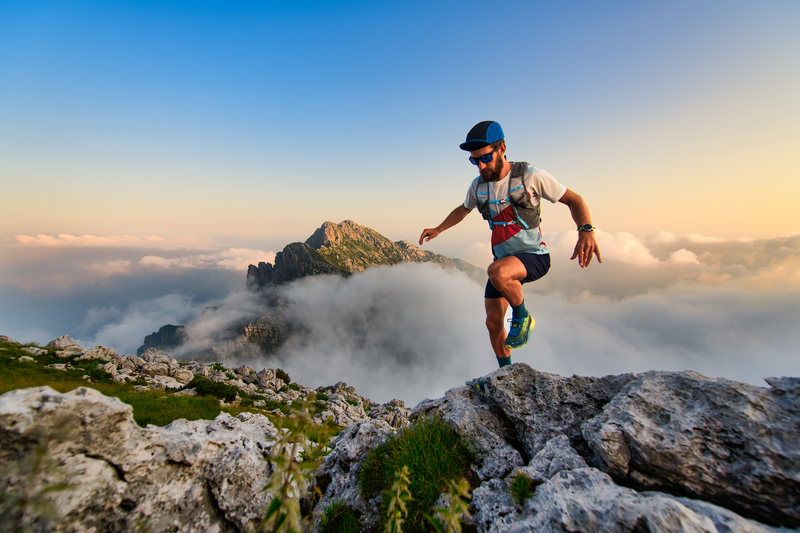
95% of researchers rate our articles as excellent or good
Learn more about the work of our research integrity team to safeguard the quality of each article we publish.
Find out more
PERSPECTIVE article
Front. Ecol. Evol. , 19 September 2024
Sec. Biogeography and Macroecology
Volume 12 - 2024 | https://doi.org/10.3389/fevo.2024.1112712
This article is part of the Research Topic Insights in Biogeography and Macroecology: 2022 View all 6 articles
Climate change significantly alters species distributions. Numerous studies project the future distribution of species using Species Distribution models (SDMs), most often using coarse resolutions. Working at coarse resolutions in forest ecosystems fails to capture landscape-level dynamics, spatially explicit processes, and temporally defined events that act at finer resolutions and that can disproportionately affect future outcomes. Dynamic Forest Landscape Models (FLMs) can simulate the survival, growth, and mortality of (stands of) trees over long time periods at small resolutions. However, as they are able to simulate at fine resolutions, study landscapes remain relatively small due to computational constraints. The large amount of feedbacks between biodiversity, forest, and ecosystem processes cannot completely be captured by FLMs or SDMs alone. Integrating SDMs with FLMs enables a more detailed understanding of the impact of perturbations on forest landscapes and their biodiversity. Several studies have used this approach at landscape scales, using fine resolutions. Yet, many scientific questions in the fields of biogeography, macroecology, conservation management, among others, require a focus on both large scales and fine resolutions. Here, drawn from literature and experience, we provide our perspective on the most important challenges that need to be overcome to use integrated frameworks at spatial scales larger than the landscape and at fine resolutions. Future research should prioritize these challenges to better understand drivers of species distributions in forest ecosystems and effectively design conservation strategies under the influence of changing climates on spatially and temporally explicit processes. We further discuss possibilities to address these challenges.
Forests are among the most species rich ecosystems, and forest associated species in turn support ecosystem functioning and provide numerous ecosystem services (Brockerhoff et al., 2017). The conservation of biodiversity in forests is amongst others challenged by land use and landcover change (henceforth referred to as landcover), which is exacerbated by climate change, and uncertainties associated with impacts of management and conservation strategies in the face of climate change (IPBES, 2019). To halt further biodiversity losses in forest ecosystems, we urgently need a better understanding of current and future combined impacts of landcover and climate change on biodiversity.
There are several different approaches to simulate the effects of such changes on spatio-temporal forest dynamics with individual requirements regarding resolution and scale. Approaches that can be used at large (global) scales and with small resolutions are Dynamic Global Vegetation Models (DGVM) and Species Distribution Models (SDMs). DGVMs can be used to simulate the interactions between climate and changes in the vegetation at a global scale while accounting for competition and disturbance (Krinner et al., 2005). The general approach of SDMs is to identify variables, amongst a set of predictor variables, that determine most of the variation in species presence to subsequently predict the relative suitability of the area under investigation for the species to occur (e.g. Phillips et al., 2006). Like DGVMs, they can be used at small resolutions and large areas, but also at high resolutions provided that the needed data are available. At the other resolution and scale spectrum are Individual Based Forest Models (IBMs), such as forest gap models. These are used to simulate the growth, development and mortality of individual trees within a forest stand (Maréchaux et al., 2021) and thus require large amounts of data at high resolution and are used at small scales. In between these two extremes are Forest Landscape Models (FLMs). FLMs are spatially explicit and designed to simulate the survival, growth, and mortality of (stands of) trees at a landscape level while accounting for landscape level interactions such as seed dispersal and natural disturbances like pest outbreaks. They were specifically designed to be able to address landscape level management issues (Scheller and Mladenoff, 2007; Shifley et al., 2017). Maréchaux et al. (2021) provide a good overview of general advantages, limitations and challenges of using DGVMs, SDMs and IBMs, but leave FLMs undiscussed. Yet, FLMs are capable to combine the simulation of detailed (stand) processes and cross-scale interactions (Temperli et al., 2013).
Many scientific questions in academic fields like biogeography, macroecology, as well as conservation management require a focus on both large regional to global scales and fine resolutions that could be relevant for management. Although biodiversity conservation generally takes place at human-scale landscapes (landscape scale hereafter) (Wu and Qi, 2000), the scale at which conservation is most effective depends on various factors. It varies with the species and communities under consideration, with goals such as conserving single populations or metapopulations, and with various anthropogenic factors. For instance, conserving a migrating bird may require cross-country approaches whilst conserving a viable population of a single species may only require a local approach (Brito and Grelle, 2006; Sodhi et al., 2011). However, successful conservation often requires larger (cross-country) scales (Kark et al., 2015). Considering this, SDMs and FLMs may be the most useful approaches to get a better understanding of current and future combined impacts of landcover and climate change on biodiversity in forest ecosystems as they both can be used at relatively large scales and with relatively high resolutions. Here we first briefly discuss both types of models and their limitations after which we offer our perspective on a possible way forward.
A growing body of studies has assessed the impacts of climate change on the distribution of numerous species using SDMs (or habitat suitability models or ecological niche models, which we here collectively refer to as SDMs). Although SDMs can be used at landscape scales and with high resolutions, these studies are generally conducted at large spatial extents (regional to global) and at coarse resolutions (generally > 30-arc seconds) and thus frequently neglect impacts compounded by landcover change (Titeux et al., 2016). Yet, decision making on managing or conserving species or ecosystems require fine resolutions. Assessments using coarse resolutions might therefore fail to capture small-grain processes that are critical for landscape-scale dynamics, such as limitations imposed by spatially explicit processes (propagule dispersal, disturbance propagation) or the effects of temporally defined perturbations on the ecosystem or microclimate (Elkin et al., 2012). The main reason that the mentioned studies are generally done at a coarse resolution is related to data limitations. Detailed data on microclimate and current landcover, let alone on future microclimate and landcover, are hardly ever available at large scales as they require large investments in monitoring or remote sensing. Moreover, using macroclimate data in fine scale predictive studies of effects of climate change on species’ presence leads to faulty predictions (Bütikofer et al., 2020; Maclean and Early, 2023). Projections in landcover change are currently only available at coarse spatial resolutions (Titeux et al., 2016) and are also considered unreliable (Stanton et al., 2012). Commonly applied downscaling methods typically focus on only a few landcover types and thus do not capture the full impact of changes in landcover on biodiversity (Titeux et al., 2016). Furthermore, changes in land management regimes and the intensity of use can have large impacts on biodiversity, yet such changes are generally ignored in predictive studies (Titeux et al., 2016). For example, Howard et al. (2023) found that changes in climate suitability were of little importance for the local colonization and extinction of birds. This finding highlights the inability to correctly predict small scale changes in species’ presence and calls for alternative approaches. Also, the needed occurrence data should be accurately georeferenced and available at a fine resolution, which is often not the case. Using occurrences with location uncertainties in high resolution studies may lead to misleading interpretations of the predictions of SDMs (Mitchell et al., 2017; Gábor et al., 2020). It is thus challenging to produce well performing SDMs at high resolutions. Instead of using correlative SDMs, which typically ignore dynamic processes and interactions, process-based models may be better at capturing the impacts of climate change on species presence at high resolutions. Process based models incorporate ecological processes and interactions and provide a mechanistic understanding of species’ responses to climate change, yet they often are complex, data and computationally demanding (Kearney and Porter, 2009; Urban et al., 2016).
In contrast to SDMs, FLMs such as LANDIS-II (Scheller et al., 2007) and iLand (Seidl et al., 2012) are process-based; they are primarily designed to capture the ecological processes of forest ecosystems in a spatially explicit manner. They simulate the impact of environmental changes on forest ecosystem dynamics typically at smaller extents (1,000–10,000 km2) and at finer resolutions (typically up to 1ha) than SDMs (Shifley et al., 2017). These finer resolutions allow for the simulation of processes driving forest dynamics that act at small scales (Elkin et al., 2012; Albrich et al., 2020). They can simulate the impacts of a variety of anthropogenic and natural disturbances such as pest outbreaks, storms, and fire, on forest structures and dynamics at a landscape/watershed/management scale and long time frames, and do this within a gridded landscape in which each grid cell represents single trees (e.g. iLand) or aggregates of species-age cohorts and their biomass (e.g. LANDIS-II). They account for growth, competition, reproduction, dispersal and mortality of individual tree species, linked through ecological processes to changing environmental drivers, thus making them suitable to capture the influence of climate change. Additionally, they were built to scale up stand-level processes to the landscape-level (e.g., seed dispersal), to track biomass accumulation, decomposition and forest composition and structure and to simulate the effect of natural and anthropogenic disturbances and land use change. Resulting outputs from FLM simulations typically include, amongst others, proportions of specific tree species, proportions of dead wood, total standing biomass, and average age of the trees per grid cell. These outputs can thus be used to assess the likelihood the landscape is suitable for forest associated species. However, when used for assessments of ecosystem change impacts on biodiversity, FLMs have thus far either considered top-down impacts on biodiversity or have been limited to changes in the distribution of tree species (Sebald et al., 2021) or a limited number of wildlife species (Hof and Hjältén, 2018; Tremblay et al., 2018). Hof and Hjältén (2018) for instance used LANDIS-II to simulate the impacts of restoration on the suitability of a forest landscape for the white-backed woodpecker (Dendrocopos leucotos) by extracting data on dead wood, the age of forest stands, and the density of broadleaved trees directly from the simulation outputs. They however based their assessment of suitability on the presence of forest characteristics associated with white-backed woodpeckers, rather than on actual presence data of the species. This approach is therefore sensitive to bias. Other studies have used indicators for biodiversity (e.g., deadwood upon which many red-listed species depend) (Thom et al., 2017) and have thus neglected other crucial aspects related to forest biodiversity, such as biogeochemistry (Xiankai et al., 2008), the vertical structure of the forest (Storch et al., 2018), its spatial heterogeneity and the presence of large, old trees accommodating dendromicrohabitats (Asbeck et al., 2021a). Furthermore, assessments of impacts of perturbations on biodiversity more often than not focus on one driver of change at a time (Titeux et al., 2017, but see, e.g., Lanzas et al., 2021). Current efforts are thus few or somewhat limited, and usually focus on relatively small spatial extents considering the needs for effective conservation management. Yet, assessments of impacts of environmental changes, including aspects like biogeochemistry and natural disturbances, on biodiversity at large spatial scales are needed as they will provide better guidance for decision making by stakeholders regarding management and conservation.
Both SDM and FLM based studies are highly likely not spread evenly across the globe. A search in Scopus with the search string (TITLE-ABS-KEY(“species distribution model*”) OR TITLE-ABS-KEY(“environmental niche model*”)) resulted in 9925 hits of which, based on the top 10 countries, 41% was tagged as being or originating from a country in Europe, 35% from Northern America, 10% from China, 9% from Australia, and 6% from Brazil. Regarding FLMs, using the search string (TITLE-ABS-KEY (“Forest landscape model*”) OR TITLE-ABS-KEY (“Forest landscape simulation model*”)) returned 246 documents from, again based on the top 10, Northern America (56%), Europe (22%), China (20%), and Japan (2%). Although this does not mean that these studies were conducted in study regions in the countries specified, there is a likelihood that the majority in fact is, suggesting a bias towards certain areas. Unfortunately, seeing the large variability in landscapes, regarding biotic, abiotic, and cultural factors, it is impossible to generalize findings from one area to others.
In brief, applying individually SDMs and FLMs is subject to the three aforementioned limitations: 1. SDM based projections of species distributions are generally done at coarse spatial resolutions, 2. FLM based simulations are typically done for a limited number of species, indicators, or drivers of change and focus on relatively small areas, and 3. efforts are not spread evenly across the globe. These limitations are currently hampering trustworthy projections of impacts of climate change and management practices on forest biodiversity at a scale relevant for biodiversity conservation. Furthermore, the large number of feedbacks between biodiversity, forest, and ecosystem processes unfortunately cannot completely be captured by either SDMs or FLMs alone. Assessments using SDMs or FLMs on their own largely exclude broader complexities associated with climate-induced impacts on biodiversity at a scale relevant for biodiversity conservation. Tehrani et al. (2021) used for instance SDMs in which they used several climatic and land cover (e.g. forest types) variables as predictors, but unfortunately not in a dynamic manner. Albeit a nice approach, such efforts fail to take the changeability of forests into account.
The first step to get a better understanding of the impacts of forest management in the face of climate change on species’ presence may well be to integrate correlative SDMs with process based FLMs. Integrated modelling frameworks in which two or more models are linked with one another have been used to assess the combined effects of changes in e.g. landcover, climate, and natural disturbance regimes on the distribution of species. Pearson et al. (2004) for instance used a multiscale hierarchical modelling framework in which land-cover data was integrated in a correlative bioclimatic model in a scale-dependent hierarchical manner, whereby an SDM was used to obtain the climatic requirements of species at a continental scale and land-cover requirements at a nationwide scale. This hierarchical approach was also followed by others for different purposes, e.g., by Regos et al. (2016, 2018) to predict the impacts of climate change scenarios as well as fire regimes on the effectiveness of protected areas to conserve bird species, predominantly in grassland areas. Pais et al. (2020) coupled a fire-landscape dynamic model with a carbon sequestration model and an SDM to identify fire-smart management strategies in a mountain farmland area that promoted amongst others biodiversity conservation. Yet, the used resolution for (parts of) these frameworks was still rather coarse (1km2). FLMs are particularly suitable to simulate the dynamics of forest landscapes using fine resolutions (1ha and smaller). Linking correlative SDMs with process based FLMs therefore seems a promising approach to project impacts of changing conditions on forest biodiversity at fine resolutions and large scales. Instead of directly extracting relevant habitat suitability data from FLM simulation outputs as has been done by Hof and Hjältén (2018), FLMs are linked to SDMs by using the FLM simulation outputs in SDMs as predictor variables to evaluate the suitability of a given area for a specific species or species group based on actual species occurrences. A flowchart of an example of an integrated framework, based on previous efforts by Pearson et al. (2004) and Regos et al. (2020), in which an FLM is coupled with an SDM is given in Figure 1. This example framework consists of three steps in which climate, biophysical, and forest characteristic variables are integrated at different scales. Step one is to use regional-scale, coarse-resolution species occurrence data and climate change scenarios to derive climate suitability maps for the target species. Step two is to derive forest characteristic maps for scenarios of e.g. climate change, natural disturbances, and forest management scenarios from a landscape scale FLM, using forest inventory and remotely sensed data at fine resolutions. Finally, step three is to use fine-resolution species occurrence data together with the outcomes of the regional-scale SDM and the landscape-scale FLM as predictors in the final set of landscape-scale, fine-resolution SDMs.
Figure 1. Flowchart of an integrated framework in which a dynamic forest landscape model (FLM) is coupled with a species distribution model (SDM).
Such type of approach has been used but, to the best of our knowledge, only a handful of times. A search in Scopus using the search string TITLE-ABS-KEY ((“forest landscape model*” OR “forest dynamic model*” OR “forest simulation model*” OR “forest succession model*”) AND (“species distribution model*” OR “ecological niche model*” OR “habitat suitability model*”)) resulted in only 8 documents (May 2023). In Web of Science the search string (“forest landscape model*” OR “forest dynamic model*” OR “forest simulation model*” OR “forest succession model*”) AND (“species distribution model*” OR “ecological niche model*” OR “habitat suitability model*”) (topic) resulted in 7 hits (February 2024). A closer analysis of the hits revealed that not all works actually integrated SDM type models with FLM type models (Dijak and Rittenhouse, 2009; Huang et al., 2018; Tremblay et al., 2018; Wang et al., 2018; García-Valdés and Morales-Castilla, 2016) and those that did, generally focused on relatively small study areas and/or on a restricted number of taxa (Di Febbraro et al., 2015 [4 species], Hoecker and Turner, 2022 [3 species], Walsh and Hudiburg, 2019 [2 species]), or used large resolutions (García-Valdés et al., 2020 [10 arcminutes]). Yet, other works that did use such an approach did not come up in our search strings. Larson et al. (2004) linked a population viability model for one bird species to landscape simulations from a habitat suitability index model. Pearman-Gillman et al. (2020) used relatively coarse scale (500m2 to 3,000m2) simulation outputs of an FLM as predictor variables in SDMs to assess the habitat suitability for 10 species. Although we may have missed more works, applying such frameworks at large, yet detailed (1ha), scales for many species may thus have not been done so far. This is likely due to the increased complexity that often comes with increased accuracy.
The current limitation for biogeographers and macro-ecologists to use such an approach is most likely the scale at which the fine resolution FLMs operate. For example, Suárez-Muñoz et al. (2021) simulated a landscape of 3,900 km2 with a 1ha resolution, Gustafson et al. (2022) simulated landscapes of respectively 530 km2 and 640 km2 at a 30m resolution, and Duveneck et al. (2015) simulated 13M ha, but at a 6ha resolution. Simulating such large areas with FLMs as has been done by Duveneck et al. (2015) at fine resolutions still appears to be challenging. Here we discuss the major challenges that need to be overcome in order to use such integrated frameworks at larger spatial scales often relevant for biodiversity conservation, and at finer resolutions based on literature and our, users of FLMs and SDMs, own perspective, them being: 1) data availability for initialization and parameterization is limited, 2) regions comprise multiple landscapes, 3) inferences cannot be made, 4) availability of accurate species occurrence and response data, and 5) uncertainty of predictive performance of models. Tackling these challenges should be a priority if we are to better understand drivers of species distributions and effectively design conservation strategies, especially for species with large geographic ranges.
Challenges related to the use of integrated frameworks in which FLMs are linked with SDMs at regional scales are first and for all related to data availability. Albeit less than Individual-based or Stand-based Forest Models, the parameterization and initialization of FLMs still require large amounts of data, which is a general limitation of high-resolution forest models (McKenzie et al., 2019; Maréchaux et al., 2021). The first challenge is that FLMs require maps representing initial landscapes, observed or expected climate, site conditions (e.g., soil characteristics) and forest structure (e.g., species composition, cohort biomass or cohort ages) which are not always available “wall to wall” at fine scales. Even though satellite remote sensing technologies are constantly increasing their capabilities to deliver such data (Shugart et al., 2015), additional challenges (e.g. related to imagery correction, registration, and interpretation, and uncertainties related to mapping; Mairota et al., 2015) are associated to their judicious use. For instance, an FLM, such as LANDIS-II, coupled with a mechanistic extension such as PnET-Succession (De Bruijn et al., 2014), requires large amounts of landscape and species-specific parameters (e.g., data on soils, biogeochemistry, growth parameters, and tree species-specific life traits). Default input parameters are typically available for some of these. However, the majority requires calibrations based on observed landscape changes and compositional trajectories, whose measurements are among the largest challenges in modelling the impacts of environmental change (Keane et al., 2015; Scheller, 2018). Suárez-Muñoz et al. (2021) give an overview of the needed data, and they also provide a step-by-step guide to initialize and calibrate dynamic FLMs. Based on personal experiences, collecting the needed data, initializing and calibrating a dynamic FLM can easily take up to a year of full time work, a sentiment that is echoed by Furniss et al. (2022).
A major specific challenge of using FLMs at large extents and fine resolution, is that large regions that include multiple landscapes (e.g. the Mediterranean biogeographic region) will require different sets of parameters and calibration runs for each landscape. This is first computationally very demanding (Maréchaux et al., 2021) and second, not easily solved by mosaication, i.e., by modelling each landscape separately and then aggregating the several results ex post, a process that increases uncertainty (Boulanger and Pascual Puigdevall, 2021). The main problem is that species parameters expressing tree species-specific life traits are not rigidly separated across landscapes but are changing gradually following environmental gradients (Gutiérrez et al., 2016), and can also vary over time adding further uncertainties. The environmental drivers of parameter values are rarely well understood, let alone implemented. Alternatively, several representative landscapes per region could be modelled (i.e. parameterized and calibrated) so that each set of landscape/region specific parameters could be used for similar conditions with relative confidence.
Yet another challenge is that inferences from one study landscape often cannot be made to another landscape (Walsh and Hudiburg, 2019; Charney et al., 2021; Maréchaux et al., 2021) and that locally sampled information, such as establishment probabilities of tree species and amount of dead wood, cannot always be used for projections elsewhere. FLM outputs can be sensitive to local and global derived species parameters (Huber et al., 2018; McKenzie et al., 2019), thus leading to uncertainty of responses in the study landscape itself, hampering transferability. Landscapes that are already parameterized are often used for several studies and are thus assumed to function as representative landscapes for larger regions, even if in reality they are not, leading to flawed inferences. The reason that parameterized landscapes are used as representatives follows from the large amount of time it takes to collect the needed data and initialize and calibrate an FLM (McKenzie et al., 2019). But the selection criteria behind the choice of the specific landscape is rarely representativeness for other regions, being more often a need to capture specific processes and unique landscapes, such as protected areas or no management areas, or address specific scientific or environmental objectives targeted at that landscape. Increasing the size of a parameterized landscape requires additional data, computation time and storage space. Yet, inferences from outcomes of, e.g., the effects of anthropogenic or natural disturbances and other perturbations on a particular ecosystem or specific species may not be valid across time and space (Johnstone et al., 2016). A major flaw, dubbed the Modifiable Areal Unit Problem (Openshaw, 1984), may already arise due to data aggregation, in which the area-specific data are aggregated into larger area units or recombined into zones with the same size but at a different location. In turn, each combination leads to different values, different conclusions and possibly flawed inferences (Jelinski and Wu, 1996). Furthermore, inferences may be faulty due to the large range of different effects of disturbances, the large range of management strategies and the variation in plant and animal species responses to disturbances and perturbations (Thompson et al., 2000). In addition, there may be confounding factors, such as landscape scale effects.
Yet another challenge is related to the quality of species occurrence and response data. When high resolution FLMs are to be linked with SDMs, accurate species occurrence data with a high resolution are needed. Many studies applying SDMs use data (partly) collected by citizens, such as those made available by the Global Biodiversity Information Facility (www.GBIF.org) (Feldman et al., 2021). Such data can be fraught of bias, caused by e.g. differences in accessibility to surveyors and mis-identification (Dickinson et al., 2010; Kosmala et al., 2016), for which statistical approaches may need to be taken to deal with them (Bird et al., 2014). Nevertheless, citizen science data can make a valuable contribution to species conservation and provide reliable predictions (Tiago et al., 2017; Van Eupen et al., 2021). The main challenge we see with using citizen science data in integrated frameworks is that they are unfortunately often provided with a low resolution.
In addition to species occurrence data – available for many species across large parts of the globe, yet important gaps exist (Feldman et al., 2021) – species requirements and response data to specific disturbances are needed as well. The increasing source of small-scale data from biodiversity monitoring programs can potentially be used for such purposes. New and ongoing studies that assess the impact of forest management and natural disturbance regimes on forest biodiversity offer a unique and rich source of information that can be used for integrated frameworks (e.g., Müller et al., 2019; Koivula and Vanha-Majamaa, 2020; Asbeck et al., 2021b). These studies often track biodiversity or ecosystem responses across time scales pertinent for simulation modelling and have significantly advanced our understanding of how plants and animals may respond to, e.g., changes in forest structure, climate, and disturbance dynamics. However, inferences from such studies are often restricted to very small spatial scales, viz. within or between stands. Integrated frameworks, such as presented in Figure 1, in which FLMs are linked with SDMs can “scale-up” such high-resolution biodiversity data obtained from field experiments, long-term monitoring programs, national inventories, published literature or remote-sensing to the spatial scale that is relevant for biodiversity conservation, such as large landscapes/regions. It is however key that the landscape-scale SDMs mentioned in step 3 in the framework should be fit at high resolutions. Coarse resolution SDMs are not able to capture the fine-scale variations in suitability of the area for the species under consideration resulted from fine-scale variations in resources and microclimates (Bütikofer et al., 2020; Maclean and Early, 2023). We therefore advocate that more biodiversity monitoring programs, especially focused on assessing impacts of perturbations – be it forest management or conservation strategies or natural disturbances – are needed.
It is important to realise that although SDMs can make useful predictions of e.g., impacts of climate change on species distribution ranges and FLMs can make useful simulations of such impacts on forest ecosystems, the predictions and simulations need not necessarily be of high quality (Petter et al., 2020; Wang and Jackson, 2023). This is for instance an issue when imprecise occurrence data are used for high resolution predictions in SDMs (Mitchell et al., 2017; Gábor et al., 2020). Unfortunately there is, more often than not, no meaningful way to assess the quality of models, especially when used to predict into future. There may be further need to explore the predictive power of SDMs and accuracy of FLMs under different climate scenarios. Hindcasting, using historical records, or using virtual species to assess the predictive performance of SDM predictions may be the only way, as has been done by e.g. Morán-Ordóñez et al. (2017) and Santini et al. (2021). Hindcasting has also been suggested as an option to validate the internal processes of FLMs (Scheller, 2018). Alternatively, multiple variants of a model can be developed to evaluate structural uncertainties in the models (Huber et al., 2020). Outcomes from such exercises may however be even more challenging to transfer and communicate to forest managers.
A majority of scientists and conservation managers may currently not be able to dedicate sufficient resources, including computing power, to build integrated modelling frameworks at large spatial scales and fine resolutions. As mentioned, our proposed framework (Figure 1) consists of three steps. First, regional-scale, low resolution species occurrence data and climate change scenarios are used in SDMs to derive low-resolution climate-suitability maps for targeted species. These low-resolution maps subsequently need to be resampled to the needed resolution in the final step. In the second step, landscape scale FLMs are used to produce high-resolution forest characteristic maps for scenarios of interest, using high-resolution forest inventory and remotely sensed data. Finally, step three is to use high-resolution species occurrence data together with the outcomes of the regional-scale SDMs and the landscape-scale FLMs as predictors in the final set of landscape-scale SDMs to obtain high-resolution maps of habitat suitability for targeted species under scenarios of interest (e.g. various scenarios of climate change and forest management). Although this exact approach has, to our knowledge, not been used before, similar approaches, albeit at smaller scales or at lower resolutions, have been used by, amongst others, Pearson et al. (2004), Di Febbraro et al. (2015), Walsh and Hudiburg (2019), Pearman-Gillman et al. (2020), Regos et al. (2020), and Hoecker and Turner (2022). Clearly, to use such an approach at the scales needed for effective conservation and at high resolutions, we need reasonable short-cuts. Identifying the problems related with the use of modelling outputs to infer responses of one specific study region through space and time, the best way forward in the foreseeable future may be to set up a range of representative landscapes for parameterisation for FLMs, covering a large range of biogeographical regions or ecotones. This could also help to identify gradients of parameters that could be used for calculating parameters in other areas based on available environmental variables. This approach should lower the problems related with faulty inferences through space. For the integration with SDMs, target species for biodiversity conservation will likely differ per region, ecosystem, and the interests of stakeholders. In addition, data regarding species requirements should be available, which is taxa dependent. For generality, it may be a good idea to target either keystone, indicator, or umbrella species or functional groups (see e.g., Walsh and Hudiburg, 2019). However, among such (groups of) species, further selection should be made as resolution of the integrated frameworks and the target species’ requirements need to be consistent with one another. One of the main problems in landscape ecology is to define resolutions (extent-grain) relevant to the perception limits of the organisms under investigation (Wiens, 1989; Kotliar and Wiens, 1990).
Ideally, integrated frameworks should also include social values placed on biodiversity, as different management objectives might need to be prioritized over a landscape, as for instance attempted by Lucet and Gonzalez (2022). Current standard processes of involving societal stakeholders and their values are iterative (e.g., Miller and Morisette, 2014; Murgue et al., 2015): model projections are shared with selected stakeholders who may rate or rank their preferred landscapes for desirable futures. Iterations help identify stakeholders’ common interests and conflicts. One should however be aware that correctly reporting results from modelling efforts to stakeholders can be challenging, particularly those relating to uncertainty around average model results (Petr et al., 2019). Incorporating social values is also central to assessing unavoidable trade-offs and capture uncertainty in biodiversity conservation (Palacios-Agundez et al., 2015; Lischka et al., 2018). Efforts have already emerged within the area of “dynamic integrated socio-environmental systems” to capture the coupled role of human actions and ecosystems dynamics (Liu et al., 2007; Aguilar and Kelly, 2019). There is a need of integrated models to be tailored to particular human and ecological conditions. Determining the “right” socio-ecological spatial scale might be a central question to such integrated frameworks.
In conclusion, we advocate for the development and application of more – and especially larger scale and finer resolution – efforts to integrate FLMs with SDMs, making use of the existing frameworks (e.g., workflows for data acquisition and preparation), parameterised landscapes which are representative for biogeographical regions, as well as the increasingly rich sources of data on environmental factors and species presence, and on species responses to environmental changes. We should however continue placing a great focus on fine resolution – landscape-scale impact assessments and data collection. These efforts should be a priority if the goal is to significantly improve our understanding of the drivers of species distributions in forest ecosystems. In turn, such knowledge would allow us to more effectively design conservation strategies, especially for species with large geographic ranges.
The original contributions presented in the study are included in the article/supplementary material. Further inquiries can be directed to the corresponding author.
AH: Conceptualization, Writing – original draft, Writing – review & editing. MM: Conceptualization, Writing – original draft, Writing – review & editing. PM: Conceptualization, Writing – original draft, Writing – review & editing. FA: Writing – review & editing. GL: Writing – review & editing. JB: Writing – review & editing. MKo: Writing – review & editing. MKl: Writing – review & editing. JS: Writing – review & editing. GV: Writing – review & editing.
The author(s) declare financial support was received for the research, authorship, and/or publication of this article. JB was supported by the long-term research development project no. RVO 67985939 from the Czech Academy of Sciences. MM acknowledges funding from the H2020-MSCA-IF project REINFORCE (Grant No. 891671). MKl was supported by the Slovenian Research and Innovation Agency (ARIS) via the research core funding P4-0059 “Forest, forestry and renewable forest resources”.
We thank two anonymous reviewers for valuable comments on an earlier draft.
The authors declare that the research was conducted in the absence of any commercial or financial relationships that could be construed as a potential conflict of interest.
All claims expressed in this article are solely those of the authors and do not necessarily represent those of their affiliated organizations, or those of the publisher, the editors and the reviewers. Any product that may be evaluated in this article, or claim that may be made by its manufacturer, is not guaranteed or endorsed by the publisher.
Aguilar F. X., Kelly M. C. (2019). US family forest management coupling natural and human systems: Role of markets and public policy instruments. Landscape Urban Plann. 188, 43–53. doi: 10.1016/j.landurbplan.2019.01.004
Albrich K., Rammer W., Turner M. G., Ratajczak Z., Braziunas K. H., Hansen W. D., et al. (2020). Simulating forest resilience: A review. Global Ecol. Biogeography 29, 2082–2096. doi: 10.1111/geb.13197
Asbeck T., Großmann J., Paillet Y., Winiger N., Bauhus J. (2021a). The use of tree-related microhabitats as forest biodiversity indicators and to guide integrated forest management. Curr. Forestry Rep. 7, 59–68. doi: 10.1007/s40725-020-00132-5
Asbeck T., Sabatini F., Augustynczik A. L., Basile M., Helbach J., Jonker M., et al. (2021b). Biodiversity response to forest management intensity, carbon stocks and net primary production in temperate montane forests. Sci. Rep. 11, 1–11. doi: 10.1038/s41598-020-80499-4
Bird T. J., Bates A. E., Lefcheck J. S., Hill N. A., Thomson R. J., Edgar G. J., et al. (2014). Statistical solutions for error and bias in global citizen science datasets. Biol. Conserv. 173, 144–154. doi: 10.1016/j.biocon.2013.07.037
Boulanger Y., Pascual Puigdevall J. (2021). Boreal forests will be more severely affected by projected anthropogenic climate forcing than mixedwood and northern hardwood forests in eastern Canada. Landscape Ecol. 36, 1725–1740. doi: 10.1007/s10531-017-1453-2
Brito D., Grelle C. E. V. (2006). Estimating minimum area of suitable habitat and viable population size for the northern muriqui (Brachyteles hypoxanthus). Biodiversity Conserv. 15, 4197–4210. doi: 10.1007/s10531-005-3575-1
Brockerhoff E. J., Barbaro L., Castagneyrol B., Forrester D. I., Gardiner B., González-Olabarrie J. R. (2017). Forest biodiversity, ecosystem functioning and the provision of ecosystem services. Biodiversity and Conservation 26, 3005–3035. doi: 10.1007/s10531-017-1453-2
Bütikofer L., Anderson K., Bebber D. P., Bennie J. J., Early R. I., Maclean I. M. D., et al. (2020). The problem of scale in predicting biological responses to climate. Global Change Biol. 26, 6657–6666. doi: 10.1111/gcb.15358
Charney N. D., Record S., Gerstner B. E., Merow C., Zarnetske P. L., Enquist B. J. (2021). A test of species distribution model transferability across environmental and geographic space for 108 western North American tree species. Front. Ecol. Evol. 393. doi: 10.3389/fevo.2021.689295
De Bruijn A., Gustafson E. J., Sturtevant B. R., Foster J. R., Miranda B. R., Lichti N. I., et al. (2014). Toward more robust projections of forest landscape dynamics under novel environmental conditions: embedding PnET within LANDIS-II. Ecol. Model. 287, 44–57. doi: 10.1016/j.ecolmodel.2014.05.004
Dickinson J. L., Zuckerberg B., Bonter D. N. (2010). Citizen science as an ecological research tool: challenges and benefits. Annu. Rev. ecology evolution systematics 41, 149–172. doi: 10.1146/annurev-ecolsys-102209-144636
Di Febbraro M., Roscioni F., Frate L., Carranza M. L., De Lisio L., De Rosa D., et al. (2015). Long-term effects of traditional and conservation-oriented forest management on the distribution of vertebrates in Mediterranean forests: a hierarchical hybrid modelling approach. Diversity Distributions 21, 1141–1154. doi: 10.1111/ddi.12362
Dijak W. D., Rittenhouse C. D. (2009). “Development and application of habitat suitability models to large landscapes,” in Models for Planning Wildlife Conservation in Large Landscapes. Eds. Millspaugh J. J., Thompson F. R. (Burlington, MA, USA: Academic Press, Elsevier), 367–389.
Duveneck M. J., Thompson J. R., Wilson B. T. (2015). An imputed forest composition map for New England screened by species range boundaries. For. Ecol. Manage. 347, 107–115. doi: 10.1016/j.foreco.2015.03.016
Elkin C., Reineking B., Bigler C., Bugmann H. (2012). Do small-grain processes matter for landscape scale questions? Sensitivity of a forest landscape model to the formulation of tree growth rate. Landscape Ecol. 27, 697–711. doi: 10.1007/s10980-012-9718-3
Feldman M. J., Imbeau L., Marchand P., Mazerolle M. J., Darveau M., Fenton N. J. (2021). Trends and gaps in the use of citizen science derived data as input for species distribution models: A quantitative review. PLoS One 16, e0234587. doi: 10.1371/journal.pone.0234587
Furniss T. J., Hessburg P. F., Povak N. A., Salter R. B., Wigmosta M. S. (2022). Predicting future patterns, processes, and their interactions: Benchmark calibration and validation procedures for forest landscape models. Ecol. Model. 473, 110099. doi: 10.1016/j.ecolmodel.2022.110099
Gábor L., Moudrý V., Lecours V., Malavasi M., Barták V., Fogl M., et al. (2020). The effect of positional error on fine scale species distribution models increases for specialist species. Ecography 43, 256–269. doi: 10.1111/ecog.04687
García-Valdés R., Estrada A., Early R., Lehsten V., Morin X. (2020). Climate change impacts on long-term forest productivity might be driven by species turnover rather than by changes in tree growth. Global Ecol. Biogeography 29, 1360–1372. doi: 10.1111/geb.13112
García-Valdés R., Morales-Castilla I. (2016). Effects of climate change on forest ecosystems: integrating inventories and models. Ecosistemas 25, 51–59. doi: 10.7818/ECOS
Gustafson E. J., Miranda B. R., Dreaden T. J., Pinchot C. C., Jacobs D. F. (2022). Beyond blight: Phytophthora root rot under climate change limits populations of reintroduced American chestnut. Ecosphere 13, e3917. doi: 10.1002/ecs2.3917
Gutiérrez A. G., Snell R. S., Bugmann H. (2016). Using a dynamic forest model to predict tree species distributions. Global Ecol. Biogeography 25, 347–358. doi: 10.1111/geb.12421
Hoecker T. J., Turner M. G. (2022). Combined effects of climate and fire-driven vegetation change constrain the distributions of forest vertebrates during the 21st century. Diversity Distributions 28, 727–744. doi: 10.1111/ddi.13470
Hof A. R., Hjältén J. (2018). Are we restoring enough? Simulating impacts of restoration efforts on the suitability of forest landscapes for a locally critically endangered umbrella species. Restor. Ecol. 26, 740–750. doi: 10.1111/rec.12628
Howard C., Marjakangas E. L., Morán-Ordóñez A., Milanesi P., Abuladze A., Aghababyan K., et al. (2023). Local colonisations and extinctions of European birds are poorly explained by changes in climate suitability. Nat. Commun. 14, 4304. doi: 10.1038/s41467-023-39093-1
Huang S., Ramirez C., McElhaney M., Evans K. (2018). F3: Simulating spatiotemporal forest change from field inventory, remote sensing, growth modeling, and management actions. For. Ecol. Manage. 415, 26–37. doi: 10.1016/j.foreco.2018.02.026
Huber N., Bugmann H., Lafond V. (2018). Global sensitivity analysis of a dynamic vegetation model: Model sensitivity depends on successional time, climate and competitive interactions. Ecol. Model. 368, 377–390. doi: 10.1016/j.ecolmodel.2017.12.013
Huber N., Bugmann H., Lafond V. (2020). Capturing ecological processes in dynamic forest models: why there is no silver bullet to cope with complexity. Ecosphere 11, e03109. doi: 10.1002/ecs2.3109
IPBES (2019). Global assessment report on biodiversity and ecosystem services of the Intergovernmental Science-Policy Platform on Biodiversity and Ecosystem Services. Brondizio E. S., Settele J., Díaz S., Ngo H. T. (editors) . (Bonn, Germany: IPBES secretariat). 1148. doi: 10.5281/zenodo.3831673
Jelinski D. E., Wu J. (1996). The modifiable areal unit problem and implications for landscape ecology. Landscape Ecol. 11, 129–140. doi: 10.1007/BF02447512
Johnstone J. F., Allen C. D., Franklin J. F., Frelich L. E., Harvey B. J., Higuera P. E., et al. (2016). Changing disturbance regimes, ecological memory, and forest resilience. Front. Ecol. Environ. 14, 369–378. doi: 10.1002/fee.1311
Kark S., Tulloch A., Gordon A., Mazor T., Bunnefeld N., Levin N. (2015). Cross-boundary collaboration: key to the conservation puzzle. Curr. Opin. Environ. Sustainability 12, 12–24. doi: 10.1016/j.cosust.2014.08.005
Keane R. E., McKenzie D., Falk D. A., Smithwick E. A., Miller C., Kellogg L. K. B. (2015). Representing climate, disturbance, and vegetation interactions in landscape models. Ecol. Model. 309, 33–47. doi: 10.1016/j.ecolmodel.2015.04.009
Kearney M., Porter W. (2009). Mechanistic niche modelling: combining physiological and spatial data to predict species’ ranges. Ecol. Lett. 12, 334–350. doi: 10.1111/j.1461-0248.2008.01277.x
Koivula M., Vanha-Majamaa I. (2020). Experimental evidence on biodiversity impacts of variable retention forestry, prescribed burning, and deadwood manipulation in Fennoscandia. Ecol. Processes 9, 11. doi: 10.1186/s13717-019-0209-1
Kosmala M., Wiggins A., Swanson A., Simmons B. (2016). Assessing data quality in citizen science. Front. Ecol. Environ. 14, 551–560. doi: 10.1002/fee.1436
Kotliar N. B., Wiens J. A. (1990). Multiple scales of patchiness and patch structure: a hierarchical framework for the study of heterogeneity. Oikos 59, 253–260. doi: 10.2307/3545542
Krinner G., Viovy N., de Noblet-Ducoudré N., Ogée J., Polcher J., Friedlingstein P., et al. (2005). A dynamic global vegetation model for studies of the coupled atmosphere-biosphere system. Global Biogeochemical Cycles 19, 1–33. doi: 10.1029/2003GB002199
Lanzas M., Hermoso V., Morán-Ordoñez A., Regos A., Bota G., Brotons L. (2021). The value of unprotected land for future conservation efforts under dynamic conditions. Biol. Conserv. 261, 109232. doi: 10.1016/j.biocon.2021.109232
Larson M. A., Thompson F. R. III, Millspaugh J. J., Dijak W. D., Shifley S. R. (2004). Linking population viability, habitat suitability, and landscape simulation models for conservation planning. Ecol. Model. 180, 103–118. doi: 10.1016/j.ecolmodel.2003.12.054
Lischka S. A., Teel T. L., Johnson H. E., Reed S. E., Breck S., Carlos A. D., et al. (2018). A conceptual model for the integration of social and ecological information to understand human-wildlife interactions. Biol. Conserv. 225, 80–87. doi: 10.1016/j.biocon.2018.06.020
Liu J., Dietz T., Carpenter S. R., Alberti M., Folke C., Moran E., et al. (2007). Complexity of coupled human and natural systems. science 317, 1513–1516. doi: 10.1126/science.1144004
Lucet V., Gonzalez A. (2022). Integrating land use and climate change models with stakeholder priorities to evaluate habitat connectivity change: a case study in southern Québec. Landscape Ecol. 37, 2895–2913. doi: 10.1007/s10980-022-01516-7
Mairota P., Cafarelli B., Didham R. K., Lovergine F. P., Lucas R. M., Nagendra H., et al. (2015). Challenges and opportunities in harnessing satellite remote-sensing for biodiversity monitoring. Ecol. Inf. 30, 207–214. doi: 10.1016/j.ecoinf.2015.08.006
Maréchaux I., Langerwisch F., Huth A., Bugmann H., Morin X., Reyer C. P. (2021). Tackling unresolved questions in forest ecology: The past and future role of simulation models. Ecology and Evolution 11 (9), 3746–3770
Maclean I. M., Early R. (2023). Macroclimate data overestimate range shifts of plants in response to climate change. Nature Climate Change, 1–7.
McKenzie P. F., Duveneck M. J., Morreale L. L., Thompson J. R. (2019). Local and global parameter sensitivity within an ecophysiologically based forest landscape model. Environ. Model. Software 117, 1–13. doi: 10.1016/j.envsoft.2019.03.002
Miller B. W., Morisette J. T. (2014). Integrating research tools to support the management of social-ecological systems under climate change. Ecol. Soc. 19. doi: 10.5751/ES-06813-190341
Mitchell P. J., Monk J., Laurenson L. (2017). Sensitivity of fine-scale species distribution models to locational uncertainty in occurrence data across multiple sample sizes. Methods Ecol. Evol. 8, 12–21. doi: 10.1111/2041-210X.12645
Morán-Ordóñez A., Lahoz-Monfort J. J., Elith J., Wintle B. A. (2017). Evaluating 318 continental-scale species distribution models over a 60-year prediction horizon: What factors influence the reliability of predictions? Global Ecol. Biogeography 26, 371–384. doi: 10.1111/geb.12545
Müller J., Boch S., Prati D., Socher S. A., Pommer U., Hessenmöller D., et al. (2019). Effects of forest management on bryophyte species richness in Central European forests. For. Ecol. Manage. 432, 850–859. doi: 10.1016/j.foreco.2018.10.019
Murgue C., Therond O., Leenhardt D. (2015). Toward integrated water and agricultural land management: Participatory design of agricultural landscapes. Land Use Policy 45, 52–63. doi: 10.1016/j.landusepol.2015.01.011
Openshaw S. (1984). “The modifiable areal unit problem.” Geo Books (Norwich, England). Available online at: https://www.uio.no/studier/emner/sv/iss/SGO9010/openshaw1983.pdf.
Pais S., Aquilué N., Campos J., Sil Â., Marcos B., Martínez-Freiría F., et al. (2020). Mountain farmland protection and fire-smart management jointly reduce fire hazard and enhance biodiversity and carbon sequestration. Ecosystem Serv. 44, 101143. doi: 10.1016/j.ecoser.2020.101143
Palacios-Agundez I., OnaIndia M., Barraqueta P., Madariaga I. (2015). Provisioning ecosystem services supply and demand: The role of landscape management to reinforce supply and promote synergies with other ecosystem services. Land Use Policy 47, 145–155. doi: 10.1016/j.landusepol.2015.03.012
Pearman-Gillman S. B., Duveneck M. J., Murdoch J. D., Donovan T. M. (2020). Drivers and consequences of alternative landscape futures on wildlife distributions in New England, United States. Front. Ecol. Evol. 8, 164. doi: 10.3389/fevo.2020.00164
Pearson R. G., Dawson T. P., Liu C. (2004). Modelling species distributions in Britain: a hierarchical integration of climate and land-cover data. Ecography 27, 285–298. doi: 10.1111/j.0906-7590.2004.03740.x
Petr M., Vacchiano G., Thom D., Mairota P., Kautz M., Goncalves L. M. D. S., et al. (2019). Inconsistent recognition of uncertainty in studies of climate change impacts on forests. Environ. Res. Lett. 14, 113003. doi: 10.1088/1748-9326/ab4670
Petter G., Mairota P., Albrich K., Bebi P., Brůna J., Bugmann H., et al. (2020). How robust are future projections of forest landscape dynamics? Insights from a systematic comparison of four forest landscape models. Environ. Model. Software 134, 104844. doi: 10.1016/j.envsoft.2020.104844
Phillips S. J., Anderson R. P., Schapire R. E. (2006). Maximum entropy modeling of species geographic distributions. Ecol. Model. 190, 231–259. doi: 10.1016/j.ecolmodel.2005.03.026
Regos A., D'Amen M., Titeux N., Herrando S., Guisan A., Brotons L. (2016). Predicting the future effectiveness of protected areas for bird conservation in Mediterranean ecosystems under climate change and novel fire regime scenarios. Diversity Distributions 22, 83–96. doi: 10.1111/ddi.12375
Regos A., Hermoso V., D'Amen M., Guisan A., Brotons L. (2018). Trade-offs and synergies between bird conservation and wildfire suppression in the face of global change. J. Appl. Ecol. 55, 2181–2192. doi: 10.1111/1365-2664.13182
Regos A., Vidal M., Lorenzo M., Domínguez J. (2020). Integrating intraseasonal grassland dynamics in cross-scale distribution modeling to support waterbird recovery plans. Conserv. Biol. 34, 494–504. doi: 10.1111/cobi.13415
Santini L., Benítez-López A., Maiorano L., Čengić M., Huijbregts M. A. (2021). Assessing the reliability of species distribution projections in climate change research. Diversity Distributions 27, 1035–1050. doi: 10.1111/ddi.13252
Scheller R. M. (2018). The challenges of forest modeling given climate change. Landscape Ecol. 33, 1481–1488. doi: 10.1007/s10980-018-0689-x
Scheller R. M., Domingo J. B., Sturtevant B. R., Williams J. S., Rudy A., Gustafson E. J., et al. (2007). Design, development, and application of LANDIS-II, a spatial landscape simulation model with flexible temporal and spatial resolution. Ecol. Model. 201, 409–419. doi: 10.1016/j.ecolmodel.2006.10.009
Scheller R. M., Mladenoff D. J. (2007). An ecological classification of forest landscape simulation models: tools and strategies for understanding broad-scale forested ecosystems. Landscape Ecol. 22, 491–505. doi: 10.1007/s10980-006-9048-4
Sebald J., Thrippleton T., Rammer W., Bugmann H., Seidl R. (2021). Mixing tree species at different spatial scales: The effect of alpha, beta and gamma diversity on disturbance impacts under climate change. J. Appl. Ecol. 58, 1749–1763. doi: 10.1111/1365-2664.13912
Seidl R., Rammer W., Scheller R. M., Spies T. A. (2012). An individual based process model to simulate landscape-scale forest ecosystem dynamics. Ecol. Model. 231, 87–100. doi: 10.1016/j.ecolmodel.2012.02.015
Shifley S. R., He H. S., Lischke H., Wang W. J., Jin W., Gustafson E. J., et al. (2017). The past and future of modeling forest dynamics: from growth and yield curves to forest landscape models. Landscape Ecol. 32, 1307–1325. doi: 10.1007/s10980-017-0540-9
Shugart H. H., Asner G. P., Fischer R., Huth A., Knapp N., Le Toan T., et al. (2015). Computer and remote-sensing infrastructure to enhance large-scale testing of individual-based forest models. Front. Ecol. Environ. 13, 503–511. doi: 10.1890/140327
Sodhi N. S., Butler R., Laurance W. F., Gibson L. (2011). Conservation successes at micro-, meso-and macroscales. Trends Ecol. Evol. 26, 585–594. doi: 10.1016/j.tree.2011.07.002
Stanton J. C., Pearson R. G., Horning N., Ersts P., Reşit Akçakaya H. (2012). Combining static and dynamic variables in species distribution models under climate change. Methods Ecol. Evol. 3, 349–357. doi: 10.1111/j.2041-210X.2011.00157.x
Storch F., Dormann C. F., Bauhus J. (2018). Quantifying forest structural diversity based on large-scale inventory data: a new approach to support biodiversity monitoring. For. Ecosyst. 5, 34. doi: 10.1186/s40663-018-0151-1
Suárez-Muñoz M., Mina M., Salazar P. C., Navarro-Cerrillo R. M., Quero J. L., Bonet-García F. J. (2021). A step-by-step guide to initialize and calibrate landscape models: A case study in the Mediterranean Mountains. Front. Ecol. Evol. 9, 209. doi: 10.3389/fevo.2021.653393
Tiago P., Pereira H. M., Capinha C. (2017). Using citizen science data to estimate climatic niches and species distributions. Basic and Applied Ecology 20, 756–85.
Tehrani N. A., Naimi B., Jaboyedoff M. (2021). Modeling current and future species distribution of breeding birds as regional essential biodiversity variables (SD EBVs): A bird perspective in Swiss Alps. Global Ecol. Conserv. 27, e01596. doi: 10.1016/j.gecco.2021.e01596
Temperli C., Bugmann H., Elkin C. (2013). Cross-scale interactions among bark beetles, climate change, and wind disturbances: a landscape modeling approach. Ecol. Monogr. 83, 383–402. doi: 10.1890/12-1503.1
Thom D., Rammer W., Dirnböck T., Müller J., Kobler J., Katzensteiner K., et al. (2017). The impacts of climate change and disturbance on spatio-temporal trajectories of biodiversity in a temperate forest landscape. J. Appl. Ecol. 54, 28–38. doi: 10.1111/1365-2664.12644
Thompson F. R. III., Brawn J. D., Robinson S., Faaborg J., Clawson R. L. (2000). Approaches to investigate effects of forest management on birds in eastern deciduous forests: how reliable is our knowledge? Wildlife Soc. Bull. 28, 1111–1122. Available online at: https://www.jstor.org/stable/3783872.
Titeux N., Henle K., Mihoub J. B., Regos A., Geijzendorffer I. R., Cramer W., et al. (2016). Biodiversity scenarios neglect future land-use changes. Global Change Biol. 22, 2505–2515. doi: 10.1111/gcb.13272
Titeux N., Henle K., Mihoub J. B., Regos A., Geijzendorffer I. R., Cramer W., et al. (2017). Global scenarios for biodiversity need to better integrate climate and land use change. Diversity distributions 23, 1231–1234. doi: 10.1111/ddi.12624
Tremblay J. A., Boulanger Y., Cyr D., Taylor A. R., Price D. T., St-Laurent M. H. (2018). Harvesting interacts with climate change to affect future habitat quality of a focal species in eastern Canada’s boreal forest. PLoS One 13, e0191645. doi: 10.1371/journal.pone.0191645
Urban M. C., Bocedi G., Hendry A. P., Mihoub J. B., Pe’er G., Singer A., et al. (2016). Improving the forecast for biodiversity under climate change. Science 353, aad8466. doi: 10.1126/science.aad8466
Van Eupen C., Maes D., Herremans M., Swinnen K. R., Somers B., Luca S. (2021). The impact of data quality filtering of opportunistic citizen science data on species distribution model performance. Ecol. Model. 444, 109453. doi: 10.1016/j.ecolmodel.2021.109453
Walsh E. S., Hudiburg T. (2019). An integration framework for linking avifauna niche and forest landscape models. PLoS One 14, e0217299. doi: 10.1371/journal.pone.0217299
Wang W. J., He H. S., Thompson F. R. III, Spetich M. A., Fraser J. S. (2018). Effects of species biological traits and environmental heterogeneity on simulated tree species distribution shifts under climate change. cience Total Environ. 634, 1214–1221. doi: 10.1016/j.scitotenv.2018.03.353
Wang L., Jackson D. A. (2023). Effects of sample size, data quality, and species response in environmental space on modeling species distributions. Landscape Ecol. 38, 4009–4031. doi: 10.1007/s10980-023-01771-2
Wu J., Qi Y. (2000). Dealing with scale in landscape analysis: an overview. Geographic Inf. Sci. 6, 1–5. doi: 10.1080/10824000009480528
Keywords: climate change, dynamic landscape modelling, species distribution modelling, species conservation, environmental niche models
Citation: Hof AR, Mina M, Mairota P, Aguilar F, Leitinger G, Brůna J, Koivula M, Klopčič M, Sjögren J and Vacchiano G (2024) A perspective on the need for integrated frameworks linking species distribution and dynamic forest landscape models across spatial scales. Front. Ecol. Evol. 12:1112712. doi: 10.3389/fevo.2024.1112712
Received: 30 November 2022; Accepted: 28 August 2024;
Published: 19 September 2024.
Edited by:
Peter Convey, British Antarctic Survey (BAS), United KingdomReviewed by:
Maurizio Marchi, National Research Council (CNR), ItalyCopyright © 2024 Hof, Mina, Mairota, Aguilar, Leitinger, Brůna, Koivula, Klopčič, Sjögren and Vacchiano. This is an open-access article distributed under the terms of the Creative Commons Attribution License (CC BY). The use, distribution or reproduction in other forums is permitted, provided the original author(s) and the copyright owner(s) are credited and that the original publication in this journal is cited, in accordance with accepted academic practice. No use, distribution or reproduction is permitted which does not comply with these terms.
*Correspondence: Anouschka R. Hof, QW5vdXNjaGthLkhvZkB3dXIubmw=
Disclaimer: All claims expressed in this article are solely those of the authors and do not necessarily represent those of their affiliated organizations, or those of the publisher, the editors and the reviewers. Any product that may be evaluated in this article or claim that may be made by its manufacturer is not guaranteed or endorsed by the publisher.
Research integrity at Frontiers
Learn more about the work of our research integrity team to safeguard the quality of each article we publish.