- 1Department of Environmental Biology, State University of New York, College of Environmental Science and Forestry, Syracuse, NY, United States
- 2Pennsylvania Animal Diagnostic Laboratory System New Bolton Center, Department of Pathobiology, University of Pennsylvania School of Veterinary Medicine, Kennett Square, PA, United States
- 3Wildlife Futures Program, Department of Pathobiology, University of Pennsylvania School of Veterinary Medicine, Kennett Square, PA, United States
- 4New Hampshire Veterinary Diagnostic Laboratory, Department of Molecular, Cellular, and Biomedical Sciences, University of New Hampshire, Durham, NH, United States
- 5Department of Environmental Conservation, State of New York, Albany, NY, United States
- 6Bureau of Wildland Management, Pennsylvania Game Commission, Harrisburg, PA, United States
- 7Fish and Game Department, State of New Hampshire, Concord, NH, United States
- 8Department of Inland Fisheries and Wildlife, State of Maine, Augusta, ME, United States
- 9Fish and Wildlife Department, State of Vermont, Springfield, VT, United States
- 10Pennsylvania Game Commission, Harrisburg, PA, United States
- 11Cornell Wildlife Health Lab, Department of Public & Ecosystem Health, Cornell University, Ithaca, NY, United States
Studies in parts of Europe, New Zealand, and North America indicate uptake of anticoagulant rodenticides (ARs) by predatory mammals to be widespread and common, with proximity to urban and agricultural areas being an important driver of exposure. Yet, little is known regarding the patterns and drivers of AR exposure in predatory mammals within more forest-dominated landscapes. Across the forest-dominated northeastern United States (US), a region spanning Pennsylvania to Maine, we tested livers from 597 fisher (Pekania pennanti) obtained from the legal harvest for 11 anticoagulant rodenticide compounds. We used kriging to determine potential hot zones (within or among states) and used regression models to test agricultural land use, developed landscapes, and protected areas as potential drivers of exposure patterns. We detected 8 AR compounds, with 78.6% of sampled individuals testing positive for ≥1 compound. The highest rate of exposure was observed in New Hampshire and Vermont at 93.3 and 100.0% and the lowest in Maine at 52.8%. The majority of individuals (55.3%) tested positive for 2-6 different compounds rather than a single compound (23.3%) or none (21.4%), indicating repeated and chronic levels of exposure among fisher. Spatial interpolation revealed a hot region of exposure spanning southern New Hampshire, Vermont, and southeastern New York. Regression models indicated the proportion of wildland-urban intermix (low density buildings within a largely forest-dominated landscape) as a consistent and strong predictor of AR exposure, with marginal and inconsistent relationships observed between AR exposure and the amount of agricultural land use, and with no apparent benefit conferred by protected areas in the region. Given that northeastern states support the highest rural human population density in the nation, with individual homes interspersed throughout a forested matrix, residential use of ARs is implicated as potentially the main driver of exposure for forest carnivores in this region. However, surveillance of a broader suite of species, and greater knowledge of AR use in commercial forestry operations, will be necessary to understand the generality of our observations.
1 Introduction
Rodents have been recognized as a threat to human health and livelihoods for millennia (Van den Brink et al., 2018). Our defense arsenal includes physical methods (e.g., traps, barriers), biological/cultural remediation (e.g., sanitation, habitat manipulation, resistant plants, house cats), and chemical methods (e.g., toxic baits, fumigants, repellents). In both commercial and private applications, use of toxic compounds is a primary choice because they can remain potent for long deployment periods and a single bait station can dose numerous individuals (compared to a snap trap that can catch only one animal at a time before needing to be rebaited and reset). The most popular toxic compounds are anticoagulant rodenticides (ARs; Eisemann et al., 2018), which interfere with blood clotting ability (specifically the vitamin-K mediated synthesis of blood clotting factors in the liver) and render animals that have ingested ARs vulnerable over time to fatal hemorrhage precipitated by minor trauma, exertion, or other factors (Stone et al., 2003; Rattner and Mastrota, 2017). The various AR compounds on the market include first-generation ARs (FGARs; developed in the 1950s) and, developed in response to resistance by target pest populations, second-generation ARs (SGARs; developed in the mid-1970s; Pelz et al., 2005; Jacob and Buckle, 2017). Being more acutely toxic than FGARs, SGARs require only a single feeding to deliver a lethal dose (Vandenbroucke et al., 2008; Rattner and Mastrota, 2017). Whereas in the US, SGARs are restricted to professional or agricultural applicators (US Environmental Protection Agency, 2008, summarized by Eisemann et al., 2018), FGARs remain widely available to the public (Supplementary Table 1). In terms of deployment, ARs are sold as powders or pellets that may be placed directly in rodent burrows or as durable paste (block) form or soft pouch placed in water-proof bait stations that can be used inside and outside of buildings. The ubiquity of ARs in the modern pest control industry stems from their efficacy, ease of acquisition and application, and lack of accumulation of carcasses in unwanted places (Jacob and Buckle, 2017).
Importantly, ARs (both FGARs and SGARs) act the same whether consumed directly by ingesting bait or indirectly by predating or scavenging an AR-laden individual (i.e., relay toxicosis; Orsted et al., 1998). Altered behavioral patterns of AR-laden lab rats, such as shifted diurnal patterns and use of areas away from cover, indicate that ARs may predispose toxic rodents to predation in the wild (Cox and Smith, 1992). Despite regulations designed to protect children, pets, and non-target wildlife from exposure, detection of ARs in predators and scavengers has proven alarmingly widespread where it has been investigated (McMillin et al., 2008; Gabriel et al., 2012; Serieys et al., 2015; Ruiz-Suárez et al., 2016; Elmeros et al., 2018; Lohr, 2018; Wiens et al., 2019; Murray, 2020; Badry et al., 2021; Cooke et al., 2022). For example, in California 83-96% of tested black bear (Ursus americanus), bobcat (Lynx rufus) and fisher (Pekania pennanti) showed evidence of ≥1 AR in their tissue, most having been exposed to 2-5 different compounds (Riley et al., 2003; Riley et al., 2007; Gabriel et al., 2012; Serieys et al., 2015; McMillin et al., 2018). As commercial products contain a single AR compound (US Environmental Protection Agency, 2008), residues of ≥1 compound would indicate different exposure events and, by extension, repeated or chronic levels of exposure. All ARs, but especially SGARs, pose substantive risks to non-target wildlife given chronic exposure (Jacob and Buckle, 2017; Rattner and Mastrota, 2017) and long elimination half-lives (taking up to hundreds of days to metabolize from the liver; Vandenbroucke et al., 2008). In addition to directly causing mortality through coagulopathy, the sublethal effects of AR exposure include increased susceptibility to parasites and disease, decreased litter size, and increased newborn death (Robinson et al., 2005; Lemus et al., 2011; Rady et al., 2013; Serieys et al., 2015). Although public concern over the high prevalence of ARs in non-target wildlife may be escalating, there remains a lack of comparably effective alternatives for protecting human health and livelihoods from the risks posed by rodents (Rattner et al., 2014; Quinn et al., 2019). As such, greater insight is needed regarding the factors that drive AR exposure in wild carnivores to balance human safety more effectively with healthy wildlife populations.
Rates of AR exposure in predators and scavengers has been observed to increase in proximity to urbanization or agricultural landscapes in a variety of locations including Germany (Geduhn et al., 2015; Badry et al., 2021), northeastern Spain, (López-Perea et al., 2019), and California (Nogeire et al., 2015; Hofstadter et al., 2021). Indeed, areas of higher human population density tend to result in higher levels of AR use due in part to the greater need for rodent control, and in part to widespread non-compliance with regulations in public applications, such as outdoor use of indoor-only products and placement of ARs further from buildings than regulations stipulate (Bartos et al., 2011; Tosh et al., 2011). Given a relative paucity of studies focused on AR spillover to mammalian carnivores, it remains unknown the degree to which previous observations might be generalized to other landscape contexts. But given that toxic prey tend to occur close to the site of outdoor AR application (Tosh et al., 2012; Geduhn et al., 2014; Walther et al., 2020) spatial clustering of AR-laden predators should point to pathways for exposure and, by extension, mitigation.
This study focuses on the northeastern United States, which ranks among the top states in the nation in terms of both forest cover, ranging from 58.6% of PA to 89.5% of ME (Vogt and Smith, 2017), and rural human population densities, ranging from 9.6 to 26.8 people/km2 from rural ME to rural PA (Demographia.com, 2000). This high amount of forested area coupled with high human presence could put forest carnivores at elevated risk for AR exposure compared to more agrarian or arid landscape contexts. Within this forest-dominated region, we focus on fisher as a model species for studying AR exposure in mesocarnivores. Fisher are widespread across the region, occupy a wide gradient of landscape conditions from forest interiors to peri-urban and agricultural landscapes (Lewis et al., 2012; Jensen and Humphries, 2019), have a diet dependent largely upon rodent prey (McNeil et al., 2017), and have been shown to be vulnerable to AR exposure in both the western and eastern US portions of their range (Gabriel et al., 2012; Thompson et al., 2014; Thomas et al., 2017; Buckley et al., 2023). Our objectives were to: 1) compare the types and prevalence of ARs ingested by fisher across a multistate region, 2) interpolate patterns of AR exposure to delineate hot zones within and among states given different landscape contexts and potential cultures with respect to AR use, and 3) test the local effects of agricultural land uses (López-Perea et al., 2019; Badry et al., 2022), human developments (Lohr, 2018; Hofstadter et al., 2021), and protected areas (Martinuzzi et al., 2013; US Geological Survey and Gap Analysis Project, 2022) on AR exposure rates. Given the high rural population density overlapping fisher habitat, we expected public application of ARs to be a more dominant force of AR exposure than professional or commercial applications, which should be evident in the prevalence of FGARs relative to SGARs. We further expected SGARs to exhibit greater association with agricultural landscapes and human population centers given regulations that restrict their ability to licensed pest control specialists or agricultural settings. We further anticipated a gradient in overall AR exposure that would increase along a northeast to southwest axis of states corresponding to increasing human population densities and decreasing forest cover (the latter consistent with increasing agricultural intensity). Lastly, we anticipated decreased uptake of ARs by fisher in and around protected areas. With the goal of illuminating pathways of AR exposure, this research should help inform educational campaigns and management actions to better mitigate the risks to forest carnivores imposed by ARs.
2 Materials and methods
2.1 Sample collection
To collect a ‘snapshot’ of AR exposure in the standing live population, we acquired liver samples from fisher largely as a by-product of the regulated fall harvest (N=569) with additional samples collected from routine carcass submissions to wildlife health labs (N=28). As states were sampling fisher regardless of our research, our collection of samples was exempt from Institutional Animal Care and Use Committee review. Livers were collected from fishers across Pennsylvania (PA; N=44 harvest samples, years 2020–2022; N=23 incidental carcasses), New York (NY; N=338, 2018–20), Vermont (VT; N=71, 2018–19 and 2021–22), New Hampshire (NH; N=15, 2019), and Maine (ME; N=101 harvest samples, 2020; N=5 incidental carcasses). We pooled harvested and opportunistically collected samples to maximize spatial coverage and sample size, but compared AR exposure rates between harvested and opportunistically collected samples in PA using a test of equal proportions with α = 0.05 to identify potential biases in opportunistically collected carcass samples. Whereas we requested samples across the full range of environmental conditions fisher were exposed to, sample locations were limited to areas open to fisher harvest where both fishers and participating trappers were available. State agency biologists requested fisher carcasses from licensed trappers and provided frozen liver samples for this study.
All liver samples were sent to the Pennsylvania Animal Diagnostic Laboratory System (New Bolton Center in Kennett Square, PA) and screened for 11 AR compounds (Vudathala et al., 2010): four SGARs (brodifacoum, bromadiolone, difenacoum, and difethialone) and seven FGARs (chlorophacinone, coumachlor, coumafuryl, dicoumarol, diphacinone, pindone, and warfarin). All four SGARs and three FGARs (chlorophacinone, diphacinone, and warfarin) are currently labelled for use in the US by the Environmental Protection Agency (EPA; US Environmental Protection Agency, 2008). The other compounds may still be detected should stockpiles of these compounds remain available for use given their long shelf life. We note that two of these compounds have potential sources other than direct use for rodent control. Warfarin is used as a medicinal blood thinner for people that can be found at low levels in urban and hospital wastewater (Santos et al., 2013; Regnery et al., 2020), while dicoumarol can be produced naturally in moldy yellow sweet clover (Melilotus officinalis; Hroboňová et al., 2018). Therefore we take caution when interpreting the point source of contamination by either of these compounds should they be prevalent.
For a given compound we recorded concentration in mg/kg when levels met or exceeded the method detection limit (MDL), else exposure was recorded as ‘trace.’ Trace detections are treated as positive detections, recognizing their presence in amounts below the quantifiable MDL. Based on the liquid chromatography mass spectrometry method used to quantify AR concentrations, the MDLs for our 11 compounds of interest were 0.010 mg/kg for brodifacoum and difenacoum, 0.025 mg/kg for bromadiolone, 0.050 mg/kg for chlorophacinone, difethialone, and diphacinone, and 0.100 mg/kg for coumachlor, coumafuryl, dicoumarol, pindone, and warfarin.
Measured AR concentration (Supplementary Table 1) was not used as a response variable in the analyses to follow because many factors affect concentrations, including time since ingestion and individual differences in metabolic rates. As a result, observed concentration levels do not reliably reflect the animal’s initial dose of ARs or consequent health effects of exposure (Vandenbroucke et al., 2008; Rattner and Mastrota, 2017). Following Van den Brink et al. (2018), we instead measured AR exposure in two ways. First, at the individual level we classified samples as positive (binary response variable) when ≥1 AR was detected in at least trace levels, and we summarized the percent of the population testing positive for ≥1 compound at the state and regional level. Second, we quantified the number of different compounds detected within a given individual (ordinal response variable), assuming each new compound to represent a different exposure event. Using both the binary and ordinal response variables we investigated broad-scale patterns in AR exposure via sample interpolation and tested finer-scale drivers of exposure using regression methods as described in the sections to follow.
2.2 Broad-scale patterns of AR exposure
We mapped broad-scale patterns in AR exposure by first summarizing exposure rates by state and second by kriging each of our two response variables from the initial sample locations. Kriging predicts values at unsampled locations based on the pattern of spatial autocorrelation among samples, and better accounts for uncertainty in exact sampling locations and values compared to other interpolation methods (Krige, 1966). Importantly, the finest resolution reported for fisher samples was the township, which varied in shape and ranged in size from 2.24–1254.98 km2. To account for uncertainty in the precise location of a sampled fisher, and by extension the potential spatial drivers of AR exposure, we adapted multiple imputation techniques (Schafer, 1999). Our approach involved iteratively simulating missing data (in this case the geographic coordinate of sample location within its assigned township) to create plausible realizations of complete data, fitting models to each replicate of completed data, and combining inference across the multiple iterations to bracket uncertainty. For our purposes, we chose 10 random imputations for each sample to balance computing demands with the need to capture sufficient variation in spatial pattern (Spratt et al., 2010). We then ran the kriging analysis separately for each of the 10 imputed datasets and averaged the resulting outputs. Spatial data were managed within ArcMap V.10.8 (ESRI, 2011) and QGIS V.3.22 (QGIS Development Team, 2021).
We conducted kriging using a grid cell size of 30 km2, which approximates the largest home range sizes reported for fishers in the region (Arthur et al., 1989; P. Jensen, NY DEC, unpub. data). This analysis was conducted first for fisher exposure to at least one AR compound (1 = exposed to any compound at trace level or higher, 0 = no compounds detected) and then for the total number of compounds an individual was exposed to (ranging from zero to six). We log-transformed the number of AR compounds to ensure predictions were ≥0 and, following the kriging prediction, back-transformed values to their original scale using the bias correction approach proposed by Laurent (1963; Yamamoto, 2007). Kriging analysis and transformation were performed in program R V.4.2.2 (R Core Team, 2022) using the gstat package (Pebesma, 2004; Gräler et al., 2016).
2.3 Fine-scale drivers of AR exposure
The same imputed datasets and dependent variables as described above were used to investigate fine-scale drivers of AR exposure. Here, we related individual samples to variables representing alternative potential routes of exposure, specifically: (1) agricultural land use, (2) human development, and (3) protected areas.
2.3.1 Variable selection process
Given uncertainty with respect to the appropriate variable to use to represent each driver, and the spatial scale over which drivers influenced AR exposure, we first screened variables using a model selection process to choose the most informative variable(s) to represent each driver in global exposure models. We calculated three variables representing agricultural land use based on the 2019 National Land Cover Database (NLCD; Dewitz and US Geological Survey, 2021). In the area surrounding each sample location we calculated the percent cover of pasture, percent cover of row crops, and percent cover of pasture and row crops combined.
To best capture spillover toxicity from application of ARs deployed near buildings, our measure of developed landscapes focused on 30-m resolution wildland-urban interface (WUI) maps of the conterminous US (Carlson et al., 2022). WUI data are divided into “intermix”, representing low density structures interspersed in an area covered by ≥50% wildland vegetation, and “interface” where structures are next to wildland areas and show a clear divide between them. Vegetated wildland includes undeveloped forest, wetland, shrubland, or grassland as delineated in the 2016 NCLD, and in the Northeast wildland predominantly reflects forest cover (Peach et al., 2019). Carlson et al. (2022) defined an area as interface, intermix, or non-WUI based on thresholds of building and wildland density calculated within radii of 100- or 500-m (Supplementary Material). We calculated percent cover of interface, percent cover of intermix, and percent cover of interface and intermix combined, each measured using the 100- or 500-m radii. Further, we calculated the count of building centroids around each sample location based on the Microsoft building footprints layer (Bing Maps Team, 2018; Heris et al., 2020).
Lastly, we extracted the boundaries and metadata on protected areas from the Protected Areas Database of the United States (PADUS; US Geological Survey and Gap Analysis Project, 2022). We calculated the percentage of area around each sample location having the highest levels of protection (GAP codes 1 and 2) separately from that covered by protected areas where land is protected from conversion but extractive uses such as logging are allowed (GAP code 3). This is the only driver category where we included two rather than a single variable to represent the category.
Uncertainty further existed in the spatial scale over which landscape variables may influence AR exposure. Based on reported fisher home range sizes (Arthur et al., 1989), we calculated percent cover of each variable within 15-, 30-, and 60-km2 buffers. To identify the most informative variable and scale within each category of landscape driver, we conducted a multi-scale modeling approach (Leblond et al., 2011; Jensen and Humphries, 2019) using Akaike’s information criterion corrected for small sample sizes (AICc; Burnham and Anderson, 2002). To capture the full range of potential variable values we pooled all 10 iterated locations for each sample, included a random effect of sample ID to account for sample replication, and fit univariate models for each variable at each buffer size. Considering that intermediate values of WUI and agricultural variables may best correspond to optimal fisher habitat (Kordosky et al., 2021), we tested for nonlinearity in these variables by including quadratic terms. All modeling was performed in program R V.4.2.2 (R Core Team, 2022).
For binary response variables, we fit logistic mixed-effects models using the package lme4 (Bates et al., 2015). For the count of AR compounds, we identified under dispersion by initially fitting a null mixed-effects model using the Poisson error distribution (ĉ = 0.03). Under-dispersion could be due to our sample selection process, which aimed to maximize spatial coverage and potentially led to a more uniform, rather than random, sample. Under-dispersion also could be due to the nature of the response variable, which ranged from zero to six compounds detected per individual. 74.1% of tested fisher livers contained zero to two AR compounds, leading to lower variance than would be expected for a Poisson distribution. To handle under dispersion in the analyses to follow, we fit under-dispersed mixed-effects models with a Conway-Maxwell-Poisson error distribution in the package glmmTMB (Brooks et al., 2017; Sellers and Premeaux, 2021). All landscape variables were centered and standardized using their standard deviation before modeling.
2.3.2 Global models of AR exposure
After identifying the most informative variable and scale within each category of landscape driver (agricultural land use, human development, and protected areas), we fit global models to each of the 10 imputed datasets to estimate final parameter values for each potential driver. When fitting each global model, we included a random effect of state to account for broad-scale spatial correlation. Ultimately, we averaged parameter estimates across the iterations and random effect levels. To interpret variable importance, we calculated the 95% confidence interval (CI) for final coefficient estimates using their summed standard errors divided by 10 (the number of iterations). A variable was considered statistically significant when the resulting 95% CI did not contain zero. We also calculated odds (for exposure models) and rate ratios (for number of compounds) by exponentiating averaged beta parameters. Ultimately, we used the final models to produce spatially-explicit predictions for the probability of fisher exposure to any AR compound, and to a specific number of compounds, across the northeastern US.
3 Results
Pooling data across states we detected ≥1 AR compound in 78.6% of sampled fishers (N=597 samples; Table 1). Of the 11 compounds tested for, fisher exhibited at least trace levels of eight—all four SGARs (brodifacoum, bromadiolone, difenacoum, and difethialone) and four FGARs (chlorophacinone, dicoumarol, diphacinone, and warfarin). The majority (55.3%) of fishers tested positive for more than one compound. The highest number of compounds detected in one individual was six (Table 1). Of the 1,022 AR detections (i.e., the sum of individual detections of each compound), 527 (51.6%) were detected at trace levels while 495 (48.4%) were present in quantifiable concentrations (Figure 1A). Of the 160 fishers exhibiting ARs in trace levels only, 96 (60%) tested positive for only a single compound while the rest had 2-4 compounds present. Diphacinone, a FGAR, was the most frequently detected compound (384fishers, 64.3% of all tested), followed by two SGARs: brodifacoum (321 fishers, 53.8%) and bromadiolone (167 fishers, 28.0%). Overall, 57.6% of diphacinone detections (N=221), 35.2% of brodifacoum detections (N=113), and 37.7% of bromadiolone detections (N=63) were present at trace levels.
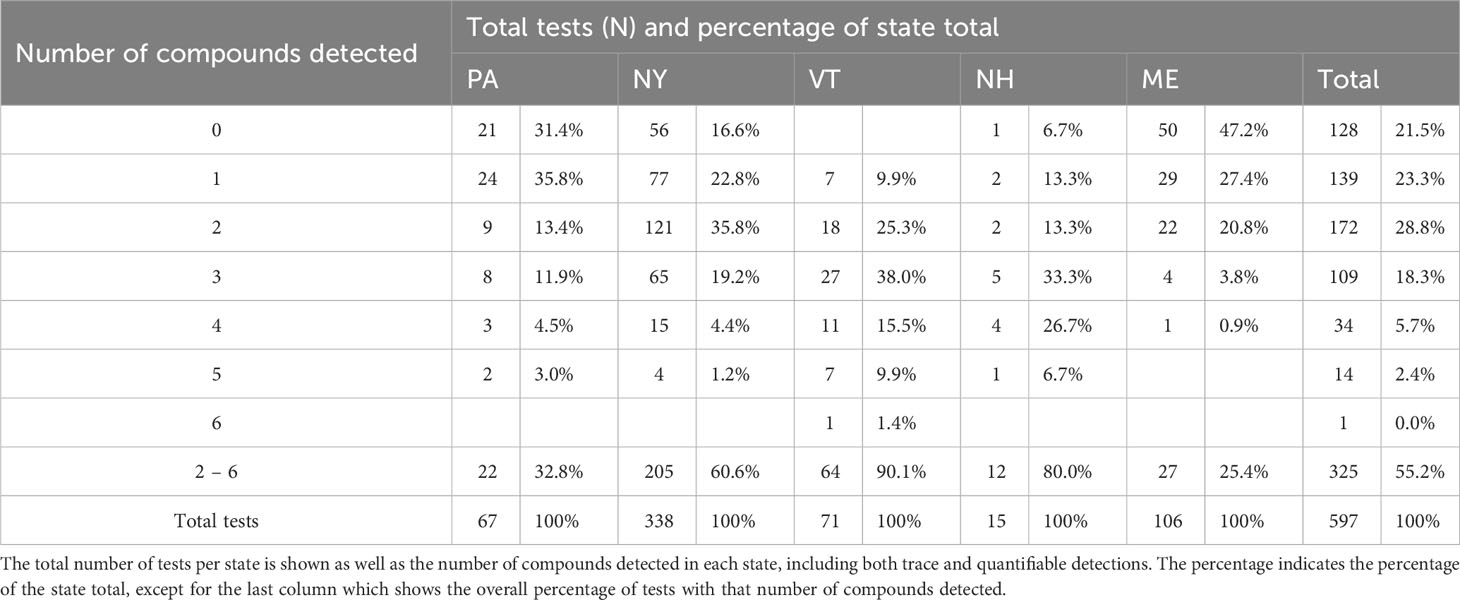
Table 1 Summary of tests for 11 anticoagulant rodenticides in 597 fishers collected as part of the legal harvest in the northeastern United States from 2018-2022.
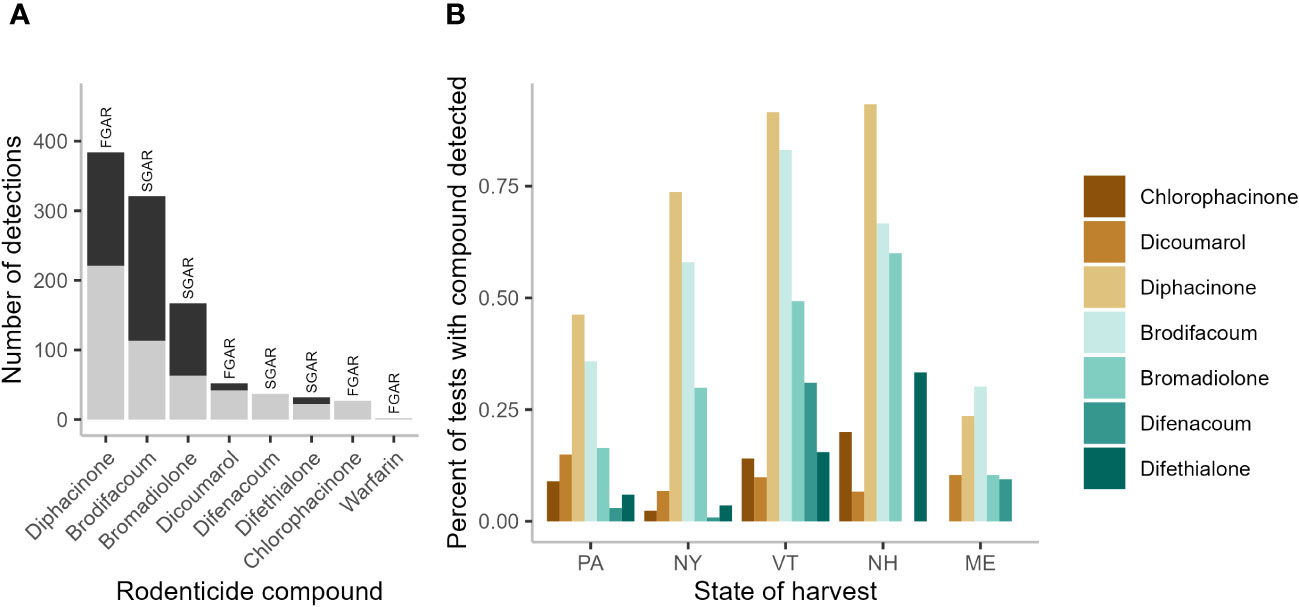
Figure 1 Summary of anticoagulant rodenticides (AR) in 597 fisher livers collected in the northeastern United States, 2018–2022. Count of AR detections (N=1,010) by compound with trace (gray bars) versus quantifiable levels (black bars) (A), and the percent of fishers testing positive for individual AR compounds in in each state: Pennsylvania (PA), New York (NY), Vermont (VT), New Hampshire (NH), and Maine (ME) (B), with first-generation ARs (FGARs) in shades of brown, and second-generation ARs (SGARs) in shades of green. Two detections of the FGAR warfarin, both in NY, were not included for clarity.
3.1 Broad-scale pattern of AR exposure
Diphacinone was the most prevalent compound detected in all states except ME where overall detection levels were lowest and brodifacoum was more common (Figure 1B). Outside of ME, brodifacoum was the second most common compound detected across states. Bromadiolone was the third most common compound across the region, with its prevalence highest in NH (Figure 1B). States differed in the overall number of compounds detected (lowest in ME, highest in NY; Figure 2A) and in the percentage of the sampled fisher population exposed to at least one compound (lowest in ME at 52.8%, highest in NH and VT at 93.3–100%; Figure 2B).
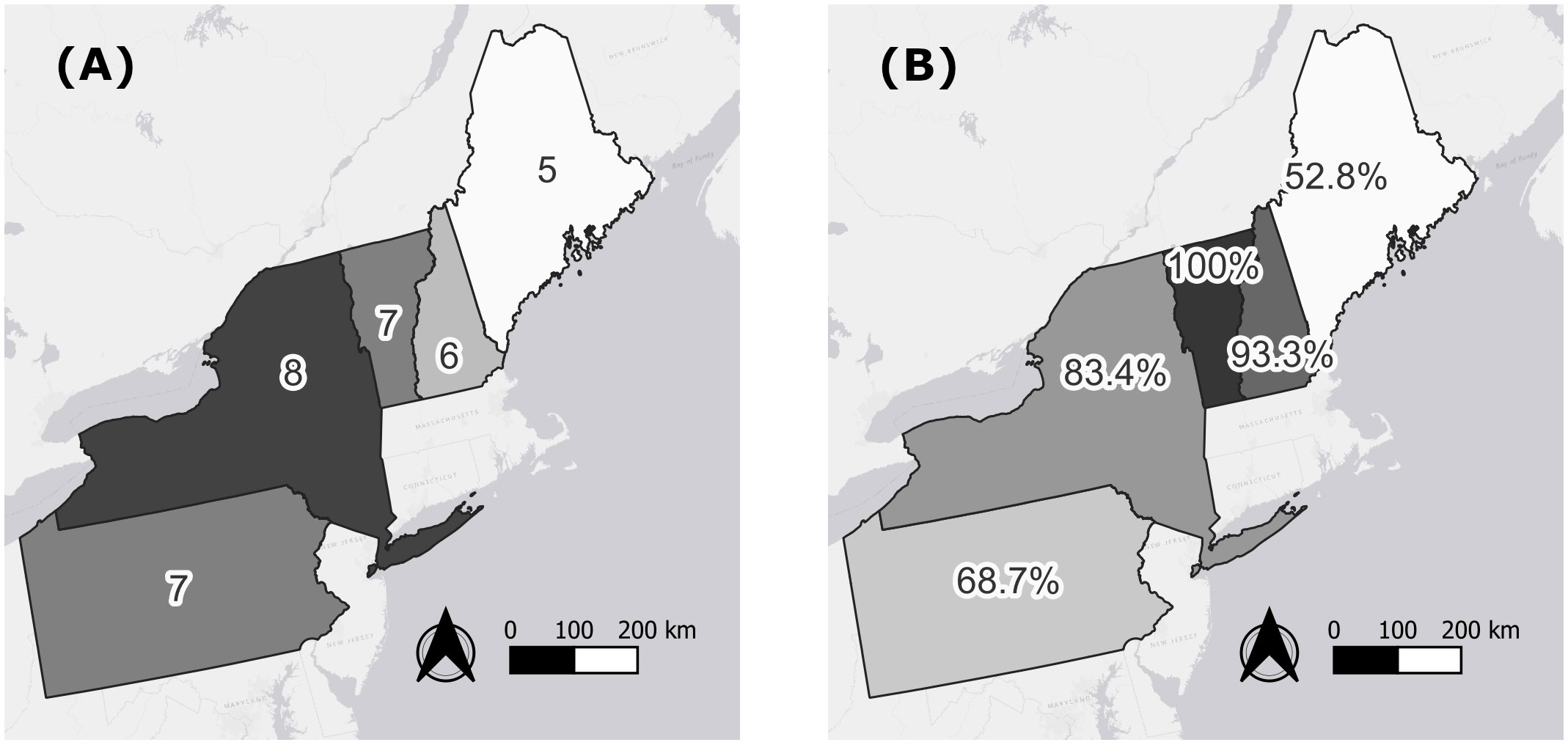
Figure 2 Number of anticoagulant rodenticide compounds detected in 597 fisher livers collected across the northeastern United States from 2018–2022 (A) and percentage of sampled fishers exposed to at least one compound (B) by state.
In PA we included samples from harvested fisher as well as opportunistic samples of which 14 (61%) were killed by vehicle collision and the rest had either unknown causes of death or were killed by gunshot. All tests from PA were included in subsequent analysis, as the proportion of fishers testing positive for ≥1 AR was not significantly different (χ2 = 0.155, df=1, P=0.69) between trapped (66%, N=44) and opportunistically collected fishers (74%, N=23).
Kriging predictions for our two response variables, averaged across the 10 imputed datasets, indicated spatial autocorrelation in AR exposure that extended over a range of ~750 km and revealed a hot zone spanning southern NH, southern VT and southeastern NY (Figure 3). Spatial autocorrelation explained ~37–40% of the total variation, leaving unexplained ~60% of the variation that may be due to finer-scale spatial processes.
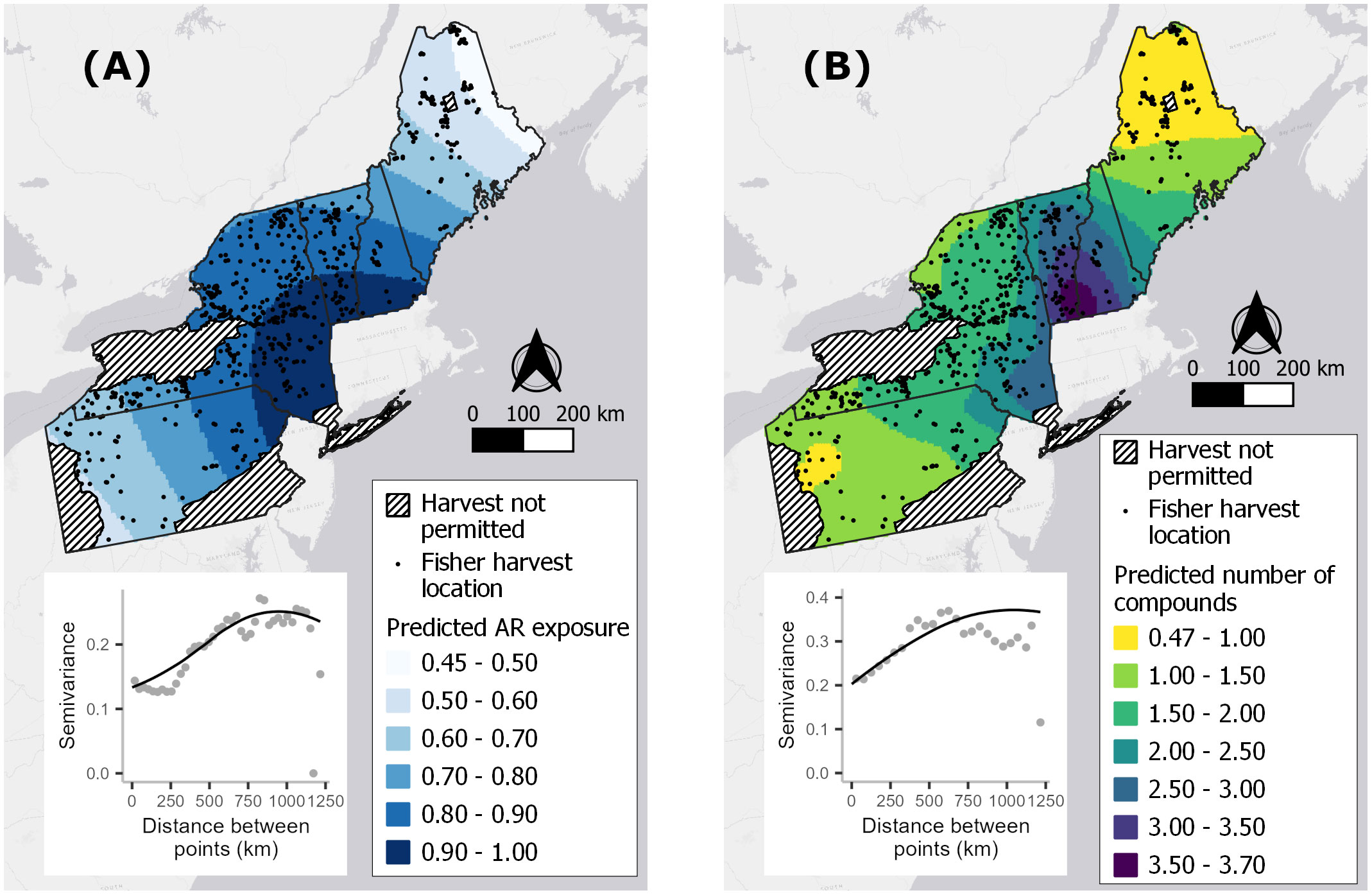
Figure 3 Averaged kriging predictions for the probability of fisher exposure to at least one anticoagulant rodenticide (AR) compound (A) and the predicted number of compounds detected per fisher (B) based on 597 fisher livers collected in the northeastern United States from 2018–2022. One of 10 imputed sets of sample locations underlying these maps (black dots), state boundaries, and map inserts of one example semivariogram used to make kriging reductions.
3.2 Fine-scale drivers of AR exposure
3.2.1 Binary exposure models
3.2.1.1 Selection of variables for inclusion in global models
We first screened alternative variables representing our three hypothesized drivers of AR exposure (agricultural land use, human development, and protected areas). Given high model selection uncertainty, we retained the top ranked variable within each category for inclusion in our global model (see Supplementary Material for model selection details). To represent agricultural land use, we retained percent cropland at the 60 km2 scale (percent cropland at 30 km2 ranked second), although equal AIC weight was prescribed to cropland, pasture, or both combined at the 15-, 30, and 60-km2 scales (Supplementary Table 2). To represent human development, percent cover of intermix, interface, or both combined were prescribed equal AIC weight at all 3 scales, while models including solely interface or building density were consistently ranked lower (Supplementary Table 3). The top ranked variable selected for inclusion in our global model was percent intermix (100-m grid cell) quantified within the 60-km2 buffer. Lastly, to represent protected areas we opted to include percent cover of highly protected areas separately from percent cover of moderately protected areas, each measured at the 60-km2 scale (Supplementary Table 4).
FGAR and SGAR compounds may be used primarily by public versus pest control and agricultural professionals, respectively, pointing to different routes of wildlife exposure and different avenues for potential mitigation. As such, we re-ran variable selection procedures to independently consider exposure to each of the top three compounds (diphacinone, brodifacoum, and bromadiolone) detected within fisher. When considering agricultural routes of exposure to diphacinone (a FGAR), the proportion of pasture at the 60-km2 ranked highest (Supplementary Table 5) whereas the proportion cropland at the 60-km2 scale ranked highest for exposure to the two SGARs brodifacoum (Supplementary Table 6) and bromadiolone (Supplementary Table 7). To represent human development, percentage intermix at the 60-km2 scale was retained for inclusion in our global model for all three ARs, with the variable derived at the 500-m grid cell size for diphacinone and at the 100-m cell size for the two SGARs (Supplementary Tables 8–10). And again, for protected areas, we separately retained proportion of high-protected areas versus proportion of moderately protected areas at the 60-km2 scale for each of the three ARs (Supplementary Tables 11–13).
All selected variables were included in our binary models as linear rather than nonlinear effects.
3.2.1.2 Global models predicting AR exposure (binary outcome)
Only WUI exhibited a positive and statistically significant effect on the probability of overall AR exposure, a pattern that remained consistent when predicting exposure to each of the top three AR compounds alone (Table 2; Figure 4A). The odds of AR exposure, overall or individually to brodifacoum and bromadiolone, increased by 1.4–1.9 times, while exposure to diphacinone increased 1.1 times, given each 10% increase in percent cover of intermix. Unexpectedly, percent agricultural cover was not a significant predictor of overall exposure or exposure to diphacinone, with high associated uncertainty (Table 2; Figure 4A). However, modeling exposure to specific AR compounds revealed stronger trends towards decreased exposure to the SGARs bromadiolone and brodifacoum with increasing amounts of agricultural land use (Table 2; Figure 4A). Lastly, percentage of moderately or highly protected areas indicated statistically non-significant trends with overall AR exposure (Table 2). Spatially-explicit predictions of overall AR exposure were therefore driven by the prevalence of wildland-urban intermix and showed high probability of exposure (> 0.80) across the region (Figure 5A).
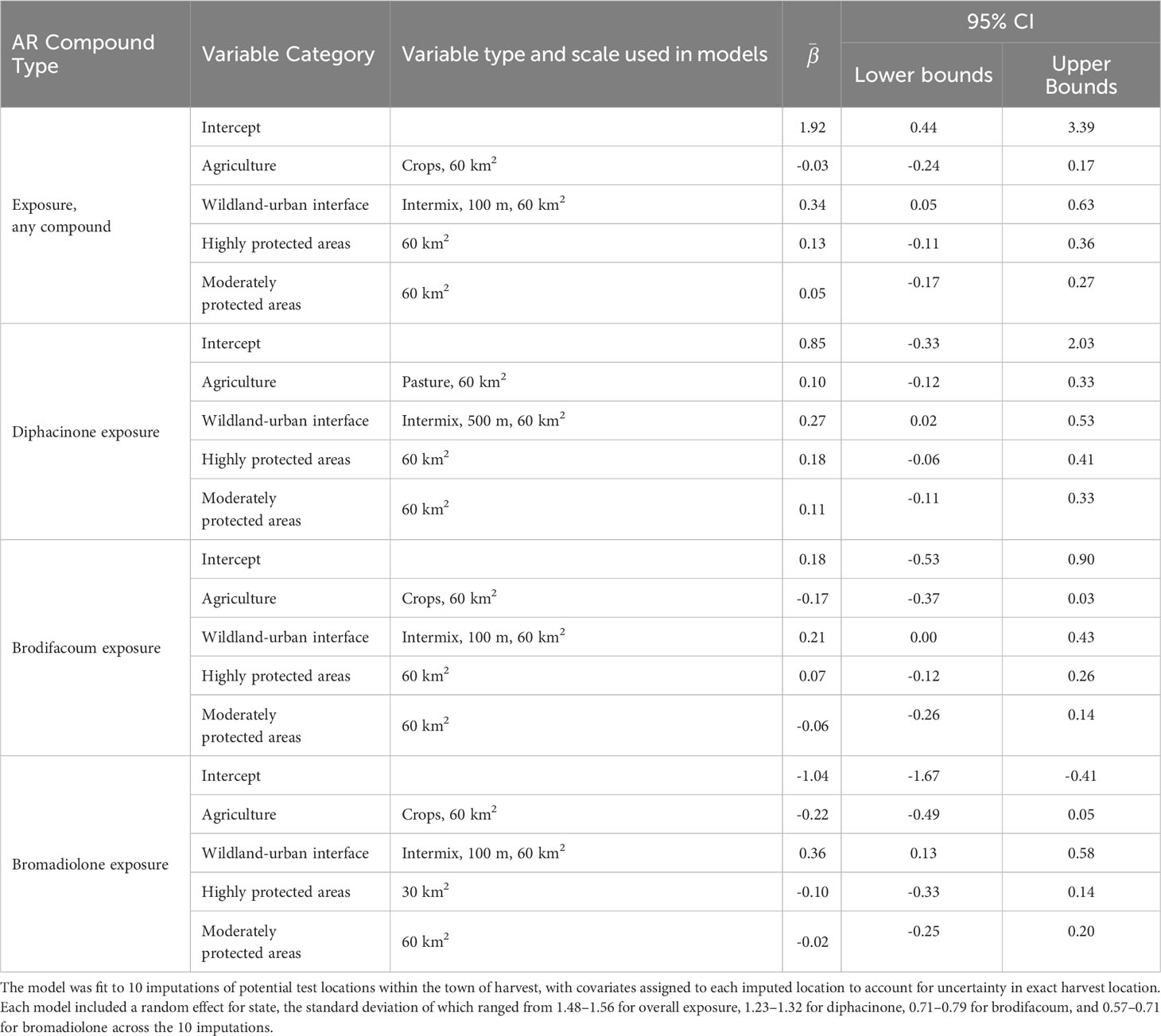
Table 2 Summary of the averaged global model comparing fisher exposure to at least one anticoagulant rodenticide (AR), and to diphacinone, brodifacoum, or bromadiolone alone to four categories of landscape variables, including the final variable type and scale used in the models, average parameter estimates (), and 95% confidence intervals (CI).
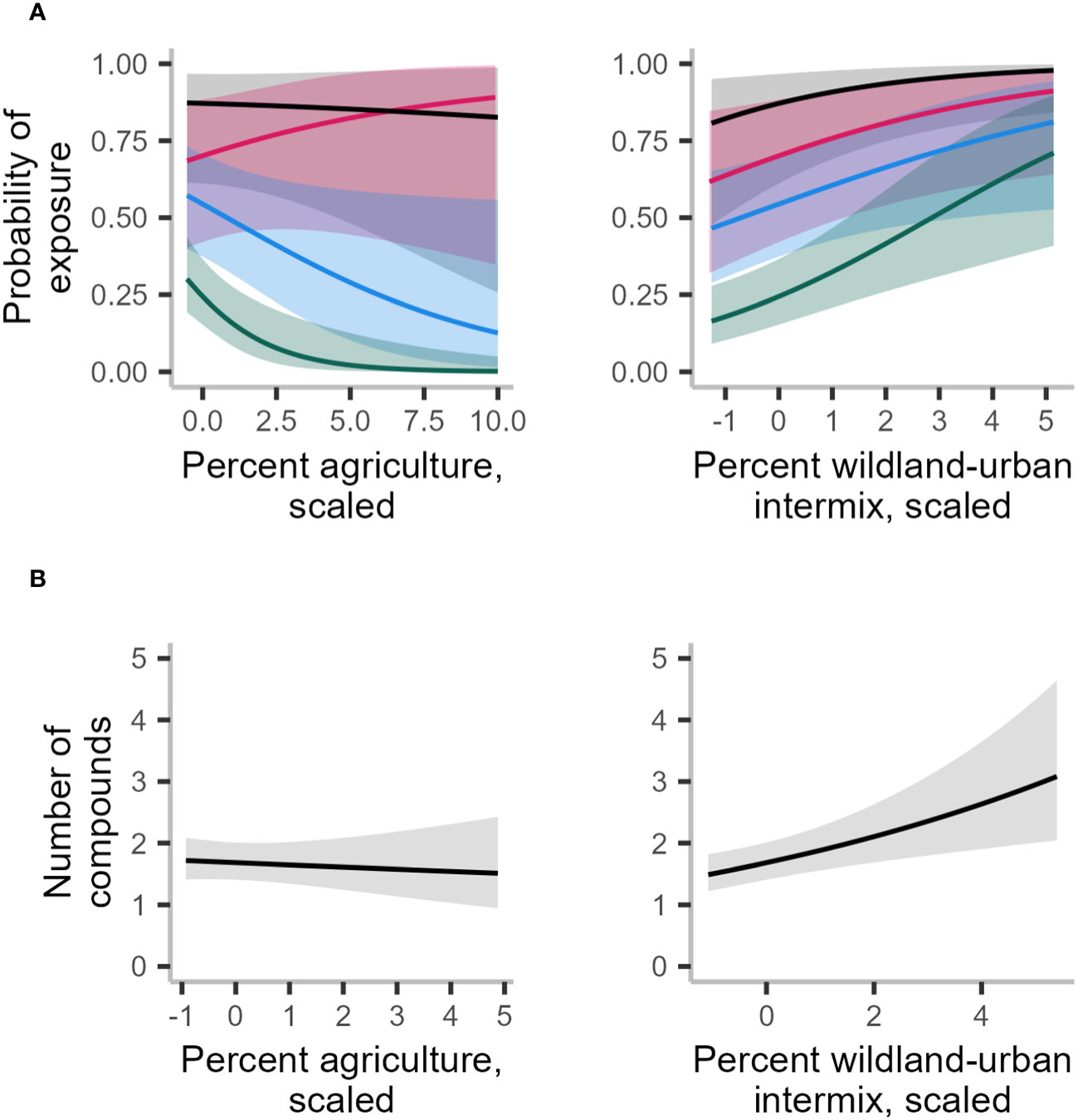
Figure 4 Averaged marginal effects of landscape variables from 10 imputed test locations on probability of exposure to any rodenticide (black line), to diphacinone (red line), to brodifacoum (blue line), and to bromadiolone (dark green line) (A) and number of compounds detected (B) in 597 fisher livers collected in the northeastern United States from 2018–2022. All variables were calculated in a 60-km2 buffer except for wildland-urban intermix for number of compounds models, which was calculated in a 15-km2 buffer. Predictions were based on 10 iterations of the global model. Predictions were also averaged across each level of the random effect for state.
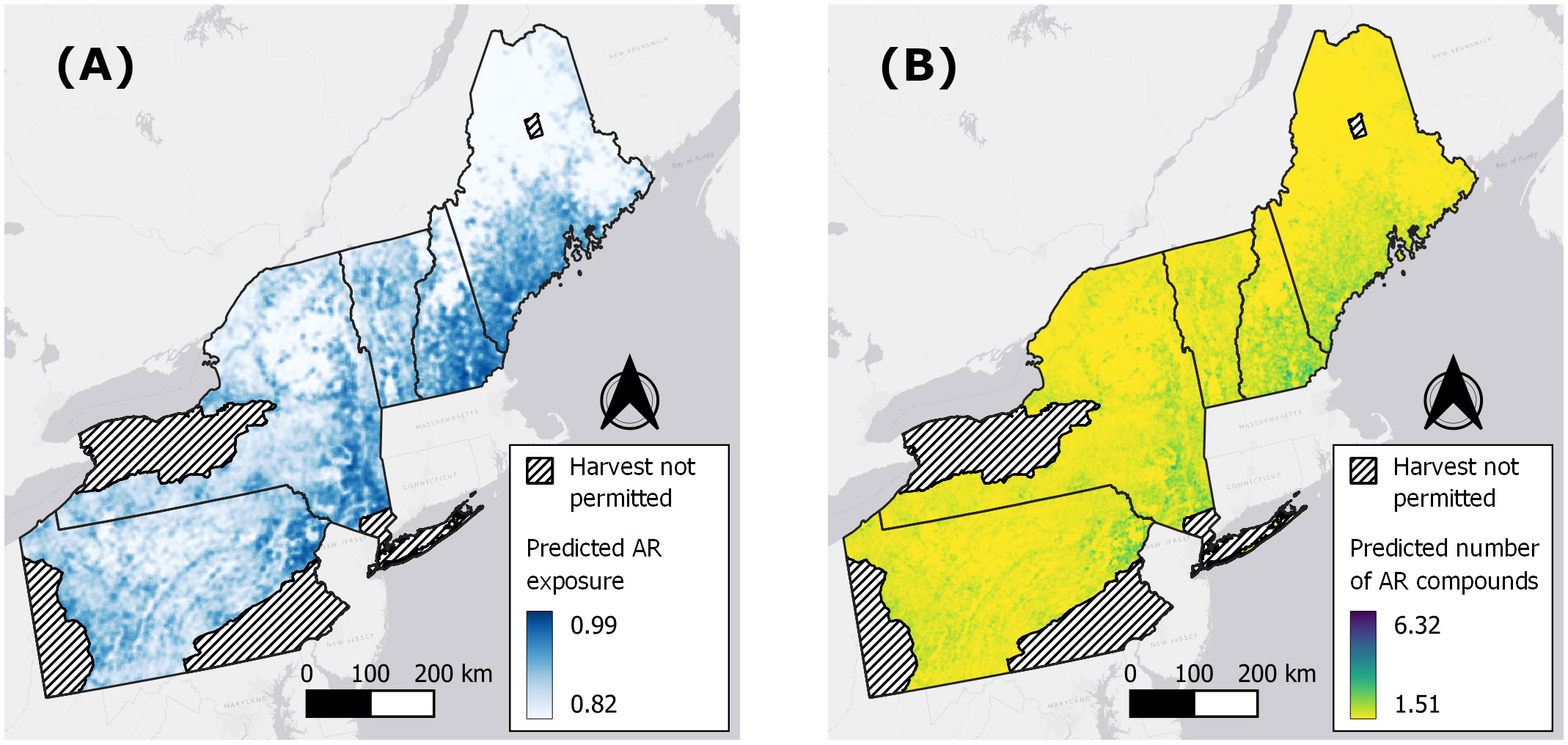
Figure 5 Spatially-explicit predictions of anticoagulant rodenticide (AR) exposure (A) and number of AR compounds (B) in fishers based on models fitted to 597 livers collected in the northeastern United States from 2018–2022.
3.2.2 Count of compounds models
3.2.2.1 Selection of variables for inclusion in global models
We followed the same variable selection process as described for the binary response models. To represent agricultural land use in our global model we retained the percentage of all agriculture (row crops + pasture) at the 30 km2 scale, which exhibited no model selection uncertainty (Supplementary Table 14). Likewise, there was no model selection uncertainty when selecting proportion intermix (100m grid cell, 15-km2 scale) to represent human development (Supplementary Table 15). Lastly, we retained the proportion of highly protected areas, and separately the proportion of moderately protected areas, quantified at the 60-km2 scale (Supplementary Table 16).
3.2.2.2 Global models predicting AR exposure (number of compounds)
As with the binary exposure model, wildland-urban-intermix exhibited a positive and statistically significant association with the number of AR compounds fisher were exposed to (Table 3; Figure 4B). With an incident rate ratio of 1.15, the number of compounds detected per fisher was predicted to increase by 15% with each 10% increase in intermix cover. Agricultural landcover and percent cover of protected areas exhibited no significant relationship with the number of compounds detected (Table 3; Figure 4B). As for overall exposure, spatially-explicit predictions for the number of compounds were driven by the prevalence of WUI (Figure 5B), the distribution of which largely explains the hot zone revealed in our kriging analysis and predicted exposure to ≥ 1 compound per fisher across the region.
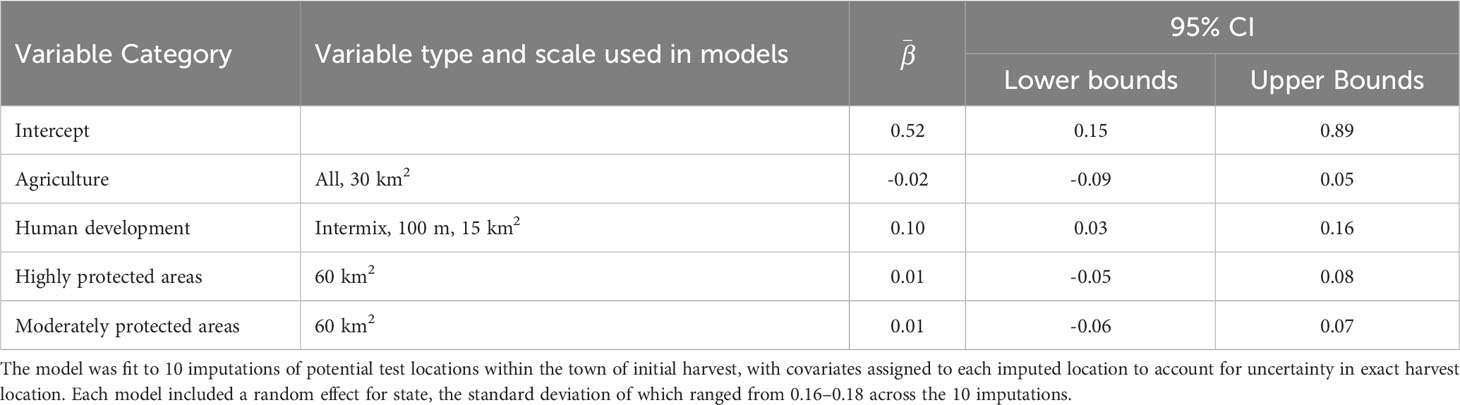
Table 3 Summary of the averaged global model comparing the number of compounds detected per fisher to four categories of landscape variables, including the final variable type and scale used in the models, average parameter estimates (), and 95% confidence intervals (CI).
4 Discussion
Consistent with studies of fisher and other mesocarnivores on the west coast of the U.S. (Gabriel et al., 2012; Serieys et al., 2015; Ruiz-Suárez et al., 2016), we observed high rates of exposure to ≥1 AR compound in fishers across the northeastern U.S, with rates ranging 52.8-94.4% depending upon state and 77.9% overall. Although Maine exhibited the lowest rates of AR exposure, our expectation of increasing AR exposure along the southwesterly axis of increasing population density by state was not realized. Instead, we revealed a hot zone of AR exposure in fisher spanning southern NH, southern VT, and southeastern NY—the region encompassing the higher population densities that emanate outward from large metropolitan centers rather than falling along state lines. This hot zone corresponded most directly with higher proportions of wildland-urban-intermix, the single most important driver of AR exposure in this region and one that we consider in more depth later. Fisher have recently expanded their range into more human-impacted landscapes following long-term reforestation trends across this region, which apparently comes at a cost in terms of exposure to environmental toxins such as ARs.
Also consistent with previous studies, the majority of fisher in our study exhibited traces of 2-6 different compounds in their system, indicative of multiple exposure events and the potential for chronic, repeated exposure over time. Three compounds, one FGAR (diphacinone; available off-the-shelf for homeowners) and two SGARs (brodifacoum and bromadiolone; restricted by the US EPA to use by commercial pest control and agricultural agents), were the most prevalent in every state—being detected in 63, 53, and 28% of fisher, respectively. Studies of fishers and bobcats in California (Gabriel et al., 2012; Serieys et al., 2015), fishers in Alberta (Thomas et al., 2017), and American mink in Scotland (Ruiz-Suárez et al., 2016) each reported regular detection of brodifacoum and bromadiolone. However, our frequent detection of diphacinone proved unusual as Ruiz-Suárez et al. (2016) and Thomas et al. (2017) failed to detect diphacinone at all, and Gabriel et al. (2012) reported that only 14% of fishers in California tested positive for the compound. Serieys et al. (2015) observed diphacinone detections to increase over time, peaking in 2012 (the last year they tested). Our results could represent a continuation of that trend or could point to regional variation in diphacinone use. In trying to determine point sources of exposure for fishers, it is important to recognize that EPA regulations cover where SGARs should be sold and used, but not who can purchase them, meaning that even SGARs remain accessible to the public at local hardware stores and online venues. Moreover, Brodifacoum and bromadiolone exhibit high liver retention rates, which may affect the frequency and magnitude at which they are detected compared to FGARs having shorter elimination half-lives (Eason et al., 2002; Erickson and Urban, 2004; Vandenbroucke et al., 2008). As a result, detection of SGARs does not point specifically to a commercial pest control or agricultural application (Bartos et al., 2011), and their frequency of detection within fisher may not reflect the relative magnitude of their use on the landscape. Moreover, while it seems logical that licensed professionals would be more likely to adhere to regulations given that state licensing requires education about safe and effective pesticide use, there are limited data available to test this assumption. There is, however, evidence of widespread non-compliance with regulations in public use of ARs (Bartos et al., 2011; Tosh et al., 2011). Moreover, up to 80% of surveyed households in California and farmers in Ireland performed pest control on their own without hiring a contractor (Bartos et al., 2011; Tosh et al., 2011). Given the patterns we observed, it seems likely that off label, non-professional use of ARs is driving the high non-target wildlife exposure rates observed in the Northeast.
Private and off-label application of ARs around primary residences, seasonal residences, and associated outbuildings would be consistent with our observation of wildland-urban intermix as the primary driver of AR exposure in fisher. Notably, a study in California indicated that residents of neighborhoods having relatively lower housing density were more likely to suffer rodent problems and employ AR products compared to more densely settled neighborhoods (Morzillo and Mertig, 2011). Proximity of wildland-urban-intermix, or the more starkly contrasted wildland-urban interface, has similarly been related to AR exposure in Barred owls (Strix varia) in California (Hofstadter et al., 2021). However, no such pattern was identified in fisher populations in California, where the species is largely constrained to public forests and parks having little to no associated urban development (Proulx et al., 2004; Lofroth et al., 2010; Suffice et al., 2020). The high-level of AR exposure in California fishers has instead been attributed to illegal marijuana cultivation rather than residential or commercial applications (Gabriel et al., 2012). We lack data on illegal marijuana grow operations within northeastern forests, although they do exist. However, potential sources of commercial application within forests also would be consistent with wildland-urban-intermix, such as logging encampments, maple sugaring operations (Houston et al., 1990), and even maintenance buildings associated with protected areas. Such deployments might help explain why the nearly 15 million acres of protected areas in the region (Radeloff et al., 2010; Martinuzzi et al., 2013) provided no respite for fisher in terms of AR exposure. That fisher home ranges cover areas larger than many protected patches in the Northeast, it is also likely that foraging fishers regularly move beyond the boundaries of protected areas and into adjacent residential or agricultural areas (Arthur et al., 1989; Purcell et al., 2012).
Livestock farming has specifically been implicated in AR exposure in other taxa (Geduhn et al., 2015; López-Perea et al., 2019; Badry et al., 2022), however the majority of studies reporting such effects stemmed from Europe. Studies of overall AR exposure in owls found no relationship to agriculture in Australia or in Oregon and Washington, USA (Wiens et al., 2019; Cooke et al., 2022). However, our study indicated that pooling together different AR compounds, and even landscapes having different agrarian contexts, may muddy inferences with respect to agricultural drivers of AR exposure. When looking at individual AR compounds, we observed a slight positive association between percentage pasture and detection of diphacinone and negative relationships between percentage cropland and exposure to brodifacoum and bromadiolone—yielding a marginal and non-significant effect when pooling multiple compounds together. Moreover, pooling data across regions that vary in agricultural intensity and practice may further muddy inferences. New Hampshire’s agricultural sector production in 2021 was valued at almost one quarter of that of Vermont, even though the states are almost exactly the same size, while NY’s production that year was valued at 7.3 times that of the much larger state of Maine (US Department of Agriculture, Economic Research Service, 2023). Another complicating factor is apparent in NY State, where the majority of concentrated agricultural land use coincides with areas where fisher populations are considered too sparse to support a sustainable harvest. As a result, working with samples from the regulated trapping season, we likely lacked sufficient geographic coverage of exposure to agricultural land use so as to effectively parameterize their impacts on fisher. Fisher may thus not be the most appropriate species to study agricultural drivers of AR exposure, and future studies might consider species more associated with open landscapes such as bobcats and red fox (Vulpes vulpes; Horne et al., 2009; McNitt et al., 2020). One final complication is that detection of dicoumarol, which can be found naturally in moldy yellow sweet clover (Hroboňová et al., 2018), may stem either from agricultural practices related to hay storage or to application of AR products from historical stockpiles. We note that although dicoumarol was detected in all five states in this study, it occurred in fishers at a low rate overall (8.7% of tested individuals), and was the sole compound detected in 14 individuals. Finer-grained information on AR deployment and wildlife foraging within agricultural landscapes will be needed to better gauge the relative importance of agricultural land use in the prevalence of AR exposure in fisher and other non-target species.
One benefit of working with data from the regulated wildlife harvest was access to a substantial sample size over a large geographic region that was collected within a short period of time. Though liver testing is one of the most used and reliable methods for measuring AR exposure, it necessitates the use of deceased animals, making the collection of large datasets challenging (Vandenbroucke et al., 2008; Valverde et al., 2021). Opportunistically collected carcasses (e.g., from sick animals or road kills) are commonly used to test for toxin exposure in wildlife, though these samples may bias population-level exposure rates high should exposure to the toxin render the individual more vulnerable to other mortality risks. Survival of AR-exposed animals may be decreased due either to direct AR toxicity or to sublethal effects that may decrease their hunting efficiency or increase their susceptibility to other causes of death such as vehicle strikes (Gabriel et al., 2012; Cox and Smith, 1992). Although we failed to detect a statistically-significant difference between AR exposure rates in harvested versus opportunistically-collected fisher in PA, small sample sizes may have hindered our ability to detect differences. Biases have been identified in other wildlife health surveillance programs based on sample collection methods (Diefenbach et al., 2004; Schuler et al., 2018). We consider the predominantly harvest-based sample of fisher used in this study to provide robust inference regarding levels of AR exposure in the standing live population. The primary drawback of using harvest data was the lack of precise location data, and by extension, uncertainty in the local drivers of AR exposure. Our multiple imputation approach bracketed some of that uncertainty, but uncertainty still arises regarding the space use patterns of the sampled individual and where their actual points of exposure occurred. To some degree, our large sample size should help compensate for some of that uncertainty. And importantly, one could readily secure another large sample in the future through harvest data to observe the degree to which changes in AR regulations improve non-target wildlife exposure rates—something that could not be achieved on the same scale when relying solely on mortality samples, radio-telemetry studies, or other means of monitoring AR exposure.
With this work we have provided a baseline assessment of AR exposure in a model forest carnivore, against which future samples may be compared to observe progress in reducing AR exposure or to study impacts to species health indices. Broadening surveillance to consider AR exposure across other mesocarnivores could help narrow down key point sources as well as improve the outcomes of any public campaign to improve compliance with AR regulations. Better information regarding public access to AR products, and use of AR products by the public and licensed professionals in the Northeast is needed to design the most effective measures to both conserve forest carnivores and protect human health and livelihoods in the region. Regardless of what actions may be taken to curb non-target wildlife exposure to ARs, we have demonstrated the value of using samples from the regulated harvest of animals as a low cost, rapid, effective, and statistically-defensible means of tracking the unintended effects of human activities on free-ranging carnivore populations.
Data availability statement
The raw data supporting the conclusions of this article will be made available by the authors, without undue reservation.
Ethics statement
Ethical approval was not required for the study involving animals in accordance with the local legislation and institutional requirements because all animal tissue used in this project was collected as part of the legal, regulated fur harvest in each state (Pennsylvania, New York, Vermont, New Hampshire, and Maine). As these animals would have been harvested and states would have collected tissue samples regardless of our research, our use of the samples was exempt from IACUC review.
Author contributions
GS: Formal analysis, Methodology, Validation, Writing – original draft. JF: Conceptualization, Funding acquisition, Methodology, Supervision, Writing – review & editing. LM: Conceptualization, Funding acquisition, Methodology, Resources, Writing – review & editing. JE: Conceptualization, Funding acquisition, Resources, Writing – review & editing, Validation. DN: Conceptualization, Funding acquisition, Resources, Writing – review & editing. SC: Conceptualization, Funding acquisition, Methodology, Writing – review & editing. AW: Conceptualization, Funding acquisition, Resources, Writing – review & editing. AF: Conceptualization, Funding acquisition, Resources, Writing – review & editing. PT: Conceptualization, Funding acquisition, Resources, Writing – review & editing. SW: Conceptualization, Funding acquisition, Resources, Writing – review & editing. KR: Conceptualization, Funding acquisition, Resources, Writing – review & editing. CB: Conceptualization, Funding acquisition, Resources, Writing – review & editing. TK: Writing – review & editing, Conceptualization, Funding acquisition, Resources. KS: Conceptualization, Funding acquisition, Methodology, Resources, Supervision, Writing – review & editing.
Funding
The author(s) declare financial support was received for the research, authorship, and/or publication of this article. Funding for anticoagulant rodenticide screening was provided by the Wildlife Health Lab at Cornell University, the Pennsylvania Department of Conservation and Natural Resources, the Veterinary Diagnostic Laboratory at the University of New Hampshire, the Grober Family Graduate Fellowship at SUNY College of Environmental Science and Forestry, the Pennsylvania Game Commission, the New York Department of Environmental Conservation, the Vermont Agency of Natural Resources, the New Hampshire Fish and Game Department, and the Maine Department of Inland Fisheries and Wildlife.
Acknowledgments
The authors would like to acknowledge and thank the trappers from Pennsylvania, New York, Vermont, New Hampshire, and Maine who provided carcasses for this study, as well as state biologists from the Pennsylvania Game Commission, the New York Department of Environmental Conservation, the Vermont Fish and Wildlife Department, the New Hampshire Fish and Game Department, and the Maine Department of Inland Fisheries and Wildlife for collecting tissue samples for testing. We would also like to thank the toxicology laboratory personnel at the Pennsylvania Animal Diagnostic Laboratory System in Kennett Square, PA for running all anticoagulant rodenticide tests used in this study. Finally, we would like to thank Jonathan Cohen for providing feedback on an earlier version of this manuscript.
Conflict of interest
The authors declare that the research was conducted in the absence of any commercial or financial relationships that could be construed as a potential conflict of interest.
Publisher’s note
All claims expressed in this article are solely those of the authors and do not necessarily represent those of their affiliated organizations, or those of the publisher, the editors and the reviewers. Any product that may be evaluated in this article, or claim that may be made by its manufacturer, is not guaranteed or endorsed by the publisher.
Supplementary material
The Supplementary Material for this article can be found online at: https://www.frontiersin.org/articles/10.3389/fevo.2024.1304659/full#supplementary-material
References
Arthur S. M., Krohn W. B., Gilbert J. R. (1989). Home range characteristics of adult fishers. J. Wildlife Manage. 53 (3), 674–679. doi: 10.2307/3809196
Badry A., Schenke D., Brücher H., Chakarov N., Grünkorn T., Illner H., et al. (2022). Spatial variation of rodenticides and emerging contaminants in blood of raptor nestlings from Germany. Environ. Sci. pollut. Res. 29, 60908–60921. doi: 10.1007/s11356-022-20089-1
Badry A., Schenke D., Treu G., Krone O. (2021). Linking landscape composition and biological factors with exposure levels of rodenticides and agrochemicals in avian apex predators from Germany. Environ. Res. 193, 110602. doi: 10.1016/j.envres.2020.110602
Bartos M., Dao S., Douk D., Falzone S., Gumerlock E., Hoekstra S., et al. (2011). Use of anticoagulant rodenticides in single-family neighborhoods along an urban-wildland interface in California. Cities Environ. 4 (1), 1–22. doi: 10.15365/cate.41122012
Bates D., Maechler M., Bolker B., Walker S. (2015). Fitting linear mixed-effects models using lme4. J. Stat. Software 67 (1), 1–48. doi: 10.18637/jss.v067.i01
Bing Maps Team (2018). Data from: Building Footprints: AnAI-Assisted Mapping Deliverable with the Capability to Solve for Many Scenarios (Microsoft). Available at: https://www.microsoft.com/en-us/maps/building-footprints.
Brooks M. E., Kristensen K., van Benthem K. J., Magnusson A., Berg C. W., Nielsen A., et al. (2017). glmmTMB balances speed and flexibility among packages for zero-inflated generalized linear mixed modeling. R J. 9 (2), 378–400. doi: 10.32614/RJ-2017-066
Buckley J. Y., Needle D., Cottrell W., Royar K., Tate P., Whittier C. (2023). High prevalence of anticoagulant rodenticide exposure in new england fishers (Pekania pennanti). Environ. Monit. Assess. 195, 1348. doi: 10.1007/s10661-023-11919-x
Burnham K. P., Anderson D. R. (2002). Model Selection and Multimodel Inference. 2nd ed (New York: NY: Springer-Verlag).
Carlson A. R., Helmers D. P., Hawbaker T. J., Mockrin M. H., Radeloff V. C. (2022). Data from: Wildland-urban interface maps for the conterminous U.S. based on 125 million building locations. US Geological Survey Data Release. doi: 10.5066/P94BT6Q7
Cooke R., Whiteley P., Jin Y., Death C., Weston M. A., Carter N., et al. (2022). Widespread exposure of powerful owls to second-generation anticoagulant rodenticides in Australia spans an urban to agricultural and forest landscape. Sci. Total Environ. 819, 153024. doi: 10.1016/j.scitotenv.2022.153024
Cox P., Smith R. H. (1992). “Rodenticide ecotoxicology: Pre-lethal effects of anticoagulants on rat behaviour,” in Proceedings of the 15th Vertebrate Pest Conference, vol. 15. Eds. Borrecco J. E., Marsh R. E. (Davis: University of California), 165–170.
Demographia.com (2000) United States (USA): Rural Population & Population Density by State: 2000. Available at: http://www.demographia.com/db-usa-staterural.htm.
Dewitz J., Geological Survey U. S. (2021). Data from: national land cover database (NLCD) 2019 products. US Geological Survey Data Release. doi: 10.5066/P9KZCM54
Diefenbach D. R., Rosenberry C. S., Boyd R. C. (2004). From the field: Efficacy of detecting chronic wasting disease via sampling hunter-killed white-tailed deer. Wildlife Soc. Bull. 32 (1), 267–272. doi: 10.2193/0091-7648(2004)32[267:ftfeod]2.0.co;2
Eason C. T., Murphy E. C., Wright G. R. G., Spurr E. B. (2002). Assessment of risks of brodifacoum to non-target birds and mammals in New Zealand. Ecotoxicology 11, 35–48. doi: 10.1023/A:1013793029831
Eisemann J. D., Fisher P. M., Buckle A., Humphrys S. (2018). “An international Perspective on the Regulation of Rodenticides,” in Anticoagulant Rodenticides and Wildlife. Eds. Van Den Brink N., Elliott J. E., Shore R. F., Rattner B. A. (Cham: Switzerland: Springer), 287–318. doi: 10.1007/978-3-319-64377-9_11
Elmeros M., Lassen P., Bossi R., Topping C. J. (2018). Exposure of stone marten (Martes foina) and polecat (Mustela putorius) to anticoagulant rodenticides: Effects of regulatory restrictions of rodenticide use. Sci. Total Environ. 612, 1358–1364. doi: 10.1016/j.scitotenv.2017.09.034
Erickson W., Urban D. (2004). Potential Risks of Nine Rodenticides to Birds and Nontarget Mammals: a Comparative Approach (Washington, D.C.: United States Environmental Protection Agency). Available at: http://pesticideresearchinstitute.com/site/docs/bulletins/EPAComparisonRodenticideRisks.pdf.
Gabriel M. W., Woods L. W., Poppenga R., Sweitzer R. A., Thompson C., Matthews S. M., et al. (2012). Anticoagulant rodenticides on our public and community lands: Spatial distribution of exposure and poisoning of a rare forest carnivore. PloS One 7 (7), e40163. doi: 10.1371/JOURNAL.PONE.0040163
Geduhn A., Esther A., Schenke D., Mattes H., Jacob J. (2014). Spatial and temporal exposure patterns in non-target small mammals during brodifacoum rat control. Sci. Total Environ. 496, 328–338. doi: 10.1016/j.scitotenv.2014.07.049
Geduhn A., Jacob J., Schenke D., Keller B., Kleinschmidt S., Esther A. (2015). Relation between intensity of biocide practice and residues of anticoagulant rodenticides in red foxes (Vulpes vulpes). PloS One 10 (9), 1–15. doi: 10.1371/journal.pone.0139191
Gräler B., Pebesma E., Heuvelink G. (2016). Spatio-Temporal Interpolation using gstat. R J. 8 (1), 204–218. doi: 10.1016/j.spasta.2023.100737
Heris M. P., Foks N., Bagstad K., Troy A. (2020). Data from: A national dataset of rasterized building footprints for the US. US Geological Survey Data release. doi: 10.5066/P9J2Y1WG
Hofstadter D. F., Kryshak N. F., Gabriel M. W., Wood C. M., Wengert G. M., Dotters B. P., et al. (2021). High rates of anticoagulant rodenticide exposure in California Barred Owls are associated with the wildland-urban interface. Ornithological Appl. 123 (4), 1–13. doi: 10.1093/ornithapp/duab036
Horne J. S., Haines A. M., Teaves M. E., Laack L. L. (2009). Habitat partitioning by sympatric ocelots and bobcats: Implications for recovery of ocelots in southern texas. Southwestern Nat. 54 (2), 119–126. doi: 10.1894/PS-49.1
Houston D. R., Allen D. C., Lachance D. (1990). “Sugarbush management: a guide to maintaining tree health,” in General Technical Report NE-129 (Broomall, PA: U.S. Department of Agriculture, Forest Service, Northeastern Forest Experiment Station). Available at: https://www.fs.usda.gov/research/treesearch/4180.
Hroboňová K., Machyňáková A., Čižmárik J. (2018). Determination of dicoumarol in Melilotus officinalis L. by using molecularly imprinted polymer solid-phase extraction coupled with high performance liquid chromatography. J. Chromatogr. A 1539, 93–102. doi: 10.1016/j.chroma.2018.01.043
Jacob J., Buckle A. (2017). “Use of anticoagulant rodenticides in different applications around the world,” in Anticoagulant Rodenticides and Wildlife. Eds. Van Den Brink N. W., Elliott J. E., Shore R. F., Rattner B. A. (Cham: Switizerland: Springer), 11–44.
Jensen P. G., Humphries M. M. (2019). Abiotic conditions mediate intraguild interactions between mammalian carnivores. J. Anim. Ecol. 88 (9), 1305–1318. doi: 10.1111/1365-2656.13024
Kordosky J. R., Gese E. M., Thompson C. M., Terletzky P. A., Purcell K. L., Schneiderman J. D. (2021). Landscape use by fishers (Pekania pennanti): core areas differ in habitat than the entire home range. Can. J. Zoology 99, 289–297. doi: 10.1139/cjz-2020-0073
Krige D. (1966). Two-dimensional weighted moving average trend surfaces for ore valuation. J. South Afr. Institute Min. Metallurgy 66, 13–38.
Laurent A. G. (1963). The lognormal distribution and the translation method: description and estimation problems. J. Am. Stat. Assoc. 58 (301), 231–235. doi: 10.1080/01621459.1963.10500844
Leblond M., Frair J., Fortin D., Dussault C., Ouellet J. P., Courtois R. (2011). Assessing the influence of resource covariates at multiple spatial scales: An application to forest-dwelling caribou faced with intensive human activity. Landscape Ecol. 26, 1433–1446. doi: 10.1007/s10980-011-9647-6
Lemus J. A., Bravo C., García-Montijano M., Palacín C., Ponce C., Magaña M., et al. (2011). Side effects of rodent control on non-target species: Rodenticides increase parasite and pathogen burden in great bustards. Sci. Total Environ. 409, 4729–4734. doi: 10.1016/j.scitotenv.2011.07.007
Lewis J. C., Powell R. A., Zielinski W. J. (2012). Carnivore translocations and conservation: Insights from population models and field data for fishers (Martes pennanti). PloS One 7 (3), e32726. doi: 10.1371/journal.pone.0032726
Lofroth E. C., Raley C. M., Higley J. M., Truex R. L., Yaeger J. S., Lewis J. C., et al. (2010). Conservation of Fishers (Martes pennanti) in South-central British Columbia, Western Washington, Western Oregon, and California-Volume I: Conservation Assessment (Denver, CO: US Department of the Interior Bureau of Land Management).
Lohr M. T. (2018). Anticoagulant rodenticide exposure in an Australian predatory bird increases with proximity to developed habitat. Sci. Total Environ. 643, 134–144. doi: 10.1016/j.scitotenv.2018.06.207
López-Perea J. J., Camarero P. R., Sánchez-Barbudo I. S., Mateo R. (2019). Urbanization and cattle density are determinants in the exposure to anticoagulant rodenticides of non-target wildlife. Environ. pollut. 244, 801–808. doi: 10.1016/j.envpol.2018.10.101
Martinuzzi S., Radeloff V. C., Higgins J. V., Helmers D. P., Plantinga A. J., Lewis D. J. (2013). Key areas for conserving United States’ biodiversity likely threatened by future land use change. Ecosphere 4 (5), 58. doi: 10.1890/ES12-00376.1
McMillin S. C., Hosea R. C., Finlayson B. F., Cypher B. L., Mekebri A. (2008). Anticoagulant rodenticide exposure in an urban population of the san joaquin kit fox. Proc. Vertebrate Pest Conf. 23, 163–165. doi: 10.5070/v423110369
McMillin S. C., Poppenga R. H., Chandler S. C., Clifford D. L. (2018). Anticoagulant rodenticide residues in game animals in California. Proc. Vertebrate Pest Conf. 28, 243–246. doi: 10.5070/v42811044
McNeil D. J., Nicks C. A., Wester J. C., Larkin J. L., Lovallo M. J. (2017). Diets of fishers (Pekania pennanti) and evidence of intraspecific consumption in pennsylvania. Am. Midland Nat. 177 (2), 200–210. doi: 10.1674/0003-0031-177.2.200
McNitt D. C., Alonso R. S., Cherry M. J., Fies M. L., Kelly M. J. (2020). Influence of forest disturbance on bobcat resource selection in the central Appalachians. For. Ecol. Manage. 465, 118066. doi: 10.1016/j.foreco.2020.118066
Morzillo A. T., Mertig A. G. (2011). Linking human behaviour to environmental effects using a case study of urban rodent control. Int. J. Environ. Stud. 68 (1), 107–123. doi: 10.1080/00207233.2010.527462
Murray M. (2020). Continued anticoagulant rodenticide exposure of red-tailed hawks (Buteo jamaicensis) in the northeastern United States with an evaluation of serum for biomonitoring. Environ. Toxicol. Chem. 39 (11), 2325–2335. doi: 10.1002/etc.4853
Nogeire T. M., Lawler J. J., Schumaker N. H., Cypher B. L., Phillips S. E. (2015). Land use as a driver of patterns of rodenticide exposure in modeled kit fox populations. PloS One 10 (8), e0133351. doi: 10.1371/journal.pone.0133351
Orsted K. M., Dubay S. A., Raisbeck M. F., Siemion R. S., Sanchez D. A., Williams E. S. (1998). Lack of relay toxicity in ferret hybrids fed carbaryl-treated prairie dogs. J. Wildlife Dis. 34 (2), 362–364. doi: 10.7589/0090-3558-34.2.362
Peach M. A., Cohen J. B., Frair J. L., Zuckerberg B., Sullivan P. J., Porter W. F., et al. (2019). The value of protected areas to avian persistence across 20 years of climate and land use change. Conserv. Biol. 33, 423–433. doi: 10.1111/cobi.13205
Pebesma E. J. (2004). Multivariable geostatistics in S: the gstat package. Comput. Geosciences 30, 683–691. doi: 10.1016/j.cageo.2004.03.012
Pelz H. J., Rost S., Hünerberg M., Fregin A., Heiberg A. C., Baert K., et al. (2005). The genetic basis of resistance to anticoagulants in rodents. Genet. Soc. America 170, 1839–1847. doi: 10.1534/genetics.104.040360
Proulx G., Aubry K., Birks J., Buskirk S., Fortin C., Frost H., et al. (2004). “World Distribution and Status of the Genus Martes in 2000,” in Martens and Fishers (Martes) in Human-Altered Environments. Eds. Harrison D. J., Fuller A. K., Proulx G. (New York: NY: Springer), 21–76. doi: 10.1007/b99487
Purcell K., Thompson C., Zielinski W. (2012). “Chapter 4: Fishers and American martens,” in Managing Sierra Nevada forests. Gen. Tech. Rep. PSW-GTR-237. Ed. North M. (Albany, CA: U.S. Department of Agriculture, Forest Service, Pacific Southwest Research Station), 47–60. Available at: https://www.fs.usda.gov/treesearch/pubs/41083.
QGIS Development Team (2021). QGIS Geographic Information System (Open Source Geospatial Foundation). Available at: http://qgis.osgeo.org.
Quinn N., Kenmuir S., Krueger L. (2019). A California without rodenticides: challenges for commensal rodent management in the future. Human–Wildlife Interact. 13 (2), 212–225. doi: 10.5070/V42811007
Radeloff V. C., Stewart S. I., Hawbaker T. J., Gimmi U., Pidgeon A. M., Flather C. H., et al. (2010). Housing growth in and near United States protected areas limits their conservation value. Proc. Natl. Acad. Sci. 107 (2), 940–945. doi: 10.1073/pnas.0911131107
Rady G. H., El-Mahrouky F. S., Abdelnabby H. M., Ahmed H. A. (2013). Sub-lethal and teratogenicity action of bromadiolone and chlorophacinone anticoagulant rodenticides on albino rats. American-Eurasian J. Toxicological Sci. 5 (1), 7–14. doi: 10.5829/idosi.aejts.2013.5.1.1107
Rattner B. A., Lazarus R. S., Elliott J. E., Shore R. F., van den Brink N. W. (2014). Adverse outcome pathway and risks of anticoagulant rodenticides to predatory wildlife. Environ. Sci. Technol. 48 (15), 8433–8445. doi: 10.1021/ES501740N
Rattner B. A., Mastrota F. N. (2017). “Anticoagulant rodenticide toxicity to non-target wildlife under controlled exposure conditions,” in Anticoagulant rodenticides and Wildlife. Eds. van den Brink N. W., Elliott J. E., Shore R. F., Rattner B. A. (Cham, Switzerland: Springer), 45–86.
R Core Team (2022). R: A language and environment for statistical computing (Vienna: R Foundation for Statistical Computing). Available at: https://www.r-project.org/.
Regnery J., Schulz R. S., Parrhysius P., Bachtin J., Brinke M., Schäfer S., et al. (2020). Heavy rainfall provokes anticoagulant rodenticides’ release from baited sewer systems and outdoor surfaces into receiving streams. Sci. Total Environ. 740, 139905. doi: 10.1016/j.scitotenv.2020.139905
Riley S. P., Bromley C., Poppenga R. H., Uzal F. A., Whited L., Sauvajot R. M. (2007). Anticoagulant exposure and notoedric mange in bobcats and mountain lions in urban southern California. J. Wildlife Manage. 71 (6), 1874–1884. doi: 10.2193/2005-615
Riley S. P., Sauvajot R. M., Fuller T. K., York E. C., Kamradt D. A., Bromley C., et al. (2003). Effects of urbanization and habitat fragmentation on bobcats and coyotes in southern California. Conserv. Biol. 17 (2), 566–576. doi: 10.1046/j.1523-1739.2003.01458.x
Robinson M. H., Twigg L. E., Wheeler S. H., Martin G. R. (2005). Effect of the anticoagulant, pindone, on the breeding performance and survival of merino sheep, Ovis aries. Comp. Biochem. Physiol. - B Biochem. Mol. Biol. 140 (3), 465–473. doi: 10.1016/j.cbpc.2004.11.011
Ruiz-Suárez N., Melero Y., Giela A., Henríquez-Hernández L. A., Sharp E., Boada L. D., et al. (2016). Rate of exposure of a sentinel species, invasive American mink (Neovison vison) in Scotland, to anticoagulant rodenticides. Sci. Total Environ. 569–570, 1013–1021. doi: 10.1016/j.scitotenv.2016.06.109
Santos L. H. M. L. M., Gros M., Rodriguez-Mozaz S., Delerue-Matos C., Pena A., Barceló D., et al. (2013). Contribution of hospital effluents to the load of pharmaceuticals in urban wastewaters: Identification of ecologically relevant pharmaceuticals. Sci. Total Environ. 461–462, 302–316. doi: 10.1016/j.scitotenv.2013.04.077
Schafer J. L. (1999). Multiple imputation: A primer. Stat. Methods Med. Res. 8, 3–15. doi: 10.1191/096228099671525676
Schuler K. L., Jenks J. A., Klaver R. W., Jennelle C. S., Bowyer R. T. (2018). Chronic wasting disease detection and mortality sources in semi-protected deer population. Wildlife Biol. 1, 1–7. doi: 10.2981/wlb.00437
Sellers K. F., Premeaux B. (2021). Conway–Maxwell–Poisson regression models for dispersed count data. Wiley Interdiscip. Reviews: Comput. Stat 13, e1533. doi: 10.1002/wics.1533
Serieys L. E. K., Armenta T. C., Moriarty J. G., Boydston E. E., Lyren L. M., Poppenga R. H., et al. (2015). Anticoagulant rodenticides in urban bobcats: exposure, risk factors and potential effects based on a 16-year study. Ecotoxicology 24, 844–862. doi: 10.1007/s10646-015-1429-5
Spratt M., Carpenter J., Sterne J. A. C., Carlin J. B., Heron J., Henderson J., et al. (2010). Strategies for multiple imputation in longitudinal studies. Am. J. Epidemiol. 172 (4), 478–487. doi: 10.1093/aje/kwq137
Stone W. B., Okoniewski J. C., Stedelin J. R. (2003). Anticoagulant rodenticides and raptors: Recent findings from New York 1998-2001. Bull. Environ. Contamination Toxicol. 70, 34–40. doi: 10.1007/s00128-002-0152-0
Suffice P., Cheveau M., Imbeau L., Mazerolle M. J., Asselin H., Drapeau P. (2020). Habitat, climate, and fisher and marten distributions. J. Wildlife Manage. 84 (2), 277–292. doi: 10.1002/jwmg.21795
Thomas P. J., Eccles K. M., Mundy L. J. (2017). Spatial modelling of non-target exposure to anticoagulant rodenticides can inform mitigation options in two boreal predators inhabiting areas with intensive oil and gas development. Biol. Conserv. 212, 111–119. doi: 10.1016/J.BIOCON.2017.06.005
Thompson C., Sweitzer R., Gabriel M., Purcell K., Barrett R., Poppenga R. (2014). Impacts of rodenticide and insecticide toxicants from marijuana cultivation sites on fisher survival rates in the sierra national forest, california. Conserv. Lett. 7 (2), 91–102. doi: 10.1111/CONL.12038
Tosh D. G., McDonald R. A., Bearhop S., Llewellyn N. R., Montgomery W. I., Shore R. F. (2012). Rodenticide exposure in wood mouse and house mouse populations on farms and potential secondary risk to predators. Ecotoxicology 21 (5), 1325–1332. doi: 10.1007/s10646-012-0886-3
Tosh D. G., Shore R. F., Jess S., Withers A., Bearhop S., Montgomery W. I., et al. (2011). User behaviour, best practice and the risks of non-target exposure associated with anticoagulant rodenticide use. J. Environ. Manage. 92 (6), 1503–1508. doi: 10.1016/j.jenvman.2010.12.014
US Department of Agriculture Economic Research Service (2023) Farm income and wealth statistics. Available at: https://data.ers.usda.gov/reports.aspx?ID=17839#P97a1ea8dc6164c83b83413b199fedaa2_2_185iT0R0x3.
US Environmental Protection Agency (2008) Risk Mitigation Decision for Ten Rodenticides. Available at: https://www.lexissecuritiesmosaic.com/resourcecenter/EPA-HQ-OPP-2006-0955-0764.pdf.
US Geological Survey, Gap Analysis Project (2022). Data from: Protected Areas Database of the United States (PAD-US) 3.0 (US Geological Survey). doi: 10.5066/P9Q9LQ4B
Valverde I., Espín S., Gómez-Ramírez P., Navas I., María-Mojica P., Sánchez-Virosta P., et al. (2021). Wildlife poisoning: a novel scoring system and review of analytical methods for anticoagulant rodenticide determination. Ecotoxicology 30 (5), 767–782. doi: 10.1007/s10646-021-02411-8
Van den Brink N. W., Elliott J. E., Shore R. F., Ratter B. A. (2018). Anticoagulant Rodenticides and Wildlife (Cham, Switzerland: Springer).
Vandenbroucke V., Bousquet-Melou A., De Backer P., Croubels S. (2008). Pharmacokinetics of eight anticoagulant rodenticides in mice after single oral administration. J. Veterinary Pharmacol. Ther. 31, 437–445. doi: 10.1111/j.1365-2885.2008.00979.x
Vogt J. T., Smith W. B. (2017). Forest inventory and analysis: fiscal year 2016 business report (Washington, D.C.: US Department of Agriculture, Forest Service). Available at: https://handle.nal.usda.gov/10113/7235756.
Vudathala D., Cummings M., Murphy L. (2010). Analysis of multiple anticoagulant rodenticides in animal blood and liver tissue using principles of QuEChERS method. J. Analytical Toxicol. 34 (5), 273–279. doi: 10.1093/jat/34.5.273
Walther B., Geduhn A., Schenke D., Schlötelburga A., Jacob J. (2020). Baiting location affects anticoagulant rodenticide exposure of non-target small mammals on farms. Pest Manage. Sci. 77, 611–619. doi: 10.1002/ps.5987
Wiens J. D., Dilione K. E., Eagles-Smith C. A., Herring G., Lesmeister D. B., Gabriel M. W., et al. (2019). Anticoagulant rodenticides in Strix owls indicate widespread exposure in west coast forests. Biol. Conserv. 238, 108238. doi: 10.1016/j.biocon.2019.108238
Keywords: toxins, mammals, fragmentation, urbanization, wildlife health, mesocarnivore
Citation: Silveira G, Frair JL, Murphy L, Ellis JC, Needle D, Cunningham SA, Watson A, Facka A, Tate P, Webb S, Royar K, Bernier C, Keller T and Schuler K (2024) Drivers of anticoagulant rodenticide exposure in fishers (Pekania pennanti) across the northeastern United States. Front. Ecol. Evol. 12:1304659. doi: 10.3389/fevo.2024.1304659
Received: 29 September 2023; Accepted: 22 January 2024;
Published: 08 February 2024.
Edited by:
Olga M.C.C. Ameixa, University of Aveiro, PortugalReviewed by:
Abdurrahman Aksoy, Ondokuz Mayıs University, TürkiyeMichael Coeurdassier, University of Franche-Comté, France
Copyright © 2024 Silveira, Frair, Murphy, Ellis, Needle, Cunningham, Watson, Facka, Tate, Webb, Royar, Bernier, Keller and Schuler. This is an open-access article distributed under the terms of the Creative Commons Attribution License (CC BY). The use, distribution or reproduction in other forums is permitted, provided the original author(s) and the copyright owner(s) are credited and that the original publication in this journal is cited, in accordance with accepted academic practice. No use, distribution or reproduction is permitted which does not comply with these terms.
*Correspondence: Georgianna Silveira, Z3NpbHZlaXJhQGVzZi5lZHU=
†Present address: Stephanie A. Cunningham, Department of Wildlife, Fisheries and Aquaculture, Mississippi State University, Mississippi State, MS, United States
Aaron Facka, Wildlands Network, Farmington, NM, United States