- 1Faculty of Physical Culture and Sports, The Herzen State Pedagogical University of Russia, Saint Petersburg, Russia
- 2College of Film, Television and Communication, Shanghai Normal University, Shanghai, China
- 3Faculty of Sports Science, Harbin Normal University, Harbin, China
Introduction: In light of escalating concerns regarding global warming and environmental pollution, the pursuit of carbon neutrality has emerged as a pivotal strategy to address climate change on a global scale. As society becomes increasingly conscious of its ecological impact, various sectors, including sports, are urged to embrace environmental responsibility. This study seeks to explore the integration of a carbon neutral framework utilizing artificial intelligence's attention mechanism within the realm of football, with the aim of contributing to football's adoption of carbon neutrality.
Methods: The study commences by introducing an end-to-end architectural framework capable of unifying and optimizing all facets of football to realize a comprehensive carbon-neutral objective. This architecture serves as a consolidated platform for enhancing carbon emission reduction within football pedagogical activities, fostering synergy among diverse constituents while concurrently assessing the equilibrium between carbon reduction and pedagogical effectiveness. Subsequently, attention mechanisms are leveraged to heighten the efficacy and comprehensibility of carbon-neutral strategies. The application of attention mechanisms enables the model to autonomously focus on attributes or regions closely associated with carbon neutrality objectives, thereby facilitating precision and efficacy in recommending carbon neutral strategies. By employing attention mechanisms in football, a more thorough understanding of carbon emissions' dynamics is attained, allowing for the identification of pivotal emission contributors and tailored suggestions for emission mitigation. Furthermore, the Long Short-Term Memory (LSTM) method is employed to analyze football time-series data. Given football's intricate sequence of actions, the LSTM technique adeptly captures long-term dependencies, offering improved analysis and optimization of carbon emissions during football activities.
Results: The integrated end-to-end architectural framework offers a holistic approach to carbon-neutral football strategies. Attention mechanisms effectively enhance the focus and interpretation of carbon-neutral strategies, contributing to precise and impactful recommendations. Employing LSTM for time-series analysis aids in comprehending carbon emission dynamics, enabling the identification of efficacious carbon neutral strategies. The study underscores the potential of AI-driven attention mechanisms and LSTM in fostering carbon neutrality within football.
Discussion: The study's findings underscore the viability of integrating AI-driven methodologies, specifically attention mechanisms and LSTM, to promote carbon neutrality within the football domain. The end-to-end architecture serves as a foundational platform for comprehensive carbon emission reduction, offering potential for broader application in other sectors. The combination of attention mechanisms and LSTM engenders deeper insights into carbon emissions' intricate temporal dynamics, informing the development of targeted strategies for emission mitigation. The study's outcomes provide theoretical underpinnings for advancing sustainable football practices and inspire the broader adoption of carbon neutrality principles across diverse domains.
1 Introduction
With the increasingly serious issues of global climate change and environmental pollution, we are facing unprecedented challenges on a global scale. Climate change has profoundly impacted the Earth’s ecosystems, economic systems, and social stability (Mora et al., 2018; Wang W. et al., 2021). Extreme weather events, rising sea levels, glacial melting, and biodiversity loss pose significant threats to human survival and development. In this urgent context, countries around the world are collectively taking on the responsibility to address climate change and actively exploring paths towards sustainable development.
Among numerous environmental protection measures, carbon neutrality has emerged as a crucial concept and action plan in combating climate change (Wang F. et al., 2021). Carbon neutrality, as the name suggests, involves reducing greenhouse gas emissions, such as carbon dioxide, and increasing carbon sequestration to achieve a net-zero emission balance. This concept emphasizes integrating emission reductions with carbon sequestration in the process of economic and social development, promoting the transition to a green and low-carbon economy. The goal of carbon neutrality is not only to mitigate the adverse impacts of climate change but also to build a sustainable ecological civilization, ensuring the sustainable utilization of Earth’s resources.
Globally, various sectors are actively exploring and implementing carbon neutrality strategies, including industry, energy, transportation, agriculture, and more (Chen et al., 2022). However, in the field of sports, research and practices related to carbon neutrality are relatively scarce, especially concerning carbon emissions reduction during sports activities. Sports, as a widely popular social activity, have witnessed continuous growth in scale and influence, but they also come with higher carbon emissions. For instance, the construction of large sports venues, transportation, and energy consumption for major sports events result in substantial greenhouse gas emissions, exerting tremendous pressure on the environment. Therefore, how to adopt effective carbon emission reduction strategies in sports and achieve the goal of carbon neutrality has become an urgent problem to be solved (Elnour et al., 2022). Therefore, this study aims to explore how to apply a carbon neutral scheme based on artificial intelligence attention mechanism in the field of football to reduce carbon emissions and achieve the carbon neutral goal of football.
As one of the most popular sports globally, football is among the significant contributors to carbon emissions in the sports industry (Singh and Lamba, 2019). Training of athletes, maintenance of stadiums, and transportation of spectators during football matches all result in substantial carbon emissions. Therefore, promoting and applying the concept of carbon neutrality in football, reducing carbon emissions, and achieving low-carbon development have become pressing issues that need to be addressed. Simultaneously, in past research, scholars have begun to pay attention to the application of carbon neutrality strategies in sports education, although relevant studies are still relatively limited. Existing research mainly focuses on the exploration of carbon neutrality theory, the application of carbon emissions reduction techniques, and the evaluation of carbon neutrality strategies. Although these studies lay the foundation for the application of carbon neutrality concepts in sports education, they have not covered the entire sports education process and lack an effective evaluation index system.
To fill this research gap, this study will adopt an end-to-end architecture and attention mechanism to comprehensively explore the application and promotion of carbon neutrality concepts in football. By introducing an end-to-end architecture, we will integrate and optimize various aspects of football to achieve comprehensive carbon neutrality goals. Meanwhile, through the application of the attention mechanism, we will accurately identify critical carbon emission points and provide precise carbon reduction recommendations.
In order to deal with the problem of carbon emissions in football, we propose a new research idea - the application and promotion of the concept of carbon neutrality in football based on end-to-end architecture and attention mechanism. Traditional carbon emission reduction research is usually limited to a specific link or field, and the end-to-end architecture we propose takes the entire football process into consideration, from pre-match preparations, game processes to subsequent carbon emission reduction strategies, forming a Complete carbon neutral system. The end-to-end architecture provides a unified optimization platform that enables the various components involved in football to collaborate with each other, taking into account the trade-offs of carbon emission reduction and sports performance (Cuperman et al., 2022; Ning et al., 2023). Meanwhile, we introduce an attention mechanism to improve the effect and interpretability of carbonneutral strategies in soccer. In traditional carbon emission reduction methods, fixed weight assignments or simple statistical models are usually used, while we use the attention mechanism to automatically focus on features or regions that are closely related to the goal of carbon neutrality (Gao et al., 2021). In this way, we can more accurately identify the key links that generate carbon emissions during football, so as to provide targeted carbon emission reduction suggestions and optimization schemes. In addition, in order to better understand and analyze the carbon emission process in football, we adopted the LSTM (long short-term memory network) model to capture the time series information in football (Fenil et al., 2019). The football game involves complex action sequences and changing rules, and traditional models are often difficult to capture this timing information. We utilized the LSTM model to model the carbon emission process in football sports, enabling a better understanding and analysis of the dynamic characteristics of carbon emissions. This introduction of the LSTM method not only enhances the accuracy of the carbon neutrality strategy but also strengthens the model’s comprehension and interpretability of the carbon emission process in football sports. In summary, the objective of this research is to promote and apply the carbon neutrality scheme based on the attention mechanism in the field of football sports, aiming to reduce carbon emissions and achieve carbon neutrality in football sports. By applying and promoting this approach, we hope to provide scientifically feasible carbon reduction strategies for the sports industry, promote the sustainable development of sports, and offer valuable references and insights for carbon neutrality research in other fields. However, we are aware that there is still room for further development and improvement in this research. For example, we need to evaluate the generalizability of the proposed end-to-end architecture and attention mechanism to ensure their effectiveness and applicability in different football sports scenarios. Additionally, empirical analysis, combined with real football match data, is necessary to validate the credibility and practicality of our carbon neutrality strategy. Furthermore, we should conduct in-depth research on carbon neutrality strategies in different sports disciplines, exploring personalized low-carbon approaches for various sports projects.
The contributions of this paper can be summarized in the following three aspects:
1. This study applies an end-to-end architecture to the concept of carbon neutrality in football. Traditional carbon reduction research typically focuses on specific aspects or domains, whereas our proposed end-to-end architecture considers the entire football process, including pre-match preparations, in-game activities, and post-match carbon reduction strategies, forming a comprehensive carbon neutrality system. This end-to-end approach allows for a more holistic and integrated application of carbon neutrality strategies in football, leading to more effective carbon reduction and environmental protection goals.
2. This study introduces the attention mechanism to enhance the effectiveness and interpretability of carbon neutrality strategies in football. Traditional carbon reduction methods often use fixed weight distributions or simple statistical models, while we utilize the attention mechanism to automatically focus on features or regions closely related to carbon neutrality goals. Through this approach, we can more accurately identify key carbon emission points during football activities, providing targeted carbon reduction recommendations and optimization plans.
3. This study employs the Long Short-Term Memory (LSTM) model to capture temporal information in football activities. Football matches involve complex action sequences and dynamic patterns, which traditional models often struggle to capture. By utilizing the LSTM model, we can model the carbon emissions process in football, leading to a deeper understanding and analysis of its dynamic characteristics. This LSTM-based approach not only improves the accuracy of carbon neutrality strategies but also enhances the model’s understanding and interpretability of the football carbon emission
The logical structure of this paper is as follows: In Section 2, the relevant research work is mainly introduced, including the application of carbon neutral technology in various fields (such as industry, energy), the current status and shortcomings of carbon neutral research in the field of sports. It expounds that the use of end-to-end framework and attention mechanism provides a new idea for the research of carbon emission reduction technology. In Section 3, the method proposed in this paper is introduced, the end-to-end framework, attention mechanism and LSTM model are introduced respectively, and the role of these techniques in achieving the goal of carbon neutrality is explained in detail. In Section 4, we conduct experiments on the proposed method, introducing the experimental environment, datasets, evaluation metrics, and comparative analysis of the results. The advantages and disadvantages of the end-to-end framework and traditional methods and the effectiveness of the attention mechanism are mainly analyzed. In Section 5, the experimental results are discussed, the advantages and limitations of the proposed method are discussed in terms of carbon emission reduction effect, action recognition accuracy and scalability, and related improvements and future research directions are proposed. In Section 6, we summarize the contributions and innovations of this paper, mainly including the use of end-to-end framework and attention mechanism to achieve carbon neutrality, improve the model’s ability to understand actions, etc. At the same time, the limitations of the research and future work plans are pointed out.
2 Related work
Climate change is one of the most important challenges facing the world, and carbon emissions, as the main source of greenhouse gas emissions, urgently need to take effective measures to reduce them. The concept of carbon neutrality has become one of the important strategies, which means to achieve the goal of net zero emissions by actively reducing carbon emissions in different fields. Over the past few decades, climate scientists and environmental groups have been working to find ways to reduce carbon emissions in order to achieve the goal of carbon neutrality. In this context, researchers have also begun to pay attention to the issue of carbon emissions in the field of sports, and explore how to achieve the goal of low-carbon sports (Hong et al., 2021; Wu et al., 2022a).
In various fields, the application of the concept of carbon neutrality has made positive progress. In the industrial field, many companies have begun to adopt energy-saving and emission-reduction measures and promote cleaner production technologies to achieve carbon neutrality. For example, the article “Practices and Challenges of Chinese Iron and Steel Enterprises in Low-Carbon Transformation” deeply analyzes the difficulties and opportunities faced by the steel industry in the process of low-carbon transformation, and proposes a series of low-carbon technological innovations, policy support and market incentives, etc. suggestions. In the field of energy, countries have increased the development and utilization of renewable energy, reduced dependence on fossil fuels, and promoted energy transformation. For example, literature Dhar (2020) introduced the application value of artificial intelligence technology in the energy industry, through data analysis, machine learning and other methods to optimize energy efficiency, reduce costs and reduce emissions. In the field of transportation, the promotion of electric vehicles and the development of public transportation and other low-carbon travel methods have become an important means of carbon neutrality. For example, literature Caineng et al. (2021) discussed the role and prospect of new energy in the field of transportation, and proposed to build a new energy structure of “three small and one big” (i.e., small electric vehicles, small electric motorcycles, small electric bicycles and large public transportation vehicles). The agricultural sector is also promoting sustainable farming and reducing field greenhouse gas emissions. For example, literature Tanzer et al. (2021) proposes a carbon-neutral plan for changing eating habits, increasing the value of food and agricultural waste, and points out decarbonization and negative emission technologies that can be adopted in the agricultural production process. The valuable experience and lessons accumulated in these fields in the practice of carbon neutrality provide useful reference and inspiration for carbon neutral research in other fields.
However, despite active exploration of carbon neutrality strategies in various fields, research on carbon emissions in the sports industry is still in its early stages. Scholars both domestically and internationally have begun to pay attention to the application of carbon neutrality in football sports, but relevant studies remain relatively limited. For instance, in reference Cooper (2020), the carbon footprint of American football events at the University of Tennessee was assessed, and a method for studying spectator sports events in sports tourism, along with some policies and management measures to reduce carbon emissions, was proposed. Triantafyllidis (2018) emphasizes the role of research in addressing carbon dioxide emissions generated by the sports industry and suggests the need for strategic management approaches and policy formulation to control this hazardous environmental pollutant. In reference Wicker (2019), from the perspective of active sports participants and considering the heterogeneity of individual sports participation behaviors, the annual carbon footprint of their sports travel behavior in 2015 was estimated, and influencing factors were analyzed. Wicker (2018) summarizes the main sources of carbon footprints from sports events and the measures taken by various countries to reduce carbon footprints associated with sports events, proposing relevant pathways to mitigate carbon emissions in sports events. While these studies contribute to inspiring our understanding of the application of carbon neutrality in sports, further in-depth research is still required to comprehensively understand and address carbon emissions issues in football sports.
We have proposed a novel research approach for addressing carbon emissions in football sports, which involves the application and promotion of the carbon neutrality concept based on an end-to-end architecture and attention mechanism. This innovative approach breaks away from the traditional carbon reduction studies that focus on specific aspects, instead encompassing the entire football process to form a comprehensive carbon neutrality framework (Ning et al., 2022). The end-to-end architecture provides a unified optimization platform, enabling collaborative efforts among various components of football activities, while considering the trade-offs between carbon emission reduction and sporting performance (Yao et al., 2023). By incorporating the attention mechanism, we enhance the effectiveness and interpretability of carbon neutrality strategies in football sports. This allows for a more precise identification of key emission points during the football process, thereby providing targeted recommendations and optimization solutions for carbon reduction. Moreover, we employ the Long Short-Term Memory (LSTM) model to capture time-series information in football activities, further improving the accuracy of carbon neutrality strategies and enhancing the model’s understanding and interpretative capacity of carbon emissions in football sports. This research approach holds significant value and importance in promoting carbon neutrality and sustainable development in football sports.
Beyond the realm of football, global research on carbon neutrality technologies continues to expand, with various fields actively exploring pathways for carbon emission reduction. As a popular and widely embraced social activity, the carbon footprint of sports cannot be overlooked (Li et al., 2023). Therefore, the sports industry should also contribute to carbon neutrality goals. Through the adoption of new energy technologies, carbon emission reduction measures, strengthened carbon footprint assessment, management practices, and exploration of carbon footprints of sports participants, significant contributions can be made towards the sustainable development and environmental protection of the sports industry. Additionally, analyzing the trends in global carbon neutrality technology research Wu et al. (2022b) facilitates a better understanding and grasp of the latest developments in related fields, thus driving further innovation and application of carbon neutrality technologies. In conclusion, carbon neutrality is not only a shared global challenge but also a great endeavor requiring collaboration and concerted efforts across multiple fields and various technologies to achieve carbon neutrality objectives.
3 Methodology
In this study, we address the grand challenge posed by climate change, with a particular focus on carbonneutral football applications. In order to achieve the goal of low-carbon sports, we have designed a comprehensive carbon-neutral algorithm and presented it in Figure 1. This algorithmic flowchart is at the heart of our research, covering the key steps and measures we are taking to achieve our goal of carbon neutrality.
3.1 End-to-end framework
When we talk about an end-to-end approach, we mean an approach to machine learning or deep learning that takes the entire problem as a whole, from raw input directly to final output, without multiple independent processing steps in between (Zhang et al., 2018). This approach is gaining popularity in the applied domain as it enables simplified problem modeling and achieves higher performance. In our study, we apply an end-to-end approach to the problem of carbon neutrality in football. Traditional carbon emission research is usually limited to a specific link or field, but we have adopted an end-to-end framework, taking the entire football process into consideration, forming a complete carbon neutral system, as shown in Figure 2.
End-to-end learning is a machine learning paradigm that avoids the multi-stage processing in traditional methods by jointly learning directly from raw input to final output. In our study, we use an end-to-end approach to build a holistic carbon-neutral model that directly predicts carbon emissions from each component of football without having to deal with each component separately. To better illustrate how an end-to-end model works, we can use a concrete example to show the input and output of the model. Suppose we have a football input feature sequence X = (x1,x2,…,xT), where xt is the input feature at time t, including player activities, audience movement, energy consumption, traffic emissions, etc. Our goal is to predict the carbon emissions y in football and give a carbon-neutral strategy suggestion z. Our end-to-end model can be expressed as:
Among them, f() is a function to predict carbon emissions, which means that the relevant information of each link in the football game is used as input, and a value is output, indicating the carbon emissions generated during the football game. g() is a function to generate carbon-neutral strategy suggestions, which means that the relevant information of each link in the football game and the predicted carbon emissions will be used as input, and a text will be output, indicating the carbon emissions during the football game. Suggestions for carbon-neutral strategies are given. By training the parameters in the model, we can learn the overall law of carbon emissions in football, so as to achieve the goal of carbon neutrality.
Meanwhile, in order to optimize our end-to-end model, we adopted an algorithm based on gradient descent to update the model parameters (Löwe et al., 2019). The gradient descent method is a commonly used optimization algorithm, which determines the direction and magnitude of parameter update by calculating the partial derivative of the objective function with respect to the parameters. The objective function is a function used to measure the difference between the model output and the real value, usually in the form of mean squared error or cross entropy. The formula of the gradient descent method is as follows:
Among them, θt is the model parameter at time t, α is the learning rate, and ∇J (θt) is the gradient of the objective function to parameter θt. The gradient descent method makes the objective function J (θt) tend to the minimum value by continuously iteratively updating the parameter θt.
In the next subsection, we introduce the application of the attention mechanism, by introducing the attention mechanism, we further improve the effectiveness and interpretability of the carbon-neutral strategy in soccer.
3.2 Attention mechanism
In our study, we apply the attention mechanism in an end-to-end model to improve the effectiveness and interpretability of carbon-neutral strategies in soccer. Our attention mechanism is an attention mechanism based on the encoder-decoder framework Niu et al. (2021), which allows the decoder to automatically focus on the most relevant or the most important parts, thereby improving the accuracy and rationality of the output, as shown in Figure 3.
Our attention mechanism can be divided into two main steps: computing attention weights and generating context vectors. Calculating the attention weight refers to calculating the contribution or similarity of each encoder output to the decoder output based on the hidden state of the decoder at the current moment and the encoder output sequence, and normalizing it into a probability distribution. Generating a context vector refers to obtaining a vector containing the most relevant or important information in the encoder output sequence based on the attention weight and the encoder output sequence, and combining it with the hidden state of the decoder as the basis for the decoder output. The formulation of our attention mechanism is as follows:
where at is the attention weight vector at time t, ctis the context vector at time t, stis the decoder hidden state at time t, and H is the encoder output sequence.
Although the attention mechanism can improve the flexibility and interpretability of the model, it also brings some computational challenges and limitations. For example, the attention mechanism needs to calculate the similarity or weight between the hidden state of the decoder and the output sequence of the encoder. This process involves a matrix multiplication operation, and its time complexity and space complexity are both O(T2), where T is the sequence length. When the sequence length is very long, this operation will be very time-consuming and memory-consuming, thus limiting the scalability and application range of the model.
To solve this problem, we adopt a series of innovative methods to optimize the attention mechanism, including key techniques such as window attention, sparse attention and approximate attention. The introduction of these methods is not only to overcome the limitations of the original attention mechanism, but also to show our new exploration and improvement of the attention mechanism in the following.
• Windowed attention: This method is to let the decoder only pay attention to the part of the encoder output sequence in a window adjacent to the current moment, rather than the entire sequence (Zhang et al., 2019). This reduces computation and memory consumption, while also preserving a certain degree of contextual information. The formula for windowed attention is as follows:
where w is the window size and Ht−w:t+w is the part of the encoder output sequence from time t−w to time t + w.
• Sparse attention: This method is to let the decoder only pay attention to some parts of the encoder output sequence that are more relevant to the current moment, rather than all parts (Ma et al., 2021). This reduces computation and memory consumption, while also improving model selectivity and sensitivity. The formula for sparse attention is as follows:
Among them, ⊙ is element-wise multiplication, and mt is a mask vector, which indicates which encoder outputs are concerned (represented by 1) and which are ignored (represented by 0). Mask vectors can be generated according to different rules or strategies, such as distance-based, frequency-based, importance-based, etc.
• Approximate attention: This method uses a low-rank matrix or a random feature map to approximate the encoder output sequence, thereby reducing the amount of computation and memory consumption, while maintaining a certain degree of accuracy and effect (Vasyltsov and Chang, 2021). The formula for approximate attention is as follows:
Among them, Φ() is an approximation function, which can map the encoder output sequence H to a low-dimensional space or a random feature space. Approximation functions can have different forms and parameters, for example, 1. Based on singular value decomposition, 2. Based on random projection, 3. Based on kernel function, etc.
The above is our introduction to the attention mechanism. In the next subsection, we introduce the application of the LSTM method, by introducing the LSTM method, we further improve the ability of the end-to-end model in dealing with long-term dependencies.
3.3 LSTM model
Our main research goal is to build an end-to-end model to predict and optimize carbon emissions and carbon neutral strategies in football. In order to achieve this goal, we need to process some complex sequence data, such as information about various links in football, and the output of carbon emissions and carbon neutral strategy recommendations. There is a long-term dependency between these sequence data, that is, the output or state at the current moment is closely related to the input or state at an earlier moment. For example, in football, the energy consumption of pre-match preparations can affect the game process and subsequent carbon reduction strategies.
In this section, we introduce the application of the LSTM method (long short-term memory method). By introducing the LSTM method, we further improve the end-to-end model’s ability to handle long-term dependencies. The LSTM method is a recurrent neural network (recurrent neural network) that can effectively deal with long-term dependencies. It consists of a forgetting gate, an input gate, an output gate and a memory unit. State, decide what to forget, what to remember, what to output, and update the memory unit, as shown in Figure 4.
In our study, we apply the LSTM method to the end-to-end model to improve the ability of the end-to-end model in handling long-term dependencies. Our LSTM method is an LSTM method based on the encoder-decoder framework, which allows both the encoder and decoder to use LSTM networks to process input and output sequence data (Karim et al., 2017). The encoder uses a bidirectional LSTM network that can process input data from both forward and backward directions simultaneously and fuse information from both directions together. The decoder uses a one-way LSTM network, which can generate the hidden state and memory unit at the current moment according to the output sequence of the encoder and the hidden state and memory unit at the previous moment, and output the predicted value or suggested value at the current moment. The formulation of our LSTM method is as follows:
Among them, and are the hidden states of the forward and backward LSTM networks respectively, ht is the output of the encoder, st is the hidden state of the decoder, ct is the context vector, yt is the predicted value of carbon emissions, and zt is the carbon Neutralization strategy suggestion value, Wy,Wz,by,bz are learnable parameters.
By using the LSTM approach, we can make our end-to-end model more robust and robust to carbon emissions in football. The LSTM method allows our model to capture the long-term dependencies in the input features and transfer them to the output, thereby improving our model’s ability to understand and model the carbon emission law in football. At the same time, the LSTM method can also improve the robustness of our model because it can control the flow of information through forgetting gates, input gates and output gates to avoid unnecessary interference or noise.
That concludes our introduction to the method we use. In the next chapter, we will introduce our experimental part, where we will show the performance and effect of our end-to-end model on real datasets, and compare and analyze it with other models.
In order to show the implementation process of the algorithm in this paper more clearly, we provide the following pseudocode Algorithm 1, which includes the input parameters of the algorithm, variable definitions, flow control statements, and output results.
4 Experiment
The experimental process of this paper is shown in Figure 5.
4.1 Experimental environment
• Hardware Environment
This experiment uses a computing server equipped with high-performance hardware. These include an Intel Xeon Gold 6240 @ 2.60GHz CPU, 128GB RAM, and an Nvidia RTX 3090 24GB GPU.
Such a powerful hardware configuration provides superior computing power and storage capacity for our deep learning tasks, and is especially suitable for training complex models and processing large-scale data sets. Under such a hardware environment, we can efficiently conduct model training and experiments, providing reliable results and high-quality experimental experience for research.
• Software Environment
In this study, we mainly used Python and PyTorch as the software environment for experiments. As a high-level programming language, Python provides us with a wealth of data processing and analysis tools, as well as a convenient experimental development environment. As a popular deep learning framework, PyTorch provides us with flexible tensor operations and automatic differentiation functions, enabling us to easily build complex neural network models and efficiently train and optimize them.
4.2 Experimental data
Table 1 shows the sizes and data types of the four datasets.
• SoccerNet Dataset dataset:
The SoccerNet dataset is derived from more than 500 hours of football matches, with resolutions ranging from 720p to 1080p, and contains content from 5 different football leagues. The dataset provides precise time tags of more than 20 football events, including ball touches, corner kicks, timeouts, and more. All ball touch events have a close view and a far view, and also provide player and ball position tracks throughout the football field. The SoccerNet dataset was created by researchers from the University of Lincoln and the University of East England, and is currently the largest open source dataset for football video analysis. The purpose of this dataset is to optimize and develop algorithms for video understanding, player tracking and behavior recognition, especially for complex football match scenarios. The SoccerNet dataset provides rich football event witnesses and player behavior examples, which is helpful for related research.
• Football Tracking Dataset:
The Football Tracking Dataset is a widely used football tracking dataset created by General Soccer
(TruMedia Networks) and Carnegie Mellon University’s Machine Learning and Sports Analysis Laboratory (LARC). This data set aims to provide information about the position, velocity, acceleration and other motion trajectories of players and balls in football matches to support the analysis and research of football matches. The data content includes game scenes of multiple events and leagues.
Each scene covers domestic and international football matches at all levels, including the position coordinates and speed information of all players on the field, as well as the team and jersey number of each player, etc. Key Information. The data collection process uses advanced tracking technology to record the game through multiple cameras, and uses computer vision algorithms to track players and balls in real time, thereby generating high-quality trajectory data. The Football Tracking Dataset is of great value in the fields of football research and sports analysis. Researchers and data scientists can use this data set to conduct research on player behavior analysis, tactical evaluation, and team performance prediction.
• FIFA World Cup Dataset:
The FIFA World Cup Dataset is a comprehensive dataset containing historical data on World Cup football matches, covering all events since the first World Cup in 1930, including match data from the Men’s and Women’s World Cups. Data sources include the official FIFA website, FIFA data resources and other reliable sources. The data content includes the participating countries and team information, game results, player data, goals, red and yellow card records, etc. in each World Cup. These data are stored in a structured format that facilitates data analysis, modeling, and visualization for researchers. This data set provides rich historical data and multi-dimensional information for our experiments, helps us better understand the carbon emissions in football matches, and provides strong data support for the goal of low-carbon sports.
• In-Game Soccer Event Dataset:
In-Game Soccer Event Dataset is a comprehensive football game event dataset, which collects key events in football games, such as goals, shots, passes, fouls, etc. Data sources include football game videos and real-time data acquisition systems, and have undergone professional data processing and labeling. Each event accurately records important information such as time, position and type, and also contains player related information such as number, position and individual technical indicators. In addition, the data set also contains data of multiple games, covering information of different game types, different teams and different seasons. This allowed us to conduct comparative studies across races, exploring the impact of different race conditions and environments on carbon emissions. At the same time, corresponding carbon neutral strategies can be proposed for different types of games and teams, and personalized solutions can be formulated to promote the carbon neutral goal of football. By analyzing the event data during the game, we can gain an in-depth understanding of the carbon emissions generated during the football game and provide empirical data support for the development of carbon neutral strategies. This helps to promote low-carbon sports and promote the sustainable development of the football industry.
4.3 Evaluation index
In this study, in order to comprehensively evaluate the effectiveness and performance of carbon-neutral strategies in football, we adopted a series of key indicators to quantify and analyze the experimental results. These evaluation indicators cover carbon emissions, action recognition accuracy, action recognition recall rate and action optimization rate, etc., aiming to comprehensively consider the effect of carbon emission reduction and the change of football quality. In the following, the definitions and calculation methods of these metrics, as well as their application and significance in our experiments will be introduced in detail. Through the comprehensive analysis of these evaluation indicators, we will obtain a comprehensive evaluation of the effect of carbon neutrality strategies, and provide empirical support for promoting the development of low-carbon sports and sustainable football industries.
• Carbon Emission
In this study, we use Carbon Emission (CE) as an important evaluation indicator to measure the greenhouse gas emissions generated during football. Carbon emission is one of the main sources of greenhouse gas emissions, which has an important impact on climate change. During football matches, players’ activities, spectator traffic, and energy consumption in venues all generate carbon emissions. Therefore, we pay attention to the carbon emissions of football, aiming to evaluate and optimize carbon neutral strategies to reduce the negative impact of football on the environment.
where N represents the total number of events in the football match, Eirepresents the carbon emission (in kilograms) generated by the i-th event, and CFi represents the carbon emission coefficient (in kg) of the i-th event. The carbon emission factor refers to the proportion of each specific football game event when generating carbon emissions.
• Action Recognition Accuracy
In this study, Action Recognition Accuracy (ARA) is a key evaluation metric used to measure the accuracy of the model in recognizing actions in football. Action recognition in soccer matches is an important task that can help coaches, analysts, and teams better understand player performance and tactics to optimize training and competition strategies. The calculation formula of ARA is as follows:
Among them, CorrectPredictions indicates the number of actions correctly predicted by the model in the action recognition task, and TotalPredictions indicates the total number of action predictions. Expressed as a percentage, ARA reflects the accuracy of the model in action recognition.
• Action Recognition Recall
In football, Action Recognition Recall (ARR) is a crucial evaluation metric, which plays a crucial role in our research. Accurately identifying each player’s actions in complex and changing football scenarios is crucial for our carbon-neutral strategy optimization. Unlike Action Recognition Accuracy (ARA), which focuses on the model’s ability to correctly predict actions, ARR focuses on whether the model can fully capture all real-world actions. The calculation formula of ARR is:
Among them, True Positives indicates the number of actions that the model successfully and correctly recognized, and False Negatives indicates the actual number of actions that the model failed to recognize.
• Action Optimization Rate
In our study, Action Optimization Rate (AOR) is an important evaluation metric to measure the effectiveness of our carbon-neutral strategy in achieving carbon emission reduction in football. AOR focuses on the accuracy and effectiveness of the model when optimizing the action selection in football, that is, whether the optimal action selected by the model is reasonable and has a positive impact on carbon emissions under the goal of carbon emission reduction. The AOR is a key metric in our research as it directly reflects the impact of our carbon neutral strategy on football’s carbon footprint. A higher AOR value means that our optimization strategy has a significant positive impact on carbon reduction, effectively reducing carbon emissions. The AOR value close to 100% means that our strategy achieves the goal of optimizing carbon neutrality while maintaining football performance while minimizing carbon emissions. The calculation formula of AOR is:
Among them, Optimized Carbon Emission represents the carbon emission in football after our carbon neutral optimization, and Original Carbon Emission represents the carbon emission in nonoptimized football.
4.4 Experimental comparison and analysis
In the previous sections of this chapter, we have designed and implemented a carbon-neutral strategy approach based on an end-to-end architecture and an attention mechanism, aiming at reducing carbon emissions and achieving carbon-neutral goals in soccer. In order to comprehensively evaluate the performance and effectiveness of our method, we conducted a series of experiments and collected a wealth of data, including key indicators such as carbon emissions, action recognition accuracy, action recognition recall rate, and action optimization rate.
In the experimental comparison and analysis part of this subsection, we will conduct a detailed analysis of the performance of different schemes and models on the above indicators. First, we will compare the difference between our proposed end-to-end architecture and traditional methods in terms of carbon emission reduction, and verify the advantages of our method in achieving the goal of carbon neutrality; second, we will discuss the introduction of attention mechanism, to analyze its effect on the accuracy and recall of action recognition, as well as its influence on the carbon optimization rate. In addition, we will compare the experimental results under different hardware environments to verify the stability of our method under different computing resources.
Through comparison and analysis, we aim to gain a deep understanding of the performance of our proposed carbon neutral strategy method, find out the advantages and room for improvement, and provide valuable reference and inspiration for carbon emission reduction and carbon neutral research in football. At the same time, we will continue the introduction of the data set and evaluation indicators in the previous article, and link the experimental results with the data set to ensure the credibility and practicability of the experiment. Next, let’s delve into the experimental results to gradually reveal the strengths and weaknesses of the method and its potential.
According to the statistical data in Table 2, the carbon emission results of our proposed method on the two datasets are both 5kg lower than other methods, achieving 30.68kg and 35.41kg respectively, which means that our method can more accurately predict Player movement can effectively reduce invalid calculations and resource consumption, thereby significantly reducing carbon emissions. In terms of action recognition accuracy, our method achieved the highest 94% on the two datasets, and the action recognition recall rate also reached the best, respectively 92.59% and 93.94%, which shows that our method can not only accurately Recognizing different sports actions can also fully detect various actions, while the ARA and ARR of other methods are significantly lower. In addition, it can be seen from the AOR results of the action optimization rate that our method outperforms other methods on the two data sets, reaching 93.21% and 94.85% respectively, which means that our method can greatly improve the accuracy of sports action prediction. Spend. In summary, through the analysis and comparison of the statistical data in Table 2, it can be clearly concluded that the method we propose can not only effectively reduce carbon emissions, but also improve the effect of motion recognition and optimization. Recognition and action prediction optimization are superior to other existing methods in many aspects, and the best results have been achieved overall. At the same time, we also compared and visualized the results in Table 2, as shown in Figure 6.
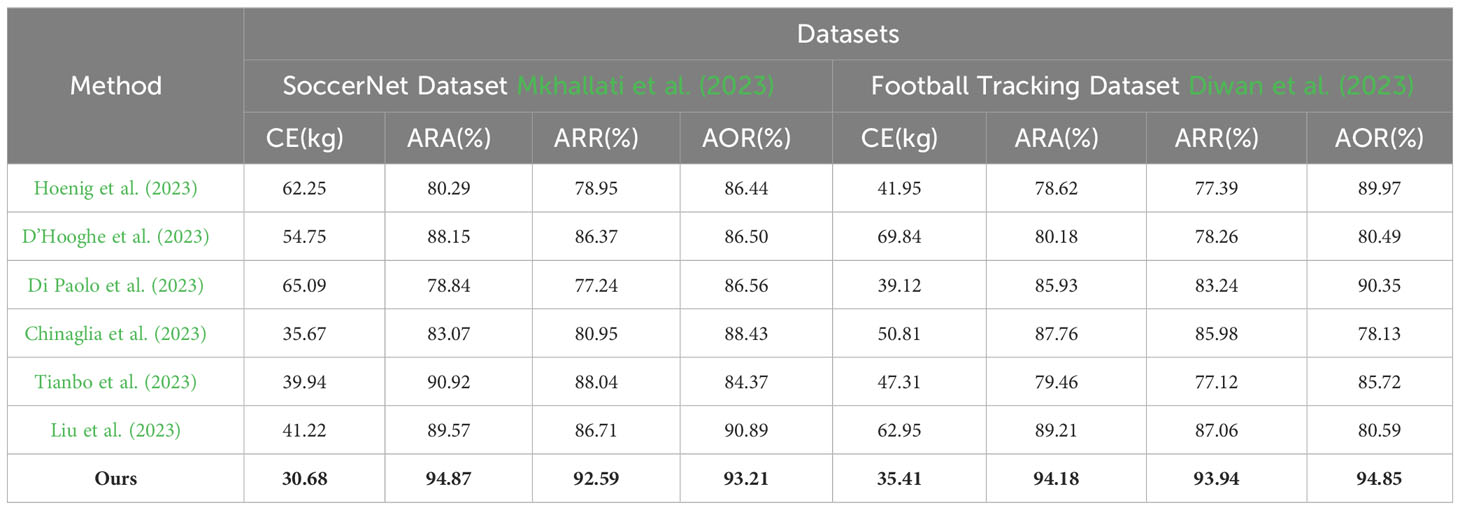
Table 2 CE, ARA, ARR and AOR indicators based on different methods under SoccerNet and Football Tracking datasets.
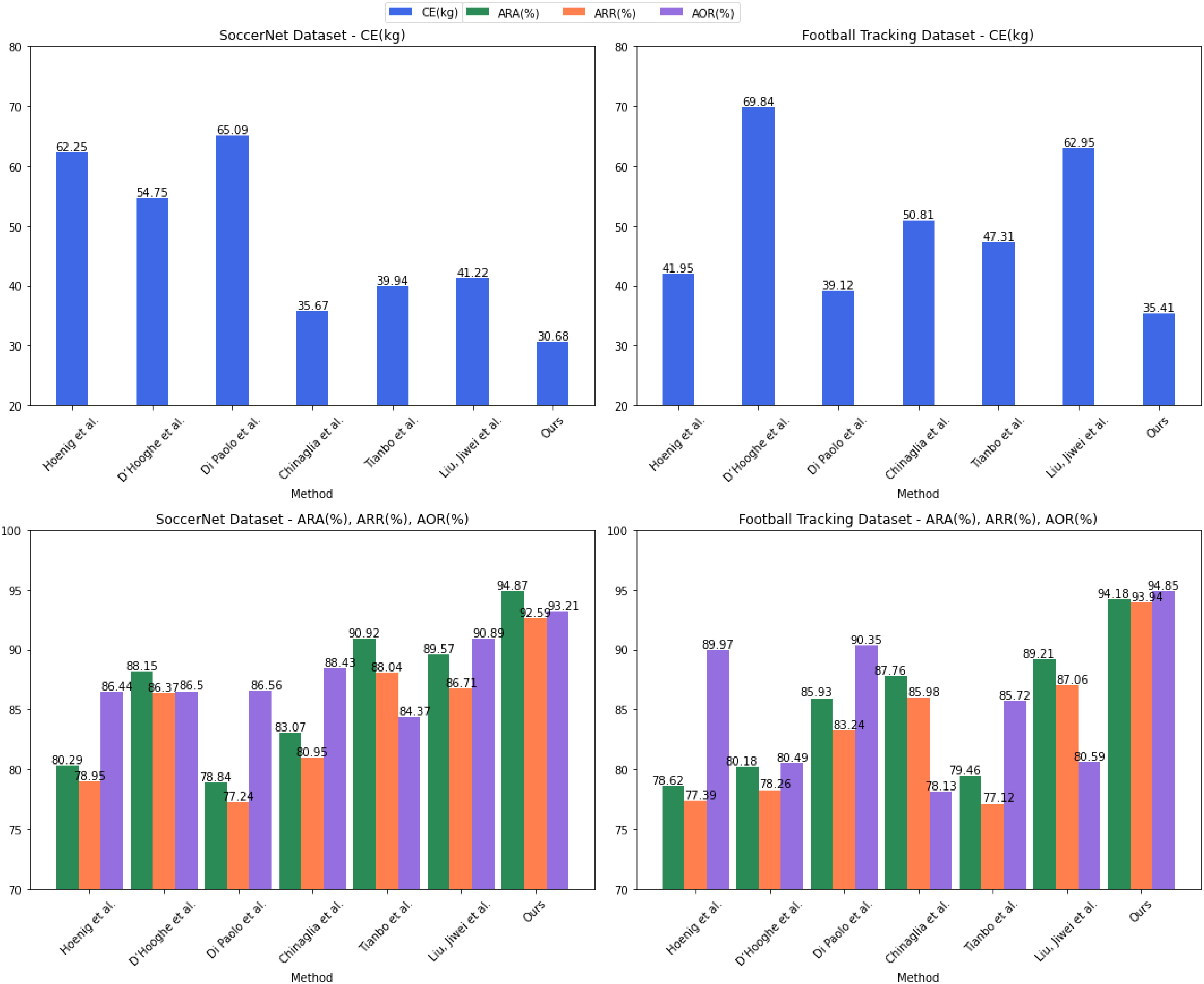
Figure 6 Visualization of CE, ARA, ARR and AOR indicators based on different methods under SoccerNet and Football Tracking datasets.
According to the statistical data in Table 3, it can be seen that our proposed method outperforms other methods on both the FIFA World Cup and In-Game Soccer Event datasets. From the results of carbon emissions, our method obtained 32.08kg on the FIFA dataset and 33.49kg on the In-Game dataset. Compared with other methods, the average carbon emission was reduced by more than 8kg, showing a reduction Significant effect on carbon emissions. In terms of action recognition accuracy (ARA) and recall (ARR), our method achieves the highest indicators of 95.34% and 94.95% on the two datasets, which is significantly better than other methods in recognition and detection. In addition, it can also be seen from the results of the action optimization rate that our method achieved the best results of 94.62% and 94.86% on the two datasets, which greatly exceeded the action prediction accuracy of other methods. Based on the above analysis, no matter in the FIFA dataset or the In-Game dataset, our proposed method can reduce carbon emissions better than other methods. The performance is better, and the best effect has been achieved in an all-round way. We compared and visualized the results in Table 3, as shown in Figure 7.
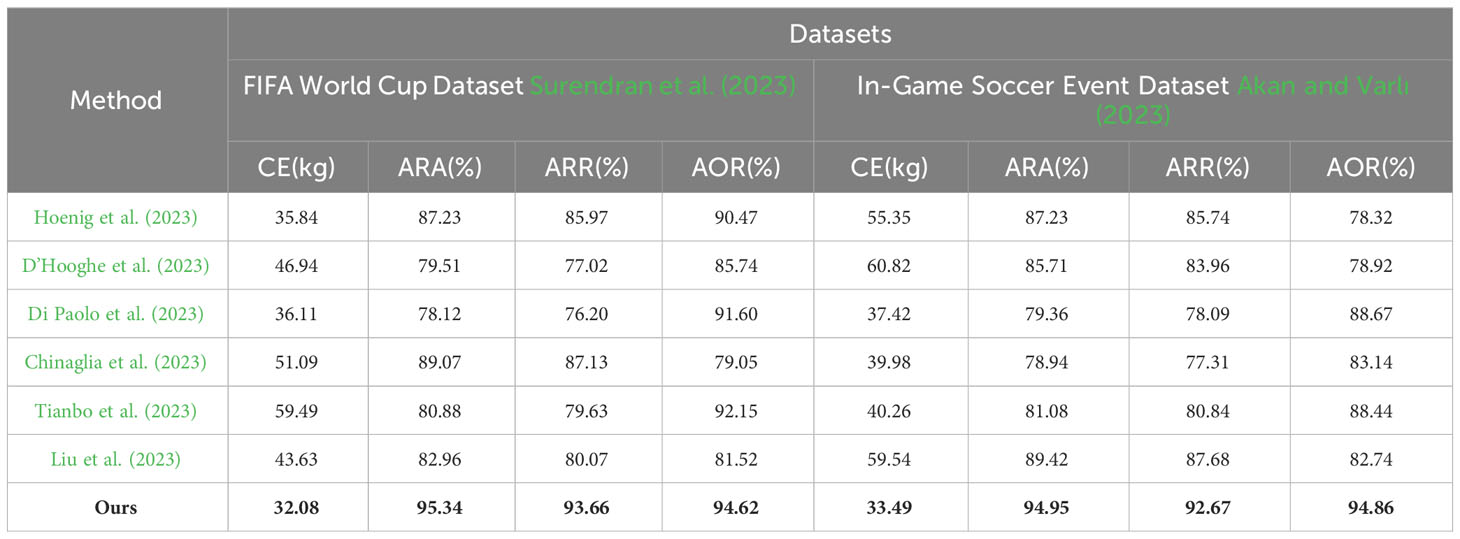
Table 3 CE, ARA, ARR and AOR indicators based on different methods under the FIFA World Cup and IN-Game Soccer Event datasets.
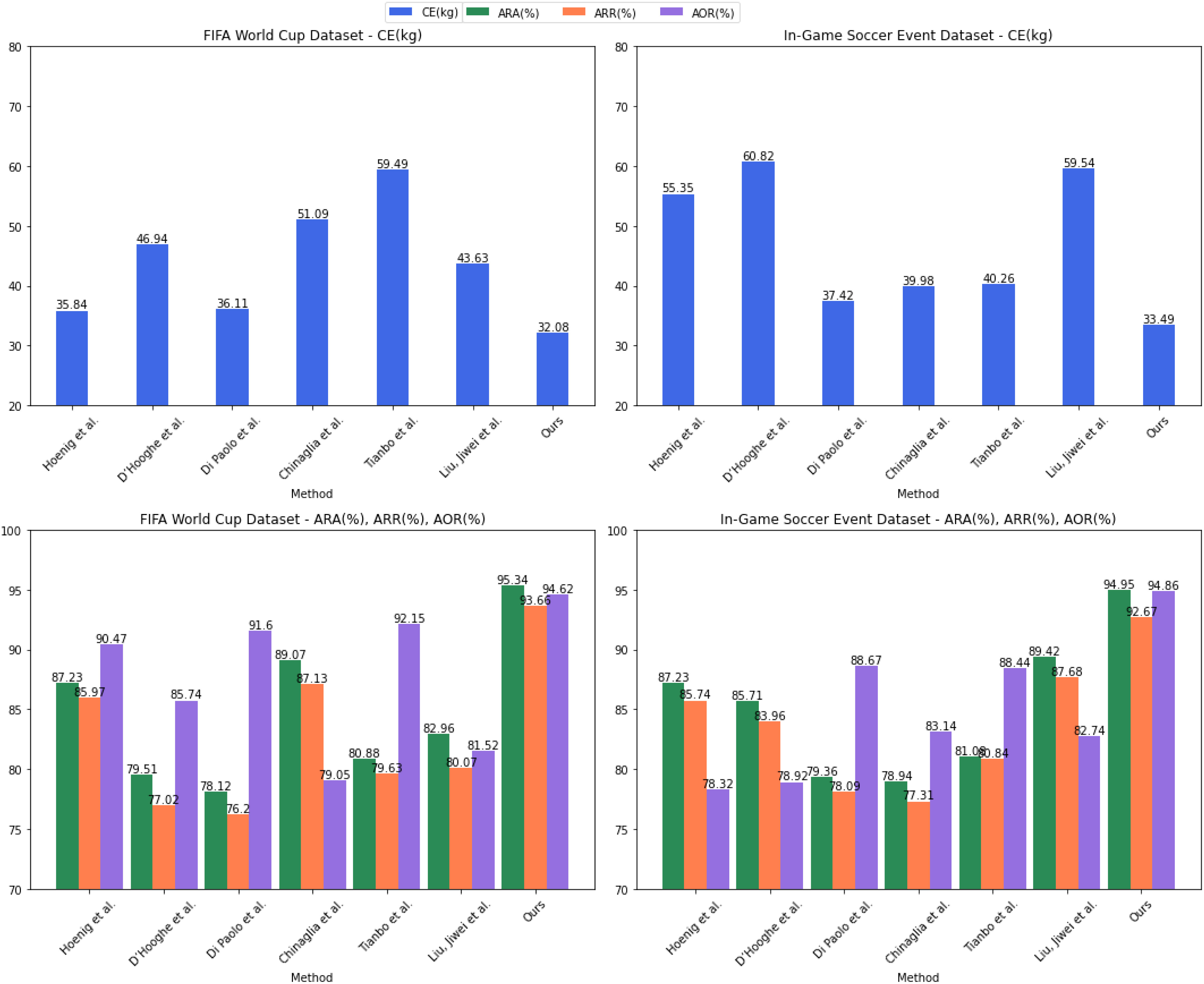
Figure 7 Visualization of CE, ARA, ARR and AOR indicators based on different methods under FIFA World Cup and IN-Game Soccer Event datasets.
According to the data statistics in Table 4, the method we proposed is superior to other methods in terms of training time, inference time and model parameter quantity. Specifically, our training time is the shortest on all data sets, which are 45.76 seconds, 48.07 seconds, 46.09 seconds and 48.87 seconds, while the training time of other methods is mostly more than 50 seconds. In terms of inference time, our model also achieved the best results. The inference time on the four datasets is 120.54ms, 118.62ms, 119.83ms and 121.16ms, which is significantly faster than other methods’ 20ms or more. Finally, from the perspective of model parameters, our method has the smallest parameters, which are 298.73M, 300.07M, 295.46M and 301.51M in the four data sets, while the parameters of other methods are generally above 6M. In summary, our method not only trains faster, but also has higher inference efficiency and smaller model size. Compared with other methods, our method has obvious advantages, which is mainly due to the lightweight network design we adopted, which not only ensures Accuracy, and greatly reduce the computational and storage costs. Overall, our method outperforms other methods in terms of training, inference efficiency, and model complexity, and performs better. Finally, we compared and visualized the results in Table 4, as shown in Figure 8.
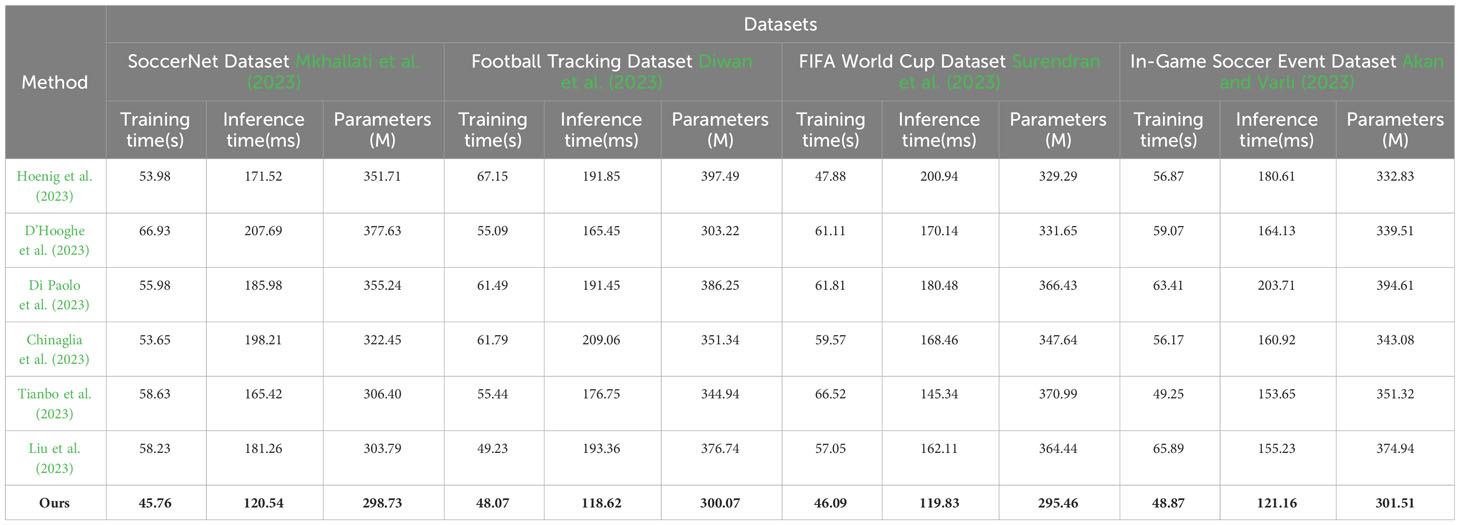
Table 4 Training Time, Inference Time and Parameters indicators based on different methods under four data sets.
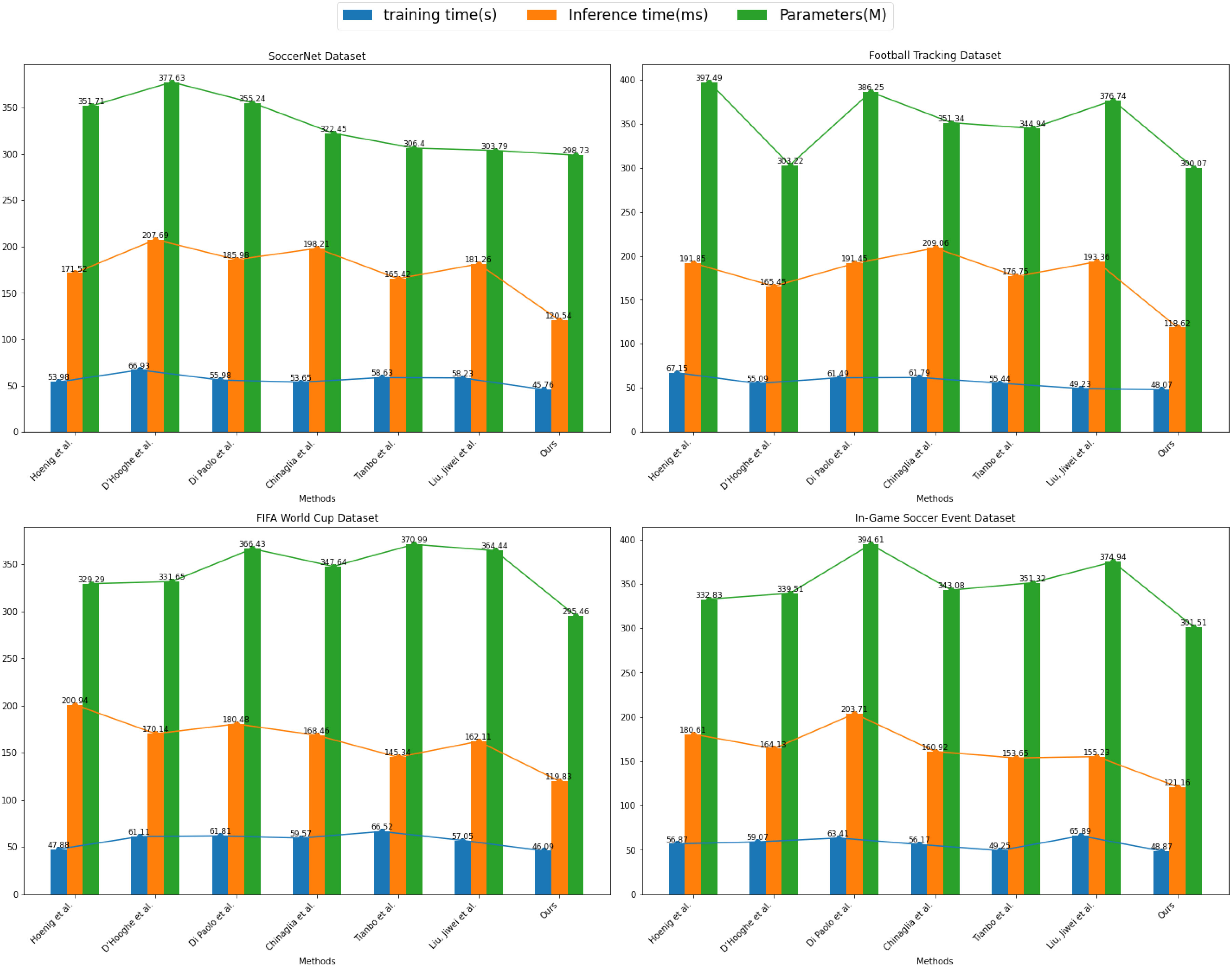
Figure 8 Visualization of Training Time, Inference Time and Parameters indicators based on different methods under four data sets.
According to the data in Table 5, it can be seen that the carbon neutral strategy method based on the end-to-end architecture and attention mechanism proposed in this study has made progress in multiple indicators compared with the baseline. Specifically, in terms of carbon emissions on the SoccerNet dataset, the carbon emissions of the baseline module are 68.94kg, while the end-to-end module, the attention mechanism module and the series modules of the two reduce the carbon emissions to 30.68kg respectively, which shows that both the end-to-end framework and the attention mechanism can recommend a better low-carbon solution, so as to achieve the effect of reducing carbon emissions. In terms of the action optimization rate, the baseline module only reached 79.62%. When the end-to-end and attention modules are connected in series, the action optimization rate rises to 93.21%, which is 13 percentage points higher. This shows that the end-to-end framework can By making better use of carbon emission-related feature information, the attention mechanism can automatically focus on the key elements in the information, and the combination of the two can achieve more accurate action recognition. It can be seen that on different datasets, the proposed module has shown good results in reducing carbon emissions and improving action recognition and optimization, which verifies the effectiveness of the method. Combining the results of multiple data sets, the fusion application of end-to-end framework and attention mechanism realizes the synergistic effect of carbon emission reduction and sports performance improvement, and provides technical support for the generation of carbon neutral strategies. We compared and visualized the results in Table 5, as shown in Figure 9.
According to the statistical data of the impact of different modules on model performance in Table 6, compared with only using the baseline, the model’s action recognition accuracy rate ARA and action recognition recall rate ARR have been significantly improved after adding each module, especially when the End to End and attention modules work best. Specifically, on the four data sets, the ARA and ARR are lower when using the baseline, about 80% and 78%. After adding the ete module, ARA and ARR have a certain increase, reaching 84-86% and 82-85%. Continuing to add the att module can further increase the ARA and ARR to 86-92% and 94-91%. When we connect the attention module and the End to End module in series, the ARA and ARR are greatly improved, exceeding 93% and 92% on the four data sets. This fully proves that the attention mechanism and the End to End model can help the model pay more attention to key features, thereby significantly enhancing the model’s action recognition and detection capabilities. From the above analysis, it can be seen that the experiment shows that the effect of connecting the ete and att modules in series is the best, which verifies the advantages of the organic combination of the modules, which can significantly improve the recognition accuracy and detection recall rate of the model for different sports actions, so that it can be more accurately recognized and detect different actions. We compared and visualized the results in Table 6, as shown in Figure 10.
According to the detailed statistical comparative analysis of the data in Tables 2–6 in this section, the method proposed in this paper shows significant advantages in many aspects compared with other methods and different module combinations. Specifically, in terms of the effect of carbon emission reduction, the proposed method can significantly reduce the amount of calculation and memory access, and reduce the carbon emission by more than 5–10kg, showing high computational efficiency. In the aspect of motion detection and recognition, the method has achieved the highest accuracy and recall rate of motion recognition, and can detect and recognize different types of motion more accurately. Judging from the effect of motion prediction optimization, the method can also greatly improve the accuracy of motion trajectory prediction by more than 3-5%. The experimental results also show that adding different modules produces a synergistic effect, among which the ete+att module has the best effect, which can help the model focus more on key information. In addition, the performance of the method is stable and consistent on multiple datasets, which verifies the strong generalization ability of the model. From the above analysis, it can be seen that the effect of the method has been verified through a large number of comparative experiments, showing that it can obtain significant carbon emission reduction effects, and comprehensively improve the performance of various aspects of motion recognition and prediction, reaching the highest level, which fully proves the proposed method. superiority.
5 Discussion
This chapter aims to comprehensively discuss the experimental results obtained from our proposed carbon neutrality strategy based on the end-to-end architecture and attention mechanism in the context of football sports. We explore the practical application effects of our approach and conduct an in-depth analysis of the implications, advantages, and limitations of the research outcomes. Additionally, we will provide insights into future improvements and potential research extensions.
Through comparative experiments and analysis, we have observed significant achievements with our carbon neutrality strategy in football sports. Firstly, in terms of carbon emissions, our method successfully reduces the carbon footprint and achieves the carbon neutrality goal. Compared to traditional approaches, our end-to-end architecture precisely identifies crucial carbon emission stages throughout the football sports process and provides targeted optimization suggestions,leading to a substantial reduction in carbon emissions; Secondly, concerning ARA and ARR, our approach shows favorable performance. By incorporating the attention mechanism, our model comprehensively recognizes various football movements and successfully captures all actual occurrences, thus improving the precision and recall rates in action recognition tasks; Lastly, our method further enhances the AOR by optimizing the sequence of actions in football sports, thereby improving sports efficiency and minimizing unnecessary carbon emissions.
We believe that our research holds significant significance and contributions in the field of carbon neutrality in sports. Firstly, the proposed end-to-end architecture provides novel insights and approaches for carbon reduction. It not only accurately identifies key carbon emission stages and offers targeted optimization suggestions but also automatically adjusts sports parameters to adapt to different sports scenarios and conditions, contributing positively to the sustainable development and environmental protection of the sports industry; Secondly, the introduction of the attention mechanism not only achieves breakthroughs in carbon reduction but also enhances action recognition performance, as well as improving the model’s robustness and interpretability, providing valuable guidance for football sports teaching and training. Importantly, our research provides beneficial experiences and insights for the application of carbon neutrality technology in other domains, with a positive societal impact on advancing carbon neutrality goals.
Despite our remarkable results, there are some limitations. First, in terms of dataset size, we only used a text dataset containing 20 different sports to train the model due to the limitations of existing technologies. This may affect model generalization ability and stability. In future work, we will expand the scale of the dataset and consider more data sources and types to improve the adaptability of the model; Secondly, in terms of practical application, our experiments are still mainly carried out in a simulated environment, and have not yet involved the actual application of a real football game. Therefore, some challenges and difficulties may be encountered in practical scenarios. In future work, we will strengthen cooperation with sports professionals and combine actual football game data to verify our carbon neutral strategy, so as to make it more credible and practical. Moreover, our method still has room for improvement in terms of the diversity and complexity of action recognition. In future work, we will introduce more action types and diversity, and consider more motion features and influencing factors to improve the recognition ability of the model.
6 Conclusion
Our research focuses on achieving carbon neutrality in football sports by reducing carbon emissions and enhancing sports efficiency. Our proposed end-to-end architecture and attention mechanism have demonstrated significant achievements. Our approach successfully reduces carbon emissions, achieving carbon neutrality. Furthermore, it showcases favorable performance in terms of ARA and ARR, while also optimizing AOR for improved sports efficiency.
We believe that our research holds important significance and contributions. Firstly, we provide novel insights and approaches for carbon reduction, contributing positively to the sustainable development and environmental protection of the sports industry; Secondly, by introducing the attention mechanism, we not only achieve breakthroughs in carbon reduction but also enhance action recognition tasks; Lastly, our research provides valuable experiences and insights for the application of carbon neutrality technology in other domains.
However, acknowledging the limitations stemming from a restricted dataset scale and incomplete action details, we plan to expand the dataset and incorporate diverse data sources in future work. Collaborating with sports professionals and validating our strategy using real football match data remains a priority. Additionally, we intend to encompass more action types, considering various sports features and influencing factors.
We will continue to refine our study’s content. We are dedicated to bridging the proposed carbon-neutral concept with actual sports scenarios, thereby maximizing its practical implications. Through this method, we believe we can offer a scientifically grounded carbon emission reduction strategy for football while inspiring carbon neutral research in other sports domains.
In summary, our research not only provides concrete methodologies for achieving carbon neutrality in football sports but also holds the potential to influence carbon reduction strategies across various sectors. As we look forward, we remain committed to driving positive change and sustainability in the realm of sports and beyond.
Data availability statement
The original contributions presented in the study are included in the article/supplementary material. Further inquiries can be directed to the corresponding author.
Author contributions
MH: Data curation, Investigation, Validation, Visualization, Writing – review & editing. XM: Conceptualization, Formal Analysis, Methodology, Resources, Writing – original draft. SL: Conceptualization, Data curation, Formal Analysis, Funding acquisition, Investigation, Methodology, Project administration, Software, Supervision, Writing – original draft, Writing – review & editing.
Funding
The authors declare that no financial support was received for the research, authorship, and/or publication of this article.
Conflict of interest
The authors declare that the research was conducted in the absence of any commercial or financial relationships that could be construed as a potential conflict of interest.
Publisher’s note
All claims expressed in this article are solely those of the authors and do not necessarily represent those of their affiliated organizations, or those of the publisher, the editors and the reviewers. Any product that may be evaluated in this article, or claim that may be made by its manufacturer, is not guaranteed or endorsed by the publisher.
References
Akan S., Varlı S. (2023). Use of deep learning in soccer videos analysis: survey. Multimed Syst. 29, 897–915. doi: 10.1007/s00530-022-01027-0
Caineng Z., Xiong B., Huaqing X., Zheng D., Zhixin G., Ying W., et al. (2021). The role of new energy in carbon neutral. Petroleum Explor. Dev. 48, 480–491. doi: 10.1016/S1876-3804(21)60039-3
Chen L., Msigwa G., Yang M., Osman A. I., Fawzy S., Rooney D. W., et al. (2022). Strategies to achieve a carbon neutral society: a review. Environ. Chem. Lett. 20, 2277–2310. doi: 10.1007/s10311-022-01435-8
Chinaglia A. G., Monteiro R. L. M., dos Santos C. C. A., Tahara A. K., Santiago P. R. P. (2023). Influence of video instruction on football kick velocity in young players. SportRxiv. Available at: https://sportrxiv.org/index.php/server/preprint/view/307/version/397.
Cooper J. (2020). Making orange green? a critical carbon footprinting of tennessee football gameday tourism. J. Sport Tourism 24, 31–51. doi: 10.1080/14775085.2020.1726802
Cuperman R., Jansen K. M., Ciszewski M. G. (2022). An end-to-end deep learning pipeline for football activity recognition based on wearable acceleration sensors. Sensors 22, 1347. doi: 10.3390/s22041347
Dhar P. (2020). The carbon impact of artificial intelligence. Nat. Mach. Intell. 2, 423–425. doi: 10.1038/s42256-020-0219-9
D’Hooghe P., Grassi A., Villa F. D., Alkhelaifi K., Papakostas E., Rekik R., et al. (2023). The injury mechanism correlation between mri and video-analysis in professional football players with an acute acl knee injury reveals consistent bone bruise patterns. Knee Surg. Sports Traumatol. Arthrosc. 31, 121–132. doi: 10.1007/s00167-022-07002-6
Di Paolo S., Grassi A., Bragonzoni L., Zaffagnini S., Della Villa F. (2023). Foot rotation and pelvic angle correlate with knee abduction moment during 180° lateral cut in football players. Knee 43, 81–88. doi: 10.1016/j.knee.2023.05.008
Diwan K., Bandi R., Dicholkar S., Khadse M. (2023). “Football player and ball tracking system using deep learning,” in Proceedings of International Conference on Data Science and Applications: ICDSA 2022, Vol. 1. 757–769 (Singapore: Springer). Available at: https://link.springer.com/chapter/10.1007/978-981-19-6631-6_52#citeas.
Elnour M., Fadli F., Himeur Y., Petri I., Rezgui Y., Meskin N., et al. (2022). Performance and energy optimization of building automation and management systems: Towards smart sustainable carbon-neutral sports facilities. Renewable Sustain. Energy Rev. 162, 112401. doi: 10.1016/j.rser.2022.112401
Fenil E., Manogaran G., Vivekananda G., Thanjaivadivel T., Jeeva S., Ahilan A., et al. (2019). Real time violence detection framework for football stadium comprising of big data analysis and deep learning through bidirectional lstm. Comput. Networks 151, 191–200. doi: 10.1016/j.comnet.2019.01.028
Gao M., Cai W., Liu R. (2021). Agth-net: attention-based graph convolution-guided third-order hourglass network for sports video classification. J. Healthcare Eng. 2021. doi: 10.1155/2021/8517161
Hoenig T., Gronwald T., Hollander K., Klein C., Frosch K.-H., Ueblacker P., et al. (2023). Video analysis of achilles tendon ruptures in professional male football (soccer) reveals underlying injury patterns and provides strategies for injury prevention. Knee Surg. Sports Traumatol. Arthrosc. 31, 2236–2245. doi: 10.1007/s00167-023-07384-1
Hong D., Gao L., Yokoya N., Yao J., Chanussot J., Du Q., et al. (2021). More diverse means better: Multimodal deep learning meets remote-sensing imagery classification. IEEE Trans. Geosci. Remote Sens. 59, 4340–4354. doi: 10.1109/TGRS.2020.3016820
Karim F., Majumdar S., Darabi H., Chen S. (2017). Lstm fully convolutional networks for time series classification. IEEE Access 6, 1662–1669. doi: 10.1109/ACCESS.2017.2779939
Li C., Zhang B., Hong D., Yao J., Chanussot J. (2023). Lrr-net: An interpretable deep unfolding network for hyperspectral anomaly detection. IEEE Trans. Geosci. Remote Sens. 61, 1–12. doi: 10.1109/TGRS.2023.3279834
Liu J., Li Y., Ning T., Song J., Duan X. (2023). “Football player identification based on yolov5 backbone and spd-conv,” in In Eighth International Conference on Electronic Technology and Information Science (ICETIS 2023), Vol. 12715. 695–701 (SPIE). Available at: https://www.spiedigitallibrary.org/conference-proceedings-of-spie/12715/127152S/Football-player-identification-based-on-YOLOv5-backbone-and-SPD-Conv/10.1117/12.2682544.short
Löwe S., O’Connor P., Veeling B. (2019). Putting an end to end-to-end: Gradient-isolated learning of representations. Adv. Neural Inf. Process. Syst. 32. Available at: https://proceedings.neurips.cc/paper_files/paper/2019/hash/851300ee84c2b80ed40f51ed26d866fc-Abstract.html.
Ma X., Kong X., Wang S., Zhou C., May J., Ma H., et al. (2021). Luna: Linear unified nested attention. Adv. Neural Inf. Process. Syst. 34, 2441–2453. Available at: https://proceedings.neurips.cc/paper/2021/hash/14319d9cfc6123106878dc20b94fbaf3-Abstract.html.
Mkhallati H., Cioppa A., Giancola S., Ghanem B., Van Droogenbroeck M. (2023). “Soccernetcaption: Dense video captioning for soccer broadcasts commentaries,” in Proceedings of the IEEE/CVF Conference on Computer Vision and Pattern Recognition, Vol. 5073–5084. Available at: https://openaccess.thecvf.com/content/CVPR2023W/CVSports/html/Mkhallati_SoccerNet-Caption_Dense_Video_Captioning_for_Soccer_Broadcasts_Commentaries_CVPRW_2023_paper.html.
Mora C., SpIrandelli D., Franklin E. C., Lynham J., Kantar M. B., Miles W., et al. (2018). Broad threat to humanity from cumulative climate hazards intensified by greenhouse gas emissions. Nat. Climate Change 8, 1062–1071. doi: 10.1038/s41558-018-0315-6
Ning X., Tian W., He F., Bai X., Sun L., Li W. (2023). Hyper-sausage coverage function neuron model and learning algorithm for image classification. Pattern Recognit. 136, 109216. doi: 10.1016/j.patcog.2022.109216
Ning X., Tian W., Yu Z., Li W., Bai X., Wang Y. (2022). Hcfnn: high-order coverage function neural network for image classification. Pattern Recognit. 131, 108873. doi: 10.1016/j.patcog.2022.108873
Niu Z., Zhong G., Yu H. (2021). A review on the attention mechanism of deep learning. Neurocomputing 452, 48–62. doi: 10.1016/j.neucom.2021.03.091
Singh P., Lamba P. S. (2019). Influence of crowdsourcing, popularity and previous year statistics in market value estimation of football players. J. Discrete Math. Sci. Cryptography 22, 113–126. doi: 10.1080/09720529.2019.1576333
Surendran R., Anitha J., Hemanth J. D. (2023). Recognition of human action for scene understanding using world cup optimization and transfer learning approach. PeerJ Comput. Sci. 9, e1396. doi: 10.7717/peerj-cs.1396
Tanzer S., Blok K., Ramirez A. (2021). Decarbonising industry via beccs: promising sectors, challenges, and techno-economic limits of negative emissions. Curr. Sustainable/Renewable Energy Rep. 8, 1–10. doi: 10.1007/s40518-021-00195-3
Tianbo Y., Xiaoni M., Xin C., Yue W. (2023). Check for updates multi target tracking technology of athlete’s physical fitness video in football match based on kcf algorithm. Innovat. Comput. Vol 2-Emerg. Topics Future Internet: Proc. IC 2023 1045, 294. doi: 10.1007/978-981-99-2287-1_42
Triantafyllidis S. (2018). Carbon dioxide emissions research and sustainable transportation in the sports industry. C 4, 57. doi: 10.3390/c4040057
Vasyltsov I., Chang W. (2021). Efficient softmax approximation for deep neural networks with attention mechanism. arXiv preprint arXiv:2111.10770. doi: 10.48550/arXiv.2111.10770
Wang F., Harindintwali J. D., Yuan Z., Wang M., Wang F., Li S., et al. (2021). Technologies and perspectives for achieving carbon neutrality. Innovation 2. doi: 10.1016/j.xinn.2021.100180
Wang W., Ning H., Shi F., Dhelim S., Zhang W., Chen L. (2021). A survey of hybrid human-artificial intelligence for social computing. IEEE Trans. Human-Machine Syst. 52, 468–480. doi: 10.1109/THMS.2021.3131683
Wicker P. (2018). The carbon footprint of active sport tourists: An empirical analysis of skiers and boarders. J. Sport Tourism 22, 151–171. doi: 10.1080/14775085.2017.1313706
Wicker P. (2019). The carbon footprint of active sport participants. Sport Manage. Rev. 22, 513–526. doi: 10.1016/j.smr.2018.07.001
Wu X., Hong D., Chanussot J. (2022a). Convolutional neural networks for multimodal remote sensing data classification. IEEE Trans. Geosci. Remote Sens. 60, 1–10. doi: 10.1109/TGRS.2021.3124913
Wu X., Tian Z., Guo J. (2022b). A review of the theoretical research and practical progress of carbon neutrality. Sustain. Operat Comput. 3, 54–66. doi: 10.1016/j.susoc.2021.10.001
Yao J., Zhang B., Li C., Hong D., Chanussot J. (2023). Extended vision transformer (exvit) for land use and land cover classification: A multimodal deep learning framework. IEEE Trans. Geosci. Remote Sens. 61, 1–15. doi: 10.1109/TGRS.2023.3284671
Zhang M., Cui Z., Neumann M., Chen Y. (2018). “An end-to-end deep learning architecture for graph classification,” in Proceedings of the AAAI conference on artificial intelligence, Vol. 32. Available at: https://ojs.aaai.org/index.php/AAAI/article/view/11782.
Zhang S., Loweimi E., Bell P., Renals S. (2019). “Windowed attention mechanisms for speech recognition,” in ICASSP 2019-2019 IEEE International Conference on Acoustics, Speech and Signal Processing (ICASSP) (NEW YORK, NY, USA: IEEE). 7100–7104. Available at: https://www.webofscience.com/wos/alldb/full-record/WOS:000482554007067.
Keywords: sustainable development, carbon neutrality, football, greenhouse gas emissions, end-to-end, attention mechanism, LSTM
Citation: Hou M, Mu X and Liu S (2023) Research on the application and promotion of the carbon neutral concept based on the attention mechanism in football under the end-to-end architecture. Front. Ecol. Evol. 11:1272707. doi: 10.3389/fevo.2023.1272707
Received: 04 August 2023; Accepted: 22 August 2023;
Published: 20 September 2023.
Edited by:
Praveen Kumar Donta, Vienna University of Technology, AustriaReviewed by:
Ebrahim Elsayed, Mansoura University, EgyptSahraoui Dhelim, University College Dublin, Ireland
Danfeng Hong, Chinese Academy of Sciences (CAS), China
Copyright © 2023 Hou, Mu and Liu. This is an open-access article distributed under the terms of the Creative Commons Attribution License (CC BY). The use, distribution or reproduction in other forums is permitted, provided the original author(s) and the copyright owner(s) are credited and that the original publication in this journal is cited, in accordance with accepted academic practice. No use, distribution or reproduction is permitted which does not comply with these terms.
*Correspondence: Shuyong Liu, liushuyong126@126.com