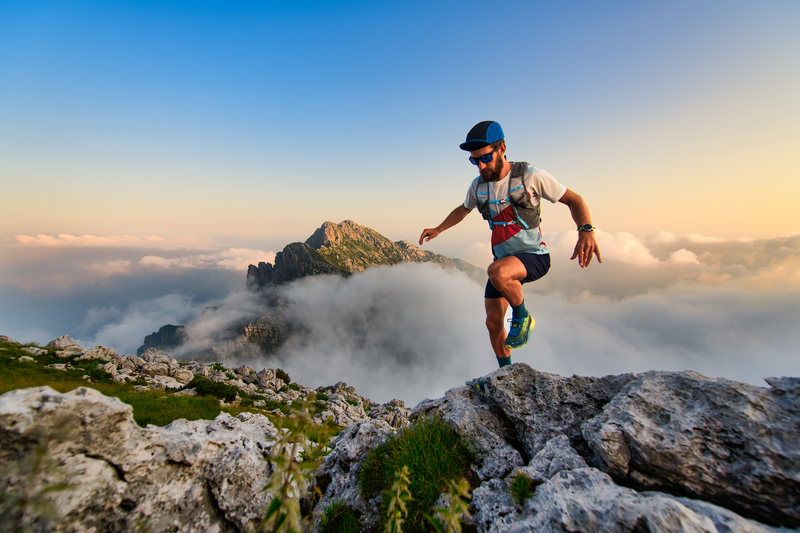
95% of researchers rate our articles as excellent or good
Learn more about the work of our research integrity team to safeguard the quality of each article we publish.
Find out more
EDITORIAL article
Front. Ecol. Evol. , 31 January 2024
Sec. Behavioral and Evolutionary Ecology
Volume 12 - 2024 | https://doi.org/10.3389/fevo.2024.1360427
This article is part of the Research Topic Cognitive Movement Ecology View all 16 articles
Editorial on the Research Topic
Cognitive movement ecology
Papers, dissertations and books devoted to the analysis of animal movement often invite interest in the subject with the incontrovertible claim that all animals move. It is no less true and no less obvious that all animals perceive, remember, and think (though cognitive scientists seem less obligated to remind everyone of the fact). Perception, memory, orientation, and navigation are all cognitive components that have been identified, in a zeitgeisty collection of simultaneous independent studies, as central to animal movement (Mueller and Fagan, 2008; Nathan et al., 2008; Schick et al., 2008). And yet, the cognitive causes and consequences of animal movement remain nearly as understudied now (Joo et al., 2022) as then (Holyoak et al., 2008).
There are several reasons behind the apparent chasm dividing these fields. Advances in movement ecology often “chase” both the data and the telemetry technology, the rapid development of which is often driven in support of concrete needs to monitor animal populations for management or conservation. Although biologists are generally aware, and often in awe, of the cognitive ability of their study species, the very thought of trying to measure or quantify something as unobservable as cognition is daunting and of limited apparent practical utility.
In contrast, the history and pedigree of ethological studies on animals is much longer. One might argue that, as an applied exercise, it includes all human groups that have ever engaged in the domestication of wild animals. In the Western scientific tradition, notably contributors include Darwin, Pavlov, and Lorenz. However, as a scientific endeavor, ethology has focused on animals that are easy to observe and therefore amenable to controlled experimentation, in almost all cases captive or domesticated (Wynne and Udell, 2020). Much as the wildlife manager may wonder what practical information can be obtained from considering cognition in a wild deer, an ethologist may wonder what can possibly be inferred about the cognition of an animal that can only be indirectly observed through blips of satellite locations and upon whom experimental manipulation is impractical. With the exception of a handful of neurological phenomena, cognitive processes are latent, and there are good reasons to shy away from studying what we cannot observe.
And yet, in the past decade there has been growing theoretical and empirical evidence that perception (Fagan et al., 2017), spatial memory (Fagan et al., 2013; Merkle et al., 2014; Avgar et al., 2015; Schlagel et al., 2017), and social and experiential learning (Mueller et al., 2013; Berdahl et al., 2018; Jesmer et al., 2018; Abrahms et al., 2021) are all fundamental to the way that free-ranging animals use space. It therefore felt timely and important to collect original research under the novel rubric of “Cognitive Movement Ecology” into a single Research Topic. We invited a wide array of conceptual, theoretical, and empirical papers, reflecting a wide range of approaches to this relatively new field of study. In so doing, we hoped to identify common themes, standardize some jargon, and generally facilitate dialog among cognitive movement ecologists.
The resulting Research Topics includes 15 contributions which strike an admirable balance between concepts, theory, methods and applications. Specifically, our Research Topic is comprised of: 2 high-level reviews, 4 explicitly theoretical contributions leaning on numerical analysis and simulation, 2 articles that propose novel heuristic approaches to inferring cognition from movement data, and, finally, 7 articles that bravely seek to make direct inference and even predictions about cognitive processes of free ranging animals based primarily on movement data. We provided no explicit guidelines outside the general rubric and were struck by the ways in which important themes emerged and similar goals were set in papers with markedly different approaches. In this editorial, we summarize the four sections of this Research Topic, making an effort to link the common themes across sections, and conclude with our view of the future of this young, but important, branch of ecology.
The Research Topic opens with a comprehensive review of the cognitive ecology of animal movement (Kashetsky et al.), setting the stage with a clear definition: that cognition is one of several processes that deal with the acquisition, retention, and use of information. The authors further explore several critical mechanisms by which such acquisition occurs, with an emphasis on the important role of social learning. The authors consider several observable spatial phenomena – all direct consequences of movement – that are exhibited by animals, in particular migration, homing, home ranging, trail following, and spatial learning. There is emphasis on the perceptual mechanisms and ranges (e.g. viewsheds, soundscapes, and smellscapes), including a consideration of the complexity and “cognitive costs” of different kinds of learning. These themes are laid out with several compelling published examples, and are all returned to explicitly and specifically (though largely independently) in almost every subsequent paper in the Research Topic. It bears noting, however, that the examples and synthesis provided are based primarily on experimental studies such as pigeon (Colomba livia domestica) releases and manipulated spatial feeding configurations for domestic sheep (Ovis aries).
The second major conceptual contribution (Lewis et al.) narrows the focus on learning (i.e. the acquisition and use of information), while broadening the disciplinary scope by pulling in vernacular, metaphors, and approaches from such fields as machine learning and robotics, as well as in psychology and behavior (their Box 1 provides a comprehensive glossary). Again, a clear definition rooted in the psychology literature is provided: that learning is a process of information acquisition that occurs via experience and leads to consistent and predictable neurophysiological or behavioral change. In the context of this Research Topic, the relevant observable behavioral change is specifically movement data. Much effort goes into covering the various mechanisms of learning (individual, social, positively reinforced, negatively reinforced, etc.). A set of rigorous criteria are proposed to identify whether actual learning is observed in a given study. Important distinctions are made between the kind of “fundamental learning” that occurs in a novel, or significantly perturbed, environment, compared to the kind of “maintenance learning” that is continuously ongoing in a dynamic but stochastically stationary environment. The former is more dramatic and categorical and can occasionally be inferred from “uncontrolled experiments” like translocations, introductions, or major environmental perturbations like habitat fragmentation or destruction. The second kind of learning is more subtle and reflects the ability of animals to continuously update information and make decisions. These two papers provide crucial conceptual context for later contributions in the Research Topic, all of which slot neatly into themes anticipated by these two overviews.
Theoretical studies lean on numerical studies and simulations and have the freedom to essentially create universes from scratch. In so doing, researchers can explore processes that are impossible to observe over a range of conditions that stretch the credible, potentially leading to profound insights into fundamental principles that produce patterns that are, in fact, widely observed in the wild.
Swain et al.– focusing on the evolution of perception – used millions of agent-based models to incorporate the relatively unexplored costs of perception to constrain the simulated emergence of optimal evolutionary scales of perception ranges. In identifying the conditions under which non-local perception is selected for, the authors found unintuitive interactions between, among others, resource density and energetic costs. Notably, low-resource environments led to the evolution of either zero perceptual range, or large perceptual ranges – pointing towards two divergent and apparently contradictory strategies in low-resource environments, consistent with observations (e.g., deep-water crustaceans either are entirely blind, or have exceptionally large eyes). The dramatic evolutionary trade-offs inherent in the evolution of perception (steep costs, high returns), leading to the wide range of evolutionary outcomes, is likely mirrored in the emergence of cognitive properties, like spatial memory and social learning, and the dizzying range of those adaptations. Indeed, it can be argued that memory itself is a kind of “temporal perceptual range”, that uses information from the past to “perceive” the future.
Gurarie et al., ask a complementary question: what possible non-genetic mechanisms can lead to the emergence, maintenance, and resilience of seasonal migrations, a very widespread and successful strategy that involves considerably uncertainty, risk, and energetic cost. Using a different computational approach from the other three theoretical studies (partial differential equations rather than agent-based simulations), the authors explore how collective memory, sociality, exploration, resource following, and learning all interact to exploit a highly seasonal and disconnected resource environment; i.e. one where the “patchiness” is extreme, but the predictability is high. For migration to emerge, all these ingredients are required, but mixed in just the right proportions: social cohesion to share information must be balanced against exploratory behavior to acquire new information, and a deep well of reference memory to lean on must be balanced against the ability to modify that reference in response to new information. Even in the highly synthetic conditions of the model, striking optimal balance is not easy; but, much as in the evolutionary model of Swain et al., the rewards can be considerable. Furthermore, though there is no selection in the model per se, it is clear that social learning as a mechanism can operate at time scales that are much more rapid than genetic selection.
Cognition is, however, not only about what the animals know (perception and memory), it is also about what they do not know, and how they might learn and make movement decision in the face of uncertainty. In the absence of perfect information, animals must rely on approximations to update their knowledge of their environment, as well as the expected outcomes of their decisions. Using individual-based simulations in a dynamic depleting and regenerating resource landscape, Avgar and Berger-Tal examine the role of two types of optimism as adaptive strategy for partially informed optimal foragers. Using a simple agent-based model, they show that moderate discounting of information from undesirable outcomes (‘positivity biased learning’ or ‘valence-dependent optimism’) results in improved fitness in environments characterized by high resource variability.
As if expressly to punish any irrationally optimistic foragers, Bracis and Wirsing introduce predators into a similar simulated dynamic resource environment to study the widely reported phenomenon of the “Landscape of Fear”. The authors build on a versatile continuous-time, continuous-space framework developed for the exploration of the role of spatial memory in guiding mobile foragers navigating dynamic landscapes (Bracis et al., 2015; Bracis et al., 2018). Within this habituated prey/resource system, the authors then release predators in high resource areas. The prey are left to learn from near escapes, and eventually to associate high quality habitat with increased risk. Somewhat analogous to Gurarie et al. This method of learning relies on two memory streams – a long-term “reference memory” (e.g. of fundamentally suitable habitat) and a short-term “working memory” which pushes the forager from recently depleted patches. Interestingly, these apparently simplistic two streams of memory are capable of both fundamentally learning about the new predator element, and of continuous maintenance learning (sensu Lewis et al.). The authors find that landscape of fear effects, in particular more time spent searching and less net consumption, do emerge with the presence of predators. However, the factors that lead to the most dramatic effects are primarily intrinsic, i.e. related to memory and personality, rather than external, i.e. related to the configuration of the environment. Specifically, the effects are greatest when animals are initially naïve to their environment and when they are highly conservative (akin to Avgar and Berger-Tal‘s pessimists). This result is important as a reminder that in real systems intrinsic states can easily be as important as the kinds of external, environmental factors that are most commonly used to model animal movements.
While very different in purpose and technique, a clear theme emerges from this suite of theoretical explorations: that the value of perception, memory, and learning for fitness is a direct consequence of the spatial structure and temporal dynamics of the environment the animal moves through. Thus, a somewhat unexpected corollary emerges: cognitive abilities serve above all else to compensate for constraints and limitations in the ability to move across the landscape itself.
While all the empirical studies rely to varying extents on methodological innovations, two contributions to this Research Topic stand out for proposing purely trajectory-based approaches to analyzing movement data, pointing towards widely observed spatial patterns that – the authors claim – can only emerge from memory-driven movement process.
Gautestad explores the topological properties of movement tracks that emerge from a model of self-reinforcing (i.e. memory-driven) returns to previously visited locations. This ultimately very simple model leads to patterns of space use that can be represented as a “scale-free network”. In other words, it has rare “dominant nodes” and very many “rarely visited” nodes, distributed in such a way that the frequency of degree centrality scores has a predictable log-log relationship. Gautestad shows that – when decomposed to a node-to-node type – empirical data on black bear movements (Ursus americanus) consistently show precisely the scale-free properties expected by this memory-driven random walk. A fascinating analogy is made with the global internet network, which is also scale-free and therefore susceptible to targeted attacks on dominant nodes. In similar ways, Gautestad makes an unexpectedly applied conclusion: that the movements and habitat-use of a free-ranging animal is structurally sensitive to disruptions to dominant nodes of patch use. There is an implicit corollary to this conclusion: if a movement track lacks these scale-free properties, this may indicate a perturbation in “normal” memory-inflected movement patterns.
Alavi et al. have a similar goal: to study the impact that simple cognitive processes have on the spatial, topological, and statistical properties of emergent movement tracks. Rather than focus, as Gautestad, on patches (network nodes) Alavi et al. focus on routes (network edges). They propose a set of metrics that can be computed directly from data that capture those properties related to linearity, absolute directionality, and recursion rates. Using a set of memory-driven simulations, the authors show the conditions under which these patterns emerge, and finally apply the methods to a set of four medium-sized tropical mammals moving in a forest in Panama. The differences in the movement patterns of these animals are striking, and well-captured by the metrics the authors proposed. Those differences are then compellingly related to very specific hypotheses about the kinds of learning and perceptual capacities (another recurring theme) that the animals likely rely on.
Notably, both of these highly original analyses depend entirely on the spatial properties of a movement track, without any environmental covariates, or even particular regard to displacement durations. Both lean on the fundamental fact that movement tracks never actually really resemble the kinds of naïve random movements that form the basis of most empirical movement modeling. In an echo of Bracis and Wirsing, they underscore the fact that a good amount of the structure of the observed animal movements can, in fact, emerge from purely intrinsic properties. Furthermore, they point to ways in which the generally unobservable process of cognition can nevertheless be inferred from movement data.
Inferring cognitive process based on observational data of free-ranging animals is a tremendous challenge (Lewis et al.). Nevertheless, seven contributions to our Research Topic attempt to do just that for a diverse set of taxa: three ungulate species (elk Cervus elaphus, mule deer Odocoileus hemionus, and bighorn sheep Ovis canadensis; Falcón-Cortés et al., Rheault et al., and Berger et al.), 2 terrestrial carnivores (fisher Pekania pennanti and wolves Canis lupus; Facka and Powell and Gurarie et al.), 1 flying mammal (Egyptian fruit bat Rousettus aegyptiacus; Lourie at al.), and 1 swimming fish (salmon Oncorhynchus spp.; Goodwin et al.). Rather than provide summaries of their findings (the authors do that in their abstracts much better than we could here), we focus on areas of notable overlap and divergence (Figure 1).
Figure 1 Venn diagram of seven empirical studies in the collection across three sets of commonalities. Three papers studied social interactions, four leveraged inference from “naive” animals (translocated ungulates, reintroduced predators, juvenile fish migrating downstream); four used some form of discrete choice modeling, whether choosing where to hunt, whether to migrate, where to move out of a discrete set of options.
The processes analyzed in these studies span a range of taxa and of spatio-temporal scales. But the fundamental question – at the level of the individual – always boils down to: where to move? At the extremes, Berger et al. predict seasonal migrations of sheep, while Goodwin et al focuses on extremely fine-scaled (3 minute) decisions made by fish in a highly dynamic environment. Rheault et al. and Falcón-Cortés et al. deal with space use and selection within a seasonal range – i.e. selection on a temporal scale of hours, while Gurarie et al. and Lourie at al. examine selection of foraging sites on the scale of diel departures from a den or roosting site. Lastly, Facka and Powell were interested less in details of movement than in large-scale interactions among conspecifics.
Six of the seven empirical contributions consider memory as a potentially important driver of animal space-use patterns or movement decisions and directly or indirectly provide a data-informed estimate of a “memory coefficient”. The most straightforward form of memory is captured as a tendency to return to previously visited locations, with or without temporal decay (Rheault et al., Falcón-Cortés et al., Lourie at al.). In all these cases, accounting for that tendency improved the ability of respective models to explain the data, or – equivalently – to match some of its emergent properties. Others add additional cognitive elements to simple attraction to previously visited locations; thus Berger et al. explicitly account for and estimate relevant perception ranges for decision-making, Gurarie et al. model multiple conflicting streams of memory that positively or negatively reinforce revisits, and Goodwin et al. incorporate a complex hierarchy of immediate behavioral responses to sensory input.
Three contributions are focused primarily on social drivers of space use (Figure 1, red set); Lourie at al. evaluate alternative hypotheses for foraging domain partitioning among neighboring bat colonies, Facka and Powell examine how established home ranges affected the formation of a newcomer’s home range, and Berger et al. compare the relative importance of the effect of a social group’s migratory culture to the effects of individual memory and sensory information. Inference on social factors requires simultaneous information on many individuals, an aspect that most observational studies lack. Each of these studies were able to examine these questions by using some innovation in their study design. Lourie at al. applied high-resolution tracking technology; they used a reverse-GPS system to track ~100 bats for an average of 24 days and at a resolution of 0.125 Hz (8 obs. x sec-1). Berger et al. and Facka and Powell had the advantage of studying reintroduced species (incidentally, both in the Sierra Nevada mountains) where many (Berger et al.) or all (Facka and Powell) individuals were tracked.
To varying degrees, four of the studies took advantage of naiveté in the animals (Figure 1, green set). Facka and Powell, Berger et al., and Falcón-Cortés et al. leveraged the “uncontrolled experiment” of releasing animals in novel environments (fisher reintroductions, and sheep and elk translocations, respectively). Facka and Powell had the further advantage of having tracked every reintroduced individual, while Berger et al. augmented their observations with the intensive monitoring associated with a high-profile recovery program. Finally, the juvenile fish in Goodwin et al were migrating downstream and entering environments and conditions, like dams, that were completely novel to them. Reintroductions and translocations – common means of ecological restoration or rewilding, augmenting struggling populations, or resolving human-wildlife conflicts – are of incredible value for studying learning in particular (Lewis et al.). Since relocated animals are naïve to the landscape they find themselves in, no behaviors can be ascribed to specific prior experience, only a moving set of expectations. The same is true of dispersal events (which also describes the juvenile salmon outmigration), which have the advantage of not requiring any handling of animals. Dispersal events, however, are generally much harder to detect in wild populations, mainly because they are relatively rare and tend to occur among subadult males, an age-sex class that is generally understudied by wildlife biologists and managers whose focus is often on adult females. Nonetheless, as tracking and monitoring efforts increase, dispersal events will be ever more available for analysis of learning in movement (Barry et al., 2020).
Two empirical contributions join the theoretical paper of Gurarie et al. to focus on seasonal migration. Berger et al. investigated why only some sheep migrate to lower elevation ranges in the fall while others remain in high-elevation ranges. Rheault et al. examined the effect of memory gained in the previous year on the space use of deer returning to their seasonal ranges. While not as tidy as translocations or dispersal events, seasonal migration also has particular benefits with respect to cognition. Beginning and end points of migrations are often well-known, or at least identifiable from movement data, and questions can focus on the repeatability of their selection. Furthermore, in some systems, proximate drivers of migration are more or less known, e.g. niche tracking or “green-wave surfing” (Merkle et al., 2016; Aikens et al., 2017), providing a well-understood null model against which the influence of perception or memory-driven choices can be compared. Finally, given long-enough tracking durations, we may have reasonable information on the animal’s prior knowledge and experience, provides researchers a null expectation about what the animal may or may not know. Studies where migratory animals are translocated and tracked as they do (or do not) adopt the migratory behavior, as was the case for several of the sheep in Berger et al., are of particular value (see also Mueller et al., 2013; Jesmer et al., 2018).
With respect to methodology, four of the contributions conducted some form of discrete choice analysis (Figure 1, blue set), where observed movement ‘decisions’ are contrasted against one or more alternative decisions that could have been made; e.g. to migrate or not to migrate (Berger et al.), which foraging area to move to (Gurarie et al., Falcón-Cortés et al.), or which “step” to take (Rheault et al.). These discrete-choice models were applied directly to observed data, and memory effects were assessed by including prior experience as a predictive covariate of the choice made. Discrete choice modeling is not often applied to wildlife studies, but echoes a long history of experimental approaches for studying memory and learning in animals (Tolman and Honzik, 1930; Wilkie and Willson, 1992; Thorpe et al., 2004). In contrast, two contributions constructed individual-based simulation models where some of the parameters are informed by observed data, but the simulation as a whole is tuned via likelihood-free (pattern-oriented) alignment with observed emerging patterns (Lourie at al.; Goodwin et al). Notably, Lourie at al. used the simulation-based approach to draw inferences about the relative contributions of individual memory vs. conformity, whereas Goodwin et al used it as a predictive tool. Lastly, Facka and Powell leverage the incredible strength of an experimental design: by simply comparing deliberate introductions of fishers into areas with and without the presence of conspecifics, a very strong signal of avoidance was detected without the need for overly complex analytical machinery.
Gurarie et al. conclude their analysis with a proposed five point checklist for the inference of memory driven processes from data on movements of free-ranging animals: (A) an observable behavior that might be driven by prior experiences; (B) identification of experienced cues that might influence that behavior; (C) a cognitive model; i.e. a plausible functional relationship between movement response A to experience B; (D) a statistical method (or pattern-matching heuristic) to estimate the model C; and (E) a metric for comparing the cognitive model against a non-cognitive model. It is instructive to apply this checklist to other studies. For example: in Berger et al. a sheep’s choice to migrate (A) is a consequence of perception viewsheds (B) which predict the probability of migration via a linear mixed model (C,D) which takes into account other potential covariates, and can be compared against a suite of non-cognitive models using maximum likelihood (E). Or, in Lourie at al., the observed property of spatially non-overlapping neighboring bat colonies (A) is hypothesized to be a consequence of prior visitations (B), a suite of agent-based models is developed to account for that behavior (C) and emergent properties of those simulations are compared to the observations (D) for agent-based simulations with and without the memory component (E). The empirical studies in this Research Topic checked off most, if not all, of these requirements, indicating that the framework may be useful for further empirical investigation into cognitive roots of movement.
Editing this Research Topic has reinforced our conviction that the cognitive processes of perception, memory and learning are fundamental to understanding any animal movements. But it may still not be clear why wildlife practitioners should care. Here, it bears noting that in two of the empirical studies (Rheault et al., Falcón-Cortés et al.) where time-scales of memory were estimated, memory was essentially infinite, consistent with prior findings (e.g., Avgar et al., 2015). Similarly, in both of the heuristic contributions (Alavi et al., Gautestad), the essential argument was that fundamental patterns of movements can be explained almost entirely by memory. These results suggest that, at least in some cases, the most effective way to predict where an animal might show up (an important goal for monitoring, conservation, and management) is not to model movement against some complex set of habitat covariates, but to simply study where the individual has been before. With that in mind, the global reality is that environmental conditions for many populations are changing extremely rapidly, whether through disturbance, habitat fragmentation, or climate change. These rapid changes put major pressures on the adaptability and behavioral plasticity of organisms. Or, to apply the jargon (and some of the paradigms) of animal cognition, the question of a population’s persistence can be summarized as its ability to modify a reference memory with updated working memories, such that the resulting behavioral innovations are adaptive with respect to fitness.
The two foundational models that underlie much of theoretical animal movement ecology are almost diametrically opposed. On the one extreme, the random walk (Berg, 1993; Turchin, 1998; Codling et al., 2008) assumes that animals move blindly and completely randomly in a restricted, slow to “diffuse” manner. On the other extreme, the ideal-free distribution (Fretwell and Lucas 1969; Křivan et al., 2008; Avgar et al., 2020) assumes that completely omniscient and optimal animals can appear anywhere and anytime, distributing themselves in proportion to resource availability. The reality is, of course, somewhere between the two: real animals in real-life scenarios are capable of moving in directed and informed ways, but not at infinite speed, and only with partial information about the environment. Cognitive movement ecology can be viewed as an essential bridge between these theoretical constructs. What does it mean to be partially informed? How does an organism act on that partial information? And how does it distribute itself through space, given its goals and given its constraints? How, in the end, do organisms manage to navigate, survive, even thrive in environments that are complex, heterogeneous, and dynamic? These questions, which are very much the realm of cognitive movement analysis, are also at the very foundation of animal ecology.
Biodiversity Pathways Ltd. is a non-profit ecological research organization for which TA serves as a scientific advisor with no financial compensation. Neither TA nor Biodiversity Pathways Ltd. have any conflict of interest associated with this research, financial, commercial, or otherwise.
EG: Writing – original draft, Writing – review & editing. TA: Writing – original draft, Writing – review & editing.
We thank the many authors (and authors) that contributed to this Research Topic and Chloe Beaupré for close reading and review of the editorial.
The authors declare that the research was conducted in the absence of any commercial or financial relationships that could be construed as a potential conflict of interest.
All claims expressed in this article are solely those of the authors and do not necessarily represent those of their affiliated organizations, or those of the publisher, the editors and the reviewers. Any product that may be evaluated in this article, or claim that may be made by its manufacturer, is not guaranteed or endorsed by the publisher.
Abrahms B., Teitelbaum C. S., Mueller T., Converse S. J. (2021). Ontogenetic shifts from social to experiential learning drive avian migration timing. Nat. Commun. 12, 7326. doi: 10.1038/s41467-021-27626-5
Aikens E. O., Kauffman M. J., Merkle J. A., Dwinnell S. P. H., Fralick G. L., Monteith K. L. (2017). The greenscape shapes surfing of resource waves in a large migratory herbivore. Ecol. Lett. 20, 741–750. doi: 10.1111/ele.12772
Avgar T., Baker J. A., Brown G. S., Hagens J. S., Kittle A. M., Mallon E. E., et al. (2015). Space-use behaviour of woodland caribou based on a cognitive movement model. J. Anim. Ecol. 84, 1059–1070. doi: 10.1111/1365-2656.12357
Avgar T., Betini G. S., Fryxell J. M. (2020). Habitat selection patterns are density dependent under the ideal free distribution. J. Anim. Ecol. 89, 2777–2787. doi: 10.1111/1365-2656.13352
Barry T., Gurarie E., Cheraghi F., Kojola I., Fagan W. F. (2020). Does dispersal make the heart grow bolder? Avoidance of anthropogenic habitat elements across wolf life history. Anim. Behav. 166, 219–231. doi: 10.1016/j.anbehav.2020.06.015
Berdahl A. M., Kao A. B., Flack A., Westley P. A. H., Codling E. A., Couzin I. D., et al. (2018). Collective animal navigation and migratory culture: from theoretical models to empirical evidence. Philos. Trans. R. Soc. B: Biol. Sci. 373, 20170009. doi: 10.1098/rstb.2017.0009
Berg H. C. (1993). Random walks in biology: new and expanded edition (Princeton, NJ, USA: Princeton University Press).
Bracis C., Gurarie E., Rutter J. D., Goodwin R. A. (2018). Remembering the good and the bad: memory-based mediation of the food-safety trade-off in dynamic landscapes. Theor. Ecol. 11, 305. doi: 10.1007/s12080-018-0367-2
Bracis C., Gurarie E., Van Moorter B., Goodwin R. A. (2015). Memory effects on movement behavior in animal foraging. PloS One. 10, e0136057. doi: 10.1371/journal.pone.0136057
Codling E. A., Plank M. J., Benhamou S. (2008). Random walk models in biology. J. R. Soc. Interface. 5 (25), 813–834. doi: 10.1098/rsif.2008.0014
Fagan W., Gurarie E., Bewick S., Howard A., Cantrell R., Cosner C. (2017). Perceptual ranges, information gathering, and foraging success in dynamic landscapes. Am. Nat. 189, 474–489. doi: 10.1086/691099
Fagan W. F., Lewis M. A., Auger-Méthé M., Avgar T., Benhamou S., Breed G., et al. (2013). Spatial memory and animal movement. Ecol. Lett. 16, 1316–1329. doi: 10.1111/ele.12165
Fretwell S. D., Lucas H. L. (1969). On territorial behavior and other factors influencing habitat distribution in birds. Acta Biotheor. 19, 16–36. doi: 10.1007/BF01601953
Holyoak M., Casagrandi R., Nathan R., Revilla E., Spiegel O. (2008). Trends and missing parts in the study of movement ecology. Proc. Natl. Acad. Sci. 105, 19060–19065. doi: 10.1073/pnas.0800483105
Jesmer B. R., Merkle J. A., Goheen J. R., Aikens E. O., Beck J. L., Courtemanch A. B., et al. (2018). Is ungulate migration culturally transmitted? Evidence of social learning from translocated animals. Science. 361, 1023–1025. doi: 10.1126/science.aat0985
Joo R., Picardi S., Boone M. E., Clay T. A., Patrick S. C., Romero-Romero V. S., et al. (2022). Recent trends in movement ecology of animals and human mobility. Movement Ecol. 10, 26. doi: 10.1186/s40462-022-00322-9
Křivan K., Cressman R., Schneider C. (2008). The ideal free distribution: a review and synthesis of the game-theoretic perspective. Theor. Popul. Biol. 73 (3), 403–425. doi: 10.1016/j.tpb.2007.12.009
Merkle J. A., Fortin D., Morales J. M. (2014). A memory-based foraging tactic reveals an adaptive mechanism for restricted space use. Ecol. Lett. 17, 924–931. doi: 10.1111/ele.12294
Merkle J. A., Monteith K. L., Aikens E. O., Hayes M. M., Hersey K. R., Middleton A. D., et al. (2016). Large herbivores surf waves of green-up during spring. Proc. R. Soc B. 283, 20160456. doi: 10.1098/rspb.2016.0456
Mueller T., Fagan W. F. (2008). Search and navigation in dynamic environments – from individual behaviors to population distributions. Oikos. 117, 654–664. doi: 10.1111/j.0030-1299.2008.16291.x
Mueller T., O’Hara R. B., Converse S. J., Urbanek R. P., Fagan W. F. (2013). Social learning of migratory performance. Science 341, 999–1002. doi: 10.1126/science.1237139
Nathan R., Getz W. M., Revilla E., Holyoak M., Kadmon R., Saltz D., et al. (2008). A movement ecology paradigm for unifying organismal movement research. Proc. Natl. Acad. Sci. 105, 19052–19059. doi: 10.1073/pnas.0800375105
Schick R. S., Loarie S. R., Colchero F., Best B. D., Boustany A., Conde D. A., et al. (2008). Understanding movement data and movement processes: current and emerging directions. Ecol. Lett. 11, 1338–1350. doi: 10.1111/j.1461-0248.2008.01249.x
Schlagel U., Merrill E. H., Lewis M. A. (2017). Territory surveillance and prey management: Wolves keep track of space and time. Ecol. Evol. 7, 8338. doi: 10.1002/ece3.3176
Thorpe C. M., Jacova C., Wilkie D. M. (2004). Some pitfalls in measuring memory in animals. Neurosci. Biobehav. Rev. 28, 711–718. doi: 10.1016/j.neubiorev.2004.09.013
Tolman E. C., Honzik C. H. (1930). Introduction and removal of reward, and maze performance in rats (Berkeley, CA, USA: University of California publications in psychology).
Turchin P. (1998). Quantitative analysis of movement: measuring and modeling population redistribution of plants and animals (Sunderland, MA, USA: Sinauer Associates).
Wilkie D. M., Willson R. J. (1992). Time-place learning by pigeons, Columbia livia. J. Exp. Anal. Behav. 57, 145–158. doi: 10.1901/jeab.1992.57-145
Keywords: animal movement, animal cognition, theoretical ecology, statistical ecology, spatial ecology, spatial memory, learning, perception
Citation: Gurarie E and Avgar T (2024) Editorial: Cognitive movement ecology. Front. Ecol. Evol. 12:1360427. doi: 10.3389/fevo.2024.1360427
Received: 23 December 2023; Accepted: 19 January 2024;
Published: 31 January 2024.
Edited and Reviewed by:
Jordi Figuerola, Spanish National Research Council (CSIC), SpainCopyright © 2024 Gurarie and Avgar. This is an open-access article distributed under the terms of the Creative Commons Attribution License (CC BY). The use, distribution or reproduction in other forums is permitted, provided the original author(s) and the copyright owner(s) are credited and that the original publication in this journal is cited, in accordance with accepted academic practice. No use, distribution or reproduction is permitted which does not comply with these terms.
*Correspondence: Eliezer Gurarie, ZWd1cmFyaWVAZXNmLmVkdQ==
Disclaimer: All claims expressed in this article are solely those of the authors and do not necessarily represent those of their affiliated organizations, or those of the publisher, the editors and the reviewers. Any product that may be evaluated in this article or claim that may be made by its manufacturer is not guaranteed or endorsed by the publisher.
Research integrity at Frontiers
Learn more about the work of our research integrity team to safeguard the quality of each article we publish.