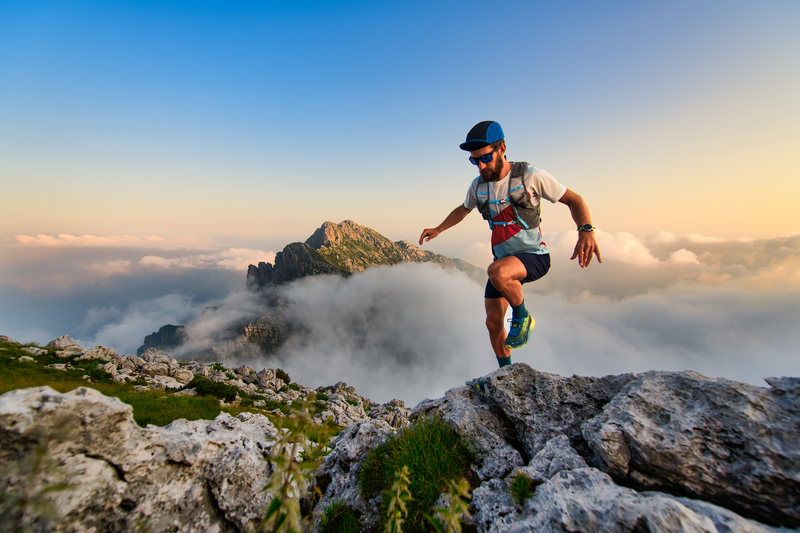
95% of researchers rate our articles as excellent or good
Learn more about the work of our research integrity team to safeguard the quality of each article we publish.
Find out more
ORIGINAL RESEARCH article
Front. Ecol. Evol. , 04 October 2024
Sec. Biogeography and Macroecology
Volume 12 - 2024 | https://doi.org/10.3389/fevo.2024.1448842
The emergence of metacommunity theory has provided a framework for studying the spatial structure of biological communities. To unravel the underlying driving mechanisms of macroinvertebrate metacommunity structure and diversity, this paper evaluates macroinvertebrate data from a water body in eastern China using variance decomposition and stratified partitioning. The results suggest that spatial processes dominate macroinvertebrate metacommunity structure, while the combined effects of spatial processes, aquatic environment, and geoclimatic factors also explain part of the macroinvertebrate community structure. Spatial processes, aquatic environment, and geoclimatic factors significantly influenced macroinvertebrate alpha diversity and indirectly influenced beta diversity through alpha diversity. Therefore, the significant effects of spatial processes and geoclimatic variables cannot be ignored in biological assessments, especially biodiversity assessments. Our findings elucidate the metacommunity dynamics in the region and provide crucial information for conservation planning in riverine ecosystems.
Metacommunity theory has gained increasing popularity among biologists, particularly for its use in unraveling the mechanisms behind the assembly of biological communities (Liu et al., 2023; Siqueira et al., 2012; Zheng and Yin, 2023). Understanding these mechanisms is crucial as it can inform biological conservation about the dominant drivers of community changes from local to regional scales (Leibold et al., 2004; Thompson et al., 2020). According to metacommunity theory, community formation is influenced by four main ecological processes (Leibold et al., 2004): (1) environmental filtering by abiotic conditions, (2) interactions between organisms, (3) dispersal of organisms among patches, and (4) ecological drift due to stochastic effects. In this study, we focus on environmental filtering and dispersal because they are likely to be closely related to human disturbance.
The mechanisms of community formation can be unraveled based on metacommunity theory, but little is known about the changes and differences in community composition (Heino et al., 2015). While alpha diversity primarily reflects variation in species composition within a community (Li et al., 2019), beta diversity reflects variation in species composition between communities (Hawkins et al., 2015). There are several ways to measure beta diversity, with the division of total beta diversity into turnover and nestedness being one of the most common methods used by community ecologists (Baselga, 2010). When the turnover component of community beta diversity is large, it is assumed that more sites should be protected, whereas when nestedness is large in community beta diversity, it is assumed that species-rich sites should be prioritized for protection (Soininen et al., 2018). Nonetheless, implementation remains difficult for managers because the specific sites to be protected remain ambiguous. This problem is well addressed by the recent emergence of the Local Contribution to Beta Diversity (LCBD) approach, which allows for the identification of sites with a high contribution to beta diversity (unique species composition) that may be prioritized for restoration (Perez Rocha et al., 2023).
A large number of studies have shown (Heino et al., 2017; Li et al., 2020; Moritz et al., 2013) that environmental filtering can be a major influence on community composition and diversity. This filtering primarily involves water environment and land use reflecting anthropogenic disturbances, as well as climatic geography reflecting natural disturbances. However, this influence may diminish with increasing spatial scale; community change at intermediate to large scales may be more influenced by spatial effects (Chase et al., 2020). Therefore, it is necessary to test the accuracy of these theories across a wider range of water bodies. In this regard, most studies have primarily focused on the relative importance of environmental filtering and spatial effects on community composition and biodiversity. However, the interrelationships between drivers and their direct and indirect effects on communities are not well understood. This problem can be effectively addressed by partial least squares path modeling (PLS-PM), which measures the causal relationships between variables and then visualizes the results using directed acyclic graphs (Oliveira et al., 2019).
Macroinvertebrates play a key role in energy and material cycling in ecosystems, are easy to collect, and exhibit differences in dispersal ability and environmental tolerance among different taxa. For example, EPT taxa (Ephemeroptera, Plecoptera, and Trichoptera) are environmentally sensitive, while oligochaetes are environmentally tolerant. Additionally, aquatic insects generally have stronger dispersal abilities, whereas other taxa tend to have weaker dispersal abilities. Therefore, macroinvertebrates are among the most commonly used organisms in metacommunity and biodiversity studies (Cai et al., 2017; Firmiano et al., 2021; Siqueira et al., 2012).
Eastern China is the most developed region in the country, with significant economic status, and is geographically located in the Lower Yangtze River Basin. Understanding the assembly mechanisms of biomes in this region is therefore of paramount importance. Specifically, we collected macroinvertebrate samples from the region in 2023 to address the following questions: (1) to determine the assembly mechanisms of macroinvertebrate communities, and (2) to identify the key drivers affecting macroinvertebrate communities diversity. We hypothesize the following: (1) Macroinvertebrate communities are determined by a combination of water environment, land use, climate, and spatial factors, but given the large spatial extent of the study area, the influence of spatial factors is likely to dominate (Heino et al., 2015). (2) Macroinvertebrate community alpha diversity is primarily influenced by localized aquatic environmental factors, whereas the contribution of a location to beta diversity (LCBD) is mainly influenced by spatial effects, as dispersal constraints increase the ecological uniqueness of locations (Heino, 2013; Wu et al., 2020). (3) Additionally, macroinvertebrate alpha diversity is expected to show a negative correlation with LCBD because sites with unique species compositions tend to have low species richness (da Silva et al., 2018).
The Yangtze River is the third longest river in the world and the longest river in China, with a total length of over 6,300 km. Its basin covers an area of approximately 1.8 million km², accounting for about 18.8% of China’s land area (http://www.cjw.gov.cn/zjzx/lypgk/zjly/). The lower reaches of the Yangtze River extend for 938 km, with a basin area of 120,000 km2. This region includes the urban agglomeration of the Yangtze River Delta, where Shanghai, China’s largest national center city (Chen et al., 2022; Luo et al., 2024; Yang et al., 2023).
A gradient of human disturbance in the study area was fully considered, and from this, 27 spatially randomly distributed points were selected for field sampling during the spring and fall of 2023 (Figure 1). Macroinvertebrate samples were collected at each site after preparation was complete, with five different habitats set up in wadeable streams to be sampled by two people using a Surber net. These samples were then combined into one composite sample. In non-wadeable streams, five habitats were sampled by two people using Peterson collectors, and these samples were similarly combined into one composite sample. The collected substrate or silt samples were washed through a sieve and sorted in the field for macroinvertebrates. Insect samples were placed in wide-mouth bottles, while larger samples, such as Mollusca, were placed in ziplock bags and preserved in a 75% alcohol solution. To ensure consistency in the identification and classification of samples by different personnel, we used a uniform reference book to identify samples at the family or genus level (Liu et al., 1979; Merritt and Cummins, 1996; Morse et al., 1994).
Water quality data were directly downloaded for subsequent analysis from the National Water Ecology Monitoring Business Application and Data Sharing Platform (https://106.37.208.244:40002/login?redirect=%2Findex). The water quality indicators include water temperature, conductivity, pH, dissolved oxygen, permanganate index, ammonia nitrogen, total phosphorus, total nitrogen, turbidity, and other related data. The monitoring times for these indicators are consistent with those of the biological samples.
We extracted the land use types (forest, cropland, water body, grassland, impervious surface, wasteland) in the upstream catchment of each sampling site and calculated the corresponding percentages based on the 2022 Chinese 30-meter land cover data (https://zenodo.org/records/8176941).
Elevation, slope direction, and slope were extracted using ArcGIS Pro based on downloaded DEM data (https://www.gscloud.cn/). Twenty-one climate factors (April minimum temperature, September minimum temperature, annual mean minimum temperature, April maximum temperature, September maximum temperature, annual mean maximum temperature, April precipitation, September precipitation, annual mean precipitation, April radiation, September radiation, annual mean radiation, April barometric pressure, September barometric pressure, annual mean barometric pressure, April mean temperature, September mean temperature, annual mean temperature, April wind speed, September wind speed, annual mean wind speed) were downloaded from WorldClim (https://worldclim.org/) and extracted using ArcGIS Pro.
The spatial factors were extracted based on the original geographic coordinates of the 27 point locations, which were first converted to Cartesian coordinates using the SoDA package for R. Subsequently, seven significant spatial variables (MEM1, MEM2, MEM3, MEM4, MEM5, MEM6, and MEM7) were extracted using the adespatial package. Spatial variables with low eigenvalues characterize large-scale spatial patterns, while spatial variables with high eigenvalues characterize fine-scale spatial patterns.
Before analyzing the data, we used the “vif” function from the car package to check for multicollinearity and retained only variables with a VIF less than 5. The macroinvertebrate community data were first transformed into Hellinger’s lattice using the “decostand” function. Following this, the “forward.sel” function was used to forward select the significant water quality, land use, natural climate, and spatial contribution factors based on RDA analysis. Only significant variables were retained. The “varpart” function was then used to decompose the variance, and the variance contributions of all factors to the macroinvertebrate community data were calculated.
To identify specific drivers affecting macroinvertebrate communities, we separately analyzed water quality, land use, physical geography, and spatial factors using the “rdacca.hp” function. To further characterize the direct and indirect effects of spatial, aquatic environmental, geoclimatic, and land use factors on macroinvertebrate communities, we first calculated macroinvertebrate alpha diversity and beta diversity using the diversity function from the vegan package and the LCBD.comp function from the adespatial package, respectively. Subsequently, partial least squares path modeling (PLSPM) was used to causally model the drivers of macroinvertebrate diversity and to identify the relationships between each latent variable (spatial, aquatic environment, geoclimatic, and land use factors) and the observed variables (alpha and beta diversity). All data analyses in this study were conducted using R version 4.3.2 (R Core Team, 2013).
A total of 96 species of macroinvertebrates at the family or genus level were identified in this study, including three phyla, 20 orders, and 43 families. Among them, Chironomidae had the largest number of species with 19, followed by Tubificidae with 8 species, and Unionidae with 7 species. Detailed data on macroinvertebrate species composition are provided in the Supplementary Document.
The significant water environment variables selected for RDA-based forward selection analysis were electrical conductivity (EC), permanganate index (CODMn), biochemical oxygen demand (BOD), and total phosphorus (TP). The significant spatial variables were MEM1, MEM2, MEM3, and MEM5. The significant land-use variables were forests and impervious surfaces, and the significant geo-climatic variables were April radiation, September mean temperature, and longitude. Variance decomposition results showed that environmental, spatial, land-use, and geo-climatic factors collectively explained 22.86% of the variance in the macroinvertebrate community, leaving 77.14% of the variance unexplained (Figure 2). Specifically, the spatial factor had the largest pure contribution (0.020) to macroinvertebrate community variance. The shared effect explained by environmental, spatial, and geo-climatic factors was the largest (0.073), followed by the shared effect of spatial and geo-climatic factors (0.068).
Figure 2. Variance decomposition of macroinvertebrate communities by water environment (env), land use (land), natural geography (nat), and spatial action (spa).
The results of the hierarchical partitioning showed (Figure 3) that among the water environmental factors, the permanganate index (CODMn) had the strongest sole effect on macroinvertebrates, followed by biochemical oxygen demand (BOD), total phosphorus (TP) and electrical conductivity (EC). Among the spatial factors, MEM1 had the strongest unique effect, followed by MEM3 and MEM5. Within the land use factors, forest had the strongest unique effect, followed by impervious surfaces. Among the geoclimatic factors, longitude had the strongest unique effect, followed by the mean temperature in September and April radiation.
Figure 3. Hierarchical partitioning of macroinvertebrate communities was conducted based on water environment, spatial factors, geoclimate, and land use.
PLS-PM analyses showed that the model had a GOF of 0.55, indicating that the results were reliable. The variance explained by the model for macroinvertebrate alpha diversity and beta diversity was 0.44 and 0.65, respectively, suggesting that the latent variables accounted for most of the variation in macroinvertebrate diversity. Specifically, alpha diversity was directly influenced by spatial factors, geoclimatic factors, and aquatic environments (standardized path coefficients: -1.33, 0.73, and 0.72, respectively). Beta diversity was directly influenced by macroinvertebrate alpha diversity (standardized path coefficient: -0.92) (Figure 4).
Figure 4. Shows the partial least squares path model, which explores the effects of spatial factors, geoclimatic factors, land-use factors, water-environmental factors, and macroinvertebrate community effects on macroinvertebrate community alpha and beta diversity. Blue and red lines indicate positive and negative significant relationships, respectively, while dashed lines represent non-significant relationships. The thickness of the lines indicates the strength of the causal relationships, supplemented by standardized path coefficients. Path coefficients are not shown for non-significant relationships. R² denotes the total variance of the dependent variable explained by the independent variables, and GOF denotes the goodness-of-fit of the entire model. *P < 0.05; **P < 0.01; ***P < 0.001.
The mechanisms of macroinvertebrate community formation have been a central topic for community ecologists, and the emergence of metacommunity theory has provided a framework for this research (Heino, 2013; Heino et al., 2015; Leibold et al., 2004). Based on this, we considered the relative roles of water environment, land use, geoclimatic, and spatial factors in the mechanisms of macroinvertebrate community assembly in the water bodies of eastern China and their impacts on diversity. We found that spatial factors contributed the largest pure effect on macroinvertebrate communities and the largest common effect explained by environmental, spatial, and geoclimatic factors. Alpha diversity was directly affected by spatial, geoclimatic, and aquatic environments (with standardized path coefficients of -1.33, 0.73, and 0.72, respectively), whereas beta diversity was directly affected by macroinvertebrate alpha diversity (with a standardized path coefficient of -0.92).
Unexpectedly, we found an overwhelming dominance of the spatial factor relative to the other variables, which contrasts with results from other riverine ecosystems where environmental filtering has often been found to play a dominant role (Firmiano et al., 2021; Li et al., 2021, Li et al., 2020). This discrepancy may be related to the large spatial extent of the study area, with a maximum distance between points of around 700 kilometers. Such a geographic distance leads to the formation of macroinvertebrate communities with a diffusion-limited spatial structure (Shurin et al., 2009). Additionally, the presence of hydroelectric power plants in the study area may reduce water body connectivity and create barriers to organism dispersal (Wang et al., 2013). Despite the geographic spacing, more than half of our points are located in the main stem of the watershed, and according to the Network Position Hypothesis (NPH) (Schmera et al., 2018), they should be more closely connected to each other. In terms of community composition, the macroinvertebrate community in the study area is dominated by Chironomidae, Oligochaetes, and Mollusca. Except for Chironomidae, which has limited terrestrial dispersal ability, the other taxa are strictly terrestrial dispersers with limited dispersal capacity, subjecting them to greater dispersal constraints over large spatial scales (Heino et al., 2015).
In addition to this, the significant effects of regional variables (land use, geoclimatic) on macroinvertebrate communities are similar to those found in other freshwater ecosystems (Firmiano et al., 2021; Van Looy et al., 2017; Wu et al., 2022). Regional variables, although not directly affecting organisms like the aquatic environment, can indirectly change community composition by influencing stressors (Van Looy et al., 2017). For example, the shift from forests to intensive land use, such as farmland and urban areas, due to anthropogenic impacts, has led to poor water quality (Uriarte et al., 2011). In this study, forested and impervious surfaces were found to be the most important, representing two very different habitats—one in a natural state and the other under high anthropogenic disturbance, resulting in distinct macroinvertebrate communities (Wang et al., 2012). Rainfall leads to surface runoff that carries sediment and suspended matter, significantly impacting the hydrological situation (Fang et al., 2012). Air temperature and radiation indirectly affect benthic communities by altering water temperature (Kelly et al., 2003). The geographic variable longitude indirectly reflects the influence of climate on benthic communities, as different longitudes correspond to different climate types. This reflects the significant influence of geographic location and climatic factors on macroinvertebrate communities, as they relate to the aquatic environment. For example, there are significant differences in the physicochemical factors of the aquatic environment at different longitudes, and the effects of air temperature on water temperature and radiation on photosynthesis.
The water environment has less explanatory power than space, land use, and geoclimate. This may be related to the dispersal capacity of species, as environmental filtration of dominant community change presupposes that the dispersal capacity of species is moderate, allowing them to respond to changes in environmental gradients (Loeuille and Leibold, 2008). Whereas the taxa in this study were predominantly mollusks and oligochaetes, and insects were dominated by Chironomidae, most of these taxa had either no active dispersal capacity or weak dispersal capacity (Sarremejane et al., 2017). Among the water environment variables, the permanganate index (CODMn), biochemical oxygen demand (BOD), total phosphorus (TP), and electrical conductivity (EC) were the significant factors affecting the macroinvertebrate community, consistent with findings from most studied riverine ecosystems (Luo et al., 2018; Qin et al., 2022; Tampo et al., 2021). Electrical conductivity (EC) reflects the salinity of a water body, and excessive conductivity can negatively impact macroinvertebrates. Higher biochemical oxygen demand (BOD), permanganate index (CODMn), and total phosphorus (TP) reflect organic pollution and eutrophication associated with anthropogenic disturbances, which can lead to a decrease in the number of intolerant taxa and an increase in the number of tolerant taxa (Friberg et al., 2010). One implication of this metacommunity-based study is the necessity to account for and potentially exclude the effects of spatial processes and natural disturbances on biological communities in bioassessment to accurately reflect the impacts of human disturbances (Birk et al., 2012; Poikane et al., 2016).
Path analysis based on PLS-PM identifies direct and indirect effects on macroinvertebrate community diversity. We found significant positive effects of spatial factors on water environment, land use, and geoclimatic factors, suggesting that these variables are spatially structured; i.e., spatial distances increase environmental differences between sites, thereby further influencing macroinvertebrate communities (Wan et al., 2015). We also found significant negative effects of spatial factors on alpha diversity, suggesting that dispersal constraints limit the movement of species between sites, thereby reducing native species diversity (Nislow et al., 2011). In contrast, there were significant positive effects of water environment and geoclimate on communities, reflecting the significant power of environmental filtering on species diversity (Cunha et al., 2019). For beta diversity, these three drivers indirectly negatively affected LCBD values, mainly through alpha diversity. Therefore, it is foreseeable that spatial variables should be the main focus of the region in terms of biodiversity conservation, followed by geoclimatic and hydroenvironmental factors. Alpha diversity’s significant negative effect on LCBD suggests lower LCBD values at sites with high alpha diversity, which aligns with the majority of studies (da Silva et al., 2018; Legendre and De Cáceres, 2013). This is because sites with many common dominant species have high alpha diversity but low contribution to beta diversity (i.e., low LCBD), whereas sites with rare species have low alpha diversity but high contribution to beta diversity (i.e., high LCBD).
Overall, we found that spatial processes significantly affect macroinvertebrate communities and their diversity, potentially masking the role of environmental filtration. Dispersal constraints can prevent organisms from responding to changes along parts of the environmental gradient because they cannot reach all sites (Heino et al., 2015). Therefore, focusing on habitat integrity and hydrological conditions at sites may be a better next step. Improving spatial connectivity could increase linkages between sites and thus reduce the effects of dispersal limitations on communities. Simultaneously, for biological assessments, adjusting evaluation indicators to identify those less affected by spatial processes and natural disturbances, and more relevant to the aquatic environment, could improve the accuracy of the evaluations.
Studies based on metacommunity theory can enhance our understanding of the ecological processes influencing biological communities. In this paper, we assessed the macroinvertebrate metacommunity structure and drivers of biodiversity in water bodies in eastern China using variance decomposition, hierarchical partitioning, and PLS-PM modeling methods. The results showed that spatial processes dominated the macroinvertebrate metacommunity structure. Additionally, land use, water environment, and geoclimatic factors significantly influenced macroinvertebrate community changes. Further analysis revealed that spatial processes, water environment, and geoclimate significantly affected macroinvertebrate alpha diversity and indirectly influenced beta diversity through alpha diversity. These findings emphasize the importance of considering spatial processes and natural disturbances in biological assessments.
The raw data supporting the conclusions of this article will be made available by the authors, without undue reservation.
BZ: Conceptualization, Methodology, Writing – original draft, Writing – review & editing. ST: Data curation, Methodology, Writing – review & editing. BS: Data curation, Methodology, Writing – review & editing. XG: Investigation, Writing – review & editing. WH: Data curation, Writing – review & editing. BW: Investigation, Writing – review & editing. SH: Investigation, Writing – review & editing. ZL: Conceptualization, Data curation, Writing – review & editing.
The author(s) declare financial support was received for the research, authorship, and/or publication of this article. The Yangtze River Joint Research Phase II Program (2022-LHYJ-02-0102)
We would also like to express our gratitude to the editors at Frontiers and the two reviewers for their valuable feedback, it is through your comments that we were able to improve the quality of the manuscript.
The authors declare that the research was conducted in the absence of any commercial or financial relationships that could be construed as a potential conflict of interest.
All claims expressed in this article are solely those of the authors and do not necessarily represent those of their affiliated organizations, or those of the publisher, the editors and the reviewers. Any product that may be evaluated in this article, or claim that may be made by its manufacturer, is not guaranteed or endorsed by the publisher.
The Supplementary Material for this article can be found online at: https://www.frontiersin.org/articles/10.3389/fevo.2024.1448842/full#supplementary-material
Baselga A. (2010). Partitioning the turnover and nestedness components of beta diversity. Global Ecol. Biogeography 19, 134–143. doi: 10.1111/j.1466-8238.2009.00490.x
Birk S., Bonne W., Borja A., Brucet S., Courrat A., Poikane S., et al. (2012). Three hundred ways to assess Europe’s surface waters: An almost complete overview of biological methods to implement the Water Framework Directive. Ecol. Indic. 18, 31–41. doi: 10.1016/j.ecolind.2011.10.009
Cai Y., Xu H., Vilmi A., Tolonen K. T., Tang X., Qin B., et al. (2017). Relative roles of spatial processes, natural factors and anthropogenic stressors in structuring a lake macroinvertebrate metacommunity. Sci. Total Environ. 601-602, 1702–1711. doi: 10.1016/j.scitotenv.2017.05.264
Chase J. M., Jeliazkov A., Ladouceur E., Viana D. S. (2020). Biodiversity conservation through the lens of metacommunity ecology. Ann. New York Acad. Sci. 1469, 86–104. doi: 10.1111/nyas.14378
Chen Y., Xu R., Jia S., Shang G., Ding S., Gao X., et al. (2022). Preliminary study on water ecology evaluation indicators in the yangtze river basin. China Environ. Monit. 38, 45–57. doi: 10.19316/j.issn.1002-6002.2022.01.05
Cunha E. R., Winemiller K. O., da Silva J. C. B., Lopes T. M., Gomes L. C., Thomaz S. M., et al. (2019). [amp]]alpha; and β diversity of fishes in relation to a gradient of habitat structural complexity supports the role of environmental filtering in community assembly. Aquat. Sci. 81, 38. doi: 10.1007/s00027-019-0634-3
da Silva P. G., Hernández M. I. M., Heino J. (2018). Disentangling the correlates of species and site contributions to beta diversity in dung beetle assemblages. Diversity Distributions 24, 1674–1686. doi: 10.1111/ddi.12785
Fang N. F., Shi Z. H., Li L., Guo Z. L., Liu Q. J., Ai L. (2012). The effects of rainfall regimes and land use changes on runoff and soil loss in a small mountainous watershed. Catena 99, 1–8. doi: 10.1016/j.catena.2012.07.004
Firmiano K. R., Cañedo-Argüelles M., Gutiérrez-Cánovas C., Macedo D. R., Linares M. S., Bonada N., et al. (2021). Land use and local environment affect macroinvertebrate metacommunity organization in Neotropical stream networks. J. Biogeography 48, 479–491. doi: 10.1111/jbi.14020
Friberg N., Skriver J., Larsen S. E., Pedersen M. L., Buffagni A. (2010). Stream macroinvertebrate occurrence along gradients in organic pollution and eutrophication. Freshw. Biol. 55, 1405–1419. doi: 10.1111/j.1365-2427.2008.02164.x
Hawkins C. P., Mykrä H., Oksanen J., Vander Laan J. J. (2015). Environmental disturbance can increase beta diversity of stream macroinvertebrate assemblages. Global Ecol. Biogeography 24, 483–494. doi: 10.1111/geb.12254
Heino J. (2013). The importance of metacommunity ecology for environmental assessment research in the freshwater realm. Biol. Rev. 88, 166–178. doi: 10.1111/j.1469-185X.2012.00244.x
Heino J., Melo A. S., Siqueira T., Soininen J., Valanko S., Bini L. M. (2015). Metacommunity organisation, spatial extent and dispersal in aquatic systems: patterns, processes and prospects. Freshw. Biol. 60, 845–869. doi: 10.1111/fwb.12533
Heino J., Soininen J., Alahuhta J., Lappalainen J., Virtanen R. (2017). Metacommunity ecology meets biogeography: effects of geographical region, spatial dynamics and environmental filtering on community structure in aquatic organisms. Oecologia 183, 121–137. doi: 10.1007/s00442-016-3750-y
Kelly D. J., Bothwell M. L., Schindler D. W. (2003). Effects of solar ultraviolet radiation on stream benthic communities: an intersite comparison. Ecology 84, 2724–2740. doi: 10.1890/02-0658
Legendre P., De Cáceres M. (2013). Beta diversity as the variance of community data: dissimilarity coefficients and partitioning. Ecol. Lett. 16, 951–963. doi: 10.1111/ele.12141
Leibold M. A., Holyoak M., Mouquet N., Amarasekare P., Chase J. M., Hoopes M. F., et al. (2004). The metacommunity concept: a framework for multi-scale community ecology. Ecol. Lett. 7, 601–613. doi: 10.1111/j.1461-0248.2004.00608.x
Li Z., Heino J., Song Z., Jiang X., Wang J., Liu Z., et al. (2021). Spatio-temporal variation of macroinvertebrate metacommunity organization in a monsoon-climate region. J. Biogeography 48, 3118–3130. doi: 10.1111/jbi.14270
Li Z., Jiang X., Wang J., Meng X., Heino J., Xie Z. (2019). Multiple facets of stream macroinvertebrate alpha diversity are driven by different ecological factors across an extensive altitudinal gradient. Ecol. Evol. 9, 1306–1322. doi: 10.1002/ece3.4841
Li Z., Xing Y., Liu Z., Chen X., Jiang X., Xie Z., et al. (2020). Seasonal changes in metacommunity assembly mechanisms of benthic macroinvertebrates in a subtropical river basin. Sci. Total Environ. 729, 139046. doi: 10.1016/j.scitotenv.2020.139046
Liu G., Lin Z., Qi X., Wang Y., Wang Y., Jiang W., et al. (2023). Environmental filtering, spatial processes and biotic interactions jointly shape different traits communities of stream macroinvertebrates. Front. Ecol. Evol. 11. doi: 10.3389/fevo.2023.1196296
Liu Y., Zhang W., Wang Y., Wang E. (1979). Freshwater molluscs of Chinese economic fauna (Beijing: Science Press).
Loeuille N., Leibold M. A. (2008). Evolution in metacommunities: on the relative importance of species sorting and monopolization in structuring communities. Am. Nat. 171, 788–799. doi: 10.1086/587745
Luo X., Guo Q., Cao J., Luo G. (2024). Remote sensing inversion of surface water turbidity and total nitrogen supported by measured data - A case study of the middle section of the Yangtze River Basin. China Environ. Monit. 40, 261–271. doi: 10.19316/j.issn.1002-6002.2024.04.27
Luo K., Hu X., He Q., Wu Z., Cheng H., Hu Z., et al. (2018). Impacts of rapid urbanization on the water quality and macroinvertebrate communities of streams: A case study in Liangjiang New Area, China. Sci. Total Environ. 621, 1601–1614. doi: 10.1016/j.scitotenv.2017.10.068
Merritt R. W., Cummins K. W. (1996). An introduction to the aquatic insects of north america (Dubuque, Iowa: Kendall/Hunt Publishing Company).
Moritz C., Meynard C. N., Devictor V., Guizien K., Labrune C., Guarini J.-M., et al. (2013). Disentangling the role of connectivity, environmental filtering, and spatial structure on metacommunity dynamics. Oikos 122, 1401–1410. doi: 10.1111/j.1600-0706.2013.00377.x
Morse J. C., Yang L., Tian L. (1994). Aquatic insects of China useful for monitoring water quality (Nanjing, China: Hohai University Press).
Nislow K. H., Hudy M., Letcher B. H., Smith E. P. (2011). Variation in local abundance and species richness of stream fishes in relation to dispersal barriers: implications for management and conservation. Freshw. Biol. 56, 2135–2144. doi: 10.1111/j.1365-2427.2011.02634.x
Oliveira C. F., do Valle Junior R. F., Valera C. A., Rodrigues V. S., Sanches Fernandes L. F., Pacheco F. A. L. (2019). The modeling of pasture conservation and of its impact on stream water quality using Partial Least Squares-Path Modeling. Sci. Total Environ. 697, 134081. doi: 10.1016/j.scitotenv.2019.134081
Perez Rocha M., Morris T. J., Cottenie K., Schwalb A. N. (2023). Limitations of beta diversity in conservation site selection. Ecol. Indic. 154, 110732. doi: 10.1016/j.ecolind.2023.110732
Poikane S., Johnson R. K., Sandin L., Schartau A. K., Solimini A. G., Urbanič G., et al. (2016). Benthic macroinvertebrates in lake ecological assessment: A review of methods, intercalibration and practical recommendations. Sci. Total Environ. 543, 123–134. doi: 10.1016/j.scitotenv.2015.11.021
Qin C., Ge Y., Gao J., Zhou S., Yu J., Wang B., et al. (2022). Ecological drivers of macroinvertebrate metacommunity assembly in a subtropical river basin in the Yangtze River Delta, China. Sci. Total Environ. 837, 155687. doi: 10.1016/j.scitotenv.2022.155687
R Core Team (2013). R: A language and environment for statistical computing. Vienna, Austria: Foundation for Statistical Computing.
Sarremejane R., Mykrä H., Bonada N., Aroviita J., Muotka T. (2017). Habitat connectivity and dispersal ability drive the assembly mechanisms of macroinvertebrate communities in river networks. Freshw. Biol. 62, 1073–1082. doi: 10.1111/fwb.12926
Schmera D., Árva D., Boda P., Bódis E., Bolgovics Á., Borics G., et al. (2018). Does isolation influence the relative role of environmental and dispersal-related processes in stream networks? An empirical test of the network position hypothesis using multiple taxa. Freshw. Biol. 63, 74–85. doi: 10.1111/fwb.12973
Shurin J. B., Cottenie K., Hillebrand H. (2009). Spatial autocorrelation and dispersal limitation in freshwater organisms. Oecologia 159, 151–159. doi: 10.1007/s00442-008-1174-z
Siqueira T., Bini L. M., Roque F. O., Marques Couceiro S. R., Trivinho-Strixino S., Cottenie K. (2012). Common and rare species respond to similar niche processes in macroinvertebrate metacommunities. Ecography 35, 183–192. doi: 10.1111/j.1600-0587.2011.06875.x
Soininen J., Heino J., Wang J. (2018). A meta-analysis of nestedness and turnover components of beta diversity across organisms and ecosystems. Global Ecol. Biogeography 27, 96–109. doi: 10.1111/geb.12660
Tampo L., Kaboré I., Alhassan E. H., Ouéda A., Bawa L. M., Djaneye-Boundjou G. (2021). Benthic macroinvertebrates as ecological indicators: their sensitivity to the water quality and human disturbances in a tropical river. Front. Water 3. doi: 10.3389/frwa.2021.662765
Thompson P. L., Guzman L. M., De Meester L., Horváth Z., Ptacnik R., Vanschoenwinkel B., et al. (2020). A process-based metacommunity framework linking local and regional scale community ecology. Ecol. Lett. 23, 1314–1329. doi: 10.1111/ele.13568
Uriarte M., Yackulic C. B., Lim Y., Arce-Nazario J. A. (2011). Influence of land use on water quality in a tropical landscape: a multi-scale analysis. Landscape Ecol. 26, 1151–1164. doi: 10.1007/s10980-011-9642-y
Van Looy K., Piffady J., Floury M. (2017). At what scale and extent environmental gradients and climatic changes influence stream invertebrate communities? Sci. Total Environ. 580, 34–42. doi: 10.1016/j.scitotenv.2016.12.009
Wan Y., Xu L., Hu J., Xu C., Wan A., An S., et al. (2015). The role of environmental and spatial processes in structuring stream macroinvertebrates communities in a large river basin. CLEAN – Soil Air Water 43, 1633–1639. doi: 10.1002/clen.201300861
Wang B., Liu D., Liu S., Zhang Y., Lu D., Wang L. (2012). Impacts of urbanization on stream habitats and macroinvertebrate communities in the tributaries of Qiangtang River, China. Hydrobiologia 680, 39–51. doi: 10.1007/s10750-011-0899-6
Wang J., Sheng Y., Gleason C. J., Wada Y. (2013). Downstream Yangtze River levels impacted by Three Gorges Dam. Environ. Res. Lett. 8, 44012. doi: 10.1088/1748-9326/8/4/044012
Wu N., Liu G., Zhang M., Wang Y., Peng W., Qu X. (2022). Spatial factors outperform local environmental and geo-climatic variables in structuring multiple facets of stream macroinvertebrates’ β-diversity. Animals 12, 2648. doi: 10.3390/ani12192648
Wu K., Zhao W., Li M., Picazo F., Soininen J., Shen J., et al. (2020). Taxonomic dependency of beta diversity components in benthic communities of bacteria, diatoms and chironomids along a water-depth gradient. Sci. Total Environ. 741, 140462. doi: 10.1016/j.scitotenv.2020.140462
Yang M., Xiong F., Lu Y., Liu H., Wang C., Chen L., et al. (2023). Analysis of benthic community patterns and driving factors of typical rivers and lakes in the source area of the Yangtze River during the period of abundant water. China Environ. Monit. 39, 11–20. doi: 10.19316/j.issn.1002-6002.2023.02.02
Keywords: metacommunities, variance partitioning, partial least squares path modeling, spatial processes, macroinvertebrates
Citation: Zheng B, Tian S, Sun B, Gao X, Han W, Wu B, Hu S and Li Z (2024) Spatial processes dominate the metacommunity structure and diversity of macroinvertebrates in the waters of eastern China. Front. Ecol. Evol. 12:1448842. doi: 10.3389/fevo.2024.1448842
Received: 14 June 2024; Accepted: 09 September 2024;
Published: 04 October 2024.
Edited by:
Mauro Fois, University of Cagliari, ItalyReviewed by:
Bianca Ramos Meira, State University of Maringá, BrazilCopyright © 2024 Zheng, Tian, Sun, Gao, Han, Wu, Hu and Li. This is an open-access article distributed under the terms of the Creative Commons Attribution License (CC BY). The use, distribution or reproduction in other forums is permitted, provided the original author(s) and the copyright owner(s) are credited and that the original publication in this journal is cited, in accordance with accepted academic practice. No use, distribution or reproduction is permitted which does not comply with these terms.
*Correspondence: Zhao Li, bGl6aGFvQGNuZW1jLmNu
Disclaimer: All claims expressed in this article are solely those of the authors and do not necessarily represent those of their affiliated organizations, or those of the publisher, the editors and the reviewers. Any product that may be evaluated in this article or claim that may be made by its manufacturer is not guaranteed or endorsed by the publisher.
Research integrity at Frontiers
Learn more about the work of our research integrity team to safeguard the quality of each article we publish.