapping reads (see Supplementary Figure S1). In all the samples, approximately 50% of the methylated cytosines was found in the CpG context (blood ~48%, muscle ~55%). Methylation in other contexts (i.e. CHH and CHG) was extremely low, accounting for less than 1% each, and higher in muscle than in blood, in line with CpG context (Supplementary Figure S2).
As expected, the highest correlations were observed among specimens derived from the same tissue type, with blood samples exhibiting particularly robust Pearson correlation coefficients (r). While muscle samples also displayed high correlation coefficients, these values were comparatively lower than those observed for blood (Figure 1). Within the blood sample group, specimens originating from the same population exhibited the highest correlation. A comparable, though less prominent, pattern was observed among muscle samples (Figure 1). The analysis of X chromosome methylation pattern produces a different picture, where correlations within each tissue are less pronounced whereas the population factor still produces evident correlation among individuals (Figure 1).
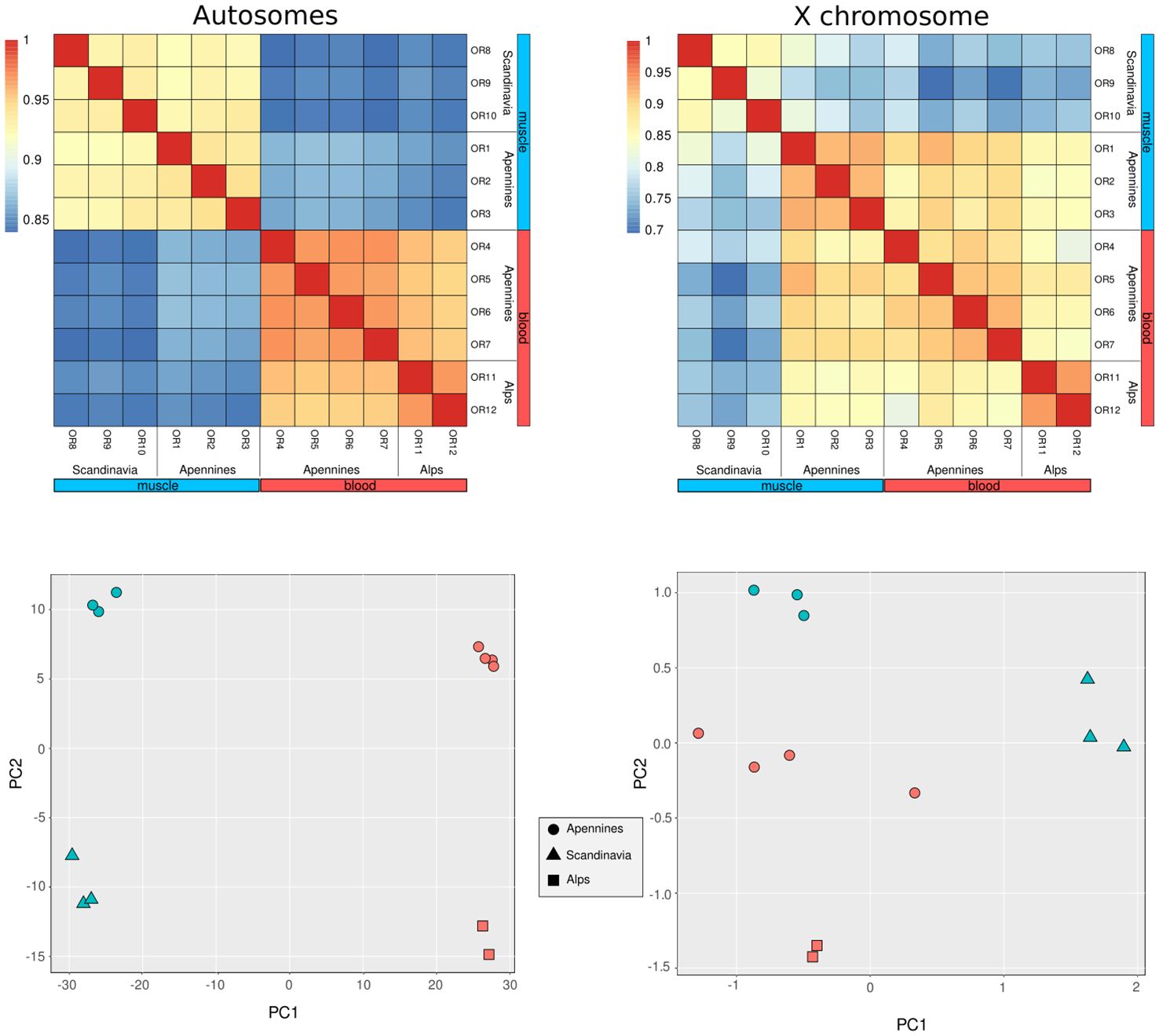
Figure 1. Heatmap of Pearson correlation coefficients (r) for autosomes and the X chromosome, calculated across all samples. Cluster dendrograms illustrate similarities based on correlation values. For autosomes, we observe low correlation between different tissue types but high correlation within samples of the same tissue type. Distinct “correlation clusters,” reflecting population membership, are particularly prominent in blood samples (highlighted in red). In contrast, the X chromosome displays high correlation not only within the same tissue type but also across different tissues from individuals within the same population. Principal Component Analysis (PCA) of autosomal methylation frequencies further reveals a clear structure in the epigenetic profiles of the 12 samples, grouping them by tissue type and population into four distinct clusters consistent with the correlation analysis. Similarly, PCA of X chromosome methylation frequencies continues to differentiate these four groups effectively.
The PCAs for both autosomes and X chromosome agree with the estimated correlation level showing a clear pattern of differentiation between tissues and populations for autosomes, less pronounced for X chromosome (Figure 1). Population differentiation is maintained in PCAs performed separately for each tissue (Supplementary Figure S3). Particularly, for both blood and muscle, PC1 is associated with population differences, whereas PC2 seems not directly linked with known variables.
Methylated cytosines were grouped in 639 different methylated regions (DMRs) in blood and 186 DMRs in muscle samples (Figure 2).
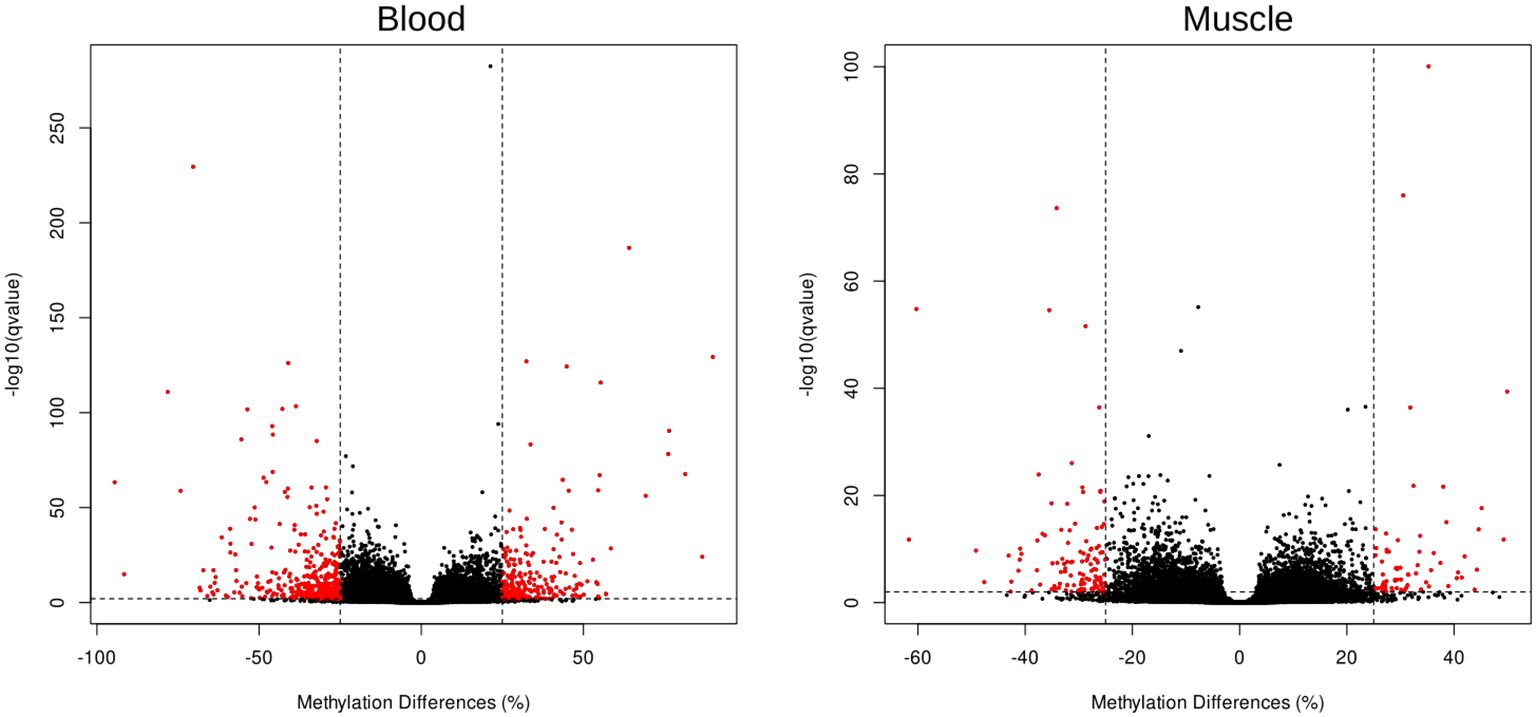
Figure 2. Volcano plots show differential methylation at regional level (DMR) in two pairwise comparisons between populations for blood and muscle (left and right respectively). Red points indicate DMRs with significant q-value < 0.05 and effect size larger than ±25%.
According to the distances from the nearest transcription start site (TSS), a larger fraction of DMRs is located within intergenic and intronic regions, distal from the TSSs, whereas promoters are a lower fraction, less than 10% (Figure 3).
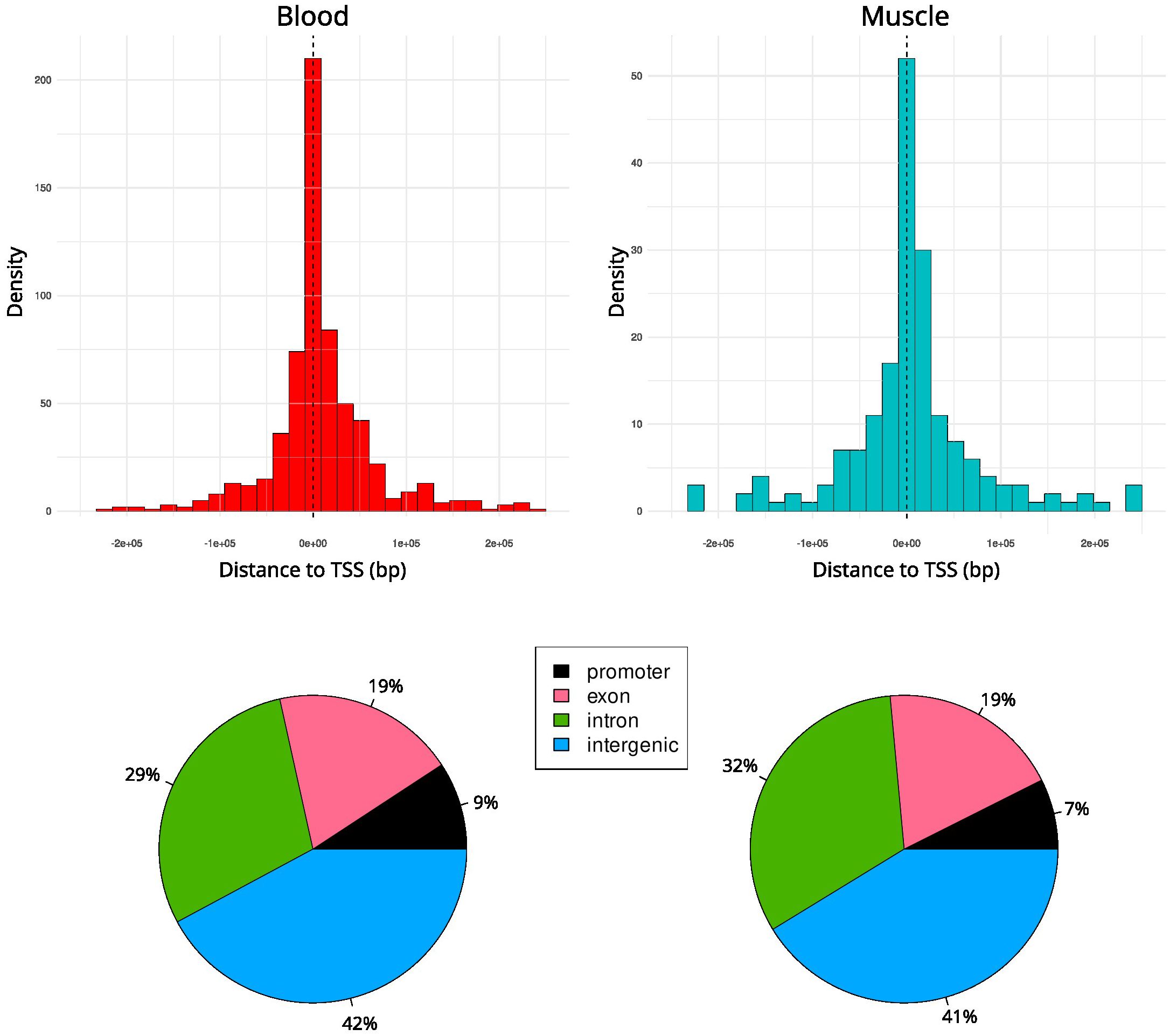
Figure 3. Histogram representing the distance of DMRs from the TSS suggests that in blood (left) and muscle (right) the DMRs are mostly found across intergenic and intronic regions.
Based on the nearest TSS, we linked DMRs to 416 and 115 annotated genes in blood and muscle respectively. According to the Gene Ontology (GO) enrichment analysis, the higher number of enriched GO terms was found in blood samples compared to muscle, with 20 and 3 GO terms respectively (Supplementary Table S2). In both the tissues most of the GO terms are related to morphogenesis and development at organismal and cellular levels.
Discussion
Our analyses highlighted a substantial divergence in methylation patterns across the three European brown bear populations we investigated. As expected, a first level of differentiation is driven by the tissue type (Figure 1), indicative of distinct cellular developmental trajectories during organismal maturation (Ehrlich and Lacey, 2013). Intriguingly, within each tissue, additional divergence was observed (Figure 1), suggesting that population-level factors have left signatures on the methylation profiles, irrespectively of the tissue. It is known that populations can exhibit environmentally associated epigenetic structures, even in the presence of a weak genetic differentiation (Meröndun et al., 2019; Bogan and Yi, 2024). In our case the robust correlation observed among specimens within the same tissue (Figure 1), while suggesting a relatively low individual variability, still conveys a clear resolution of population-level divergence. We observed significant methylation differentiation at a regional (DMR) level in the analyzed tissues. Notably, blood emerged as a promising tissue for further investigation of methylation patterns in brown bears due to its higher number of DMR. Despite the recognized tissue-specific nature of methylation patterns, many studies face the challenge of obtaining tissue-specific samples, often requiring the sacrifice of individuals. In contrast, blood, routinely collected during any live-trapping management interventions, offers a practical avenue for research involving live animals. Previous studies on wild mammals have highlighted the potential of blood methylation in understanding additional aspects of adaptation. For instance, Lea et al. (2016) demonstrated how blood methylation can be relevant in linking the genetic patterns with phenotypic traits associated with resource availability in baboons. Additionally, Vilgalys et al. (2019) showed that DNA methylation can reflect both the genetic and geographic structure of populations and can be a target of positive selection. The observed methylation patterns on the X chromosome intriguingly appear to correspond to the geographic structure of our dataset. While the X chromosome is typically excluded from methylation analyses due to potential biases introduced by X-inactivation in females (Inkster et al., 2023), our analysis underscores methylation profiles associated with population structure. This pattern may reflect shared ancestry of X chromosomes among individuals within populations; however, further investigation with a larger dataset is warranted to substantiate these findings.
The two tissue types we analyzed showed a high number of DMRs distinguishing populations. According to the GO enrichment analysis we identified only three GO terms in the muscle, relative to regulation of morphogenetic processes at cellular and organ levels (e.g. GO:0000902 cell morphogenesis, GO:0009653 anatomical structure morphogenesis and GO:0048731 system development). On the contrary, blood yielded a wider array of significant enriched GO terms. The terms showing the highest significance are related to development at organismal (e.g. GO:0032502 developmental process and GO:0048856 anatomical structure development) and cellular level (e.g. GO:0048869 cellular developmental process, GO:0030154 cell differentiation and GO:0048468 cell development) (Supplementary Table S2). These terms refer to the wide array of genes whose specific outcome is the progression of an integrated organism influencing the development of an anatomical structure over time from an initial condition to the mature stage. Mutation or alteration of expression of these genes can have a relevant role for the evolution of morphological novelties on which natural selection can subsequently act. The presence of significant GO terms related to anatomical development in blood may be explained by the capacity of blood methylation patterns to reflect those of other tissues. Ebrahimi et al. (2021) demonstrated that blood can reflect the bone methylome, identifying sites shared between the two tissues and associated with major pathways relevant to bone regulation. This suggests that peripheral blood may serve as a viable proxy tissue for large scale genome-wide methylation study in living animals.
As expected, an inspection of DMRs close to promoter regions (approximately +/- 2000 bp from TSS) highlight the possible regulative role on genes such Colony Stimulating Factor 3 (CSF3), Class II Major Histocompatibility Complex Transactivator (CIITA), DLA class I histocompatibility antigen, and Interferon Regulatory Factor 7 (IRF7), all involved in immune response. Moreover, we found evidence of a close association of DMRs to genes directly involved in developmental processes such as Bone Morphogenetic Protein 7 (BMP7) and Homeobox B3 (HOXB3) and genes involved in metabolic pathways such as Galanin Receptor 3 (GALR3), Arachidonate 15-Lipoxygenase (ALOX15) and LDL receptor related protein 5.
While disclosing the direct linkage between observed differential methylation and phenotypic differences remains still an unexplored challenge at this stage, differential methylation associated to development seems particularly relevant in terms of intraspecific phenotypic plasticity. This strongly supports Loy et al.’s (2021) hypothesis that skull morphological variations among brown bear populations may stem from alterations in skull integration patterns, indicative of changes in gene network expression during development. The progression of development is a critical process, and its disruption can lead to an unfit adult phenotype. Nonetheless, accumulating empirical evidence indicates that developmental plasticity can serve as a driver of evolutionary change (Moczek et al., 2011; Colangelo et al., 2019; González-Forero, 2023). Although brown bear populations in Europe exhibit genetic homogeneity (Benazzo et al., 2017; Tumendemberel et al., 2023), they display conspicuous phenotypic differences in traits such as size, pelage color, and skull morphology (Swenson et al., 2023), and it has been suggested that variation in skull morphology might be driven by adaptation to local environmental conditions (Colangelo et al., 2012; Loy et al., 2021). Interestingly, Benazzo et al. (2017), highlighted the occurrence of several putative deleterious mutations associated with human disorders such as craniofacial and ocular anomalies, small body size, and skeletal muscle-related diseases, that could partially explain the phenotypic divergence of the Apennine brown bear.
In conclusion, our results suggest that epigenomics could complement genomics in the study of intraspecific diversification, particularly concerning phenotypic evolution in wild species. The study on gene expression and epigenomic profile can provide informative insights into phenotypic plasticity (Bogan and Yi, 2024). Although such studies are more rarely conducted in non-controlled conditions, our study shows a robust clustering pattern observed among samples from the same tissue and population. In turn, this indicates that wild, non-model organisms could be viable subjects for epigenomic research. Moreover, exploring epigenomic profiles at the population level holds promise for generating novel insights and providing crucial data to inform conservation strategies (Rey et al., 2020), aimed at safeguarding the adaptive and evolutionary potential of the species.
Data availability statement
The datasets presented in this study can be found in online repositories. The names of the repository/repositories and accession number(s) can be found below: https://www.ncbi.nlm.nih.gov/, PRJNA1104803.
Ethics statement
The study was conducted in accordance with the local legislation and institutional requirements.
Author contributions
PCo: Conceptualization, Data curation, Formal analysis, Funding acquisition, Investigation, Methodology, Project administration, Resources, Software, Supervision, Validation, Visualization, Writing – original draft, Writing – review & editing. IB: Conceptualization, Funding acquisition, Writing – review & editing, Resources, Validation. LGr: Data curation, Formal Analysis, Visualization, Writing – review & editing, Investigation, Methodology. ES: Methodology, Writing – review & editing. ED: Formal Analysis, Writing – review & editing. PF: Conceptualization, Methodology, Writing – review & editing. LGe: Writing – review & editing, Resources. RG: Writing – review & editing, Resources. OK: Writing – review & editing, Resources. JK: Writing – review & editing, Resources. AK: Writing – review & editing, Resources. PCi: Conceptualization, Funding acquisition, Investigation, Supervision, Writing – review & editing, Resources, Validation.
Funding
The author(s) declare financial support was received for the research, authorship, and/or publication of this article. This project was founded by PRIN (Progetti di Rilevante Interesse Nazionale) 2022PYJWJA “Epigenetics and conservation of small populations: microevolution and adaptive divergence in relict bear populations” CUP: B53D23012260006. ES and PCo were also funded by the National Recovery and Resilience Plan (NRRP), Mission 4 Component 2 Investment 1.4 - Call for tender No. 3138 of 16 December 2021, rectified by Decree n.3175 of 18 December 2021 of Italian Ministry of University and Research funded by the European Union – NextGenerationEU; Project code CN_00000033, Concession Decree No. 1034 of 17 June 2022 adopted by the Italian Ministry of University and Research, CUP B83C22002930006 Project title “National Biodiversity Future Center - NBFC”.
Conflict of interest
The authors declare that the research was conducted in the absence of any commercial or financial relationships that could be construed as a potential conflict of interest.
The author(s) declared that they were an editorial board member of Frontiers, at the time of submission. This had no impact on the peer review process and the final decision.
Generative AI statement
The author(s) declare that no Generative AI was used in the creation of this manuscript.
Publisher’s note
All claims expressed in this article are solely those of the authors and do not necessarily represent those of their affiliated organizations, or those of the publisher, the editors and the reviewers. Any product that may be evaluated in this article, or claim that may be made by its manufacturer, is not guaranteed or endorsed by the publisher.
Supplementary material
The Supplementary Material for this article can be found online at: https://www.frontiersin.org/articles/10.3389/fevo.2024.1504225/full#supplementary-material.
References
Akalin A., Kormaksson M., Li S., Garrett-Bakelman F. E., Figueroa M. E., Melnick A., et al. (2012). methylKit: a comprehensive R package for the analysis of genome-wide DNA methylation profiles. Genome Biol. 13, R87. doi: 10.1186/gb-2012-13-10-r87
Akalin A., Franke V., Vlahoviček K., Mason C. E., Schübeler D. (2015). Genomation: a toolkit to summarize, annotate and visualize genomic intervals. Bioinf. 31, 1127–1129. doi: 10.1093/bioinformatics/btu775
Andrews S. (2010). FastQC: A quality control tool for high throughput sequence data. Available online at: http://www.bioinformatics.babraham.ac.uk/projects/fastqc/ (Accessed April 1 , 2023).
Baltazar-Soares M., Balard A., Heckwolf M. (2024). Epigenetic Diversity and the Evolutionary Potential of Wild Populations. Evol Appl 17, e70011. doi: 10.1111/eva.70011
Benazzo A., Trucchi E., Cahill J. A., Delser P. M., Mona S., Fumagalli S., et al. (2017). Survival and divergence in a small group: The extraordinary genomic history of the endangered Apennine brown bear stragglers. Proc. Natl. Acad. Sci. 114, E9589–E9597. doi: 10.1073/pnas.1707279114
Bogan S. N., Yi S. V. (2024). Potential role of DNA methylation as a driver of plastic responses to the environment across cells, organisms, and populations. Genome Biol. Evol. 16, evae022. doi: 10.1093/gbe/evae022
Caizergues A. E., Le Luyer J., Grégoire A., Szulkin M., Senar J. C., Charmantier A., et al. (2022). Epigenetics and the city: non-parallel DNA methylation modifications across pairs of urban-forest great tit populations. Evolutionary Appl. 15, 149–165. doi: 10.1111/eva.13334
Cavalli G., Heard E. (2019). Advances in epigenetics link genetics to the environment and disease. Nature 571, 489–499. doi: 10.1038/s41586-019-1411-0
Cecalev D., Viçoso B., Galupa R. (2024). Compensation of gene dosage on the mammalian X. Development 151, dev202891. doi: 10.1242/dev.202891
Colangelo P., Loy A., Huber D., Gomerčić T., Vigna Taglianti A., Ciucci P. (2012). Cranial distinctiveness in the Apennine brown bear: genetic drift effect or ecophenotypic adaptation? Biol. J. Linn. Soc. 107, 15–26. doi: 10.1111/j.1095-8312.2012.01926.x
Colangelo P., Ventura D., Piras P., Bonaiuti J. P. G., Ardizzone G. (2019). Are developmental shifts the main driver of phenotypic evolution in Diplodus spp. (Perciformes: Sparidae)?. BMC Evol Biol 19, 106. doi: 10.1186/s12862-019-1424-1
Ebrahimi P., Luthman H., McGuigan F. E., Akesson K. E. (2021). Epigenome-wide cross-tissue correlation of human bone and blood DNA methylation - can blood be used as a surrogate for bone? Epigenetics 6, 92–105. doi: 10.1080/15592294.2020.1788325
Ehrlich M., Lacey M. (2013). DNA methylation and differentiation: silencing, upregulation and modulation of gene expression. Epigenomics 5, 553–568. doi: 10.2217/epi.13.43
Feinberg A. P., Irizarry R. A. (2010). Evolution in health and medicine Sackler colloquium: Stochastic epigenetic variation as a driving force of development, evolutionary adaptation, and disease. Proc. Natl. Acad. Sci. United States America 107 Suppl 1, 1757–1764. doi: 10.1073/pnas.0906183107
González-Forero M. (2023). How development affects evolution. Evolution 77, 562–579. doi: 10.1093/evolut/qpac003
Hu J., Barrett R. D. H. (2017). Epigenetics in natural animal populations. J. Evolutionary Biol. 30, 1612–1632. doi: 10.1111/jeb.13130
Inkster A. M., Wong M. T., Matthews A. M., Brown C. J., Robinson W. P. (2023). Who’s afraid of the X? Incorporating the X and Y chromosomes into the analysis of DNA methylation array data. Epigenet. Chromatin 16, 1. doi: 10.1186/s13072-022-00477-0
Kolberg L., Raudvere U., Kuzmin I., Vilo J., Peterson H. (2020). gprofiler2 – an R package for gene list functional enrichment analysis and namespace conversion toolset g:Profiler. F1000Research 9, ELIXIR–ELI709. doi: 10.12688/f1000research.24956.2
Krueger F., Andrews S. R. (2011). Bismark: a flexible aligner and methylation caller for Bisulfite-Seq applications. Bioinformatics 27, 1571–1572. doi: 10.1093/bioinformatics/btr167
Lea A. J., Altmann J., Alberts S. C., Tung J. (2016). Resource base influences genome-wide DNA methylation levels in wild baboons (Papio cynocephalus). Mol. Ecol. 25, 1681–1696. doi: 10.1111/mec.13436
Leung C., Breton S., Angers B. (2016). Facing environmental predictability with different sources of epigenetic variation. Ecol. Evol. 6, 5234–5245. doi: 10.1002/ece3.2283
Lokk K., Modhukur V., Rajashekar B., Märtens K., Mägi R., Kolde R., et al. (2014). DNA methylome profiling of human tissues identifies global and tissue-specific methylation patterns. Genome Biol. 15, r54. doi: 10.1186/gb-2014-15-4-r54
Loy A., Ciucci P., Guidarelli G., Roccotelli E., Colangelo P. (2021). Developmental instability and phenotypic evolution in a small and isolated bear population. Biol. Lett. 17, 20200729. doi: 10.1098/rsbl.2020.0729
Loy A., Genov P., Galfo M., Jacobone M. G., Vigna Taglianti A. (2008). Cranial morphometrics of the Apennine brown bear (Ursus arctos marsicanus) and preliminary notes on the relationships with other southern European populations. Ital. J. Zoology 75, 67–75. doi: 10.1080/11250000701689857
Meröndun J., Murray D. L., Shafer A. B. A. (2019). Genome-scale sampling suggests cryptic epigenetic structuring and insular divergence in Canada lynx. Mol. Ecol. 28, 3186–3196. doi: 10.1111/mec.15131
Mittelstaedt N. N., Becker A. L., de Freitas D. N., Zanin R. F., Stein R. T., de Souza A. P. D. (2021). DNA methylation and immune memory response. Cells 10, 2943. doi: 10.3390/cells10112943
Moczek A. P., Sultan S., Foster S., Ledón-Rettig C., Dworkin I., Nijhout H. F., et al. (2011). The role of developmental plasticity in evolutionary innovation. Proc. R. Soc. B. 278, 2705–2713. doi: 10.1098/rspb.2011.0971
Peaston A. E., Whitelaw E. (2006). Epigenetics and phenotypic variation in mammals. Mamm. Genome: Off. J. Int. Mamm. Genome Soc. 17, 365–374. doi: 10.1007/s00335-005-0180-2
Reimand J., Kull M., Peterson H., Hansen J., Vilo J. (2007). g:Profiler–a web-based toolset for functional profiling of gene lists from large-scale experiments. Nucleic Acids Res. 35, W193–W200. doi: 10.1093/nar/gkm226
Rey O., Eizaguirre C., Angers B., Baltazar-Soares M., Sagonas K., Prunier J. G., et al. (2020). Linking epigenetics and biological conservation: Towards a conservation epigenetics perspective. Funct. Ecol. 34, 414–427. doi: 10.1111/1365-2435.13429
Swenson J. E., Ciucci P., Huber Đ., Penteriani V., Zedrosser A. (2023). “Brown bear ursus arctos linnaeus 1758,” in Handbook of the mammals of europe. Eds. Hackländer K., Zachos F. E. (Cham: Springer, Cham), 1–36. doi: 10.1007/978-3-319-65038-8_147-1
Tumendemberel O., Hendricks S. A., Hohenlohe P. A., Sullivan J., Zedrosser A., Sæbø M., et al. (2023). Range-wide evolutionary relationships and historical demography of brown bears (Ursus arctos) revealed by whole-genome sequencing of isolated central Asian populations. Mol. Ecol. 32, 5156–5169. doi: 10.1111/mec.17091
Venney C. J., Anastasiadi D., Wellenreuther M., Bernatchez L. (2023). The evolutionary complexities of DNA methylation in animals: from plasticity to genetic evolution. Genome Biol. Evol. 15, evad216. doi: 10.1093/gbe/evad216
Verhoeven K. J. F., Preite V. (2014). Epigenetic variation in asexually reproducing organisms. Evolution; Int. J. Organic Evol. 68, 644–655. doi: 10.1111/evo.12320
Vilgalys T. P., Rogers J., Jolly C. J., Baboon Genome Analysis, Mukherjee S., Tung J. (2019). Evolution of DNA methylation in papio baboons. Mol. Biol. Evol. 36, 527–540. doi: 10.1093/molbev/msy227
Vogt G. (2022). Paradigm shifts in animal epigenetics: Research on non-model species leads to new insights into dependencies, functions and inheritance of DNA methylation. BioEssays: News Rev. Molecular Cell. Dev. Biol. 44, e2200040. doi: 10.1002/bies.202200040
Keywords: RRBS, methylome, non-model, gene ontology, conservation, population
Citation: Colangelo P, Bonapace IM, Gramolini L, Solano E, Desiato E, Franchini P, Gentile L, Guadagnini R, Kleven O, Kindberg J, Kopatz A and Ciucci P (2025) Intraspecific epigenomics divergence in brown bears (Ursus arctos): insights from genome-wide DNA methylation patterns. Front. Ecol. Evol. 12:1504225. doi: 10.3389/fevo.2024.1504225
Received: 30 September 2024; Accepted: 23 December 2024;
Published: 24 January 2025.
Edited by:
Clement Fisher Kent, York University, CanadaReviewed by:
Guinevere O. U. Wogan, Oklahoma State University, United StatesBlair Perry, University of California, Santa Cruz, United States
Copyright © 2025 Colangelo, Bonapace, Gramolini, Solano, Desiato, Franchini, Gentile, Guadagnini, Kleven, Kindberg, Kopatz and Ciucci. This is an open-access article distributed under the terms of the Creative Commons Attribution License (CC BY). The use, distribution or reproduction in other forums is permitted, provided the original author(s) and the copyright owner(s) are credited and that the original publication in this journal is cited, in accordance with accepted academic practice. No use, distribution or reproduction is permitted which does not comply with these terms.
*Correspondence: Laura Gramolini, bGF1cmEuZ3JhbW9saW5pQGlyZXQuY25yLml0