- School of Economics and Management, Beijing Forestry University, Beijing, China
Introduction: In the search for sustainable development, urban eco-development is becoming a core agenda for all countries. China’s low-carbon city pilot (LCCP) policy is an important initiative to promote urban low-carbon governance. And exploring the direction of LCCP policy is an important step towards sustainable urban development.
Methods: Super-SBM is employed to calculate urban ecological efficiency using panel data from 254 prefecture-level cities between 2007 and 2020. The methods of kernel density estimation and spatial Markov chains are applied to the spatial analysis of urban ecological efficiency. The spatial analyses examine the spatiotemporal patterns and dynamic evolving trends of urban ecological efficiency. Additionally, the multiperiod difference-in-differences method is used to assess the impact of the LCCP policy on urban ecological efficiency. On this basis, we apply the method of mechanism analysis to discuss the influence mechanism.
Results: The results indicate that urban ecological efficiency gradually forms a continuous spatial clustering pattern, although there is a widening tendency in the absolute differences. In the subsequent transfer process, the spatial factor plays a highly significant role. Benchmark regressions and auxiliary robustness tests demonstrate that the LCCP policy is effective in improving urban ecological efficiency. Further analysis shows that the effect of LCCP policies on ecological efficiency can be transmitted by promoting green technology innovation as well as facilitating a low-carbon transition in the energy mix. The above findings indicate the presence of the "Green Innovation Effect" and the "Decoupling Effect".
Discussion: These discoveries enhance the theoretical framework of urban ecology and offer valuable insights for other regions seeking to implement low-carbon urban governance.
1 Introduction
A thriving and flourishing ecological environment is crucial for urban sustainable development. Effective environmental systems form the foundation and core driving force for this development (Shen et al., 2023a). With the energy crisis deepening, the frequency of extreme meteorological events increasing, and biodiversity loss escalating, the pursuit of ecological wellbeing has recently become a priority in international policy discussions (Ramzan et al., 2023). In developing countries, weather-related economic losses are nearing $35 billion per year (Wen et al., 2024a). In China, the heavy industry and manufacturing-focused development strategy adopted after the reform and opening up has resulted in significant urban pollution and ecological damage (Wang and Wang, 2023). Solutions in policy, technology, and resource management are needed to address these issues. The creation of high-quality urban ecosystems has become a global policy consensus (Xu et al., 2022). Therefore, accelerating the ecological development of cities and exploring ways to implement the concept of ecological civilization is one of today’s most urgent and practical challenges.
Cities play an important role in addressing environmental problems caused by excessive carbon emissions, which are an important module in the development of low-carbon governance. In the process of rapid urbanization, industrialization has become the driving force behind human agglomeration activities (Wu et al., 2024). Being one of the countries with rich population agglomeration activities and rapid urbanization, China has aimed to peak carbon emissions by 2030 and achieve carbon neutrality by 2060. In 2009, China first proposed integrating “low-carbon city” concepts with “ecological city” ideals, introducing the concept of a low-carbon urban environment. Building on early successes, the National Development and Reform Commission (NDRC) launched the low-carbon city pilot (LCCP) in 2010, with subsequent strategic policy phases in 2012 and 2017. These policies aim to enhance energy efficiency, foster low-carbon industries, allocate resources with ecological considerations, and establish a sustainable roadmap amid rapid urbanization (Cheng et al., 2019). What remains is to consolidate this experimental framework with urban low-carbon governance and ecological development, advancing existing theories to address pressing environmental imperatives. It is therefore of great interest to study whether China’s low-carbon city policies have been effective in promoting low-carbon governance and solving environmental problems and whether their experience can provide references and lessons for sustainable development in other regions and countries.
To begin the review of efficiency evolution within the framework of urban ecology theory, it is essential first to outline urban ecological efficiency. This concept has been proposed and has been studied more extensively in the existing literature. This concept aims to establish the appropriate balance needed to synergize economic growth with resource utilization while mitigating negative impacts on the environment (Wen et al., 2024b). Emphasis is placed on achieving sustainable development across economic, social, and environmental spheres (Sun et al., 2019; Yasmeen et al., 2020). In this pursuit, this study has traced back to a host of techniques developed over the years to measure ecological efficiency. Some of these include the alternative indicator approach (Wang et al., 2015), multilevel analysis (Gao et al., 2018), and the DPSIR model (Sobhani et al., 2023), among others. The DEA technique stands out for efficiency appraisal, as it can perform the appraisal without using specific functional form structures, especially in cases involving multi-input evaluations. For instance, Wang et al. (2020) examined the detrimental effects of corruption and resource misalignments on ecological efficiency through a three-factor model. Additionally, Dong et al. (2020) utilized the ecological footprint model as input into the environmental efficiency measurement model using data envelopment analysis to depict the spatiotemporal characteristics of ecological efficiency among 30 Chinese provinces. Zhao et al. (2020) applied the super efficiency slack-based model to measure the ecological efficiency of 286 Chinese cities at a prefecture level, considering industrial structure distortions to explore possible causal relationships. The latter is discussed further to provide strong support for the presented ecological efficiency measurement model in current studies.
Optimizing the urban ecosystem is a multidimensional and comprehensive issue, encompassing sustainable land use planning (Das et al., 2023), enhancement of urban innovation dynamics (Li et al., 2021), and increasing electricity supply (Fan et al., 2021), among others. Creating low-carbon cities has become a crucial task worldwide for addressing environmental issues and tackling the climate crisis (Haarstad, 2016; Holtz et al., 2018). For instance, in China, Zhao et al. (2023b) identified characteristic CO2 emission features of urban clusters and developed collective programs to reduce emissions based on this information. Cheng et al. (2019) employed difference-in-differences analysis to examine the relationship between low-carbon city construction and the greening of city economies. Chen et al. (2023a) explored urban sustainable development through the reconceptualization of LCCP policies. Zhao et al. (2023a) analyzed urban carbon lock-in attributes and considered potential critical impacts of variations in LCCP policy. A synthesis of these studies suggests that achieving a high degree of ecological efficiency is a prominent outcome of urban low-carbon governance.
By applying the super-efficiency SBM method, we establish an ecological efficiency analysis index system and design a quasi-natural experiment based on the pilot low-carbon city policy proposed by the Chinese government in 2010. At the same time, this study also handles carbon dioxide emission data to better fit the connotation of eco-efficiency indicators. This study empirically investigates whether urban low-carbon governance can effectively improve eco-efficiency by utilizing panel data from 254 Chinese cities. This study also utilizes a combination of kernel density estimation and spatial Markov chain methods to portray the dynamic evolutionary trend of eco-efficiency. In addition, we investigate the mechanisms by which low-carbon governance affects eco-efficiency.
This study is anticipated to contribute to the existing literature in three key ways. Firstly, it introduces ecological outcomes and establishes a framework for assessing ecological efficiency, encompassing 11 indicators of input and output. This approach offers a more nuanced definition of urban ecological efficiency, thereby enhancing the applicability of existing theories. Secondly, the study analyzes a sample of 254 Chinese prefecture-level cities to examine the policy effects of urban low-carbon governance from the municipalities’ perspective. Robustness tests were conducted to ensure the reliability of the core findings. Finally, the paper investigates green technological innovation and energy structure as two mechanism variables that influence the relationship between low-carbon city policies and ecological efficiency, aiming to understand their intermediary effects. Regional variations in the sample were also considered to observe the diverse impacts of LCCP policies across different geographic locations, including urban clusters, and resource endowments.
The remainder of this paper is organized as follows. Section 2 provides a critical review of the literature, focusing on the LCCP policy. In Section 3, the theoretical framework of this investigation is elaborated in response to the policy background of the LCCP. Section 4 details the research methodology, including the variables used and data acquisition methods. Section 5 discusses the geographical distribution and dynamic characteristics of ecological efficiency. Finally, Section 6 explores studies on the causal relationship between the LCCP policy and ecological efficiency. Section 7 explores the mechanisms by which both green technology innovations and the energy mix are affected, and demonstrates the heterogeneity of policy impacts. The final section of the paper, Section 8, is named “Conclusions and policy implications.”
2 Literature review and theoretical analysis
Most of the existing literature suggests that urban low-carbon governance improves ecological efficiency mainly by promoting technological innovation and optimizing energy structure. For instance, Du et al. (2022) indicated that China’s LCCP policy could impact urban ecological construction by reducing carbon emissions and improving infrastructure. Song et al. (2020) explored the influencing mechanism of technological innovation paths but did not definitively confirm the energy efficiency transmission channel. Hou et al. (2023) demonstrated the driving role of environmental regulatory policies and financial incentives in urban low-carbon governance and urban ecological construction. Lan et al. (2023) analyzed the operational mechanism of urban functions in low-carbon cities and carbon emission reduction using functional data from 178 cities in China. In general, we find that the LCCP policy has increased urban ecological supply by adjusting industrial structures, implementing financial incentives, improving energy efficiency, and promoting urban innovation. However, it remains questionable whether the policy will continue to increase urban ecological supply through technological innovation and energy structure. Therefore, this paper addresses both aspects in more detail.
2.1 Green technology innovation and ecological efficiency
On the one hand, urban technological innovation does not necessarily benefit the natural environment (Zhao et al., 2023d; Zhou et al., 2022). The mismatch of factors may lead to technological innovation that ignores environmental costs, thereby supporting the “pollution paradise hypothesis.” Technological innovation and economic freedom can sometimes pose significant obstacles to improving urban eco-quality (Sakariyahu et al., 2023). This is why the focus on ecological cost-oriented green technology innovation has been chosen. Green technology innovation can be defined as a technological concept that avoids harmful environmental consequences and aligns with natural ecosystems to mitigate the adverse impacts of invasive ecological characteristics (Zhang et al., 2023). It typically includes pollution inhibition, abatement technology, recycling technology, ecological process technology, and similar approaches (Xu et al., 2023). Ozkan et al. (2023) further reveal in their study that the long-term impact of GTI on CEMS in Chinese cities is positive, using autoregressive distributed lag simulation in a dynamic framework. Danish et al. (2023) also demonstrate that simulating environmentally friendly technological innovation can help smooth policy effects. In conclusion, green technology innovation appears more suitable for the LCCP policy, fostering a pattern of green innovative growth (Stucki and Woerter, 2017).
2.2 Energy structure and ecological efficiency
It remains to be verified whether low-carbon urban governance can effectively detach from economic development and energy use by altering the energy structure to achieve the “decoupling effect.” Another crucial point is the predominance of research focused on explaining the formation mechanism of the relationship between low-carbon governance and energy efficiency. For example, Wang et al. (2023a) suggest that the enhancement of the low-carbon governance effect on energy efficiency in cities is delayed. Additionally, Shen et al. (2023b) established the causality of the LCCP policy concerning the energy transition index in their research. Yang et al. (2023a) found that while the LCCP policy can significantly reduce energy efficiency in industries, this is not always the case. Nevertheless, much attention is not given to the energy structure in the city level and thus further empirical research may be immensely useful to this relationship.
2.3 Research gaps
Through the literature review, we find the following research gaps in past studies. First, there are few works exploring the prosperity of ecological output, and most scholars tend to define ecological efficiency as merely the correlation between economic development and the consumption of resources, which in return, overlooks the need to build up ecology. Second, due to the limitation of the definition of urban ecological efficiency in previous studies, it is necessary to explore whether urban low-carbon governance can still promote ecological efficiency with richer connotations. Moreover, the existing literature mostly conducted research from the level of city agglomerations and provinces, which makes it difficult to provide pilot experiences for city-level sample units. Third, in our opinion, more solid data are required to verify the mechanism of IT and energy structure in the context of LCCP policy as well as ecological efficiency.
3 Policy background and research hypotheses
3.1 Policy background
The concept of “low carbon” is a relatively new paradigm of this century, emerging in response to escalating global warming and energy crises. The term “low-carbon city” was initially introduced by the UK in 2003 and formally included in the Energy White Paper. Encouraged by market trends and bolstered by substantial logistical support from the central government, this concept aims to promote the widespread adoption of low-carbon technologies across the industry and construction sectors. Its primary goal is to foster economic preparedness and establish a sustainable economic structure. In 2010, China’s NDRC issued the “Notice on the Pilot Work of Low-Carbon Provinces, Regions, and Cities,” marking the beginning of efforts to reshape the traditional urbanization model and lead the way in low-carbon industrial development. The initial phase focused on pilot cities such as Tianjin, Chongqing, and Shenzhen. Building on the success of these initial pilots, NDRC expanded the program nationwide, garnering positive responses from 2 provinces and 37 cities in subsequent rounds of policy implementation. In 2017, NDRC reaffirmed its commitment to low-carbon development with the “Notice on the Pilot Work of Low-Carbon Communities,” underscoring the nation’s dedication to sustainable development and the integration of low-carbon principles into everyday life.
Experiences from various cities have demonstrated that the transformation to low-carbon cities is a complex and systematic endeavor, encompassing all urban elements, territories, and progress (Lin et al., 2022a). This strategic undertaking demands meticulous planning, coordination, and harmonization. In China, the objective is to achieve high-quality modernization and development guided by a low-carbon economic framework.
3.2 Research hypotheses
In essence, Figure 1 presents a conceptual map of one of the most crucial instruments in research aimed at reducing carbon emissions. Initially, the LCCP policy focuses on developing and enhancing low-carbon growth-related policies. It aims to restructure industries to significantly reduce carbon emissions. Simultaneously, the policy supports research into managing carbon emissions operationally.
At the governmental level, low-carbon cities encourage local governments to shift from pursuing “economic championships” to prioritizing “ecological performance.” They develop corresponding low-carbon development plans and formulate policies that support low-carbon initiatives. Effective incentives guide resources toward environmentally friendly industries (Wang et al., 2023b). At the corporate level, the LCCP enhances existing procedures while advancing low-carbon technologies. It provides incentives for firms to adopt these technologies and optimizes industrial frameworks, integrating cleaner, innovative manufacturing and service sectors. These measures are outlined by Zhao et al. (2023c).
Degrees of influence on different economic and social bodies: At the community level, the state advocates for low-carbon consumption patterns and lifestyles. It promotes the use of low-carbon products and encourages the adoption of low-carbon living (Zhang and Zheng, 2023). From this perspective, it delves deeply into various aspects of economic society while addressing the relationship between energy consumption and economic development, aiming to construct a new urban ecological civilization (Wang et al., 2022). In this regard, the paper advances the following hypothesis:
H1: The LCCP policy can significantly promote urban ecological efficiency.
Moreover, as indicated in the literature review section, there is a lack of adequate theoretical evidence regarding the mechanisms linking green technology innovation and energy structure. The LCCP is essentially a government-led formal environmental regulation. From the perspective of neoclassical economics, the LCCP promotes the adoption of low-carbon technologies to achieve program initiatives, compelling firms to engage in R&D focused on these technologies. This stimulation fosters their expansion into sectors such as energy conservation, environmental protection, and new energy sources, positioning them as burgeoning strategic industries. From a market demand perspective, LCCP policies create investment opportunities in “low-carbon and emission reduction” initiatives, incentivizing enterprises to adopt and develop green technologies (Jie and Jiahui, 2023). Simultaneously, these policies impose stricter admission criteria on high-emission and high-pollution industries, compelling them to adjust their business strategies. Porter’s hypothesis also suggests that appropriate environmental regulations can stimulate technological innovation (Feng et al., 2023). On the supply side, the gradual enhancement of supportive policies and infrastructure reduces development costs and investment risks. Incentive implementations further encourage market favorability toward technologies and concepts with promising prospects (Wang and Deng, 2021). Based on this, we propose the following hypothesis:
H2a: The LCCP policy can increase urban ecological efficiency by encouraging green technology innovation and producing the “green innovation effect.”
The past growth path of China has involved extensive energy use and considerable emissions, creating significant challenges as urban infrastructures advance and resulting in considerable carbon lock-in, also known as “high-carbon lock-in” (Chen et al., 2023b). The LCCP blueprint aims to break the “strong linkage” pattern that connects economic growth with increased resource consumption. Instead, it seeks to reshape the pattern of economic growth by imposing “ecological constraints” on market agents. From the supply side, it will motivate firms to increase investment in R&D, supported by increased incentives and rewards (Zhang et al., 2023). From the perspective of the social identity theory and the social pressure theory, the LCCP policy will have a demonstration effect and a regulatory effect on urban residents. Individuals will be empowered to enhance energy efficiency through new concepts such as green travel, low-carbon commuting, and sustainable living. On the demand side, the pilot policy of phasing out outdated industries imposes higher environmental costs on high-emitting and energy-intensive firms. Fluidity among these factors will also provide a pathway for firms with higher efficiency in clean production to capture a larger market share (Yin et al., 2023). In other words, the hypothesis can be stated as:
H2b: The LCCP policy can promote urban ecological efficiency by reducing energy structure, thus exerting the “decoupling effect.”
4 Materials and methods
4.1 Model specification
4.1.1 Benchmark model
It has become particularly useful in empirical policy evaluation, as previously mentioned when describing the difference-in-differences (DID) approach. Essentially, this method attempts to analyze the comparison between individuals before encountering a policy and after being subjected to it, allowing deduction of the policy’s effects on individuals (Callaway and Sant’Anna, 2018). This analysis depends on the variation between these two times, which can effectively offset biases resulting from variations in endogenous variables. For instance, China initiated the LCCP policy in 2010, subsequently extending it in 2012 and 2017. Currently, the NDRC has announced the names of cities participating in three rounds of pilot projects. Therefore, following the specifications of this paper, the policy is considered a quasi-natural experiment, and a multiperiod DID method is employed to analyze its outcomes.
One of the city-level indicators for identifying the policy’s inception is the date when a city first attempts to launch pilot projects. Indeed, in some cities, first-, second-, and third-stage pilot experiments were conducted. Furthermore, if an entire province is designated as a pilot low-carbon area, then all cities related to that province are considered to have adopted the low-carbon city policy. The overall sample under investigation includes panel data from 254 cities spanning from 2007 to 2020, with 112 cities in the treatment group and 142 cities in the control group.
Based on the above analysis, this paper establishes a benchmark regression model to examine changes in ecological efficiency resulting from the LCCP policy, incorporating individual and time fixed effects. The model is structured as follows:
Here, represents urban ecological efficiency index. is the cross-multiplier term of the pilot policy and the pilot timing. If city i conducts the LCCP policy in year t, is equal to 1 in year t and subsequent years; otherwise, it will be equal to 0; is the controlling variables, as described in detail in the variable description below; and represent individual fixed effect and time fixed effect, respectively. is a stochastic disturbance term.
4.1.2 Mediating effect model
Our hypothesis goes on to state that the LCCP policy should boost urban ecological efficiency further by innovating opportunities for green technology and lowering the linkage between economic growth and energy use. We test this hypothesis using the mediating effect model:
In this paper, we respectively regard and as the mediating variables of green technology innovation and energy structure. The meanings of other variables are consistent with the baseline model. Equation 1 estimates the total effect ; Equation 2 estimates the allocation effect ; and Equation 3 estimates the direct effect and indirect effects .
In addition to traditional tests, this research employs other methods to evaluate the validity of the proposed mediating effect. One such method is the Sobel test, which assumes that the parameter of interest is normally distributed—a condition that is often unrealistic in practical applications. Therefore, this study also utilizes the bootstrap approach, as recommended by Preacher and Hayes (2008). According to this method, if the confidence interval based on bootstrapping does not include 0, the mediating effect can be considered statistically significant.
4.2 Variables
4.2.1 Explained variable: ecological efficiency
The explained variable of this paper is ecological efficiency (EE). We construct the ecological efficiency evaluation index system based on previous research (He and Hu, 2022; Lin et al., 2022b; Wu et al., 2022; Zhang and Liu, 2021). First, we select 11 input and output indicators, considering energy, land, labor, capital, and other factors as the core input indicators. The desirable output indicators are regional GDP and green coverage of building-up area. Secondly, since carbon emission has become the considerable issue in the field of ecological environment (Li et al., 2023b), we try to incorporate CO2 emissions into the index system of ecological efficiency evaluation. Finally, we select CO2 emissions, industrial waste gas, industrial wastewater, and industrial smoke and dust emission as undesirable output indicators. The specific indicator system is shown in Table 1.
The primary issue with computing the ecological efficiency metric in both the radial or angular CCR and BBC models lies in their significant limitations. Unintended outputs are incorporated into the super-SBM model, which is a non-radial and non-angular model developed by Tone to compute ecological efficiency. The computational steps are as follows: Assume there are n1 decision-making units (DMUs), each with n inputs. Let s1 represent the desired output and s2 the undesired output. The objective function, , defines the super-SBM model as follows:
Here, is a weight vector, when , it indicates that the decision unit is in an ineffective state; when , it means that the input remains unchanged. At this time, reducing or maintaining output can achieve an effective state.
4.2.2 Core explanatory variable
The primary explanatory variable in this paper is the dummy variable for the LCCP policy. This paper focuses on the coefficient of the LCCP policy, which impacts urban ecological efficiency. Positive coefficients indicate a promotion effect, while negative coefficients indicate an inhibitory effect.
4.2.3 Control variables
Based on the previous research foundation of scholars (Ahmad and Wu, 2022; Hou et al., 2022; Su et al., 2021; Yin et al., 2022), the control variables are selected as follows:
1. Level of economic development (pgdp): This refers to the per capita gross regional product, established for the population of a given region. A higher level of economic development typically entails greater resource usage. However, according to the environmental Kuznets curve, economic development can enhance environmental quality and ecological balance once per capita income surpasses a “critical point” (Niu et al., 2024).
2. Size of local finance (gov): This is determined by local government general budget expenditures as a percentage of GDP. The extent of government influence can determine the degree of governmental interference in the distribution of regional resources. On one hand, the “visible hand” of the government can effectively implement strategies for ecological protection and green development. On the other hand, “GDP worship” may cause the government to neglect ecological protection and lead the market toward “profit competition” (Zhang et al., 2024).
3. Degree of fiscal decentralization (fid): This can be measured by the relationship between local government’s own-source revenues and total expenditures. Greater fiscal space allows local governments to promote inclusiveness and foster sustainable development through improved renewable energy resources (Sun et al., 2023).
4. Financial development (fdp) levels are measured by the ratio of financial intermediaries’ credit aggregates to GDP. This metric indicates the efficiency of resource allocation, particularly in direct investments and the rapid implementation of related policies and decisions. However, this can lead to inefficient resource allocation favoring resource-based sectors, potentially increasing ecological and environmental degradation (Ahmad and Wu, 2022).
5. The security of a society, especially in terms of social welfare, is often assessed by the proportion of the government’s budget allocated to social security and employment-related spending (social). A robust social security system acts as a buffer, ensuring the smooth operation of a market economy. This not only enhances the overall wellbeing of the population but also encourages greener production methods and lifestyles among citizens (Brendler, 2022). However, a highly developed social security network can have a drawback; a densely constructed social network may influence people to engage in environmentally adverse activities, which contradicts the goal of improving urban environmental quality.
Of these variables, we drop the natural logarithm of the level of economic development into the variable lnpgdp for analysis below.
4.2.4 Mechanism variables
In our exploration of the fundamental forces driving change, we have identified green technology innovation and energy intensity as critical variables. To measure the progress of urban green technology, we are tracking two key indicators: the annual count of eco-friendly inventions () and the number of green utility patents (). Recognizing that some cities may experience periods with no activity in these areas, leading to 0 values, we have refined our methodology to address this issue. To avoid division by 0 and better reflect the scale of innovation, we add one to the count and then take the logarithm. This approach allows us to capture a city’s innovative efforts in eco-technology even in the absence of documented green breakthroughs or patents.
As mentioned earlier, the low-carbon transition of the energy mix (ES) is the mechanism variable that is the focus of this paper. Among many economic indicators, we finally choose coal share as the variable to measure ES for theoretical and practical research reasons. As the main category of energy consumption in most regions, the utilization efficiency of coal directly reflects the urban energy structure. Moreover, with the continued global attention to sustainable development issues, reducing the use of fossil fuels such as coal has become an international consensus. The research of some scholars further supports the validity of this economic variable in economic analysis (Shen et al., 2024; Wang and Feng, 2021).
For the calculation of this indicator, Chinese prefecture-level cities have not yet published specific information on the consumption of all energy categories. We draw on the China Urban Statistical Yearbook and the study by Bie et al. (2024) to derive the share of coal by using all-society electricity consumption/(natural gas + liquefied petroleum gas + all-society electricity consumption).
4.3 Data sources and descriptive statistics
In this context, the material was collected from different official sources to compare the indicators of the ecological efficiency of cities. Based on the aspects of data collection, the main data sources are derived from the National Bureau of Statistics, including the China Statistical Yearbook, China Urban Statistical Yearbook, China Energy Statistical Yearbook, China Economic and Social Big Data Research Platform, and Wind Macroeconomic Database. To have a basic understanding of any missing information, the statistical yearbooks of the specific city can be referred to. In the same way, the CSMAR database provides information about the amount of green spaces in urban areas.
To follow the number of invention patents in urban green, we use the Sate Intellectual Property Office statistics. Technically, to define a patent as “green,” use is made of the green patent classification code adopted at the global level by the World Intellectual Property Organization.
The deficient data are obtained by linear interpolation method. Table 2 shows the descriptive statistics of the main variables.
5 Spatiotemporal pattern and dynamic evolution of urban ecological efficiency level
First, as preliminary research before discussing the causal relationship between the LCCP policy and urban ecological efficiency, it is essential to examine the scope, time evolution, and spatial evolution of Chinese urban ecological efficiency. All three methods can comprehensively illustrate the relationship and changes in the spatial–temporal pattern of urban ecological efficiency. Static inscriptions are mainly used to provide a brief snapshot of the spatiotemporal characteristics. Robust trends involving the dynamics of evolution are assessed using kernel density estimation. Additionally, employing the spatial Markov chain method is suggested to gain further empirical support for the results based on objective spatial factors.
Among the methods, the first one is a parametric estimation method, while the other two are non-parametric estimation methods. The most significant difference between these and parametric estimation methods is that the latter often does not require prior knowledge of the type of basic distribution being used. This means that if there are incorrect assumptions about the overall distribution, the errors can be significant, making the robustness of the estimation results superior. Moreover, the principle of kernel density estimation is entirely different from that of the evaluation tool, which aims at an overall approach to tracking the development of ecological efficiency. Spatial Markov chains focus on the spatial factors in the transition process and can compensate for the limitation of traditional Markov chains, which cannot describe spatial spillover effects.
5.1 Spatiotemporal pattern of ecological efficiency
Based on Stata 17.0 statistical software, this paper analyzes urban ecological efficiency levels for the years 2007, 2011, 2015, and projected 2020 and presents a spatial distribution map (Figure 2). The detailed breakdown of the study’s results is as follows:
In 2007, the overall scale of urban ecological efficiency was lower, showing a more dispersed pattern compared to subsequent years. By 2011, there was a continuous improvement in urban ecological efficiency levels in the central and eastern regions, with a trend toward dispersal. Regional distribution patterns showed characteristics of small-scale agglomeration. In 2015, overall urban ecological efficiency levels continued to rise, with some cities making significant advancements that influenced the efficiency of surrounding areas. By 2020, urban ecological efficiency levels in the central and eastern regions had risen significantly. This improvement trend extended westward, forming a more cohesive agglomeration pattern along the horizontal axis.
5.2 Dynamic evolution trend of ecological efficiency
5.2.1 Kernel density estimation
To compare and further study the dynamic evolution of urban ecological efficiency levels in China, this paper will utilize KDF analysis to examine the distribution, flexibility, and polarization trends among different cities. The specific method is as follows: Assuming that the density function of random variable Y is , the probability density at point Y can be estimated by Equations 7 and 8. The specific expressions are as follows:
In the above equation, N is the number of observations, and h is the bandwidth to control the degree of smoothing. Equation 9 is the optimal broadband expression, Se is the standard deviation of the observations of the random variable (Silverman, 1987), is the independently distributed observations, and Y is the mean value.
This paper presents a three-dimensional kernel density map to illustrate the calculation results, as depicted in Figure 3. From a distribution standpoint, the center of the kernel density estimation curve shows a tendency to shift rightward, indicating an increasing trend in urban ecological efficiency levels. Regarding distribution spread, the curve exhibits a distinct right-trailing characteristic, suggesting a higher degree of regional development imbalance. Analyzing the wave peaks, each year shows two or more peaks, with the main peak significantly higher than the side peaks, implying the presence of multiple centers and a noticeable trend toward multipolarization. The curve shape transitions from “tall and narrow” to “flat and narrow,” indicating an expanding gap in urban ecological efficiency levels among cities.
5.2.2 Spatial Markov chain model
The application of the spatial Markov chain model employs the aspect of the spatial lag to analyze the influence of the spatial factors which are the level of neighbor cities on the transfer probability of a given city.
For a Markov transition matrix in the form of , when k spatial lag types are considered as the transition conditions of regions, k conditional transition matrices in the form of will be generated, denoted as . The specific meaning of is that under the condition of the ecological efficiency of spatial lag in that year is λ, the probability of ecological efficiency transferring from type i to type j in the region after d years. In this paper, the phenomenon of the change of ecological efficiency is defined as the upward (or downward) n-order transfer; for example, a change in a neighboring type is an upward or downward first-order transfer.
In this paper, we use the economic spatial weight matrix () to calculate the spatial Markov chain. can be calculated from Equation 10, and matrix elements can be obtained from Equations 11 and 12. Where and , respectively, represent the per capita GDP of the i and j city during the observation period.
In addressing whether space factors can impact the growth of the ecological efficiency index in urban development, this article employs the chi-square test. The formula used is as follows:
In Equation (13), k is the level type, and , respectively, represents the sum of the element values of the two types of transition matrices and the number of regions belonging to this type of transition when the time length is d; is the transition probability value calculated after merging the two types of data; Q obeys the chi-square distribution and its degree of freedom is subtract the number of transition probabilities of 0.
Initially, based on the ecological efficiency index of 254 cities in China, these are categorized into four levels: low (L), low to medium (ML), medium high (MH), and high (H). Subsequently, cross-entropy is employed to estimate the 1-year lag of the transition probability matrix for the urban ecological efficiency index. For preliminary analysis of transition paths, the transitional Markov chain model is utilized. The results are summarized in Table 3.
Overall, the following conclusions can be drawn: 1) Evaluation of the stepping stone model probabilities shows a higher likelihood on the diagonal and a convergence phenomenon among the four types of clubs; 2) regarding urban ecological efficiency at low and medium high levels, there is a gradual upward trend, with higher transfer probabilities compared to downward transitions; and 3) despite cross-level transfers occurring, the process is not entirely smooth or straightforward; most transitions between different levels tend to move directly to the next higher level. For instance, the relatively mobile transition probability from low-level cities to the upper order is 14.4%, while the probability of transitioning to the higher two orders is only 1%.
The conventional theory of Markov chains assumes that each city is an isolated entity, solely analyzing the temporal dynamic properties of the ecological efficiency level of each city. Due to the constant evolution of policies, it is believed that the development level of neighboring cities will influence local eco-efficiency. Therefore, based on the results of the economic spatial weight analysis, we proceed with the study using the spatial Markov chain method. These findings are summarized in the table below. However, if viewed without considering their previous experience in database design, they would appear as presented in Table 4.
Overall, the following conclusions are drawn: First, there is a clear indication of convergence toward clusters within Chinese cities. For instance, there are no low-level cities around high-level cities, and the possibility of downward transfer approaches 0. This also supports the observation of a trend toward multipolarization in the kernel density estimation above. Second, spatial dynamics play a significant role in the transfer of relative ecological efficiency within urban areas. Higher levels of policy implementation and technological resource advantages have positive effects in stimulating surrounding cities and reducing the likelihood of bottom-up transfers.
At the same time, this paper uses the chi-square test method to test whether the spatial factors are significant to the growth of urban ecological efficiency. The final calculation result is that the Q value is 81.44, the degree of freedom is 36, is 58.619, and the p-value is 0.0000. The Q value is greater than the critical value and rejects the original hypothesis, which indicates that neighboring cities do have a significant impact on the transfer of the local ecological efficiency index.
6 Empirical results
6.1 Correlation test
First of all, we calculate the values of the Pearson coefficient for those main variables which were used in this article; we also draw the correlation coefficient matrix. A tabular representation of the identified results is presented in Table 5.
6.2 Benchmark regression
In this paper, we conducted tests and analyses on the data using the statistical software STATA 17.0 to estimate the model. Consequently, Histogram 0 depicts the model estimated using the software. It is also presented in Table 6, where the first two columns contain coefficients estimated based on the OLS model, while the last two contain fixed effect estimators. The estimates are presented across four columns: Column (1) includes no control variables, column (2) adds control variables, column (3) lacks control variables, and column (4) includes them. All model biases arising from heteroskedasticity are mitigated using the heteroskedasticity robust standard error method.
Additionally, the estimated coefficients of the LCCP pass the significance test at a 1% level, indicating a positive impact of the LCCP policy on urban ecological efficiency. Comparing the models, we find that the regression coefficient steadily declines to 0.259 when other city characteristics and a two-way fixed effect of a person’s year in-transition are controlled for. These regression results support the study’s hypothesis H1, suggesting that the implementation of the LCCP policy may enhance urban ecological efficiency.
6.3 Parallel trend test
However, there is an important precondition for the DID method: assuming a parallel trend between the treatment group and the control group. This assumes that the trends in variation of urban ecological efficiency levels in the pilot cities should be parallel to those in non-pilot cities before the policy implementation. Thus, it is crucial to justify the benchmark regression results with a parallel trend test.
The base period of this paper is defined as the first period within the research scope, identified as 2007. Due to the unavailability of potentially valuable data beyond the longitudinal range of 2001 to 2005, the examination period is defined as the 2 years before and the 5 years after the policy implementation.
The results of the parallel trend test are depicted in Figure 4, where the horizontal axis represents the year and the vertical axis represents the coefficient of the policy variable. Since all coefficient values are negative, it indicates that prior to policy implementation, none of the coefficients were significant in the context of the treatment and control group analysis. Therefore, the fluctuation of urban ecological efficiency levels shown in the two groups meets the requirement of the parallel trend test. Coefficients become meaningful in the year when the policy was implemented and in the subsequent 4 years.
6.4 Robust test
6.4.1 PSM-DID test
On one hand, the formulation of policies and the establishment of institutions in any country are not solely determined by external random factors; some are self-selected and often influenced by a series of economic and social reasons. Consequently, they concurrently impact the urban ecological efficiency of a city (Niu et al., 2023). Under such circumstances, our research design may face a significant self-selection problem. It becomes challenging to discern whether the LCCP policy affects urban ecological efficiency or if differences and changes in urban ecological efficiency drive the emergence and evolution of the policy. Therefore, using cities that do not adopt the pilot policy as counterfactual results for those that do could introduce sample selection bias.
To address this issue, we employ the PSM-DID method for testing. Initially, control variables represent covariates, and we utilize one-to-one nearest neighbor matching with replacement, caliper matching with a radius of 0.01, and kernel matching to enhance result credibility, effectively mitigating selection bias. This paper ensures that matching effects between the treatment and control groups are observed using density function graphs of propensity score distributions. Specific findings from the study are detailed as follows. Figure 5A denotes the propensity score before matching; Figures 5B–D denotes the propensity score figure after using nearest neighbor matching, kernel matching, and caliper matching, respectively. These figures demonstrate that post-matching, the density distributions of propensity scores for the treatment and control groups align more closely than pre-matching, with kernel and radius matching results overlapping. This indicates successful matching.
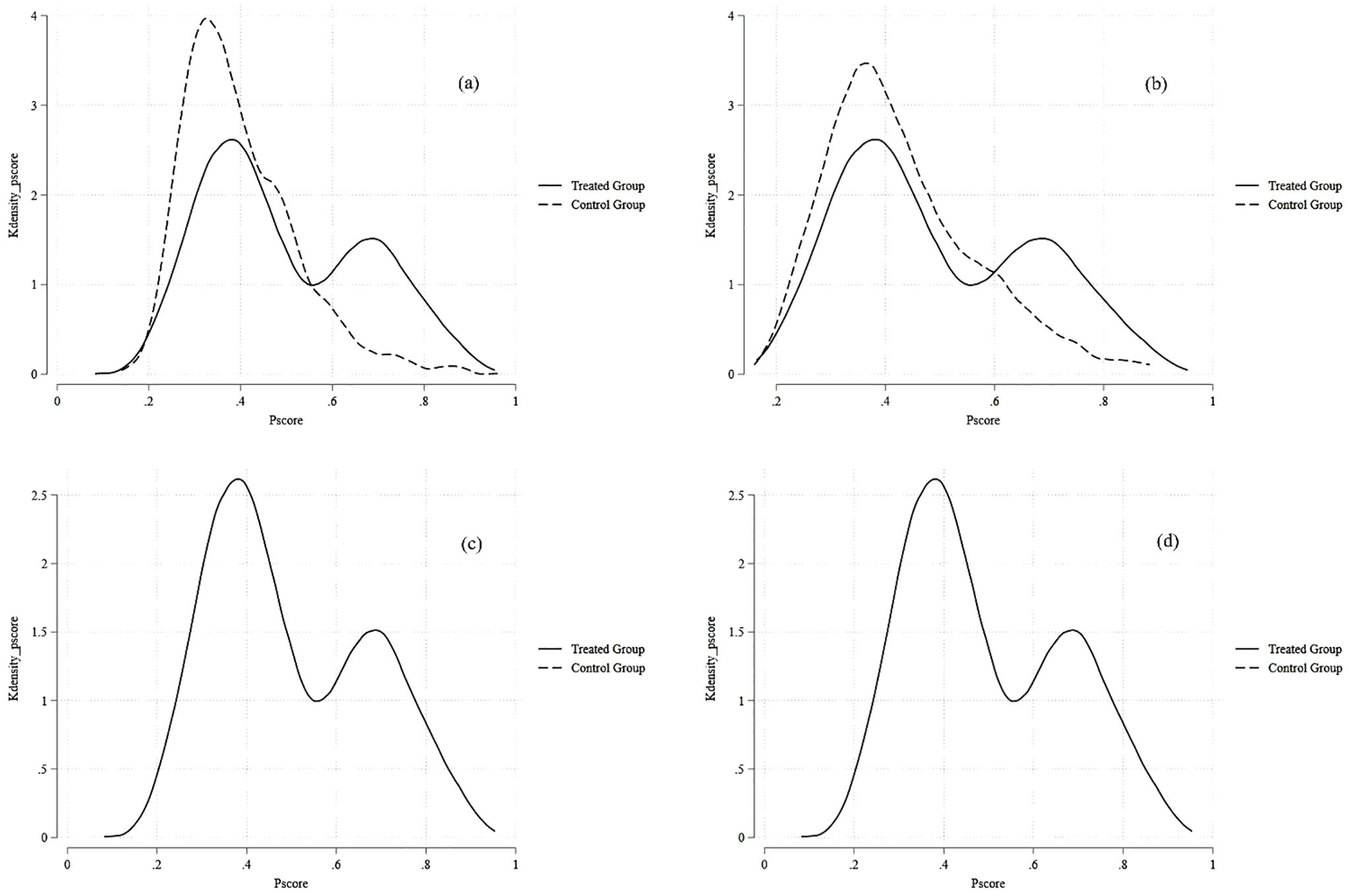
Figure 5. Propensity score (A) before matching and after using (B) nearest neighbor matching, (C) kernel matching, and (D) caliper matching.
Following PSM, DID regression is conducted on the filtered samples, yielding results shown in Table 7. The coefficients for LCCP in regression results, screened through the three matching methods, are 0.0259, 0.0236, and 0.0253, all significant at the 1% level. These results largely mirror those of the benchmark regression in the preceding section, confirming the robustness of benchmark regression findings and further supporting hypothesis H1.
6.4.2 Placebo test
To exclude interference from other policies or random factors and enhance the credibility of the benchmark regression results, this paper conducts a placebo test using a fictitious treatment group. Figure 6 presents the final distribution graph of the regression estimated coefficients, indicating that the coefficients from random sampling follow a normal distribution centered around 0. The results of 500 regressions all lie to the left of the true regression estimate, which is 0.0253. This suggests that our estimates are unlikely to have been obtained by chance and are therefore unlikely to have been influenced by other policies or random factors.
6.4.3 Policy lag order
Because policy lag is also a contingency factor influencing the occurrence of a policy in this paper, the policy occurrence is shifted backward in time, and the results are presented in Table 8. The estimated effect of the LCCP policy is consistently greater than 0, aligning with the regression results mentioned earlier.
6.4.4 Special samples deletion
Initially, the inclusion of data spanning from 2007 to 2020 encompassed cities that only began implementing low-carbon pilot policies in 2017. Given this short timeframe, it was challenging to discern the policy’s full impact. Therefore, one particular sample was excluded and re-estimated using the transformation sample method, as indicated in the first two columns of Table 9 below. Following the exclusion of these samples, the estimated coefficients for the LCCP policy showed a slight increase while maintaining statistical significance at the 1% level. This strengthens the credibility of the analysis and supports the conclusions drawn.
6.4.5 Exclusion of other policies
In this paper, the policies conducted concurrently with the LCCP may introduce biases in assessing policy impacts. To mitigate this, we focus on pilot policies implemented simultaneously in smart cities and introduce a dummy variable (Smart) into our regression model. The results are presented in the rightmost columns of Table 9, where the regression findings reveal a significantly positive sign and magnitude for the estimated coefficient of the LCCP. This suggests that the outcomes of this policy within the LCCP framework are not influenced by other policies, affirming its contribution to the benchmark regression results.
6.4.6 Bacon decomposition
We also found that due to a multiyear DID approach, the timing of policy changes varies across different research units, and some treatment groups can transition to the control group in any given year (Goodman-Bacon, 2018). Therefore, this paper applies the Bacon decomposition to diagnose such biases. Table 10 and Figure 7 present the results of the Bacon decomposition.
Upon examining the data in Table 8, it is evident that 86.3% of the variation in LCCP stems from the treated and untreated groups, while the time group (including × and ▲) has a smaller impact, accounting for only 13.7%. The influence of the ineffective control group (×) amounts to 6.6%, exerting minimal impact on the bias of the LCCP estimator.
Analysis of the results depicted in Figure 7 reveals that the time groups predominantly cluster around point 0, with the total estimator and the red line plots showing insignificant deviations in most cases. This further indicates that the LCCP model estimator is not significantly biased.
7 Further analysis
7.1 Mechanism testing
As mentioned earlier, both the results of the robustness test as well as the benchmark regression provide evidence for our conclusion based on the LCCP policy—that high urban ecological efficiency is increased. However, it is still necessary to uncover an aspect in which the LCCP policy works, that is, to some extent, how does this policy contribute to enhancing urban ecological efficiency? We think that as for the LCCP policy, the mechanism enables the green innovation effect and the decoupling effect for raising the level of urban ecological efficiency. Table 11 also presents the summary of regression results. In general, the overall regression value represents the contribution of each individual variable to the overall model.
The regression results of the first two columns show that the LCCP policy increases at the level of 1% the degree of green technology development and then improves the ecological efficiency of urban areas. In support of hypothesis H2a, the purpose and effectiveness of the LCCP policy has been identified in this study, where it has been found to be capable of boosting the vitality of green technology innovation in urban areas, facilitating the flow of technology factor into eco-friendly industries, enhancing the efficiency of ecological utility, and translating innovation in green technology into the “green innovation effect.”
Analysis of the results of the last two columns most likely related to the regression analysis showed that the impact of the LCCP policy is rather significant in the negative direction to the energy structure at the level of 5%, enabling an enhanced level of urban ecological efficiency. Consequently, hypothesis H2b is valid: the LCCP policy can take the place of optimizing the energy structure, enhancing the energy utilization efficacy, changing the kernel of the driving force of economic growth, and realizing the “decoupling” between the development economy and energy consumption as carrying out the “decoupling effect.”
In the following part of the analysis, we use the bootstrap method to perform auxiliary tests to apply the test on the assumption of mediation effect. About the test of green technology innovation and energy intensity, the 95% CI values after bias correction are, respectively, as follows: (0.0061, 0.0139) and (0.0013, 0.0040). X2 is greater than X1 and the confidence intervals do not contain 0, and they are significant at different levels, particularly at the 1% level, suggesting that there is a mediating effect of green technology innovation and energy intensity in the LCCP policy.
7.2 Heterogeneity analysis
7.2.1 Urban geographic location heterogeneity
China is a huge country and that makes it quite large both in geographical size and population size. The economic and social development of different cities is not similar, and the implementation of policies differs with the local government; this factor may be playing a role that can differently affect the proportion of ecological efficiency due to the LCCP policy. This paper thus partitions the sample into groups by region—east, west, and central—and carries out the regression for each separately; the findings are presented in Table 12 in the first three columns. The results of this hypothesis report that the impact of the introduced LCCP policy is more profound in the western cities in contrast to that observed in the eastern and central cities. Therefore, we can also conclude that geographic location plays a vital role in determining how the implementation of the LCCP policy will affect the ecological efficiency of cities.
This may be because, on the one hand, the eastern region, as a more economically developed region in China, tends to be more industrialized but also faces more serious resource bottleneck constraints and social problems, which make the policy effectiveness to be more constrained. On the other hand, industrialization and urbanization are the main features of the development history of the central region, but the pollution during industrialization has brought about a serious “ecological deficit.” Therefore, the costs of the policy implementation are particularly high, while there is a lack of capital for development with the resources flowing to the east continually. It is difficult to choose between the development and ecological construction in the central region of China.
7.2.2 Urban resources heterogeneity
Taking into account the dissimilar nature of the development processes in the industries of various cities and the fact that some cities, on the one hand, manage urban advancement through the RBIs is important. In this paper, the scope of the 254 prefecture-level cities is extended and is divided into two groups of resource-based cities and non-resource-based cities as defined by the China No. 45 National Development Document in 2013. We perform the regressive analysis of the two groups, and the results are presented in the last two columns of Table 12. This result demonstrated the importance of LCCP construction toward the non-resource-based cities which is significantly positive at the 1% level.
However, the promoting effect on resource-based cities is negative, as shown by the following reasons: This might be due to the fact that the resource-based cities have over a long time of development, gradually developing a natural resource-oriented industrial development system which not only discharges huge amounts of greenhouse gases but also lacks the impetus of innovating and constructing environmentally friendly industries and sustaining resource-friendly industries.
The simple conventional low-carbon cities based on the so-called “low-carbon” concept might be hard to have a definite positive impact on ecological efficiency.
7.2.3 Urban agglomerations heterogeneity
This paper also therefore provides an analysis of how heterogeneity of the LCCP construction has affected the ecological efficiency of three selected major urban agglomerations (Beijing-Tianjin-Hebei, Yangtze River Delta, and Pearl River Delta). Table 13 presents the specific regression results of each variable on urban agglomerations heterogeneity. The empirical analysis shows that the establishment of LCCP has a positive influence on the ecological construction of the Pearl River Delta and Yangtze River Delta’s agglomerations, and the relevant estimated parameters are 0.0543 and 0.5825. However, it is not large in the Beijing-Tianjin-Hebei tripartite region. Hence, the LCC construction has a more obvious consequence for the ecological construction as an external environment element in the Pearl River Delta and the Yangtze River Delta agglomeration, whereas it has no apparent result on the Beijing-Tianjin-Hebei agglomeration.
8 Conclusions and implications
The rapid pace of urbanization has resulted in a disregard for environmental care, contributing to the proliferation of “urban ills” such as prioritizing economic growth over ecological harmony, industrial expansion leading to environmental degradation, and urban sprawl accompanied by carbon-intensive activities. The low-carbon city model, which integrates sustainability with progress, aims to achieve a harmonious coexistence between humanity and the planet. As previously noted, recent studies have identified causal relationships between effective low-carbon city management and environmental improvement. However, the absence of an indicator system for ecological output and carbon footprints skews evaluations of ecological efficiency, often focusing solely on the economic growth–energy consumption equation. Additionally, the impact of technological innovation and energy consumption on ecological efficiency requires more robust scientific validation.
This study utilizes balanced panel data encompassing 254 Chinese prefectural-level cities from 2007 to 2020 to establish a framework for estimating ecological efficiency. It seeks to delineate the nature and chronology of changes, as well as overall trends within these urbanized settings and their definitive or indeterminate impacts on ecosystem efficiency. To investigate the three aforementioned hypotheses, this study employs the DID method to analyze the effects of the LCCP on ecological efficiency. Furthermore, it examines the mediating influence of green technology innovation and energy structure on the relationship between the LCCP and ecological efficiency. Based on the outlined study, the following conclusions have been drawn:
Firstly, our analysis of ecological efficiency across Chinese cities reveals an overall improving trend, both spatially and temporally, leading to the emergence of distinct groups or clusters. As we track changes over time, a clear divide in efficiency levels among cities becomes evident, with an increasing gap between them. Utilizing the classic Markov chain analysis, we have identified a “club convergence” effect, indicating the difficulty for cities to transition between different efficiency levels. The influence of location on changes in ecological efficiency is significant. Regions with higher ecological efficiency leverage their extensive experience in policy implementation and technological prowess, benefiting themselves and creating spillover effects that uplift nearby regions and reduce the likelihood of regression in efficiency.
Secondly, the implementation of the LCCP policy has notably enhanced the ecological efficiency of urbanization, a finding supported by various robustness checks. This study builds upon prior research exploring diverse, interconnected, and dynamic aspects related to low-carbon urban strategies. These include the relationship between low-carbon urban initiatives and air quality (Yang et al., 2023b), strategies to promote green transport and transform resident lifestyles toward sustainability (Li et al., 2023a; Zhang and Zheng, 2023), and strategies to break away from carbon lock-in (Zhao et al., 2023a). Other scholars have also supported our conclusions from various perspectives, such as industrial structure and innovation (Ling et al., 2024), industrial agglomeration (Wu et al., 2022), and digital financial inclusion (Liu et al., 2024). In contrast to evaluating single indicators or the necessity of ecological efficiency indicators within specific fields, this paper delves into redefining ecological efficiency in detail, offering robust data to support the causal relationship between the LCCP policy and ecological efficiency. Based on this foundation, the authors propose that future steps should focus on piloting low-carbon cities to amplify their pioneering and demonstrative effects, thereby assisting neighboring cities in exploring ecological civilization construction. Economically, to balance economic development and ecological construction, governments should formulate emission reduction strategies and foster the emergence and development of cities specializing in low-carbon industries. From an ecological sensitivity standpoint, expanding urban green spaces and cultivating social norms favoring low-carbon transportation are crucial.
Thirdly, in our key finding, we observe that the LCCP policy is effectively enhancing the eco-friendliness of our cities. It aims to promote innovative green technologies and reduce energy consumption while maintaining productivity. We refer to these dual benefits as the “green innovation effect” and the “decoupling effect.” These concepts are not new; previous researchers have discussed similar ideas, particularly when it comes to understanding how we can push for more of this good stuff (Ali et al., 2023) and “inhibition” (Zhao et al., 2023d; Zhou et al., 2022) on the “innovation effect.” Few studies, however, have explored how energy structure influences the relationship between the LCCP policy and a city’s ecological efficiency. Our research addresses this gap by focusing on green technology innovation and energy consumption. Consequently, we suggest that governments should discourage environmentally harmful industries and incentivize companies to invest more in green research and development. This can be achieved through tax incentives, performance-based rewards, and funding support for research initiatives. Moreover, it is imperative to reduce reliance on fossil fuels to safeguard our natural resources and environment.
Fourthly, geographical location, natural resources, and urban agglomerations significantly influence the effectiveness of pilot policies. The impact of the LCCP policy appears more pronounced in western regions, cities less dependent on natural resources, and urban clusters like the Pearl River and Yangtze River Deltas. This underscores the role of favorable location and transportation infrastructure in policy efficacy. However, there is a caveat: The policy seems less effective in resource-dependent cities, potentially due to the “resource curse” hindering ecological efficiency (Wu et al., 2023). Therefore, when implementing such policies, it is crucial to tailor approaches to local conditions and characteristics.
Finally, employing the DID approach to assess the impact of the LCCP policy on ecological efficiency initiates a critical dialogue that is still unfolding. Several areas warrant further investigation. Firstly, our study is limited to Chinese cities, necessitating a broader global perspective. Secondly, a deeper examination at the provincial and county levels could provide richer insights. Additionally, recognizing the limitations of our methodology, continuous monitoring of policy effects over time is essential, alongside consideration of other factors influencing ecological efficiency.
Data availability statement
The original contributions presented in the study are included in the article/supplementary material. Further inquiries can be directed to the corresponding author.
Author contributions
JL: Conceptualization, Formal analysis, Methodology, Software, Visualization, Writing – original draft, Validation, Writing – review & editing. KX: Writing – review & editing, Validation. DJ: Formal analysis, Methodology, Writing – original draft. CW: Validation, Visualization, Writing – review & editing, Data curation. YZ: Funding acquisition, Project administration, Resources, Supervision, Writing – review & editing, Investigation.
Funding
The author(s) declare financial support was received for the research, authorship, and/or publication of this article. This work was supported by the Beijing Forestry University Science and Technology Innovation Project (Grant number 2021SCL01).
Conflict of interest
The authors declare that the research was conducted in the absence of any commercial or financial relationships that could be construed as a potential conflict of interest.
Publisher’s note
All claims expressed in this article are solely those of the authors and do not necessarily represent those of their affiliated organizations, or those of the publisher, the editors and the reviewers. Any product that may be evaluated in this article, or claim that may be made by its manufacturer, is not guaranteed or endorsed by the publisher.
References
Ahmad M., Wu Y. (2022). Natural resources, technological progress, and ecological efficiency: Does financial deepening matter for G-20 economies? Resour. Policy 77, 102770. doi: 10.1016/j.resourpol.2022.102770
Ali M., Joof F., Samour A., Tursoy T., Balsalobre-Lorente D., Radulescu M. (2023). Testing the impacts of renewable energy, natural resources rent, and technological innovation on the ecological footprint in the USA: Evidence from Bootstrapping ARDL. Resour. Policy 86, 104139. doi: 10.1016/j.resourpol.2023.104139
Bie F., Yang Y., Shen H., Zhao Q. (2024). Inclusive digital economy, resource dependence and changes in the urban energy mix: City level analysis from China. Resour. Policy 92, 105027. doi: 10.1016/j.resourpol.2024.105027
Brendler P. (2022). Rising earnings inequality and optimal income tax and social security policies. J. Monetary Economics. 134, 35–52. doi: 10.1016/j.jmoneco.2022.10.004
Callaway B., Sant’anna P. H. C. (2018). Difference-in-differences with multiple time periods. CJRN: Criminol. Res. Method. (Topic). 225, 200–230. doi: 10.1016/j.jeconom.2020.12.001
Chen W., Liu J., Ning X., Du L., Zhang Y., Wu C. (2023a). Low-carbon city building and green development: new evidence from quasi natural experiments from 277 cities in China. Sustainability. 15, 11609. doi: 10.3390/su151511609
Chen Y., Liu K., Ni L., Chen M. (2023b). Impact of carbon lock-in on green economic efficiency: Evidence from Chinese provincial data. Sci. Total Environ. 892, 164581. doi: 10.1016/j.scitotenv.2023.164581
Cheng J., Yi J., Dai S., Xiong Y. (2019). Can low-carbon city construction facilitate green growth? Evidence from China’s pilot low-carbon city initiative. J. Cleaner Production 231, 1158–1170. doi: 10.1016/j.jclepro.2019.05.327
Cong J., Liu X., Zhao X. (2014). Demarcation problems and the corresponding measurement methods of the urban carbon accounting. Chin. J. Population Resour. Environ. 24, 19–26.
Danish, Ulucak R., Baloch M. A. (2023). An empirical approach to the nexus between natural resources and environmental pollution: Do economic policy and environmental-related technologies make any difference? Resour. Policy. 81, 103361. doi: 10.1016/j.resourpol.2023.103361
Das M., Das A., Pereira P. (2023). Developing an integrated urban ecological efficiency framework for spatial ecological planning: A case on a tropical mega metropolitan area of the global south. Geosci. Front. 14, 101489. doi: 10.1016/j.gsf.2022.101489
Dong F., Zhang Y., Zhang X. (2020). Applying a data envelopment analysis game cross-efficiency model to examining regional ecological efficiency: Evidence from China. J. Cleaner Production 267, 122031. doi: 10.1016/j.jclepro.2020.122031
Du M., Feng R., Chen Z. (2022). Blue sky defense in low-carbon pilot cities: A spatial spillover perspective of carbon emission efficiency. Sci. total Environ. 846, 157509. doi: 10.1016/j.scitotenv.2022.157509
Fan Y., Meng J., Ye H., Wang P., Wang Y., Wang Y. (2021). Sustainability and ecological efficiency of low-carbon power system: A concentrating solar power plant in China. J. Environ. Manage. 290, 112659. doi: 10.1016/j.jenvman.2021.112659
Feng Y., Zhang J., Geng Y., Jin S., Zhu Z., Liang Z. (2023). Explaining and modeling the reduction effect of low-carbon energy transition on energy intensity: Empirical evidence from global data. Energy 281, 128276. doi: 10.1016/j.energy.2023.128276
Gao P. P., Li Y. P., Sun J., Li H. W. (2018). Coupling fuzzy multiple attribute decision-making with analytic hierarchy process to evaluate urban ecological security: A case study of Guangzhou, China. Ecol. Complexity 34, 23–34. doi: 10.1016/j.ecocom.2018.03.001
Goodman-Bacon A. (2018). Difference-in-differences with variation in treatment timing. Econometrics: Multiple Equation Models eJournal. 225, 254–277. doi: 10.3386/w25018
Haarstad H. (2016). Where are urban energy transitions governed? Conceptualizing the complex governance arrangements for low-carbon mobility in Europe. Cities 54, 4–10. doi: 10.1016/j.cities.2015.10.013
He J., Hu S. (2022). Ecological efficiency and its determining factors in an urban agglomeration in China: The Chengdu-Chongqing urban agglomeration. Urban Climate 41, 101071. doi: 10.1016/j.uclim.2021.101071
Holtz G., Xia-Bauer C., Roelfes M., Schüle R., Vallentin D., Martens L. (2018). Competences of local and regional urban governance actors to support low-carbon transitions: Development of a framework and its application to a case-study. J. Cleaner Production 177, 846–856. doi: 10.1016/j.jclepro.2017.12.137
Hou H., Guo H., Yun X. (2022). Exploring the impact of environmental regulation and economic agglomeration on ecological efficiency in China. Polish J. Environ. Stud. 31, 1109–1124. doi: 10.15244/pjoes/140298
Hou X., Hu Q., Liang X., Xu J. (2023). How do low-carbon city pilots affect carbon emissions? Staggered difference in difference evidence from Chinese firms. Economic Anal. Policy 79, 664–686. doi: 10.1016/j.eap.2023.06.030
Jie G., Jiahui L. (2023). Media attention, green technology innovation and industrial enterprises’ sustainable development: The moderating effect of environmental regulation. Economic Anal. Policy 79, 873–889. doi: 10.1016/j.eap.2023.07.003
Lan T., Shao G., Xu Z., Tang L., Dong H. (2023). Considerable role of urban functional form in low-carbon city development. J. Cleaner Production 392, 136256. doi: 10.1016/j.jclepro.2023.136256
Li J., Tang F., Zhang S., Zhang C. (2023a). The effects of low-carbon city construction on bus trips. J. Public Transportation 25, 100057. doi: 10.1016/j.jpubtr.2023.100057
Li R., Wang Q., Li L. (2023b). Does renewable energy reduce per capita carbon emissions and per capita ecological footprint? New evidence from 130 countries. Energy Strategy Rev. 49, 101121. doi: 10.1016/j.esr.2023.101121
Li Y., Zhang J., Yang X., Wang W., Wu H., Ran Q., et al. (2021). The impact of innovative city construction on ecological efficiency: a quasi-natural experiment from China. Sustain. Production Consumption 28, 1724–1735. doi: 10.1016/j.spc.2021.09.012
Lin C. L., Chan E. H. W., Chiang W.-H. (2022a). Urban renewal governance and manipulation of plot ratios: A comparison between Taipei, Hong Kong and, Singapore. Land Use Policy 119, 106158. doi: 10.1016/j.landusepol.2022.106158
Lin X., Tang Z., Long H. (2022b). Spatial and temporal research on ecological total factor energy efficiency in China: Based on “Ecology-Economy-Geography” heterogeneity framework. J. Cleaner Production 377, 134143. doi: 10.1016/j.jclepro.2022.134143
Ling S., Jin S., Wang H., Zhang Z., Feng Y. (2024). Transportation infrastructure upgrading and green development efficiency: Empirical analysis with double machine learning method. J. Environ. Manage. 358, 120922. doi: 10.1016/j.jenvman.2024.120922
Liu F., Liu G., Wang X., Feng Y. (2024). Whether the construction of digital government alleviate resource curse? Empirical evidence from Chinese cities. Resour. Policy 90, 104811. doi: 10.1016/j.resourpol.2024.104811
Niu S., Zhang J., Luo R., Feng Y. (2023). How does climate policy uncertainty affect green technology innovation at the corporate level? New evidence from China. Environ. Res. 237, 117003. doi: 10.1016/j.envres.2023.117003
Niu S., Zhang K., Zhang J., Feng Y. (2024). How does industrial upgrading affect urban ecological efficiency? New evidence from China. Emerging Markets Finance Trade 60, 899–920. doi: 10.1080/1540496X.2023.2260544
Ozkan O., Sharif A., Mey L. S., Tiwari S. (2023). The dynamic role of green technological innovation, financial development and trade openness on urban environmental degradation in China: Fresh insights from carbon efficiency. Urban Climate 52, 101679. doi: 10.1016/j.uclim.2023.101679
Preacher K. J., Hayes A. F. (2008). Asymptotic and resampling strategies for assessing and comparing indirect effects in multiple mediator models. Behav. Res. Methods 40, 879–891. doi: 10.3758/BRM.40.3.879
Ramzan M., Abbasi K. R., Salman A., Dagar V., Alvarado R., Kagzi M. M. (2023). Towards the dream of go green: An empirical importance of green innovation and financial depth for environmental neutrality in world’s top 10 greenest economies. Technological Forecasting Soc. Change. 189, 122370. doi: 10.1016/j.techfore.2023.122370
Sakariyahu R., Lawal R., Etudaiye-Muhtar O. F., Ajide F. M. (2023). Reflections on COP27: How do technological innovations and economic freedom affect environmental quality in Africa? Technological Forecasting Soc. Change 195, 122782. doi: 10.1016/j.techfore.2023.122782
Shen L., Yang Y., Bao H. D. X., Du X., He H. (2023a). Residents’ Perceptions on the urban resources environment in Chinese large cities. SSRN Electronic J. 100, 107080. doi: 10.1016/j.eiar.2023.107080
Shen Q., Wu R., Pan Y., Feng Y. (2024). Explaining and modeling the impacts of inclusive finance on CO2 emissions in China integrated the intermediary role of energy poverty. Humanities Soc. Sci. Commun. 11, 82. doi: 10.1057/s41599-023-02595-w
Shen Y., Shi X., Zhao Z., Sun Y., Shan Y. (2023b). Measuring the low-carbon energy transition in Chinese cities. iScience 26, 105803. doi: 10.1016/j.isci.2022.105803
Silverman B. W. (1987). Density Estimation for Statistics and Data Analysis (Monographs on Statistics and Applied Probability, London: Chapman and Hall).
Sobhani P., Esmaeilzadeh H., Wolf I. D., Deljouei A., Marcu M. V., Sadeghi S. M. M. (2023). Evaluating the ecological security of ecotourism in protected area based on the DPSIR model. Ecol. Indic. 155, 110957. doi: 10.1016/j.ecolind.2023.110957
Song M., Zhao X., Shang Y. (2020). The impact of low-carbon city construction on ecological efficiency: Empirical evidence from quasi-natural experiments. Resources Conserv. Recycling 157, 104777. doi: 10.1016/j.resconrec.2020.104777
Stucki T., Woerter M. (2017). Green inventions: is wait-and-see a reasonable option? Energy J. 38, 43–71. doi: 10.5547/01956574.38.4.tstu
Su Y., Li Z., Yang C. (2021). Spatial interaction spillover effects between digital financial technology and urban ecological efficiency in China: an empirical study based on spatial simultaneous equations. Int. J. Environ. Res. Public Health 18, 8535. doi: 10.3390/ijerph18168535
Sun Y., Gao P., Razzaq A. (2023). How does fiscal decentralization lead to renewable energy transition and a sustainable environment? Evidence from highly decentralized economies. Renew. Energy. 206, 1064–1074. doi: 10.1016/j.renene.2023.02.069
Sun X., Loh L., Chen Z. (2019). Effect of market fragmentation on ecological efficiency: evidence from environmental pollution in China. Environ. Sci. pollut. Res. 27, 4944–4957. doi: 10.1007/s11356-019-06548-2
Wang Y. (2017). An industrial ecology virtual framework for policy making in China. Economic Syst. Res. 29, 252–274. doi: 10.1080/09535314.2017.1313199
Wang J., Deng K. (2021). Impact and mechanism analysis of smart city policy on urban innovation: Evidence from China. Economic Anal. Policy. 73, 574–587. doi: 10.1016/j.eap.2021.12.006
Wang X., Feng Y. (2021). The effects of National High-tech Industrial Development Zones on economic development and environmental pollution in China during 2003–2018. Environ. Sci. pollut. Res. 28, 1097–1107. doi: 10.1007/s11356-020-10553-1
Wang L., Shao J., Ma Y. (2023a). Does China’s low-carbon city pilot policy improve energy efficiency? Energy 283, 129048. doi: 10.1016/j.energy.2023.129048
Wang Y., Sun M., Wang R.-Q., Lou F. (2015). Promoting regional sustainability by eco-province construction in China: A critical assessment. Ecol. Indic. 51, 127–138. doi: 10.1016/j.ecolind.2014.07.003
Wang Y., Wang H. (2023). Spatial spillover effect of urban sprawl on total factor energy ecological efficiency: Evidence from 272 cities in China. Energy 273, 127217. doi: 10.1016/j.energy.2023.127217
Wang X., Wang G., Chen T., Zeng Z., Heng C. K. (2022). Low-carbon city and its future research trends: a bibliometric analysis and systematic review. Sustain. Cities Society. 90, 104381. doi: 10.1016/j.scs.2022.104381
Wang Y., Wei S., He X., Gu H. (2023b). Environmental regulation and entrepreneurial activity: Evidence from the low-carbon city pilot policy in China. Sustain. Cities Soc. 98, 104829. doi: 10.1016/j.scs.2023.104829
Wang S., Zhao D., Chen H. (2020). Government corruption, resource misallocation, and ecological efficiency. Energy Economics 85, 104573. doi: 10.1016/j.eneco.2019.104573
Wen H., Hu K., Nghiem X.-H., Acheampong A. O. (2024a). Urban climate adaptability and green total-factor productivity: Evidence from double dual machine learning and differences-in-differences techniques. J. Environ. Manage. 350, 119588. doi: 10.1016/j.jenvman.2023.119588
Wen H., Yu H., Nghiem X.-H. (2024b). Impact of urban sprawl on carbon emission efficiency: Evidence from China. Urban Climate 55, 101986. doi: 10.1016/j.uclim.2024.101986
Wu G., Gao Y., Feng Y. (2023). Assessing the environmental effects of the supporting policies for mineral resource-exhausted cities in China. Resour. Policy 85, 103939. doi: 10.1016/j.resourpol.2023.103939
Wu K., You K., Ren H., Gan L. (2022). The impact of industrial agglomeration on ecological efficiency: An empirical analysis based on 244 Chinese cities. Environ. Impact Assess. Rev. 96, 106841. doi: 10.1016/j.eiar.2022.106841
Wu Y., Zong T., Shuai C., Jiao L. (2024). How does new-type urbanization affect total carbon emissions, per capita carbon emissions, and carbon emission intensity? An empirical analysis of the Yangtze River economic belt, China. J. Environ. Manage. 349, 119441. doi: 10.1016/j.jenvman.2023.119441
Xu G., Chang H. P., Meng L., Sine Marma K. J. (2022). Green development level, resource utilization, and ecological protection across China from 2006 to 2017: Based on the national standard indicator system. Environ. Dev. 44, 100776. doi: 10.1016/j.envdev.2022.100776
Xu Y., Dong Z., Wu Y. (2023). The spatiotemporal effects of environmental regulation on green innovation: Evidence from Chinese cities. Sci. total Environ. 876, 162790. doi: 10.1016/j.scitotenv.2023.162790
Xu L., Wang Y. (2022). City carbon emission accounting in China: international statistical standards measurement and methodology construction. Stat. Res. 39, 12–30. doi: 10.19343/j.cnki.11-1302/c.2022.07.002
Yang S., Jahanger A., Hossain M. R. (2023a). Does China’s low-carbon city pilot intervention limit electricity consumption? An analysis of industrial energy efficiency using time-varying DID model. Energy Economics 121, 106636. doi: 10.1016/j.eneco.2023.106636
Yang Z., Yuan Y., Tan Y. (2023b). The impact and nonlinear relationship of low-carbon city construction on air quality: Evidence from a quasi-natural experiment in China. J. Cleaner Production 422, 138588. doi: 10.1016/j.jclepro.2023.138588
Yasmeen H., Tan Q., Zameer H., Tan J., Nawaz K. (2020). Exploring the impact of technological innovation, environmental regulations and urbanization on ecological efficiency of China in the context of COP21. J. Environ. Manage. 274, 111210. doi: 10.1016/j.jenvman.2020.111210
Yin Y., Hou X., Liu J., Zhou X., Zhang D. (2022). Detection and attribution of changes in cultivated land use ecological efficiency: A case study on Yangtze River Economic Belt, China. Ecol. Indicators. 137, 108753. doi: 10.1016/j.ecolind.2022.108753
Yin H., Qian Y., Zhang B., Pérez R. (2023). Urban construction and firm green innovation: Evidence from China’s low-carbon pilot city initiative. Pacific-Basin Finance J. 80, 102070. doi: 10.1016/j.pacfin.2023.102070
Zhang S., Dong R., Jiang J., Yang S., Cifuentes-Faura J., Peng S., et al. (2024). Whether the green credit policy effectively promote green transition of enterprises in China? Empirical analysis and mechanism verification. Environ. Res. 244, 117910. doi: 10.1016/j.envres.2023.117910
Zhang R.-L., Liu X.-H. (2021). Evaluating ecological efficiency of Chinese industrial enterprise. Renewable Energy 178, 679–691. doi: 10.1016/j.renene.2021.06.119
Zhang Z., Shi K., Gao Y., Feng Y. (2023). How does environmental regulation promote green technology innovation in enterprises? A policy simulation approach with an evolutionary game. J. Environ. Plann. Manage., 1–30. doi: 10.1080/09640568.2023.2276064
Zhang J., Zheng T. (2023). Can dual pilot policy of innovative city and low carbon city promote green lifestyle transformation of residents? J. Cleaner Production 405, 136711. doi: 10.1016/j.jclepro.2023.136711
Zhao C., Dong K., Wang K., Taghizadeh-Hesary F. (2023a). How can Chinese cities escape from carbon lock-in? The role of low-carbon city policy. Urban Climate 51, 101629. doi: 10.1016/j.uclim.2023.101629
Zhao X., Long L., Yin S., Zhou Y. (2023d). How technological innovation influences carbon emission efficiency for sustainable development? Evidence from China. Resources Environ. Sustainability 14, 100135. doi: 10.1016/j.resenv.2023.100135
Zhao F., Qian S., Zhao X. (2023b). Collaborative governance of carbon reduction in urban agglomerations in the China Yangtze River Economic Belt based on a spatial association network. Ecol. Indic. 154, 110663. doi: 10.1016/j.ecolind.2023.110663
Zhao X., Shang Y., Song M. (2020). Industrial structure distortion and urban ecological efficiency from the perspective of green entrepreneurial ecosystems. Socio-Economic Plann. Sci. 72, 100757. doi: 10.1016/j.seps.2019.100757
Zhao S., Zhang L., An H., Peng L., Zhou H., Hu F. (2023c). Has China’s low-carbon strategy pushed forward the digital transformation of manufacturing enterprises? Evidence from the low-carbon city pilot policy. Environ. Impact Assess. Rev. 102, 107184. doi: 10.1016/j.eiar.2023.107184
Keywords: low-carbon city, super-SBM model, ecological efficiency, kernel density estimation, spatial Markov chain model, multi-period difference-in-differences (DID), green innovation effect, decoupling effect
Citation: Liu J, Xu K, Jin D, Wu C and Zhang Y (2024) Urban low-carbon governance and ecological efficiency: new evidence from prefecture-level cities in China. Front. Ecol. Evol. 12:1442755. doi: 10.3389/fevo.2024.1442755
Received: 02 June 2024; Accepted: 09 August 2024;
Published: 03 September 2024.
Edited by:
Salvador García-Ayllón Veintimilla, Polytechnic University of Cartagena, SpainCopyright © 2024 Liu, Xu, Jin, Wu and Zhang. This is an open-access article distributed under the terms of the Creative Commons Attribution License (CC BY). The use, distribution or reproduction in other forums is permitted, provided the original author(s) and the copyright owner(s) are credited and that the original publication in this journal is cited, in accordance with accepted academic practice. No use, distribution or reproduction is permitted which does not comply with these terms.
*Correspondence: Yang Zhang, emhhbmd5YW5nMDUyMDEyQGFsaXl1bi5jb20=