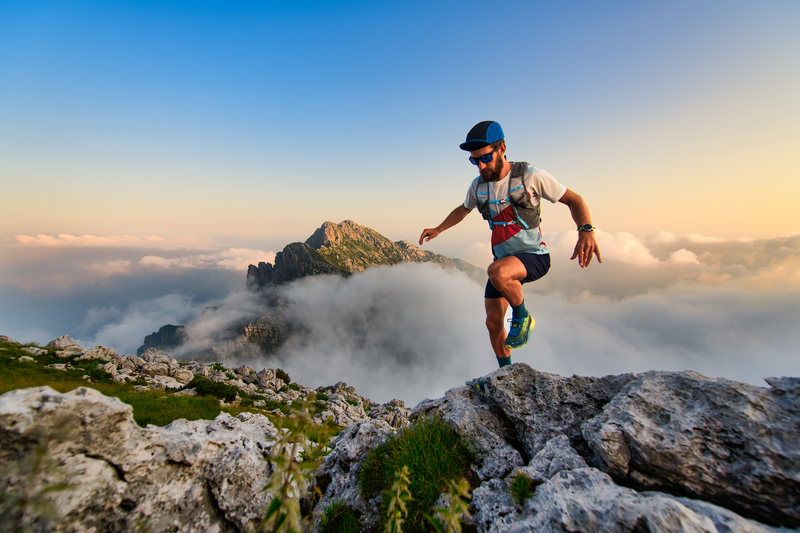
95% of researchers rate our articles as excellent or good
Learn more about the work of our research integrity team to safeguard the quality of each article we publish.
Find out more
BRIEF RESEARCH REPORT article
Front. Ecol. Evol. , 15 November 2024
Sec. Conservation and Restoration Ecology
Volume 12 - 2024 | https://doi.org/10.3389/fevo.2024.1482783
This article is part of the Research Topic Conservation of the World’s Butterflies: Recent Advances in Science and Practice View all 6 articles
Scientists have documented effects of climate and land use change across a range butterfly species. However, incorporating future climate and land use change into butterfly conservation plans is a difficult task. These difficulties arise mainly from assumptions that future processes are the same as past processes (stationarity) and because scientists cannot reliably predict the future (deep uncertainty). In this case study, I used land use and climate change scenarios to compare possible futures for the regal fritillary, a grassland butterfly in the central United States. My analysis indicated that climate and land use change have the potential to influence species persistence, but that climate change has the larger effect. Moderate warming scenarios may improve the possibility of persistence, whereas extreme warming reduces this possibility. My analysis demonstrates the importance of considering nonstationarity and alternative plausible futures in butterfly conservation planning.
Scientists and conservation practitioners have documented abundance and distribution loss across butterfly species from around the globe. In Europe, analyses indicated that butterfly species declines were related to land use practices and climate change (Warren et al., 2021). In North America, researchers found similar trends, but concluded these declines were mediated by climate change (Crossley et al., 2019). Whether studies like these point to a general global decline is debatable (Saunders et al., 2019). But studies like these show that many butterfly species may be affected by both climate and land use change.
One may expect that some regions of the landscape may serve as refugia as the effects of climate and landscape changes accumulate (Habel et al., 2023). The specter of these potential changes highlights the importance of designing butterfly conservation plans that respond to the threats posed by future climate and land use change. Incorporating these considerations into conservation planning exercises is not an easy task, though. The simplest approach is to apply past knowledge (e.g. a model) to projections of future climate or land use and then forecast how a species will respond to those new conditions. But this approach assumes that the same processes that generated past data are operating in the future (i.e., stationarity). Assuming stationary processes under climate and land use change can lead to inferential errors and the misallocation of conservation effort (Nichols et al., 2011). These errors arise because scientists have no reliable way to estimate the risk or assign probabilities to future events in the face of non-stationary processes (Post van der Burg and Tyre, 2011; Ben-Haim, 2023). This lack of reliable estimates makes uncertainty about the future different from the uncertainty most managers work with (i.e. probabilities). Often scientists refer to this kind of uncertainty as “deep uncertainty” (Stanton and Roelich, 2021). It is this deep uncertainty that makes accounting for the effects of future climate and land use change especially challenging for managers.
Perhaps the most practical way to account for deeply uncertain threats in butterfly conservation planning is to adopt the use of climate and land use change scenarios. Scenarios are used in planning efforts to represent plausible futures, rather than as predictions of the future (Peterson et al., 2003). Scenarios allow managers to plan for possible futures. For examples, Lütolf et al. (2009) used land use scenarios to explore the potential effects of changes in major vegetation types (e.g., grassland, forests, cropland) on butterfly abundance in Switzerland. Their findings indicate that more active management approaches will be needed in the future to maintain butterfly diversity. Diengdoh et al. (2023) used scenarios to explore the joint effects of climate and land use change on multiple butterfly species in Australia. Still others have used scenarios to map the projected habitat mismatch for multiple European butterfly species (Schweiger et al., 2008) or to disentangle the relative strength of climate or land use change (Vermaat et al., 2017).
While the scenarios used in these approaches do not assume stationary climate or land use change processes, the predictive models used to forecast how species will respond to those changes often do assume stationarity. In other words, the average parameter values in these predictive models are assumed to remain the same in the future. The implications of this assumption may be particularly impactful for threatened and endangered butterfly species. Often, the focus of managers is to ensure that these species persist on the landscape. However, persistence is typically measured as the number of times a species abundance drops to zero or below a threshold using simulations that rely on species-specific predictive models (McCarthy et al., 2003). If the processes underlying these relationships change in the future (i.e., are nonstationary), then one is not able to reliably forecast species persistence for different climate or land use futures using the same predictive model. This means that some scenario analyses based on such a model may misrepresent the level of persistence managers can expect for a given conservation action or policy.
An alternative approach is to assess the robustness of different actions using tools from the field of information-gap decision theory (a.k.a. info-gap decision theory; Ben-Haim, 2006). The info-gap framework defines robustness as the amount of uncertainty one can tolerate before reaching a point of failure. In the context of threatened and endangered species, we might compare two actions or scenarios in terms of how much uncertainty in performance we can tolerate before reaching an extinction threshold. Actions that require more uncertainty before reaching that threshold are said to be more robust. In other words, we can be more wrong about mechanisms and processes that underly these models, while ensuring a minimum level of conservation performance.
In this case study, I explored the use of scenarios and info-gap decision theory to assess the abundance and distributional response of a grassland-specialist butterfly in the Central United States. I conducted the analysis under different land use and climate futures to understand how the relative effects of climate and land use change influence the possibility that this species will persist into the future.
My analysis focused on the Prairie Pothole Region of the United States (PPR; Figure 1 inset). This region is comprised of thousands of closed-basin wetlands that were historically surrounded by uplands covered with tall and mixed-grass native prairies (Dahl, 2014). The grasslands and wetlands of this region are a conservation priority for wildlife managers (Doherty et al., 2013; Prairie Pothole Joint Venture, 2017). Scientists have recognized the importance of this region in supporting invertebrate pollinators such as European honeybees (Apis mellifera), which are economically important for supporting crop pollination and commercial honey production (Otto et al., 2022).
Figure 1. Maps of grasslands in the Prairie Pothole Region of the United States. These maps express the proportion of grassland within a 1-km buffer around each pixel. Dark green pixels contain more grass (maximum of 1.0) and white indicates where there is no grass (minimum of 0.0). Each map represents a different land use scenario: (A) current condition (year 2014), (B) business-as-usual (year 2100), (C) billion-ton-update (year 2100), (D) Intergovernmental Panel on Climate Change Special Report on Emissions Scenarios (SRES) A2 Storyline (year 2100). Inset shows the location of the Prairie Pothole Region in the conterminous United States.
The species I selected for this study was the regal fritillary (Argynnis idalia), a grassland butterfly that was once widespread throughout North America. Its current distribution is focused in the U.S. Midwest and Great Plains, but there remains one small relict population in the mid-Atlantic region (Selby, 2007). Because of the decline in regal fritillary abundance and distribution, this species is now a candidate for listing under the U.S. Endangered Species Act, which is enforced by the U.S. Fish and Wildlife Service (FWS). As part of the listing process, the FWS has begun assembling a species status assessment (Smith et al., 2018), which consolidates the needed information about the species’ biology and its conservation status. The current distribution of the regal fritillary is much larger than the study area I am focusing on here. However, previous analyses of air temperature warming showed regal distributions were likely to shift northward within Great Plains and Midwest (Post van der Burg et al., 2023). Additionally, land use change scenarios had already been developed for this region (see section 2.3).
I used the model published by Post van der Burg et al. (2023), which is an inhomogeneous point process model (IPP; see Hefley and Hooten, 2016) that estimates intensity of use for the regal fritillary across the landscape. This model was built using abundance data from the North American Butterfly Association Fourth-of-July Counts, which is a citizen science butterfly monitoring program (Swengel, 1990). I used the predictions from this model as the basis of my analysis. The model contains four fixed parameters: two land cover variables (proportion of grass and proportion of trees within 1 km of a point) and two climate variables (average temperature and total precipitation in May and June). The choice of these variables is summarized in Post van der Burg et al. (2023), but they based their choices on previously documented analyses and observations for the regal fritillary. The model estimates show that regal fritillary habitat use is positively related to the proportion of grass within 1 km of a point, negatively related with the proportion of tree cover, and quadratically related to both spring temperature and precipitation, indicating the existence of an optimal combination of precipitation (72-216 mm) and temperature (15-20°C). These latter two parameters had been suggested as important in unpublished reports but was confirmed by Post van der Burg et al. (2023). This model was originally fit to data at a 30 m resolution, and I maintained that resolution in this analysis. Since the model is a Poisson regression, it estimates the average intensity of use at a point on the natural log scale. These estimates can be translated back to the response scale by applying an inverse-log transformation. The estimates on the response scale can be summed to estimate abundance over a larger geographic area (Gotway and Young, 2002).
I considered 11 different land use change scenarios based on different storylines for the Prairie Pothole Region (Sohl et al., 2018). These storylines reflect different assumptions about the future: business-as-usual, prioritizing environmental protection, or prioritizing economic development. Each land use scenario was combined with three different climate change scenarios developed by the Intergovernmental Panel on Climate Change (IPCC, 2000). These climate scenarios included the Representative Concentration Pathways (RCP) 4.5 and 8.5, as well as a scenario that was the average between the two. For context, RCP 4.5 (4.5 W/m2) can be considered a moderate warming scenario whereas RCP 8.5 represents a more extreme warming scenario. Each scenario is served as a 30 m resolution raster of land cover over a period spanning 2014 – 2100. Land cover classes were developed by the creators by combining land cover classes in the National Land Cover Database (Dewitz, 2023) and Cropland Data Layer (U.S. Department of Agriculture, 2023). From 2020 on, land cover rasters are provided every decade. My analysis focused on the 2014 land cover (i.e., “reference condition”) and land cover in the year 2100. For this analysis, I combined pixels classified as “grassland” and “perennial grass” into a single “grass” category. I also combined three forest classes into a single “tree” class.
I selected three land use scenarios to represent “bookends” of plausible futures. The first represented a “business-as-usual” (BAU) scenario in which current trends of agricultural development remain constant over time. The second was based on the “Billion-ton-update” (BTU) developed by the U.S. Department of Energy (U.S. Department of Energy, 2024). Under this scenario, perennial vegetation cover is maximized as a biofuel feedstock. The scenarios from Sohl et al. (2018) represent perennial vegetation as grass cover. The last scenario I chose was based on the IPCC Special Report on Emissions Scenarios (SRES) A2 storyline (IPCC, 2000). Under this story line, conventional cropland expands to meet a growing population and the highest number of grass pixels are lost of any of the 11 scenarios.
I also considered whether habitat protection could affect regal fritillary responses under each of these land use scenarios. I created three new land use scenarios which represented protection of grass beginning in 2014 and up to 2100. To simulate protection, I simply assumed that grass pixels that were lost between 2014 and 2100 were replaced in 2100 for each of the three scenarios described above. I then modified the name of each scenario with the term “protection”, if lost grass pixels were added back in 2100, and “do nothing” if they were not.
The model I used to predict regal fritillary use included parameters for spring (May and June) temperature and precipitation, so I created weather rasters to go along with each land use scenario. I downloaded temperature and precipitation forecasts for both RCP scenarios mentioned earlier from the Northwest Knowledge Network website (Northwest Knowledge Network, 2024). The data served on this site are projections from 20 different Global Circulation Models (GCM) associated with the Coupled Model Inter-Comparison Project 5 (CMIP5), which have been downscaled to 4-km resolution using the Multivariate Adaptive Constructed Analogs (MACA) method (Abatzoglou and Brown, 2012). I ensemble averaged the 20 model projections to create a single average projection for both the 4.5 and 8.5 climate scenarios. I resampled the ensemble climate futures to match the 30 m resolution of land use scenario rasters. With all land use and climate scenarios combined, I considered 12 different scenarios (Supplementary Material, Supplementary Table 1).
I performed my analyses in the R programming environment (R Core Team, 2024). I conducted a qualitative comparison of predicted habitat use for regal fritillaries in 2014 and 2100 by generating maps of estimated use values and plotting them side-by-side. I used these estimates from the model to generate cumulative relative abundance estimates across the PPR. I use these cumulative estimates to quantitatively compare the performance of the 12 scenarios.
I used an info-gap analysis to assess how uncertainty impacts the possibility of regal fritillaries persisting. This kind of analysis requires a system model, a model of uncertainty, and a minimum performance criterion (Ben-Haim, 2006). The system model is already specified as the IPP model I mentioned earlier. I used a proportional error model for the model of uncertainty: . Here µ is the nominal estimate of intensity of use for pixel j under scenario i, σ is the standard error, alpha is the horizon of uncertainty (or a measure of robustness), and is the new abundance estimate once uncertainty (i.e. error) has been subtracted from the original nominal estimates. I compared these abundance estimates to a minimum performance criterion, which was 5,000 individuals (Traill et al., 2010). I am using this criterion as a surrogate for persistence since I am not able to estimate extinction probabilities reliably using these scenarios. One should note that to mimic how most species-specific scenario analyses are done, one should assume that α is zero (i.e., there is no uncertainty being considered). My analysis, by comparison, asks how large is when drops below 5,000. Scenarios that exhibit higher values of are regarded as more robust (Ben-Haim, 2006).
The twelve scenarios I considered presented distinctly different futures for the landscape of the PPR. When compared with the reference condition (Figure 1A), the “business-as-usual scenario” showed a decline in grass cover (Figure 1B). This loss of grass was more pronounced under a future where agricultural production was prioritized (Figure 1D), whereas grass increased under the scenario where perennial vegetation was prioritized as a potential biofuel feedstock (Figure 1C).
From a climate change point of view, the RCP 4.5 scenario indicated increased warming could be expected in the southern portion of the PPR (Figure 2B) when compared to the reference condition (Figure 2A). The RCP 8.5 scenario showed a pronounced pattern of warming when compared to either the 4.5 scenario or reference condition (Figure 2C). Precipitation in the region was expected to increase under the 4.5 scenario (Figure 2E) when compared to the reference condition (Figure 2D), but precipitation patterns were comparable to the reference condition under the 8.5 scenario (Figure 2F).
Figure 2. Maps of temperature (A–C) and precipitation (D–F) values for the Prairie Pothole Region of the United States. Panels represent mapped values for the year 2014 (A, D); and projections for the year 2100 under an Intergovernmental Panel on Climate Change Representative Concentration Pathway 4.5 W/m2 (IPCC RCP 4.5) warming scenario (B, E) and under an 8.5 scenario (C, F).
I compared the effects of the two future climate scenarios to inspect how the broader scale impact of climate change might affect regal fritillary use of the landscape. Inspection of these maps showed that, when compared with the reference condition (Figure 3A), the area of useable habitat conditions increased under the 4.5 scenario, with decreases in the southern portion of the region (Figure 3B). The area of used habitat decreased over a wide portion of the region under the 8.5 scenario (Figure 3C). Further comparison of these climate scenarios shows that total relative abundance was between 1 million and 2.5 million regal fritillaries under RCP 4.5 assuming average parameter values in the model (Figure 4; i.e., α = 0). In contrast, under RCP 8.5 the predicted relative abundance of regal fritillaries was about three orders of magnitude lower (~30,000 individuals).
Figure 3. Maps of regal fritillary habitat use (i.e. the abundance of use points in a 30-m pixel) in the Prairie Pothole Region of the United States. Mapped values are estimated means from an inhomogeneous Poisson point process model and are expressed on the natural log scale. Panels represent use in the 2014 (A); and in 2100 under an Intergovernmental Panel on Climate Change Representative Concentration Pathway 4.5 W/m2 (IPCC RCP 4.5) warming scenario (B) and an RCP 8.5 scenario (C).
Figure 4. Robustness curves comparing estimated relative abundances of regal fritillaries in the Prairie Pothole Region of the United States in the year 2100. (A) represents the robustness curves for six different land use and conservation scenarios under an Intergovernmental Panel on Climate Change Representative Concentration Pathway 4.5 W/m2 (IPCC RCP 4.5) warming scenario. (B) represents the robustness curves for the same set of land use scenarios, but under the RCP 8.5 warming scenario. The dashed line represents the minimum performance criterion (i.e., 5,000 individuals). Note the different scales on the y-axis. BAS = business-as-usual, BTU= billion-ton-update, SRES = IPCC Special Report on Emissions Scenarios.
Land use change also appears to have affected regal fritillary use. For example, a future where agricultural production increased resulted in the fewest number of regal fritillaries, whereas a future where perennial vegetation increased resulted in the highest number (Figure 4A). Overall, scenarios where grass was protected up to the year 2100 generally resulted in higher abundances. However, the largest effect of protecting grass was in the case where agricultural production increased (Figure 4A). In terms of the effect on regal fritillary persistence, all six scenarios were robust to the range of uncertainty I considered. In other words, abundance never dropped below the minimum performance threshold for the values of α I considered. I found a similar pattern of the relative effects of land use change and protection on abundance under the RCP 8.5 scenario, but absolute performance was so similar that the lines overlapped (Figure 4B). Despite these similarities, these scenarios showed much less robustness to uncertainty. In fact, relative abundance dropped below the minimum performance criterion at around one standard deviation. This indicates that continued regal fritillary persistence is much less possible under the RCP 8.5 warming scenario.
I found that climate change appears to have a strong effect on the possibility of regal fritillaries persisting on the landscape of the PPR. In fact, when comparing the population level response of regal fritillaries to climate change, the differences in total abundance were orders of magnitude different between the two climate change scenarios I considered. Some studies of contemporary changes in butterfly distributions have found that land use is more influential on butterfly population trends (Kwon et al., 2021), while studies that forecast future change have found that climate change has a larger influence on some species (Diengdoh et al., 2023). It is likely that the differences in findings between these and other studies are related to the timescale over which their analyses were performed. Perhaps the most comparable study to mine was conducted by Crossley et al. (2021) who found similar patterns when comparing land cover and climate variables. That is, they found that butterfly abundance generally decreased as locations became warmer and drier and increased where locations became cooler and wetter. Interestingly, they used the same data set that was used to parameterize the model I used in this analysis, North American Butterfly Fourth-of-July Counts (4JCs). The authors of the model I used did point out that scaling issues and the haphazard design of the 4JC program initially obscured their ability to detect relationships between abundance and landcover (Post van der Burg et al., 2023). However, their final analysis corrected for many of those design issues and allowed them to detect some influence of land cover on abundance. Still the fact that climate variables had a much stronger influence on habitat use and abundance may be reflective of the importance of microsite conditions for larvae (Post van der Burg et al., 2023), which has been reported for other species (Hill et al., 2021).
With that said, land use change did have some effects on abundance. For example, under a scenario where agricultural production increased, regal fritillary abundance decreased because there was less available grass. This pattern was a little more muted under the business-as-usual scenario since this scenario simply extended current trends of agricultural land use. Of course, the BTU scenario appeared to improve over the other two land use scenarios because there was more perennial grassland cover available. This last finding should be interpreted with caution, however. The BTU scenario is based on the idea that perennial grassland cover, mainly in the form of Miscanthus, will be present on the landscape. Regal fritillaries rely on numerous forb species as nectaring and nursery plants, so it may be that fields dominated with Miscanthus, but with little of the necessary forb species would not sustain this species. This implies that one component of management targeting regal fritillary recovery may be to ensure that any perennial grasslands also contain this forb component.
In the context of land use change, protecting grassland pixels resulted in higher regal fritillary abundances, which was true across the scenarios I considered. The marginal increase in abundance was mediated by climate indicating that managers would need to determine whether the marginal gains in abundance and robustness to uncertainty are worth investing in. Diengdoh et al. (2023) suggested that expanding protected area networks will likely be key when aiming to restore landscape functional connectivity. However, Hodgson et al. (2009) expressed concern over overemphasizing the importance of connectivity in protecting habitat. Rather, they suggest that improving connectivity should be assessed relative to other strategies that may enhance robustness to climate change, such as increasing habitat area and habitat quality.
My analysis of the robustness of a policy of protection illustrates why such assessments are important. Obviously, a policy of protection under RCP 4.5 was generally robust to climate change in the sense that it resulted in more useable habitat. However, under the RCP 8.5 scenario, a protection policy did very little to increase robustness. This indicates that other strategies will need to be considered under the 8.5 scenario. These strategies might include restoring grasslands in the portion of the PPR that contains optimal spring weather conditions or looking beyond the PPR. Initial targeting of these areas might be done through climate envelope modeling. Then this strategy could be compared in terms of its robustness to a policy of protection or a policy of grassland restoration.
This analysis also points to a fundamental issue with conservation planning under uncertainty. That is, the seemingly inevitable tradeoff between conservation performance and robustness to uncertainty (Ben-Haim, 2023). Understanding this tradeoff in the context of scenario exploration is important for decision makers to grasp because it sets the stage for designing new strategies that may improve robustness. Similarly, relying on models that were built under past conditions necessarily opens the door to egregious failures of the model because novel conditions in the future are likely given the nonstationarity of climate and ecological systems. Had I not incorporated uncertainty into my scenario analysis (i.e. assumed α = 0), I would have reached the conclusion that regal fritillaries were likely to persist regardless of future conditions. Shifting to focusing on robustness illustrates how a different conclusion could be reached regarding regal persistence.
Because my analysis was nonprobabilistic, I had to rely on an abundance surrogate to represent persistence. There are obvious problems with this approach. As others have pointed out, specifying a general threshold minimum population size may not be supported by data or theory (Flather et al., 2011), though others have shown that population viability analyses may provide reliable relative predictions (McCarthy et al., 2003). On the other hand, minimum viable population sizes may be useful for giving decision makers a relative sense of when a population may have declined too much (Traill et al., 2010). Smith et al. (2018) has used the concept of population viability to guide the development of the biological assessment process that is used by the U.S. Fish and Wildlife Service to inform their development of policy. Thus, I would argue that my use of a minimum criterion was intended to serve as a relative measure of performance. In other words, this threshold does not provide an actual extinction threshold, but rather a single number that can be used to compare two or more scenarios.
With that in mind, this case study demonstrates how important it is to consider a range of plausible futures when considering how to conserve butterflies. That is, the benefits of different conservation policies could change depending on the type of future one is considering. Analyzing a range of potential futures serves at least two purposes in conservation planning. First, it gives decision makers a sense of how to calibrate their expectations about the effectiveness of conservation actions. In other words, is it even plausible that one would avoid population decline or potential extinction under a given scenario? Second, it allows them the opportunity to explore why certain actions may fail, which should help them develop strategies to mitigate those failures. Lastly, this case study emphasizes the notion of nonstationarity and demonstrates the utility of assuming our models of the future may not be reliable. Taken together, combining scenarios with other robust decision-making tools (Bonjean Stanton and Roelich, 2021) may be one effective way to develop more durable and long-lasting butterfly conservation plans for the future.
Publicly available datasets were analyzed in this study. This data can be found here: ScienceBase Archive: https://doi.org/10.5066/P9YQMNHF, Northwest Knowledge Network Archive of Multivariate Adaptive Constructed Analogs (MACA) Datasets: https://climate.northwestknowledge.net/MACA/index.php, The model used in this study can be found at https://doi.org/10.1002/ecs2.4429.
MP: Conceptualization, Data curation, Formal analysis, Methodology, Software, Visualization, Writing – original draft, Writing – review & editing.
The author(s) declare financial support was received for the research, authorship, and/or publication of this article. This work was funded by the Ecosystem Mission Area of the U.S. Geological Survey.
The author declares that the research was conducted in the absence of any commercial or financial relationships that could be construed as a potential conflict of interest.
All claims expressed in this article are solely those of the authors and do not necessarily represent those of their affiliated organizations, or those of the publisher, the editors and the reviewers. Any product that may be evaluated in this article, or claim that may be made by its manufacturer, is not guaranteed or endorsed by the publisher.
Any use of trade, firm, or product names is for descriptive purposes only and does not imply endorsement by the U.S. Government.
The Supplementary Material for this article can be found online at: https://www.frontiersin.org/articles/10.3389/fevo.2024.1482783/full#supplementary-material
Abatzoglou J. T., Brown T. J. (2012). A comparison of statistical downscaling methods suited for wildfire applications. Int. J. Climatol. 32, 772–780. doi: 10.1002/joc.2312
Ben-Haim Y. (2006). Information-gap decision theory: decisions under severe uncertainty (London, UK: Academic Press).
Ben-Haim Y. (2023). Managing uncertainty in decision-making for conservation science. Conserv. Biol. 37, e14164. doi: 10.1111/cobi.14164
Crossley M. S., Smith O. M., Berry L. L., Phillips-Cosio R., Glassberg J., Holman K. M., et al. (2021). Recent climate change is creating hotspots of butterfly increase and decline across North America. Global Change Biol. 27, 2702–2714. doi: 10.1111/gcb.15582
Dahl T. E. (2014). Status and Trends of Prairie Wetlands in the United States 1997 to 2009 (Washington D.C: U.S. Department of the Interior, Fish and Wildlife Service, Ecological Services).
Dewitz J. (2023). “National land cover database (NLCD) 2021 products,” in U.S. Geological Survey data release. Reston, VA: U.S. Geological Survey. doi: 10.5066/P9JZ7AO3
Diengdoh V. L., Ondei S., Amin R. J., Hunt M., Brook B. W. (2023). Landscape functional connectivity for butterflies under different scenarios of land-use, land-cover, and climate change in Australia. Biol. Conserv. 277, 109825. doi: 10.1016/j.biocon.2022.109825
Doherty K. E., Ryba A. J., Stemler C. L., Niemuth N. D., Meeks W. A. (2013). Conservation planning in an era of change: state of the U.S. Prairie pothole region. Wildlife. Soc. Bull. 37, 546–563. doi: 10.1002/wsb.284
Flather C. H., Hayward G. D., Beissinger S. R., Stephens P. A. (2011). Minimum viable populations: is there a ‘magic number’ for conservation practitioners? Trends Ecol. Evol. 26, 307–316. doi: 10.1016/j.tree.2011.03.001
Gotway C. A., Young L. J. (2002). Combining incompatible spatial data. J. Am. Stat. Assoc. 97, 632–648. doi: 10.1198/016214502760047140
Habel J. C., Ulrich W., Gros P., Teucher M., Schmitt T. (2023). Butterfly species respond differently to climate warming and land use change in the northern Alps. Sci. Total. Environ. 890, 164268. doi: 10.1016/j.scitotenv.2023.164268
Hefley T. J., Hooten M. B. (2016). Hierarchical species distribution models. Curr. Landscape Ecol. Rep. 1, 87–97. doi: 10.1007/s40823-016-0008-7
Hill G. M., Kawahara A. Y., Daniels J. C., Bateman C. C., Scheffers B. R. (2021). Climate change effects on animal ecology: butterflies and moths as a case study. Biol. Rev. 96, 2113–2126. doi: 10.1111/brv.12746
Hodgson J. A., Thomas C. D., Wintle B. A., Moilanen A. (2009). Climate change, connectivity and conservation decision making: back to basics. Journal of Applied Ecology 46, 964–969. doi: 10.1111/j.1365-2664.2009.01695.x
IPCC. (2000). Special report on Emission s Scenarios. Eds. Nakicenovic N., Swart R. (Cambridge, UK: Cambridge University Press).
Kwon T.-S., Lee C. M., Kim E.-S., Won M., Kim S.-S., Park Y.-S. (2021). Habitat change has greater effects than climate change on butterfly occurrence in South Korea. Global Ecol. Conserv. 26, e01464. doi: 10.1016/j.gecco.2021.e01464
Lütolf M., Bolliger J., Kienast F., Guisan A. (2009). Scenario-based assessment of future land use change on butterfly species distributions. Biodiversity and Conservation 18, 1329–1347. doi: 10.1007/s10531-008-9541-y
McCarthy M. A., Andelman S. J., Possingham H. P. (2003). Reliability of relative predictions in population viability analysis. Conserv. Biol. 17, 982–989. doi: 10.1046/j.1523-1739.2003.01570.x
Nichols J., Koneff M., Heglund P., Knutson M., Seamans M., Lyons J., et al. (2011). Climate change, uncertainty, and natural resource management. Journal of Wildlife Management 75, 6–18. doi: 10.1002/jwmg.33
Northwest Knowledge Network (2024). Multivariate Adaptive Constructed Analogs (MACA) Datasets. Available online at: https://climate.northwestknowledge.net/MACA/index.php (Accessed October 1, 2024).
Otto C. R., Zheng H., Hovick T., Post van der Burg M., Geaumont B. (2022). Grassland conservation supports migratory birds and produces economic benefits for the commercial beekeeping industry in the U.S. Great Plains. Ecol. Econ. 197, 107450. doi: 10.1016/j.ecolecon.2022.107450
Peterson G. D., Cumming G., Carpenter S. (2003). Scenario planning: a tool for conservation in an uncertain world. Conserv. Biol. 17, 358–366. doi: 10.1046/j.1523-1739.2003.01491.x
Post van der Burg M., MacDonald G., Hefley T., Glassberg J. (2023). Point-scale habitat and weather patterns influence the distribution of regal fritillaries in the central United States. Ecosphere 14, e4429. doi: 10.1002/ecs2.4429
Post van der Burg M., Tyre A. (2011). Integrating info-gap decision theory with robust population management: a case study using the Mountain Plover. Ecol. Appl. 21, 303–312. doi: 10.1890/09-0973.1
Prairie Pothole Joint Venture. (2017). Prairie Pothole Joint Venture Implementation Plan (Denver, Colorado: U.S. Fish and Wildlife Service).
R Core Team. (2024). _R: A Language and Environment for Statistical Computing (Vienna, Austria: R Foundation for Statistical Computing). Available at: https://www.R-project.org/.
Saunders M. E., Janes J., O’Hanlon J. C. (2019). Moving on from the insect apocalypse narrative: engaging with evidence-based insect conservation. BioScience 70, 80–89. doi: 10.1093/biosci/biz143
Schweiger O., Settele J., Kudrna O., Klotz S., Kühn I. (2008). Climate change can cause spatial mismatch of trophically interacting species. Ecology 89, 3472–3479. doi: 10.1890/07-1748.1
Selby G. (2007). Regal Fritillary (Drury):A Technical Conservation Assessment (Rocky Mountain Region: USDA Forest Service).
Smith D. R., Allan N. L., McGowan C. P., Szymanski J. A., Oetker S. R., Bell H. M. (2018). Development of a species status assessment process for decisions under the U.S. Endangered species act. J. Fish. Wildlife. Manage. 9, 302–320. doi: 10.3996/052017-JFWM-041
Sohl T. L., Dornbierer, Wika S. (2018). 33 high-resolution scenarios of land use and vegetation change in the Prairie Potholes of the United States (Reston, VA: U.S. Geological Survey data release). doi: 10.5066/P9YQMNHF
Stanton M., Roelich K. (2021). Decision making under deep uncertainties: A review of the applicability of methods in practice. Technol. Forecasting. Soc. Change 171, 120939. doi: 10.1016/J.TECHFORE.2021.120939
Swengel A. B. (1990). Monitoring butterfly populations using the fourth of July butterfly count. Am. Midland. Nat. 124, 395–406. doi: 10.2307/2426190
Traill L. W., Brook B. W., Frankham R. R., Bradshaw C. J. (2010). Pragmatic population viability targets in a rapidly changing world. Biol. Conserv. 143, 28–34. doi: 10.1016/j.biocon.2009.09.001
U.S. Department of Agriculture. (2023). National Agricultural Statistics Service Cropland Data Layer. Available online at: http://nassgeodata.gmu.edu/CropScape/ (Accessed October 1, 2024).
U.S. Department of Energy. (2024). 2023 Billion-Ton Report: An Assessment of U.S. Renewable Carbon Resources (Oak Ridge, TN: U.S. Department of Energy, Oak Ridge National Laboratory).
Vermaat J., Hellmann F., Teeffelen A., Minnen J., Alkemade R., Billeter R., et al. (2017). Differentiating the effects of climate and land use change on European biodiversity: A scenario analysis. Ambio 46, 277–290. doi: 10.1007/s13280-016-0840-3
Keywords: regal fritillary, climate change, land use change, uncertainty, decision making
Citation: Post van der Burg M (2024) Measuring butterfly persistence in the face of deep uncertainty: a case study using the regal fritillary. Front. Ecol. Evol. 12:1482783. doi: 10.3389/fevo.2024.1482783
Received: 18 August 2024; Accepted: 18 October 2024;
Published: 15 November 2024.
Edited by:
Carolina Puerta-Pinero, Joint Research Centre (JRC), ItalyReviewed by:
Jaime A Collazo, North Carolina State University, United StatesCopyright © 2024 Post van der Burg. This is an open-access article distributed under the terms of the Creative Commons Attribution License (CC BY). The use, distribution or reproduction in other forums is permitted, provided the original author(s) and the copyright owner(s) are credited and that the original publication in this journal is cited, in accordance with accepted academic practice. No use, distribution or reproduction is permitted which does not comply with these terms.
*Correspondence: Max Post van der Burg, bWF4cG9zdHZhbmRlcmJ1cmdAdXNncy5nb3Y=
Disclaimer: All claims expressed in this article are solely those of the authors and do not necessarily represent those of their affiliated organizations, or those of the publisher, the editors and the reviewers. Any product that may be evaluated in this article or claim that may be made by its manufacturer is not guaranteed or endorsed by the publisher.
Research integrity at Frontiers
Learn more about the work of our research integrity team to safeguard the quality of each article we publish.