- 1State Key Laboratory of Simulation and Regulation of Water Cycle in River Basin, China Institute of Water Resources and Hydropower Research, Beijing, China
- 2Key Laboratory of Water Safety for Beijing-Tianjin-Hebei Region of Ministry of Water Resources, Beijing, China
- 3Institute of International Rivers and Eco-security, Yunnan University, Kunming, China
- 4Yunnan Key Laboratory of International Rivers and Transboundary Eco-security, Yunnan University, Kunming, China
- 5Department of Geography and Spatial Information Techniques, Ningbo University, Ningbo, China
Effective riverine ecosystem management and restoration are predicated upon comprehending the relationship between land use patterns, the aquatic environment, and macroinvertebrates. However, the processes by which land use affects riverine ecosystems are exceedingly complex, influenced by factors across varying special scales. To explore these multi-scale relationships, we centered our study on the Taizi River Basin (TRB) in northeastern China, encompassing data on land use, macroinvertebrates, physical habitats, and water quality. We employed a synergy of correlation analysis, multiple stepwise regression, and redundant analysis techniques to discern these relationships at diverse spatial extents. This approach led to the development of 24 structural equation models (SEMs) that delineate the pathways bridging land use patterns to various biological indices. Remarkably, the interpretive capacity of SEM-Benthic Index of Biotic Integrity (SEM-BIBI), SEM-Shannon Wiener index (SEM-H’), and SEM-Average Score Per Taxon (SEM-ASPT) models was 76%, 70%, and 66% respectively. Within the TRB, the primary pathway from land use pattern to biological index consisted of: land use (predominantly rural and agricultural) feeding into physical habitat (habitat complexity, combined characteristic of speed and depth), which in turn affected benthic indicators. A secondary pathway charts a course from land use, through water quality (electrical conductivity and total dissolved solids) and culminating in benthic variations. Furthermore, our findings indicated that inverse-distance-weighted (IDW) metrics, specifically the IDW distance based on flow length to the stream outlet and the nearest stream (iFLO_m and iFLS_m, respectively) could provide more accurate interpretations of the river’s ecological health status. Given the effects of rural areas and agriculture on macroinvertebrates, we recommend establishing 100-m wide riparian buffers. The optimal buffer range should be determined by evaluating various biological needs and considerations.
1 Introduction
Land use change is intimately associated with regional hydrological processes, including evapotranspiration and base flow formation. These processes in turn influence the water balance (Schilling et al., 2008; Li et al., 2020), riparian vegetation (Palt et al., 2022), and biodiversity (Fierro et al., 2017; Luo et al., 2021) of rivers. Urbanization and agriculture have reduced water yield and deteriorated water quality. (Atasoy et al., 2006; Chen et al., 2016; Fan et al., 2022; Kaufmann et al., 2022; Feio et al., 2023). These shifts subsequently alter the composition and diversity of aquatic organisms such as fish, algae, and macroinvertebrates in rivers, lakes, and other water bodies (Liu et al., 2023).
Among these affected aquatic organisms, macroinvertebrate assemblages serve as optimal indicators of river ecosystem alterations. Macroinvertebrates exhibit inherent sensitivity to changes in riverine health, exist in diverse groupings, have broad life-history requirements, and can be readily collected (Wallace and Webster, 1996; O’Brien et al., 2016). Therefore, macroinvertebrates have been widely employed in water ecological monitoring in Australia (Kefford et al., 2020), the United States (Carlisle and Hawkins, 2008; Wilkins et al., 2015), and many other regions (Eriksen et al., 2021).
The reactions of macroinvertebrates to land use patterns are intricate and influenced by various factors at different spatial extents. According to the hierarchical framework proposed by Frissell et al. (1986), smaller-scale systems are shaped within the constraints delineated by the larger-scale systems they are a part of. Various factors, including the climate, geology, and vegetation at the basin level significantly affect the water environment and physical habitats, thereby directly affecting macroinvertebrates. Numerous studies have explored the effects of environmental factors on macroinvertebrates at different spatial extents, such as land use (Hettetronquart et al., 2017; Dalu et al., 2022) and riparian vegetation (Palt et al., 2022). However, varied key influential factors have been identified according to the choice of scale in the analysis (Gove et al., 2010; Colas et al., 2017). Earlier work by Kaufmann et al. (1999) laid foundational methodologies for quantifying the physical habitats in wadable streams, presenting a crucial reference for contemporary aquatic research. Typically, at larger spatial extents, land use changes exert long-term, comprehensive effects on macroinvertebrates. However, at the reach scale, factors such as the riparian zone, water quality, and sediment significantly affect the ecology of macroinvertebrates (Hettetronquart et al., 2017; Luo et al., 2018; He et al., 2020). Many studies have investigated how land use and environmental factors affect macroinvertebrates in specific regions in the Americas and in South America (Macedo et al., 2014; Chen et al., 2019; Herlihy et al., 2020; Hughes et al., 2023). However, there is a lack of comprehensive research in China examining land use patterns and their impact pathways at multiple spatial scales. This multiscale perspective is required to obtain global insight on these associations and offer practical guiding significance for effective management.
Basin conditions are typically characterized according to lumped attributes, such as the percentage of land use, as a lumped method offers operational advantages for ecosystem research. In particular, lumped attributes can be readily obtained through a geographic information system (GIS); however, this comes at a cost of losing spatially explicit information. Several GIS-based methods are more suited to explain the ecological processes of regions affected by land use changes, but cannot reveal crucial information on the spatial distribution of distinct land use activities. For instance, Lorion and Kennedy (2010) discovered that riparian forest buffers significantly influence macroinvertebrate diversity in neotropical headwater streams. An alternative method to lumped metrics is a distance-weighted approach with the advantage of a spatially explicit analysis that can better reflect macroinvertebrates as indicators of aquatic system health. A distance-weighted approach also improves the predictive power of the relationship between land use and benthic characteristics (Peterson et al., 2011; Zhang et al., 2015; Meyer et al., 2022). Taking these considerations into account, we compared the effectiveness of lumped metrics, Euclidean distance buffer metrics, and inverse distance-weighted (IDW) metrics in determining the impacts of land use on macroinvertebrate assemblages at multiple key spatial extents.
Structural equation models (SEMs) have been widely used in psychology and social science (MacCallum and Austin, 2000), and their application in ecological research has been expanding in recent years (Capmourteres and Anand, 2016; Villeneuve et al., 2018; Jia et al., 2023). SEMs are instrumental in the modeling and testing of causal relationships among factors, leveraging the covariance structure between variables (Shipley, 2002). Thus, SEMs show promising applications in various studies of the natural environment, including those focused on soil, biology (Eisenhauer et al., 2015; Beaumelle et al., 2016; Fan et al., 2016; Cui et al., 2022), and freshwater ecosystems (Leitão et al., 2018; Alvarenga et al., 2021).
Recognizing the influence of environmental factors on macroinvertebrates via diverse pathways, we employed SEMs to identify the pivotal factors shaping these relationships. We further explored the intricate and variable nature of the ecological pathways across different spatial extents. Toward this end, we established SEMs of land use patterns related to macroinvertebrates in the Taizi River Basin (TRB) of China. The goals of our research were to (i) evaluate the influence of land use metrics on macroinvertebrates and (ii) discern the primary ecological pathways concerning land use patterns, the water environment and physical habitat, and macroinvertebrates. Notably, the multiscale approach adopted herein enabled identification of impacts at various spatial extents. Elucidation of these impact pathways will serve as a baseline and foundation for regional land use planning and river ecological management.
2 Materials and methods
2.1 Study area
The Taizi River (122°25’ E–124°56’ E, 40°28’ N–41°40’N) is located in the southeastern part of Liaoning Province in northeastern China. This river serves as a key tributary of the Liaohe River upstream, spanning a total length of 413 km with coverage of 1.4 × 104 km2 (Figure 1). The TRB is characterized by a complex terrain with increasing elevation and human activities from east to west. The upper reaches are mainly low hills with high vegetation cover and limited human interventions, while the middle and lower reaches are marked by hilly and plain terrains. Flow paths through Benxi, Liaoyang, and Anshan reveal a dominantly industrial landscape. The lower reach underpins the agricultural economy of Liaoning Province. The diversity in climatic conditions and topography across different geographical regions results in a differential distribution of land use changes across the TRB.
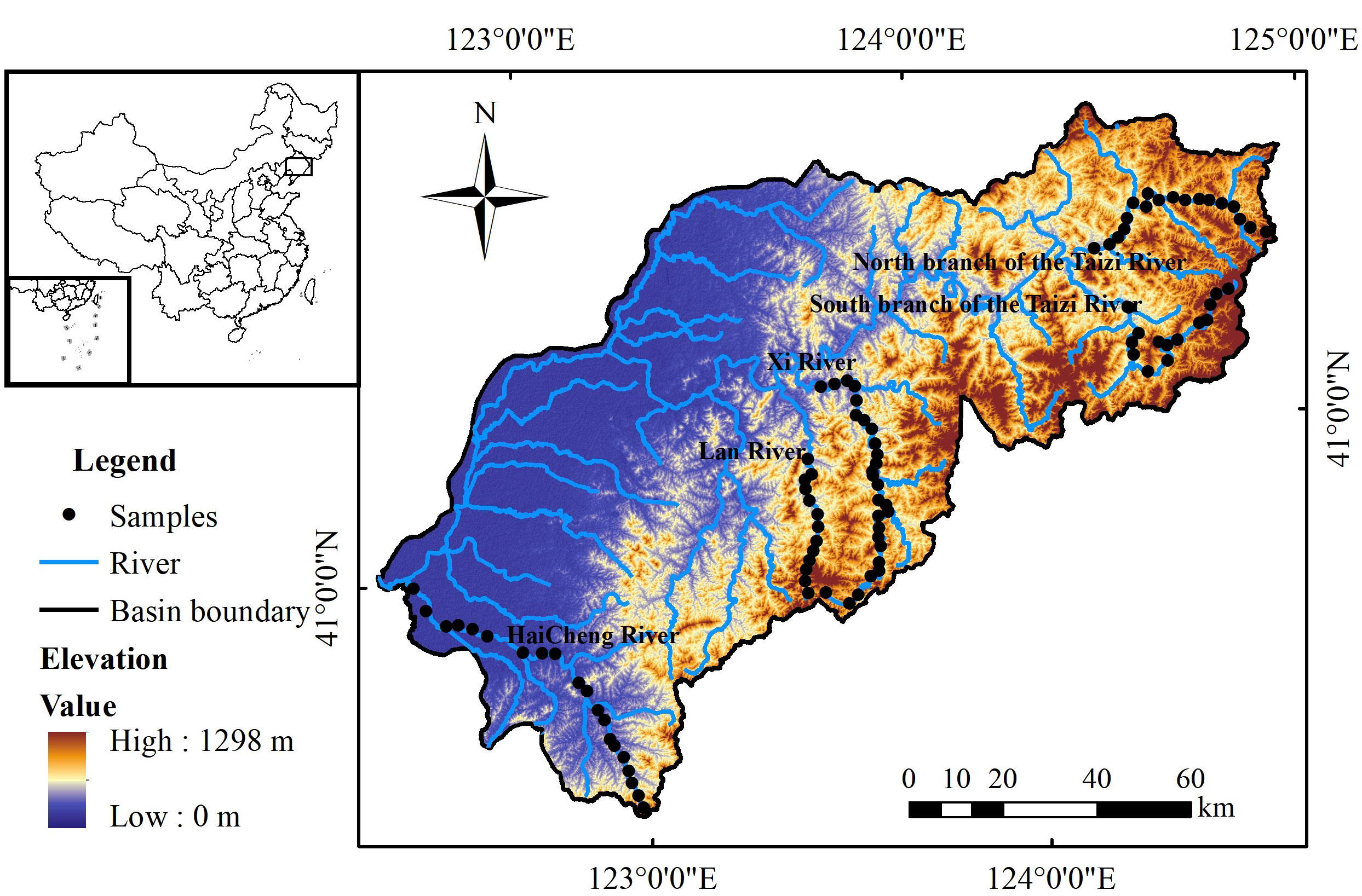
Figure 1 Location of the study area in the Taizi River Basin. The black dots denote the distribution of sampling sites.
2.2 Macroinvertebrate sampling
We selected 92 sampling locations spread across the TRB (Figure 1), encompassing the Haicheng River, Lanhe River, Xihe River, and southern and northern tributaries flowing into the Taizi River. Macroinvertebrate samples were collected with a Surber net (30 × 30 cm, 0.4mm). At locations where wading was possible, we gathered three replicate samples from two riffles and a shallow pool. For sites where wading was not feasible, three replicates samples were collected from shallow waters along the river bank using the same method. All specimens were passed through a 0.4-mm mesh sieve. The remaining organisms were fixed, preserved using 70% alcohol, and brought back to the laboratory for identification under a stereomicroscope (Olympus SZ511), according to the identification keys of Merritt and Cummins (1996) and Morse et al. (1984). A total of 76 families were determined, enabling establishment of assemblage indicators. The overall sample was largely dominated by insects, crustaceans, and gastropods, comprising 90.8%, 2.0%, and 1.7% of the total sample, respectively. Among the identified taxa, Orthocladinae, Chironomidae, and Baetidae are density-predominant species, contributing to 25.6%, 13.9%, and 9.3% respectively (see the Appendix for details). To adequately characterize the macroinvertebrate assemblages within the TRB, each sample was evaluated using the benthic index of biotic integrity (B-IBI), average score per taxon (ASPT), and Shannon–Wiener index (H’). The evaluation of B-IBI was based on reported river health assessment indicators from North America and Europe. Through distribution tests, sensitivity analysis, and correlation tests, the following core parameters of B-IBI for the study area were determined: total taxa, Plecoptera taxa, Trichoptera%, EPT% (total Ephemeroptera, Plecoptera, and Trichoptera individuals divided by the total number of macroinvertebrates of all taxa), tolerant taxa%, dominant taxa%, filterers%, and predators%. The B-IBI was then computed using a standardized method, as described by Qu et al. (2012). The formulas for calculating B-IBI, ASPT, and H’ are provided in Table S1 and Text S1.
2.3 Water environment and physical habitat variables
Across the 92 sampling sites, we identified 14 variables emblematic of the physical habitat and water environment (Table 1). River width measurements were based on three random transects per sampling station, while the water depth was measured only at the macroinvertebrate sampling locations. A multiparameter water quality probe (YSI-Pro Plus, YSI Inc., USA) was utilized to measure the water temperature, electrical conductivity (EC), dissolved oxygen (DO), total dissolved solids (TDS), and pH. The total phosphorus (TP), total nitrogen (TN), ammonia nitrogen (NH3-N), nitrate-nitrogen (NO3-N), and chemical oxygen demand (COD) were evaluated in the laboratory per the “Environmental quality standards for surface water” standard of China (GB3838-2002). Water samples were collected in sterilized polyethylene bottles and immediately acidified to pH<2 for preservation. The samples were stored in a cooler with ice packs to maintain a temperature below 4°C during transport. In the lab, samples were maintained at 4°C and analyzed within 48 h. Suspended solids (SS) were quantified via filtration through pre-dried cellulose acetate membranes (0.45 μm) per the Chinese standard (GB11901-89).
Our analysis approach involved the adoption of 10 quality indicators for river habitat, as detailed in Table S2: substrate, habitat complexity, comprehensive depth and flow velocity, embankment stability, channel change, river flow, vegetation diversity, water quality, human activities, and riparian land use. Together, these indicators provide a comprehensive perspective on water quality, water yield, human activity, and the physical structure of the river habitat. The rating system used in the Liaohe Basin (Zheng et al., 2007) served as our primary criteria for evaluation, which was complemented by methods from Hughes et al. (2010) on agricultural stream habitats.
2.4 Landscape pattern and metrics
Land use classification was conducted based on the 2010 Landsat Thematic Mapper data with a spatial resolution of 30 m. We utilized a supervised classification approach in ERDAS IMAGINE, defining training areas for each land use type to interpret the imagery. The resulting land use map was divided into five categories: rural area, urban area, forest, grassland, and agricultural land. Although rural areas might also contain forests, grasslands, and agricultural lands, these terms primarily describe types of landscapes or land uses rather than the human settlement patterns characterizing rural areas. We utilized both lumped metrics and IDW metrics for comparison (Figure 2). The former quantifies the percentage values of different land uses at the watershed or buffer scales. The latter involves the use of spatial weight layers, giving higher importance to landscape cells closer to streams or monitoring sites. We calculated the IDW metrics by superimposing land use layers and spatial weight layers via a distance-decay function. The IDW metrics calculated based on their flow length to the stream outlet (iFLO) and the flow length to nearest stream (iFLS) were used to represent the distance in spatial weight layers. This approach results in higher weight values for areas closer to streams or monitoring sites (Peterson et al., 2011; Walsh and Webb, 2014).
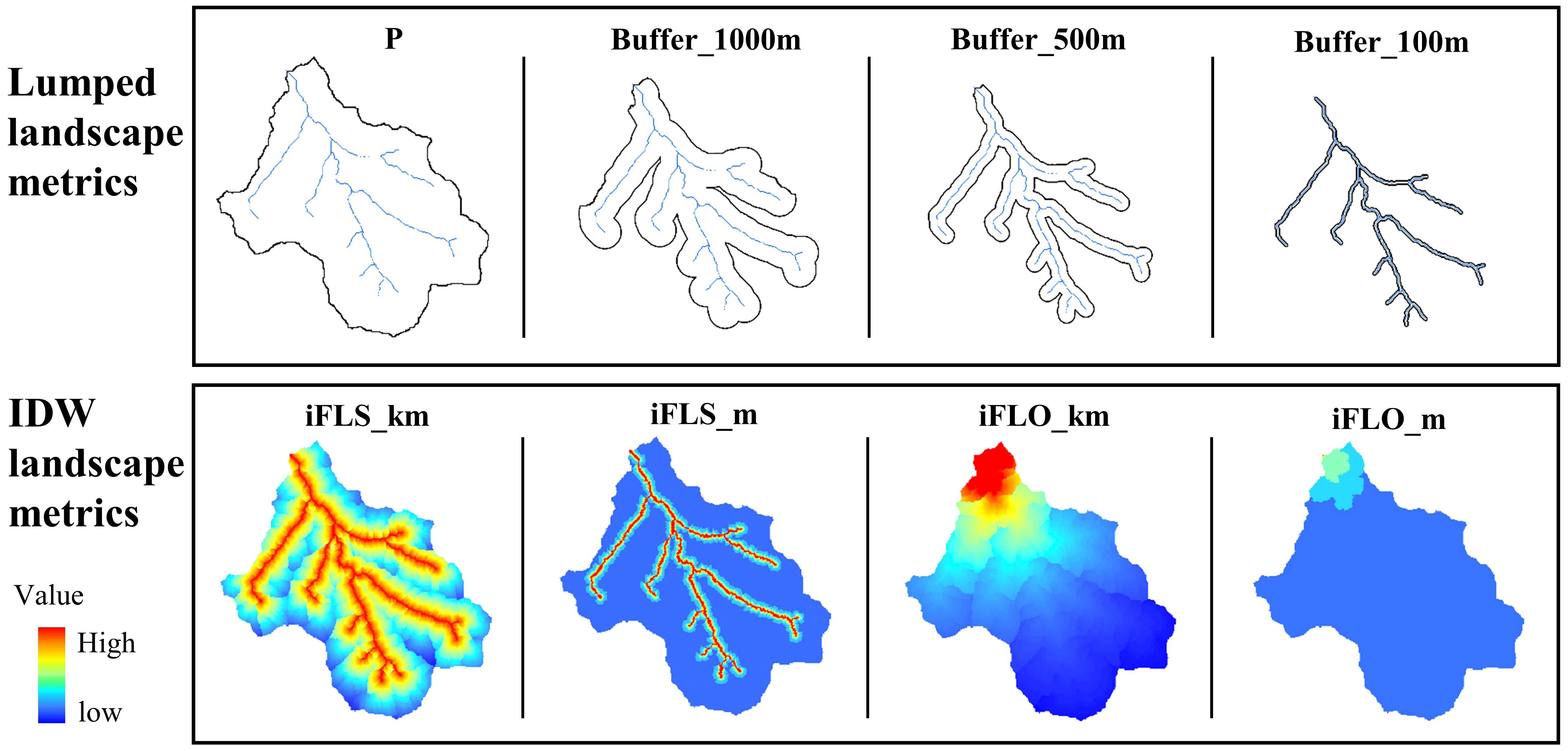
Figure 2 Lumped landscape metrics considering all cells as having an equivalent impact due to their non-spatial characteristics, using the entire basin’s percentage (P) of specific land use types and buffers of varying ranges (Buffer_1000m, Buffer_500m, and Buffer_100m). The inverse-distance-weighted (IDW) landscape metrics consider the spatial distribution and assign weights to distances based on their flow length to the stream outlet (iFLO) or to the nearest stream (iFLS).
3 Statistical analysis
3.1 Data preprocessing
Redundancy analysis (RDA) was employed to discern water environment and physical habitat factors that significantly affect the structural characteristics of macroinvertebrates. The species data used for the RDA were the absolute numbers for each species. The eigenvalues for each axis were deduced through a detrending analysis performed prior to the RDA, with a maximum value of 3.38 (range: 3–4). Subsequently, RDA was utilized to delve deeper into the association between environmental factors and macroinvertebrates. We determined the variables using abundance data, conducted significance tests, and then screened the key environmental factors through the pre-selection process. Environmental factors contributing significantly (p< 0.05) to species variation were evaluated using the Monte Carlo permutation test with 999 permutations. Variables with a variance inflation factor less than 5 were selected for inclusion in the model.
RDA offers a descriptive global view of the relationships among all variables. Therefore, to ascertain which variables have the greatest predictive importance, we employed multivariate stepwise regression models to investigate the influence of land use patterns on river chemistry, physical parameters, and macroinvertebrates. The adjusted R2 value from the regression model quantifies the explanatory power of these metrics, helping to elucidate the impacts of land use under various landscape metrics from a predictive modeling perspective. Finally, we developed SEMs to examine the pathways between land use pattern, water environment, physical habitat, and macroinvertebrates. RDA and multivariate stepwise regression were performed using CANOCO4.5 and STATISTICA7.0 software, respectively. To ensure adherence to normality assumptions, all environmental data and evaluation indicators (S1–S10), with the exception of pH, were subjected to log (x + 1) transformation and standardization prior to RDA; pH was not log-transformed given its inherent logarithmic nature.
3.2 SEM construction
SEMs were implemented using AMOS 7.0 software (Arbuckle, 2006) to examine the pathway and assess the pathway coefficient (Pc) of the influence of human activities on river ecosystems across diverse spatial extents. Prior to model construction, a conceptual model of the regional river ecosystem was required. The conceptual model was informed by the fundamental theory and associated findings from prior Taizi River ecosystem studies (Figure 3).
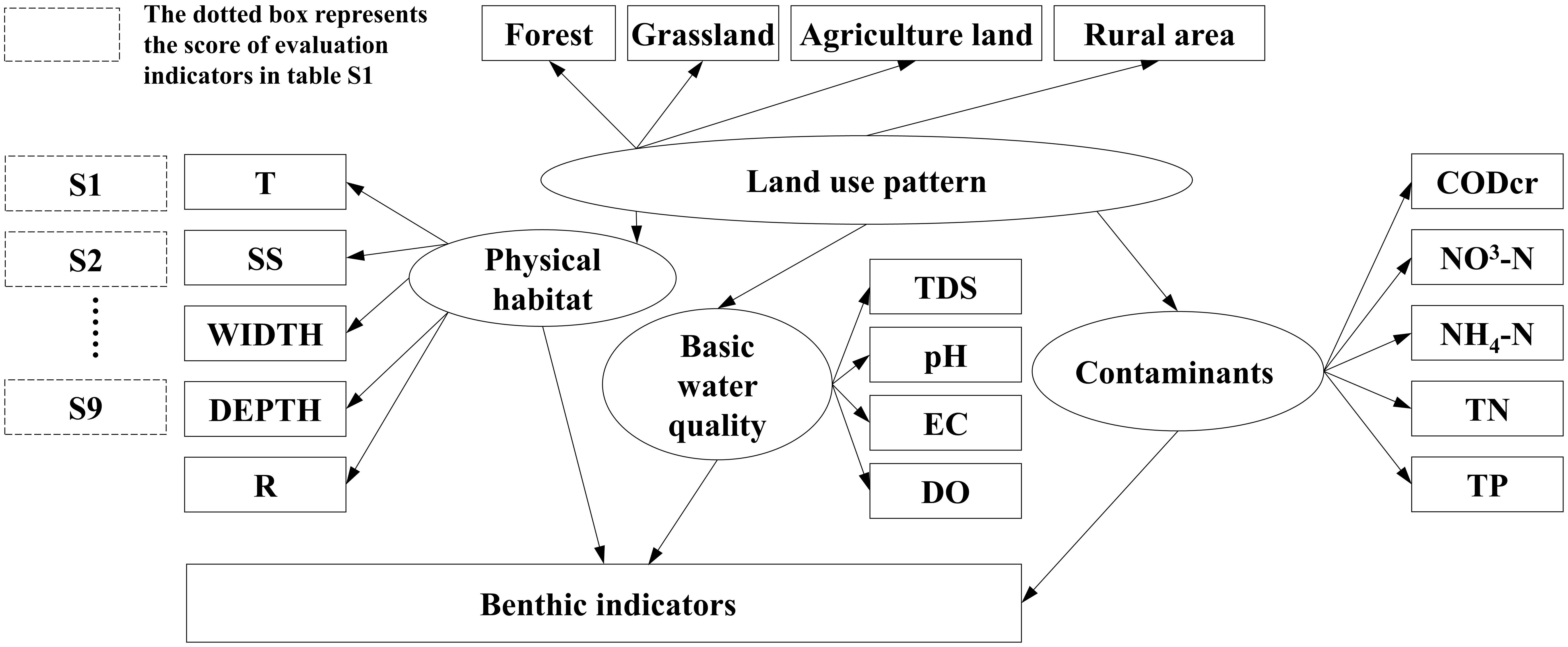
Figure 3 Diagrammatic representation of the study’s conceptual model. Rectangles represent the observation variables and ellipses represent the latent variables. To satisfy the normality assumption of the structural equation model (SEM), a log(x + 1) transformation was applied to parameters S1–S10. The transformed data for S1–S10, along with other physical habitat and water quality variables that underwent the same transformation, were then input into the SEM for analysis.
Following creation of the conceptual model, observational data were incorporated into the models. Model refinement was achieved by adjusting the Modified Index (MI) by adding or removing variables to better align the theoretical models with the observational data. The models’ reliability was verified using Chi-square (χ2), root mean square error of approximation (RMSEA), non-centrality parameter (NC), adjusted goodness-of-fit index (AGFI), and comparative fit index (CFI) (Bentler and Bonett, 1980; Browne and Cudeck, 1992). R2 is used to interpret the macroinvertebrate index, while Pc delineates the impact of different paths. Common grounds for model adjustments include insufficient ecological data, non-compliance with normality assumptions, autocorrelation between different environmental factors, and model definition inconsistency with the actual situation.
4 Results
4.1 Identification of environment and physical habitat impacts
At the spatial extent of the river, we predominantly focused on the impacts of the water environment and physical habitat on macroinvertebrate assemblages. Among the correlations between land use and water quality, temperature exhibited significant correlations with iFLS_km_agriculture% (R = 0.575, p< 0.01), iFLS_km_rural% (R = 0.597, p< 0.01), iFLO_km_rural% (R = 0.541, p< 0.01), and iFLO_m_rural% (R = 0.526, p< 0.01). Moreover, EC exhibited strong associations with iFLO_km_rural% (R = 0.520, p< 0.01) and iFLO_km_rural% (R = 0.52, p< 0.01). Furthermore, TDS demonstrated significant correlations with iFLO_km_rural% (R = 0.474, p< 0.01) and iFLO_m_rural% (R = 0.425, p< 0.01).
Informed by the correlation analysis between water environment and physical habitat, the preliminary findings showed a close correlation between TDS and T (R = 0.669, p< 0.01), EC (R = 0.837, p< 0.01), and TN (R = 0.515, p< 0.01), prompting its exclusion in the RDA. Additionally, macroinvertebrate species with a low frequency of occurrence (less than 5%) were omitted at each site. The RDA results indicated substantial eigenvalues on the first and second axes (values of 0.205 and 0.065 respectively) (Table 2), accounting for 30.7% of the total variation in accumulated species variance. The arrows in Figure 4 represent the significant water environmental and physical habitat factors with an impact on macroinvertebrate communities.
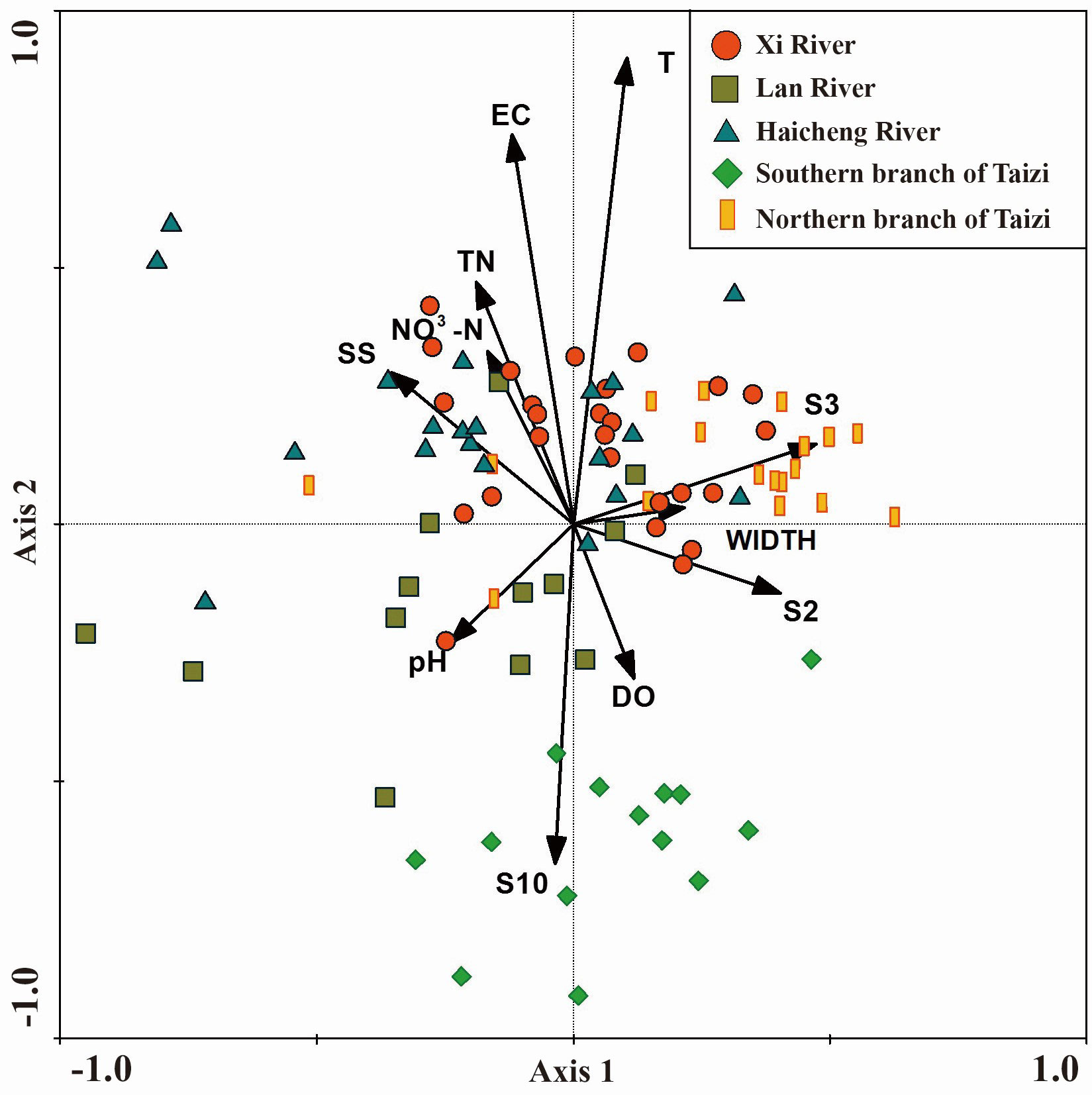
Figure 4 Redundancy analysis (RDA) plot, illustrating the relationships between benthic assemblages and water environment and habitat variables. The arrow direction denotes positive and negative correlations between each factor and the macroinvertebrate distribution, while the length of the arrow indicates the strength of the correlation. The arrow sizes represent the correlation between each factor and sorting axis.
The Monte Carlo test demonstrated that all axis interpretation rates were significant (F = 2.873, p< 0.001). Major contributors to the first axis were S2 (habitat complexity) (F = 2.735, p< 0.001). The second axis was significantly influenced by temperature (F = 7.838, p< 0.001), EC (F = 2.364, p< 0.01), and TN (F = 2.033, p< 0.01).
Therefore, our results demonstrated that variations in the macroinvertebrate community are primarily driven by environmental differences and human impacts in the tributaries (Figure 4). A clear divergence was noted in the macroinvertebrate assemblage distribution across spatial extents. In the northern branch of the Taizi River, the distribution focused in the first quadrant, primarily influenced by S2, whereas in the southern section, the distribution was concentrated in the third and fourth quadrants. In the Xi and Haicheng rivers, the macroinvertebrate assemblage distribution was focused in the first and second quadrants, with a greater influence from T, EC, and TN. However, in the Lan River, the distribution was primarily in the third quadrant, largely influenced by pH. Overall, the RDA findings indicated that habitat complexity constituted the main physical habitat factor directly affecting the macroinvertebrate distribution. Temperature, EC, and TN were the major water quality factors influencing the macroinvertebrate distribution.
4.2 Impact of landscape metrics on macroinvertebrate indices
Multiple linear stepwise regression was employed with land use landscape metrics and macroinvertebrate biological indices. We utilized a diverse set of landscape indicators to better understand how different land use patterns influence the macroinvertebrate distribution along river pathway distance gradients (Table 3). The B_ metric underscores the prominence of agriculture, highlighting its protective zones and interactions around water bodies. As we shifted our focus to iFLO and iFLS, which measure the distance gradients to the stream outlet and nearest stream, respectively, the influence of rural landscapes became increasingly evident. In contrast, the P_ metric offers a more holistic perspective on the overall pattern, which recognizes the influence of rural landscapes while simultaneously capturing the impact of forested areas.
Macroinvertebrate indices also highlight differences among tributaries. The B-IBI showed that the north and south branches exhibit good biological integrity, suggesting a healthy river state. However, as the river flows downstream, with the expansion of urbanized areas and agricultural land use, the health status of the sampling sites progressively deteriorated. The Haicheng River comprised the poorest assessment indicators, which further corroborated the RDA findings.
In evaluating the relationship between landscape metrics and bioindicators, it became evident that certain metrics played a pivotal role in explaining macroinvertebrate biological indices. The optimal land use landscape metrics varied among the indices B-IBI, ASPT, and H’. Specifically, iFLO_km had the highest explanatory power for B-IBI (R2 = 0.382, p< 0.001). For ASPT, both iFLO_km and P had similar explanatory power (R2 = 0.371, p< 0.001). The optimal landscape metric for H’ was the lumped land use percentage (P), with an R2 value of 0.172, which was significant at p< 0.0011. However, this explanatory power was notably weaker compared to that for B-IBI and ASPT. Additionally, under the optimal landscape for B-IBI, ASPT, and H’, the predictive variables were predominantly rural.
4.3 Multi-scale pathways identified by SEMs
Based on the RDA and regression analysis results, 24 SEMs of B_IBI, ASPT, and H’ were developed; these models and their adaptability indicators are presented in Table 4. Of the 24 models, 11 exhibited a good fit (p > 0.05). The errors in these models were all below 0.06, indicating that the latent variables in the models were properly fitted and explained. The most interpretable models for B_IBI, ASPT, and H’ were then selected for more detailed analysis. The SEM-BIBI, SEM-ASPT, and SEM-H’ models, along with the standardized Pc, are depicted in Figure 5. The landscape pattern metric with the highest interpretability for macroinvertebrate indices was iFLO_m.
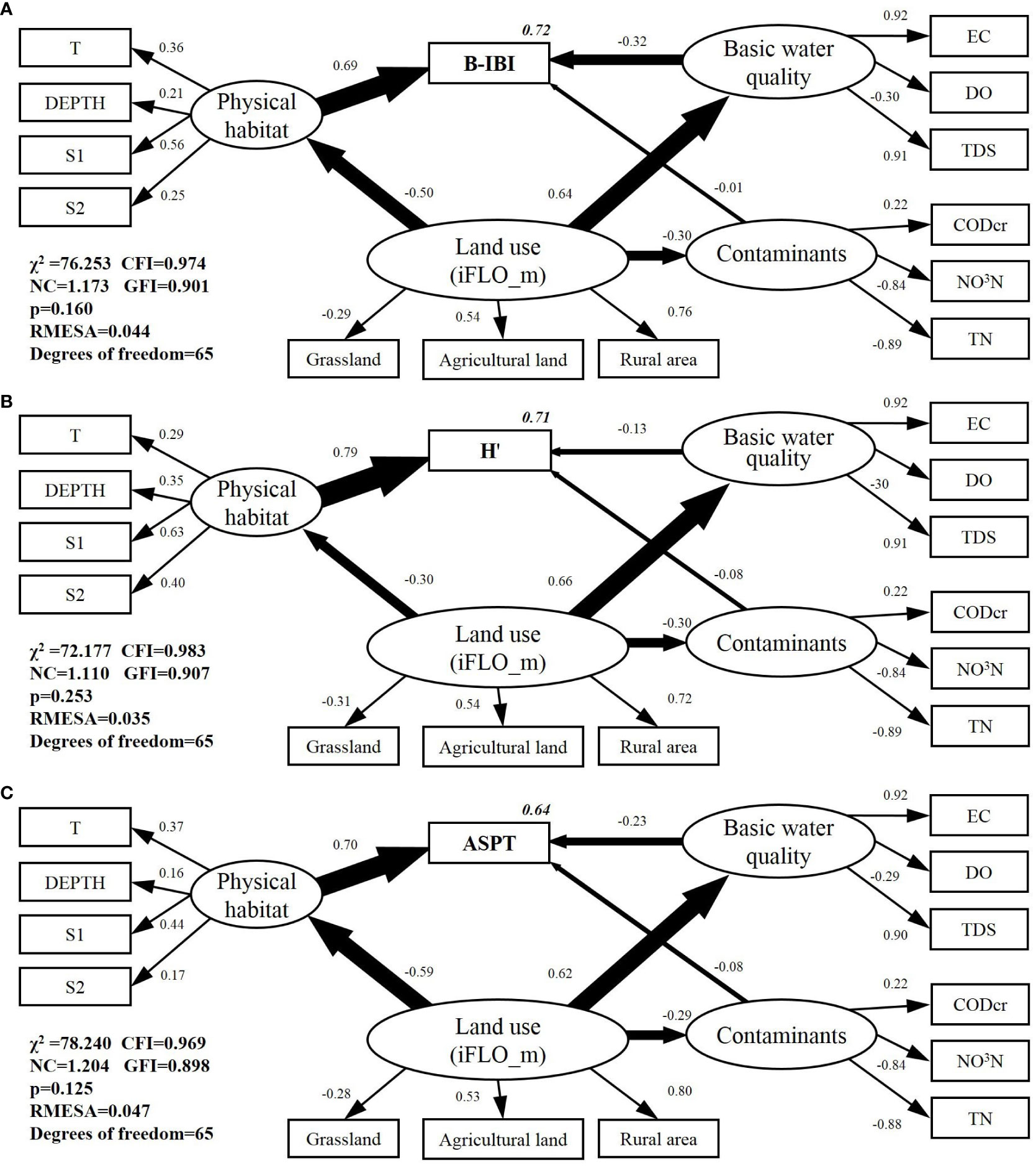
Figure 5 Structural equation models (SEMs) for biological indicators: (A) SEM-BIBI, (B) SEM-H’, (C) SEM-ASPT. (Rectangles represent the observation variables, ellipses represent the latent variables, and circles represent the error term for each variable. The number on the single arrow indicates the path coefficient, with + and – representing a positive and negative correlation, respectively. The number on the box represents the explain ability of the model (R2). S1 and S2 represent substrate and habitat complexity parameters, respectively, defined in Table S1.
The SEM-BIBI model accounted for 76% of the variation in B-IBI. From the viewpoint of impact pathways, land use patterns primarily affect macroinvertebrates by modifying their physical habitat conditions (Pc = –0.41), largely focusing on habitat complexity (0.53), combined speed-depth characteristics (0.24), and water depth (0.21). The main influence of land use patterns depends on the river’s basic water quality (Pc = 0.53), which further impacts the B-IBI (Pc = -0.33). Nutrient and organic pollution factors, such as NO3-N and TN, were mainly influenced by land use (Pc = –0.24), but exert a limited impact on B-IBI (Pc = –0.01). With respect to land use pattern metrics, the rural area showed the largest contribution to the land use pattern based on the iFLO_m metric (0.92), followed by agricultural land (0.46) and grassland (–0.33).
The SEM-H’ model explained 70% of the variance in the Shannon–Wiener index. Land use has a major impact on the physical habitat (Pc = –0.28), thereby affecting macroinvertebrates. The primary areas of influence include habitat complexity (0.65), water depth (0.36), and the combined characteristic of speed and depth (0.41). Land use patterns primarily influence the river’s basic water quality (Pc = 0.61), whereas water quality deterioration only demonstrated a minor effect on the biological index (Pc = –0.14). Nutrient and organic pollution were also influenced by land use patterns (Pc = –0.30), although their impact on the Shannon–Wiener index was minimal (Pc = 0.07). Using the iFLO_m metric, the largest contribution from the land use pattern was derived from the rural residential area (0.84), followed by agricultural land (0.51) and grassland (–0.23).
Overall, the SEM-ASPT model accounted for 66% of the variation in the ASPT. Consistently, land use patterns mainly impact macroinvertebrates through modifications to physical habitat conditions (Pc = –0.51), primarily emphasizing habitat complexity (0.41), water depth (0.15), and the combined characteristic of speed and depth (0.15). Land use also showed an influence on the river’s basic water quality (Pc = 0.52), with EC (0.93) and TDS (0.90) being the most significant contributors. Nutrient and organic pollution, influenced by land use patterns (Pc = –0.24), exert minimal impacts on the ASPT (Pc = –0.07). According to the iFLO_m metric, the rural residential area demonstrated the highest contribution to the land use pattern (0.94), followed by agricultural land (0.46) and grassland (–0.29).
5 Discussion
5.1 Land use pattern metrics
This study utilized lumped and IDW metrics to examine the influence of land use on environmental and physical factors affecting macroinvertebrate communities at various river ecosystem spatial scales. The findings were particularly notable in comparing the explanatory power (R2) of multiple stepwise regression (Table 3) with that of SEM (Table 4). The results from multivariate stepwise regression highlighted P and iFLO_km as the most explanatory metrics. However, SEM analysis revealed iFLO_m and iFLO_km as the top predictors, followed by iFLS_m, iFLS_km, Buffer metrics, with P showing the lowest explanatory strength.
The landscape metrics (iFLO_m and IFLO_km) with inverse distance weights showed commendable explanatory ability, whereas the lumped attribute P showed a divergence according to the model used for evaluation. As a fundamental and widely used landscape descriptor, P metrics concentrate on analyzing the proportions of different land use types. This analysis is crucial for understanding ecological processes and for identifying the specific effects of various land use types on related factors. (Mello et al., 2018). According to Table 3, P metrics exhibit robust explanatory capacity for H’ (0.172) and ASPT (0.371). Moreover, the capability of P metrics to interpret B-IBI (0.363) is only slightly less effective than that of IDW metrics (0.382), underscoring the broad applicability of P.
However, P metrics exhibited a substantial difference in predictive ability according to the various indices in SEMs. The explanatory capacity of P for the biological index B-IBI did not show improvement in the SEM (0.35) compared to multiple regression results (0.363); a similar effect was observed for the ASPT and H’ indices. This implies a low upper limit for the explanatory ability of P, indicating that an SEM cannot further enhance this ability of P metrics. Conversely, SEM analysis revealed an improved explanatory capacity for the ‘Buffer’ attribute, suggesting its greater importance for benthic organisms compared to the broader impact of the entire basin. However, determining the most crucial range of the buffer zone still requires further investigation.
While the efficacy of iFLO_km and iFLO_m metrics differed among methods, they were generally more effective than other landscape metrics in elucidating biological indices. Multiple regression analysis indicated that iFLO_km had the highest interpretative strength for B-IBI (0.382) and ASPT (0.371). In SEMs, however, iFLO_m models exhibited slightly better performance than iFLO_km, albeit not significantly so. In SEMs, iFLO_m and iFLO_km’s explanatory abilities were 47–63% greater compared to multiple regression, demonstrating their substantial benefits. Of all eight landscape metrics tested, only the SEM using iFLS_m metrics demonstrated a high level of fitness, with its capacity to interpret H’ being strong (0.70), closely followed by iFLO_m (0.69). Hence, selecting the most suitable landscape metrics for accurate ecological description in a specific area requires thorough verification and comparison using diverse methodologies.
By integrating the statistical results with existing research theories, SEM can precisely describe ecological conditions. This method underscores the comparative advantage of improved landscape metrics. Peterson et al. (2011) corroborate that the use of ameliorated land use landscape metrics can amplify the description ability for river habitats. This is congruent with our original intent to perpetually enhance landscape metrics. Consequently, employing SEM brings analytical approaches and systems closer to the dynamics of natural ecology, thereby enhancing the accuracy and reliability of ecological description.
5.2 Multiple pathways of the SEMs and management recommendations
This research utilized SEM to explore the relationship between macroinvertebrates and environmental variables at different spatial extents (Figure 5). In the TRB, the main pathway from land use (rural and agriculture) to biological indices was through physical habitat factors (habitat complexity, speed and depth) to benthic indicators. A secondary pathway connected these land use types to water quality parameters (electrical conductivity and total dissolved solids), impacting benthic indicators.
In the context of basin-wide spatial extents, the presence of rural and agricultural lands plays a crucial role in shaping macroinvertebrate populations. It predominantly influences their distribution by modifying the river channel, physical habitat, and water quality in riparian zones. The influence of rural areas and agricultural land on macroinvertebrates has been widely investigated across different regions. Among various environmental factors examined, Zhang et al. (2018) found that escalating agriculture activities led to a decrease in richness and diversity of pollution-sensitive macroinvertebrate species. Probst et al. (2005) concluded that agricultural runoff significantly contributes to biological assemblage variations. They noted that increased agricultural land often leads to reduced vegetation cover, which affects the runoff process. This change typically results in expanded shallow water areas in the river’s biological habitat, thereby simplifying the habitat structure (Allan, 2004). The influence of rural areas on macroinvertebrates is largely due to human activities, as pollution from these activities significantly alters water quality. Water pollution therefore leads to changes in physical and chemical variables like conductivity, turbidity, and total suspended solids, which in turn affect benthic organism assemblages. Similar responses of benthic assemblages to pollution and habitat alterations have been observed in various studies (Minaya et al., 2013; Boehme et al., 2016), which align with our results.
We employed data related to water quality, nutrient and organic pollution, habitat, and biological indicators to investigate the characteristics of the five tributaries on the Taizi River. The RDA results illustrated that physical habitat factors (S2, S3, S10) primarily govern the macroinvertebrate assemblage distribution. Following a substantial period of evolution, the riverbeds manifest diverse geological characteristics across the five rivers. Aquatic vegetation, litter, fallen wood, inverted embankments, and boulders enrich a variety of habitats. The absence of arable land, coupled with nutrient interception and high nutrient content, creates ideal conditions for benthic habitats. This is particularly evident in the northern and southern branches of the Taizi River, near the Dadi Forest Park, an area characterized by lush vegetation and excellent water quality.
However, analysis of the correlation between land use patterns and water quality has inherent limitations. Water quality is influenced by the instantaneous effects of point-source emissions and the combined effects of both point and non-point sources; these often demonstrate a non-linear relationship with static land use patterns and singular water quality test data (Wu et al., 2012). Within the TRB, conductivity and TN emerged as the principal water quality factors affecting macroinvertebrates, notably in the Xi River and Haicheng River. This effect is largely attributed to urban and industrial activities within the region, particularly the presence of petrochemical, electroplating, and mining factories near the city spanning the middle and lower reaches of the Taizi River (Qu et al., 2017; Wang et al., 2018). Metal mines and heavy industries are significant sources of pollution. The chemicals from these industries, released through wastewater discharge, affect the distribution of macroinvertebrate communities in the river.
The comparison of landscape metrics underscored that rural and agricultural areas closer to the river more significantly influence river water quality and biological habitats. This influence is especially pronounced in terms of benthic biodiversity. This indicates that the efficacious management of riparian areas within rural and agricultural lands forms the key to reviving the health of river ecosystems. A judicious approach to management could balance the dual goals of pollution control and biodiversity conservation. For instance, a study by McCracken et al. (2012) in the United Kingdom suggested that when the farm fence set aside for river protection is wider than 5.4 m, there is a marked increase in aquatic invertebrate diversity. Similarly, Hughes and Vadas (2021) highlighted the influence of agriculture on the quality of streams and rivers in the western United States. Accordingly, they proposed that policies encouraging the preservation and restoration of riparian zones would increase the chances of improved biological conditions in streams. Sweeney and Newbold (2014) proposed that the ideal buffer zone width could vary based on the location; they recommended a buffer width of at least 30 m to optimally protect the water quality and ecological health of small streams. However, in the TRB and northern China, farmlands are typically less than 5.4 meters from rivers, a practice stemming from traditional farming habits. This proximity, along with the characteristics of the surrounding landscape, notably affects riparian zones in agricultural and residential areas (Zhang et al., 2013). Consequently, it is recommended that the government and agricultural management department establish a buffer protection zone along the riverbanks of agricultural lands to enhance the water quality and ecological health of the TRB. Given the effects of large-scale agriculture and proximity of rural areas to rivers on benthic organisms, we recommend establishing 100-m wide riparian buffers. However, when implementing these measures, the impacts on other regional wildlife, such as fish and wild animals, should be considered to determine the optimal buffer range.
6 Summary and conclusions
Utilizing a comprehensive dataset from the TRB, including macroinvertebrates, water environmental factors, and physical habitat, this study effectively used SEMs to examine land use effects on macroinvertebrates across multiple scales. The findings provide several key insights with practical implications for enhanced management.
Along the spatial extent of the river, macroinvertebrate assemblages were significantly influenced by water and habitat parameters. In particular, temperature, EC, TN, and habitat complexity emerged as dominant influencers across different river reaches. Correlation and regression analyses in this study showed that land use landscape metrics, especially those related to rural areas, have a significant impact on macroinvertebrate biological indices along river pathways. These metrics varied in their explanatory power for different indices like B-IBI, ASPT, and H’.
Of the 24 structured SEMs, 11 exhibited an exemplary model fit. Collectively, these models articulate a narrative where land use, especially in rural areas, affects macroinvertebrates by altering physical habitats and water quality. In this analysis, the iFLO_m metric proved particularly effective, exhibiting strong interpretative capabilities in our refined SEM models. Its explanatory power for SEM-BIBI, SEM-H’, and SEM-ASPT was observed to be 76%, 70%, and 66%, respectively. The primary pathway of influence within the TRB is delineated as: land use (predominantly rural and agricultural) feeding into physical habitat (habitat complexity, combined characteristic of speed and depth), which in turn affects benthic indicators. A secondary pathway charts a course from land use through water quality (electrical conductivity and total dissolved solids), culminating in benthic variations.
SEMs have proven to be invaluable in discerning the complex relationships between land use and macroinvertebrates. These diverse land use descriptions underscore that IDW metrics, especially iFLO_m and iFLS_m, offer substantial advantages in deciphering the ecological status of river health under various impact pathways. Moreover, the present findings further emphasize the need for verification of diverse landscape metrics, demonstrating the benefits of structural equation modeling in delivering a refined ecological understanding.
Overall, our results demonstrate that close-proximity rural and agricultural land use in the TRB significantly shape macroinvertebrate distributions by altering river habitats and water quality. This highlights the need for the construction of strategic riparian buffer zones. Specifically, from the perspective of macroinvertebrates in the TRB, we recommend establishing 100-m wide riparian buffers. Buffer zones that take into account a broader range of biological needs will further enhance the ecosystem health of the river basin.
Data availability statement
The raw data supporting the conclusions of this article will be made available by the authors, without undue reservation.
Author contributions
KM: Conceptualization, Data curation, Investigation, Methodology, Software, Visualization, Writing – original draft. HZ: Funding acquisition, Methodology, Supervision, Writing – review & editing. MZ: Writing – review & editing. XQ: Resources, Supervision, Writing – review & editing. NW: Writing – review & editing.
Funding
The author(s) declare financial support was received for the research, authorship, and/or publication of this article. This work was supported by the National Key R&D Program of China (No. 2021YFC3200102), the national Natural Science Foundation of China(No. 32071588, No. 41501204, No.42201040), and the China Postdoctoral Science Foundation (2023M733006).
Acknowledgments
We thank Daming He for supporting Kai Ma in research. We would like to clarify that, based on the nature of our research and institutional guidelines, this study did not require ethics approval.
Conflict of interest
The authors declare that the research was conducted in the absence of any commercial or financial relationships that could be construed as a potential conflict of interest.
The author(s) declared that they were an editorial board member of Frontiers, at the time of submission. This had no impact on the peer review process and the final decision.
Publisher’s note
All claims expressed in this article are solely those of the authors and do not necessarily represent those of their affiliated organizations, or those of the publisher, the editors and the reviewers. Any product that may be evaluated in this article, or claim that may be made by its manufacturer, is not guaranteed or endorsed by the publisher.
Supplementary material
The Supplementary Material for this article can be found online at: https://www.frontiersin.org/articles/10.3389/fevo.2023.1292721/full#supplementary-material
Text S1 | The formulas for calculating ASPT and H’.
Supplementary Table 1 | Formula for calculating of the core attributes of B-IBI.
Supplementary Table 2 | The evaluation indicators and evaluation criteria of river habitat.
References
Allan J. D. (2004). Landscapes and riverscapes: the influence of land use on stream ecosystems. Annu. Rev. Ecol. Evol. System. 35 (1), 257–284. doi: 10.1146/annurev.ecolsys.35.120202.110122
Alvarenga L. R. P., Pompeu P. S., Leal C. G., Hughes R. M., Fagundes D. C., Leitão R. P. (2021). Land-use changes affect the functional structure of stream fish assemblages in the Brazilian Savanna. Neotrop. ichthyol. 19, e210035. doi: 10.1590/1982-0224-2021-0035
Atasoy M., Palmquist R. B., Phaneuf D. J. (2006). Estimating the effects of urban residential development on water quality using microdata. J. Environ. Manage. 79 (4), 399–408. doi: 10.1016/j.jenvman.2005.07.012
Beaumelle L., Vile D., Lamy I., Vandenbulcke F., Gimbert F., Hedde M. (2016). A structural equation model of soil metal bioavailability to earthworms: confronting causal theory and observations using a laboratory exposure to field-contaminated soils. Sci. Total Environ. 569-570, 961–972. doi: 10.1016/j.scitotenv.2016.06.023
Bentler P. M., Bonett D. G. (1980). Significance tests and goodness of fit in the analysis of covariance structures. psychol. Bull. 88 (3), 588–606. doi: 10.1037/0033-2909.88.3.588
Boehme E. A., Zipper C. E., Schoenholtz S. H., Soucek D. J., Timpano A. J. (2016). Temporal dynamics of benthic macroinvertebrate communities and their response to elevated specific conductance in Appalachian coalfield headwater streams. Ecol. Indic. 64, 171–180. doi: 10.1016/j.ecolind.2015.12.020
Browne M. W., Cudeck R. (1992). Alternative ways of assessing model fit. Sociol. Methods Res. 21 (2), 230–258. doi: 10.1177/0049124192021002005
Capmourteres V., Anand M. (2016). Assessing ecological integrity: A multi-scale structural and functional approach using Structural Equation Modeling. Ecol. Indic. 71, 258–269. doi: 10.1016/j.ecolind.2016.07.006
Carlisle D. M., Hawkins C. P. (2008). Land use and the structure of western US stream invertebrate assemblages: predictive models and ecological traits. J. North Am. Benthol. Soc. 27 (4), 986–999. doi: 10.1899/07-176.1
Chen K., Rajper A. R., Hughes R. M., Olson J. R., Wei H., Wang B., et al. (2019). Incorporating functional traits to enhance multimetric index performance and assess land use gradients. Sci. Total Environ. 691, 1005–1015. doi: 10.1016/j.scitotenv.2019.07.047
Chen X., Zhou W., Pickett S. T., Li W., Han L. (2016). Spatial-temporal variations of water quality and its relationship to land use and land cover in Beijing, China. Int. J. Environ. Res. Public Health 13 (5), 449. doi: 10.3390/ijerph13050449
Colas F., Baudoin J.-M., Gob F., Tamisier V., Valette L., Kreutzenberger K., et al. (2017). Scale dependency in the hydromorphological control of a stream ecosystem functioning. Water Res. 115, 60–73. doi: 10.1016/j.watres.2017.01.061
Cui H., Wang L., Zhang J. (2022). Synergistic influence on microbial communities ascribed to copper and tetracycline during aerobic composting: Insights into bacterial and fungal structures. Front. Ecol. Evol. 10. doi: 10.3389/fevo.2022.1019494
Dalu T., Mwedzi T., Wasserman R. J., Madzivanzira T. C., Nhiwatiwa T., Cuthbert R. N. (2022). Land use effects on water quality, habitat, and macroinvertebrate and diatom communities in African highland streams. Sci. Total Environ. 846, 157346. doi: 10.1016/j.scitotenv.2022.157346
Eisenhauer N., Bowker M. A., Grace J. B., Powell J. R. (2015). From patterns to causal understanding: Structural equation modeling (SEM) in soil ecology. Pedobiol. J. Soil Ecol. 58 (2-3), 65–72. doi: 10.1016/j.pedobi.2015.03.002
Eriksen T. E., Brittain J. E., Søli G., Jacobsen D., Goethals P., Friberg N. (2021). A global perspective on the application of riverine macroinvertebrates as biological indicators in Africa, South-Central America, Mexico and Southern Asia. Ecol. Indic. 126, 107609. doi: 10.1016/j.ecolind.2021.107609
Fan X., Zhang L., Yuan L., Guo B., Zhang Q., Huang H. (2022). Urbanization and water quality dynamics and their spatial correlation in coastal margins of mainland China. Ecol. Indic. 138, 108812. doi: 10.1016/j.ecolind.2022.108812
Fan Y., Chen J., Shirkey G., John R., Wu S. R., Park H., et al. (2016). Applications of structural equation modeling (SEM) in ecological studies: an updated review. Ecol. Processes 5 (1), 19. doi: 10.1186/s13717-016-0063-3
Feio M. J., Hughes R. M., Serra S. R. Q., Nichols S. J., Kefford B. J., Lintermans M., et al. (2023). Fish and macroinvertebrate assemblages reveal extensive degradation of the world’s rivers. Global Change Biol. 29, 355–374. doi: 10.1111/gcb.16439
Fierro P., Bertrán C., Tapia J., Hauenstein E., Peña-Cortés F., Vergara C., et al. (2017). Effects of local land-use on riparian vegetation, water quality, and the functional organization of macroinvertebrate assemblages. Sci. Total Environ. 609 (7), 724–734. doi: 10.1016/j.scitotenv.2017.07.197
Frissell C. A., Liss W. J., Warren C. E., Hurley M. D. (1986). A hierarchical framework for stream habitat classification: Viewing streams in a watershed context. Environ. Manage. 10 (2), 199–214. doi: 10.1007/BF01867358
Gove N. E., Edwards R. T., Conquest L. L. (2010). Effects of scale on land use and water quality relationships: A longitudinal basin-wide perspective. Jawra J. Am. Water Resour. Assoc. 37 (6), 1721–1734. doi: 10.1111/j.1752-1688.2001.tb03672.x
He S., Soininen J., Chen K., Wang B. (2020). Environmental factors override dispersal-related factors in shaping diatom and macroinvertebrate communities within stream networks in China. Front. Ecol. Evol. 8. doi: 10.3389/fevo.2020.00141
Herlihy A. T., Sifneos J. C., Hughes R. M., Peck D. V., Mitchell R. M. (2020). The relation of lotic fish and benthic macroinvertebrate condition indices to environmental factors across the conterminous USA. Ecol. Indic. 112, 105958. doi: 10.1016/j.ecolind.2019.105958
Hettetronquart N., Oberdorff T., Tales E., Zahm A., Belliard J. (2017). Biological impacts of local vs. regional land use on a small tributary of the Seine River (France): insights from a food web approach based on stable isotopes. Environ. Sci. Pollut. Res. 25, 23583–23594. doi: 10.1007/s11356-017-8771-5
Hughes R. M., Herlihy A. T., Comeleo R., Peck D. V., Mitchell R. M., Paulsen S. G. (2023). Patterns in and predictors of stream and river macroinvertebrate genera and fish species richness across the conterminous USA. Knowl. Manage. Aquat. Ecosyst. 424 (19), 1-16. doi: 10.1051/kmae/2023014
Hughes R. M., Herlihy A. T., Kaufmann P. R. (2010). An evaluation of qualitative indexes of physical habitat applied to agricultural streams in ten U.S. states. JAWRA J. Am. Water Resour. Assoc. 46, 792–806. doi: 10.1111/j.1752-1688.2010.00455.x
Hughes R. M., Vadas R. L. (2021). Agricultural effects on streams and rivers: A Western USA focus. Water 13, 1901. doi: 10.3390/w13141901
Jia H., Luo P., Yang H., Luo C., Li H., Cheng Y., et al. (2023). Constructing an indices system for evaluating the ecological integrity of forests in western Sichuan, China based on structural equation modeling. Ecol. Indic. 146, 109745. doi: 10.1016/j.ecolind.2022.109745
Kaufmann P.R., Levine P., Robison E.G., Seeliger C., D.V., Peck. (1999). Quantifying physical habitat in wadeable streams. U.S. Environmental Protection Agency (Washington, D.C.). EPA/620/R-99/003
Kaufmann P. R., Hughes R. M., Paulsen S. G., Peck D. V., Seeliger C. W., Weber M. H., et al. (2022). Physical habitat in conterminous US streams and rivers, Part 1: Geoclimatic controls and anthropogenic alteration. Ecol. Indic. 141, 109046. doi: 10.1016/j.ecolind.2022.109046
Kefford B. J., Botwe P. K., Brooks A. J., Kunz S., Marchant R., Maxwell S., et al. (2020). An integrated database of stream macroinvertebrate traits for Australia: concept and application. Ecol. Indic. 114, 106280. doi: 10.1016/j.ecolind.2020.106280
Leitão R. P., Zuanon J., Mouillot D., Leal C. G., Hughes R. M., Kaufmann P. R., et al. (2018). Disentangling the pathways of land use impacts on the functional structure of fish assemblages in Amazon streams. Ecography 41, 219–232. doi: 10.1111/ecog.02845
Li C., Sun G., Caldwell P. V., Cohen E., Fang Y., Zhang Y., et al. (2020). Impacts of urbanization on watershed water balances across the conterminous United States. Water Resour. Res. 56. doi: 10.1029/2019WR026574
Liu G., Lin Z., Qi X., Wang Y., Wang Y., Jiang W., et al. (2023). Environmental filtering, spatial processes and biotic interactions jointly shape different traits communities of stream macroinvertebrates. Front. Ecol. Evol. 11. doi: 10.3389/fevo.2023.1196296
Lorion C. M., Kennedy B. P. (2010). Relationships between deforestation, riparian forest buffers and benthic macroinvertebrates in neotropical headwater streams. Freshw. Biol. 54 (1), 165–180. doi: 10.1111/j.1365-2427.2008.02092.x
Luo Q., Chiu M.-C., Tan L., Cai Q. (2021). An environmental flow framework for riverine macroinvertebrates during dry and wet seasons through non-linear ecological modeling. Front. Ecol. Evol. 9. doi: 10.3389/fevo.2021.734716
Luo K., Hu X., He Q., Wu Z., Cheng H., Hu Z., et al. (2018). Impacts of rapid urbanization on the water quality and macroinvertebrate communities of streams: A case study in Liangjiang New Area, China. Sci. Total Environ. 621 (10), 1601. doi: 10.1016/j.scitotenv.2017.10.068
MacCallum R. C., Austin J. T. (2000). Applications of structural equation modeling in psychological research. Annu. Rev. Psychol. 51, 201–226. doi: 10.1146/annurev.psych.51.1.201
Macedo D. R., Hughes R. M., Ligeiro R., Ferreira W. R., Castro M. A., Junqueira N. T., et al. (2014). The relative influence of catchment and site variables on fish and macroinvertebrate richness in cerrado biome streams. Landscape Ecol. 29, 1001–1016. doi: 10.1007/s10980-014-0036-9
McCracken D. I., Cole L. J., Harrison W., Robertson D. (2012). Improving the farmland biodiversity value of riparian buffer strips: conflicts and compromises. J. Environ. Qual. 41 (2), 355–363. doi: 10.2134/jeq2010.0532
Mello K. D., Valente R. A., Randhir T. O., Santos A. C. A. D., Vettorazzi C. A. (2018). Effects of land use and land cover on water quality of low-order streams in Southeastern Brazil: Watershed versus riparian zone. Catena 167, 130–138. doi: 10.1016/j.catena.2018.04.027
Merritt R. W., Cummins K. W. (1996). An introduction to the aquatic insects of North America. J. North Am. Benthol. Soc. 81 (2), 593–595. doi: 10.2307/1467288
Meyer M. F., Ozersky T., Woo K. H., Shchapov K., Galloway A. W. E., Schram J. B., et al. (2022). A unified dataset of colocated sewage pollution, periphyton, and benthic macroinvertebrate community and food web structure from Lake Baikal (Siberia). Limnol. Oceanogr. Lett. 7, 62–79. doi: 10.1002/lol2.10219
Minaya V., Mcclain M. E., Moog O., Omengo F., Singer G. A. (2013). Scale-dependent effects of rural activities on benthic macroinvertebrates and physico-chemical characteristics in headwater streams of the Mara River, Kenya. Ecol. Indic. 32 (3), 116–122. doi: 10.1016/j.ecolind.2013.03.011
Morse J. C., Yang L., Tian L. (1984). Aquatic insects of China useful for monitoring water quality (Hohai University Press).
O’Brien A., Townsend K., Hale R., Sharley D., Pettigrove V. (2016). How is ecosystem health defined and measured? A critical review of freshwater and estuarine studies. Ecol. Indic. 69, 722–729. doi: 10.1016/j.ecolind.2016.05.004
Palt M., Le Gall M., Piffady J., Hering D., Kail J. (2022). A metric-based analysis on the effects of riparian and catchment landuse on macroinvertebrates. Sci. Total Environ. 816, 151590. doi: 10.1016/j.scitotenv.2021.151590
Peterson E. E., Sheldon F., Darnell R., Bunn S. E., Harch B. D. (2011). A comparison of spatially explicit landscape representation methods and their relationship to stream condition. Freshw. Biol. 56 (3), 590–610. doi: 10.1111/j.1365-2427.2010.02507.x
Probst M., Berenzen N., Lentzengodding A., Schulz R., Liess M. (2005). Linking land use variables and invertebrate taxon richness in small and medium-sized agricultural streams on a landscape level. Ecotoxicol. Environ. Saf. 60 (2), 140–146. doi: 10.1016/j.ecoenv.2004.04.003
Qu X., Liu Z., Zhang Y. (2012). Discussion on the standardized method of reference sites selection for establishing the Benthic-Index of Biotic Integrity. Acta Ecol. Sin. 32 (15), 4661–4672. doi: 10.5846/stxb201107181065
Qu X., Ren Z., Zhang M., Liu X., Peng W. (2017). Sediment heavy metals and benthic diversities in Hun-Tai River, northeast of China. Environ. Sci. pollut. Res. Int. 24 (11), 10662–10673. doi: 10.1007/s11356-017-8642-0
Schilling K. E., Jha M. K., Zhang Y. K., Gassman P. W., Wolter C. F. (2008). Impact of land use and land cover change on the water balance of a large agricultural watershed: historical effects and future directions. Water Resour. Res. 44 (7), 636–639. doi: 10.1029/2007WR006644
Shipley B. (2002). Cause and Correlation in Biology: a user’s guide to path analysis, structural equations, and causal inference. Cambridge Univ. Press 82 (4), 646–649.
Sweeney B. W., Newbold J. D. (2014). Streamside forest buffer width needed to protect stream water quality, habitat, and organisms: A literature review. J. Am. Water Resour. Assoc. 50, 560–584. doi: 10.1111/jawr.12203
Villeneuve B., Piffady J., Valette L., Souchon Y., Usseglio-Polatera P. (2018). Direct and indirect effects of multiple stressors on stream invertebrates across watershed, reach and site scales: A structural equation modelling better informing on hydromorphological impacts. Sci. Total Environ. 612, 660–671. doi: 10.1016/j.scitotenv.2017.08.197
Wallace J. B., Webster J. R. (1996). The role of macroinvertebrates in stream ecosystem function. Annu. Rev. Entomol. 41 (1), 115–139. doi: 10.1146/annurev.en.41.010196.000555
Walsh C. J., Webb J. A. (2014). Spatial weighting of land use and temporal weighting of antecedent discharge improves prediction of stream condition. Landscape Ecol. 29 (7), 1171–1185. doi: 10.1007/s10980-014-0050-y
Wang Q., Chen Q., Yan D., Xin S. (2018). Distribution, ecological risk, and source analysis of heavy metals in sediments of Taizihe River, China. Environ. Earth Sci. 77 (16), 569. doi: 10.1007/s12665-018-7750-6
Wilkins P. M., Cao Y., Heske E. J., Levengood J. M. (2015). Influence of a forest preserve on aquatic macroinvertebrates, habitat quality, and water quality in an urban stream. Urban Ecosyst. 18 (3), 1–18. doi: 10.1007/s11252-015-0464-6
Wu M. Y., Xue L., Jin W. B., Xiong Q. X., Ai T. C., Li B. L. (2012). Modelling the linkage between landscape metrics and water quality indices of hydrological units in Sihu Basin, Hubei Province, China: an allometric model. Proc. Environ. Sci. 13 (10), 2131–2145. doi: 10.1016/j.proenv.2012.01.202
Zhang Y., Cheng L., Tolonen K. E., Yin H., Gao J., Zhang Z., et al. (2018). Substrate degradation and nutrient enrichment structuring macroinvertebrate assemblages in agriculturally dominated Lake Chaohu Basins, China. Sci. Total Environ. 627 (15), 57–66. doi: 10.1016/j.scitotenv.2018.01.232
Zhang H., Wu D., Wang Z., Sun R., Chen L. (2015). The impact of landscape class and patterns at the watershed scale on Benthic Macroinvertebrate Index of Biological Integrity (B-IBI). Acta Ecol. Sin. 19), 6237–6249. doi: 10.5846/stxb201403080395
Zhang Y., Zhao R., Kong W., Geng S., Bentsen C. N., Qu X. (2013). Relationships between macroinvertebrate communities and land use types within different riparian widths in three headwater streams of Taizi River, China. J. Freshw. Ecol. 28 (3), 307–328. doi: 10.1080/02705060.2013.779941
Keywords: land use pattern, macroinvertebrate, structural equation models, IDW, multiple pathways
Citation: Ma K, Zhang H, Zhang M, Qu X and Wu N (2023) Impact of land use on macroinvertebrates from a multiscale perspective: enhancing structural equation models with inverse distance-weighted metrics. Front. Ecol. Evol. 11:1292721. doi: 10.3389/fevo.2023.1292721
Received: 12 September 2023; Accepted: 23 November 2023;
Published: 07 December 2023.
Edited by:
Qiang Wang, Southwest University, ChinaCopyright © 2023 Ma, Zhang, Zhang, Qu and Wu. This is an open-access article distributed under the terms of the Creative Commons Attribution License (CC BY). The use, distribution or reproduction in other forums is permitted, provided the original author(s) and the copyright owner(s) are credited and that the original publication in this journal is cited, in accordance with accepted academic practice. No use, distribution or reproduction is permitted which does not comply with these terms.
*Correspondence: Xiaodong Qu, quxiaodong@iwhr.com