- 1School of Marxism, Hunan University of Science and Technology, Xiangtan, China
- 2School of Marxism, Hunan University of Finance and Economics, Changsha, China
- 3Economic College, Hunan Agricultural University, Changsha, China
Introduction: Protecting the ecosystem of the Dongting Lake area is of utmost importance for maintaining ecological balance and achieving human well-being.
Methods: This study identifies the key factors influencing the remote sensing based ecological index (RESI) in the Dongting Lake area based on the spatial and temporal evolution characteristics of the RESI and environmental changes and anthropogenic disturbance factors. The priority zoning for ecological restoration was delineated in combination with the anthropogenic composite index (ACI). By exploring the influence of anthropogenic disturbances on RESI, the zoning locations were determined using spatial statistics and linear regression methods.
Results: The overall RESI of the Dongting Lake area showed a decline from 2001 to 2020, with the mean value decreasing from 0.52 to 0.48. High-quality zones were mainly located in mountainous and forested areas, while low-quality zones were mainly distributed in more developed cities in the east urban area. Anthropogenic factors were the main reasons for the decline in the ecological environment, while natural factors showed a positive correlation with RESI. Based on the RESI and ACI, four ecological control zones (H-H, H-L, L-H, and L-L) were delineated, which accounted for a total of 45.66% of the Dongting Lake area. Among them, 3.91% required immediate control and management, while 17.80% required artificial maintenance. This study explores the influencing factors and mechanisms of the ecological environment quality in the Dongting Lake area, and explores the effective spatial paths for the implementation of ecological restoration zoning control and differentiated restoration strategies in the Dongting Lake area.
Discussion: This study provides a scientific basis for mitigating ecological and environmental problems in the Dongting Lake area, and provides a reference for ecological restoration and regulation and the realization of sustainable development goals in China and global regions with complex environmental problems.
1 Introduction
Environmental changes, such as precipitation and temperature, along with human disturbances, can lead to a range of ecological effects that impact ecological health (Su et al., 2010; He et al., 2019). In recent decades, the Dongting Lake area has faced numerous threats to its ecological quality due to intensive and continuous human activities, including lake encirclement, dam construction, urban expansion, agricultural surface pollution, and irrational exploitation. Of particular concern is the over-exploitation of resource elements (Yu et al., 2018; Zhang et al., 2018b). These activities have resulted in the decline of wetland area, deterioration of water quality, destruction of ecosystem structure and habitats, and loss of biodiversity, ultimately leading to a significant decline in ecological quality and weakening of ecological service function (Wang et al., 2022). Consequently, there is an urgent need to effectively evaluate the RESI within the Dongting Lake area and implement comprehensive spatial protection measures for this national territory.
Numerous studies have been conducted to monitor and evaluate the status or changes in the ecological environment. Among these studies, remote sensing indices have been employed for evaluation purposes. These indices include the Normalized Vegetation Index (Kobayashi and Dye, 2005), enhanced vegetation index (Matsushita et al., 2007), Normalized Difference Water Index (Taloor et al., 2021), and the Normalized Difference moisture Index (Jin and Sader, 2005). Scholars have increasingly combined these remote sensing indices with socio-economic factors to construct pressure-state-response models for evaluating ecological health (Zhang et al., 2012; Qin et al., 2023). However, the subjectivity involved in applying hierarchical analysis to determine the weight of each factor reduces the credibility of the evaluation results (Cheng et al., 2022). Alternatively, some scholars have proposed a comprehensive ecological index, known as the Remote Sensing Based Ecological Index (RESI), which integrates four major ecological indicators: moisture, dryness, heat, and greenness. The principal component analysis of covariance is used to determine the weights of each factor on ecological quality. This approach emphasizes the objectivity and reasonability of the evaluation results by highlighting the influence of each indicator’s impact on ecological quality based on data characteristics rather than subjective factors (Xu et al., 2018). Thus, the use of the RESI to evaluate changes in ecological quality in the Dongting Lake area is both feasible and reliable.
Researchers have identified various factors that influence RESI, including climate, land use change, population, and economy(Ouyang et al., 2019; Hasan et al., 2020; Yang et al., 2022). The composition and health of different land use types serve as direct indicators of ecological quality (Hasan et al., 2020). Temperature and precipitation are significant climatic influences (Zou et al., 2020), while population density and socio-economic development contribute as prominent anthropogenic disturbances (Liu et al., 2023). It is reasonable to expect that higher population density will have a greater negative impact on ecological health (Wei et al., 2023). Therefore, it is imperative to study the factors influencing RESI in the Dongting Lake area and identify key factors responsible for the changes in RESI. However, current research on RESI has certain limitations. Firstly, it primarily focuses on urban areas, neglecting the lake area. Secondly, it fails to consider long-term changes in RESI and the varying intensity of influencing factors across different time periods.
Climate change and large-scale land development have led to changes in the structure and function of the lake and wetland ecosystems in the region, and it is necessary to curb ecological degradation by promoting ecological restoration. Efficient zoning control strategies and rapid improvement of ecological quality remain challenging. In this study, based on a 2 km × 2 km grid scale covering the Dongting Lake area, the ecological environment quality index (EEQI) was calculated from 2001 to 2020 by integrating four indicators, namely, humidity, dryness, heat and greenness, and the influencing factors of EEQ were analyzed by using GWR (geographically-weighted regression), and the relationship between ACI (Anthropogenic Composite Index) and RSEI was quantified to determine the priority areas for ecological restoration by combining the Anthropogenic Finally, the relationship between ACI and RSEI was quantified by combining the ACI to determine the ecological restoration priority areas. The study aims to address the following scientific questions: (1) What are the temporal and spatial characteristics of continuous changes in the RESI in the Dongting Lake area during the study period. (2) What are the potential factors that affect changes in the RESI in the Dongting Lake area. The ultimate goal is to provide guidance for formulating ecological restoration plans in the Dongting Lake area.
2 Materials and methods
2.1 Study area
The Dongting Lake area, centered on the waters of Dongting Lake, exhibits diverse landscapes transitioning into wetlands, plains, hills, and mountains (Tan et al., 2020). It serves as a significant ecological zone for regulating water levels in the middle and lower reaches of the Yangtze River. Additionally, it represents a fragile zone where land and water ecosystems intertwine at the confluence of four rivers: Xiang, Zizhi, Yuan, and Li. The region has a subtropical monsoon climate characterized by abundant precipitation, simultaneous rain and heat, and rich water, air, soil, and biological resources. It hosts diverse ecosystems, including wetlands, grasslands, forests, farmlands, and urban areas (Tan et al., 2020; Xiong et al., 2022). At present, the ecological environment of Dongting Lake is mainly facing problems such as unstable water quality, degradation of wetlands, declining water levels, loss of biodiversity, floods and land use conflicts. These problems affect the ecological balance of the lake, the sustainable use of water resources and the livelihoods of local residents.
To enhance the ecological quality of the Dongting Lake Basin, the Hunan Provincial People’s Government implemented the Three-Year Action Plan for the Ecological Environment of Dongting Lake (2018–2020). This plan encompassed measures such as addressing agricultural surface pollution, reforestation and wetland conservation, the removal of invasive poplars in the core area of the Dongting Lake Nature Reserve, and wetland restoration. The implementation of these measures has resulted in notable improvements in the habitat quality of the Dongting Lake Basin. However, serious threats to habitat quality persist. Hence, studying the habitat quality in the Dongting Lake area is both typical and representative. The study area includes selected counties (cities and districts) in Yueyang, Yiyang, and Changde, three prefecture-level cities located in the Dongting Lake area (Figure 1). It covers an area of approximately 25,800 km2, accounting for about 12.18% of Hunan Province. The resident population was approximately 10,705,800 by the end of 2020, constituting 16.11% of the province’s total, with a gross domestic product (GDP) of around 710,440 million yuan, representing approximately 17% of the province’s total.
2.2 Study methods
(1) Remote Sensing Based Ecological Index (RESI)
The Remote Sensing Based Ecological Index (RSEI) evaluates the quality of the ecological environment using four indicators: moisture, dryness, heat, and greenness. These indicators are closely related to the human living environment. They can be obtained by analyzing remote sensing images, and the specific data acquisition methods can be found in the data source section. Compared with the Ecological Environment Status Index, RSEI provides a more accurate assessment of the strengths and weaknesses of regional ecological environments (Lakes and Kim, 2012; Xu, 2013; Zheng et al., 2022). In this study, we utilized the ENVI 5.3 platform to calculate the indicators of moisture, dryness, heat, and greenness based on different bands of remote sensing images. Moisture was represented by WET values, dryness was determined by integrating surface building and surface bare soil indices, heat was derived through the inversion of a single window algorithm, and greenness was expressed using the Normalized Vegetation Index (NVI).
(2) Selection of Factors Influencing RSEI
When it comes to the selection of variables that affect RSEI, moisture, dryness, heat, and greenness are the main factors. These factors directly reflect the status of RESI. Moreover, the quality of the ecological environment is affected by both natural and human factors (Ouyang et al., 2021). Natural factors encompass climatic and geographical aspects, with temperature and precipitation being key climatic influences, while slope is an important geographical factor (Peng et al., 2017). Human factors incorporate disturbances such as proximity to the county government, population size, and socio-economic development (Retallack, 2021; Li et al., 2022). Since RSEI is constructed based on the metrics of moisture, dryness, heat, and greenness, these factors were not taken into account during the analysis of potential influencing factors. This study quantitatively analyzed the relationship between the entire RSEI mean and factors such as slope, temperature, rainfall, population density, GDP, and proximity to the county government using geographically weighted regression to dissect the correlation between natural factors and natural factors on RSEI.
(3) Selection of spatial priority areas for ecological restoration
This study utilized the Anthropogenic Composite Index (ACI) to measure the intensity of human activities within each 2 km × 2 km grid in the Dongting Lake area of Hunan Province, quantify the relationship between ACI and RSEI” for concision (Equation 1), and identify priority zones for ecological restoration using a spatial statistical method that considers the removal of water bodies. The calculation formula is as follows:
Where, Gstd is the standardized value of GDP, Pstd is the standardized value of population density, Nstd is the standardized value of nighttime lighting index, all expressed in terms of a 2 km × 2 km grid.
Based on the bivariate local Moran’s I (bi-LISA) method (Equation 2), the spatial correlation between the RSEI and the ACI was investigated to quantify the significance of ΔRSEI and ΔACI in image elements and their neighbouring shares at the 2 km grid scale. The calculation is as follows:
Where, x is the average change in the ΔACI of a pixel and y represents the average change in ΔRSEI of pixels nearby called j. The first-order queen-neighbor matrix serves as the foundation for the spatial weight matrix, Wi,j. Using significant values of p 0.001, 0.005, 0.01, and 0.05 as the discriminating criterion, the results revealed that the restoration priority locations had four significant value types: high-high (H-H), high-low (H-L), low-high (L-H), and low-low (L-L).
2.3 Data sources
The data used in this study were mainly derived from the Resource and Environment Science Data Centre of the Chinese Academy of Sciences (www.resdc.cn); China statistical yearbook (www.stats.gov.cn); OpenStreetMap (www.openstreetmap.org); Landscan (https://landscan.ornl.gov); and National Aeronautics and Space Administration (www.earthdata.nasa.gov). This study considers paddy fields, drylands, construction land, and bare land as potential threat sources due to their frequent human activities or harsher natural environments. In this study, NDVI, NDBSI, LST and WET data were calculated based on Landsat surface reflectance data, and then RSEI was further measured. The Landsat surface reflectance data were obtained from the USGS (United States Geological Survey) (https://lpdaac.usgs.gov) with a resolution of 500m. The parameter settings, including habitat suitability, weights of stressors, maximum stress distance, and sensitivity of habitat types to stressors, were adopted from relevant literature.
3 Results
3.1 Temporal and spatial characteristics of RESI
Based on the results of the spatial and temporal evolution of the ecological quality of Dongting Lake at a raster scale of 2 km × 2 km from 2001 to 2020 (Figure 2) and the categorical statistics of the ecological quality area (Table 1), there was an overall slight downward trend in the mean value of ecological quality. Over time, the mean value of RESI in the Dongting Lake area decreased from 0.52 in 2000 to 0.48 in 2020. The minimum value of RESI fluctuated with a decrease from 0.28 in 2000 to 0.22 in 2020, while the maximum value showed a fluctuating trend of ups and downs.
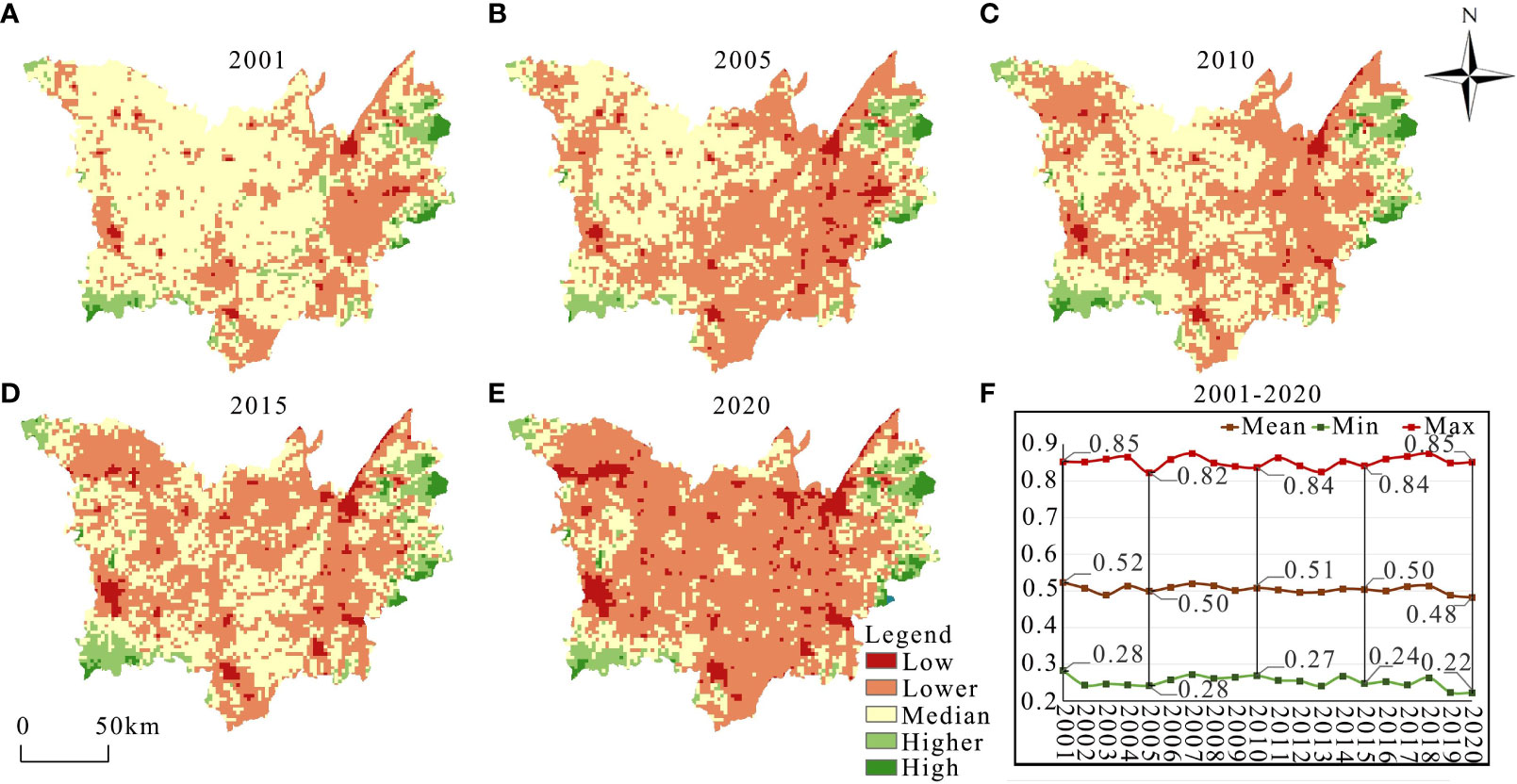
Figure 2 Spatial and temporal evolution of RESI in Dongting Lake region, 2001–2020. (A–E) 2001, 2005, 2010, 2015 and 2020 of RESI, (F) mean, min and max of RESI from 2001 to 2020.
From a spatial perspective, the RESI in the Dongting Lake region exhibits a concentration of high values and a dispersion of low values. High-value and higher-value areas are primarily found in Dingcheng District, Linxiang City, and other mountainous areas with abundant vegetation cover. On the other hand, low-value areas cluster in the eastern part of the Dongting Lake Basin, particularly in regions characterized by extensive urban construction and development, such as Heshan District, Yueyanglou District, and Yunxi District. Between 2000 and 2020, the process of urban expansion has led to a more extensive spatial distribution of low-value and lower-value areas of ecological quality in the Dongting Lake area. The area occupied by low-value areas increased by 4.43 times, while lower-value areas increased by 1.24 times. These areas have experienced rapid economic development, intense exploitation of land resources, and high population concentration, resulting in significant disturbances to the ecosystem.
During the period from 2001 to 2020, there were variations in the transfer of RESI levels in Hunan Province, demonstrating an overall gradient downward trend from high values to medium values and then to low values (Figure 3).
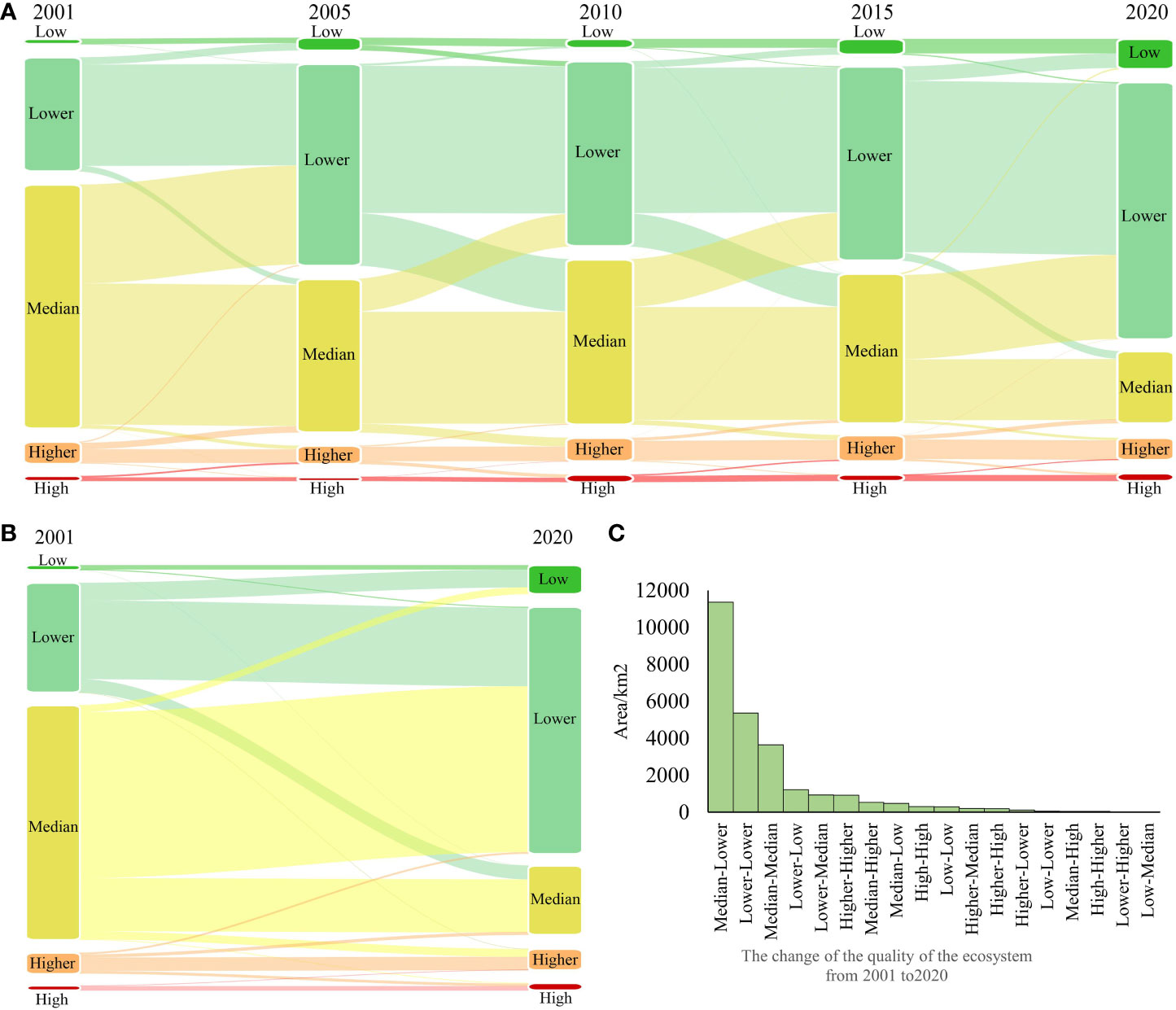
Figure 3 Transfer of RESI level in Dongting Lake region, 2001–2020. (A) Transfer of RESI in 2001–2005, 2005–2010, 2010–2015 and 2015–2020; (B) transfer of RESI from 2001 to 2020; (C) change of RESI from 2001 to 2020.
Specifically, the transfer of different RSEI levels occurred in an area of 15,222.85 km2 within the Dongting Lake area. The largest proportion of transfers was from the middle value to the lower value, accounting for 74.62% of the area (11,359.01 km2), followed by transfers from the lower value to the lower value, accounting for 8.04% of the area (1,223.52 km2). Transfers from the high value to the higher value accounted for 1.38%, and transfers from the higher value to the lower value accounted for 1.4%. Higher values were transferred to higher values in 1.38% of the area, covering an area of 43.42 km2.
3.2 Analysis of influencing factors
In this study, the Geographically Weighted Regression (GWR) model was developed to assess the ecological environment’s quality in the Dongting Lake area. The dependent variable was ecological environmental quality, while the independent variables included population (POP), GDP, road network density (RND), nighttime light index (NLI), elevation (DEM), slope (SP), temperature (TEM), precipitation (PRE), sunshine duration (SD), and NDVI. The GWR model’s goodness of fit was evaluated using R2, corrected R2, residual sum of squares (RSS), and AICC. A fixed bandwidth of 3113.034 was selected for the regression. Table 2 provides the corresponding parameters used in this study. The results indicated that the GWR model yielded a corrected R2 of 0.58, 0.68, and 0.72 for the years 2001, 2010, and 2020, respectively, which demonstrated a good fit.
The spatial distribution of each factor’s influence on the quality of the ecological environment was visualized and analyzed using the GWR model, indicated by the regression coefficients (Figure 4). Areas with the highest significance of influence were primarily located in regions with high ecological environment’s quality values and concentrated human activities. These regions included Dingcheng District, Linxiang City, Wuling District, and Yueyanglou District. Moreover, the center of gravity of the significantly influential areas gradually shifted from the western to the eastern part of the country during the period of 2001–2020.
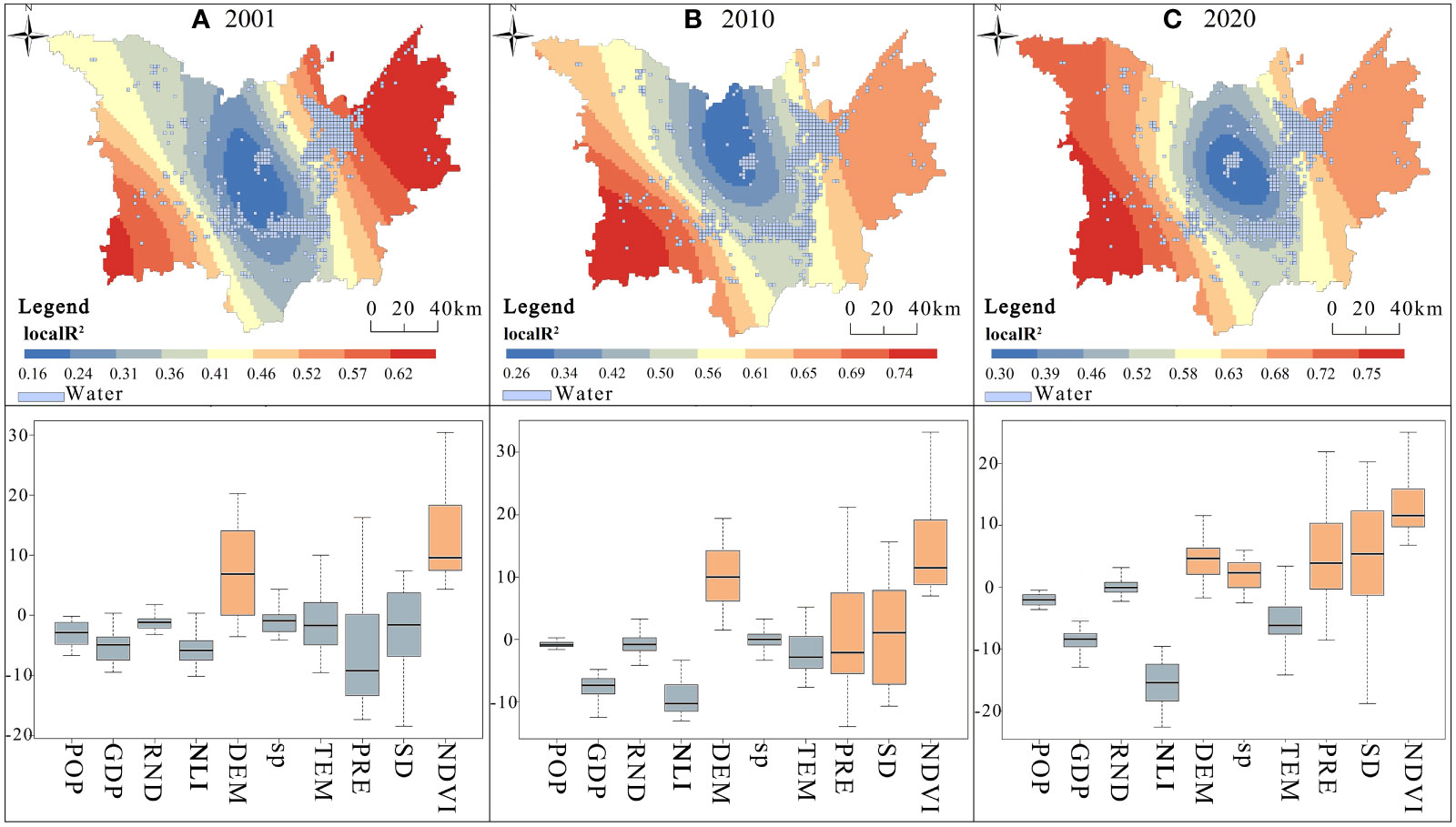
Figure 4 Spatial distribution of GWR residual sum of squares and visualization of regression coefficients. (A) 2001; (B) 2010; (C) 2020.
Regarding human factors, POP, GDP, RND, and NLI were found to be negatively correlated with the RSEI of the Dongting Lake area. The mean regression coefficients for POP in 2001, 2010, and 2020 were −0.14, −1.62, and −2.13. For GDP, the mean regression coefficients were −5.16, −8.35, and −9.19 during the same years. The RND had mean regression coefficients of −1.24, −0.65, and −0.45, while NLI had mean regression coefficients of −5.41, −9.45, and −15.70.
The negative influence of POP, GDP, and NLI on the RSEI showed a gradual increase over time. This can be attributed to accelerated industrialization and urbanization, which have led to higher levels of disturbance and pollution in densely populated areas, resulting in a decline in the ecological quality of the Dongting Lake area. However, the negative impact of RND on RESI decreased gradually as there were fewer excavation projects in the past two decades, leading to a relatively stable spatial pattern.
As for natural factors, DEM, SP, TEM, PRE, SD, and NDVI were positively correlated with the quality of the ecological environment during 2001–2020. The mean regression coefficients for elevation in 2001, 2010, and 2020 were 7.27, 10.10, and 4.36, respectively. The mean regression coefficients for SP were −0.95, −0.15, and 1.91. TEM had mean regression coefficients of −1.51, −2.16, and −5.39, while PRE had mean regression coefficients of −6.8 and 0.8. SD had mean regression coefficients of −2.39, 1.10, and 5.09, and NDVI had mean regression coefficients of 13.10, 14.97, and 14.44.
The positive influence of natural factors on RSEI increased from two factors (DEM and NDVI) in 2001 to five factors (DEM, SP, PRE, SD, and NDVI) in 2020. Specifically, SP, PRE, and SD exhibited an increasing positive trend, while the impact of NDVI consistently showed a high positive correlation with the quality of the ecological environment. Furthermore, under the backdrop of global warming, the annual mean temperature in the Dongting Lake area gradually exerted a negative effect on the ecological quality.
3.3 Delineation of ecological priority zones
In order to identify potential areas for ecological restoration, RSEI and ACI were used to perform spatial statistical tests. After the spatial calculations, ΔRSEI and ΔACI for the period 2001–2020 were utilized for significance tests to classify the grids in the Dongting Lake region of Hunan Province into four categories. The four ecological restoration priority areas include critical ecological restoration areas, important ecological restoration site areas, artificial ecological restoration site areas and natural base ecological restoration areas. The ecological restoration priority areas are considered as potential targets for artificial reconstruction or natural restoration. Critical ecological restoration areas represent pixels with large changes in ACI and large changes in neighboring RSEI, which have the potential to provide more high-quality ecosystem services. Critical ecological restoration site areas indicate pixels with large changes in ACI and small changes in neighboring RSEI, which have higher ecological sensitivity. Artificial ecological restoration site areas indicate pixels with small changes in ACI and large changes in the surrounding RSEI, which have a high urbanization intensity, and changes in the intensity of urbanization at a low level have a greater impact on the RSEI. Natural-based ecological restoration areas represent image elements with low ACI change and surrounding RSEI change, which are characterized by low ACI and low RSEI within the ecological red line, and ecosystems can be restored through nature-based solutions. We classified 3106 grids measuring 2 km × 2 km as priority control areas for ecological conservation in the Dongting Lake region, accounting for 45.66% of all grids. Among these, 3.91% exhibited significant ecological significance as the high-high type, 7.95% as the high-low type, 17.80% as the low-high type, and 16.00% as the low-low type (Figure 5).
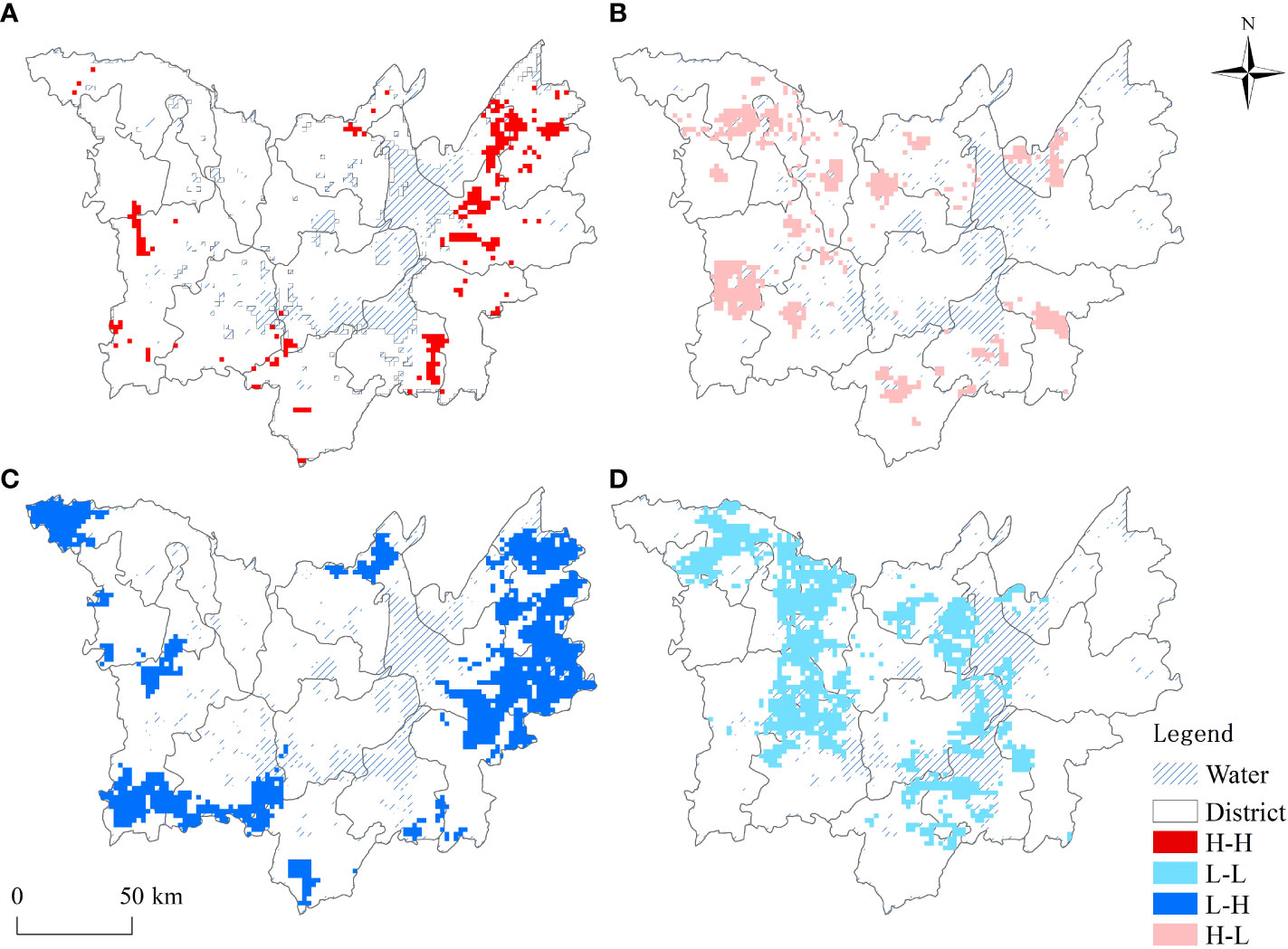
Figure 5 Priority areas for ecological restoration in the Dongting Lake region of Hunan Province. (A) H-H; (B) H-L; (C) L-H; (D) L-L.
The high-high type areas were primarily located in the municipal districts of Yueyang City, Changde City, Yiyang City, and surrounding areas such as Linli County. The high-low-type areas indicated regions with long-term urbanization and high levels of human disturbance, mainly concentrated in the central built-up areas of cities and counties. The low-high type areas were primarily distributed in regions with relatively favorable ecological conditions, such as the suburbs of Linxiang City, Yueyang County, and Li County. These areas are characterized by abundant vegetation and minimal human interference, and their ecological quality is mainly influenced by natural conditions such as climate and topography. The low-low type areas were mainly found in Anxiang County, Huarong County, and Hanshou County, exhibiting more stable ecological environments.
4 Discussion
4.1 Mechanism of ecological quality impact
The RESI in this area is a complex process influenced by a variety of factors, according to the findings of the spatio-temporal characteristics of the RESI and the analysis of influencing factors through GWR in the Dongting Lake region of Hunan Province (Figure 6). Specifically, urbanization and the level of economic development are considered to be among the most important factors affecting the ecological environment (Li et al., 2023). The advancement of urban expansion and industrialization has intensified pollutant emissions and land resource exploitation, thus exerting pressure on the ecosystem. Areas with high road network density contribute to land fragmentation and ecosystem destruction, negatively impacting the ecosystem.
Natural factors such as DEM, SP, PRE, SD, and NDVI positively influence the quality of the ecosystem. The positive effect of NDVI suggests that vegetation cover has a beneficial impact on ecosystem improvement, with higher NDVI values indicating healthier vegetation. Areas at higher elevations typically exhibit better ecological conditions, while regions with sufficient PRE and SD are favorable for vegetation growth and maintaining ecological balance.
However, the gradual increase in average annual temperature has a negative impact on ecological conditions. Global warming may lead to water scarcity, ecosystem disturbances, and species extinction, thus affecting the quality of the ecological environment. By comprehensively understanding and summarizing the mechanisms influencing the quality of the ecological environment, we can promote the orderly functioning of both natural and artificial ecosystems, ultimately improving the regional ecological environment. We can play a good role as a spatial carrier of the ecological environment in urban activities, which is essential in supporting human survival and promoting economic and social development in the Dongting Lake region.
4.2 Suggestions for zoning control
High-high type zoning refers to image elements with significant changes in anthropogenic disturbance intensity and surrounding RESI. High-low type zoning denotes image elements with significant changes in anthropogenic disturbance intensity but minimal changes in the quality of the surrounding ecological environment. Low-high type zoning indicates pixels with low changes in anthropogenic disturbance intensity but high changes in the quality of the surrounding ecosystem. Low-low type zoning represents image elements with low changes in anthropogenic disturbance intensity and low changes in the quality of the surrounding ecological environment. These categories, respectively, represent critical control areas (KERS), important control areas (IERS), artificially maintained areas (AERS), and naturally maintained areas (NERS). This study put forward suggestions for zoning control, which was in line with the findings of Lv et al. (2023) and Zhang et al. (2023).
Therefore, to address the decline in RESI in the Dongting Lake region during the period 2001–2020, it is crucial to exercise strict control over construction land expansion while considering the restoration of other land use types, such as land remediation and lake restoration (Zhang et al., 2018a; Niu et al., 2022). Changes in both the extent and quality of different land use types can better explain variations in the RESI of the Dongting Lake basin. It is strongly recommended to implement effective management plans, particularly those focused on ecological restoration, for crucial land use types within areas exhibiting low RSEI values.
4.3 Limitation and future research directions
This study presents a case study on the spatial and temporal variations in RSEI within the Dongting Lake area. Moreover, it quantifies the influence of ten key drivers on RSEI changes at a 2 km × 2 km grid scale, taking into account the contributions of human activities and natural factors. These findings offer valuable insights for future urbanization and ecological preservation efforts in the Dongting Lake region. However, it is essential to acknowledge the limitations of this study.
Firstly, it should be noted that the findings of this study are specific to the Dongting Lake region and may not be generalizable. While the Dongting Lake area possesses valuable wetland ecosystems and represents a typical level of ecological damage, conducting experiments on a larger scale and in additional regions would yield a more comprehensive understanding. This approach would enable researchers to assess if similar conclusions can be drawn for other urban areas.
Secondly, this study underscores that anthropogenic factors exert the most significant influence on regional RSEI, aligning with previous research findings. Notably, human activities such as urban expansion largely contribute to the ecological disparities among the three key urban agglomerations in the Yangtze River Economic Belt. However, balancing societal development and environmental preservation remains a challenging task. Therefore, it is essential to identify other influential factors that impact RSEI, thus facilitating its improvement through effective urban planning. For instance, factors such as the distribution of green infrastructure, the establishment of ecological redlines, and energy consumption play pivotal roles in addressing the regional disparity between RSEI and human social development.
In conclusion, despite certain limitations, this study offers valuable insights into the dynamics and determinants of RSEI in the Dongting Lake region. Conducting future research on a broader scale, encompassing multiple cities, and considering additional factors like the distribution of green infrastructure will enhance our comprehensive understanding of the underlying reasons behind the RSEI imbalance and provide pathways towards its resolution. Moreover, such research will contribute to well-informed urban planning and ecological enhancement efforts.
5 Conclusion
This study is to evaluate the spatial and temporal evolution of RESI in the Dongting Lake area and to analyze its relationship with various anthropogenic and natural factors. The overall RESI of the Dongting Lake area exhibited a declining trend from 2001 to 2020, with the mean value dropping from 0.52 in 2001 to 0.48 in 2020. The high-quality areas were primarily located in mountainous and forested regions, whereas the low-quality areas were concentrated in zones characterized by extensive urban construction and development, particularly in the eastern watershed area. The GWR results show that urban expansion and economic development have significant negative impacts on the ecological environment, which are related to economic development, land resource development and population agglomeration, and also reflect the importance of balance and sustainability between human factors and natural factors. The geographically weighted regression (GWR) analysis revealed a positive relationship between urban expansion and the expansion of low-quality areas. This can be attributed to economic development, land resource exploitation, and population concentration. POP, GDP, RND, and NLI displayed negative correlations with ecological quality, and these detrimental effects intensified over time. Natural factors, including DEM, SP, PRE, SD and NDVI, exhibited a positive influence on ecological quality, with NDVI consistently showing a positive effect. The mean annual temperature had an incrementally adverse impact on RESI, possibly attributable to global warming. These divisions provide governments and decision makers with information on how to undertake sustainable ecological management and policy development. Policies and plans can be developed accordingly for each type of area to balance the need for resource utilization and ecological protection. It provides a strong basis for targeted control and management, ensuring effective resource utilization and ecological protection.
Data availability statement
The raw data supporting the conclusions of this article will be made available by the authors, without undue reservation.
Author contributions
XY: Conceptualization, Data curation, Formal analysis, Writing – original draft, Writing – review & editing. ZL: Visualization, Writing – review & editing. BZ: Formal analysis, Supervision, Validation, Writing – review & editing, Writing – original draft.
Funding
The author(s) declare financial support was received for the research, authorship, and/or publication of this article. Humanities and Social Science Fund of Ministry of Education (23YJA710051).
Conflict of interest
The authors declare that the research was conducted in the absence of any commercial or financial relationships that could be construed as a potential conflict of interest.
Publisher’s note
All claims expressed in this article are solely those of the authors and do not necessarily represent those of their affiliated organizations, or those of the publisher, the editors and the reviewers. Any product that may be evaluated in this article, or claim that may be made by its manufacturer, is not guaranteed or endorsed by the publisher.
References
Cheng H., Zhu L., Meng J. (2022). Fuzzy evaluation of the ecological security of land resources in mainland China based on the Pressure-State-Response framework. Sci. Total Environ. 804, 150053. doi: 10.1016/j.scitotenv.2021.150053
Hasan S. S., Zhen L., Miah M. G., Ahamed T., Samie A. (2020). Impact of land use change on ecosystem services: A review. Environ. Dev. 34, 100527. doi: 10.1016/j.envdev.2020.100527
He J., Pan Z., Liu D., Guo X. (2019). Exploring the regional differences of ecosystem health and its driving factors in China. Sci. Total Environ. 673, 553–564. doi: 10.1016/j.scitotenv.2019.03.465
Jin S., Sader S. A. (2005). Comparison of time series tasseled cap wetness and the normalized difference moisture index in detecting forest disturbances. Remote Sens. Environ. 94, 364–372. doi: 10.1016/j.rse.2004.10.012
Kobayashi H., Dye D. G. (2005). Atmospheric conditions for monitoring the long-term vegetation dynamics in the Amazon using normalized difference vegetation index. Remote Sens. Environ. 97, 519–525. doi: 10.1016/j.rse.2005.06.007
Lakes T., Kim H.-O. (2012). The urban environmental indicator “Biotope Area Ratio”—An enhanced approach to assess and manage the urban ecosystem services using high resolution remote-sensing. Ecol. Indic. 13, 93–103. doi: 10.1016/j.ecolind.2011.05.016
Li F., Yin X., Shao M. (2022). Natural and anthropogenic factors on China’s ecosystem services: Comparison and spillover effect perspective. J. Environ. Manage. 324, 116064. doi: 10.1016/j.jenvman.2022.116064
Li W., Kang J., Wang Y. (2023). Spatiotemporal changes and driving forces of ecological security in the Chengdu-Chongqing urban agglomeration, China: Quantification using health-services-risk framework. J. Clean. Prod. 389, 136135. doi: 10.1016/j.jclepro.2023.136135
Liu C., Liu Y., Giannetti B. F., Almeida C. M. V. B., Sevegnani F., Li R. (2023). Spatiotemporal differentiation and mechanism of anthropogenic factors affecting ecosystem service value in the Urban Agglomeration around Poyang Lake, China. Ecol. Indic. 154, 110733. doi: 10.1016/j.ecolind.2023.110733
Lv T., Zeng C., Lin C., Liu W., Cheng Y., Li Y. (2023). Towards an integrated approach for land spatial ecological restoration zoning based on ecosystem health assessment. Ecol. Indic. 147, 110016. doi: 10.1016/j.ecolind.2023.110016
Matsushita B., Yang W., Chen J., Onda Y., Qiu G. (2007). Sensitivity of the enhanced vegetation index (EVI) and normalized difference vegetation index (NDVI) to topographic effects: a case study in high-density cypress forest. Sensors 7, 2636–2651. doi: 10.3390/s7112636
Niu L., Shao Q., Ning J., Huang H. (2022). Ecological changes and the tradeoff and synergy of ecosystem services in western China. J. Geographical Sci. 32, 1059–1075. doi: 10.1007/s11442-022-1985-6
Ouyang X., Shao Q., Zhu X., He Q., Xiang C., Wei G. (2019). Environmental regulation, economic growth and air pollution: Panel threshold analysis for OECD countries. Sci. Total Environ. 657, 234–241. doi: 10.1016/j.scitotenv.2018.12.056
Ouyang X., Tang L., Wei X., Li Y. (2021). Spatial interaction between urbanization and ecosystem services in Chinese urban agglomerations. Land Use Policy 109, 105587. doi: 10.1016/j.landusepol.2021.105587
Peng J., Liu Y., Li T., Wu J. (2017). Regional ecosystem health response to rural land use change: A case study in Lijiang City, China. Ecol. Indic. 72, 399–410. doi: 10.1016/j.ecolind.2016.08.024
Qin X., Hu X., Xia W. (2023). Investigating the dynamic decoupling relationship between regional social economy and lake water environment: The application of DPSIR-Extended Tapio decoupling model. J. Environ. Manage. 345, 118926. doi: 10.1016/j.jenvman.2023.118926
Retallack M. (2021). The intersection of economic demand for ecosystem services and public policy: A watershed case study exploring implications for social-ecological resilience. Ecosyst. Serv. 50, 101322. doi: 10.1016/j.ecoser.2021.101322
Su M., Fath B. D., Yang Z. (2010). Urban ecosystem health assessment: A review. Sci. Total Environ. 408, 2425–2434. doi: 10.1016/j.scitotenv.2010.03.009
Taloor A. K., Drinder Singh M., Chandra Kothyari G. (2021). Retrieval of land surface temperature, normalized difference moisture index, normalized difference water index of the Ravi basin using Landsat data. Appl. Comput. Geosci. 9, 100051. doi: 10.1016/j.acags.2020.100051
Tan J., Yu D., Li Q., Tan X., Zhou W. (2020). Spatial relationship between land-use/land-cover change and land surface temperature in the Dongting Lake area, China. Sci. Rep. 10, 9245. doi: 10.1038/s41598-020-66168-6
Wang H., Huang L., Guo W., Zhu Y., Yang H., Jiao X., et al. (2022). Evaluation of ecohydrological regime and its driving forces in the Dongting Lake, China. J. Hydrology: Regional Stud. 41, 101067. doi: 10.1016/j.ejrh.2022.101067
Wei G., He B.-J., Sun P., Liu Y., Li R., Ouyang X., et al. (2023). Evolutionary trends of urban expansion and its sustainable development: Evidence from 80 representative cities in the belt and road initiative region. Cities 138, 104353. doi: 10.1016/j.cities.2023.104353
Xiong J., Wang X., Zhao D., Zhao Y. (2022). Spatiotemporal pattern and driving forces of ecological carrying capacity during urbanization process in the Dongting Lake area, China. Ecol. Indic. 144, 109486. doi: 10.1016/j.ecolind.2022.109486
Xu H. (2013). A remote sensing urban ecological index and its application. Acta Ecol. Sin. 33, 7853–7862. doi: 10.5846/stxb201208301223
Xu H., Wang M., Shi T., Guan H., Fang C., Lin Z. (2018). Prediction of ecological effects of potential population and impervious surface increases using a remote sensing based ecological index (RSEI). Ecol. Indic. 93, 730–740. doi: 10.1016/j.ecolind.2018.05.055
Yang S., Liu J., Wang C., Zhang T., Dong X., Liu Y. (2022). Vegetation dynamics influenced by climate change and human activities in the Hanjiang River Basin, central China. Ecol. Indic. 145, 109586. doi: 10.1016/j.ecolind.2022.109586
Yu Y., Mei X., Dai Z., Gao J., Li J., Wang J., et al. (2018). Hydromorphological processes of dongting lake in China between 1951 and 2014. J. Hydrology 562, 254–266. doi: 10.1016/j.jhydrol.2018.05.015
Zhang D., Jia Q., Xu X., Yao S., Chen H., Hou X. (2018a). Contribution of ecological policies to vegetation restoration: A case study from Wuqi County in Shaanxi Province, China. Land Use Policy 73, 400–411. doi: 10.1016/j.landusepol.2018.02.020
Zhang X., Ma C., Zhan S., Chen W. (2012). Evaluation and simulation for ecological risk based on emergy analysis and Pressure-State-Response Model in a coastal city, China. Proc. Environ. Sci. 13, 221–231. doi: 10.1016/j.proenv.2012.01.021
Zhang Y., Hu X., Wei B., Zhang X., Tang L., Chen C., et al. (2023). Spatiotemporal exploration of ecosystem service value, landscape ecological risk, and their interactive relationship in Hunan Province, Central-South China, over the past 30 years. Ecol. Indic. 156, 111066. doi: 10.1016/j.ecolind.2023.111066
Zhang Y., Tian Y., Shen M., Zeng G. (2018b). Heavy metals in soils and sediments from Dongting Lake in China: occurrence, sources, and spatial distribution by multivariate statistical analysis. Environ. Sci. Pollut. Res. 25, 13687–13696. doi: 10.1007/s11356-018-1590-5
Zheng Z., Wu Z., Chen Y., Guo C., Marinello F. (2022). Instability of remote sensing based ecological index (RSEI) and its improvement for time series analysis. Sci. Total Environ. 814, 152595. doi: 10.1016/j.scitotenv.2021.152595
Keywords: Dongting Lake area, ecological environment, zoning control, influencing factors, ecological restoration
Citation: Yin X, Lu Z and Zhang B (2024) Study on the factors influencing ecological environment and zoning control: a study case of the Dongting Lake area. Front. Ecol. Evol. 11:1308310. doi: 10.3389/fevo.2023.1308310
Received: 06 October 2023; Accepted: 11 December 2023;
Published: 10 January 2024.
Edited by:
Xue-Chao Wang, Beijing Normal University, ChinaCopyright © 2024 Yin, Lu and Zhang. This is an open-access article distributed under the terms of the Creative Commons Attribution License (CC BY). The use, distribution or reproduction in other forums is permitted, provided the original author(s) and the copyright owner(s) are credited and that the original publication in this journal is cited, in accordance with accepted academic practice. No use, distribution or reproduction is permitted which does not comply with these terms.
*Correspondence: Benqing Zhang, zhang1667799@foxmail.com