- 1Colorado Cooperative Fish and Wildlife Research Unit, Colorado State University, Fort Collins, CO, United States
- 2U.S. Geological Survey, Colorado Cooperative Fish and Wildlife Research Unit, Colorado State University, Fort Collins, CO, United States
- 3U.S. Fish and Wildlife Service, Migratory Bird Office, Albuquerque, NM, United States
- 4GeoSystems Analysis, Inc., Wildlife and Renewables Program, Corvallis, OR, United States
The Rocky Mountain Population (RMP) of greater sandhill cranes uses a key stopover area, the San Luis Valley (SLV) in Colorado. Parameters of migration phenology can differ between autumn and spring and are affected by weather and environmental factors. We hypothesized that sandhill cranes in the SLV would have a longer stopover duration in autumn than in spring, and that wind assistance, crosswinds, temperature change, barometric air pressure, and surface water area would influence persistence probability. We used data from sandhill cranes fitted with transmitters that spanned autumn and spring, 2015-2022. We used an open robust design mark-recapture model to estimate stopover duration, arrival probability, and persistence probability. We examined the effects of weather and surface water on the persistence probability for 106 sandhill cranes in the SLV. Stopover duration was longer in autumn than in spring and had higher variability across years. Arrival probability to the SLV peaked on 13 October in autumn and 21 February in spring. Persistence probability declined around mid-December in autumn and mid-March in spring. We found that several weather covariates influenced persistence in both seasons. In autumn, sandhill cranes departed the SLV with higher tailwinds, lower crosswinds, and higher surface water availability. In spring, sandhill cranes departed the SLV with lower crosswinds and higher barometric air pressure at the surface and higher wind speeds at altitudes of about 3,000 m. The effect of wind speed was stronger later in the spring. Given the lower variability of arrival and persistence probability and shorter stopover duration in spring compared to autumn, we suspect that RMP sandhill cranes are using a time-minimization strategy during spring. However, given the use of supportive winds and weather conditions ideal for soaring, RMP sandhill cranes appear to be using strategies that save energy in both seasons. Our study identifies the optimal timing of water management and surveys for RMP sandhill cranes and confirms that weather influences their persistence. Understanding differences in migration patterns between seasons and the factors that influence persistence at stopover sites will also be important for anticipating phenological impacts from climate change and land use alterations.
1 Introduction
Migration is energetically costly and exposes individuals to predators and sometimes unpredictable weather, but fast migration and early arrival to the breeding grounds can improve reproductive performance (Alerstam and Lindström, 1990; Kokko, 1999; Alerstam, 2011). Optimal migration theory predicts that birds should balance the time it takes to get to their destination with energy expenditure to maximize the benefits of migration (Alerstam and Lindström, 1990; Alerstam and Hedenström, 1998; Alerstam, 2011). There are several aspects of migration behavior that allow birds to optimize time and energy during migration, including the use of stopover areas, as they can provide refueling opportunities and allow individuals to rapidly increase nutrient reserves (e.g., lipids), which may improve fitness potential (Ankney and MacInnes, 1978; Alisauskas, 2002; Chapman et al., 2011; Schmaljohann et al., 2022). However, stopovers may increase the overall duration of migration, incur energetic settling costs, expose individuals to predation risk, and potentially result in decreased survival probability (Newton, 2007). Migratory behavior, such as total migration duration, the travel route, and optimal stopover departure timing, will vary among individuals and across seasons based on environmental and intrinsic factors (Weber et al., 1998; Karlsson et al., 2012; Nilsson et al., 2013; Åkesson and Helm, 2020). Understanding these decisions at known, key stopover areas can provide information on how populations respond to weather and habitat conditions, which may help identify potential impacts from climate and land use changes.
Migration in birds is hypothesized to evolve in response to temporal (e.g., seasonal) environmental variation, allowing for individuals to access high quality resources throughout the year and respond to changing conditions (Cox, 1985; Lundberg, 1988; Winger et al., 2019). While some aspects of migration are genetically determined (e.g., response to day length; Møller, 2001; Pulido, 2011; Åkesson and Helm, 2020), migratory behaviors are also mediated and influenced by weather and habitat (Åkesson and Hedenström, 2007; Bauer et al., 2008; Haest et al., 2020). Previous work has identified temperature, wind assistance, cloud cover, precipitation, and barometric air pressure as factors influencing migratory decisions, such as departure probability (Richardson, 1978, 1990; Shamoun-Baranes et al., 2017). Often the effects of weather covariates on migration are related to the ability of birds to optimize time and energy (Åkesson and Hedenström, 2007; Shamoun-Baranes et al., 2017). For example, wind speed and wind assistance both have been cited as important in the departure decisions of many species (Schaub et al., 2004; Mellone et al., 2012; Gill et al., 2014; Panuccio et al., 2016; O’Neal et al., 2018). Higher wind speeds, increased tailwinds, and reduced crosswinds may all speed up migration and reduce energy use (Pennycuick et al., 1979; Panuccio et al., 2016; O’Neal et al., 2018; Roques et al., 2021; Rüppel et al., 2023). Temperature changes are often associated with arrival and departure during migration, where migration usually occurs in spring with rising temperatures and in autumn with falling temperatures (Richardson, 1990; Bauer et al., 2008). Both temperature and wind can interact or be correlated with barometric air pressure, which has been shown to influence departure decisions and other migratory behaviors (Richardson, 1978; Shamoun-Baranes et al., 2006; Cooper et al., 2023). Growing evidence indicates that birds can detect changes in barometric air pressure (Kreithen and Keeton, 1974; von Bartheld, 1994) and may change their flight or foraging behavior in response (Breuner et al., 2013; Metcalfe et al., 2013).
Topography and habitat may also influence the effects of environmental covariates on migratory behavior and impact migratory phenology. For example, orographic uplift (i.e., the movement of air over terrain) may be more important in migratory performance for birds flying over mountain ranges, whereas thermal uplift may have a greater impact on birds flying over flatter terrain (Mandel et al., 2011). Birds may also decide to go around ecological barriers, such as mountains or the open ocean, rather than over them, even if it increases overall migration duration (Åkesson and Hedenström, 2007). The suitability and availability of habitat can affect the stopover duration of migrating birds, where birds might remain at a stopover area longer if adequate resources are available (Obernuefemann et al., 2013; Jorgensen and Brown, 2017; Lawrence et al., 2021). The availability of resources can also interact with climatic shifts to alter migration phenology. In the Intermountain West, for example, increasing temperatures and changing precipitation and water regimes influence water availability, which can affect the availability of foraging and roosting sites for migrating waterbirds (Donnelly et al., 2019). Other studies have confirmed that some individuals and populations are departing wintering and stopover areas sooner or shifting their distribution in response to changing climate and habitat conditions (Jenni and Kéry, 2003; Visser et al., 2009; Usui et al., 2017; Cox et al., 2023). Understanding migratory behaviors and how individuals respond to weather, climate, and habitat is an important initial step to anticipating how climate and land use changes may adversely affect population numbers.
The importance of weather patterns and habitat may differ between northbound and southbound migration (Nilsson et al., 2013; Kemp et al., 2023) and be based on other intrinsic factors of the bird, such as individual body condition and age or experience (Piersma and Jukema, 1990; Mueller et al., 2013; Mitchell et al., 2015). Considerable attention has been given to whether birds migrate based on time-minimization or energy-minimization as the primary goal (Alerstam and Lindström, 1990; Alerstam and Hedenström, 1998; Duijns et al., 2019). Minimizing time is expected to be the preferred strategy during spring migration, as arriving to the breeding grounds at an optimal time may allow individuals to take advantage of high-quality resources and secure territory before the arrival of competitors (Kokko, 1999; Nilsson et al., 2013; Illan et al., 2017). In contrast, autumn migrants may be less time-constrained because they are not competing for breeding areas and may prioritize acquiring and saving energy (Nilsson et al., 2013). Several studies have confirmed that migrants travel faster in spring than in autumn (Karlsson et al., 2012; Mellone et al., 2012; Illan et al., 2017; Duijns et al., 2019), while others have found the opposite to be true (Shamoun-Baranes et al., 2003; Nolet, 2006; Kölzsch et al., 2016). Migrants generally travel faster by reducing stopover time, which is the main determinant of total migration duration, but they may also increase their flight speed (Karlsson et al., 2012; Nilsson et al., 2013; Duijns et al., 2019).
Cranes in the subfamily Gruinae are composed of species known to be impacted by various weather conditions during migration, especially due to their relatively large size and reliance on a mix of flapping-powered and soaring flight (Pennycuick et al., 1979; Alonso et al., 1990a; Ojaste et al., 2020). There are six distinct migratory populations of sandhill cranes in North America, and they are composed of up to two subspecies (Collins et al., 2016; Petersen et al., 2003; Johnson et al., 2005). The greater sandhill crane (Antigone canadensis tabida) is the largest subspecies of sandhill cranes and makes up the Rocky Mountain Population (RMP). The San Luis Valley (SLV) in Colorado has been identified as a key stopover site during spring and autumn migration for the RMP (Donnelly et al., 2021). During stopover, sandhill cranes rely on foraging areas (e.g., harvested grain fields) and roosting areas (e.g., wetland and riverine habitat) in the SLV for up to 30 days and sometimes more as they move to breeding areas throughout several western states (i.e., Colorado, Idaho, Montana, Wyoming, and Utah) and their wintering areas in New Mexico, Arizona, and northern Mexico (Drewien and Bizeau, 1974; Donnelly et al., 2021). Water availability in the SLV is highly variable within and between seasons, which may impact stopover patterns, as the juxtaposition of nearby surface water with foraging areas may dictate distribution (Krapu et al., 1984; Boggie et al., 2018). A thorough examination of the stopover ecology and the role of weather and surface water in stopover decisions will be informative for both monitoring and management (e.g., survey timing, habitat manipulation), particularly as land use changes and increasing water scarcity have the potential to affect RMP cranes in the Intermountain West (Gerber et al., 2015; Donnelly et al., 2021, 2023).
Our specific objectives were to (1) determine stopover duration, (2) investigate arrival and persistence probability (i.e., the probability of staying in the SLV during stopover), and (3) examine the influence of temperature, wind components (i.e., wind speed, tailwind, and crosswind) at the surface and higher altitudes, barometric air pressure, and surface water availability on the persistence probability of RMP sandhill cranes in autumn and spring in the SLV. Day of season or the amount of time an individual has been at a stopover (i.e., time-since-arrival) may be more influential than weather or other factors on persistence for an individual prioritizing the minimization of time over energy use (Alerstam and Lindström, 1990; Roques et al., 2022). Because the time spent at stopovers largely determines total migration duration, we expected RMP sandhill cranes to have a shorter stopover duration in spring than in autumn. There may also be less variability among years in the timing and duration of spring migration. For example, other studies have confirmed sandhill cranes migrate longer during autumn compared to spring and found evidence of greater variability in stopover duration and total migration duration in autumn (Krapu et al., 2014; Fronczak et al., 2017; Donnelly et al., 2021). Thus, we predicted that there would be less variability in arrival and persistence probabilities in spring compared to autumn.
Several weather metrics have been shown to be correlated with the stopover decisions of other crane species and similarly sized migrants (Pennycuick et al., 1979; Shamoun-Baranes et al., 2006; Vansteelant et al., 2017; Ojaste et al., 2020). Specifically, the metrics wind speed, tailwinds, crosswinds, temperature, and barometric air pressure play a role in shaping conditions for migration and the amount of energy required to depart and fly (Richardson, 1990; Gordo, 2007). We predicted higher wind speeds, the presence of a tailwind, and reduced crosswinds would negatively influence persistence probability for RMP sandhill cranes in spring and autumn. We also predicted that wind covariates at higher altitudes would be more influential in spring than autumn because sandhill cranes must fly over or bypass mountains during northward migration. We hypothesized that barometric air pressure would have a negative influence on persistence probability in both seasons, as higher air pressure generally indicates light winds and no precipitation (Ahrens, 2013), which are favorable conditions for migration (Richardson, 1990). We also expected daily temperature change to negatively influence persistence probability in spring, as an increase in temperature might indicate more optimal migratory conditions (Richardson, 1990; Shamoun-Baranes et al., 2003; Panuccio et al., 2016). At the same time, temperature can influence habitat availability (Richardson, 1990; Ojaste et al., 2020). In the SLV, lower temperatures in early spring or late autumn may result in freezing conditions, which can incur thermoregulatory costs for sandhill cranes and limit roosting potential (Richardson, 1990). Water is becoming a limited resource in much of the Intermountain West (Llewellyn and Vaddey, 2013; Dettinger et al., 2015), and so it may affect the ability of sandhill cranes to roost and forage while maintaining adequate energy reserves. As a result, we predicted that temperature and surface water availability would both positively influence persistence probability during autumn.
2 Materials and methods
2.1 Study area
The SLV is in south-central Colorado, USA and is a high elevation, intermontane basin that is the northernmost part of the Upper Rio Grande Basin (Figure 1). The average elevation is approximately 2,100 m, and the total area of the valley floor is about 8,200 km2 (Emery et al., 1969). Climate in the SLV is largely driven by a combination of its location and elevation and the sea surface temperatures in the Pacific Ocean and the Gulf of Mexico, which in turn influence the movement of air masses throughout the year and the North American Monsoon in the summer (Ahrens, 2013; Llewellyn and Vaddey, 2013). The SLV is an arid region with an average annual precipitation of 180 mm but with evapotranspiration rates of 1150 to 1300 mm per year (U.S. Fish and Wildlife Service, 2015). The surrounding mountain ranges receive more precipitation (850 to 1100 mm on average) mostly in the form of snow. As a result, the major source of water input in the SLV is spring snowmelt from the Sangre de Cristo Mountains to the east and the San Juan Mountains to the west (Elias et al., 2015). Snowmelt is transported to the valley floor via the Rio Grande River and other creeks and tributaries (Emery et al., 1969). Monsoonal rains in the summer can provide water inputs, but surface water tends to become more limited throughout the summer and autumn due to evapotranspiration and irrigation withdrawals for agriculture (Rio Grande Basin Roundtable, 2015; U.S. Fish and Wildlife Service, 2015). Two aquifers also provide water for irrigation and natural inflows, which influence hydrological conditions. The annual average temperature in the SLV is approximately 6°C, but temperatures range from as low as -20°C in winter to over 20°C in the summer (Western Regional Climate Center, 2013). Recent research has shown increasing temperatures in the Upper Rio Grande Basin (Llewellyn and Vaddey, 2013) and the SLV in recent decades (Mix et al., 2010). Winds are highly variable and are primarily from the south and southwest throughout the year.
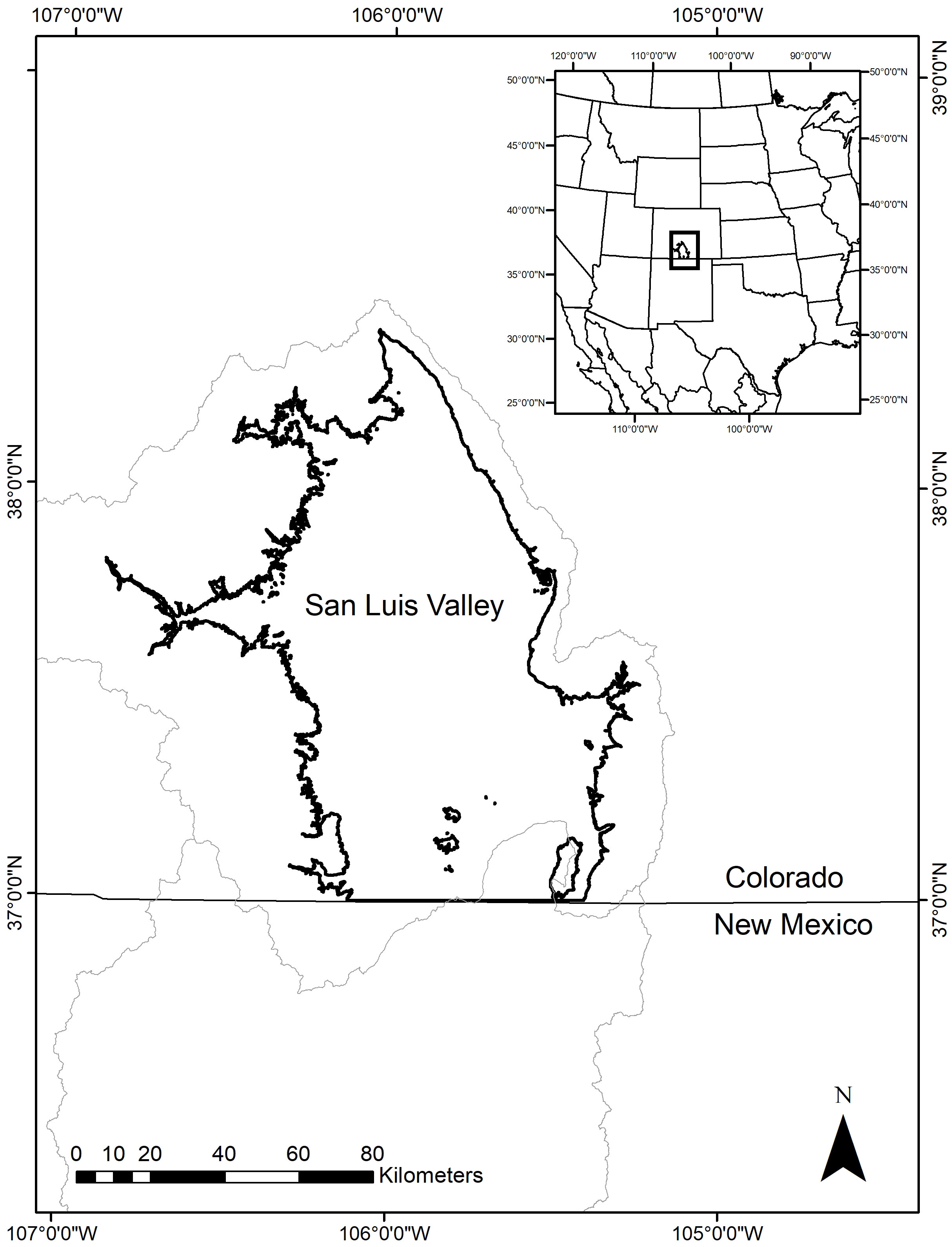
Figure 1 A map showing the location of the San Luis Valley, Colorado. The light gray lines indicate the boundaries of the Upper and Middle Rio Grande Basin.
Much of the SLV is composed of shrub-scrub habitat, privately-owned agricultural land, and interspersions of pastures and publicly managed wetland and wet meadow habitat. Willows (Salix sp.) and cottonwood (Populus deltoides) grow along the Rio Grande and its tributaries. Many of the remaining wetland habitats, composed of cattail (Typha sp.), rushes, and other emergent vegetation, exist on public lands, including the Monte Vista National Wildlife Refuge, Alamosa National Wildlife Refuge, and Russell Lakes State Wildlife Area. Agricultural production is the dominant economic driver in the SLV and the greatest user of water (Rio Grande Basin Roundtable, 2022). Agricultural fields are mostly irrigated by pivot sprinkler systems with water transported from ditches, canals, and underground wells. The primary crops grown include alfalfa, potatoes, grass hay, and barley (Rio Grande Basin Roundtable, 2022). Livestock ranching is also a major industry in the area. Irrigation water for crops come from both surface water and groundwater but diminishing surface water resources due to over-appropriation and increasing temperatures have resulted in a greater reliance on groundwater in recent decades (Rio Grande Basin Roundtable, 2022).
During the spring and the fall, RMP cranes will roost along the rivers and creeks and in emergent wetlands and wet meadows on both public and private lands. They forage primarily on waste grain (e.g., barley) remaining after autumn harvest or provided by the Monte Vista National Wildlife Refuge, but they will also forage in potato fields, grasslands, wetlands, and pastures for other seeds, vegetation, and invertebrates. Greater sandhill cranes generally forage within 2-5 km of their roosting areas (Ivey et al., 2015; Boggie et al., 2018) and are central-place foragers that gradually move farther from the roost as foraging resources are depleted over time (Ashmole, 1963; Pearse et al., 2010; Ivey et al., 2015).
2.2 Transmitter deployment and data processing
A total of 106 greater sandhill cranes were captured and fitted with global system for mobile communication (GSM) platform transmitter terminal (PTT) tags (n = 72; Evolution Series-400, 15-g; Cellular Tracking Technologies, Rio Grande, New Jersey, USA) or global positioning system (GPS) PTT tags (n = 34; PTT-100, 22-g Solar Argos/GPS PTT, Microwave Telemetry, Columbia, Maryland, USA). Most RMP cranes (n = 86) were captured in wintering areas in New Mexico and Arizona, but a few individuals were captured in Colorado (n = 3), Idaho (n = 14), and Montana (n = 3) during the summer. Details on the capture methods can be found in Collins et al. (2016), Boggie et al. (2018), and Hays et al. (2021).
Data were formatted to an individual’s presence or absence in the SLV on each day of autumn or spring for a given year (2015-2022). The GSM/GPS units were programmed to acquire positions every 15 minutes, while the GPS units acquired positions at specified time intervals throughout the day. Because we were only interested in presence or absence in the SLV, the different fix rates and the exact location of fixes in the SLV did not influence formatting. We did not use individuals for which we had data for less than three days in the SLV. Based on the GSM/GPS data, we identified the spring season to be between 18 January and 9 May and the autumn season to be between 20 August and 13 December.
All appropriate banding permits were acquired from the U.S. Geological Survey (USGS) Bird Banding Laboratory, the U.S. Fish and Wildlife Service, and New Mexico Department of Game and Fish permits to band and attach transmitters to sandhill cranes.
2.3 Covariate development
2.3.1 Weather data
Local weather covariates were acquired from up to five weather stations in the SLV through the National Oceanic and Atmospheric Administration (NOAA, 2022). We averaged daily maximum temperature (C°) from all five weather stations and calculated a daily change in temperature, where zero indicated no change in temperature, a positive value indicated an increase in temperature, and a negative value indicated a decrease in temperature. All other temperature covariates (e.g., daily maximum temperature) were highly correlated (r > 0.70) with time of season, so we did not consider other temperature covariates. Local wind covariates were available for one weather station in the SLV. In addition to using wind speed (m/s), we calculated a tailwind variable (b) according to Åkesson and Hedenström (2000) and Arizaga et al. (2011),
where V is the wind speed, is the departure direction, and is the wind direction (i.e., the direction from which the wind comes). The departure direction differed for each season and was based on the mean departure direction of RMP cranes in autumn and spring during the study period. We determined departure direction by examining the bearing between the location of the sandhill crane on the last day it was observed in the SLV and the following location outside the SLV. Mean departure direction was less variable in autumn (=190°, standard deviation=10.97°) than in spring (=285°, standard deviation=91.59°), but both seasons showed a normal distribution in the frequency of departure directions among individuals. Positive values of b corresponded to a tailwind, while negative values corresponded to a headwind (Arizaga et al., 2011). We also considered the influence of crosswinds c, which we calculated as
where a positive value indicates an easterly crosswind and a negative value indicates a more westerly crosswind. Values at or close to zero indicate no or minimal crosswind. We also considered the absolute value of c, which represents the effect of crosswind without consideration to direction.
Other covariates were obtained from the National Centers for Environmental Prediction (NCEP) and Department of Energy (DOE) Reanalysis II dataset (Kanamitsu et al., 2002) using the RNCEP package in R (Kemp et al., 2012). The data are publicly available and cover a spatial resolution of 2.5 x 2.5° (i.e., approximately 220 km x 250 km) and temporal resolution of 6-h intervals. We downloaded u (east-west) and v (north-south) wind components and wind direction at the pressure levels of 600 and 700 hPa, which correspond to approximately 4,500 and 3,000 m above sea level, respectively. These are altitudes where cranes have been documented during migratory flights (Gerber et al., 2020). We calculated wind speed as and wind direction (in radians) as . We then calculated the same tailwind and crosswind variables as with the local weather variables. Finally, we also considered barometric air pressure (hPa) measured at the surface of the study area and the daily change in barometric air pressure.
2.3.2 Water data
We used spectral mixture analysis to measure daily surface water availability in the SLV for each year and season. Spectral mixture analysis is a process that utilizes remotely sensed images and determines the proportion of habitat classes within pixels using endmembers (Adams and Gillespie, 2006; Jin et al., 2017). Endmembers are areas representative of the habitat class of interest and are static. We used Landsat 8 and Landsat 9 Operational Land Imager satellite imagery corrected for surface reflectance. The spatial resolution is 30 x 30 m, and images are acquired approximately every two weeks. Images were processed to remove clouds, shadow, and snow. Although we were only interested in surface water, spectral mixture analysis requires that all pixels be classified into proportional habitat classes. We chose the classes sandbar, soil, shrub, and vegetation along with water. Static images of sandbar, soil, and shrub were chosen based on examination of satellite imagery, focusing on areas where there was little change in these classes over the study period. Endmembers for water were acquired using the mean of the 98th percentile of the Normalized Difference Water Index for each two-week period in every year and season (Boggie et al., 2018). We used a similar method for vegetation, which was estimated using the Normalized Difference Vegetation Index. Spectral mixture analysis was completed in Google Earth Engine (Gorelick et al., 2017). To get daily values of surface water, we used cubic interpolation using the “ss” function (Bunting et al., 2022; Helwig, 2022) in Program R (R Core Team, 2022).
2.4 Statistical analysis
We examined stopover decisions using the within-season component of a multistate open robust design mark-recapture model (Kendall et al., 2019). For each season this model allows for estimation of arrival and persistence probabilities, in addition to detection probability. We modeled autumn and spring separately, so each season was composed of a different number of secondary occasions, and for each we used year as a grouping variable. Each season consisted of a set of J total secondary occasions (i.e., days), where individual i was either detected or not detected on day j. The start and end of each autumn and spring depended on the first and last encounter of all RMP cranes among all years. These dates were buffered by two additional occasions to improve estimation of arrival and persistence probabilities. The parameters included arrival probability detection probability , and persistence probability of individual i on day j.
We were also interested in three derived parameters. The average total stopover duration was calculated using Method 1 described in Kendall et al. (2019), applicable where the arrival of the first individual and the departure of the last individual is bounded by sampling effort. We determined the expected arrival and departure periods across all years. Finally, the intensity parameter is the probability that individual i is present in period j (Kendall et al., 2019).
We examined a different set of covariates for and . We assumed would likely be driven by conditions at areas prior to sandhill crane arrival in the SLV, so we only considered the effects of time (linear and quadratic). The list of covariates we considered for included a linear trend of time and time-since-arrival, total surface water availability, the daily change in the maximum temperature, wind speed (daily and a five-day rolling average), crosswind speed (daily and a five-day rolling average), and barometric air pressure (daily and the daily change). We considered wind speed at the surface and at barometric air pressures of 600 hPa and 700 hPa, corresponding to 4,500 and 3,000 m above sea level, respectively. We did not include covariates with a correlation coefficient > 0.40 in the same model, including covariates that were correlated with time, as we were interested in modeling the effect of time or time-since-arrival on . We also examined one-way interactions between time or time-since-arrival and a time-varying weather covariate to determine if weather covariates were more influential later in the season. We did not consider more than a one-way interaction to maintain simplicity and to avoid overfitting. Fixes from GSM/GPS units are sometimes unsuccessful and therefore detection is not perfect but is reliably high, so we constrained to vary only by year [i.e., ] to allow for the reduced fix rate of the older GSM/GPS units and the greater potential for missing a signal from cranes during the day. All weather covariates and surface water were scaled to have a mean of zero.
Model selection for was composed of four steps using a build-up strategy to identify the best-supported models (Morin et al., 2020). First, we determined the best time trend for both and by comparing all possible combinations of time and time-since-arrival. Time-since-arrival is an important covariate to consider because the decision to migrate on a given day may depend more on the accumulated time on site of an individual, rather than the day of the season (Roques et al., 2022). This is particularly pertinent for spring, when stopovers are used to build up energy reserves needed for reproduction and completing migration for some migrant birds (Hedenström and Alerstam, 1997). We also examined models with a time trend across years for to allow for a trend in environmental conditions not accounted for by our chosen predictor variables. We compared models using Akaike’s Information Criterion corrected for small sample sizes (AICc), and the time model with the lowest AICc score was used in the next step (Akaike, 1973; Burnham and Anderson, 2002). Second, we compared a set of models each with the best time covariate (on ) and a single time-varying covariate. Covariates in the models that improved the fit of the time-only model were used in the next step, but we removed covariates that were highly (> 0.40) correlated with one another or time. We used the covariate that produced a lower AICc value unless it was correlated with time. Third, covariates in the most competitive models from the previous step were used to examine the interaction of time with a time-varying weather covariate and additive effects of the remaining covariates. These models were compared to one another, and we included interactions from the most competitive models in the next step. Finally, we examined all combinations of covariates, including both main effects only and interactive models, and identified the most competitive models (<2 ΔAICc from the top model). To remove potentially uninformative covariates, we did not consider models competitive at this stage if they were nested versions of other competitive models (Arnold, 2010).
There is currently no way to assess goodness-of-fit of multistate open robust design models directly (Kendall et al., 2019), so we used the median approach described by Cooch and White (2017) and recommended by Kendall et al. (2019). Estimating can reveal the amount of overdispersion in a model due to more variance in the capture history than expected (Cooch and White, 2017). Conditioning on first detection within a season and using the Cormack-Jolly-Seber framework (CJS; Cormack, 1964; Seber, 1965), we examined models for autumn and spring separately with a time trend on and with p(year).
To examine the change in in response to covariates, we used the most competitive models to predict across the range of observed values for the covariate being examined while keeping the other values at their means. If we found the interaction of time or time-since-arrival with a weather covariate to be influential, we examined the effect of the covariate during three periods of the season: early (day 15), middle (half the season length), and late (day 100). We estimated variances using the delta method. All model building, comparison, goodness-of-fit assessment, and predictions were completed directly in Program MARK (White and Burnham, 1999) or through RMark (Laake, 2013; R Core Team, 2022).
3 Results
We monitored a total of 106 unique individual sandhill cranes across all years (2015-2022) in spring and 55 unique individuals across all years in autumn (Table 1). Some individuals (n =36) were only monitored during the spring following their initial capture due to transmitter failure, loss, or crane death. A total of 10 individuals never used the SLV in autumn throughout their monitoring periods, and five individuals were removed from the autumn season due to too few (i.e.,<3) daily fixes in the SLV. Finally, of the 106 individuals, one in autumn and eight in spring were last detected in the SLV, which might indicate the GSM/GPS units stopped transmitting while the individuals were in the study area. We reran the most competitive model for each season and censored the days after the first day of no detection for these individuals to determine if results were biased. We found no substantial differences in coefficient estimates, so we kept all data for these individuals uncensored.
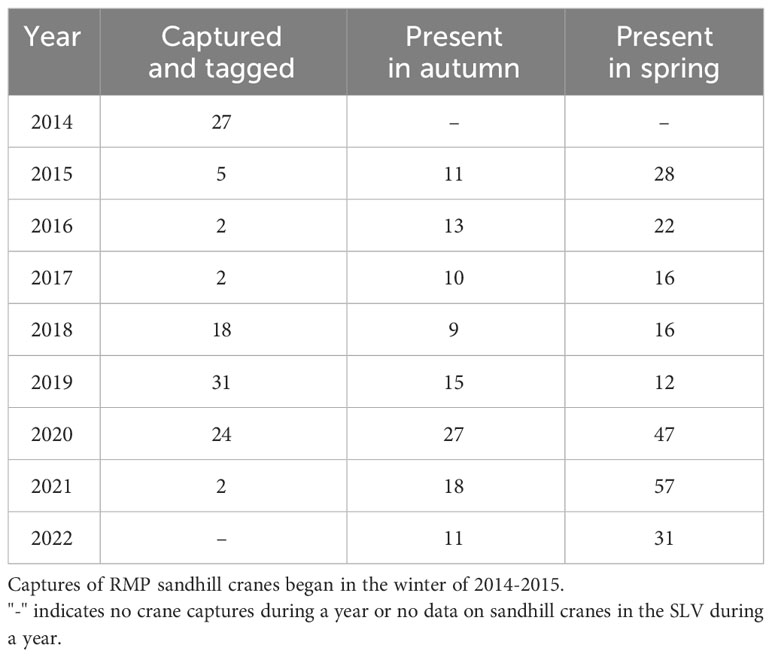
Table 1 The number of sandhill cranes that were captured and tagged during the winter season and the number migrating through the San Luis Valley, Colorado the following spring and autumn.
Weather patterns in the SLV during this time showed declining temperature in autumn and increasing temperature in spring (Table 2; Supplementary Figure 1). Surface winds primarily came from the west and southwest in both seasons (Table 2; Supplementary Figure 2). Wind speeds at higher altitudes were more northerly on average (Table 2; Supplementary Figure 2). Tailwinds were variable for both seasons but were generally more favorable in autumn than in spring (Table 2; Supplementary Figure 3). Barometric air pressure was variable in both seasons and showed a slight negative trend in autumn (Table 2; Supplementary Figure 4). Surface water declined throughout autumn and increased throughout spring on average (Table 2; Supplementary Figure 5).
For the autumn CJS model, we estimated a of 1.41 (95% CI -0.45, 3.27), so we used the quasi-likelihood adjusted AICc (QAICc) to compare autumn models (Burnham and Anderson, 2002). A value of 1 > < 3 is likely due to overdispersion, rather than a lack of model fit (Lebreton et al., 1992). We did not detect overdispersion in the spring models and used AICc for model comparison. Models for in both seasons included a quadratic time trend. Based on the most competitive models, the probability of arriving in the SLV throughout the season peaked on 13 October in autumn and 21 February in spring (Figure 2). The value of remained close to one for much of autumn and showed its greatest decrease around 12 December (Figure 3). The value of was also high at the beginning of the spring season but declined in mid-March (Figure 3). The average expected departure date varied across years in both seasons (Table 3). Autumn stopover duration was generally greater and more variable among years than spring stopover duration (Figure 4). The derived values of indicated that the probability of presence peaked around 6 November in autumn and 6 March in spring (Figure 5).
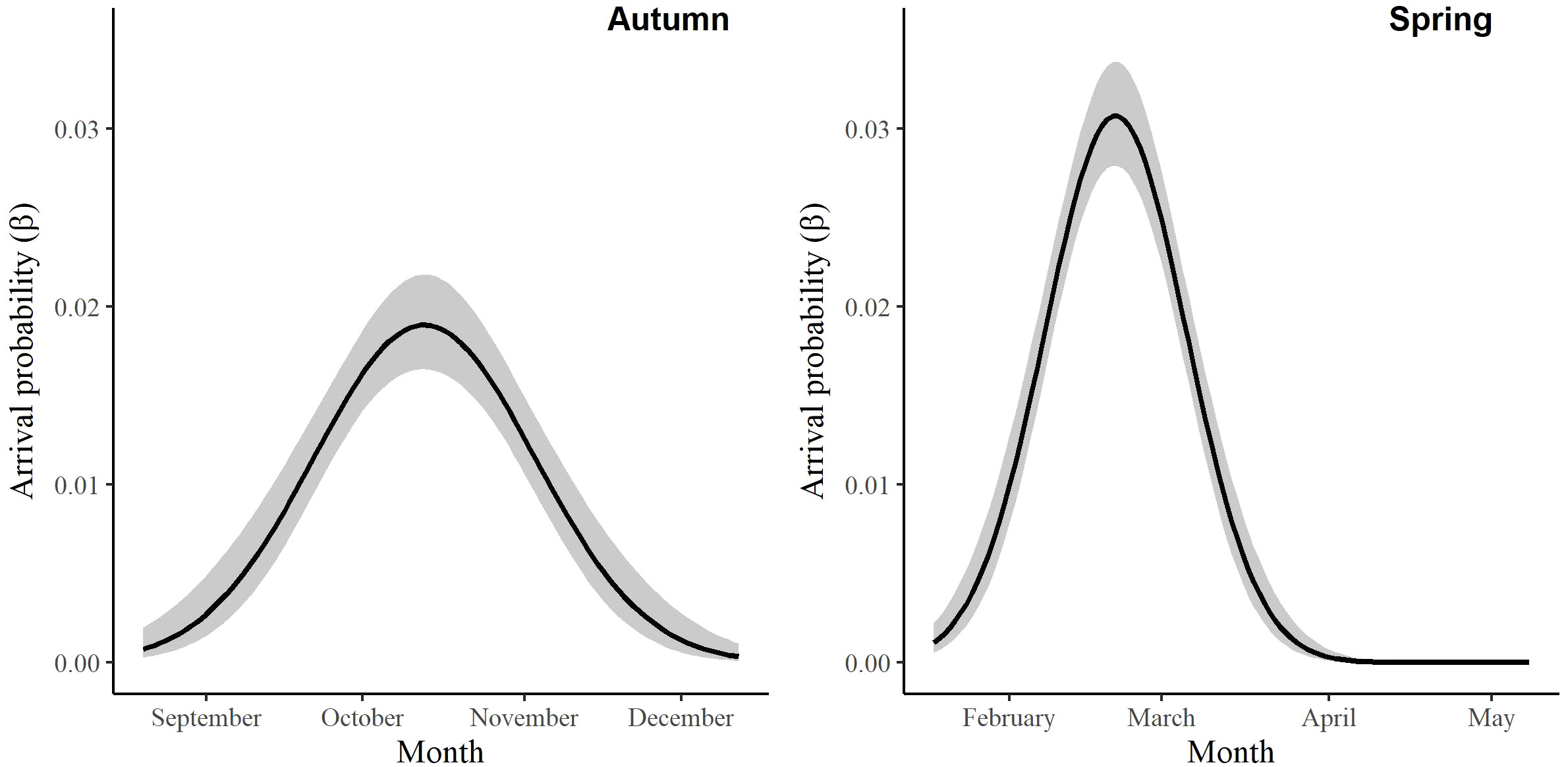
Figure 2 The change in the arrival probability (β) of migrating Rocky Mountain Population greater sandhill cranes in the San Luis Valley, Colorado, 2015-2022 in autumn and spring.
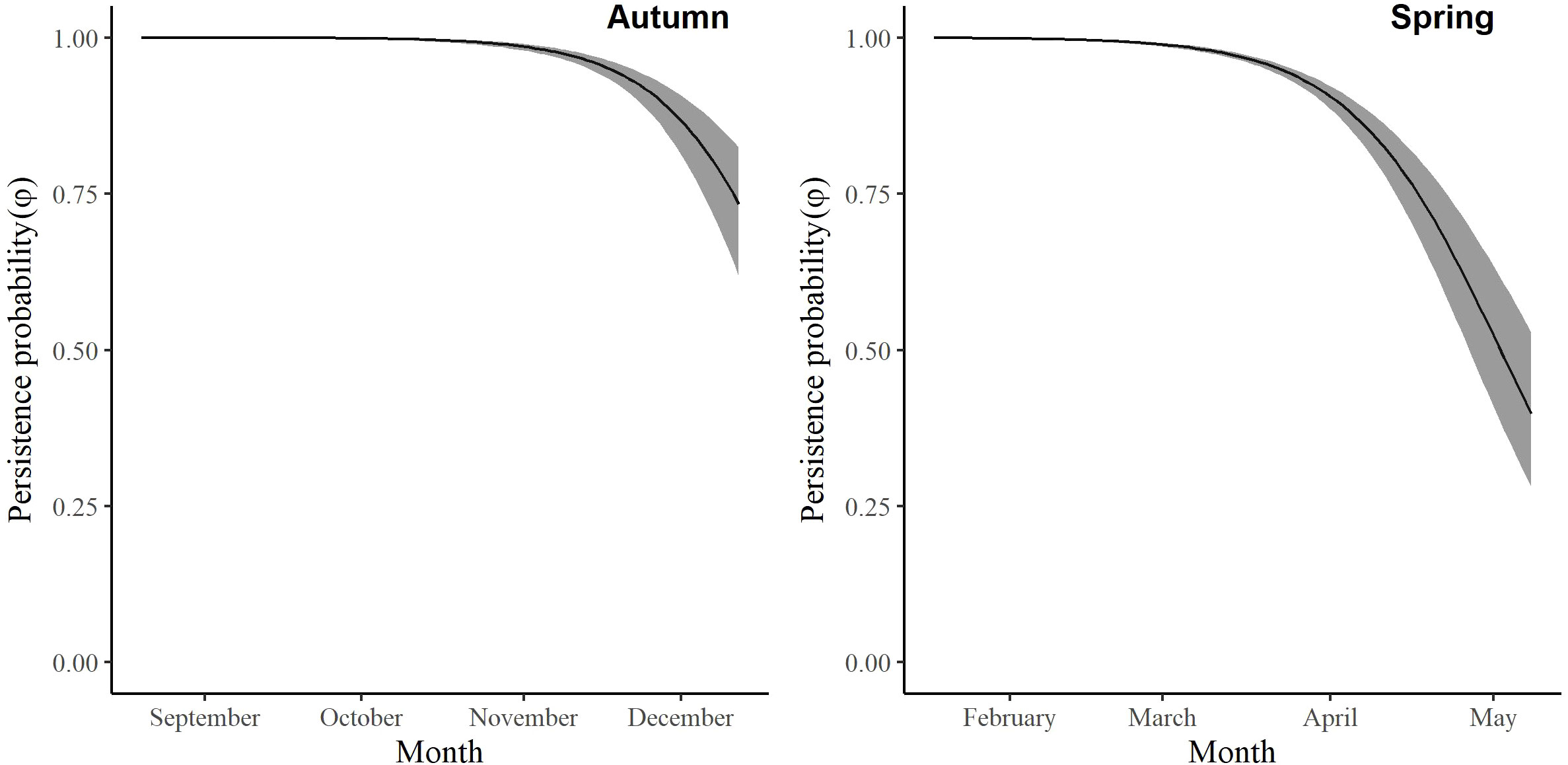
Figure 3 The change in the persistence probability (φ) of migrating Rocky Mountain Population greater sandhill cranes in the San Luis Valley, Colorado, 2015-2022 in autumn and spring.
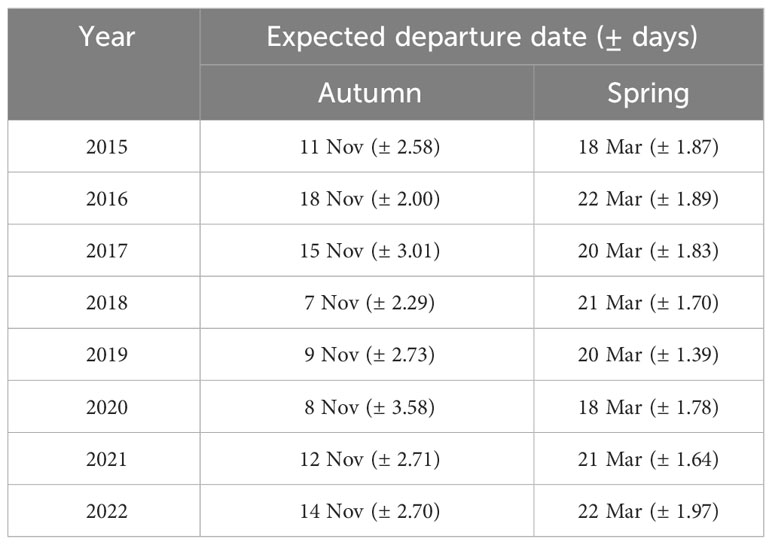
Table 3 Mean expected departure date (± days) derived from the multistate open robust design model for Rocky Mountain Population greater sandhill cranes in the San Luis Valley, Colorado.
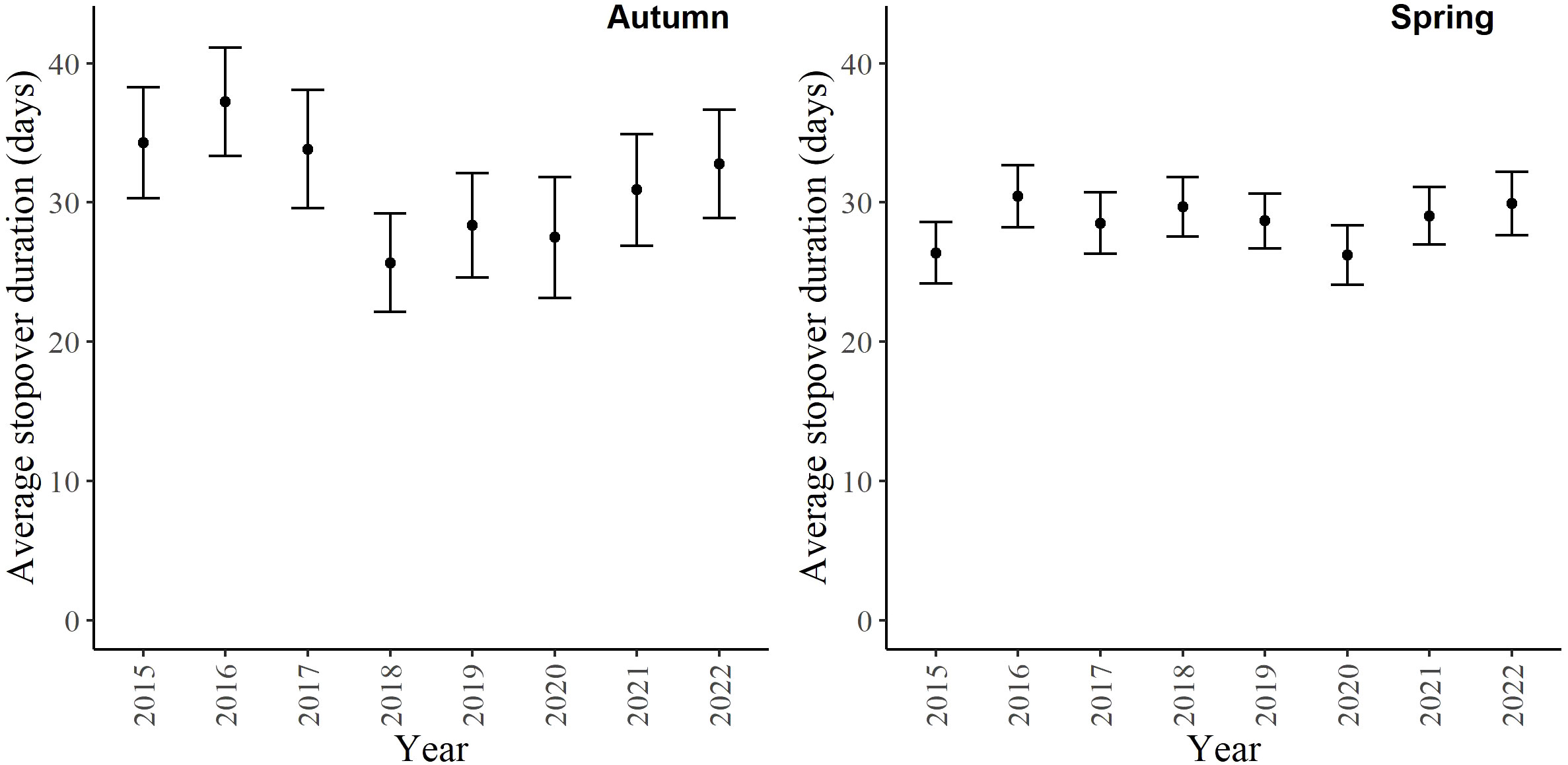
Figure 4 The stopover duration of migrating Rocky Mountain Population greater sandhill cranes in the San Luis Valley, Colorado, 2015-2022 in autumn and spring. The black bars are 95% confidence intervals.
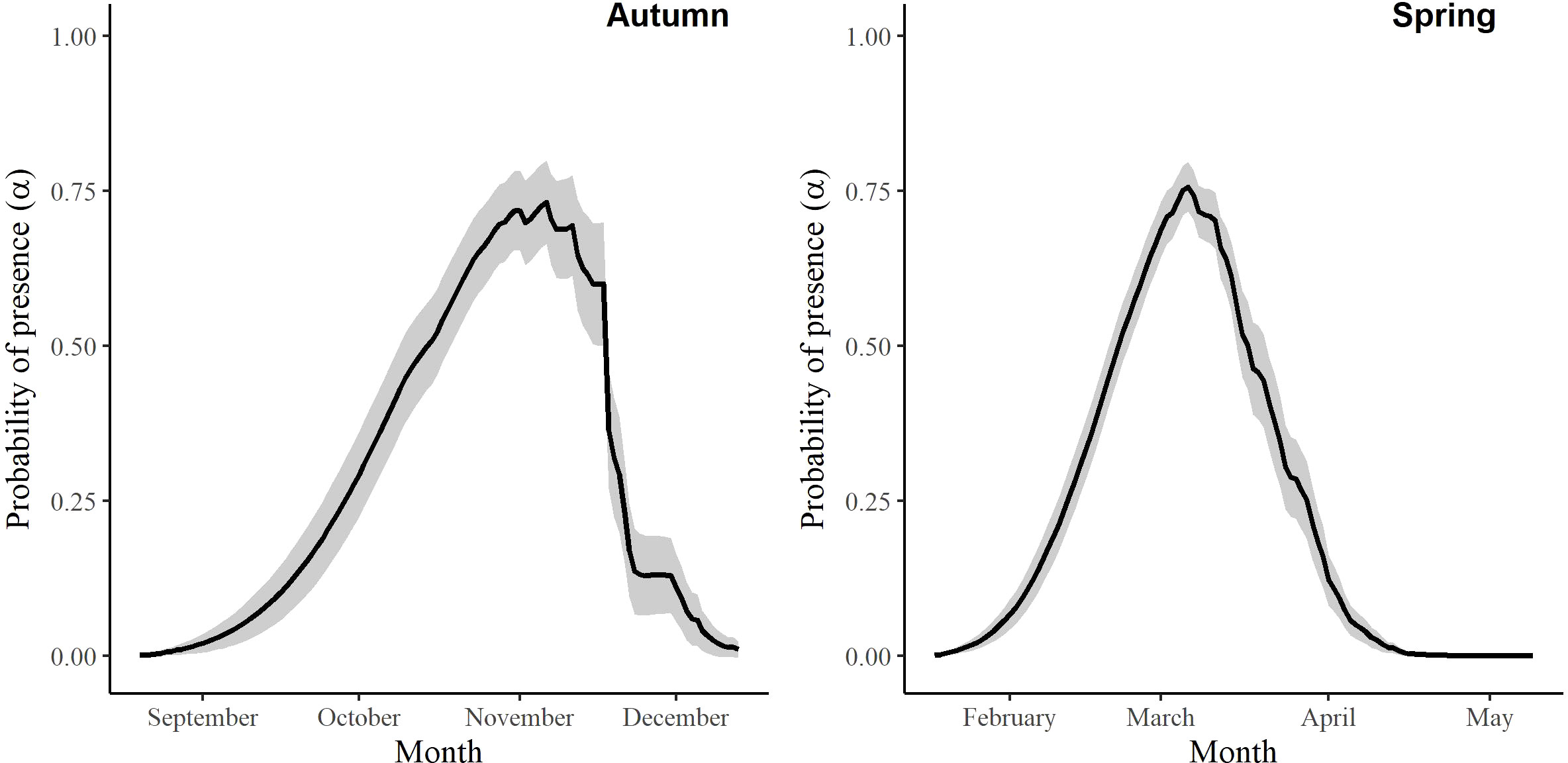
Figure 5 The probability of presence (α) of Rocky Mountain Population greater sandhill cranes in the San Luis Valley, Colorado, 2015-2022 in autumn and spring. The shaded area represents 95% confidence intervals.
The model with a time trend over years did not improve model fit for either the time-only models or the top model with covariates. There were small apparent trends in each season, negative for autumn and positive for spring (Supplementary Table 1), but the 95% confidence intervals overlapped zero for both seasons.
The most competitive model for in autumn included tailwind, crosswinds at 700 hPa, and surface water (Table 4). Several models with an interaction between time and temperature change or tailwind were also competitive. Surface water was included in all competitive models. The effect of tailwind indicated that RMP sandhill cranes were more likely to depart the SLV in autumn with greater tailwinds (Figures 6, 7). The effect of crosswinds at 700 hPa showed that as winds became more easterly and perpendicular to the migratory direction, RMP sandhill cranes were more likely to remain in the SLV. The opposite was true as surface water increased, with RMP sandhill cranes less likely to stay as surface water increased.
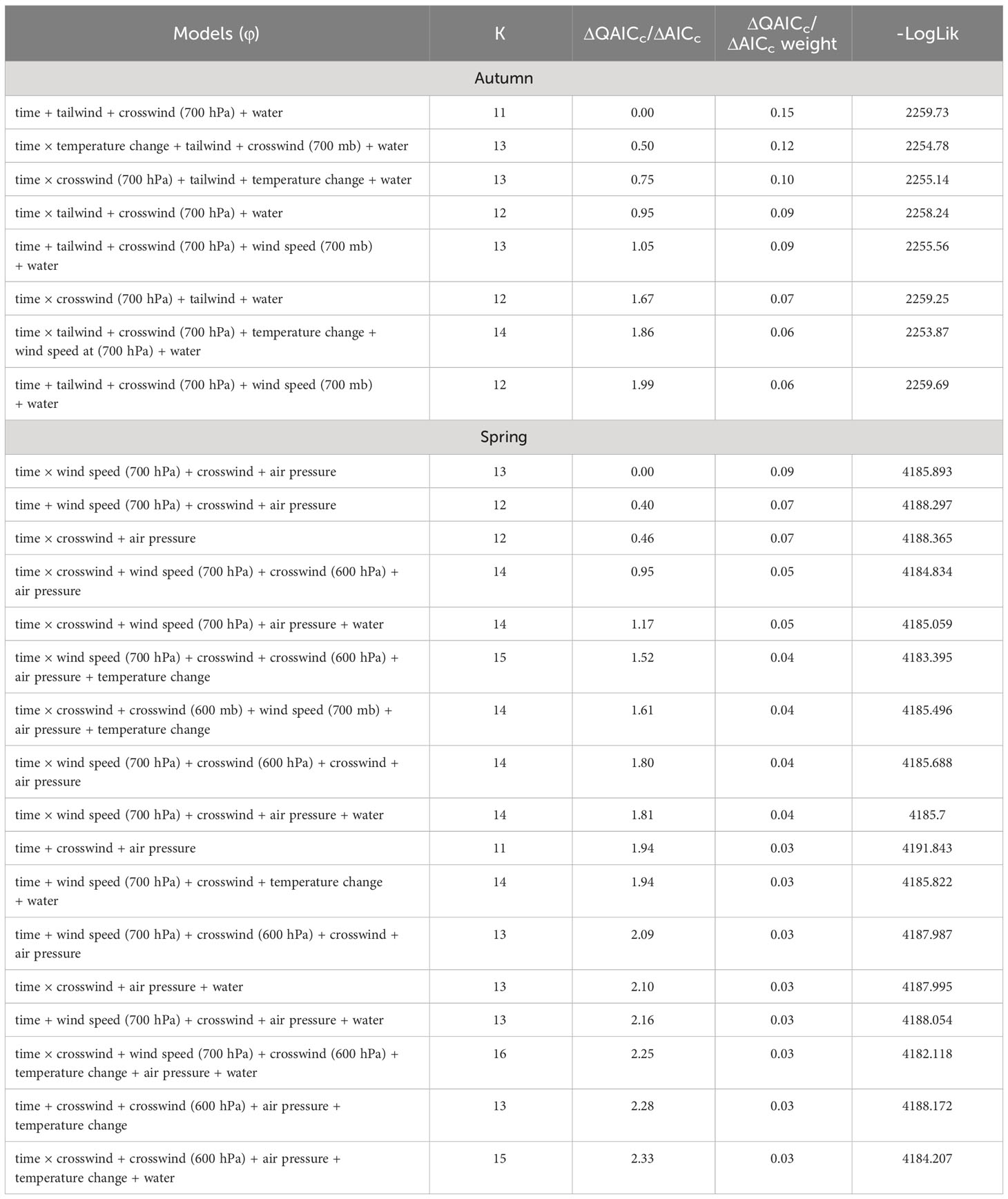
Table 4 Model selection results with the number of parameters (K), ΔQAICc (autumn models) or ΔAICc (spring models), model weights, and the negative log likelihood for the effects of weather covariates and surface water on persistence probability (φ) of sandhill cranes in the San Luis Valley, Colorado, 2015-2022.
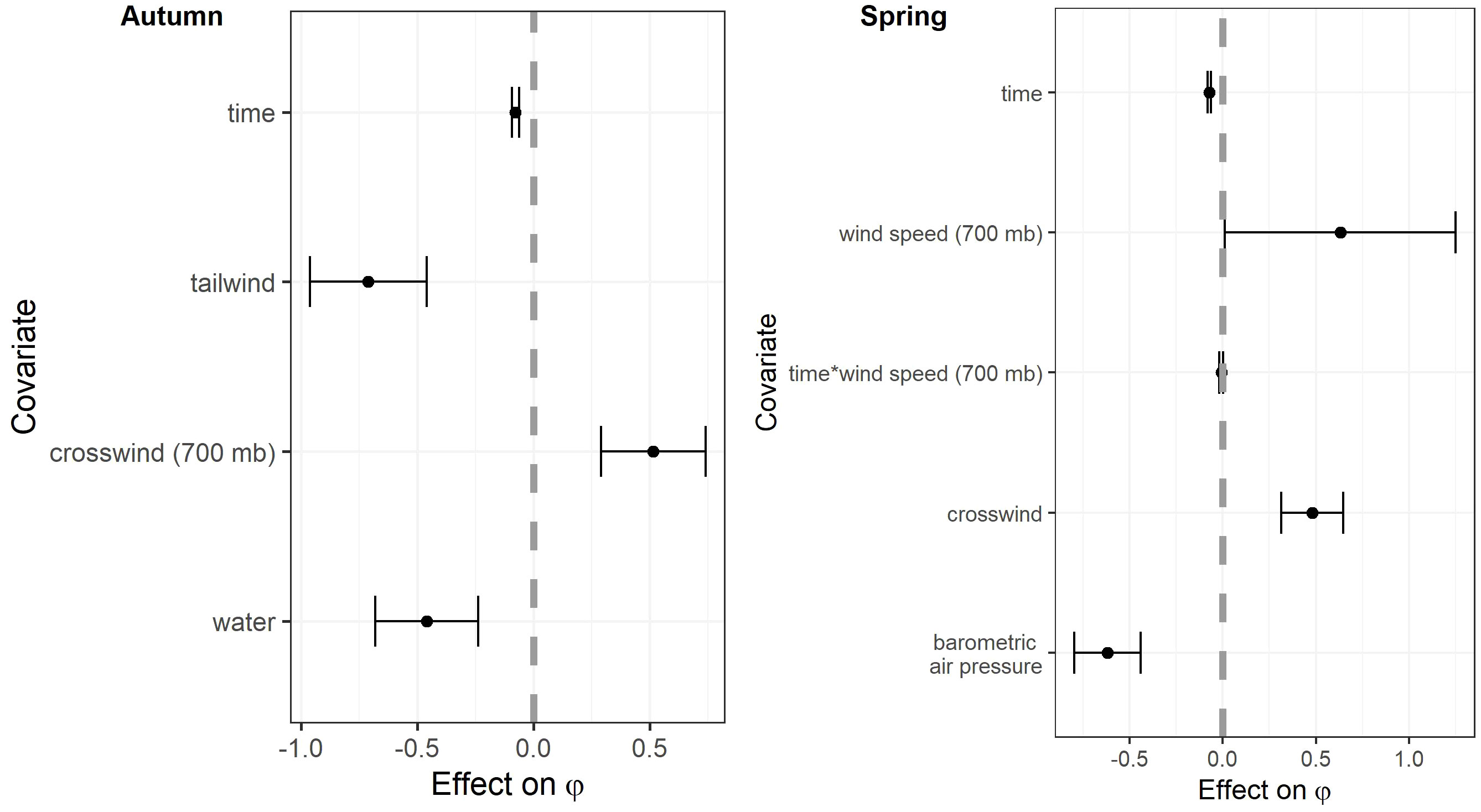
Figure 6 Coefficient estimates in the top models examining the effects of weather and habitat covariates on the probability of persistence (φ) of migrating Rocky Mountain Population greater sandhill cranes in the San Luis Valley, Colorado, 2015-2022 in autumn and spring. Covariates were standardized to have a mean of zero. The black bars are 95% confidence intervals.
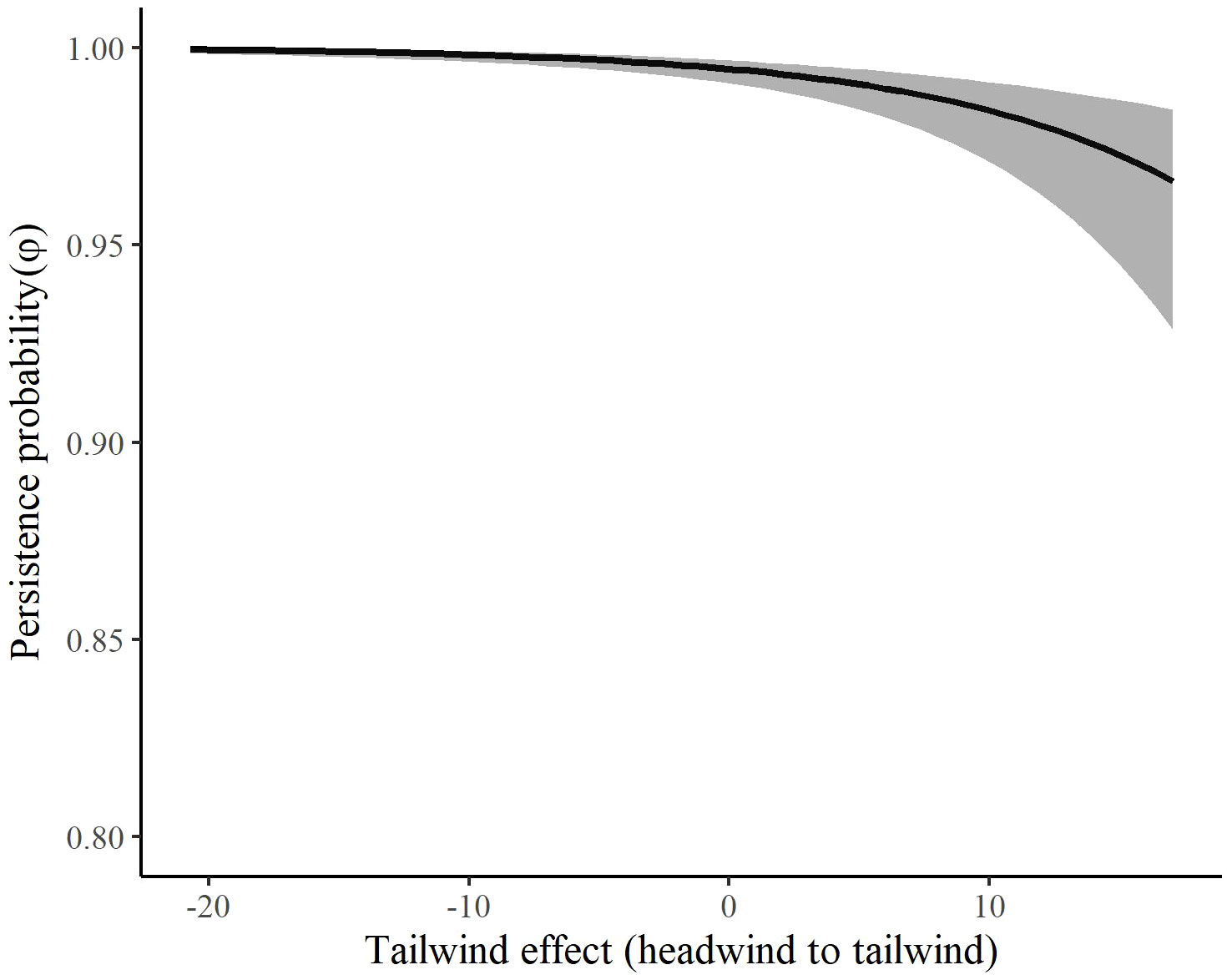
Figure 7 The effect of tailwinds on the persistence probability (φ) of migrating Rocky Mountain Population greater sandhill cranes in the San Luis Valley, Colorado, 2015-2022 in autumn. The shaded area represents 95% confidence intervals.
The most competitive model for in spring included barometric air pressure, crosswinds, and an interaction between time and wind speed at 700 hPa, but several other models were competitive (Table 4). Models with an interaction between time and crosswinds at 700 hPa were among the competitive models, as were models with main effects of temperature change and surface water. The most competitive model indicated that as the spring season progressed, the effect of wind speed at 700 hPa on became stronger and RMP sandhill cranes were less likely to remain in the SLV with strong wind speeds at 700 hPa (Figure 8). As local winds became more easterly and perpendicular to the preferred migratory direction (i.e., crosswinds), RMP sandhill cranes were more likely to remain in the SLV, while they were less likely to remain when barometric air pressure increased (Figure 9).
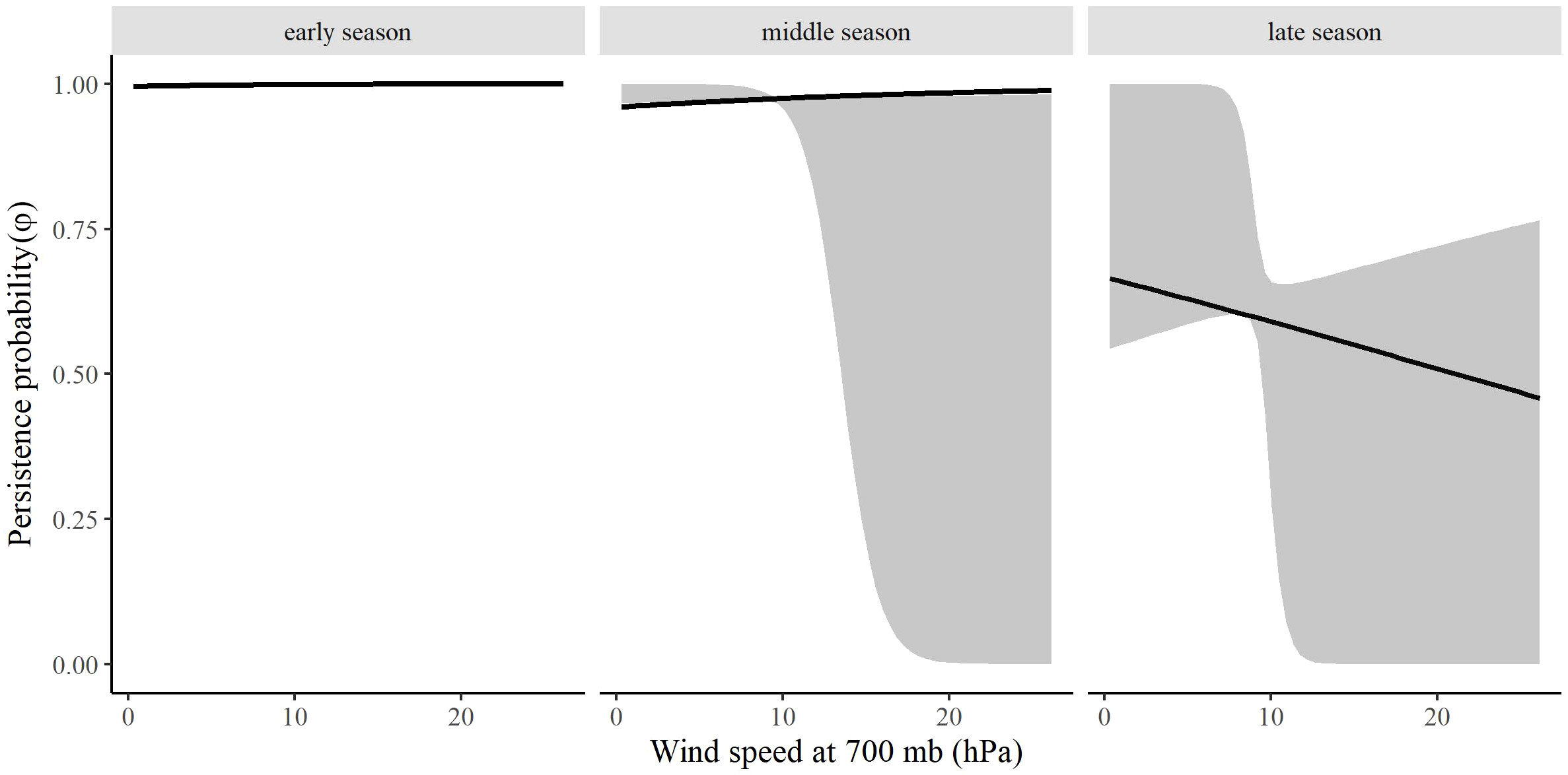
Figure 8 The effect of surface wind speed on the persistence probability (φ) of migrating Rocky Mountain Population greater sandhill cranes in the San Luis Valley, Colorado, 2015-2022 during early (1 February), middle (13 March), and late (27 April) spring. The shaded area represents 95% confidence intervals.
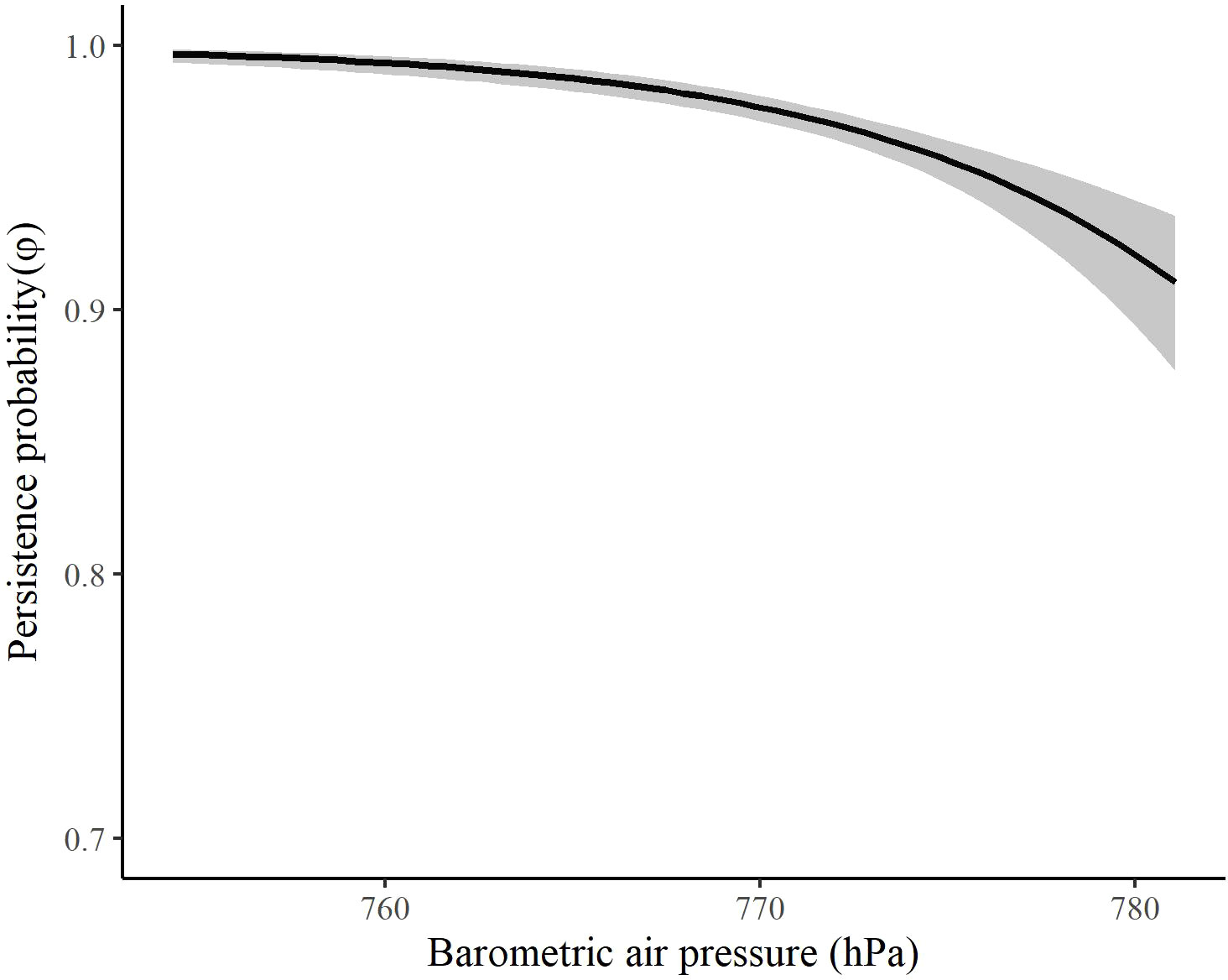
Figure 9 The effect of barometric air pressure on the persistence probability (φ) of migrating Rocky Mountain Population greater sandhill cranes in the San Luis Valley, Colorado, 2015-2022 in spring. The shaded area represents 95% confidence intervals.
4 Discussion
Patterns of sandhill crane phenology in the SLV during the spring and autumn of 2015-2022 confirmed our hypotheses that there was greater variability in the stopover patterns of RMP sandhill cranes in autumn than in spring and that autumn stopover duration was longer than spring stopover duration. Several of our hypotheses concerning the influence of weather covariates on persistence probability were confirmed, including the negative influence of tailwinds in autumn, the positive effect of barometric air pressure in spring, and the positive effects of wind speed and crosswinds in spring. In contrast to our expectation, surface water area had a negative influence on persistence probability in autumn and the daily change in temperature had no effect in either season. Our study provides information on migration phenology in the SLV and highlights the potential impacts of weather and habitat on the stopover ecology of RMP sandhill cranes.
Estimates of arrival and persistence probabilities provide support that RMP sandhill cranes in the SLV might prioritize minimizing time during spring migration when compared to autumn, which is consistent with findings from other studies of migrating birds in both spring and autumn (Mellone et al., 2012; Nilsson et al., 2013; Illan et al., 2017). The arrival day of RMP sandhill cranes in the SLV showed a quadratic pattern in both seasons, with greater within-year variability in autumn compared to spring. The RMP sandhill cranes also demonstrated a longer duration of high persistence probability and an overall greater stopover duration in autumn than in spring. Other migratory populations of cranes, including sandhill cranes (Krapu et al., 2014; Fronczak et al., 2017; Donnelly et al., 2021) and whooping cranes (Grus americana; Pearse et al., 2020), also show greater variability in arrival and departure dates and longer stopover durations in autumn compared to spring. The higher variation in autumn compared to spring is likely due to several individual-specific intrinsic factors that we could not measure, such as experience and reproductive status. For example, during southbound autumn migration, adult RMP sandhill cranes that have successfully bred may be accompanied by 1-2 young, and these family units may depart breeding or stopover areas later than nonbreeding adults (Carlisle and Tacha, 1983; Tacha et al., 1985; Pearse et al., 2020). The timing of migration in spring is likely more related to the benefits of optimal arrival to the breeding grounds. Consistency in arrival to the breeding grounds, which is mediated by stopover duration in spring, can allow birds to arrive at a time that provides the best resources for egg-laying and raising young (Kokko, 1999; Both et al., 2004). Donnelly et al. (2021) estimated that total migration duration for RMP sandhill cranes is approximately 35 days in spring and 60 days in autumn. Our results indicate that RMP sandhill cranes spend most of their migration period in the SLV in both seasons, so conditions within the SLV are likely the main determinants of total migration duration for RMP sandhill cranes.
Identifying the peak of arrival probability, the change in persistence probability, and the length of stopover duration may ultimately be useful for focusing research, monitoring, and management efforts in both seasons. Annual productivity surveys in autumn provide information for hunting regulations of RMP sandhill cranes (Seamans, 2022), and productivity surveys are conducted when it is thought that the greatest proportion of RMP sandhill cranes are in the SLV (Collins and Vanausdall, 2023). Current monitoring efforts of the RMP align well with our findings, which suggested that the optimal timing for annual surveys is early to mid-October for autumn and late-February to early March for spring when both the entry and persistence probabilities are relatively high. It will be important to continue to monitor potential changes in migration timing. Additional research on arrival and departure dates for other stopover areas may also be informative for annual autumn population surveys conducted to estimate the abundance of RMP sandhill cranes (Bunting et al., 2022).
Weather factors played an important role in the persistence probability of RMP sandhill cranes and were mostly consistent with our hypotheses. The importance of wind components may provide some evidence that RMP sandhill cranes prioritize energy maximization in both seasons but particularly autumn. Tailwind had a negative effect on persistence probability in autumn, while crosswinds at 700 hPa, equivalent to about 3,000 m in altitude, had a positive effect. Supportive winds can reduce the energetic costs of flight (Cooper et al., 2023), particularly for a large bird like a sandhill crane (Hedenström, 1993; Weber et al., 1998), as the cost of flapping flight increases with increasing body mass (Peters, 1986). Other studies have shown that tailwinds have a negative effect on persistence probability of migrating passerines (Schaub et al., 2004; Roques et al., 2022) and soaring raptors (Mellone et al., 2012; Limiñana et al., 2013). However, we did not find tailwinds at any altitude to be important for the spring season, which was inconsistent with our hypothesis. This could be due to the fact that winds at higher altitudes primarily come from the north, reducing the frequency of days with tailwinds (Supplementary Figure 2). Because RMP sandhill cranes must fly over or bypass a mountain range, winds at higher altitudes are likely more important than at the surface. We found that crosswind at the surface and wind speed at 700 hPa had a positive effect on persistence in spring, with some evidence of a stronger wind speed effect later in the season. Other studies have found wind speed or wind support at higher altitudes to influence departure (Mateos-Rodríguez and Liechti, 2012; Carneiro et al., 2020). Cranes use a mix of flapping powered and soaring flight and will take advantage of thermals to gain altitude and glide (Pennycuick et al., 1979; Galtbalt et al., 2022). Sandhill cranes in the SLV have been observed using thermals, and high wind speeds from the opposite direction at high altitudes are likely not favorable for northward migration.
The finding that barometric air pressure also had a negative influence on persistence probability provides evidence that ideal weather and/or soaring conditions might be important for spring departure, but this may require further validation. The influence or correlation of barometric air pressure with persistence has been documented in studies of both small and large migrants (Shamoun-Baranes et al., 2006; Sapir et al., 2011; Cooper et al., 2023), including other crane species (Pennycuick et al., 1979; Alonso et al., 1990a, Alonso et al., 1990b). Increasing barometric air pressure is generally associated with calm, fair weather conditions with light winds. Studies have found spring migrants depart or increase migration intensity during high pressure conditions (Richardson, 1978, 1990; Maransky et al., 1997), as these conditions might be suitable for northbound migration and potentially provide better conditions for thermal uplift. There is also evidence that birds can detect changes in barometric air pressure (Kreithen and Keeton, 1974), an ability that might allow for maintaining a certain altitude during migration or detection of conditions favorable for migration.
The effect of surface water did not appear to be more important than time and weather covariates on persistence probability, but water is still a critical component to consider given its role in sandhill crane roosting and foraging (Boggie et al., 2018; Donnelly et al., 2021). We had hypothesized that surface water would have little to no effect on spring persistence and have a positive influence on persistence in autumn, as more surface water means more roosting potential. Our findings were consistent with our hypothesis in spring, but we found a negative influence on persistence in autumn. Sandhill cranes typically choose roosting sites in areas with shallow water (Pearse et al., 2017; Boggie et al., 2018), and the proximity of foraging areas near roost sites tends to determine the distribution of sandhill cranes (Krapu et al., 1984; Sparling and Krapu, 1994). A negative effect of surface water on persistence in autumn could indicate that the water available is not ideal for roosting or foraging (e.g., too deep). It is also possible that with enough water, RMP sandhill cranes can acquire energetic requirements more efficiently and depart sooner. However, we acknowledge that our estimates of water were spatially and temporally coarse. Surface water is dynamic throughout spring and autumn in the SLV (Wetland Dynamics, 2019). Our seasonal estimates may not have been able to capture effects on persistence within seasons, such as late autumn, which may have a greater influence on persistence probability than total surface water throughout the season. The impact of water on RMP sandhill cranes will be important to investigate further, as water is an increasingly limited resource in the SLV and other parts of the Intermountain West due to over-allocation for irrigation and the effects of climate change (Ray et al., 2008; Mix et al., 2011). Our results provide information on when water can be distributed on the landscape for RMP sandhill cranes, particularly on public areas that are managed for waterbirds. Allocating water when the probability of arrival is high and persistence probability has not greatly declined will allow managers to use a depleting resource more efficiently throughout both seasons.
The finding that persistence is partially linked to weather and environmental covariates indicates that shifts in these cue values may alter migratory behavior and/or generate phenological mismatches if RMP sandhill cranes are unable to adapt or show plasticity when responding to climate and landscape changes (Visser et al., 2012; Visser and Gienapp, 2019). There is already documentation of weather and environmental covariates shifting in the western United States (Llewellyn and Vaddey, 2013; Dettinger et al., 2015). Reduced streamflow and earlier spring snowmelt have been documented in mountainous areas due to increasing temperatures (Barnett et al., 2008; Dettinger et al., 2015; Elias et al., 2015), and temperatures are projected to continue to increase within the coming decades (Ray et al., 2008; Llewellyn and Vaddey, 2013). Mix et al. (2011) also reported evidence of increasing temperatures in the SLV in the last century, and Donnelly et al. (2021) showed a decline in surface water throughout the region. Furthermore, other weather covariates, including wind speed and precipitation, are projected to shift under climate change (Liu et al., 2013; La Sorte et al., 2019), which could influence migratory decisions of RMP sandhill cranes. On the one hand, a mistiming in the response to an environmental or climatic cue that initiates a migratory behavior and results in arriving at a destination when conditions are suboptimal may lead to reduced fitness potential and, in some cases, population decline (Both et al., 2006; Møller et al., 2008; Visser et al., 2012; Visser and Gienapp, 2019). On the other hand, there is evidence that species and populations that are more rapidly able to respond to climatic and environmental variation (i.e., show phenotypic plasticity) are less threatened by climate change and demonstrate a lower rate of decline (Møller et al., 2008). For example, while cranes and other long-lived, gregarious birds show evidence of a genetic component to migration, the experience of older individuals seems to play an important role and may allow these groups to respond to changing environmental conditions over time (Chernetsov et al., 2004; Mueller et al., 2013; Teitelbaum et al., 2016). However, a population-level shift in migratory performance or behavior may take years in long-lived birds (Mueller et al., 2013), making such species still susceptible to phenological mismatches. An analysis of migratory and movement decisions throughout the full annual cycle of RMP sandhill cranes could provide more insight into the potential impacts of climate and land use change on this population.
There are other important factors that may influence the stopover decisions of migrating birds that we did not address, including waste grain availability, the accumulation of energy reserves at stopover areas, and the sex, age, or experience of individuals. Previous work on other migratory species has suggested that birds may delay the initiation of migration and remain at wintering or stopover areas longer if adequate resources are available and environmental conditions are favorable (Schummer et al., 2010; Thurber et al., 2020; Cox et al., 2023). Because waste grain is an important component of the diet of RMP sandhill cranes in the SLV, it is possible that the amount and distribution of grain may influence their stopover decisions. Indeed, other species of cranes have shown evidence of shifting their winter distributions and migratory timing in response to climate and availability of agricultural foods (Alonso et al., 2008; Jorgensen and Brown, 2017). However, we were not able to assess waste grain availability throughout the SLV and estimates of grain planted would be an overestimate, but future work could consider its role in stopover decisions. Additionally, evidence from earlier studies suggests that cranes vary in their stopover decisions as they age and gain more experience (Mueller et al., 2013; Teitelbaum et al., 2016; Abrahms et al., 2021). Family groups may behave differently at stopover areas than adults without young (Gerber et al., 2020). While males are typically larger than females, there can be some overlap (Krapu et al., 1985; Gerber et al., 2020), so we were not able to sex all individuals or confidently identify family groups. Our analysis was also limited to adult individuals, and we were not able to determine body condition throughout migration. The rate of fuel deposition can be a major determinant in the timing of departure for migrant birds, particularly for those prioritizing time over energy (Alerstam and Lindström, 1990; Lindström, 1991; Hedenström and Alerstam, 1997). Departure probability has been found to be related to increasing lipid levels or body condition in other migrant birds (Anderson et al., 2019; Vanausdall and Dinsmore, 2021). Given that sandhill cranes are rapidly depositing fat during spring migration in other populations at key stopover areas (Krapu et al., 1985, 2014; Fronczak et al., 2017), examining the influence of body condition on departure decisions of RMP sandhill cranes could be valuable.
Data availability statement
The raw data supporting the conclusions of this article will be made available by the authors, without undue reservation.
Ethics statement
The animal study was approved by New Mexico State University Institutional Animal Care and Use Committee. The study was conducted in accordance with the local legislation and institutional requirements.
Author contributions
RV: Conceptualization, Formal analysis, Investigation, Validation, Visualization, Writing – original draft, Writing – review & editing. WK: Conceptualization, Formal analysis, Funding acquisition, Investigation, Methodology, Supervision, Validation, Writing – original draft, Writing – review & editing. DC: Conceptualization, Data curation, Funding acquisition, Investigation, Methodology, Project administration, Resources, Supervision, Validation, Writing – review & editing. QH: Data curation, Funding acquisition, Resources, Supervision, Writing – review & editing.
Funding
The author(s) declare financial support was received for the research, authorship, and/or publication of this article. Financial and logistical support for this research was provided by the U.S. Fish and Wildlife Service (USFWS) Southwest Region Migratory Bird Program and the Mountain Prairie Region National Wildlife Refuge System, Science Applications, and Migratory Bird Management Office. Funding was also provided by the U.S. Geological Survey (G19AC00342) and the USFWS Cooperative Ecosystems Studies Units (F18AC00986).
Acknowledgments
We thank the Bosque del Apache NWR staff, New Mexico Department of Game and Fish staff at Ladd S. Gordon Waterfowl Complex, and Idaho Fish and Game for field and technical support over the course of the unit deployment. Any use of trade, firm, or product names is for descriptive purposes only and does not imply endorsement by the U.S. Government. Data are not currently available from the USFWS.
Conflict of interest
Author QH was employed by GeoSystems Analysis, Inc.
The remaining authors declare that the research was conducted in the absence of any commercial or financial relationships that could be construed as a potential conflict of interest.
Publisher’s note
All claims expressed in this article are solely those of the authors and do not necessarily represent those of their affiliated organizations, or those of the publisher, the editors and the reviewers. Any product that may be evaluated in this article, or claim that may be made by its manufacturer, is not guaranteed or endorsed by the publisher.
Supplementary material
The Supplementary Material for this article can be found online at: https://www.frontiersin.org/articles/10.3389/fevo.2024.1279279/full#supplementary-material
References
Abrahms B., Teitelbaum C. S., Mueller T., Converse S. J. (2021). Ontogenetic shifts from social to experiential learning drive avian migration timing. Nat. Commun. 12, 1–8. doi: 10.1038/s41467-021-27626-5
Adams J. B., Gillespie A. R. (2006). Remote Sensing of Landscapes with Spectral Images (Cambridge: Cambridge University Press).
Ahrens C. D. (2013). Meteorology Today: An Introduction to Weather, Climate, and the Environment. 10th ed (Belmont, CA: Brooks/Cole).
Akaike H. (1973). “Information theory and an extension of the maximum likelihood principle,” in 2nd International Symposium on Information Theory, vol. 276–281 . Eds. Petran B. N., Csaki F. (Akadémiai Kiadó, Budapest, Hungary).
Åkesson S., Hedenström A. (2000). Wind selectivity of migratory flight departures in birds. Behav. Ecol. Sociobiol. 47, 140–144. doi: 10.1007/s002650050004
Åkesson S., Hedenström A. (2007). How migrants get there: migratory performance and orientation. BioScience 57, 123–133. doi: 10.1641/B570207
Åkesson S., Helm B. (2020). Endogenous programs and flexibility in bird migration. Front. Ecol. Evol. 8. doi: 10.3389/fevo.2020.00078
Alerstam T. (2011). Optimal bird migration revisited. J. Ornithol. 152, S5–S23. doi: 10.1007/s10336-011-0694-1
Alerstam T., Hedenström A. (1998). The development of bird migration theory. J. Avian Biol. 29, 343–369. doi: 10.2307/3677155
Alerstam T., Lindström A. (1990). “Optimal bird migration: the relative importance of time, energy, and safety,” in Bird Migration: Physiology and Ecophysiology. Ed. Gwinner E. (Heidelberg, Germany: Springer-Verlag), 331–351.
Alisauskas R. T. (2002). Arctic climate, spring nutrition, and recruitment in Midcontinent lesser snow geese. J. Wildl. Manage. 66, 181–193. doi: 10.2307/3802884
Alonso J. A., Alonso J. C., Cantos F. J., Bautista L. M. (1990a). Spring crane Grus grus migration through Gallocanta, Spain: II. Timing and pattern of daily departures. Ardea 78, 379–388.
Alonso J. C., Alonso J. A., Cantos F. J., Bautista L. M. (1990b). Spring crane Grus grus migration through Gallocanta, Spain: I. Daily variations in migration volume. Ardea 78, 365–378.
Alonso J. A., Alonso J. C., Nowald G. (2008). Migration and wintering patterns of a central European population of common cranes Grus grus. Bird Study 55, 1–7. doi: 10.1080/00063650809461499
Anderson A. M., Duijns S., Smith P. A., Friis C., Nol E. (2019). Migration distance and body condition influence shorebird migration strategies and stopover decisions during southbound migration. Front. Ecol. Evol. 7. doi: 10.3389/fevo.2019.00251
Ankney C. D., MacInnes C. D. (1978). Nutrient reserves and reproductive performance of female lesser snow geese. Auk 95, 459–471. doi: 10.1093/auk/95.3.459
Arizaga J., Belda E. J., Barba E. (2011). Effect of fuel load, date, rain and wind on departure decisions of a migratory passerine. J. Ornithol. 152, 991–999. doi: 10.1007/s10336-011-0685-2
Arnold T. W. (2010). Uninformative parameters and model selection using Akaike’s Information Criterion. J. Wildl. Manage. 74, 1175–1178. doi: 10.2193/2009-367
Ashmole N. P. (1963). The regulation of numbers of tropical oceanic birds. Ibis 103b, 458–473. doi: 10.1111/j.1474-919X.1963.tb06766.x
Barnett T. P., Pierce D. W., Hidalgo H. G., Bonfils C., Santer B. D., Das T., et al. (2008). Human-induced changes in the hydrology of the western United States. Sci. (1979) 316, 1180–1183. doi: 10.1126/science.1139601
Bauer S., Gienapp P., Madsen J. (2008). The relevance of environmental conditions for departure decision changes en route in migrating geese. Ecology 89, 1953–1960. doi: 10.1890/07-1101.1
Boggie M. A., Collins D. P., Patrick Donnelly J., Carleton S. A. (2018). Land use, anthropogenic disturbance, and riverine features drive patterns of habitat selection by a wintering waterbird in a semiarid environment. PloS One 13, 1–26. doi: 10.1371/journal.pone.0206222
Both C., Artemyev A. V., Blaauw B., Cowie R. J., Dekhuijzen A. J., Eeva T., et al. (2004). Large-scale geographical variation confirms that climate change causes birds to lay earlier. Proc. R. Soc B Biol. 271, 1657–1662. doi: 10.1098/rspb.2004.2770
Both C., Bouwhuis S., Lessells C. M., Visser M. E. (2006). Climate change and population declines in a long-distance migratory bird. Nature 441, 81–83. doi: 10.1038/nature04539
Breuner C. W., Sprague R. S., Patterson S. H., Woods H. A. (2013). Environment, behavior and physiology: Do birds use barometric pressure to predict storms? J. Exp. Biol. 216, 1982–1990. doi: 10.1242/jeb.081067
Bunting D. P., Boggie M. A., Collins D. P., Thorpe P. P., Donnelly J. P. (2022). Linking ecological processes and animal movements to inform timing of long-term surveys of a migratory game bird. Ecosphere 13, 1–19. doi: 10.1002/ecs2.4298
Burnham K. P., Anderson D. R. (2002). Model selection and multimodal inference: A practical information-theoretic approach. 1st ed (New York, New York: Springer-Verlag).
Carlisle M. J., Tacha T. C. (1983). Fall migration of sandhill cranes in West Central North Dakota. J. Wildl. Manage. 47, 818–821. doi: 10.2307/3808617
Carneiro C., Gunnarsson T. G., Alves J. A. (2020). Linking weather and phenology to xtopover dynamics of a long-distance migrant. Front. Ecol. Evol. 8. doi: 10.3389/fevo.2020.00145
Chapman B. B., Brönmark C., Nilsson J. Å., Hansson L. A. (2011). The ecology and evolution of partial migration. Oikos 120, 1764–1775. doi: 10.1111/j.1600-0706.2011.20131.x
Chernetsov N., Berthold P., Querner U. (2004). Migratory orientation of first-year white storks (Ciconia ciconia): Inherited information and social interactions. J. Exp. Biol. 207, 937–943. doi: 10.1242/jeb.00853
Collins D. P., Grisham B. A., Conring C. M., Knetter J. M., Conway W. C., Carleton S. A., et al. (2016). New summer areas and mixing of two greater sandhill crane populations in the Intermountain West. J. Fish Wildl. Manage. 7, 141–152. doi: 10.3996/042015-JFWM-036
Collins D., Vanausdall R. A. (2023). October 2022 recruitment survey of the Rocky Mountain Population of greater sandhill cranes (U.S. Fish and Wildlife Service), 1–10. Available at: https://www.fws.gov/media/survey-rocky-mountain-population-greater-sandhill-cranes-2022.
Cooch E. G., White G. C. (2017) Program MARK: a gentle introduction. Available online at: www.phidot.org/software/mark/docs/book.
Cooper N. W., Dossman B. C., Berrigan L. E., Brown J. M., Cormier D. A., Bégin-Marchand C., et al. (2023). Atmospheric pressure predicts probability of departure for migratory songbirds. Mov. Ecol. 11, 1–11. doi: 10.1186/s40462-022-00356-z
Cormack R. M. (1964). Estimates of survival from the sighting of marked animals. Biometrika 51, 429–438. doi: 10.1093/biomet/51.3-4.429
Cox G. W. (1985). The evolution of avian migration systems between temperate and tropical regions of the world. Am. Nat. 126, 451–474. doi: 10.1086/284432
Cox A. R., Frei B., Gutowsky S. E., Baldwin F. B., Bianchini K., Roy C. (2023). Sixty-years of community-science data suggest earlier fall migration and short-stopping of waterfowl in North America. Ornithol. App. 125, 1–14. doi: 10.1093/ornithapp/duad041
Dettinger M., Udall B., Georgakakos A. (2015). Western water and climate change. Ecol. App. 25, 2069–2093. doi: 10.1890/15-0938.1
Donnelly J. P., Collins D. P., Knetter J. M., Gammonley J. H., Boggie M. A., Grisham B. A., et al. (2023). Flood-irrigated agricultural mediates climate-induced wetland scarcity for summering sandhill cranes in western North America. Available at: https://www.biorxiv.org/content/10.1101/2023.11.03.565509v1.
Donnelly J. P., King S. L., Knetter J., Gammonley J. H., Dreitz V. J., Grisham B. A., et al. (2021). Migration efficiency sustains connectivity across agroecological networks supporting sandhill crane migration. Ecosphere 12. doi: 10.1002/ecs2.3543
Donnelly J. P., Naugle D. E., Collins D. P., Dugger B. D., Allred B. W., Tack J. D., et al. (2019). Synchronizing conservation to seasonal wetland hydrology and waterbird migration in semi-arid landscapes. Ecosphere 10, 1–22. doi: 10.1002/ecs2.2758
Drewien R. C., Bizeau E. G. (1974). Status and distribution of greater sandhill cranes in the Rocky Mountains. J. Wildl. Manage. 38, 720–742. doi: 10.2307/3800039
Duijns S., Anderson A. M., Aubry Y., Dey A., Flemming S. A., Francis C. M., et al. (2019). Long-distance migratory shorebirds travel faster towards their breeding grounds, but fly faster post-breeding. Sci. Rep. 9, 1–13. doi: 10.1038/s41598-019-45862-0
Elias E. H., Rango A., Steele C. M., Mejia J. F., Smith R. (2015). Assessing climate change impacts on water availability of snowmelt-dominated basins of the Upper Rio Grande basin. J. Hydrol. Reg. Stud. 3, 525–546. doi: 10.1016/j.ejrh.2015.04.004
Emery P. A., Boettcher A. J., Snipes R. J., Mcintyre H. J. (1969). Hydrology of the San Luis Valley, south-central Colorado (Denver, Colorado: U.S. Geological Survey).
Fronczak D. L., Andersen D. E., Hanna E. E., Cooper T. R. (2017). Distribution and migration chronology of Eastern population sandhill cranes. J. Wildl. Manage. 81, 1021–1032. doi: 10.1002/jwmg.21272
Galtbalt B., Batbayar N., Sukhbaatar T., Vorneweg B., Heine G., Müller U., et al. (2022). Differences in on-ground and aloft conditions explain seasonally different migration paths in Demoiselle crane. Mov. Ecol. 10, 1–11. doi: 10.1186/s40462-022-00302-z
Gerber B. D., Dwyer J. F., Nesbitt S. A., Drewien R. C., Littlefield C. D., Tacha T. C., et al. (2020). “Sandhill Crane (Antigone canadensis), version 1.0,” in Birds of the World. Ed. Poole A. F. (Cornell Lab of Ornithology, Ithaca, NY, USA).
Gerber B. D., Kendall W. L., Hooten M. B., Dubovsky J. A., Drewien R. C. (2015). Optimal population prediction of sandhill cranes recruitment based on climate-mediated habitat limitations. J. Anim. Ecol. 84, 1299–1310. doi: 10.1111/1365-2656.12370
Gill R. E., Douglas D. C., Handel C. M., Tibbitts T. L., Hufford G., Piersma T. (2014). Hemispheric-scale wind selection facilitates bar-tailed godwit circum-migration of the Pacific. Anim. Behav. 90, 117–130. doi: 10.1016/j.anbehav.2014.01.020
Gordo O. (2007). Why are bird migration dates shifting? A review of weather and climate effects on avian migratory phenology. Clim. Res. 35, 37–58. doi: 10.3354/cr00713
Gorelick N., Hancher M., Dixon M., Ilyushchenko S., Thau D., Moore R. (2017). Google Earth Engine: Planetary-scale geospatial analysis for everyone. Remote. Sens. Environ. 202, 18–27. doi: 10.1016/j.rse.2017.06.031
Haest B., Hüppop O., Bairlein F. (2020). Weather at the winter and stopover areas determines spring migration onset, progress, and advancements in Afro-Palearctic migrant birds. Proc. Nat. Acad. Sci. 117, 17056–17062. doi: 10.5281/zenodo.3629178
Hays Q. R., Tredennick A. T., Carlisle J. D., Collins D. P., Carleton S. A. (2021). Spatially explicit assessment of sandhill crane exposure to potential transmission line collision risk. J. Wildl. Manage. 85, 1440–1449. doi: 10.1002/jwmg.22100
Hedenström A. (1993). Migration by soaring or flapping flight in birds: the relative importance of energy cost and speed. Philso. Trans. R. Soc B 342, 353–361. doi: 10.1098/rstb.1993.0164
Hedenström A., Alerstam T. (1997). Optimum fuel loads in migratory birds: Distinguishing between time and energy minimization. J. Theor. Biol. 189, 227–234. doi: 10.1006/jtbi.1997.0505
Helwig N. E. (2022) npreg: Nonparametric Regression via Smoothing Splines. R package version 1.0-9. Available online at: https://CRAN.R-project.org/package=npreg.
Illan J. G., Wang G., Cunningham F. L., King D. T. (2017). Seasonal effects of wind conditions on migration patterns of soaring American white pelican. PloS One 12, 1–15. doi: 10.1371/journal.pone.0186948
Ivey G. L., Dugger B. D., Herziger C. P., Casazza M. L., Fleskes J. P. (2015). Wintering ecology of sympatric subspecies of Sandhill Crane: Correlations between body size, site fidelity, and movement patterns. Condor 117, 518–529. doi: 10.1650/condor-14-159.1
Jenni L., Kéry M. (2003). Timing of autumn bird migration under climate change: Advances in long-distance migrants, delays in short-distance migrants. Proc. R. Soc B Biol. Sci. 270, 1467–1471. doi: 10.1098/rspb.2003.2394
Jin H., Huang C., Lang M. W., Yeo I.-Y., Stehman S. V. (2017). Monitoring of wetland inundation dynamics in the Delmarva Peninsula using Landsat time-series imagery from 1985 to 2011. Remote. Sens. Environ. 190, 26–41. doi: 10.1016/j.rse.2016.12.001
Johnson D., Austin J., Shaffer J. (2005). A fresh look at the taxonomy of Midcontinental sandhill cranes. Proc. North American Crane Workshop. 9, 37–45.
Jorgensen J. G., Brown M. B. (2017). Temporal migration shifts in the Aransas-Wood Buffalo Population of whooping cranes (Grus americana) across North America. Waterbirds 40, 195–308. doi: 10.1675/063.040.0302
Kanamitsu M., Ebisuzaki W., Woollen J., Yang S.-K., Hnilo J. J., Fiorino M., et al. (2002). NCEP-DOE AMIP-II Reanalysis (R-2). American Meteorological Society. 1631–1643. doi: 10.1175/BAMS-83-11
Karlsson H., Nilsson C., Bäckman J., Alerstam T. (2012). Nocturnal passerine migrants fly faster in spring than in autumn: A test of the time minimization hypothesis. Anim. Behav. 83, 87–93. doi: 10.1016/j.anbehav.2011.10.009
Kemp J., Boyd W. S., Forstner T. M., Esler D., Bowman T. D., Douglas D. C., et al. (2023). Pacific Barrow’s Goldeneye refine migratory phenology in response to overwintering temperatures and annual snowmelt. Ornithol. 140, 1–13. doi: 10.1093/ornithology/ukad024
Kemp M. U., Emiel van Loon E., Shamoun-Baranes J., Bouten W. (2012). RNCEP: Global weather and climate data at your fingertips. Methods Ecol. Evol. 3, 65–70. doi: 10.1111/j.2041-210X.2011.00138.x
Kendall W. L., Stapleton S., White G. C., Richardson J. I., Pearson K. N., Mason P. (2019). A multistate open robust design: population dynamics, reproductive effort, and phenology of sea turtles from tagging data. Ecol. Monogr. 89, 1–17. doi: 10.1002/ecm.1329
Kokko H. (1999). Competition for early arrival in migratory birds. J. Anim. Ecol. 68, 940–950. doi: 10.1046/j.1365-2656.1999.00343.x
Kölzsch A., Müskens G. J. D. M., Kruckenberg H., Glazov P., Weinzierl R., Nolet B. A., et al. (2016). Towards a new understanding of migration timing: slower spring than autumn migration in geese reflects different decision rules for stopover use and departure. Oikos. 125, 1496–1507. doi: 10.5441/001/1.31c2v92f
Krapu G. L., Brandt D. A., Kinzel P. J., Pearse A. T. (2014). Spring migration ecology of the Mid-Continent sandhill crane population with an emphasis on use of the Central Platte River Valley, Nebraska. Wildl. Monogr. 189, 1–41. doi: 10.1002/wmon.l013
Krapu G. L., Facey D. E., Fritzell E. K., Johnson D. H. (1984). Habitat use by migrant sandhill cranes in Nebraska. J. Wildl Manage. 48, 407–417. doi: 10.2307/3801172
Krapu G. L., Iverson G. C., Reinecke K. J., Boise C. M. (1985). Fat deposition and usage by arctic-nesting Sandhill Cranes during spring. Auk 102, 362–368. doi: 10.2307/4086780
Kreithen M. L., Keeton W. T. (1974). Detection of changes in atmospheric pressure by the homing pigeon, Columba livia. J. Comp. Physiol. 89, 73–82. doi: 10.1007/BF00696164
Laake J. L. (2013). “RMark: an interface for analysis of capture-recapture data with MARK,” in AFSC Processed Rep. 2013-01 (Alaska Fish. Sci. Cent., NOAA, Natl. Mar. Fish. Serv., 7600 Sand Point Way, NE, Seattle, WA 98115), 25 p.
La Sorte F. A., Horton K. G., Nilsson C., Dokter A. M. (2019). Projected changes in wind assistance under climate change for nocturnally migrating bird populations. Glob. Chang Biol. 25, 589–601. doi: 10.1111/gcb.14531
Lawrence K. B., Barlow C. R., Bensusan K., Perez C., Willis S. G. (2021). Phenological trends in the pre- and post-breeding migration of long-distance migratory birds. Glob. Change Biol. 28, 375–389. doi: 10.1111/gcb.15916
Lebreton J.-D., Burnham K. P., Clobert J., Anderson D. R. (1992). Modeling survival and testing biological hypotheses using marked animals: a unified approach with case studies. Ecol. Mon. 62, 67–118. doi: 10.2307/2937171
Limiñana R., Romero M., Mellone U., Urios V. (2013). Is there a different response to winds during migration between soaring and flapping raptors? An example with the Montagu’s harrier and the lesser kestrel. Behav. Ecol. Sociobiol. 67, 823–835. doi: 10.1007/s00265-013-1506-9
Lindström Å. (1991). Maximum fat deposition rates in migrating birds. Scandinavian J. Ornithol. 22, 12–19. doi: 10.2307/3676616
Liu Y., Goodrick S. L., Stanturf J. A. (2013). Future U.S. wildfire potential trends projected using a dynamically downscaled climate change scenario. For. Ecol. Manage. 294, 120–135. doi: 10.1016/j.foreco.2012.06.049
Llewellyn D., Vaddey S. (2013). West-Wide Climate Risk Assessment: Upper Rio Grande Impact Assessment (Albuqurque, NM: Bureau of Reclamation).
Lundberg P. (1988). The evolution of partial migration in birds. Trends Ecol. Evol. 3, 172–175. doi: 10.1016/0169-5347(88)90035-3
Mandel J. T., Bohrer G., Winkler D. W., Barber D. R., Houston C. S., Bildstein K. L. (2011). Migration path annotation: Cross-continental study of migration-flight response to environmental conditions. Ecol. App. 21, 2258–2268. doi: 10.1890/10-1651.1
Maransky B., Goodrich L., Bildstein K. (1997). Seasonal shifts in the effects of weather on the visible migration of Red-Tailed Hawks at Hawk Mountain, Pennsylvania 1992-1994. Wilson Bull. 109, 246–252.
Mateos-Rodríguez M., Liechti F. (2012). How to diurnal long-distance migrants select flight altitude in relation to wind. Behav. Ecol. 23, 403–409. doi: 10.1093/beheco/arr204
Mellone U., Klaassen R. H. G., García-Ripollés C., Limiñana R., López-López P., Pavón D., et al. (2012). Interspecific comparison of the performance of soaring migrants in relation to morphology, meteorological conditions and migration strategies. PloS One 7, 1–11. doi: 10.1371/journal.pone.0039833
Metcalfe J., Schmidt K. L., Bezner Kerr W., Guglielmo C. G., MacDougall-Shackleton S. A. (2013). White-throated sparrows adjust behaviour in response to manipulations of barometric pressure and temperature. Anim. Behav. 86, 1285–1290. doi: 10.1016/j.anbehav.2013.09.033
Mitchell G. W., Woodworth B. K., Taylor P. D., Norris D. R. (2015). Automated telemetry reveals age specific differences in flight duration and speed are driven by wind conditions in a migratory songbird. Mov. Ecol. 3, 1–13. doi: 10.1186/s40462-015-0046-5
Mix K., Lopes V. L., Rast W. (2011). Annual and growing season temperature changes in the San Luis Valley, Colorado. Water Air Soil pollut. 220, 189–203. doi: 10.1007/s11270-011-0746-4
Mix K., Rast W., Lopes V. L. (2010). Increases in growing degree days in the alpine desert of the San Luis Valley, Colorado. Water Air Soil pollut. 205, 289–304. doi: 10.1007/s11270-009-0074-0
Møller A. P. (2001). Heritability of arrival date in a migratory bird. Proc. R. Soc B Biol. Sci. 268, 203–206. doi: 10.1098/rspb.2000.1351
Møller P. A., Rubolini D., Lehikoinen E. (2008). Populations of migratory bird species that did not show a phenological response to climate change are declining. Proc. Nat. Acad. Sci. 105, 16195–16200. doi: 10.1073/pnas.0803825105
Morin D. J., Yackulic C. B., Diffendorfer J. E., Lesmeister D. B., Nielsen C. K., Reid J., et al. (2020). Is your ad hoc model selection strategy affecting your multimodel inference? Ecosphere 11, 1–16. doi: 10.1002/ecs2.2997
Mueller T., O’Hara R. B., Converse S. J., Urbanek R. P., Fagan W. F. (2013). Social learning of migratory performance. Sci. (1979) 341, 999–1002. doi: 10.1126/science.1239794
Newton I. (2007). Weather-related mass-mortality events in migrants. Ibis 149, 453–467. doi: 10.1111/j.1474-919X.2007.00704.x
Nilsson C., Klaassen R. H. G., Alerstam T. (2013). Differences in speed and duration of bird migration between spring and autumn. American Naturalist 181, 837–845. doi: 10.5061/dryad.82d4q
NOAA (2022) Climate Data Online. Available online at: https://www.ncei.noaa.gov/cdo-web/ (Accessed August 31, 2022).
Nolet B. A. (2006). Speed of migration of tundra swans Cygnus columbianus in accordance with income or capital breeding strategy? Ardea. 94, 579–591.
Obernuefemann K. P., Collazo J. A., Lyons J. E. (2013). Local movements and wetland connectivity at a migratory stopover of semipalmated sandpipers (Calidris pusilla) in the Southeastern United States. Waterbirds 36, 63–76. doi: 10.1675/063.036.0110
Ojaste I., Leito A., Hedenström A., Sepp K., Leivits M., Sellis U., et al. (2020). From northern Europe to Ethiopia: long-distance migration of Common Cranes (Grus grus). Ornis Fenn 97, 12–25. doi: 10.51812/of.133962
O’Neal B. J., Stafford J. D., Larkin R. P., Michel E. S. (2018). The effect of weather on the decision to migrate from stopover sites by autumn-migrating ducks. Mov. Ecol. 6, 1–19. doi: 10.1186/s40462-018-0141-5
Panuccio M., Barboutis C., Chiatante G., Evangelidis A., Nicolantonio A., Panuccio A. M., et al. (2016). Pushed by increasing air temperature and tailwind speed: weather selectivity of raptors migrating across the Aegean Sea. Ornis. Fenn. 93, 159–171. doi: 10.51812/of.133898
Pearse A. T., Krapu G. L., Brandt D. A. (2017). Sandhill crane roost selection, human disturbance, and forage resources. J. Wildl. Manage. 81, 477–486. doi: 10.1002/jwmg.21215
Pearse A. T., Krapu G. L., Brandt D. A., Kinzel P. J. (2010). Changes in agriculture and abundance of snow geese affect carrying capacity of sandhill cranes in Nebraska. J. Wildl. Manage. 74, 479–488. doi: 10.2193/2008-539
Pearse A. T., Metzger K. L., Brandt D. A., Bidwell M. T., Harner M. J., Baasch D. M., et al. (2020). Heterogeneity in migration strategies of Whooping Cranes. Condor 122, 1–15. doi: 10.1093/condor/duz056
Pennycuick C. J., Alerstam T., Larsson B. (1979). Soaring migration of the common crane Grus grus observed by radar and from an aircraft. Ornis Scand. 10, 241–251. doi: 10.2307/3676068
Petersen J. L., Bischof R., Krapu G. L., Szalanski A. L. (2003). Genetic variation in the Midcontinental population of sandhill cranes. Biochem. Gen. 41, 1–12. doi: 10.1023/A:1020985427461
Peters R. H. (1986). The ecological implications of body size (Cambridge: Cambridge University Press).
Piersma T., Jukema J. (1990). Budgeting the flight of a long-distance migrant: changes in nutrient reserve levels of bar-tailed godwits at successive spring staging sites. Ardea 78, 315–337. doi: 10.5253/arde.v78.p315
Pulido F. (2011). Evolutionary genetics of partial migration - the threshold model of migration revis(it)ed. Oikos 120, 1776–1783. doi: 10.1111/j.1600-0706.2011.19844.x
Ray A. J., Barsugli J. J., Averyt K. B., Wolter K., Hoerling M., Doesken N., et al. (2008). Climate change in Colorado: A synthesis to support water resources management and adaptation (Boulder, Colorado, USA: Western Water Assessment).
R Core Team (2022). R version 4.2.3: A language and environment for statistical computing (Vienna, Austria: R Foundation for Statistical Computing).
Richardson W. J. (1978). Timing and amount of bird migration in relation to weather: a review. Oikos 30, 224–272. doi: 10.2307/3543482
Richardson W. J. (1990). Timing of bird migration in relation to weather: updated review. Oikos 30, 78–101. doi: 10.2307/3543482
Rio Grande Basin Roundtable (2015) Rio Grande Basin Implementation Plan. Available online at: https://engagecwcb.org/basin-implementation-plans (Accessed August 31, 2022).
Rio Grande Basin Roundtable (2022) Rio Grande Basin Implementation Plan. Available online at: https://engagecwcb.org/basin-implementation-plans (Accessed August 31, 2022).
Roques S., Henry P.-Y., Guyot G., Bargain B., Cam E., Pradel R. (2022). When to depart from a stopover site? Time since arrival matters more than current weather conditions. Ornithol. 139, 1–13. doi: 10.1093/ornithology/ukab057
Roques S., Lank D. B., Cam E., Pradel R. (2021). More than just refuelling: lengthy stopover and selection of departure weather by sandpipers prior to transoceanic and transcontinental flights. Ibis 163, 519–535. doi: 10.1111/ibi.12891
Rüppel G., Hüppop O., Lagerveld S., Schmaljohann H., Brust V. (2023). Departure, routing and landing decisions of long-distance migratory songbirds in relation to weather. R. Soc Open Sci. 10, 1–13. doi: 10.1098/rsos.221420
Sapir N., Wikelski M., Avissar R., Nathan R. (2011). Timing and flight mode of departure in migrating European bee-eaters in relation to multi-scale meteorological processes. Behav. Ecol. Sociobiol. 65, 1353–1365. doi: 10.1007/s00265-011-1146-x
Schaub M., Liechti F., Jenni L. (2004). Departure of migrating European robins, Erithacus rubecula, from a stopover site in relation to wind and rain. Anim. Behav. 67, 229–237. doi: 10.1016/j.anbehav.2003.03.011
Schmaljohann H., Eikenaar C., Sapir N. (2022). Understanding the ecological and evolutionary function of stopover in migrating birds. Biol. Rev. 97, 1231–1252. doi: 10.1111/brv.12839
Schummer M. L., Kaminski R. M., Raedeke A. H., Graber D. A. (2010). Weather-related indices of autumn-winter dabbling duck abundance in middle North America. J. Wildl. Manage. 74, 94–101. doi: 10.2193/2008-524
Seamans M. E. (2022) Status and harvests of sandhill cranes: Mid-Continent, Rocky Mountain, Lower Colorado River Valley and Eastern Populations (U.S. Fish and Wildlife Service). Available online at: https://www.fws.gov/library/collections/sandhill-crane-population-status-reports (Accessed 6 June 2023).
Seber G. A. F. (1965). A note on the multiple-recapture census. Biometrika 52, 249–259. doi: 10.1093/biomet/52.1-2.249
Shamoun-Baranes J., Baharad A., Alpert P., Berthold P., Yom-Tov Y., Dvir Y., et al. (2003). The effect of wind, season and latitude on the migration speed of white storks Ciconia Ciconia, along the eastern migration route. J. Avian Biol. 34, 97–104. doi: 10.1034/j.1600-048X.2003.03079.x
Shamoun-Baranes J., Liechti F., Vansteelant W. M. G. (2017). Atmospheric conditions create freeways, detours, and tailbacks for migrating birds. J. Comp. Physiol. A. 203, 509–529. doi: 10.1007/s00359-017-1181-9
Shamoun-Baranes J., van Loon E., Alon D., Alpert P., Yom-Tov Y., Leshem Y. (2006). Is there a connection between weather at departure sites, onset of migration and timing of soaring-bird autumn migration in Israel? Global Ecol. Biog. 15, 541–552. doi: 10.1111/j.1466-822x.2006.00261.x
Sparling D. W., Krapu G. L. (1994). Communal roosting and foraging behavior of staging sandhill cranes. Wilson Bull. 106, 62–77.
Tacha T. C., Jorgenson C., Taylor P. S. (1985). Harvest, migration, and condition of Sandhill Cranes in. J. Wildl. Manage. 49, 476–480. doi: 10.2307/3801556
Teitelbaum C. S., Converse S. J., Fagan W. F., Böhning-Gaese K., O’Hara R. B., Lacy A. E., et al. (2016). Experience drives innovation of new migration patterns of whooping cranes in response to global change. Nat. Commun. 7, 1–7. doi: 10.1038/ncomms12793
Thurber B. C., Roy C., Zimmerling J. R. (2020). Long-term changes in the autumn migration phenology of dabbling ducks in southern Ontario and implications for waterfowl management. Wildl. Biol. 2, 1–11. doi: 10.2981/wlb.00668
U.S. Fish and Wildlife Service (2015). Comprehensive conservation plan: San Luis Valley National Wildlife Refuge Complex (Colorado: Lakewood).
Usui T., Butchart S. H. M., Phillimore A. B. (2017). Temporal shifts and temperature sensitivity of avian spring migratory phenology: a phylogenetic meta-analysis. J. Anim. Ecol. 86, 250–261. doi: 10.1111/1365-2656.12612
Vanausdall R. A., Dinsmore S. J. (2021). Stopover ecology of the least sandpiper (Calidris minutilla) in Iowa: implications for reservoir management. Lake Reserv. Manage. 37, 300–312. doi: 10.1080/10402381.2021.1920071
Vansteelant W. M. G., Shamoun-Baranes J., McLaren J., van Diermen J., Bouten W. (2017). Soaring across continents: decision-making of a soaring migrant under changing atmospheric conditions along an entire flyway. J. Avian Biol. 48, 887–896. doi: 10.1111/jav.01298
Visser M. E., Gienapp P. (2019). Evolutionary and demographic consequences of phenological mismatches. Nat. Ecol. Evol. 3, 879–885. doi: 10.1038/s41559-019-0880-8
Visser M. E., Marvelde L., Log M. E. (2012). Adaptive phenological mismatches of birds and their food in a warming world. J. Ornithol. 153, 75–84. doi: 10.1007/s10336-011-0770-6
Visser M. E., Perdeck A. C., van Balen J. H., Both C. (2009). Climate change leads to decreasing bird migration distances. Glob. Change Biol. 15, 1859–1865. doi: 10.1111/j.1365-2486.2009.01865.x
von Bartheld C. S. (1994). Functional morphology of the paratympanic organ in the middle ear of birds. Brain Behav. Evol. 44, 61–73. doi: 10.1159/000113570
Weber T. P., Alerstam T., Hedenström A. (1998). Stopover decisions under wind influence. J. Avian Biol. 29, 552–560. doi: 10.2307/3677175
Western Regional Climate Center (2013) Cooperative Climatological Data Summaries. Available online at: https://wrcc.dri.edu/Climate/summaries.php (Accessed August 31, 2022).
Wetland Dynamics L. (2019). San Luis Valley wetland and wildlife conservation assessment (Monte Vista, CO).
White G. C., Burnham K. P. (1999). Program mark: Survival estimation from populations of marked animals. Bird Study 46, S120–S139. doi: 10.1080/00063659909477239
Keywords: climate, migration, phenology, San Luis Valley, water
Citation: Vanausdall RA, Kendall WL, Collins DP and Hays QR (2024) Time of year and weather influence departure decisions of sandhill cranes at a primary stopover. Front. Ecol. Evol. 12:1279279. doi: 10.3389/fevo.2024.1279279
Received: 17 August 2023; Accepted: 26 February 2024;
Published: 14 March 2024.
Edited by:
Sebastien Devillard, Université Claude Bernard Lyon 1, FranceReviewed by:
Natalie Beenaerts, Hasselt University, BelgiumSean V. Zimin, Ben-Gurion University of the Negev, Israel
Michiel Lathouwers, Hasselt University, Belgium, in collaboration with reviewer NB
Copyright © 2024 Vanausdall, Kendall, Collins and Hays. This is an open-access article distributed under the terms of the Creative Commons Attribution License (CC BY). The use, distribution or reproduction in other forums is permitted, provided the original author(s) and the copyright owner(s) are credited and that the original publication in this journal is cited, in accordance with accepted academic practice. No use, distribution or reproduction is permitted which does not comply with these terms.
*Correspondence: Rachel A. Vanausdall, cmFjaGVsLnZhbmF1c2RhbGxAbWRjLm1vLmdvdg==
†These authors have contributed equally to this work