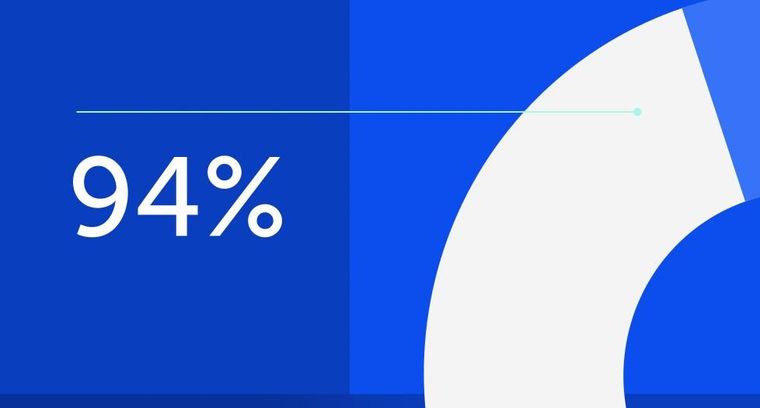
94% of researchers rate our articles as excellent or good
Learn more about the work of our research integrity team to safeguard the quality of each article we publish.
Find out more
ORIGINAL RESEARCH article
Front. Ecol. Evol., 26 June 2024
Sec. Conservation and Restoration Ecology
Volume 12 - 2024 | https://doi.org/10.3389/fevo.2024.1377214
Introduction: Terrestrial species in riverine ecosystems face unique constraints leading to diverging patterns of population structure, connectivity, and disease dynamics. Desert bighorn sheep (Ovis canadensis nelsoni) in Grand Canyon National Park, a large native population in the southwestern USA, offer a unique opportunity to evaluate population patterns and processes in a remote riverine system with ongoing anthropogenic impacts. We integrated non-invasive, invasive, and citizen-science methods to address questions on abundance, distribution, disease status, genetic structure, and habitat fragmentation.
Methods: We compiled bighorn sightings collected during river trips by park staff, commercial guides, and private citizens from 2000–2018 and captured bighorn in 2010–2016 to deploy GPS collars and test for disease. From 2011–2015, we non-invasively collected fecal samples and genotyped them at 9–16 microsatellite loci for individual identification and genetic structure. We used assignment tests to evaluate genetic structure and identify subpopulations, then estimated gene flow and recent migration to evaluate fragmentation. We used spatial capture-recapture to estimate annual population size, distribution, and trends after accounting for spatial variation in detection with a resource selection function model.
Results and discussion: From 2010–2018, 3,176 sightings of bighorn were reported, with sightings of 56–145 bighorn annually on formal surveys. From 2012–2016, bighorn exhibiting signs of respiratory disease were observed along the river throughout the park. Of 25 captured individuals, 56% were infected by Mycoplasma ovipneumoniae, a key respiratory pathogen, and 81% were recently exposed. Pellet sampling for population estimation from 2011–2015 yielded 1,250 genotypes and 453 individuals. We detected 6 genetic clusters that exhibited mild to moderate genetic structure (FST 0.022–0.126). The river, distance, and likely topography restricted recent gene flow, but we detected cross-river movements in one section via genetic recaptures, no subpopulation appeared completely isolated, and genetic diversity was among the highest reported. Recolonization of one large stretch of currently empty habitat appears limited by the constrained topology of this system. Annual population estimates ranged 536–552 (95% CrI range 451–647), lamb:ewe ratios varied, and no significant population decline was detected. We provide a multi-method sampling framework useful for sampling other wildlife in remote riverine systems.
Riverine habitats are a unique form of terrestrial systems, offering striking contrast in topography, structure, resources, and species diversity compared to surrounding areas, particularly in arid landscapes (Free et al., 2015). Rivers often provide access to water and concentrated resources even in desert or semi-desert ecosystems, along with steep and sometimes nearly continuous topography due to erosion on long time scales (Free et al., 2013). Occasional high-water flows further influence biodiversity (Leigh et al., 2010), but riverine ecosystems are often markedly narrow and linear, sometimes dendritic: therefore, terrestrial species with limited dispersal capability may be bisected by the water itself or the unique habitats associated with the river (Varty, 1990; Dudgeon, 2000; Fagan, 2002; Wiens, 2002; Nilsson et al., 2007; Leigh et al., 2010; Free et al., 2013; McCluney et al., 2014; Thorp, 2014; Free et al., 2015; Naka and Pil, 2020). Thus, species taking advantage of belts of riverine habitat may be subject to particular spatial constraints, leading to, for instance, increased importance of stepwise gene flow or mismatches between the geometry of dispersal and the geometry of disturbance (Fagan, 2002). An important interacting characteristic of species using riverine systems is that water frequently facilitates human visitation, leading to high levels of anthropogenic impacts such as rapid resource extraction or impacts of flow regulation by dams in otherwise remote systems (Leigh et al., 2010; McCluney et al., 2014). Yet, rivers are often the only practical means for accessing and assessing wildlife species in such remote areas. Collecting sufficient data to understand population dynamics and threats poses a unique challenge in such environments due to limited access and sampling opportunities along a relatively narrow corridor.
Many species occur in both riverine and non-riverine systems. Species such as hippopotamus (Hippopotamus amphibius), American beavers (Castor canadensis), and river otters (Lontra canadensis) are not solely restricted to rivers, but populations in riverine systems may exhibit fundamentally different patterns of population structure, movement, and distribution (Crawford et al., 2008; Latch et al., 2008; Stears et al., 2019). In these linear or dendritic distributions, riverine wildlife susceptible to disease and other stressors may have increased vulnerability to fragmentation and local extirpation of subpopulations with recolonization potential limited by constraints of the riparian corridor (e.g., platypus Ornithorhyncus anatinus, Bino et al., 2020). Indeed, for both terrestrial and aquatic species, observed and theoretical metapopulation dynamics in riverine systems have received much research attention because of their particular topologies (e.g., Bellard and Hugueny, 2020), with one study even concluding that classical metapopulation dynamics are more likely in dendritic networks (Fronhofer and Altermatt, 2017).
Bighorn sheep (Ovis canadensis), a large terrestrial herbivore, are strongly tied to steep, treeless topography in western North America. Bighorn locate predators visually and use steep, rocky slopes as escape terrain (e.g., McKinney et al., 2003). As such, bighorn are typically found in three types of landscapes: high alpine areas above tree line (e.g., the mountains of the Sierra Nevada California, Teton Range of Wyoming), isolated island-like mountain ranges in desert or sage brush steppe (e.g., Mojave Desert habitats in California, eastern Oregon), and canyon habitats along rivers that are too steep or arid to support trees (e.g., Hells Canyon in Oregon, Idaho, Washington; Dinosaur National Monument in Colorado). Bighorn sheep are often managed as metapopulations (Bleich et al., 1990; Singer et al., 2000), and population dynamics, metapopulation structure, and disease transmission have been well described in alpine (Johnson et al., 2010) and island-type systems (Epps et al., 2004; Creech et al., 2014; Dekelaita et al., 2020, Dekelaita et al., 2023), but these processes are less well understood in riverine systems, with one exception. Population and disease dynamics have been well described in the reintroduced Hells Canyon metapopulation for the Rocky Mountain subspecies (Ovis canadensis canadensis, Cassirer and Sinclair, 2007; Cassirer et al., 2013). However, population and disease dynamics in large riverine systems of desert bighorn sheep (O. c. nelsoni), or in native riverine metapopulations of bighorn, have not been systematically evaluated.
Grand Canyon in northern Arizona, a relatively isolated, deep canyon bisected by the Colorado River, holds what is likely the largest remaining example of native (i.e., not reestablished by translocation) desert bighorn populations in a riverine system, albeit one that has been heavily influenced by anthropogenic change. Located primarily within Grand Canyon National Park (hereafter park), desert bighorn in this population play important roles in the ecology of the canyon as large herbivores and as sources of food for predators and scavengers. They are also an important cultural resource, considered an iconic symbol of the desert southwest in both traditional native and modern contexts. Bighorn are largely restricted to the rocky slopes below the relatively flat or tree-lined rim of the canyon, which is generally oriented along an east–west gradient with numerous side canyons carved out by ephemeral and perennial streams. Historically, the Colorado River exhibited large seasonal fluctuations that likely allowed opportunities for wildlife species such as bighorn to regularly cross over between the south and north side during low flows (Bendt, 1957). Since the completion of the Glen Canyon Dam in 1963, river flows in Grand Canyon have been held relatively constant, resulting in an abundant, perennial water source that appears to form a landscape barrier for some terrestrial vertebrates, as much of the river is now wide and deep year-round. For some species, flow stabilization could have caused greater population structuring or unoccupied habitats along the east–west gradient due to numerous insurmountable cliffs that can no longer be bypassed by movements across the river (e.g., Stevens, 2012). Desert bighorn are adapted to arid conditions, but are limited by available surface water and vulnerable to drought over most of their range (e.g., Epps et al., 2004; Bender and Weisenberger, 2005). Here, while undoubtedly benefiting from access to perennial water, bighorn may now be constrained to varying degrees by the combination of river and topography, resulting in a linear distribution of desert bighorn. Yet, population trends, habitat use, genetic and demographic structure, or and disease exposure are unknown—indeed, no reliable population estimates have been made in Grand Canyon, despite several assertions regarding abundance from previous attempts to monitor and estimate numbers (Bendt, 1957; Guse, 1975; Wilson, 1976; Walters, 1979).
Disease dynamics are of particular concern, as bighorn are susceptible to outbreaks of respiratory pneumonia caused by pathogens transmitted from domestic sheep and goats and then passed among bighorn (Besser et al., 2008, Besser et al., 2012). Spatial structuring of populations may strongly influence dynamics of respiratory disease (e.g., Cassirer et al., 2018; Dekelaita et al., 2020). In island-like systems of bighorn, webs of connections among populations maintain genetic diversity but can facilitate disease transmission, while natural fragmentation accelerates genetic drift but may allow some populations to escape significant disease impacts in some years (e.g., Spaan et al., 2021). Yet, high genetic diversity in well-connected native systems, particularly those with multiple connections per population as in some arid montane regions, may ameliorate impacts of disease outbreaks (Dugovich et al., 2023). Bighorn in riverine systems have proved highly vulnerable to respiratory disease, even when metapopulation structure exists, particularly in restored populations such as Hells Canyon (Cassirer and Sinclair, 2007). Near Grand Canyon, recent respiratory disease outbreaks in desert bighorn have occurred in eastern California, southern Nevada, and northern Arizona (Kamath et al., 2019; Shirkey et al., 2021). Determining whether this population is spatially structured, the impact of such structure on genetic diversity, and describing connectivity and potential for disease spread into, out of, and within Grand Canyon is an important component of anticipating long-term consequences of disease.
Here, we present the first systematic assessment of bighorn in Grand Canyon, using annually repeated sampling along the river corridor, which we use to refer to an area within a short walk or view of the river. Research and monitoring of bighorn in Grand Canyon have been limited due to the challenging logistics of the canyon environment, including a nearly complete lack of roads, few trails, limited access points into the rugged terrain, and highly restricted ability to conduct survey flights due to steep terrain, low sightability of animals across a massive landscape, and preservation of wilderness and soundscape values. We integrated observational methods, GPS collar data, disease sampling, non-invasive genetic sampling, and novel variations on statistical techniques address the following questions: 1) Is the distribution and population structure of desert bighorn sheep in Grand Canyon continuous, given the continuity of steep topography along the river, or is the distribution fragmented into discrete subpopulations by distance, topography, or other habitat elements? 2) If the population is fragmented, are those subpopulations strongly divergent or linked by gene flow? 3) Is the Colorado River currently a barrier to movement and gene flow, and potentially pathogens, after changes in flow regulation in the last 55 years? 4) Are key respiratory pathogens present in this population, and, if so, are they distributed throughout the system or isolated by population substructure? 5) Can we apply spatially explicit models in this linear system to provide population size and uncertainty estimates? To those ends, we collated citizen science and park survey data, captured animals and tested for disease, characterized habitat use and movement, identified individual animals from fecal samples, estimated population size, quantified characteristics associated with variation in density, evaluated components of sampling related to detection of individuals, assessed whether a detectable trend in population size occurred during sampling, described genetic structure, diversity, and gene flow at multiple time scales, and evaluated the barrier effects of the Colorado River. Understanding these processes would support actions to conserve wide-ranging species of concern in arid environments increasingly affected by climate change, facilitate landscape-scale conservation efforts, and provide a benchmark for understanding future changes, particularly given the previous lack of comprehensive population and disease assessment in this riverine system, while informing studies of other species in anthropogenically-influenced riverine systems.
Grand Canyon is an isolated, 2.4 km-deep (1.6 km average), 28 km-wide (16 km average) canyon on the southern Colorado Plateau in northern Arizona that spans nearly 450 river kilometers and is located at the geographic limits of the Great Basin, Mojave Desert, and Sonoran Desert. Grand Canyon consists of plateaus and other comparatively flat topography incised by shallow drainages on the plateaus and steep canyons leading into the Colorado River gorge. On the rims, natural surface water is uniformly scarce, but sparsely distributed springs and seeps serve as perennial sources of water in the inner canyon. Collectively, the area includes approximately 6,750 km2 of relatively steep terrain below the rims of Grand Canyon. Our study area primarily focused on 364 km of the river, which started at Lees Ferry (river mile (RM 0) and ended at Diamond Creek (RM 226) but included light-intensity sampling and observations along another 85 km of river, terminating at Pearce Ferry on Lake Mead (RM 279). We use RM (Gushue, 2019) to denote location (instead of km) due to the widespread, universal use of this nomenclature for the Colorado River in Grand Canyon. Similarly, given the meandering nature of the river, we use the term river left (RL) or river right (RR) to denote river side as when viewing downstream from RM 0 to RM 226.
Hoover and Glen Canyon Dams on the Colorado River create the nation’s two largest reservoirs, Lake Mead and Lake Powell, which roughly form the lower and upper ends, respectively, of Grand Canyon. The Grand Canyon is contained within the park and lands of the Hualapai Indian Tribe. The Havasupai Tribe, the Navajo Nation, and a mosaic of other federal land management entities. Elevations range from 600 meters along the canyon bottom, where the Colorado River flows, to over 2,400 meters on north rim of Grand Canyon (Figure 1).
Figure 1 Map of study area for Grand Canyon bighorn research, showing major rivers, sub-regions of Grand Canyon, river miles (RM), the area of steep topography generally considered to be within the physiogeographic boundary of the canyon system (shaded relief), location within the southwestern United States (inset), and land jurisdiction including Grand Canyon National Park (light green shading), tribal lands (tan), including Navajo Nation, Havasupai, and Hualapai Indian Reservations (east to west), National Forest (blue), Bureau of Land Management (aqua), other National Park Service lands (red), and private and state lands in light blue or gray. Important locations and river miles (RM) noted in the paper are illustrated on the map.
Grand Canyon is geologically divided into the eastern and western basins (Billingsley and Hampton, 1999), separated by the steep, cliff-bound Muav Gorge that serves as the primary upstream and downstream barrier for dispersal of several plant, invertebrate, and vertebrate taxa (Miller et al., 1982; Phillips et al., 1987; Stevens and Polhemus, 2008). River flow rates change depending on daily discharge, which varies in response to hydropower demand and river reach, averaging 226–340 cubic meters per second (8,000–12,000 cubic feet per second). The rims of Grand Canyon routinely experience snowfall in winter. In contrast, the lower levels of Grand Canyon near the river rarely drop below freezing (January minimum of 2.4°C), are hot during the summer (mean July maximum of 42.0°C), and receive an average of only 225 mm of precipitation. Precipitation occurs throughout the year except during the strong spring drought during May and June, with highest precipitation during a summer monsoonal period from July through September.
Below the canyon rim, Mojave and Sonoran Desert scrub communities dominate the lowest elevations, rising to more cold-tolerant species indicative of Great Basin Desert communities. Along the riparian zone, vegetation structure has been modified since the completion of the Glen Canyon Dam, which generally has prevented floods that used to scour the river channel and limit plant colonization (Sankey et al., 2015). Stabilized water flows have since resulted in dense vegetation along the shoreline (Sankey et al., 2015), frequently dominated by nonnative tamarisk (Tamarix ramosissima). Above the shoreline zone, vegetation composition varies with aspect and solar radiation (Stevens, 2012), but is dominated by species including honey mesquite (Prosopis glandulosa var. torreyana), catclaw acacia (Seneglia greggii), brittlebush (Encelia farinosa), creosote (Larrea tridentata), and numerous yuccas and cactuses. More than 300 plant species have been described within Grand Canyon.
Bighorn in Grand Canyon are most commonly observed along the river corridor. Since the 1950’s, anecdotal observations of bighorn along the river were periodically collected by park and commercial (tourism) river companies; for decades, this was the primary source of information about the population. In 2000, park staff began to work with private and commercial river guides to report bighorn sightings in a standardized way, reporting sex/age class, group composition, river mile, and river side. We generated location coordinates at the river mile intersection with the modeled shoreline for 20,000 cfs (566 cms) flows. We used 2000–2018 bighorn demographic data collected by park, commercial, and private river trips across all seasons to inform numbers and distribution of bighorn along the Colorado River through the park. Data collected between 2000–2010 provided a more comprehensive assessment of bighorn distribution along the river than was previously available and helped guide our study beginning in 2010. Bighorn congregate along the river during the breeding season (August–October) and are most concentrated during that time. Thus, in 2010, we began counting and classifying bighorn during September bighorn-specific river trips. Three to four experienced observers surveyed for bighorns on each trip, but depending on the timing of the observation relative to the position of the raft and the ability of the boat operator to stop in that location, the time for counting and classifying a group of bighorn varied, and observers worked together to document all sightings. Detectability of bighorns from the river via visual observations may vary based on time of day, temperature, terrain ruggedness, and activity level, and robust population estimates from this approach would require independent double observations or large numbers of marked animals. However, this can provide insight to distribution over time and classifications provide an index to reproduction with enough observations. In 2011, we broadened the study to include information on disease surveillance and genetic structure. River trips usually consisted of four biologists and one boat operator in a single 22-foot motor raft that allowed us to hold position except in the largest rapids, but did not allow efficient upriver movement. In 2011 we had 2 boats. In total, we made seven annual river trips between 2010 and 2016, with each lasting approximately two weeks. Demographic counts continued through 2018, by which time all collared bighorn either dropped their collars or died.
We recorded all observations of bighorn with symptoms of respiratory disease and mortality. We classified bighorn as sick if they expressed clinical signs of respiratory complications including nasal discharge, coughing, and labored breathing, and we investigated sick or dead bighorn reported by commercial river trips when feasible from 2010–2018 (Supplementary Tables A1, A2). To determine current and prior exposure to disease pathogens, we collected antemortem diagnostic samples from bighorn captures from 2013–2016 (see below), and postmortem diagnostic samples were collected from recent mortalities when feasible from 2011–2018. For captured bighorn, approximately 20 mL of blood was collected into serum-separating tubes as well as a 5 ml tube with EDTA for whole blood preservation for DNA work and kept on ice while in the field; after finishing each river trip, serum-separating tubes were immediately spun to extract serum which was then frozen. For captured bighorn and relatively intact carcasses, nasopharyngeal swabs were used to collect nasal and tonsillar samples, stored in tubes with tryptic soy broth (TSB) buffer in glycerol, and frozen. Serum and swabs were analyzed by Washington Animal Disease Diagnostics Laboratory (WADDL) at Washington State University: swabs were tested by PCR for evidence of infection by Mycoplasma ovipneumoniae, a key respiratory pathogen, while ELISA was used on serum samples to test for presence of antibodies to M. ovipneumoniae and was used as a metric for exposure. Strain typing for M. ovipneumoniae was conducted on a subset of samples by WADDL as described by Kamath et al. (2019). On a subset of samples, the Colorado State Veterinary Laboratory conducted PCR tests for other respiratory pathogens, including Biberstenia trehalosi and Mannheimia (Pasteurella) haemolytica. Although respiratory pneumonia in bighorn is a polymicrobial disease, M. ovipneumoniae has been identified as a primary causal agent (Cassirer et al., 2018).
We fitted GPS collars to bighorn to characterize habitat use and movement and to inform the population estimation model. We captured bighorn from 2010–2016 along the Colorado River in Grand Canyon using river- and ground-based darting techniques during the same river trips described above. River-based captures involved darting bighorn from the boat in eddies or slow-moving areas of the river where distance to the animal was less than 30m. Ground-based captures involved approaching and darting bighorn from the shoreline or farther from the river if darting could not be accomplished safely from the boat. Capture efforts were concentrated during fall when bighorn tend to congregate along the river, and to reduce temperature- and gestation-related stress during captures. We attempted to capture animals equally on either side of the river, and spatially distributed throughout the river corridor, except where suitable habitat or shoreline access was absent.
Bighorn were immobilized with BAM, a pre-mixed combination of Butorphanol (B), Azaperone (A), and Medetomidine (M), with a dosage of 0.50 mg/kg B, 0.17 mg/kg A, and 0.20 mg/kg of M. Bighorn were aged and classified based on annual horn rings and time of year (Elbroch, 2006). We fitted bighorn with GPS-satellite uplink (Telonics TGW-4583) or GPS-direct download (Telemetry Solutions Quantum 5000) collars programmed to collect GPS fixes every four hours and equipped with a mortality sensor and a programmable drop-off mechanism. Locations of marked bighorn were transmitted via Argos or Iridium satellite uplink or downloaded directly via UHF antenna during aerial surveys. Bighorn captures were approved by the National Park Service (NPS) Institutional Animal Care and Use Committee (IMR_GRCA_Holton_Bighorn Sheep) and the park (GRCA-2011-SCI-0018, GRCA-2012-SCI-0020, GRCA-2013-SCI-0057, CE-2010-2015 PEPC-21840, CE-2016-2021 PEPC-62989).
We created two genetic datasets to address different purposes. For the primary dataset, used for population estimation, we obtained DNA via non-invasive sampling of fecal pellets during annual river trips in September–early October from 2011–2015. Where accessibility allowed, we collected fecal pellets by 1) searching at locations where bighorn routinely access water, such as the mouths of side drainages, 2) searching where bighorn were observed from the boat, 3) searching where bighorn tracks were observed at the river’s edge, and 4) opportunistic searching at river camps. In the first 3 years, searches of shorelines and adjacent areas (canyons and hills) for fecal pellets was a mix of systematic stops at any promising and accessible location or in response to observations of bighorn or tracks, but we also identified 74 high-priority planned search areas after 2013. These sites were distributed more evenly along the canyon than initial sampling and were either at side canyon–river junctions likely to funnel bighorn or at places with consistent observations in initial sampling years. We stopped at 43 of these in 2014 and 56 in 2015. Samples ranged in age from fresh to those estimated to be up to 2 weeks old based on pellet condition and tracks. Pellet condition, date, UTM location, and river side were noted for each sample, which were collected by gloved hands or using sticks to prevent contamination and placed into a paper envelope. Samples collected wet from freshness or light rain were dried by placing envelopes in direct sun, or by placing envelopes into a pot of dry sand and heating on a camp stove until no moisture was detectable through the envelope; samples were then stored in the laboratory in dry, dark conditions at room temperature.
We used the second genetic dataset for estimation of genetic structure, gene flow, and river crossings (hereafter, “combined” dataset). This dataset was created by merging the primary dataset for population estimation (9 microsatellite loci for 1,250 samples; see Results) with data from samples collected from 2011–2013 and genotyped at 16 microsatellite loci including the 9 used for population estimation (see details in Creech et al., 2017, Creech et al., 2020). Like the primary dataset, that effort relied on non-invasive sampling of fecal pellets, but also included a small number of tissue and blood samples from live captures, euthanized animals, or carcasses found in the field.
We used a modified AquaGenomic Stool and Soil protocol (MultiTarget Pharmaceuticals LLC, Salt Lake City, UT) to extract DNA from material scraped from the surface of fecal pellets. The primary dataset (population estimation) was genotyped at a single panel of 9 microsatellite loci and a sex-identification marker to allow individual identification (see Pfeiler et al., 2020 for details on markers and amplification conditions). The primer pair used for sex identification amplifies the amelogenin gene located on both the X and Y chromosomes (Yamamoto et al., 2002); in bighorn, the Y chromosomal fragment exhibits a 44-base-pair (bp) deletion relative to the X chromosome (214 bp vs. 258 bp). The Creech et al. (2017) dataset was genotyped at 16 dinucleotide microsatellite loci in three multiplex PCRs of 4–6 loci using a Qiagen Multiplex PCR kit (Qiagen, Valencia, CA) and included numerous samples also used in the primary dataset. We submitted PCR products to the Center for Quantitative Life Sciences at Oregon State University for analysis on an ABI 3730 capillary sequencer (Applied Biosystems [ABI], Foster City, CA, USA) and used GENEMAPPER (version 4.1; ABI) to score genotypes. Each sample was amplified in at least three replicate PCRs to generate consensus genotypes. Samples that produced ≥3 alleles at any locus were eliminated. Samples that produced partial genotypes at ≥50% of the microsatellite loci were subjected to 3 more attempted amplifications. For a genotype to be accepted, each allele in a heterozygous genotype had to be observed twice, while the single allele in a homozygous genotype had to be observed 3 times.
We used CERVUS version 3.0.3 (Kalinowski et al., 2007) to identify duplicate genotypes, i.e., those derived from different fecal samples from the same individual bighorn, and used GIMLET version 1.3.3 (Valière, 2002) to estimate genotyping error rates (false allele occurrence rate and allelic dropout rate). We used a maximum probability of identity (PID) of 0.01 for unrelated individuals and 0.05 for full siblings (PIDsibs), and on that basis determined the minimum number of loci (working from least to most informative) needed to be amplified for a genotype to be used. Duplicate genotypes in the primary dataset (9 loci) were identified for population estimation analysis. For the combined dataset, we used genotypes of unique individuals from the primary and Creech et al. (2017) datasets, checked for duplicates after combining them, and removed all but one sample from any individual detected multiple times, retaining the most complete genotypes or selecting at random. Any individual detected on both sides of the Colorado River at either stage was noted as evidence of river crossings. Thus, the combined dataset for evaluation of genetic structure consisted of unique individuals genotyped at either 9 or 16 loci. As Creech et al. (2017) tested these markers and found no evidence of linkage disequilibrium in this system after controlling for genetic structure, we used GENEPOP version 4.2 (Raymond and Rousset, 1995) to test for deviations from Hardy–Weinberg proportions on the combined dataset for genetic structure, after assigning individuals to populations, but did not reevaluate linkage disequilibrium (see below).
We used the combined dataset for analyses of genetic structure and gene flow. Because the distribution of bighorn within the park is discontinuous, but clear geographic boundaries other than the Colorado River were largely not apparent, we used STRUCTURE (Pritchard et al., 2000) with no prior information on population or sampling location to describe clusters of genetically similar individuals. We used a burn-in of 50,000 iterations followed by 100,000 sampling iterations on 5 replicate runs, reviewing consistency across runs to determine that these lengths were adequate. We determined the largest number of clusters diagnosable in the dataset using the “high assignment criterion” (HAC) approach, in which the largest supported value of K is that for which all clusters have at least 1 individual assigned to them at high confidence (Epps et al., 2018). We used CLUMPP to average assignment probabilities (q values) across replicate runs and used a cutoff of q > 0.90 when applying the HAC approach. We also plotted ΔK values (Evanno et al., 2005) against K to evaluate whether peaks at higher K values occurred.
After determining the largest supported value of K, we mapped average assignment probabilities for each sampled individual, choosing at random among multiple locations at which any individual was detected if observed more than once. We then defined populations spatially based on concentrations of samples assigned to coherent clusters, defining them by side (RR or RL) and RM. We reviewed population assignments, observations of bighorn (2011–2018), locations of all genotyped samples, and GPS collar data to guide choices of divisions along each river side, seeking to identify gaps in bighorn distribution where assignment values differed. We used those populations as the basis for subsequent population-based analyses. We tested for migrants among those spatially defined populations using the average q values from STRUCTURE analyses, defining migrants as any animal assigned at q > 0.9 to another cluster.
We evaluated genetic structure among those populations by estimating FST using GENEPOP (Raymond and Rousset, 1995). We also tested for evidence of additional structure or other processes such as selection by testing for deviation from Hardy–Weinberg Proportions using the probability test in GENEPOP by population and locus, using default settings for Markov chains.
Finally, we estimated recent gene flow (previous generation) using BIMr (Faubet and Gaggiotti, 2008) among those spatially defined populations. We tested two environmental factors that might influence migration among populations: 1) whether populations were on the same or different sides of the Colorado River and 2) distance, measured along the river from the center of each spatially defined population, where center was defined as the middle point of that stretch of river. We used 10 replicate runs with 20,000 iterations for burn-in and 20,000 iterations for parameter estimation, with other model settings as default. We evaluated posterior model probabilities for 4 models: 1 for each environmental factor, 1 with both factors, and 1 with both factors and an interaction term. We used mean migration rates for each population pair estimated from the run with the lowest Bayesian deviance as a comparison with patterns of migration inferred from STRUCTURE.
Estimating population size posed a particular challenge. Many traditional methods to estimate population size require even sampling across a population and multiple sampling events within a short time window. In the last two decades, spatial capture–recapture methods that allow for a heterogeneous distribution of sampling and integration of data sources have expanded options to estimate population size and understand the role of habitat on distributions simultaneously (Borchers and Efford, 2008; Royle et al., 2013a, Royle et al., 2013c). Ongoing developments now support estimates for wildlife distributed along linear features, such as rivers (Royle et al., 2013b; Fuller et al., 2016; Sutherland et al., 2018), and the use of a single annual sampling session (Morin et al., 2018; Schmidt et al., 2022).
We used a spatial capture–recapture (SCR) model to estimate population size of Grand Canyon bighorn (e.g., Royle and Young, 2008). SCR models detection using a function where the probability of detection declines as a function of distance between the coordinates of a trap, j, and an activity center, s, of an individual animal, i. The activity center is a location, with coordinates, that is analogous to a home range center for a particular period of time, which here likely comprises a few weeks before sampling as the DNA from pellets decays over time. The activity center is a latent variable, that is estimated in this model based on a grid of points (the state space) that describes the potential activity centers considered possible during modeling. We used the half-normal detection function:
This model thus contains 3 parameters, namely density and 2 parameters describing detection, the baseline encounter probability, p0, and the spatial scale parameter, σ, which determines the rate of decrease in detection probability with the distance between the trap location, xj, and the activity center for an individual, si. The incorporation of a spatial model for detection allows estimation of detection parameters even with a single detection session per year. We used the approximate place where the boat stopped for the search for pellets to define the trap location and assigned detections of individuals to the trap closest to the pellet collection coordinates. Potential activity centers (the state space) had a spacing of 1 km and included a buffer of 5 km from the river where sampling occurred but extended farther in places to include the entire park.
We summarized sample sizes and calculated the mean maximum distance and the maximum distance among individual detections, confirming >30% of recaptured animals had spatial recaptures (Schmidt et al., 2022) and assessing for extreme distances moved across detected individuals to prevent unusual movements from biasing the σ estimate (Kendall et al., 2019).
We fit models in the software environment R (R Core Team, 2023), conducting model selection using the package oSCR (Sutherland et al., 2018). For each parameter, we fit univariate models to ensure variables captured the intended ecological effect, compare similar covariates (e.g., two hypotheses describing temperature), and understand univariate effect size and direction. We calculated pairwise correlations and retained only variables with correlations < 0.7 (Dormann et al., 2013). Because density was our primary interest, we considered detection as nuisance parameters and first identified best models for p0 and σ, using those in all hypothesized density models. For each model component (p0, σ, and density), we compared all possible combinations of the reduced variable set using Akaike’s Information Criteria (AIC, Burnham and Anderson, 2002).
We assessed constant, sex-specific, year-specific, and the combination of sex and year-specific variation in σ. We evaluated several hypotheses for influences on baseline detection: trap type and observer, plus trap covariates reflecting temperature in days before sampling and habitat conditions. For trap type, we competed hypotheses for categorizing trap type based on the initial reason for the stop (bighorn sign seen, targeted search location, bighorn seen, capture location, and opportunistic) comparing 2–5 categories. We tested the effect of the presence of two lead observers. We assessed temperature across the previous three and five days because warm days could drive bighorn to move closer to the river and increase detection. Habitat covariates summarized the area within 500 m of the trap site that we tested and included mean slope, maximum slope, terrain ruggedness, measured as the standard deviation of curvature (Ironside et al., 2018), exponentiated terrain ruggedness to account for neighboring steep cliffs, and summaries of habitat selection around the trap.
Because we expected more bighorn activity centers and higher detection of bighorn in places that they used more frequently, we developed a resource selection function (RSF) based on GPS locations from September, the month of pellet sampling, to use for detection and density covariates in the SCR model. Because this coincides with the breeding season when bighorn occur in mixed-sex groups and generally use the same areas, we included both sexes in a single model. We evaluated selection within a boundary that included the park plus the length of the Colorado River from Lees Ferry to the Diamond Creek takeout. Within that study area, we assigned each 30 m pixel as either used or unused depending on the presence of a telemetry point. We randomly assigned each of the unused sites proportionally to an individual. Thus, every location in the study area was included to completely describe the habitat in the analysis. We fit logistic models with a random intercept for individual, weighting each location based on the probability of fix acquisition, a spatially explicit probability based on topography that mitigates for the low fix success of some individuals (Ironside et al., 2018; Graves et al., 2024). We calculated aspect [northness = cos(aspect), eastness = sin(aspect)], the standard deviation of curvature and its quadratic, elevation and its quadratic, and solar radiation during the month of September as well its quadratic. We defined additional covariates based on the distance to a feature, namely distances to escape terrain >40° slope, to helicopter overflight paths, to trails, and to the river. For each distance covariate we also assessed a model with the effect of distance log-transformed, which can effectively model a threshold effect. After removing correlated variables, we fit univariate models. We eliminated the distance to helicopter covariate because the coefficient suggested attraction to helicopters, which is unlikely (Bleich et al., 1994), suggesting this was a spurious correlation (Wisdom et al., 2020). We fit a global model with all remaining variables. To use the RSF covariate as covariates on detection in the SCR model, we extracted mean and maximum values of predicted selection for each trap (location where boat stopped), competing linear and quadratic forms of RSF variables.
For the density portion of the model, we evaluated whether the mean or max of September RSF in a 500m radius, distance to the river, or both would best constrain activity centers to areas used by bighorn. We used binary variables to assess whether density changed inside the park boundary or in areas where domestic sheep grazing was possible based on land ownership. We considered three temporal model forms for changes in density: no time dependent covariate, linear yearly change, and year-specific density. We also assessed sex-specific variation in density.
Following model selection, we refit the best model from the maximum likelihood approach using a Bayesian approach to get credible intervals for estimated abundance (Woodruff et al., 2021), as detailed in Supplementary Appendix 1. Abundance is a derived parameter based on spatially explicit density estimates. This model did not have separate intercepts for density by year, nor did it incorporate a trend term for density, as those model structures were less supported in model selection (see Results). The Bayesian model combined 1) delineation of individual activity centers across an inhomogeneous state space with covariates modeling the spatial variation in population density, 2) an observation model with categorical covariates to allow for variation in detection probabilities across J traps and K sessions, and 3) data augmentation to model the true population size. To implement data augmentation, we included an excess of all-zero encounter histories for individuals that were not detected during sampling but could be present in the population, M=1,200 individuals (Royle and Young, 2008; Royle et al., 2018; Woodruff et al., 2021). Our formulation assumed activity centers, s, for each individual, i, did not change over these 5 years. It also assumed the relationship of covariates with density was constant over time. We fit the Bayesian model using NIMBLE in the statistical computing environment R v.4.3.0 (de Valpine et al., 2017, de Valpine et al., 2023; R Core Team, 2023) and carried out 5 chains of MCMC sampling over 20,000 iterations with no thinning, of which we excluded the first 5,000 iterations for adaptation and burn-in.
From 2010–2018, we summarized 1,047 unique observations of bighorn groups, totaling 3,176 sightings of individuals, recorded on 94 river trips (private, commercial, and bighorn survey) to describe bighorn distribution along the river (Figure 2). Bighorn counts averaged ~30 individuals per trip and were recorded between river miles 1 and 261. Overall patterns of distribution during this period appeared the same as the longer time series including pre-study dates (2000–2018). Our dedicated seven bighorn survey trips along the river (RM 0–RM 226) recorded 165 unique observations of bighorn groups totaling 535 bighorn sightings, and under the assumption that bighorn did not move down-canyon faster than our boats, yielded minimum counts that declined from 145 individuals (2011) to a low of 56–61 individuals (2012–2014), before increasing to 88–92 bighorn in 2015–2016 (Table 1). The number of individuals detected via direct genetic identification of individuals from fecal pellets declined more slowly, from 134 in 2011 to 65 in 2013, before a rapid increase in genetic detections to 128 individuals in 2014 and nearly 200 in 2015, although the trap type varied substantially across years as did the numbers of individuals detected by trap type. For example, the number of traps where the reason for stopping involved seeing either bighorn or other sign varied from 17 to 49 across years. More stops in 2015 resulted in more samples collected. In contrast, fall lamb–ewe ratios generally declined from 50:100 in 2012 to an average of 24.3:100 from 2014–2016 (Table 1).
Figure 2 Distribution of bighorn observations (n = 1,047 observations of 3,176 bighorn) by park staff, members of the public, or commercial river guides along the Colorado River in Grand Canyon National Park (green shading), 2010–2018, depicted by river side: (A) river right, blue; (B) river left, red. Important locations and river miles (RM) noted in the paper are illustrated on the map.
Table 1 Number of bighorn sighted during river sampling trips, fall lamb:ewe ratios, number of fecal pellet samples collected during population estimation sampling, numbers of individuals inferred via genotype matching of fecal samples, and spatially explicit capture–recapture estimates of population size (N) with 95% credible intervals (CrI) based on the final model.
We observed bighorn with signs of disease beginning in 2012, when a collared ewe died at RM 6 on RR and a necropsy revealed bronchopneumonia, although a ewe captured in 2010 exhibited nasal discharge. Reports of animals with symptoms congruent with respiratory disease (hereafter “sick”; see Methods) increased after 2012 and the combination of observations of symptoms and diagnostic samples suggested that a widespread disease event occurred with the maximum number of sick (n = 11) and dead (n = 16) bighorn reports in 2014, ranging from RM 8–196 on RR and RM 79–216 on RL (Figure 3). The number of coughing and dead bighorn increased each year between 2012 and 2014 (Supplementary Tables A1, A2). From 2011–2016, non-native respiratory disease pathogens were detected in 77% of dead and captured bighorn sampled for disease diagnostics (Table 2). Testing of samples from collared bighorn indicated that 81% had been exposed to M. ovipneumoniae (serology) and 56% had active M. ovipneumoniae infections (PCR; Table 2). Most bighorn mortalities reported were not possible to investigate due to remoteness. Seven post-mortem investigations were completed, of which 2 yielded viable samples that both tested positive for M. ovipneumoniae, but most carcasses examined were too heavily scavenged or autolyzed to determine cause of death or test for M. ovipneumoniae or other pathogens (Supplementary Table A2). Strain typing for M. ovipneumoniae, conducted by WADDL on 3 samples from collared animals at 4 loci (16S, gyrB, rpoB, IGS), indicated that 2 strains were detected in Grand Canyon during this study, none of which has been detected outside Grand Canyon (WADDL, unpublished data).
Figure 3 Distribution of sick and dead bighorn along the Colorado River in Grand Canyon National Park, 2011–2018, depicted by river side: (A) river right; (B) river left. Red crosses indicate bighorn mortalities where respiratory disease was either confirmed or suspected. Blue triangles indicate observations of bighorn showing clinical signs of respiratory disease, including coughing and labored breathing. Important locations and river miles (RM) noted in the paper are illustrated on the map.
Table 2 Disease testing results and disposition for bighorn captured or sampled at Grand Canyon 2011–2016.
Between October 2010 and September 2016, we captured 25 individual adult bighorn (16 female and 9 males) between RM 6 and 214. Mean age across all sexes was 5.6 years. Estimated age of females at capture ranged from 3.5–7.5 years old (n = 16, mean = 5.5 years old) and males ranged from 2.5–8.5 years old (n = 9, mean = 5.9 years old). We captured 14 ewes and 5 rams on RR, and 2 ewes and 4 rams on RL (Figure 4). All captured bighorn were collared except one ewe on RR. Two collars did not acquire GPS fixes and were not included in the RSF analysis but were monitored via VHF for 627–832 days. Collars with functioning GPS (n = 22) averaged 480 days (range = 178–754 days) and accumulated 10,560 collar-days (one collar day = one bighorn wearing a collar for one day) with 25,700 GPS fixes (Supplementary Figure A1; Graves et al., 2024). Overall GPS fix success rates were poor, averaging 0.33 of programmed acquisitions (range = 0.07–0.72). Bighorn typically inhabit regions in the park with complex rugged topography and narrow sky views that constrain GPS fix success rates (Ironside et al., 2017). Although GPS fixes on individual collared bighorn were occasionally acquired on the opposite side of the river, these were single location events, and determined to be associated with GPS error based on topography, poor sky view, and pre- and post-locations.
Figure 4 Distribution of GPS-collared bighorn (n = 25) along the Colorado River in Grand Canyon National Park, 2010–2018, depicted by river side: (A) river right; (B) river left. Colored dots indicate individual bighorn. Collared bighorn generally moved along the river, creating relatively linear distributions of locations, and no collared bighorn were documented crossing the river. Insets (right panels) show distribution of GPS-collared bighorn by river side within the RR-Mid and RL-Mid populations, near the junction of Kanab Creek with the Colorado River. Six bighorn sheep were detected genetically on both sides of the river; all were sampled within RM 136–141 (inset, exact sample locations not shown). Important locations and river miles (RM) noted in the paper are illustrated on the map.
For the global September RSF model, distance to the river, distance to escape terrain, and northness had the largest effects, with all covariates statistically significant (Table 3). Bighorn had higher use of areas closer to the river closer to escape terrain, on southerly and easterly aspects, and in areas with moderately low variation in curvature and medium amounts of solar radiation (Supplementary Figures A2–A4). Nearly 60% of September GPS locations were <500 m from the river, ~83% were <1 km from the river, and >90% were <1.5 km from the river.
Table 3 Odds ratios and confidence intervals for the global resource selection function model for bighorn sheep in September in Grand Canyon National Park, 2011–2017.
For the population estimation dataset, we collected 1,539 fecal pellet samples (Supplementary Figure A5; Graves et al., 2024). We successfully genotyped 1,250 samples at sufficient loci (4 minimum; Table 4) to resolve individual identity, yielding 453 individuals (Table 1). After merging that dataset with the Creech et al. (2017) dataset (n = 262 individual genotypes, of which 208 were already represented within the population estimation dataset), the final combined dataset included 529 individuals, of which 257 individuals were genotyped at up to 16 loci (yielding 224 individuals with at least 15 loci resolved) and 273 were genotyped at up to 9 loci (yielding 233 individuals with 9 loci resolved); the full distribution of locus number and genotypes used for estimation of genetic structure is presented in Supplementary Figure A6.
Table 4 Number of alleles (k), sample size (n; number of individuals genotyped at that locus in the population estimation dataset/combined data set), observed (Ho) and expected (He) heterozygosity (calculated for all samples treated as one population), probability of identity (PID), probability of identity-siblings (PIDsibs), and cumulative PID and PIDsibs for 9 loci used for individual identification of 453 bighorn sheep from fecal DNA samples collected during capture–recapture sampling in Grand Canyon, Arizona.
Based on individual identification from genotypes, we detected only 6 (3 male, 3 female) bighorn on both sides of the Colorado River, all between RM 136–141 (Figure 4). All other redetections occurred on the same sides of the river and distances between individual redetections across the population estimation dataset ranged from 0–38 km (Table 5; Supplementary Figure A7).
Table 5 Sample size and distance summaries for genetically detected individuals used in spatial capture recapture analyses of 787 bighorn sheep detections in the Grand Canyon during September boat trips, 2011–2015.
Estimates of the number of genetic clusters (K) using STRUCTURE with no informed priors on population or sampling location suggested K = 6 best represented structure within the data. At K = 6, the maximum q values ranged 0.97–0.98; at K = 7, the maximum q values per cluster ranged 0.77–0.98. Thus, applying the high assignment criterion, K = 6 was the highest supported value of K. ΔK values (Supplementary Figure A8) suggested divisions within the data primarily at K = 2, 3, and 6. At K = 2, the primary division in the data reflected river side (Supplementary Figure A9). After spatially defining populations based on individual assignments at K = 6 (Figure 5), tests for Hardy–Weinberg proportions indicated that with Bonferroni correction for 16 tests, only one locus from the 16-locus data showed consistent violations. That locus, BL4, showed evidence of selection in another bighorn study area (Epps et al., 2018), but we retained it in this analysis. Of the six clusters, described hereafter as subpopulations, only 1 subpopulation (RL-East) showed 2 loci departing from HW proportions after Bonferroni correction for 16 tests; all other subpopulations showed 0 to 1 locus departing from HW proportions, suggesting that subpopulations we defined did not contain substantial additional substructure. Population genetic distance (FST) ranged from 0.022, between RR-Mid and RR-West subpopulations, to 0.126, between RL-East and RR-West (Table 6).
Figure 5 Assignment of individual bighorn sheep to 6 populations using 9–16 microsatellite loci genotype assignments with Program STRUCTURE. Cluster assignments, reflected in different colors were used to define populations spatially for population-type analyses of gene flow (e.g., Table 6). Individual assignment pie charts are staggered for visibility, and thus are not in their exact locations; because most samples were collected along the river, for clarity, samples are presented separately depicted by river side, (A) river right, (B) river left, even if depiction moves sample across river to avoid overlap. Multiple colors in a single dot indicate that individual was proportionally assigned to more than one cluster, potentially indicating recently mixed ancestry. Important locations and river miles (RM) noted in the paper are illustrated on the map.
Table 6 Sample size and genetic structure (FST, below diagonal) and migration rates from the previous generation (estimated using BIMr, above diagonal) among 6 populations of bighorn sheep defined spatially and by genetic assignment tests, as well as measures of genetic diversity [observed (Ho) and expected (He) heterozygosity], estimated using 9–16 microsatellite loci and 529 individuals in total.
Tests using STRUCTURE indicated migrants from both current and previous generations were detected among subpopulations on the same side of the river as well as among those separated by the river, particularly in the central section (Supplementary Table A3). Estimation of migration rates among subpopulations in the previous generation using BIMr best supported a model where both factors (distance along the river and the river itself) limited migration. The run with the lowest Bayesian deviance estimated the posterior probability of the two-factor model at 0.75, compared to 0.10 for the null model with neither factor, 0.05 for the river alone, and 0.11 for distance alone; the model with river and distance was strongly supported in all 10 replicate simulations (mean posterior probability of the river and distance model was 0.72, range: 0.43–0.92). Migration rates (estimated for previous generation) were highest among RR-Mid and RR-West subpopulations on the same side of the river, but a high migration rate also was observed across the river between the middle subpopulations (Table 6). Self-migration estimates clearly showed that both RR-Mid and RL-Mid subpopulations experienced a relatively high rate of migration (in the genetic sense) to and from other subpopulations (Table 6).
Spatial recaptures ranged from 14 to 35 individuals per year (Supplementary Table A4), suggesting sampling was sufficient to avoid bias (Schmidt et al., 2022), as sample size can influence bias and precision of population estimates from SCR approaches (Efford and Boulanger, 2019; Dupont et al., 2021). Because we used a single sampling session in each year, recaptures within sites did not exist in the analysis. We excluded one detection of an individual bighorn that was 38 km away from another detection (Supplementary Figure A7; Table 5), exceeding 5 standard deviations of the maximum distance, because rare extreme movements can bias estimates of σ (Kendall et al., 2019). We retained movements <20km in the same year because we detected the same animals at this distance across different years for multiple animals, suggesting that this was a regular movement pattern. The proportion of males detected genetically in the SCR dataset ranged from 39–61% (Table 1). Recaptures across years, albeit not used analytically, were generally consistent, with 23–39% of individuals detected 2012–2015 previously detected (Supplementary Table A5).
Two models had high parsimony (<2 ΔAIC, next best model ΔAIC = 4.5), sharing identical form except that density either was constant (best model) or declined linearly with year. In the Bayesian fit of the best model, estimates generally aligned with likelihood-based estimates. Mean annual point estimates of abundance ranged from 536–552 with 95% credible intervals ranging from 451–647 across years (Table 1). Both top models included the maximum September RSF value, distance to river, and park boundary indicator. More animals occurred in areas of better habitat, inside the park, and near the river, consistent with bighorn observations (Table 7; Figure 6). Baseline detection included trap type as 4 categories and presence of 2 experienced observers. Detection was highest at sites where the team stopped because they saw bighorn or bighorn sign, followed by predefined search areas (2014–2015), systematic sites searched based on a congruence of good habitat and the ability to stop the raft (2011–2013), and finally by opportunistic locations. The best model for σ was session-specific, with the lowest estimated activity range in 2013 (Table 7). Sex differences in density or detection were not supported.
Table 7 Coefficient estimates for the best spatial capture recapture model of bighorn sheep in Grand Canyon, 2011–2015, based on scaled variables.
Figure 6 Predicted distribution of density across the study area. Map illustrates how the covariates of distance to river and maximum resource selection in the density portion of the model led to the highest predicted density near the river and predicts more areas of high density in the central and eastern sections. The model does not account for subpopulations.
Integrating observational, invasive, and non-invasive approaches along a riverine system yielded new insights for a large native population of desert-adapted ungulates in a remote region. We assessed population fragmentation, gene flow among population subcomponents, whether the river acted as a barrier to movement of individuals, genes, or pathogens, the presence and distribution of key respiratory pathogens, and tested a method to estimate population size with uncertainty in this complex system. Our investigation determined that Grand Canyon is a moderately fragmented landscape for desert bighorn, due to the linear distribution of animals disrupted by complex topography and biogeographic barriers, as well as possible anthropogenic influences. Multiple lines of evidence indicated that existing landscape barriers are not absolute, and therefore are unlikely to prevent spread of respiratory disease, but limit gene flow to varying degrees and may impede recolonization where local extirpation has occurred. However, we also established a viable method for population estimation that verified the size, stability, and regional importance of this population.
Despite apparently continuous habitat along a relatively narrow river corridor, our investigations revealed that the degree, although not the geometry, of spatial structuring of bighorn in Grand Canyon in some ways resembles some of the well-documented Mojave Desert metapopulations (Schwartz et al., 1986). Indeed, Grand Canyon bighorn had similar levels of structure and pairwise FST values to those in the Mojave Desert of California and southern Nevada (Epps et al., 2018; Creech et al., 2020). Despite that degree of fragmentation, genetic diversity estimates of the 6 subpopulations we detected (Table 6) are among the highest reported for desert bighorn populations across the southwestern United States (Creech et al., 2020), pointing to the importance of this system as a long-term refugium for native desert bighorn. Although there are no obvious anthropogenic developments that stifle movements among subpopulations (e.g., highways), the combination of robust topography, areas with lower habitat suitability or at least low bighorn presence, and the partial barrier imposed by the Colorado River maintain enough separation to create six subpopulations of bighorn in Grand Canyon. In the Mojave, genetic investigations of bighorn with the same or similar microsatellite loci demonstrated that FST <0.05 between populations that regularly exchange migrants (Epps et al., 2010; Epps et al., 2018). Here, we observed FST< 0.05 only between two pairs of subpopulations on the same side of the river (RR-Mid & RR-West, RL-East & RL-Mid), mirroring the highest estimated recent migration rates (previous generation). However, gene flow across the river between subpopulations in the central section approached that level (FST = 0.07), where we also observed high migration rates in the previous generation (Table 6).
We observed direct and indirect genetic evidence for river crossings by bighorn, including recaptures of individuals on both sides as well as migrants from subpopulations on the other side of the river, particularly in the midsection of the study area (Supplementary Table A3). Genetic structure and migration indices, however, clearly indicate bighorn successfully move and reproduce at a higher rate between subpopulations on the same side of the river, despite partial barriers imposed by topography (Table 6). We found direct evidence for river crossing only in one small section that also has relatively high bighorn density (~5 river miles, RM 136–141, above the junction with Kanab Creek at RM 144, Supplementary Figure A5; Figure 6), although migrant tests and assignments tests suggest cross-river gene flow has occurred elsewhere. Anecdotal observations of bighorn swimming across the river are uncommon and have solely included rams (Holton personal observation). No collared bighorn crossed the river. However, our genetic recaptures indicated both males (n = 3) and females (n = 3) crossed. No major rapids occur in the reach of RM 137–144, topography allows relatively easy access to the river, and the apparently high density of bighorn on both sides in this section may increase incentive for crossings, as bighorn are drawn to conspecifics. Indeed, visual inspection of assignment tests and individual locations suggested that the greatest genetic mixing among subpopulations occurred in this stretch of river just above Kanab Creek (Figures 1, 4, 5). This river reach also has regional importance. Gille et al. (2019) determined that a translocated bighorn population outside the park in the upper Kanab Creek drainage showed evidence of significant gene flow from bighorn within Grand Canyon. That population tested positive for a strain of M. ovipneumoniae that has not yet been detected within the Grand Canyon region (A. E. Justice-Allen, AZGFD, written communication, January 21, 2022), although we note that few samples have been strain typed. Although our individual assignment tests did not suggest that influx of genetic variants from another “outside” population has yet occurred, this river section will be important to monitor for influx or egress of new strains of M. ovipneumoniae.
We were unable to determine whether the barrier effect imposed by the Colorado River increased with stabilization of water flows, which, since the Glen Canyon Dam was completed in 1963, generally have remained above 6,000–8,000 cfs (170–227 cms) during 1995–2020 (USGS, 2022). Patterns of migration inferred by FST, which typically represent inferences over a longer timeframe than other measures presented here (Epps and Keyghobadi, 2015), were similar to those inferred from estimates of recent migration rates or inferences from detection of migrants or individual movements. That similarity suggests movement patterns have been consistent for more than the last few generations. Human-made barriers in other metapopulations of desert bighorn caused large increases in FST within only about 8 generations due to the high rate of genetic drift observed in small bighorn populations (42 years, Epps et al., 2005). The FST levels in this system are therefore unlikely to represent pre-dam levels of genetic structure and gene flow. However, at this time, observed rates of migration throughout Grand Canyon appear sufficient to prevent inbreeding or rapid loss of genetic diversity due to genetic drift, with the possible exception of RL-West: all other subpopulations showed at least one link with migration rates in the previous generation exceeding 0.10 and FST values< 0.05 (Table 6). Therefore, while movements across the river are infrequent, we do not conclude that river flows would need to be altered to encourage additional migration at this time. If, however, local extirpation were to occur due to disease or some other issue in the central region, where cross-river movements were observed to be most common, barrier effects from the river could increase more rapidly. Populations on RL (southern side) are most isolated from other bighorn populations outside Grand Canyon, so any disruptions of gene flow within Grand Canyon would likely influence those populations disproportionately.
We estimated mean population sizes of 536–552 (95% CrI range 451–647) bighorn per year across the six Grand Canyon subpopulations during this study. We did not detect population declines with this dataset. Although we illustrated that sufficient data for reasonable estimates with sufficient precision to detect large declines could be obtained with a single sampling trip per year when combined with data from multiple years (Schmidt et al., 2022), model selection uncertainty and these credible intervals suggest that multiple trips, longer trips with more locations sampled, or models allowing same-site recaptures may be needed to gain enough precision to detect small changes in abundance, particularly if goals included estimates for each sub-population. We attempted to estimate density separately by side of the river, but data were sparse, few models converged, and those that did had very large confidence intervals. The administrative boundary of the park included some large areas with little observed use, such as the area on RR between RM 77–95 (i.e., between RR-Mid and RR-East) and distant from the river. Our resource selection model predicted high probability of use near the river and along some side drainages, but few bighorn were observed (Figure 2) and no samples were collected in this section (Supplementary Figure A5). Our model of predicted N suggested lower use along the river in this section compared to some other sections, and little use distant from the river (Figure 6). Sheer cliffs leading down to the river’s edge throughout much of this river reach may hamper accessibility for bighorn.
We confirmed for the first time that respiratory pneumonia was widespread in this system. All six Grand Canyon subpopulations displayed common signs of respiratory disease in bighorn (Cassirer et al., 2018) such as coughing individuals, dead bighorn with signs of bronchiopneumonia, or bighorn with M. ovipneumoniae-positive tests. The distribution of impacts was consistent with our estimates of genetic structure and migration, which indicated that all subpopulations are linked by movements ranging from occasional to frequent. Disease transmission can occur even from incidental contact (Besser et al., 2014). A spike in mortalities peaking in 2014, apparent declines in lamb:ewe ratios later in the study (Table 1), and high rates of infected and exposed individuals support the conclusion that an outbreak was occurring during the study period, but adverse effects appeared short-term. Although we detected fewer individuals genetically and in river surveys in 2013–2014, the population did not suffer a clear decline during our 5-year population estimation, and credible intervals were reasonably small suggesting reasonable power to detect a severe decline. Population declines exceeding 50% of adults have been observed in outbreaks of novel strains of M. ovipneumoniae in other bighorn populations (Cassirer et al., 2018). Here, consistent recapture rates across years (Supplementary Table A5) likewise suggested a relatively stable population. Shifts in bighorn movement, weather, or variation in survey periods and personnel could have decreased detection rates and sightings in some years. We assessed lamb:ewe ratios in September well after the typical period of high mortality for newly-exposed lambs (4–14 weeks, Cassirer et al., 2013). Yet, even the lowest lamb:ewe ratio observed (22:100 in 2015) did not reflect the extremely low lamb:ewe ratios observed within the central Mojave Desert metapopulation after an outbreak of respiratory pneumonia detected in 2013. There, lamb:ewe ratios were as low as zero in the worst-affected populations in the following year, although ratios in other affected populations were as high as 69:100 within 3 years following the first detection of the outbreak (Dekelaita, 2020). Elsewhere in Arizona, lamb:ewe ratios for desert bighorn are often less than 30:100 (Wakeling, 2007; (AGFD), 2014); in other subspecies of bighorn sheep undergoing acute or chronic outbreaks, recurring lamb mortality can approach 100% (Spaan et al., 2021). Thus, we conclude that either the strain(s) of M. ovipneumoniae involved had low virulence (Johnson et al., 2022), or more likely, have been circulating in the population for some unknown previous time period (e.g., Shirkey et al., 2021).
The degree of connectivity we inferred from the genetic data clearly indicates that a novel strain of M. ovipneumoniae or any other pathogen transmitted by contact could likely spread throughout the Grand Canyon region. Yet, the spatial structuring we observed may still influence long-term impacts in the population. Once a strain of M. ovipneumoniae is established in a population and most adults have been exposed and either recovered or died, ewes that are chronically infected with M. ovipneumoniae may play a key role in the annually recurring infections of lambs with high subsequent mortality as observed in other bighorn systems (Plowright et al., 2017). Those “chronic carriers” may cause severe impacts even when present at very low frequency and maintain those impacts for years (e.g., Cassirer et al., 2013; Spaan et al., 2021). We hypothesize that the high degree of spatial segregation we described within Grand Canyon might allow more lambs to escape exposure and yield more recruitment in some areas, particularly during the later phases of a disease outbreak when chronic carriers are the primary source of infection. In the Hells Canyon system, local outbreaks in Rocky Mountain bighorn were asynchronous but still severe (Cassirer and Sinclair, 2007). In contrast, in the arid montane-island system in the Mojave Desert where desert bighorn do not appear to form large stable ewe groups, rapid local recovery in many populations after the 2013 outbreak (Shirkey et al., 2021) suggested that strong spatial structure, flexible behaviors, and lower survival and reduced movement for previously-infected bighorn (Dekelaita et al., 2020, Dekelaita et al., 2023) could buffer lambs from exposure across time and space as chronic carriers die or avoid long-distance movements. For desert bighorn in the riverine landscape of Grand Canyon, intensive demographic and disease sampling is needed to rigorously evaluate that hypothesis and determine the long-term impacts of disease on this population. The estimates of population size and disease distribution reported here will provide a baseline for future monitoring for ongoing or novel disease outbreaks.
The constraints on bighorn distribution and movement within this riverine system may have limited potential for recolonization at the edges: notably, bighorn were largely absent in the eastern portion of the study area, on RL upstream of the confluence with the Little Colorado River (RM 62), where no genetic samples were found and very few individuals were observed (Figures 2, 5). Although the population model predicted bighorn presence on both sides of the river (Figure 6), as no habitat covariates included differed significantly by river side, the estimated population for that portion of the system incorporates the detections on RR as well as undetected animals on both sides (Figure 7). We speculate that absence of bighorn from apparently otherwise suitable habitat stems from disease-related impacts of domestic sheep present on the adjacent lands above the rim, with recolonization of empty habitats slowed by the barrier effect of the Colorado River and the need for any recolonizing individuals to traverse along the river where steep cliffs may make travel difficult. Habitat does not appear to be limiting, and bighorn are common on RR (Figure 2), so the most likely explanation is local extirpation, perhaps because of past disease events, with recolonization limited by the river, steep cliffs, and distance to the subpopulation in the midsection of the park on RL. Observations, genetic samples, and estimated population densities provided consistent patterns of high densities of bighorn sheep on both sides of the river in the central section (~RM 125–190), as well as on RL at ~RM 215–225 (Figures 2, 5–7). However, recolonization potential is lower with dispersers limited to a single direction, compared to better-connected island-type arid montane systems (e.g., Epps et al., 2010).
Figure 7 Abundance of bighorn sheep in Grand Canyon by river mile, summarized at 2.5-mile intervals incorporating 2 km buffers on both sides of the river, showing the average of estimated population sizes from 2011–2015. Observations suggested few bighorn occurred in the eastern river section on RL before river mile 62 at the confluence with the Lower Colorado River.
Rivers provide resources, alter terrain on geological and ecological time scales, and may also act as barriers, slowing or preventing dispersal, or for some species, facilitating dispersal. For desert bighorn in Grand Canyon, the river clearly provides a major resource in late summer, including water and forage on nearby vegetation. It does not provide a sufficient barrier to prevent spread of respiratory pathogens, but does limit dispersal, gene flow, and likely recolonization. Other river-dependent or river-influenced terrestrial vertebrates in the system, such as hog-nosed skunks (Holton et al., 2021), may exhibit very different constraints. The non-invasive and integrated methods that formed the backbone of our study may offer a useful approach for other populations of bighorn or other large mammals in canyon-dominated or riverine systems. Non-invasive genetic sampling combined with SCR methods allow simultaneously estimating population size, delineating potential subpopulations or metapopulation structure, evaluating whether the river or canyon acts as a barrier, and potentially other information such as diet or parasites, all of which may contribute to a fuller understanding of the importance of riverine systems for biodiversity. Where disease assessment also is possible by limited captures or other means, careful evaluation of whether rivers act as barriers to different degrees for pathogens or genes likewise would be informative for disease and population ecology.
The original contributions presented in the study are publicly available. This data can be found here: US Geological Survey data release, 10.5066/P9K89AA3.
The animal study was approved by National Park Service Institutional Animal Care and Use Committee. The study was conducted in accordance with the local legislation and institutional requirements.
CE: Conceptualization, Data curation, Formal analysis, Funding acquisition, Investigation, Methodology, Project administration, Resources, Writing – original draft, Writing – review & editing. PH: Conceptualization, Data curation, Funding acquisition, Investigation, Methodology, Project administration, Resources, Supervision, Writing – original draft, Writing – review & editing. RM: Conceptualization, Funding acquisition, Investigation, Writing – review & editing. RC: Data curation, Formal analysis, Investigation, Methodology, Writing – original draft, Writing – review & editing. SG: Formal analysis, Writing – review & editing. WJ: Formal analysis, Visualization, Writing – review & editing. TC: Data curation, Formal analysis, Investigation, Writing – review & editing. TG: Conceptualization, Data curation, Formal analysis, Investigation, Methodology, Supervision, Writing – original draft, Writing – review & editing.
The author(s) declare financial support was received for the research, authorship, and/or publication of this article. Funding for this research was provided by the NPS Climate Change Response Program grant through cooperative agreement #P10AC00485, a USGS-NPS Natural Resource Preservation Program grant, U.S. Geological Survey Ecosystem Mission Area Species Management Program, Oregon State University, and the Grand Canyon Conservancy.
We thank Scott Ratchford, Jenny Powers, and Liz Wheeler (NPS Wildlife Health Program) for assisting with bighorn captures and disease work, Janice Stroud-Settles, Greg Holm, Kirsten Ironside, Miles Brown, Skye Salganek, and the Grand Canyon Conservancy for assisting with bighorn captures and fecal sampling, Nate Alvord, Caroline Alvord, and Drew Podany for safely navigating the Colorado River through Grand Canyon, and numerous Grand Canyon river guides for recording bighorn observations along the river. We thank Ken Honeycutt and Nate Mikle for preliminary analysis. We thank the numerous commercial and private river guides for collecting bighorn observations over the last decades. Any use of trade, firm, or product names is for descriptive purposes only and does not imply endorsement by the U.S. Government.
The authors declare that the research was conducted in the absence of any commercial or financial relationships that could be construed as a potential conflict of interest.
All claims expressed in this article are solely those of the authors and do not necessarily represent those of their affiliated organizations, or those of the publisher, the editors and the reviewers. Any product that may be evaluated in this article, or claim that may be made by its manufacturer, is not guaranteed or endorsed by the publisher.
The Supplementary Material for this article can be found online at: https://www.frontiersin.org/articles/10.3389/fevo.2024.1377214/full#supplementary-material
Arizona Department of Game and Fish (2014) Kofa National Wildlife Refuge. Available online at: http://www.azgfd.gov/w_c/bhsheep/index.shtml (Accessed October 15, 2014).
Bellard C., Hugueny B. (2020). Importance of metapopulation dynamics to explain fish persistence in a river system. Freshw. Biol. 65, 1858–1869. doi: 10.1111/fwb.13571
Bender L. C., Weisenberger M. E. (2005). Precipitation, density, and population dynamics of desert bighorn sheep on San Andres National Wildlife Refuge, New Mexico. Wildlife Soc. Bull. 33, 956–964. doi: 10.2193/0091-7648(2005)33[956:PDAPDO]2.0.CO;2
Bendt R. H. (1957). Status of bighorn sheep in Grand Canyon National Park and Monument. Desert Bighorn Council Trans. 1, 16–19.
Besser T. E., Cassirer E. F., Potter K. A., Lahmers K., Oaks J. L., Shanthalingam S., et al. (2014). Epizootic pneumonia of bighorn sheep following experimental exposure to Mycoplasma ovipneumoniae. PloS One 9. doi: 10.1371/journal.pone.0110039
Besser T. E., Cassirer E. F., Potter K. A., VanderSchalie J., Fischer A., Knowles D. P., et al. (2008). Association of Mycoplasma ovipneumoniae infection with population-limiting respiratory disease in free-ranging rocky mountain bighorn sheep (Ovis canadensis canadensis). J. Clin. Microbiol. 46, 423–430. doi: 10.1128/JCM.01931-07
Besser T. E., Cassirer E. F., Yamada C., Potter K. A., Herndon C., Foreyt W. J., et al. (2012). Survival of bighorn sheep (Ovis canadensis) commingled with domestic sheep (Ovis aries) in the absence of Mycoplasma ovipneumoniae. J. Wildlife Dis. 48, 168–172. doi: 10.7589/0090-3558-48.1.168
Billingsley G. H., Hampton H. M. (1999). Physiographic rim of the Grand Canyon, Arizona: a digital database. U.S. Geological Survey Open-File Report USGS. U.S. Geological Survey, Reston, VA. doi: 10.3133/ofr9930
Bino G., Kingsford R. T., Wintle B. A. (2020). A stitch in time - Synergistic impacts to platypus metapopulation extinction risk. Biol. Conserv. 242, 12. doi: 10.1016/j.biocon.2019.108399
Bleich V. C., Bowyer R. T., Pauli A. M., Matthew M. C., Anthes R. W. (1994). Mountain sheep Ovis canadensis and helicopter surveys: ramifications for the conservation of large mammals. Biol. Conserv. 70, 1–7. doi: 10.1016/0006-3207(94)90292-5
Bleich V. C., Wehausen J. D., Holl S. A. (1990). Desert-dwelling mountain sheep: conservation implications of a naturally fragmented distribution. Conserv. Biol. 4, 383–390. doi: 10.1111/j.1523-1739.1990.tb00312.x
Borchers D. L., Efford M. G. (2008). Spatially explicit maximum likelihood methods for capture-recapture studies. Biometrics 64, 377–385. doi: 10.1111/j.1541-0420.2007.00927.x
Burnham K. P., Anderson D. R. (2002). Model selection and multimodel inference: a practical information-theoretic approach (New York: Springer).
Cassirer E. F., Manlove K. R., Almberg E. S., Kamath P. L., Cox M., Wolff P., et al. (2018). Pneumonia in bighorn sheep: risk and resilience. J. Wildlife Manage. 82, 32–45. doi: 10.1002/jwmg.21309
Cassirer E. F., Plowright R. K., Manlove K. R., Cross P. C., Dobson A. P., Potter K. A., et al. (2013). Spatio-temporal dynamics of pneumonia in bighorn sheep. J. Anim. Ecol. 82, 518–528. doi: 10.1111/1365-2656.12031
Cassirer E. F., Sinclair A. R. E. (2007). Dynamics of pneumonia in a bighorn sheep metapopulation. J. Wildlife Manage. 71, 1080–1088. doi: 10.2193/2006-002
Crawford J. C., Liu Z. W., Nelson T. A., Nielsen C. K., Blooniquist C. K. (2008). Microsatellite analysis of mating and kinship in beavers (Castor canadensis). J. Mammalogy 89, 575–581. doi: 10.1644/07-MAMM-A-251R1.1
Creech T. G., Epps C. W., Landguth E. L., Wehausen J. D., Crowhurst R. S., Holton B., et al. (2017). Simulating the spread of selection-driven genotypes using landscape resistance models for desert bighorn sheep. PloS One 12. doi: 10.1371/journal.pone.0176960
Creech T. G., Epps C. W., Monello R. J., Wehausen J. D. (2014). Using network theory to prioritize management in a desert bighorn sheep metapopulation. Landscape Ecol. 29, 605–619. doi: 10.1007/s10980-014-0016-0
Creech T. G., Epps C. W., Wehausen J. D., Crowhurst R. S., Jaeger J. R., Longshore K., et al. (2020). Genetic and environmental indicators of climate change vulnerability for desert bighorn sheep. Front. Ecol. Evol. 8. doi: 10.3389/fevo.2020.00279
Dekelaita D. (2020). Assessing apparent effects on survival and movement of desert bighorn sheep (Ovis canadensi nelsoni) following a pneumonia outbreak. Corvallis, Oregon, U.S.A.: Ph.D., Oregon State University.
Dekelaita D. J., Epps C. W., German D. W., Powers J. G., Gonzales B. J., Abella-Vu R. K., et al. (2023). Animal movement and associated infectious disease risk in a metapopulation. R. Soc. Open Sci. 10. doi: 10.1098/rsos.220390
Dekelaita D. J., Epps C. W., Stewart K. M., Sedinger J. S., Powers J. G., Gonzales B., et al. (2020). Survival of adult female bighorn sheep following a pneumonia epizootic. J. Wildlife Manage. 84, 1268–1282. doi: 10.1002/jwmg.21914
de Valpine P., Turek D., Paciorek C. J., Anderson-Bergman C., Lang D. T., Bodik R. (2017). Programming with models: writing statistical algorithms for general model structures with NIMBLE. J. Comput. Graphical Stat 26, 403–413. doi: 10.1080/10618600.2016.1172487
de Valpine P., Paciorek C., Turek D., Michaud N., Anderson-Bergman C., Obermeyer F., et al. (2023). “NIMBLE: MCMC, Particle Filtering, and Programmable Hierarchical Modeling”. R package version 1.0.1 ed. doi: 10.5281/zenodo.1211190
Dormann C. F., Elith J., Bacher S., Buchmann C., Carl G., Carre G., et al. (2013). Ecography 36, 27–46. doi: 10.1111/j.1600-0587.2012.07348.x
Dudgeon D. (2000). The ecology of tropical Asian rivers and streams in relation to biodiversity conservation. Annu. Rev. Ecol. Systematics 31, 239–263. doi: 10.1146/annurev.ecolsys.31.1.239
Dugovich B. S., Beechler B. R., Dolan B. P., Crowhurst R. S., Gonzales B. J., Powers J. G., et al. (2023). Population connectivity patterns of genetic diversity, immune responses and exposure to infectious pneumonia in a metapopulation of desert bighorn sheep. J. Anim. Ecol. 92, 1456–1469. doi: 10.1111/1365-2656.13885
Dupont G., Royle J. A., Nawaz M. A., Sutherland C. (2021). Optimal sampling design for spatial capture-recapture. Ecology 102. doi: 10.1002/ecy.3262
Efford M. G., Boulanger J. (2019). Fast evaluation of study designs for spatially explicit capture-recapture. Methods Ecol. Evol. 10, 1529–1535. doi: 10.1111/2041-210X.13239
Elbroch M. (2006). Animal skulls: a guide to North American species (Mechanicsburg, Pennsylvania, USA: Stackpole Books).
Epps C. W., Crowhurst R. S., Nickerson B. S. (2018). Assessing changes in functional connectivity in a desert bighorn sheep metapopulation after two generations. Mol. Ecol. 27, 2334–2346. doi: 10.1111/mec.14586
Epps C. W., Keyghobadi N. (2015). Landscape genetics in a changing world: disentangling historical and contemporary influences and inferring change. Mol. Ecol. 24, 6021–6040. doi: 10.1111/mec.13454
Epps C. W., McCullough D. R., Wehausen J. D., Bleich V. C., Rechel J. L. (2004). Effects of climate change on population persistence of desert-dwelling mountain sheep in California. Conserv. Biol. 18, 102–113. doi: 10.1111/j.1523-1739.2004.00023.x
Epps C. W., Palsboll P. J., Wehausen J. D., Roderick G. K., Ramey R. R. II, McCullough D. R. (2005). Highways block gene flow and cause a rapid decline in genetic diversity of desert bighorn sheep. Ecol. Lett. 8, 1029–1038. doi: 10.1111/j.1461-0248.2005.00804.x
Epps C. W., Wehausen J. D., Palsboll P. J., McCullough D. R. (2010). Using genetic tools to track desert bighorn sheep colonizations. J. Wildlife Manage. 74, 522–531. doi: 10.2193/2008-448
Evanno G., Regnaut S., Goudet J. (2005). Detecting the number of clusters of individuals using the software STRUCTURE: a simulation study. Mol. Ecol. 14, 2611–2620. doi: 10.1111/j.1365-294X.2005.02553.x
Fagan W. F. (2002). Connectivity, fragmentation, and extinction risk in dendritic metapopulations. Ecology 83, 3243–3249. doi: 10.1890/0012-9658(2002)083[3243:CFAERI]2.0.CO;2
Faubet P., Gaggiotti O. E. (2008). A new Bayesian method to identify the environmental factors that influence recent migration. Genetics 178, 1491–1504. doi: 10.1534/genetics.107.082560
Free C. L., Baxter G. S., Dickman C. R., Leung L. K. P. (2013). Resource pulses in desert river habitats: productivity-biodiversity hotspots, or mirages? PloS One 8. doi: 10.1371/journal.pone.0072690
Free C. L., Baxter G. S., Dickman C. R., Lisle A., Leung L. K. P. (2015). Diversity and community composition of vertebrates in desert river habitats. PloS One 10. doi: 10.1371/journal.pone.0144258
Fronhofer E. A., Altermatt F. (2017). Classical metapopulation dynamics and eco-evolutionary feedbacks in dendritic networks. Ecography 40, 1455–1466. doi: 10.1111/ecog.02761
Fuller A. K., Sutherland C. S., Royle J. A., Hare M. P. (2016). Estimating population density and connectivity of American mink using spatial capture-recapture. Ecol. Appl. 26, 1125–1135. doi: 10.1890/15-0315
Gille D. A., Buchalski M. R., Conrad D., Rubin E. S., Munig A., Wakeling B. F., et al. (2019). Genetic outcomes of translocation of bighorn sheep in Arizona. J. Wildlife Manage. 83, 838–854. doi: 10.1002/jwmg.21653
Graves T. A., Epps C. W., Janousek W. M., Ironside K., Monello R. J., Crowhurst R. S., et al. (2024). Desert bighorn sheep (Ovis canadensis nelsoni) datasets from Grand Canyon National Park, 2010-2018. U.S Geological Survey data release. doi: 10.5066/P9K89AA3
Gushue T. (2019). Colorado River Mile System, Grand Canyon, Arizona (USGS). Available at: https://www.usgs.gov/data/colorado-river-mile-system-grand-canyon-arizona. U.S. Geological Survey, Reston, VA. doi: 10.5066/P9IRL3GV
Holton B., Theimer T., Ironside K. E. (2021). First records and possible range extension of American hog-nosed skunk into the Grand Canyon. Small Carnivore Conserv. 59, e59001.
Ironside K. E., Mattson D. J., Arundel T., Theimer T., Holton B., Peters M., et al. (2018). Geomorphometry in landscape ecology: issues of scale, physiography, and application. Environ. Ecol. Res. 6, 397–412. doi: 10.13189/eer.2018.060501
Ironside K. E., Mattson D. J., Choate D., Stoner D., Arundel T., Hansen J., et al. (2017). Variable terrestrial GPS telemetry detection rates: addressing the probability of successful acquisitions. Wildlife Soc. Bull. 41, 329–341. doi: 10.1002/wsb.758
Johnson H. E., Mills L. S., Stephenson T. R., Wehausen J. D. (2010). Population-specific vital rate contributions influence management of an endangered ungulate. Ecol. Appl. 20, 1753–1765. doi: 10.1890/09-1107.1
Johnson B. M., Stroud-Settles J., Roug A., Manlove K. (2022). Disease ecology of a low-virulence Mycoplasma ovipneumoniae strain in a free-ranging desert bighorn sheep population. Animals 12. doi: 10.3390/ani12081029
Kalinowski S. T., Taper M. L., Marshall T. C. (2007). Revising how the computer program cervus accommodates genotyping error increases success in paternity assignment. Mol. Ecol. 16, 1099–1106. doi: 10.1111/j.1365-294X.2007.03089.x
Kamath P. L., Manlove K., Cassirer E. F., Cross P. C., Besser T. E. (2019). Genetic structure of Mycoplasma ovipneumoniae informs pathogen spillover dynamics between domestic and wild Caprinae in the western United States. Sci. Rep. 9, 14. doi: 10.1038/s41598-019-51444-x
Kendall K. C., Graves T. A., Royle J. A., Macleod A. C., McKelvey K. S., Boulanger J., et al. (2019). Using bear rub data and spatial capture-recapture models to estimate trend in a brown bear population. Sci. Rep. 9. doi: 10.1038/s41598-019-52783-5
Latch E. K., Scognamillo D. G., Fike J. A., Chamberlain M. J., Rhodes O. E. (2008). Deciphering ecological barriers to North American river otter (Lontra canadensis) gene flow in the Louisiana landscape. J. Heredity 99, 265–274. doi: 10.1093/jhered/esn009
Leigh C., Sheldon F., Kingsford R. T., Arthington A. H. (2010). Sequential floods drive ‘booms’ and wetland persistence in dryland rivers: a synthesis. Mar. Freshw. Res. 61, 896–908. doi: 10.1071/MF10106
McCluney K. E., Poff N. L., Palmer M. A., Thorp J. H., Poole G. C., Williams B. S., et al. (2014). Riverine macrosystems ecology: sensitivity, resistance, and resilience of whole river basins with human alterations. Front. Ecol. Environ. 12, 48–58. doi: 10.1890/120367
McKinney T., Boe S. R., deVos J. C. (2003). GIS-based evaluation of escape terrain and desert bighorn sheep populations in Arizona. Wildlife Soc. Bull. 31, 1229–1236.
Miller D. M., Young R. A., Gatlin T. W., Richardson J. A. (1982). Amphibians and reptiles of the Grand Canyon. Grand Canyon Natural History Assoc. Monogr. 4, 133.
Morin D. J., Waits L. P., McNitt D. C., Kelly M. J. (2018). Efficient single-survey estimation of carnivore density using fecal DNA and spatial capture-recapture: a bobcat case study. Population Ecol. 60, 197–209. doi: 10.1007/s10144-018-0606-9
Naka L. N., Pil M. W. (2020). Moving beyond the riverine barrier vicariant paradigm. Mol. Ecol. 29, 2129–2132. doi: 10.1111/mec.15465
Nilsson C., Jansson R., Malmqvist B., Naiman R. J. (2007). Restoring riverine landscapes: The challenge of identifying priorities, reference states, and techniques. Ecol. Soc. 12. doi: 10.5751/ES-02030-120116
Pfeiler S. S., Conner M. M., McKeever J. S., Stephenson T. R., German D. W., Crowhurst R. S., et al. (2020). Costs and precision of fecal DNA mark-recapture versus traditional mark-resight. Wildlife Soc. Bull. 44, 531–542. doi: 10.1002/wsb.1119
Phillips B. G., Phillips A. M. III, Schmidt-Bernzott M. A. (1987). Annotated checklist of vascular plants of Grand Canyon National Park. Grand Canyon Natural History Assoc. Monogr. 7.
Plowright R. K., Manlove K. R., Besser T. E., Paez D. J., Andrews K. R., Matthews P. E., et al. (2017). Age-specific infectious period shapes dynamics of pneumonia in bighorn sheep. Ecol. Lett. 20, 1325–1336. doi: 10.1111/ele.12829
Pritchard J. K., Stephens M., Donnelly P. (2000). Inference of population structure using multilocus genotype data. Genetics 155, 945–959. doi: 10.1093/genetics/155.2.945
R Core Team. (2023). R: a language and environment for statistical computing (Vienna, Austria: R Foundation for Statistical Computing).
Raymond M., Rousset F. (1995). GENEPOP (Version1.2): population genetics software for exact tests and ecumenicism. J. Heredity 86, 248–249. doi: 10.1093/oxfordjournals.jhered.a111573
Royle J. A., Chandler R. B., Gazenski K. D., Graves T. A. (2013b). Spatial capture-recapture models for jointly estimating population density and landscape connectivity. Ecology 94, 287–294. doi: 10.1890/12-0413.1
Royle J. A., Chandler R. B., Sun C. C., Fuller A. K. (2013c). Integrating resource selection information with spatial capture-recapture. Methods Ecol. Evol. 4, 520–530. doi: 10.1111/2041-210X.12039
Royle J., Changler R. B., Sollmann R., Gardner B. (2013a). Spatial capture-recapture (Waltham, MA, USA: Academic Press). doi: 10.1016/B978-0-12-405939-9.00005-0
Royle J. A., Fuller A. K., Sutherland C. (2018). Unifying population and landscape ecology with spatial capture-recapture. Ecography 41, 444–456. doi: 10.1111/ecog.03170
Royle J. A., Young K. V. (2008). A hierarchical model for spatial capture-recapture data. Ecology 89, 2281–2289. doi: 10.1890/07-0601.1
Sankey J. B., Ralston B. E., Grams P. E., Schmidt J. C., Cagney L. E. (2015). Riparian vegetation, Colorado River, and climate: Five decades of spatiotemporal dynamics in the Grand Canyon with river regulation. J. Geophysical Research-Biogeosciences 120, 1532–1547. doi: 10.1002/2015JG002991
Schmidt G., Graves T. A., Pederson J. C., Carroll S. L. (2022). Evaluating uncertainty in spatial capture-recapture estimates with a multi-site, multi-year sampling framework: A Utah black bear case study. Ecol. Appl. 32, e2618. doi: 10.1002/eap.2618
Schwartz O. A., Bleich V. C., Holl S. A. (1986). Genetics and the conservation of mountain sheep Ovis canadensis nelsoni. Biol. Conserv. 37, 179–190. doi: 10.1016/0006-3207(86)90090-X
Shirkey N., Roug A., Besser T., Bleich V. C., Darby N., Dekelaita D., et al. (2021). Previously unrecognized exposure of desert bighorn sheep (Ovis canadensis nelsoni) to Mycoplasma ovipneumoniae in the California Mojave Desert. J. Wildlife Dis. 57, 447–452. doi: 10.7589/JWD-D-20-00098
Singer F. J., Bleich V. C., Gudorf M. A. (2000). Restoration of bighorn sheep metapopulations in and near western National Parks. Restor. Ecol. 8, 14–24. doi: 10.1046/j.1526-100x.2000.80062.x
Spaan R. S., Epps C. W., Crowhurst R., Whittaker D., Cox M., Duarte A. (2021). Impact of Mycoplasma ovipneumoniae on juvenile bighorn sheep (Ovis canadensis) survival in the northern Basin and Range ecosystem. Peerj 9, 28. doi: 10.7717/peerj.10710
Stears K., Nuñez T. A., Muse E. A., Mutayoba B. M., McCauley D. J. (2019). Spatial ecology of male hippopotamus in a changing watershed. Sci. Rep. 9. doi: 10.1038/s41598-019-51845-y
Stevens L. E. (2012). The biogeographic significance of a large, deep canyon: Grand Canyon of the Colorado River, southwestern USA. Global Advances in Biogeography. InTech, Rijeka, Croatia, 169–208.
Stevens L. E., Polhemus J. T. (2008). Biogeography of aquatic and semi-aquatic Heteroptera in the Grand Canyon ecoregion, southwestern USA. Monogr. Western North Am. Nat. 4, 38–76. doi: 10.3398/1545-0228-4.1.38
Sutherland C., Fuller A. K., Royle J. A., Hare M. P., Madden S. (2019). Large-scale variation in density of an aquatic ecosystem indicator species (vol 8, 8958, 2018). Sci. Rep. 8, 8958. doi: 10.1038/s41598-019-53158-6
Thorp J. H. (2014). Metamorphosis in river ecology: from reaches to macrosystems. Freshw. Biol. 59, 200–210. doi: 10.1111/fwb.12237
USGS (2022). USGS Surface-Water Daily Data for the Nation (Reston, VA: United States Geological Survey). Available at: https://waterdata.usgs.gov/nwis/dv.
Valière N. (2002). GIMLET: a computer program for analysing genetic individual identification data. Mol. Ecol. Notes 2, 377–379. doi: 10.1046/j.1471-8286.2002.00228.x-i2
Varty N. (1990). Ecology of the small mammals in the riverine forests of the Jubba Valley, southern Somalia. J. Trop. Ecol. 6, 179–189. doi: 10.1017/S0266467400004272
Wakeling B. F. (2007). Status of bighorn sheep in Arizona 2006-2007. Desert Bighorn Council Trans. 49, 52–54.
Walters J. E. (1979). Bighorn sheep population estimate for the south Tonto Plateau, Grand Canyon. Desert Bighorn Council Trans. 23, 96–106.
Wiens J. A. (2002). Riverine landscapes: taking landscape ecology into the water. Freshw. Biol. 47, 501–515. doi: 10.1046/j.1365-2427.2002.00887.x
Wilson L. O. (1976). Past and present distribution of bighorn sheep on the Colorado and Green rivers in Utah. Desert Bighorn Council Trans. 11, 65–72.
Wisdom M. J., Nielson R. M., Rowland M. M., Proffitt K. M. (2020). Modeling landscape use for ungulates: forgotten tenets of ecology, management, and inference. Front. Ecol. Evol. 8. doi: 10.3389/fevo.2020.00211
Woodruff S. P., Eacker D. R., Waits L. P. (2021). Estimating coyote densities with local, discrete Bayesian capture-recapture models. J. Wildlife Manage. 85, 73–86. doi: 10.1002/jwmg.21967
Keywords: abundance, desert bighorn, respiratory disease, genetic structure, movement, spatial capture recapture
Citation: Epps CW, Holton PB, Monello RJ, Crowhurst RS, Gaulke SM, Janousek WM, Creech TG and Graves TA (2024) Population and spatial dynamics of desert bighorn sheep in Grand Canyon during an outbreak of respiratory pneumonia. Front. Ecol. Evol. 12:1377214. doi: 10.3389/fevo.2024.1377214
Received: 26 January 2024; Accepted: 09 April 2024;
Published: 26 June 2024.
Edited by:
Mark S. Boyce, University of Alberta, CanadaReviewed by:
Ian Gazeley, University of Alberta, CanadaCopyright At least a portion of this work is authored by Brandon Holton, Ryan J. Monello, Sarah M. Gaulke, William M. Janousek, and Tabitha A. Graves on behalf of the U.S. Government and as regards Dr. Holton, Dr. Monello, Dr. Gaulke, Dr. Janousek, and Dr. Graves and the U.S. Government, is not subject to copyright protection in the United States. Foreign and other copyrights may apply. This is an open-access article distributed under the terms of the Creative Commons Attribution License (CC BY). The use, distribution or reproduction in other forums is permitted, provided the original author(s) and the copyright owner(s) are credited and that the original publication in this journal is cited, in accordance with accepted academic practice. No use, distribution or reproduction is permitted which does not comply with these terms.
*Correspondence: Clinton W. Epps, Y2xpbnRvbi5lcHBzQG9yZWdvbnN0YXRlLmVkdQ==
†Present address: Sarah M. Gaulke, Colorado Cooperative Fish and Wildlife Research Unit, Colorado State University, Fort Collins, CO, United States
‡ORCID: Clinton W. Epps, orcid.org/0000-0001-6577-1840
P. Brandon Holton, orcid.org/0009-0001-6436-4546
Rachel S. Crowhurst, orcid.org/0000-0003-2281-9693
Sarah M. Gaulke, orcid.org/0000-0002-2657-5844
William M. Janousek, orcid.org/0000-0003-3978-1775
Tyler G. Creech, orcid.org/0000-0001-8049-6680
Tabitha A. Graves, orcid.org/0000-0001-5145-2400
Disclaimer: All claims expressed in this article are solely those of the authors and do not necessarily represent those of their affiliated organizations, or those of the publisher, the editors and the reviewers. Any product that may be evaluated in this article or claim that may be made by its manufacturer is not guaranteed or endorsed by the publisher.
Research integrity at Frontiers
Learn more about the work of our research integrity team to safeguard the quality of each article we publish.