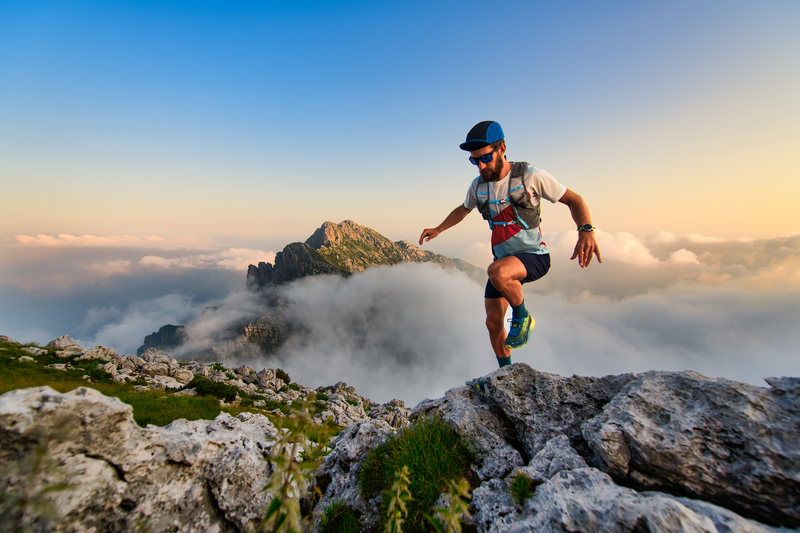
95% of researchers rate our articles as excellent or good
Learn more about the work of our research integrity team to safeguard the quality of each article we publish.
Find out more
ORIGINAL RESEARCH article
Front. Ecol. Evol. , 28 November 2023
Sec. Biogeography and Macroecology
Volume 11 - 2023 | https://doi.org/10.3389/fevo.2023.1277058
This article is part of the Research Topic Global Change Ecology: Threats and Solutions View all 10 articles
Chelydra serpentina, a species introduced to China for aquaculture purposes, is commonly found in its natural habitats within the country. The invasion of C. serpentina poses potential threats to both the biodiversity of China and human health. The potential distribution of C. serpentina has been simulated using the species distribution model – MaxEnt, incorporating global distribution data, climate, and land cover variables. Our simulations encompasses both current conditions and four future climate change scenarios. Currently, the potential distribution is concentrated in central, eastern, and southeastern regions of China, with the central and eastern regions facing the highest risk of invasion. Under future climate change scenarios, the distribution area may expand by 30–90%, and multiple provinces will face a more severe threat of invasion. This study presents the inaugural simulation of the potential invasion range of C. serpentina under current climatic conditions. Moreover, it reveals that climate change is likely to contribute to the expansion of its invasive range, thus furnishing a reference foundation for scientific prevention and control measures. We propose integrating citizen science and eDNA technologies into species monitoring to enhance the efficiency of detecting invasive species. This research has filled the gap in the research on the invasive distribution range of C. serpentina in China and globally, while also providing novel perspectives on the invasion control of this species.
Globally, non-native freshwater turtles have been intentionally or unintentionally introduced into various habitats (Sung et al., 2021), causing damage to local biodiversity and ecosystems through competition (Díaz-Paniagua et al., 2011), hybridization (Ueno et al., 2021), and the spread of parasites (Mendoza-Roldan et al., 2020) and diseases (Bosch et al., 2015). To prevent the further spread of invasive alien species (IAS), employing species distribution models (SDMs) to analyze the current and future ranges of IAS can provide a scientific basis for decision-making (Bertolino et al., 2020).
It is expected that the global surface temperature will continue to increase at least until the middle of the century (Masson-Delmotte et al., 2021). Climate change and biological invasions occur simultaneously in both space and time, and there is a potential for synergistic effects in the future (Stephens et al., 2019). The persistent climate change poses an escalating threat to biodiversity and ecosystems (Watson et al., 2019). Forecasts suggest that global warming will alter the geographical ranges of various species (Duan et al., 2016). The survival of species in response to climate change will likely depend on their dispersal capabilities (Capinha et al., 2013). Species with narrow ecological niches and limited dispersal abilities, including many endangered species, are significantly more vulnerable to environmental changes (Malcolm et al., 2006) compared to species with extensive dispersal abilities (Slatyer et al., 2013) like IAS. IAS are often better adapted to local conditions, outcompeting slower-spreading species and potentially leading to their extinction (Urban et al., 2012).
China’s complex terrain and climatic diversity contribute to its abundant biodiversity compared to other countries at similar latitudes (SEP A (State Environmental Protection Administration), 1998). China has identified over 660 IAS (Xian et al., 2018), including four species from the Testudines order: pond slider turtle (Trachemys scripta), Florida softshell turtle (Apalone ferox), alligator snapping turtle (Macrochelys temminckii), and common snapping turtle (Chelydra serpentina) (Ji, 2023). The original natural range of C. serpentina extends from the southeastern region of Alberta, Canada, eastward in the United States to the east of the 105th meridian, and southwards to the Gulf Coast (Ernst and Lovich, 2009). C. serpentina has been introduced in various countries across Asia (including China, Japan, Singapore), Europe (Germany, the Netherlands, France, Spain), and South America (Mexico, Honduras, Costa Rica, Panama, Ecuador) primarily through the pet and food trade (Koo and Sung, 2020). Around 1997, China began introducing C. serpentina due to its rapid growth, high egg production, substantial meat yield, simple feeding requirements, and low susceptibility to disease. This led to rapid development in aquaculture, and in 2005, the Ministry of Agriculture of the People’s Republic of China endorsed this species for aquaculture promotion (Liu et al., 2007). C. serpentina is frequently discovered in the natural habitats of China and has been featured in various online news outlets (Liu et al., 2021). In the region of Hong Kong, C. serpentina has also been witnessed on no less than 10 occasions (Sung et al., 2021). Therefore, we hold the belief that China is currently confronted with a significant risk of C. serpentina invasion. As apex predators, these formidable creatures exert a significant cascading effect on freshwater ecosystems (Wilbur, 1997; Lovich et al., 2018; Garig et al., 2020). Even their brief visits can induce substantial changes in freshwater communities (Garig et al., 2020).
SDMs are statistical models that utilize observed distribution data to infer species ecological requirements and map their potential distribution (Austin, 2002). They are widely used to quantify species responses to climate change (Araújo et al., 2011; Newbold et al., 2020). This study aims to simulate the potential global and China-specific distributions of C. serpentina by utilizing existing records of its occurrence and high-resolution data on climate warming and water bodies. The study sets forth three distinct research objectives: (1) Assessing the potential distribution of C. serpentina in both current and future climatic conditions; (2) Spatially delineating the contraction and expansion patterns within the distribution range of C. serpentina; (3) Identifying the principal environmental variables that strongly correlate with the distribution of C. serpentina. This study presents the inaugural simulation of the potential invasion range of C. serpentina under current climatic conditions. Additionally, it reveals the impact of climate change on the invasive range of C. serpentina, thus furnishing a reference foundation for scientific prevention and control measures. Moreover, based on the biological characteristics of C. serpentina, suggestions for conducting field investigations have been proposed to enhance monitoring efficiency. This study has filled the gap in the research on the invasive distribution range of C. serpentina in China and globally, while also providing novel perspectives on the invasion control of this species.
We obtained the global occurrence records for C. serpentina from the Global Biodiversity Information Facility (GBIF), accessed on 23 May 2023 (GBIF, 2023). Any records that fell outside the range of bioclimatic variables were excluded. In order to reduce the impact of sampling bias, we performed spatial thinning using the spThin R package (R Version 4.3.0), with a minimum distance of 10km between pairs of sites (Aiello-Lammens et al., 2015). In the end, we obtained 7,897 occurrence records of C. serpentina for our study, of which 97.34% were within the original range (Figure 1). We utilized global occurrence data for model calibration because relying solely on occurrence data from native habitats may lead to an underestimation of potential distribution areas. This is primarily because it overlooks the essential survival strategies species employ to mitigate the effects of environmental changes. These mechanisms include exploiting microclimates, regulating body temperature, adjusting life history traits, and exhibiting evolutionary adaptations (Bonebrake et al., 2014; Faye et al., 2014; Bush et al., 2016). This is particularly relevant for IAS, which often exhibit heightened adaptability (Kolar and Lodge, 2001).
Nineteen global bioclimatic and elevation variables were sourced from the WorldClim databas (Hijmans et al., 2005), derived from the Coupled Model Intercomparison Project Phase 5 (CMIP5). The current climatic variables are based on the long-term average data for 1960–1990. As turtles are aquatic organisms, land cover data of Chen et al. (2022) were utilized to generate data on water bodies and distance from water bodies. The current land cover variables use 2015 data. Water bodies were represented using binary data (1 for water, 0 for non-water) (Gábor et al., 2022), while the distances from water bodies were represented using continuous data. For future predictions, the Beijing Climate Center Climate System Model (BCC-CSM1-1) was employed, as it is widely utilized in the Asian region, and our primary focus is on species invasion issues in China (Dakhil et al., 2021). We selected climate and land cover variables for four “Representative Concentration Pathways” (RCPs: 2.6, 4.5, 6.0, and 8.5) for 2050 and 2070. The four RCPs represent hypothetical future greenhouse gas concentrations, ranging from low to high (van Vuuren et al., 2011). All environmental data were obtained at a high resolution of 2.5 arcminutes (equivalent to 5 km × 5 km). To avoid collinearity in our statistical models, we used Pearson’s rank correlation coefficient to identify and remove highly correlated climate variables (|r| > 0.70) (Dormann et al., 2013). The final environmental variables incorporated into the model and subsequent analysis were Annual Mean Temperature, Mean Diurnal Range, Mean Temperature of Wettest Quarter, Annual Precipitation, Precipitation Seasonality, Precipitation of Warmest Quarter, Elevation, Water Bodies and Distance from Water Bodies.
Maximum Entropy Modeling (MaxEnt) is a useful method to simulate the potential habitat redistribution under climate change, due to high predictive accuracy and strong stability (Phillips et al., 2006; Wisz et al., 2008; West et al., 2016). Parameter optimization is critical for rigorously developing the model, as its purpose is to determine the optimal parameter combination that best represents the phenomenon of interest through finding the best fit to the data (Steele and Werndl, 2013; Jayasinghe and Kumar, 2019). We utilized the R 4.3.0 program and the kuenm package (Cobos et al., 2019) to evaluate candidate solutions on a global scale, encompassing all 31 possible combinations of the feature types (linear = l, quadratic = q, product = p, threshold = t, and hinge = h), and 10 regularization multiplier settings (0.1, 0.3, 0.6, 0.9, 1, 2, 3, 4, 5, and 6). The selection of optimal parameters for modeling hinged on three criteria: statistical significance, predictive power, and model complexity. The Partial ROC method was employed to gauge statistical significance (Peterson et al., 2008). Model performance was appraised via the omission rate (Anderson et al., 2003), whereas model complexity was ascertained by the AICc value (Warren et al., 2010). Previous research has shown that the model with the lowest AICc value (delta AICc = 0) is considered the best (Cobos et al., 2019). The final MaxEnt model’s feature and regularization multiplier were selected based on the optimization process outcomes. The maximum number of background points was set to 10,000. For calibration, 70% of the occurrence records were utilized, with the remaining 30% used for model prediction evaluation. Extrapolation with clamping settings was applied, treating environmental conditions not encountered during model training as if they were at the limits of the training range. This approach holds fitted species responses at constant probabilities outside of training conditions, thereby limiting model extrapolations when projecting into novel environments (Elith et al., 2011). To ensure stable model predictions, the analysis included 10 replicate runs with cross-validation. Logistic regression was chosen as the output format, while all other parameters were set to the MaxEnt model defaults.
The predictive efficacy of the models was evaluated using the True Skill Statistic (TSS). Within the framework of species distribution models (SDMs), TSS is a threshold-dependent measure derived from sensitivity and specificity, or the probability that the model correctly predicts true presences and true absences, respectively (Allouche et al., 2006; Liu et al., 2009). TSS is extensively applied in assessing the predictions of SDMs. Interpretation of TSS values can be categorized as follows: values < 0.4 were poor, 0.4–0.8 useful, and > 0.8 good to excellent (Allouche et al., 2006). The impact of environmental variables on species distribution was assessed through percentage contribution (PC) analysis. PC is an intuitive and continuous measure of variable importance, was the most frequently reported metric (Bradie and Leung, 2017).
We incorporated the results produced by MaxEnt software 3.3.4 (AMNH, New York, NY, USA) into ArcGIS 10.5 (ESRI, Redlands, CA, USA). The conversion tool was used to convert the data into raster data, and then the classification of suitable C. serpentina habitat was carried out using the reclassify tool. The comprehensive probability of suitable distribution regions was classified into four classes: unsuitable area (0 ≤ p ≤ 0.1), low-suitability area (0.1 < p ≤ 0.3), medium-suitability area (0.3 < p ≤ 0.5), and high-suitability area (0.5 < p ≤ 1) (Yan et al., 2021).
We reclassified the MaxEnt output to a binary grid using the 10th training presence logistic threshold values (1 = above the threshold; 0 = below). The 10th training presence logistic threshold categorizes fewer than 10% of the training presence locations as unsuitable area (Capinha et al., 2013). To quantify the extent of changes in C. serpentina habitat distributions under future climate scenarios, we utilized the binary map and Python 2.7-based geographic information system (GIS) toolkits SDMtoolbox 2.5 (Brown et al., 2017). We calculated the change in suitable habitat for C. serpentina in China from the present to the future. The focus is on the central, eastern and southeastern regions, as well as 19 provincial administrative regions that currently have or will have suitable habitats in the future.
From an initial pool of 310 candidate models, only one set of model parameters met our selection criteria. In this candidate model (regularization multiplier = 1, feature class combination = lq), the mean AUC ratio was 1.686, the partial ROC was 0, the omission rate was 0.047, and the AICc was 149045.283. The average TSS value for 10 repeated runs is 0.76 (SD = 0.002), indicating that the MaxEnt model output based on model parameters can accurately simulate the potential distribution of C. serpentina.
On a global scale, our results showed that optimal habitats for C. serpentina, apart from their indigenous regions, are predominantly located in western North America, southeastern and southern South America, southern Europe, a small range of northern central and southern Africa, eastern Asia and southern of Australia and New Zealand in Oceania. (Figure 2A). In China, high and middle suitable habitats are mainly distributed in the central and eastern regions, and there are scattered low suitable habitats in the southeast region (Figure 2B). Currently, the suitable habitat for C. serpentina in China only accounts for 4.34% of the total area (Figure 3). However, in the specific regions we are focusing on, the proportion of suitable habitat reaches 15.05% (Figure 4). Shanghai (91.74%), Anhui (58.69%), Hubei (56.73%), Henan (47.90%) and Jiangsu (42.72%) provinces face the highest risk of C. serpentina invasion, then followed by Jiangxi (23.35%), Zhejiang (18.09%) and Fujian (14.77%) provinces (Table 1). The most important environmental factors affecting the distribution of C. serpentina were Annual Precipitation (31.5%) and Annual Mean Temperature (27.5%), then followed by Precipitation Seasonality (17.6%) and Mean Diurnal Range (11.0%). The other five environmental factors had little effect on C. serpentina distribution (Table 2).
Figure 2 Under current climate conditions, the potential distribution area of C. serpentina in the world (A) and China (B).
Figure 3 Under current climatic conditions, the binary map of potential distribution area of C. serpentina in China.
Figure 4 The changes in the potential distribution of C. serpentina in central, eastern, and southeastern China from the current conditions to 2070 under the climate scenarios of RCP2.6, RCP4.5, RCP6.0, and RCP8.5.
Table 1 Share of current and 2050 C. serpentina habitat areas in 20 provincial administrative regions.
Table 2 Average percentage contribution (PC) of environmental variables in MaxEnt for C. serpentina.
Under various future climatic conditions, the potential geographic distribution range for the four climate change scenarios demonstrates a significant outward expansion. Within China, the future suitable habitat of C. serpentina is projected to expand by 30% to 90%, with new suitable habitat emerging in northeast China (Supplementary Figure 1). In the central, eastern, and southeastern regions of China that are the focus of our study, the changes in the area of suitable habitat align with the national scale changes. The suitable habitats in the central and eastern regions exhibit varying degrees of expansion, while there is some contraction in the southeast. In the RCPs2.6 and 4.5 scenarios, the suitable habitat in 2070 is expected to decrease to some extent compared to 2050, whereas the RCPs6.0 and 8.5 scenarios show an increase (Figure 4). Among the eight provinces with more than 10% suitable habitat, only Fujian Province is projected to experience a downward trend in the future, while the remaining seven provinces are expected to see an upward trend, particularly Jiangsu and Zhejiang Province, which may experience two to three times growth. Among the twelve provinces where the current proportion of suitable habitats is less than 10%, Guangdong and Guangxi will show a downward trend in some scenarios, while the remaining ten provinces will show an upward trend, especially Shaanxi and Hunan provinces, where the proportion of suitable habitats may exceed 20% in the future (Tables 1, 3).
Table 3 Share of current and 2070 C. serpentina habitat areas in 20 provincial administrative regions.
Risk maps play a crucial role in the strategic management of IAS by visually displaying potential settlement areas. The research findings indicate that, given current climate conditions, the potential invasion range of C. serpentina in China is relatively low. But the central, eastern, and southeastern regions of China that we are focusing on have a high risk of invasion currently and in the future. Presently, Shanghai, Anhui, Hubei, Henan, and Jiangsu provinces face a high risk of invasion, while Jiangxi, Zhejiang, and Fujian provinces have a relatively low risk. Existing research reports indicate that only the Hong Kong region in China has recorded dense wild sightings of C. serpentina (Sung et al., 2021). However, Hong Kong does not fall within the suitable habitat range according to our findings. Therefore, we believe that the individuals found in the region were more likely released into the wild during the captive breeding period, though this requires further field investigation. Through online information retrieval, we discovered news reports indicating the presence of C. serpentina in the wild environment across all eight provinces that face a high risk of invasion. C. serpentina has been discovered in China for over a decade (Liu et al., 2021), but the status of its invasion in the country remains entirely unknown. It is imperative that China conducts an urgent investigation into the status of C. serpentina invasion.
Annual precipitation and annual mean temperature are the most significant variables limiting the suitable habitat of the C. serpentina. Established populations of C. serpentina are most frequently occur in ponds, marshes, swamps, peat bogs, shallow bays, river and lake edges, and slow-moving streams (Harding and Mifsud, 1997; Ernst and Lovich, 2009; Paterson et al., 2012). The dependence on water bodies makes annual precipitation the most important factor in predicting the distribution of C. serpentina. Although C. serpentina can hibernate in soil (Brown and Brooks, 1994), or raise their body temperature by sunning their backs (Obbard and Brooks, 1979), their eggs require a hatching temperature of above 20°C to survive, and the appropriate hatching temperature can increase the survival rate of C. serpentina hatchlings (McKnight and Gutzke, 1993; O’Steen, 1998; Rollinson et al., 2012). Consequently, the average annual temperature is also important for the distribution of C. serpentina. The lack of effect of water bodies and distance from water bodies on the distribution of C. serpentina may be due to their extensive nesting and terrestrial migration in wetlands within their range to cope with extreme conditions such as drought (Steen et al., 2010), leading to a lower dependency on water bodies. Another explanation lies in the fact that climate variables can directly provide mechanisms and physiological explanations for species distribution, but land cover are supposed to have little direct physiological relevance for species (Guisan and Zimmermann, 2000; Pearson and Dawson, 2003). Because land cover can only be applied within limited geographical ranges without significant errors, as the same land cover type can correspond to different climate combinations in different regions or time periods (Guisan and Zimmermann, 2000). Hence, the distribution of a species at the continental scale primarily depends on its climate tolerance rather than the type of land cover (Pearson and Dawson, 2003; Thuiller et al., 2004).
Climate change is expected to cause the expansion of suitable habitats in all scenarios. The suitable habitat shows a pattern of significant initial increase with the intensity of climate change, followed by a slight decrease, and ultimately increasing again. The central and eastern regions’ suitable habitats will significantly expand toward the north and south. Among the eight provinces currently facing higher risk, except for Fujian Province, the other seven provinces will indeed face even more severe invasion risks in the future. The suitable habitats in Shaanxi and Hunan provinces may account for more than 20% in the future. We strongly recommend the establishment of long-term monitoring plans for C. serpentina in Shaanxi, Hunan, and the eight provinces currently facing higher risk.
Turtles, symbolizing luck and longevity in Chinese culture, are popular as pets and extensively used for food and traditional medicine (Cheung and Dudgeon, 2006; Zhou and Jiang, 2008). The turtle farming industry in China has seen rapid expansion since the 1990s to meet this significant demand (Wu et al., 2020). Freshwater turtles, especially favored in the pet trade, are frequently abandoned by their owners or intentionally released into the wild during religious events, potentially leading to their establishment in new habitats (Perry et al., 2007; Masin et al., 2014). Despite being a relatively species-poor order, turtles have been introduced more frequently than any other reptiles (Kraus, 2009). The worldwide pet trade has documented at least 61 species of chelonians (Gong et al., 2009). In the Chinese turtle trade market, alien species constitute approximately 67.05% (Hong et al., 2022). As per 2023 data, 43 species of the order Testudines inhabit China, including 39 native and 4 invasive alien species (Ji, 2023). China’s rapidly developing e-commerce industry is facilitating the purchase and release of potential invasive turtle species within the country (Liu et al., 2021). Among the eight provinces currently facing higher risk of invasion, except for Jiangxi Province, the Gross Domestic Product (GDP) of the other seven provinces ranks within the top ten in China, and their developed economy will further promote the establishment of IAS (Gallardo, 2014). Moreover, turtle farms are heavily concentrated in the provinces of Zhejiang, Jiangsu, and Guangdong within these regions (Zhou and Huang, 2007), which heightens the risk of species escaping. The existence of irrigation channels could further aid the dispersal of this specie to more remote areas (Alles et al., 2022). In conclusion, human activities are likely to increase the chances of C. serpentina establishing stable populations in their suitable habitats.
According to the Convention on Biological Diversity, which establishes a “three-stage hierarchical approach,” priority should be given to prevention in high-suitability areas where the species has not yet been recorded (UNEP Mittermeier, 2002). Given the limited knowledge we currently have about the extent of C. serpentina invasion in China, it is essential to prioritize monitoring efforts in the areas indicated by our risk map. Citizen science, which involves public participation in data collection and analysis, has proven to be an effective approach for monitoring biological invasions and detecting IAS at an early stage (Kalaentzis et al., 2023). Although the unique shape traits of C. serpentina make it easier to identify in the wild (Ernst and Lovich, 2009), underwater observation can still be challenging. A solution to this challenge is the use of environmental DNA (eDNA) technology, which detects genetic material from the organisms’ surroundings (Kelly et al., 2014). By combining citizen science engagement with a simple eDNA sampling toolkit, public awareness of invasive organisms such as C. serpentina can be increased, and the efficiency of scientific monitoring can be greatly improved (Zhang et al., 2023). Using trained dogs to detect C. serpentina through olfaction would be an efficient investigation method (Kapfer et al., 2012). During May-August of the year and in the morning and afternoon of the day, C. serpentina is the most active period and the best time to investigate (Obbard and Brooks, 1981). The primary area of investigation is the surrounding vicinity of a permanent body of water (Graves and Anderson, 1987).
For government agencies, effectively managing invasive species from a legal and enforcement perspective is crucial. In America, C. serpentina lacks endangered species classification yet faces significant threat from unprecedented harvesting meeting international market demands. In response to the over-commercial harvest and the resulting decline in C. serpentina populations, certain states in the United States have already taken steps to ban this practice (Colteaux and Johnson, 2017). C. serpentina was listed in the Appendix II of the Washington Convention (CITES, Convention on International Trade in Endangered Species) in 2023. The illegal possession and trafficking of these animals will be punished under the Chinese Wildlife Protection Law. However, the specific implementation of the law is still being explored, especially given the challenges posed by China’s large market for this species.
The reliability of extrapolating SDMs to new ranges and future climates has been extensively debated (Sequeira et al., 2018). One important factor influencing the model’s ability to extrapolate is the number of occurrences used in model calibration. In our study, we utilized 7,897 occurrence data for model calibration. This abundance of occurrence data helps mitigate the influence of outliers during model calibration (Guisan and Thuiller, 2005). We studied a species that has a very wide distribution in North America, and the wide range and many native occurrence data mean that C. serpentina live in a wider range of climates, resulting in a relatively large native niche and a more conservative niche than specialist species (Li et al., 2014; Liu et al., 2020). The conservatism of the climate niche in C. serpentina enhances the reliability of our results when extrapolating the calibrated model to new ranges (Liu et al., 2020). Despite the limitations of SDMs (Pearson and Dawson, 2003; Record et al., 2018), this method remains one of the most promising tools for predicting the potential distribution of invasive species (Bellard et al., 2013; Hill et al., 2017), which is a fundamental objective of conservation biology (Jeschke and Strayer, 2008).
In addition to the controversy over model extrapolation, there are some limitations to our simulation results. We did not consider source populations, biotic interactions, and the dispersal capacity and pathway of C. serpentina. The species has multiple geographic lineages within its native rang (Iverson et al., 1997; Ewert et al., 2005), and if the environmental tolerances of these geographic lineages differ, the introduced population may only occupy a portion of the entire niche (Jeschke and Strayer, 2008; Tingley et al., 2016). As we do not know which geographic lineages were introduced to China, we may have overestimated the suitable habitat for C. serpentina in China. Biotic interactions, such as competition and predation, directly influence the distribution of species at a local scale (Jeschke and Strayer, 2008; Yates et al., 2018). Our results can only be interpreted as suitable habitats under abiotic environmental factors. The dispersal capacity and pathway of invasive species significantly affect the scope and speed of invasion (Hulme, 2009). As C. serpentina has weak dispersal abilities (Obbard and Brooks, 1981), releasing them into the wild is likely the primary way they spread in China (Liu et al., 2021; Sung et al., 2021). Therefore, it is crucial to consider local customs and habits when investigating their distribution. The occurrence data we used on a global scale comes from the GBIF database, which has spatial bias that is challenging to eliminate, even when carrying out spatial sparsity processing (Beck et al., 2014). Furthermore, there are several inherent limitations to using presence-only data (Yackulic et al., 2013).
Our modeling indicates that suitable habitats for C. serpentina are currently concentrated in central, eastern, and southeastern China, with Shanghai, Anhui, Hubei, Henan and Jiangsu provinces facing the highest risk of invasion. Climate change may substantially expand future suitable areas, and multiple provinces will face a more severe threat of invasion. To prevent the establishment and spread of C. serpentina, we urgently recommend implementing monitoring programs that leverage the integration of citizen science, eDNA technology, and other methods, particularly in high-risk regions. Moreover, the government should address legal and enforcement challenges associated with the trade of this species. While our modeling has some inherent limitations, it nonetheless offers valuable scientific support for managing C. serpentina invasion in China. Further research incorporating source populations, biotic interactions and dispersal abilities would provide greater insight into the invasion dynamics of this generalist turtle species. Actively preventing and early detecting C. serpentina is of paramount importance for the conservation of China’s freshwater ecosystems.
The datasets presented in this study can be found in online repositories. The names of the repository/repositories and accession number(s) can be found below: https://doi.org/10.15468/dl.rvmyvk.
Ethical approval was not required for the study involving animals in accordance with the local legislation and institutional requirements because, although we conducted research on vertebrates, we only obtained occurrence data and analyzed it through databases. We did not conduct animal experiments nor had direct contact with animals.
CM: Conceptualization, Data curation, Formal Analysis, Investigation, Methodology, Resources, Software, Validation, Visualization, Writing – original draft, Writing – review & editing. PL: Conceptualization, Funding acquisition, Project administration, Supervision, Writing – review & editing.
The author(s) declare financial support was received for the research, authorship, and/or publication of this article. This research was funded by Method of the Invasive Vertebrate Species Survey from National Forestry and Grassland Administration, and the Current Situation of Invasive Amphibians and Reptiles in China from National Forestry and Grassland Administration.
The authors declare that the research was conducted in the absence of any commercial or financial relationships that could be construed as a potential conflict of interest.
All claims expressed in this article are solely those of the authors and do not necessarily represent those of their affiliated organizations, or those of the publisher, the editors and the reviewers. Any product that may be evaluated in this article, or claim that may be made by its manufacturer, is not guaranteed or endorsed by the publisher.
The Supplementary Material for this article can be found online at: https://www.frontiersin.org/articles/10.3389/fevo.2023.1277058/full#supplementary-material
Supplementary Figure 1 | Changes in the potential distribution of C. serpentina in China from the current conditions to the 2070 under the climate scenarios of RCP2.6, RCP4.5, RCP6.0, and RCP8.5.
Aiello-Lammens M. E., Boria R. A., Radosavljevic A., Vilela B., Anderson R. P. (2015). spThin: an R package for spatial thinning of species occurrence records for use in ecological niche models. Ecography 38, 541–545. doi: 10.1111/ecog.01132
Alles J., Banther-McConnell J., Montgomery J., Suriyamongkol T., Mali I. (2022). Irrigation canals as potential dispersal routes for the Common Snapping Turtle, Chelydra serpentina, in the southern High Plains of New Mexico, USA. Herpetology Notes 15, 193–195.
Allouche O., Tsoar A., Kadmon R. (2006). Assessing the accuracy of species distribution models: prevalence, kappa and the true skill statistic (TSS): Assessing the accuracy of distribution models. J. Appl. Ecol. 43, 1223–1232. doi: 10.1111/j.1365-2664.2006.01214.x
Anderson R. P., Lew D., Peterson A. T. (2003). Evaluating predictive models of species’ distributions: criteria for selecting optimal models. Ecol. Model. 162, 211–232. doi: 10.1016/S0304-3800(02)00349-6
Araújo M. B., Alagador D., Cabeza M., Nogués-Bravo D., Thuiller W. (2011). Climate change threatens European conservation areas. Ecol. Lett. 14, 484–492. doi: 10.1111/j.1461-0248.2011.01610.x
Austin M. P. (2002). Spatial prediction of species distribution: an interface between ecological theory and statistical modelling. Ecol. Model. 157, 101–118. doi: 10.1016/S0304-3800(02)00205-3
Beck J., Böller M., Erhardt A., Schwanghart W. (2014). Spatial bias in the GBIF database and its effect on modeling species’ geographic distributions. Ecol. Inform. 19, 10–15. doi: 10.1016/j.ecoinf.2013.11.002
Bellard C., Thuiller W., Leroy B., Genovesi P., Bakkenes M., Courchamp F. (2013). Will climate change promote future invasions? Glob. Change Biol. 19, 3740–3748. doi: 10.1111/gcb.12344
Bertolino S., Sciandra C., Bosso L., Russo D., Lurz P. W. W., Di Febbraro M. (2020). Spatially explicit models as tools for implementing effective management strategies for invasive alien mammals. Mammal Rev. 50, 187–199. doi: 10.1111/mam.12185
Bonebrake T. C., Boggs C. L., Stamberger J. A., Deutsch C. A., Ehrlich P. R. (2014). From global change to a butterfly flapping: biophysics and behaviour affect tropical climate change impacts. Proc. R. Soc B. 281, 20141264. doi: 10.1098/rspb.2014.1264
Bosch S., Tauxe R. V., Behravesh C. B. (2015). Turtle-associated salmonellosis, United States 2006–2014. Emerg. Infect. Dis. 22, 1149–1155. doi: 10.3201/eid2207.150685
Bradie J., Leung B. (2017). A quantitative synthesis of the importance of variables used in MaxEnt species distribution models. J. Biogeogr. 44, 1344–1361. doi: 10.1111/jbi.12894
Brown G. P., Brooks R. J. (1994). Characteristics of and fidelity to hibernacula in a northern population of snapping turtles, Chelydra serpentina. Copeia 1994, 222–226. doi: 10.2307/1446689
Brown J. L., Bennett J. R., French C. M. (2017). SDMtoolbox 2.0: the next generation Python-based GIS toolkit for landscape genetic, biogeographic and species distribution model analyses. PeerJ 5, e4095. doi: 10.7717/peerj.4095
Bush A., Mokany K., Catullo R., Hoffmann A., Kellermann V., Sgrò C., et al. (2016). Incorporating evolutionary adaptation in species distribution modelling reduces projected vulnerability to climate change. Ecol. Lett. 19, 1468–1478. doi: 10.1111/ele.12696
Capinha C., Larson E. R., Tricarico E., Olden J. D., Gherardi F. (2013). Effects of climate change, invasive species, and disease on the distribution of native european crayfishes: global change and european crayfishes. Conserv. Biol. 27, 731–740. doi: 10.1111/cobi.12043
Chen G., Li X., Liu X. (2022). Global land projection based on plant functional types with a 1-km resolution under socio-climatic scenarios. Sci. Data 9, 125. doi: 10.1038/s41597-022-01208-6
Cheung S. M., Dudgeon D. (2006). Quantifying the Asian turtle crisis: market surveys in southern China 2000–2003. Aquat. Conserv. Mar. Freshw. Ecosyst. 16, 751–770. doi: 10.1002/aqc.803
Cobos M. E., Peterson A. T., Barve N., Osorio-Olvera L. (2019). kuenm: an R package for detailed development of ecological niche models using Maxent. PeerJ 7, e6281. doi: 10.7717/peerj.6281
Colteaux B. C., Johnson D. M. (2017). Commercial harvest and export of snapping turtles (Chelydra serpentina) in the United States: trends and the efficacy of size limits at reducing harvest. J. Nat. Conserv. 35, 13–19. doi: 10.1016/j.jnc.2016.11.003
Dakhil M. A., Halmy M. W. A., Liao Z., Pandey B., Zhang L., Pan K., et al. (2021). Potential risks to endemic conifer montane forests under climate change: integrative approach for conservation prioritization in southwestern China. Landscape Ecol. 36, 3137–3151. doi: 10.1007/s10980-021-01309-4
Díaz-Paniagua C., Pérez-Santigosa N., Hidalgo-Vila J., Florencio M. (2011). Does the exotic invader turtle, Trachemys scripta elegans, compete for food with coexisting native turtles? Amphibia-reptilia 32, 167–175. doi: 10.1163/017353710X552795
Dormann C. F., Elith J., Bacher S., Buchmann C., Carl G., Carré G., et al. (2013). Collinearity: a review of methods to deal with it and a simulation study evaluating their performance. Ecography 36, 27–46. doi: 10.1111/j.1600-0587.2012.07348.x
Duan R.-Y., Kong X.-Q., Huang M.-Y., Varela S., Ji X. (2016). The potential effects of climate change on amphibian distribution, range fragmentation and turnover in China. PeerJ 4, e2185. doi: 10.7717/peerj.2185
Elith J., Phillips S. J., Hastie T., Dudík M., Chee Y. E., Yates C. J. (2011). A statistical explanation of MaxEnt for ecologists. Divers. Distrib. 17, 43–57. doi: 10.1111/j.1472-4642.2010.00725.x
Ernst C. H., Lovich J. E. (2009). Turtles of the United States and Canada (Baltimore: Johns Hopkins University Press).
Ewert M. A., Lang J. W., Nelson C. E. (2005). Geographic variation in the pattern of temperature-dependent sex determination in the American snapping turtle (Chelydra serpentina). J. Zool. 265, 81–95. doi: 10.1017/S0952836904006120
Faye E., Herrera M., Bellomo L., Silvain J.-F., Dangles O. (2014). Strong discrepancies between local temperature mapping and interpolated climatic grids in tropical mountainous agricultural landscapes. PloS One 9, e105541. doi: 10.1371/journal.pone.0105541
Gábor L., Šímová P., Keil P., Zarzo-Arias A., Marsh C. J., Rocchini D., et al. (2022). Habitats as predictors in species distribution models: Shall we use continuous or binary data? Ecography 2022, e06022. doi: 10.1111/ecog.06022
Gallardo B. (2014). Europe’s top 10 invasive species: relative importance of climatic, habitat and socio-economic factors. Ethol. Ecol. Evol. 26, 130–151. doi: 10.1080/03949370.2014.896417
Garig D. F., Ennen J. R., Davenport J. M. (2020). The effects of common snapping turtles on a freshwater food web. Copeia 108, 132. doi: 10.1643/CE-19-258
Gong S.-P., Chow A. T., Fong J. J., Shi H.-T. (2009). The chelonian trade in the largest pet market in China: scale, scope and impact on turtle conservation. Oryx 43, 213. doi: 10.1017/S0030605308000902
Graves B. M., Anderson S. H. (1987). Habitat suitability index models: snapping turtle (Washington: U.S. Fish and Wildlife Service).
Guisan A., Thuiller W. (2005). Predicting species distribution: offering more than simple habitat models. Ecol. Lett. 8, 993–1009. doi: 10.1111/j.1461-0248.2005.00792.x
Guisan A., Zimmermann N. E. (2000). Predictive habitat distribution models in ecology. Ecol. Model. 135, 147–186. doi: 10.1016/S0304-3800(00)00354-9
Harding J. H., Mifsud D. A. (1997). Amphibians and reptiles of the Great Lakes region. (Ann Arbor: University of Michigan Press).
Hijmans R. J., Cameron S. E., Parra J. L., Jones P. G., Jarvis A. (2005). Very high resolution interpolated climate surfaces for global land areas. Int. J. Climatol. 25, 1965–1978. doi: 10.1002/joc.1276
Hill M. P., Gallardo B., Terblanche J. S. (2017). A global assessment of climatic niche shifts and human influence in insect invasions. Glob. Ecol. Biogeogr. 26, 679–689. doi: 10.1111/geb.12578
Hong X., Zhang X., Liu X., Wang Y., Yu L., Li W., et al. (2022). Status and analysis of artificial breeding and management of aquatic turtles in China. Biology 11, 1368. doi: 10.3390/biology11091368
Hulme P. E. (2009). Trade, transport and trouble: managing invasive species pathways in an era of globalization. J. Appl. Ecol. 46, 10–18. doi: 10.1111/j.1365-2664.2008.01600.x
Iverson J. B., Higgins H., Sirulnik A., Griffiths C. (1997). Local and geographic variation in the reproductive biology of the snapping turtle (Chelydra serpentina). Herpetologica 53, 96–117.
Jayasinghe S. L., Kumar L. (2019). Modeling the climate suitability of tea [Camellia sinensis(L.) O. Kuntze] in Sri Lanka in response to current and future climate change scenarios. Agric. For. Meteorol. 272–273, 102–117. doi: 10.1016/j.agrformet.2019.03.025
Jeschke J. M., Strayer D. L. (2008). Usefulness of bioclimatic models for studying climate change and invasive species. Ann. New York Acad. Sci. 1134, 1–24. doi: 10.1196/annals.1439.002
Ji L. (2023). “Catalogue of life China: 2023 annual checklist,” in China checklist of animals China checklist of animals (Beijing: Biodiversity Committee of Chinese Academy of Sciences).
Kalaentzis K., Kazilas C., Strachinis I., Tzoras E., Lymberakis P. (2023). Alien freshwater turtles in Greece: citizen science reveals the hydra-headed issue of the pet turtle trade. Australas. I. Min. Met. 15, 691. doi: 10.3390/d15050691
Kapfer J. M., Munoz D. J., Tomasek T. (2012). Use of wildlife detector dogs to study Eastern Box Turtle (Terrapene carolina carolina) populations. Herpetol. Conserv. Bio. 7, 169–175.
Kelly R. P., Port J. A., Yamahara K. M., Martone R. G., Lowell N., Thomsen P. F., et al. (2014). Harnessing DNA to improve environmental management. Science 344, 1455–1456. doi: 10.1126/science.1251156
Kolar C. S., Lodge D. M. (2001). Progress in invasion biology: predicting invaders. Trends Ecol. Evol. 16, 199–204. doi: 10.1016/S0169-5347(01)02101-2
Koo K. S., Sung H.-C. (2020). New record of the non-native snapping turtle Chelydra serpentina (Linnaeus 1758) in the wild of the Republic of Korea. BIR 9, 444–449. doi: 10.3391/bir.2020.9.2.30
Kraus F. (2009). Alien reptiles and amphibians: a scientific compendium and analysis (Dordrecht: Springer Science & Business Media).
Li B., Wei S., Li H., Yang Q., Lu M. (2014). “Invasive species of China and their responses to climate change,” in Invasive species and global climate change. Eds. Ziska L. H., Dukes J. S. (Wallingford UK: Centre for Agriculture and Bioscience International), 198–216.
Liu J., Li R., Liu C., Cai J. (2007). Techniques of artificial breeding and breeding of Chelydra serpentina. J. Aquaculture 28, 23–24.
Liu S., Newman C., Buesching C. D., Macdonald D. W., Zhang Y., Zhang K.-J., et al. (2021). E-commerce promotes trade in invasive turtles in China. Oryx 55, 352–355. doi: 10.1017/S0030605319001030
Liu C., White M., Newell G. (2009). “Measuring the accuracy of species distribution models: a review,” in Proceedings of 18th World IMACs/MODSIM Congress Cairns (Citeseer).
Liu C., Wolter C., Xian W., Jeschke J. M. (2020). Most invasive species largely conserve their climatic niche. Proc. Natl. Acad. Sci. U.S.A. 117, 23643–23651. doi: 10.1073/pnas.2004289117
Lovich J. E., Ennen J. R., Agha M., Gibbons J. W. (2018). Where have all the turtles gone, and why does it matter? Bioscience 68, 771–781. doi: 10.1093/biosci/biy095
Malcolm J. R., Liu C., Neilson R. P., Hansen L., Hannah L. E. E. (2006). Global warming and extinctions of endemic species from biodiversity hotspots. Conserv. Biol. 20, 538–548. doi: 10.1111/j.1523-1739.2006.00364.x
Masin S., Bonardi A., Padoa-Schioppa E., Bottoni L., Ficetola G. F. (2014). Risk of invasion by frequently traded freshwater turtles. Biol. Invasions 16, 217–231. doi: 10.1007/s10530-013-0515-y
Masson-Delmotte V., Zhai P., Pirani A., Connors S. L., Péan C., Berger S., et al. (2021). Climate change 2021: the physical science basis. Contribution of working group I to the sixth assessment report of the intergovernmental panel on climate change (Cambridge: Cambridge University Press).
McKnight C. M., Gutzke W. H. N. (1993). Effects of the embryonic environment and of hatchling housing conditions on growth of young snapping turtles (Chelydra serpentina). Copeia 1993, 475. doi: 10.2307/1447148
Mendoza-Roldan J. A., Modry D., Otranto D. (2020). Zoonotic parasites of reptiles: A crawling threat. Trends Parasitol. 36, 677–687. doi: 10.1016/j.pt.2020.04.014
Newbold T., Oppenheimer P., Etard A., Williams J. J. (2020). Tropical and Mediterranean biodiversity is disproportionately sensitive to land-use and climate change. Nat. Ecol. Evol. 4, 1630–1638. doi: 10.1038/s41559-020-01303-0
Obbard M. E., Brooks R. J. (1979). Factors affecting basking in a northern population of the common snapping turtle, Chelydra serpentina. Can. J. Zool. 57, 435–440. doi: 10.1139/z79-051
Obbard M. E., Brooks R. J. (1981). A radio-telemetry and mark-recapture study of activity in the common snapping turtle, Chelydra serpentina. Copeia 1981, 630–637. doi: 10.2307/1444568
O’Steen S. (1998). Embryonic temperature influences juvenile temperature choice and growth rate in snapping turtles chelydra serpentina. J. Exp. Biol. 201, 439–449. doi: 10.1242/jeb.201.3.439
Pearson R. G., Dawson T. P. (2003). Predicting the impacts of climate change on the distribution of species: are bioclimate envelope models useful? Glob. Ecol. Biogeogr. 12, 361–371. doi: 10.1046/j.1466-822X.2003.00042.x
Perry G., Owen J. L., Petrovic C., Lazell J., Egelhoff J. (2007). The red-eared slider, Trachemys scripta elegans, in the British Virgin Islands. Appl. Herpetol. 4, 88. doi: 10.1163/157075407779766723
Peterson A. T., Papeş M., Soberón J. (2008). Rethinking receiver operating characteristic analysis applications in ecological niche modeling. Ecol. Model. 213, 63–72. doi: 10.1016/j.ecolmodel.2007.11.008
Paterson J. E., Steinberg B. D., Litzgus J. D. (2008). Generally specialized or especially general? Habitat selection by Snapping Turtles (Chelydra serpentina) in central Ontario. Can. J. Zool. 90, 139–149. doi: 10.1139/z11-118
Phillips S. J., Anderson R. P., Schapire R. E. (2006). Maximum entropy modeling of species geographic distributions. Ecol. Model. 190, 231–259. doi: 10.1016/j.ecolmodel.2005.03.026
Record S., Strecker A., Tuanmu M.-N., Beaudrot L., Zarnetske P., Belmaker J., et al. (2018). Does scale matter? A systematic review of incorporating biological realism when predicting changes in species distributions. PloS One 13, e0194650. doi: 10.1371/journal.pone.0194650
Rollinson N., Farmer R. G., Brooks R. J. (2012). Widespread reproductive variation in North American turtles: temperature, egg size and optimality. Zoology 115, 160–169. doi: 10.1016/j.zool.2011.10.005
SEP A (State Environmental Protection Administration) (1998). China’s biodiversity: A country study (Beijing: China Environmental Science Press).
Sequeira A. M. M., Bouchet P. J., Yates K. L., Mengersen K., Caley M. J. (2018). Transferring biodiversity models for conservation: Opportunities and challenges. Methods Ecol. Evol. 9, 1250–1264. doi: 10.1111/2041-210X.12998
Slatyer R. A., Hirst M., Sexton J. P. (2013). Niche breadth predicts geographical range size: a general ecological pattern. Ecol. Lett. 16, 1104–1114. doi: 10.1111/ele.12140
Steele K., Werndl C. (2013). Climate models, calibration, and confirmation. Br. J. Philos. Sci. 64, 609–635. doi: 10.1093/bjps/axs036
Steen D. A., Sterrett S. C., Heupel A. M., Smith L. L. (2010). Snapping turtle, Chelydra serpentina, overland movements near the southeastern extent of its range. Georgia J. Sci. 68, 196–201.
Stephens K. L., Dantzler-Kyer M. E., Patten M. A., Souza L. (2019). Differential responses to global change of aquatic and terrestrial invasive species: evidences from a meta-analysis. Ecosphere 10, e02680. doi: 10.1002/ecs2.2680
Sung Y.-H., Lee W.-H., Wai-neng Lau M., Lau A., Wong P., Dingle C., et al. (2021). Species list and distribution of non-native freshwater turtles in Hong Kong. BIR 10, 960–968. doi: 10.3391/bir.2021.10.4.20
Thuiller W., Araújo M. B., Lavorel S. (2004). Do we need land-cover data to model species distributions in Europe? J. Biogeogr. 31, 353–361. doi: 10.1046/j.0305-0270.2003.00991.x
Tingley R., Thompson M. B., Hartley S., Chapple D. G. (2016). Patterns of niche filling and expansion across the invaded ranges of an Australian lizard. Ecography 39, 270–280. doi: 10.1111/ecog.01576
Ueno S., Kamezaki N., Mine K., Suzuki D., Hosoya S., Kikuchi K., et al. (2021). Reproductive Ability of Hybrids between Japanese Pond Turtle (Mauremys japonica) and Reeves’ Pond Turtle (Mauremys reevesii). Zoolog. Sci. 39, 186–192. doi: 10.2108/zs210047
UNEP Mittermeier (2002). United nations environmental program—Convention on biological diversity (Montreal: UNEP Mittermeier).
Urban M. C., Tewksbury J. J., Sheldon K. S. (2012). On a collision course: competition and dispersal differences create no-analogue communities and cause extinctions during climate change. P Roy Soc. B-Biol Sci. 279, 2072–2080. doi: 10.1098/rspb.2011.2367
van Vuuren D. P., Stehfest E., den Elzen M. G. J., Kram T., van Vliet J., Deetman S., et al. (2011). RCP2.6: exploring the possibility to keep global mean temperature increase below 2°C. Climatic Change 109, 95–116. doi: 10.1007/s10584-011-0152-3
Warren D. L., Glor R. E., Turelli M. (2010). ENMTools: a toolbox for comparative studies of environmental niche models. Ecography 33, 607–611. doi: 10.1111/j.1600-0587.2009.06142.x
Watson R., Baste I., Larigauderie A., Leadley P., Pascual U., Baptiste B., et al. (2019). Summary for policymakers of the global assessment report on biodiversity and ecosystem services of the Intergovernmental Science-Policy Platform on Biodiversity and Ecosystem Services (Bonn: IPBES Secretariat).
West A. M., Kumar S., Brown C. S., Stohlgren T. J., Bromberg J. (2016). Field validation of an invasive species Maxent model. Ecol. Inform. 36, 126–134. doi: 10.1016/j.ecoinf.2016.11.001
Wilbur H. M. (1997). Experimental ecology of food webs: complex systems in temporary ponds. Ecology 78, 2279–2302. doi: 10.1890/0012-9658(1997)078[2279:EEOFWC]2.0.CO;2
Wisz M. S., Hijmans R. J., Li J., Peterson A. T., Graham C. H., Guisan A., et al. (2008). Effects of sample size on the performance of species distribution models. Diversity Distributions 14, 763–773. doi: 10.1111/j.1472-4642.2008.00482.x
Wu J., Wu Y., Rao D., Zhou T., Gong S. (2020). China’s wild turtles at risk of extinction. Science 368, 838–838. doi: 10.1126/science.abc0997
Xian X., Wang R., Guo J., Liu W., Zhang G., Sun Y., et al. (2018). Analysis of new invasive alien species in China’s agricultural and forestry ecosystems in recent 20 years. Plant Prot. 44, 168–175. doi: 10.16688/j.zwbh.2018332
Yackulic C. B., Chandler R., Zipkin E. F., Royle J. A., Nichols J. D., Campbell Grant E. H., et al. (2013). Presence-only modelling using MAXENT: when can we trust the inferences? Methods Ecol. Evol. 4, 236–243. doi: 10.1111/2041-210x.12004
Yan X., Wang S., Duan Y., Han J., Huang D., Zhou J. (2021). Current and future distribution of the deciduous shrub Hydrangea macrophylla in China estimated by MaxEnt. Ecol. Evol. 11, 16099–16112. doi: 10.1002/ece3.8288
Yates K. L., Bouchet P. J., Caley M. J., Mengersen K., Randin C. F., Parnell S., et al. (2018). Outstanding challenges in the transferability of ecological models. Trends Ecol. Evol. 33, 790–802. doi: 10.1016/j.tree.2018.08.001
Zhang H., Yang J., Zhang L., Gu X., Zhang X. (2023). Citizen science meets eDNA: A new boom in research exploring urban wetland biodiversity. Environ. Sci. Ecotechnology 16, 100275. doi: 10.1016/j.ese.2023.100275
Zhou T., Huang C. (2007). Current situation and characteristics of turtle breeding industry in China. J. Economic Anim. 11, 238–242+245. doi: 10.13326/j.jea.2007.04.018
Keywords: Chelydra serpentina, invasive alien species, species distribution models, climate change, potential distribution, management
Citation: Mu C and Li P (2023) Assessing the invasion risk of Chelydra serpentina in China under current and future climate change scenarios. Front. Ecol. Evol. 11:1277058. doi: 10.3389/fevo.2023.1277058
Received: 13 August 2023; Accepted: 08 November 2023;
Published: 28 November 2023.
Edited by:
Ming-Chih Chiu, Chinese Academy of Sciences (CAS), ChinaReviewed by:
Viorel Dan Popescu, Columbia University, United StatesCopyright © 2023 Mu and Li. This is an open-access article distributed under the terms of the Creative Commons Attribution License (CC BY). The use, distribution or reproduction in other forums is permitted, provided the original author(s) and the copyright owner(s) are credited and that the original publication in this journal is cited, in accordance with accepted academic practice. No use, distribution or reproduction is permitted which does not comply with these terms.
*Correspondence: Pipeng Li, MTA0NDY2NjA2QHFxLmNvbQ==
Disclaimer: All claims expressed in this article are solely those of the authors and do not necessarily represent those of their affiliated organizations, or those of the publisher, the editors and the reviewers. Any product that may be evaluated in this article or claim that may be made by its manufacturer is not guaranteed or endorsed by the publisher.
Research integrity at Frontiers
Learn more about the work of our research integrity team to safeguard the quality of each article we publish.