- 1U.S. Geological Survey, Western Ecological Research Center, Boulder, NV, United States
- 2Department of Biology, California State University Northridge, Northridge, CA, United States
- 3Department of Biology, Willamette University, Salem, OR, United States
- 4Department of Geography, University of Nevada – Reno, Reno, NV, United States
Introduction: Forecasting range shifts in response to climate change requires accurate species distribution models (SDMs), particularly at the margins of species' ranges. However, most studies producing SDMs rely on sparse species occurrence datasets from herbarium records and public databases, along with random pseudoabsences. While environmental covariates used to fit SDMS are increasingly precise due to satellite data, the availability of species occurrence records is still a large source of bias in model predictions. We developed distribution models for hybridizing sister species of western and eastern Joshua trees (Yucca brevifolia and Y. jaegeriana, respectively), iconic Mojave Desert species that are threatened by climate change and habitat loss.
Methods: We conducted an intensive visual grid search of online satellite imagery for 672,043 0.25 km2 grid cells to identify the two species' presences and absences on the landscape with exceptional resolution, and field validated 29,050 cells in 15,001 km of driving. We used the resulting presence/absence data to train SDMs for each Joshua tree species, revealing the contemporary environmental gradients (during the past 40 years) with greatest influence on the current distribution of adult trees.
Results: While the environments occupied by Y. brevifolia and Y. jaegeriana were similar in total aridity, they differed with respect to seasonal precipitation and temperature ranges, suggesting the two species may have differing responses to climate change. Moreover, the species showed differing potential to occupy each other's geographic ranges: modeled potential habitat for Y. jaegeriana extends throughout the range of Y. brevifolia, while potential habitat for Y. brevifolia is not well represented within the range of Y. jaegeriana.
Discussion: By reproducing the current range of the Joshua trees with high fidelity, our dataset can serve as a baseline for future research, monitoring, and management of this species, including an increased understanding of dynamics at the trailing and leading margins of the species' ranges and potential for climate refugia.
1 Introduction
Accurate geographic distribution information for sensitive species is fundamental to evaluating habitat changes in response to disturbances and environmental variation (Pitelka and Plant Migration Workshop Group, 1997), and for conservation science and resource management planning (Guisan and Thuiller, 2005; Neilson et al., 2005; Elith and Leathwick, 2009). Short of mapping the location of every individual in a species, geographic distributions are frequently studied using species distribution modeling (SDM), a collection of statistical methods to correlate species presence and absence records with spatially explicit environmental factors, and to predict probabilities of species’ presences in locations where direct observation is not available (Franklin, 1995; Guisan and Thuiller, 2005). Practitioners often use SDMs to close the gap between incomplete records of a species’ presence on the landscape and its full geographic range. However, SDMs are limited by the power of the modeling methods used, the selection of relevant environmental variables used as predictors, and, perhaps most critically, the quality of input observation data (e.g., Phillips et al., 2009; Lobo and Tognelli, 2011; Bean et al., 2012).
Range-wide distribution modeling can be hindered by the lack of robust presence and absence data across broad areas occupied by the focal species (Brown and Griscom, 2022). Acquiring presence and/or absence data may be challenging because of species crypsis, difficulties accessing remote habitats, or the sheer size of many widespread species’ distributions. Moreover, while presence data may often be incomplete, true absence data for many species are simply unobtainable (Lobo et al., 2010), because many animals easily travel into areas that would seem unlikely as habitat. This is especially true for volant species such as birds and bats, or large mammals with increased capacity for movement across landscapes. Many models are instead estimated using “pseudoabsence” data drawn at random from locations within a known or estimated dispersal range from presence locations (Lobo and Tognelli, 2011; Barbet-Massin et al., 2012). Because most SDM methods hinge on the contrast between environmental conditions at presence and absence locations, the method of pseudoabsence selection influences a model’s power to identify the environmental factors that meaningfully contribute to habitat suitability (e.g., VanDerWall et al., 2009).
One recent alternative is afforded by remote-sensing datasets, which are increasingly accessible and offer the potential to develop high-resolution distribution data encompassing both presence and true absence information. For a species that can be reliably identified in satellite imagery or LiDAR (Light Detection And Ranging) scans, it should be possible to collect presence and absence records in regions that may be inaccessible to direct survey, at a spatial resolution and geographic scope limited only by the remote-sensing method (Esque et al., 2020a; Hu et al., 2021). High quality remotely sensed satellite data are available near human population centers, but quality declines in remote areas, and regions where national security concerns preempt public availability of high-resolution imagery (e.g., near Department of Energy and Department of Defense installations; http://apps.nationalmap.gov/lidar-explorer/).
Joshua trees (Yucca brevifolia Engelm. and Y. jaegeriana McKelvey ex Lenz; Figure 1) offer a unique demonstration of the possibilities created by high-resolution remote sensing. The two species are sister taxa of large tree-like yuccas broadly inhabiting four states and an area upwards of 25,000 km2 at low to middle elevations across the Mojave Desert ecoregion (McKelvey, 1938; Rowlands, 1978; Lenz, 2007). In most of the plant communities where they occur, Joshua trees are the largest plants on the landscape (reproductive individuals grow >2 m tall), which has facilitated the collection of unusually comprehensive presence records to inform species distribution models (Godsoe et al., 2009; Cole et al., 2011; Smith et al., 2011). The trees’ association with the Mojave Desert more broadly has made them a focal species for the use of SDMs to predict plant community shifts in response to projected climate change (Cole et al., 2011; Barrows and Murphy-Mariscal, 2012; Sweet et al., 2019; Smith et al., 2023) as well as historical distribution changes since the last glacial maximum (Smith et al., 2011). However, even in the case of these well-studied, conspicuous species, SDMs published to date have significant limitations. The largest observation datasets for Joshua trees contain only validated presence records and rely on pseudoabsences for SDM estimation (Godsoe et al., 2009). Observation records in these datasets are also distributed unevenly across the Mojave Desert, limited by the accessibility of remote habitats in which the trees occur and substantial regions where access is restricted for national security concerns.
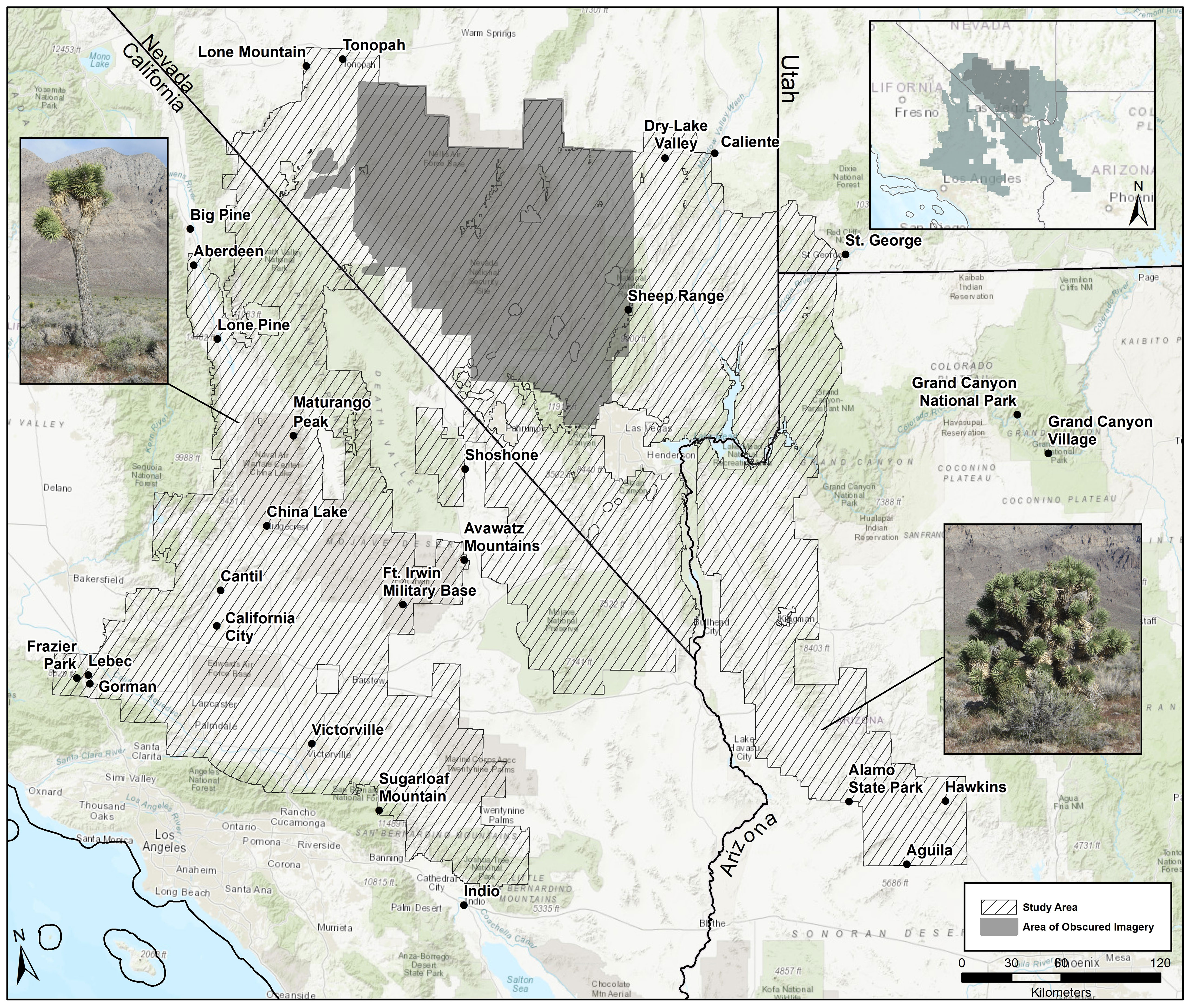
Figure 1 Study area, placenames, and area of obscured imagery. Photograph on left is an example of the western Joshua tree (Yucca brevifolia), which is unbranched for the first 2 m of its stem; photograph on right is the eastern Joshua tree (Y. jaegeriana), which has many branches from as low as 1 m. Photo credit – Christopher I. Smith.
The division of the two species presents a further complication: the possibility that they are adapted to different climate regimes in the eastern and western Mojave. The two species of Joshua tree were formally recognized following the discovery that they are each exclusively pollinated by separate, sister species of yucca moths with obligate seed-feeding larvae (Pellmyr and Segraves, 2003; Lenz, 2007; Godsoe et al., 2008). Yucca jaegeriana and Y. brevifolia hybridize in a narrow conterminous zone, where the moths’ (Tegeticula. antithetica, and T. synthetica; respectively) host specificity is thought to be the primary barrier to gene flow (Smith et al., 2008; Starr et al., 2013). Genome-wide patterns of differentiation between the two Joshua tree species, however, indicate that the moths are not solely responsible for maintaining reproductive isolation, and other environmental factors, such as climate differences between the eastern and western Mojave, may also contribute (Starr et al., 2013; Royer et al., 2016; Royer et al., 2020). The most comprehensive range-wide SDM studies of Joshua trees have attempted to compare the range of climates in which the two species grow and find that they occupy overlapping climate regimes (Godsoe et al., 2009), but studies done at the highest spatial resolution have only been conducted within the range of Y. brevifolia, in Joshua Tree National Park and its vicinity (Barrows et al., 2019).
Understanding Joshua trees’ climate requirements has become more urgently necessary to plan for their conservation in the face of a suite of interlocking threats to the natural communities of the Mojave Desert (Smith et al., 2023). Recruitment of Joshua trees requires that seedlings survive a gauntlet of life history challenges (Reynolds et al., 2012). Furthermore, increasingly frequent wildfires fueled by invasive introduced grasses (Loik et al., 2000; DeFalco et al., 2010; Reynolds et al., 2012; St. Clair et al., 2022), land use changes (Esque et al., 2020b; Esque et al., 2020c; Smith et al., 2023; State of California, 2023) and climate change (Dole et al., 2003; Cole et al., 2011; Barrows and Murphy-Mariscal, 2012; Sweet et al., 2019) all complicate conservation and management. Slow growing and long-lived plant species with wide distributions are frequently left out of conservation planning (Kwit et al., 2004), but widespread concern for Joshua tree populations has prompted petitions to the California Fish and Game Commission and the US Fish and Wildlife Service to protect them (WildEarth Guardians, 2015; California Fish and Game Commission, 2019; State of California, 2023). Listing under the Endangered Species Act was determined to be not warranted (USFWS, 2023), and the Western Joshua Tree Conservation Act (State of California, 2023) was passed. However, high-resolution distribution mapping and demographic information remain major unknowns in understanding the population status of Y. jaegeriana and Y. brevifolia across their ranges.
Identifying presence and absence of adult Joshua trees can be easier than for many other species because of their conspicuous height and unique branching patterns (reproductive plants >2 m tall), occurring among sparse desert shrubs with shorter canopies. Pre-reproductive Joshua trees (usually <2 m tall) are mostly undetectable because they cast a small shadow and primarily exist within the canopy of nurse plants (Esque et al., 2021). Adults can be reliably identified using commercial satellite data (i.e., Google Maps, satellite view) or Light Detection and Radar Data (LiDAR; Esque et al., 2020a). Image-based empirical maps of the trees’ distribution can be used with SDMs to enhance representative rangewide habitat suitability maps and explore occupied and potential habitat with great accuracy (Brown and Griscom, 2022).
Here, we paired remotely sensed satellite data with species distribution modeling of Joshua trees across the Mojave and portions of the Sonoran Deserts (132,441 km2), evaluated those data using extensive field validation with other spatially explicit data sources, and provide high-resolution distribution maps for Y. brevifolia and Y. jaegeriana. We found we could reliably identify adult Joshua trees at a resolution of 0.25 km2 and validated the trees’ presence and absence across 672,043 grid cells to develop a nearly comprehensive distribution dataset to inform SDM-based range mapping for this iconic species. We use these data to understand the underlying environmental variables related to Joshua tree distributions and to compare habitat, modeled habitat, and potential habitat between these two closely related species. We distinguish habitat as currently occupied areas derived directly from empirical presence/absence data, modeled habitat as the regions identified by SDMs as having a high probability of species presence, and potential habitat as modeled habitat that is outside of the empirical habitat area (e.g., where occupancy was not detected in imagery or field surveys). Our distribution maps represent the first rangewide, survey-based models for both species and can inform decision-makers and the public about the status of Joshua trees, describe the environmental factors that shape their current distributions, help establish an informed network for demographic monitoring, and provide the foundation for high-resolution predictions of future and paleoclimate distributions.
2 Methods
2.1 Study area
The study area encompasses 132,441 km2, including the Level III Mojave Desert ecoregion and some adjacent ecoregions (Omernik and Griffith, 2014) in Utah, Arizona, Nevada, and California, USA (Figure 1). The study area perimeter was determined using the probability threshold of 0.3 or higher from a previous species distribution model for Joshua trees (Godsoe et al., 2009) and based on field observation. Baseline survey elevations were from 400 m to 2,200 m to encompass the range of Joshua trees but exclude extraneous search areas because of the size of the study area. The elevational range across the study region was −86 m in Death Valley, California, to 3,632 m at the summit of Charleston Peak, Clark County, Nevada.
2.2 Initial surveys using satellite imagery
Publicly available Google Earth satellite imagery was used to identify presence or absence of adult Joshua trees of both species (Esque et al., 2020a). We created a structured data set for both species with a repeatable protocol (Isaac et al., 2020). Rather than a stratified sampling design, we aimed to sample 100% of the study area at 500 m resolution. Using the Fishnet toolbox in ArcMap 10.5 we created a grid of 811,900 500 m × 500 m cells in the USA Contiguous Albers Equal Area Conic USGS coordinate system (SR-ORG:7301). Observers inspected 672,043 of the survey cells during our initial mapping phase, encompassing 82.7% of the gridded study area. The remainder of the gridded study area, or 139,857 cells, occurred in south-central Nevada near US Department of Energy and Defense facilities (i.e., National Nuclear Testing Facility and Nellis Air Force Base) where we found obscured imagery presumably due to national security concerns. Thus, presence/absence determinations were precluded in this area (Figure 2). As an alternative, we modeled Joshua tree habitat in this region using SDM algorithms based on the true presence and absences for the rest of both species’ ranges (see below).
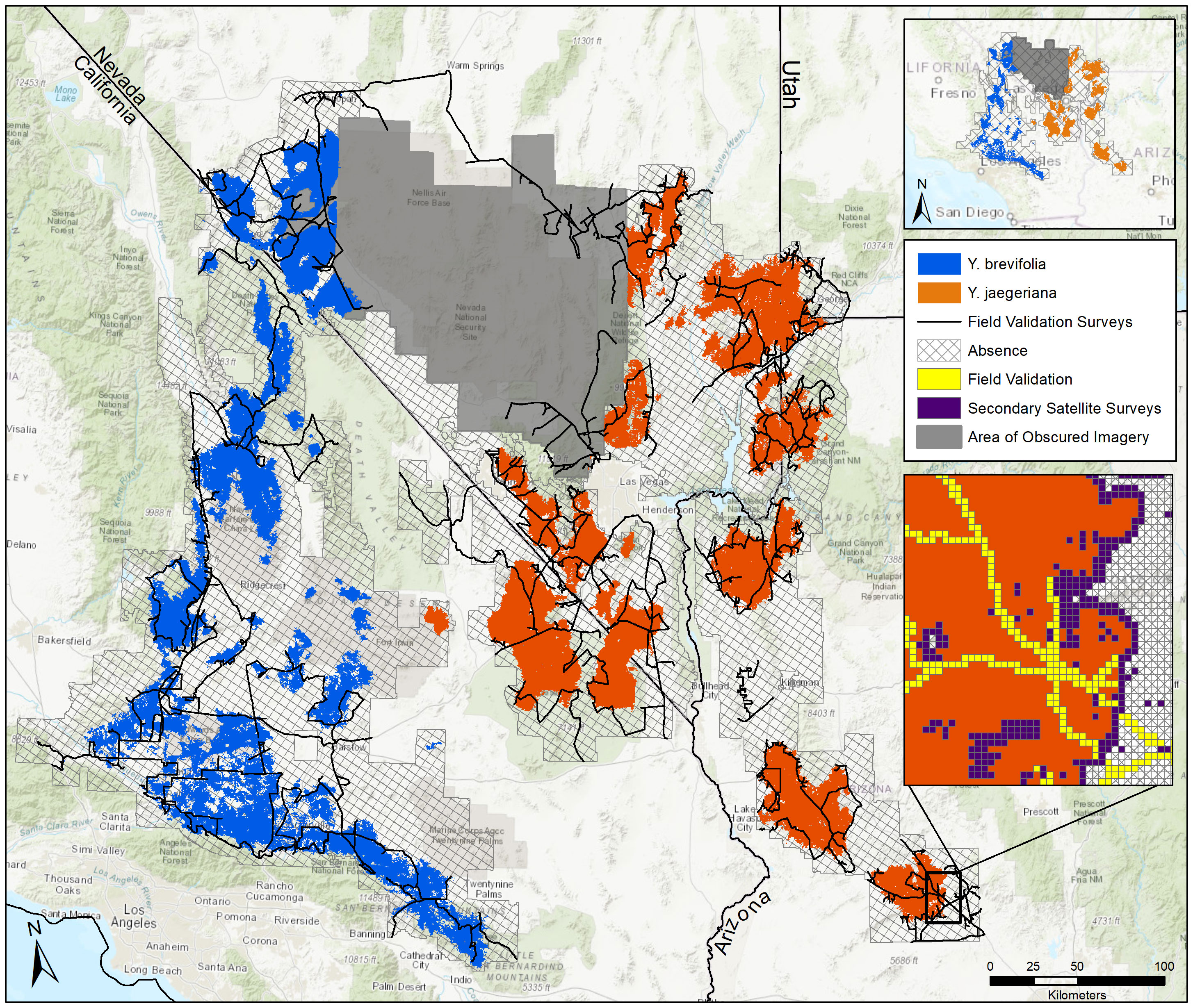
Figure 2 Geographical distribution for Y. brevifolia and Y. jaegeriana illustrating true presences and absences and the areas of obscured imagery where field and satellite surveys were missing. Inset is an example of field validation and secondary satellite surveys across the distributions for both species.
Visual scans of adult Joshua tree presence and absence were conducted at a standardized eye elevation of ~250 m (i.e., the altitude above the land surface) with a target search time of roughly 45 s per cell. Observers either placed waypoints within each cell at the location of a prominent Joshua tree or designated absence. Surveys of satellite imagery were mostly limited to adult Joshua trees (usually branched trees >2 m height) because initial field surveys indicated that smaller trees are usually not distinguishable, and those <1 m tall are undetectable (Esque et al., 2021).
2.3 Refinement of habitat map
The quality of Google Earth imagery was variable, but usable, across the gridded study area except for the region of obscured imagery described above (see Completing coverage for Joshua tree distributions in obscured area using SDMs). The most recent imagery (2021) was evaluated first, but if presence/absence was not readily assigned because of image quality, the eye altitude or time frame within the historical satellite imagery (2003 to 2021) was varied to try and detect Joshua trees. Remaining questionable cells were re-evaluated using secondary satellite surveys (see below).
We further refined the habitat map by correcting errors using field validation, secondary satellite searches, empirical point data from co-authors’ unpublished datasets, points provided by staff at Nellis Air Force Base, filtered research grade iNaturalist observations (GBIF, 2023), and some SEInet observations (Esque et al., 2020a; SEINet, 2023). Field validation included 15,001 km of driving along both paved and dirt roads during twenty site visits throughout the grid system (29,050 cells; Figure 2). Where site visits were not possible, the most experienced observers re-evaluated cells using secondary satellite searches (from any year available, with the best imagery) in the following three scenarios: 1) cells determined to be without Joshua trees but adjacent to cells having Joshua trees present; 2) cells having Joshua trees present but surrounded by cells without Joshua trees; and 3) cells in areas known to cause confusion because of other issues (e.g., plants, rocky outcrops, fire scars, the urban/wildland interface). Data from iNaturalist and SEInet that were inconsistent with our database were also field validated.
2.4 Environmental variables
We derived 18 environmental variables to serve as covariates in the species distribution models (SDMs), which together characterize climate, topography, vegetation (e.g., NDVI variables), and soil surface properties for the study region (Table 1). Precipitation and temperature layers were created using ClimateNA v. 7.3 (Wang et al., 2016), which downscales PRISM data (Daly et al., 2008) and corrects for elevational variation. Our contemporary climate analyses included data from the 30-year period between 1980–2010. Satellite metrics incorporating plant canopy and soil surface data from the moderate-resolution imaging spectroradiometer (MODIS) satellite were averaged across 17 y (2003–2020) to represent a norm for the study region (NDVI amplitude and maximum – USGS eMODIS Remote Sensing Phenology, https://doi.org//10.5066/F7PC30G1). A layer representing soil surface texture was downloaded and mosaicked from the SoilGrids 2.0 web portal (Poggio et al., 2021). All topographic metrics were calculated by aggregating a 30 m digital elevation model to the 500 m × 500 m resolution used for modeling (National Elevation Dataset, http://ned.usgs.gov/).
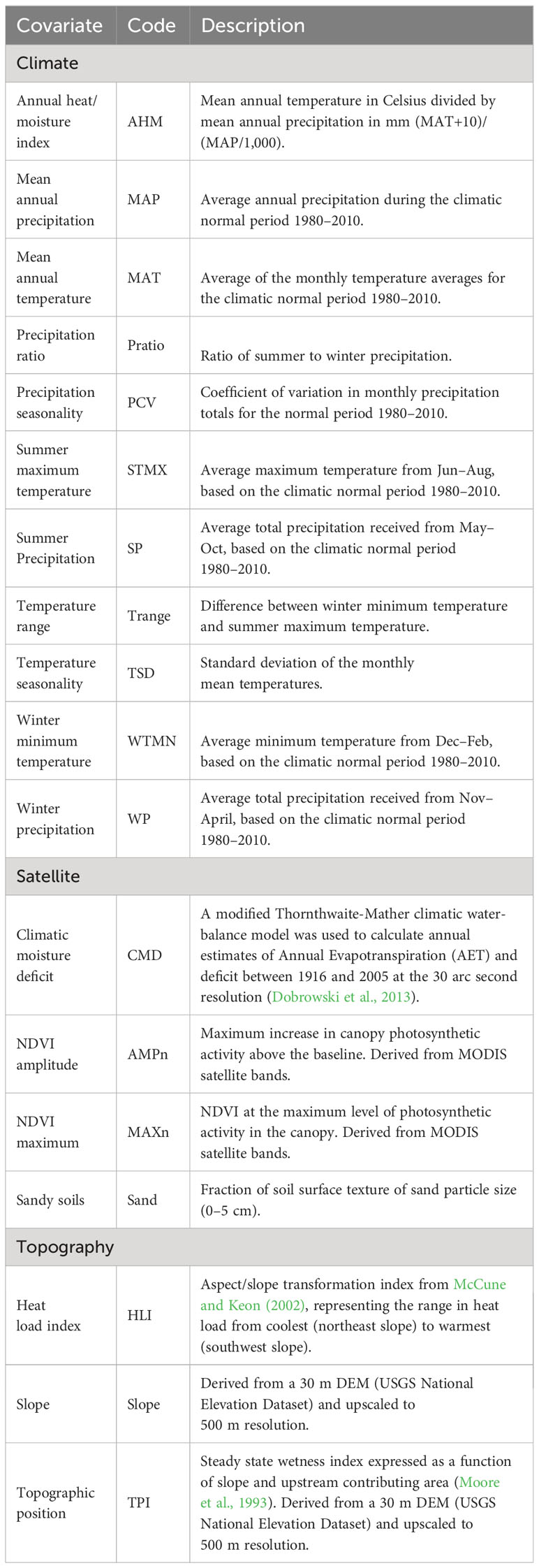
Table 1 Environmental covariates (climate, satellite, and topography) used to fit species distribution models (SDMs) for eastern and western Joshua tree species.
Climatographs were generated for Y. brevifolia and Y. jaegeriana from the final corrected presence grid cells using gaussian kernel density estimates from cell values for each environmental variable. The climatographs were used to compare the climate between adult Joshua tree species’ occupied habitat and can be compared with partial response curves resulting from SDMs.
2.5 Species distribution modeling
We used an ensemble modeling approach to create SDMs for Y. brevifolia, Y. jaegeriana, and a rangewide model with both species’ datasets (hereafter, rangewide model). Presence and absence points were assigned to each species’ range based on Rowlands (1978); Lenz (2007), and author’s unpublished genetic data (CIS). The species are non-overlapping except in the hybrid zone which was obscured by poor imagery; in that area the data for both species were used in combination for modeling (i.e., species specific points were not designated). Next, we fit SDMs to the individual species occurrences separately, along with a rangewide SDM featuring all points (i.e., from both species). We used a custom script in R version 4.1.3 (R Core Team, 2022) to implement model cross-validation, model averaging, parallel processing, and partial response curves for model terms. Our ensemble modeling approach included two algorithms: generalized additive models (GAM; R package “mgcv” version 1.8-22; Wood, 2017) and random forests (RF; R package “ranger” version 0.12.1; Wright and Ziegler, 2017). Both algorithms have been consistently strong performers among SDM algorithms (Franklin, 2010). However, due to consistently better cross-validation performance, we only fit RF models for the rangewide dataset, whereas both GAM and RF were compared for the individual species models. All GAM models were fit with restricted maximum likelihood (REML) and an extra penalty allowing smooth terms to be penalized to zero (“gam” option select=TRUE in “mgcv” package) to aid model selection. Random forest models were fit with 1,500 random trees and “ranger” package defaults for the regression model (Wright and Ziegler, 2017).
For each algorithm and dataset, we considered eight candidate models that included 10 uncorrelated terms and another 8 terms used such that we avoided multicollinearity as environmental predictors (Table S1). All models included the same 10 uncorrelated terms (NDVI amplitude – AMPn, NDVI maximum – MAXn, HCL, precipitation seasonality – PCV, precipitation ratio – Pratio, Sand, Slope, topographic position – TPI, temperature seasonality – TSD, and temperature range – Trange; Table 1), but varied with respect to eight additional terms to avoid multicollinearity (Climate moisture deficit – CMD, Annual heat/moisture index – AHM, mean annual precipitation – MAP, mean annual temperature – MAT, summer precipitation – SP, summer maximum temperature – STMX, winter precipitation – WP, and winter temperature minimum – WTMN). This set of models allowed us to contrast seasonal and annual climate variables which, though correlated, may relate to different critical life stages or phenological processes for the different Joshua tree species.
To account for potential bias due to spatial aggregation (Veloz, 2009), and for computational efficiency, we rasterized presence and absence points to the modeling resolution (500 m × 500 m) and applied a spatial thinning procedure in which a maximum of 50 points could be randomly sampled from any 5 km2 area (Fourcade et al., 2014). This procedure reduced data density without changing the spatial arrangement of points. Following this procedure, the models included 128,438 points for Y. brevifolia (including presences and absences), 114,705 points for Y. jaegeriana, and 198,305 points for the rangewide model (note: rangewide points are not additive because of potential species overlap in a large area). This procedure was repeated five times for each species and for the rangewide dataset. Next, for each set of thinned points, we applied a 5-fold cross-validation procedure to split the data into training folds (used for model fitting) and testing folds (used for model evaluation). Overall, this process resulted in 25 total cross-validation runs used to evaluate models (5 sets of randomly thinned points × 5-fold cross-validation). It has been shown that conventional random cross-validation may underestimate model error and/or transferability, particularly when applied to spatially structured data (Bahn and McGill, 2012; Wenger and Olden, 2012). For this reason, we used a spatial cross-validation approach developed by Valavi et al. (2019) and implemented in the R package “blockCV” v2.1.4. Using the packages’ “spatial blocking feature”, we split data into random training and testing blocks that were both geographically separated and optimized to contain relatively equal numbers of presences and absences. With this procedure, we intended to reduce overfitting to the training data while identifying models that were generalizable and performed well across the study extent.
To measure model performance of the cross-validated models, we considered several metrics of model prediction accuracy including AUC (i.e., the area under the receiver operating characteristic; Fielding and Bell, 1997), the Boyce Index (Hirzel et al., 2006), and the True Skill Statistic (TSS; Allouche et al., 2006). For GAM, we also calculated each model’s average AIC (with each model being fit to the same subsets of data during cross validation) to help identify well-performing, parsimonious models. These metrics were averaged across cross-validation runs for each model to obtain an overall estimate of performance. Moreover, for each cross-validation run, we also generated model predictions as raster grids for the study extent constituting the predicted habitat suitability probabilities. We then created a final prediction raster for each model as the weighted average of the 25 individual cross-validation predictions based on the TSS scores (such that better performing model predictions from the cross-validation runs featured more heavily in the final raster prediction surface for each model). Similarly, we created overall algorithm ensemble predictions as the weighted average of raster predictions from individual models, again based on TSS scores. For each ensemble model, we also calculated model calibration curves by binning the predicted habitat probalities into 5 probability classes of width 0.2 (e.g., 0–0.2, 0.2–0.4, etc.) and comparing the midpoints of each bin with the observed frequency of presences within that subset of data. Calibration curves are useful in illustrating how well habitat probabilities correspond to the actual frequency of presence observations.
To aid model interpretation, we derived relative importance values for each predictor present in the candidate models for each algorithm. Relative importance for predictors in random forest models were based on the mean decrease in accuracy from permutations leaving out each term, while for GAM, relative performance was based on the parameter’s chi-square statistics. We also derived partial variable response curves for each of the top nine predictors present in the candidate models for each species, as well as the rangewide dataset. These curves indicate the shape and direction of relationships between predictors and habitat probability values. For GAM, response curve functions for predictors were also averaged across all the models in which each predictor occurred to create a model-averaged response curve for each predictor, which we overlay on the individual curves from candidate models. For random forest models, we calculated partial dependence curves using the R package “pdp” (Greenwell, 2017).
2.6 Modeled and potential habitat
We define the modeled habitat for Joshua trees as areas where SDM-predicted habitat probability values were above the threshold that maximized the sum of model sensitivities (true positive rate) and specificities (true negative rate; Liu et al., 2005). SDM ensemble habitat layers were thresholded separately for each species and for the combined dataset. Potential habitat for Joshua trees includes locations with modeled habitat where empirical Joshua tree presences were not detected (e.g., where there were no presence points from surveys). We quantified the area of habitat resulting from empirical distribution data versus the modeled and potential habitat generated by SDMs.
2.7 Comparison of habitat overlap
To compare the modeled habitats of Y. brevifolia and Y. jaegeriana, we calculated Schoener’s D statistic and the I statistic defined in Warren et al. (2009), both measures of environmental overlap which range from 0 (no overlap in covariates) to 1 (complete overlap). As a second measure, we calculated AUC values for two scenarios: (1) Y. brevifolia’s habitat probabilities (from surveyed presences) within the SDM predicted for Y. jaegeriana and projected across the full range of both species; and (2) Y. jaegeriana’s habitat probabilities (from surveyed presences) within the SDM predicted for Y. brevifolia and projected across the full range. These calculations illustrate how well each species’ individual SDMs predict occupied habitat for the other species, as well as whether any such relationships are spatially symmetric. Finally, to contrast the environmental tolerances of each species, we overlaid the response curves for environmental predictors that were among the top nine predictors in the individual SDMs for either species (overlaid separately for GAM and random forest). These response curve overlays contrast the relationships between each species’ modeled habitat by each environmental covariate.
3 Results
3.1 Refinement of habitat map
Field validation of 29,050 grid cells resulted in status changes for 3,703 of them (12.8%) (i.e., changes from absence to presence, or vice versa; Table S2). Experienced observers re-evaluated 76,578 cells using secondary satellite searches, changed the status of 36,857 cells (48.1% of re-surveyed cells, or 5.5% of all cells surveyed). The majority of these cells were changed from absence to presence (72.4% of changed cells; Table S2).
3.2 Joshua tree habitat
Based on the gridded image surveys and final corrections, Yucca jaegeriana currently occupies more than 16,683 km2 of habitat (excluding the area of obscured imagery) in the eastern Mojave Basin and Range ecoregion, and conterminous ecotones of Sonoran Basin and Range, Arizona/New Mexico Mountains, and Southern Basin and Range ecoregions (Figure 2; Omernik and Griffith, 2014). Nevada has the greatest amount of occupied Y. jaegeriana habitat (7,137 km2), followed by Arizona, California, and Utah (Table 2). Yucca jaegeriana generally occurs in 11 large and homogeneous populations, along with a few smaller peripheral populations. Unoccupied cells within these populations are rare (Figure 2). Occupied cells outside the well-defined populations are infrequent and mostly within 0.4 to 2.0 km of population edges, with only two individual cells isolated by >3 km. While uncommon, such isolates occur throughout the range of Y. jaegeriana (77 out of 102,274, 0.25 km2 grid cells, Figure 2). The elevational limits of Y. jaegeriana are 392 m near Alamo State Park, AZ and 2,319 m in the Sheep Range, NV. The latitudinal limits are near Aguila, AZ in the south and Dry Lake Valley, near Caliente, NV in the north. Longitudinal limits are near Hawkins, AZ in the east, and the Avawats Mountains on Ft. Irwin Military Base, CA in the west.
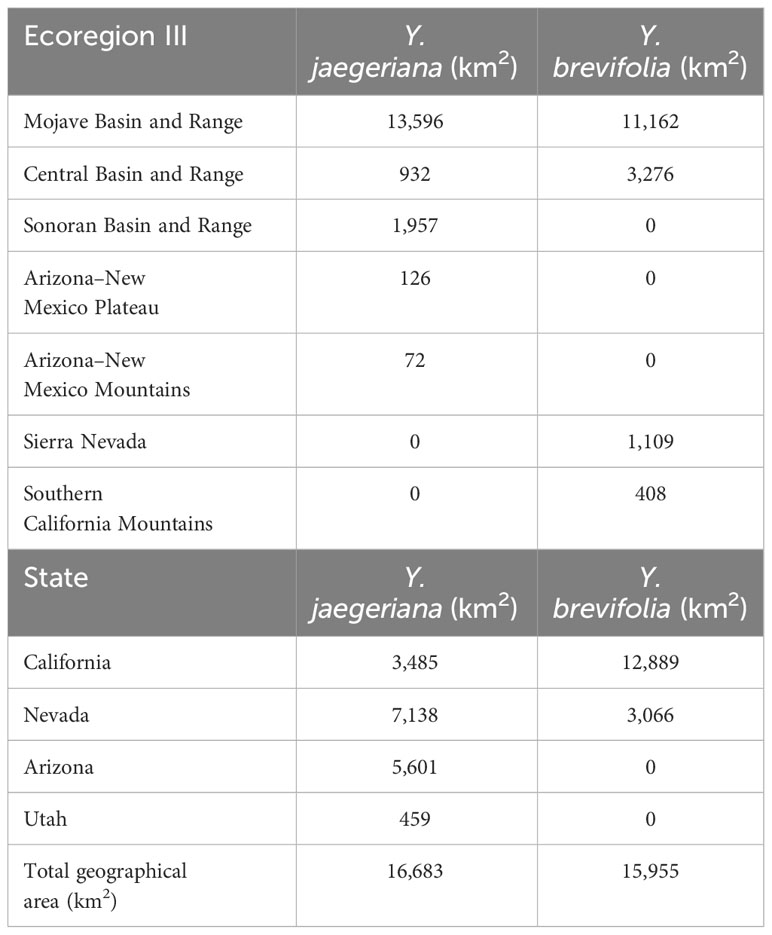
Table 2 Area of geographic distribution (i.e., occupied habitat in square km) for Yucca juaegeriana and Yucca brevifolia by Class III Ecoregions and States.
Yucca brevifolia occupies 15,955 km2 of habitat (excluding the area of obscured imagery) in the western Mojave Basin and Range ecoregion and adjacent Sierra Nevada and Southern California Mountain ecoregions (Omernik and Griffith, 2014; Figure 2). California has the most occupied habitat for Y. brevifolia followed by Nevada. Native populations do not occur in Utah or Arizona (Table 2). Yucca brevifolia has an extensive population on the southwestern edge of its range with many narrow pinch points throughout. Besides another large stand in the northwest periphery of its range that is contiguous with the region of obscured imagery, there are several smaller isolated populations of Y. brevifolia southward and eastward throughout California (Figure 2). Occupied cells that are isolated outside of large Joshua tree stands are infrequent with 170 cells isolated by <3 km and only 2 occupied cells isolated by >3 km from larger populations. In the southwestern range of Y. brevifolia, unoccupied clusters of cells are more frequently interspersed within occupied populations, creating a more diffuse pattern of occupancy in comparison with Y. jaegeriana (Figure 2). The elevational limit of Y. brevifolia is 600 m near Cantil, CA and the upper is 2,605 m on Maturango Peak, CA on the Naval Air Weapons Station – China Lake. The latitudinal limits are from near Indio, CA in the south and just south of Tonopah, NV in the north, and longitudinal limits are near Twentynine Palms in the east and the western limit is currently at the junction of Orwin Way Road and the Quail Canyon Motocross Road in Los Angeles, Co, CA.
3.3 Environmental variables within species habitats
Climatographs were used to display the frequency distributions of environmental variables within occupied Joshua tree habitat (Figure 3). While annual heat/moisture index (AHM) and winter precipitation (WP) had similar distributions between Y. brevifolia and Y. jaegeriana habitats, other variables – particularly summer precipitation (SP), precipitation ratio (Pratio), temperature standard deviation (TSD), and precipitation coefficient of variation (PCV) – showed marked discrepancies (Figure 3). Yucca jaegeriana experiences a greater range of temperature variation (higher TSD) than Y. brevifolia throughout the year, but a more even precipitation distribution (i.e., lower PCV). Interestingly, due to differences in the precipitation regime, Y. jaegeriana and Y. brevifolia share a similar profile of annual aridity (AHM), despite Y. jaegeriana experiencing higher mean annual temperatures (MAT). For Y. brevifolia, annual precipitation is largely restricted to the winter months due to its western position in the Mojave precipitation gradient. However, Y. jaegeriana receives more bi-modal precipitation benefitting from tropical monsoonal storms in its easterly position (Hereford et al., 2006; Figure 3), and consequently higher annual precipitation. However, the climatic moisture deficit model (based on Dobrowski et al., 2013), which incorporates additional variables beyond MAP and MAT (e.g., downward shortwave radiation and wind velocity), suggested that Y. jaegeriana has a greater overall moisture deficit than Y. brevifolia in portions of its range.
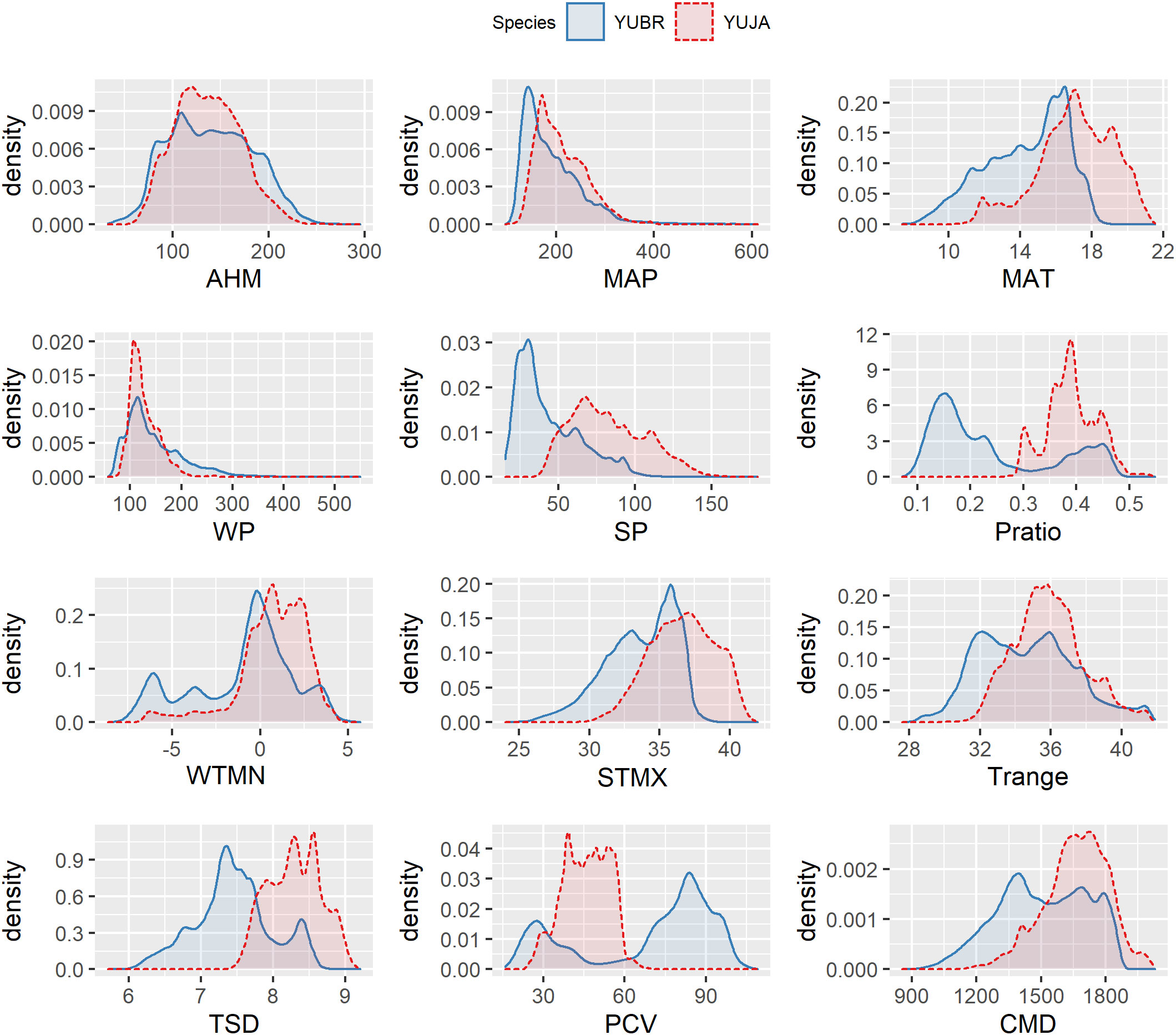
Figure 3 Climatographs showing relationships between locations where Yucca brevifolia (YUBR) and Y. jaegeriana (YUJA) occur and the environmental variables associated with these locations on a 0.25 km2 grid across the ranges of both species. Graphs are derived from gaussian kernel density estimates for each variable.
3.4 Species distribution models
SDMs predicted the Joshua tree habitats with high model performance (Tables 3, S3). Most AUC values from spatial cross-validation were greater than 0.8, and scores for the algorithm ensemble models were higher for RF than for GAM (Table 3). The RF model for the rangewide map performed similarly compared to the separate species models, with an AUC = 0.868 and R2 = 0.809 (Table 3, Figure 4). Given that spatial cross-validation is a more stringent approach for model evaluation than traditional random cross-validation (i.e., models are scored based on their ability to predict into distinct geographic areas), these metrics suggest that the SDMs are generalizable rather than overfit and accurate in their predictions across the full distribution. Inter-model standard deviations were generally low (<0.2 in habitat probabilities) but more pronounced in areas with fewer data points, such as the northern part of the range where empirical coverage is low. Standard deviations for the individual species models were also higher where we extrapolated predictions into the range of the other species to facilitate habitat comparisons (Figures 5, 6), but these areas were typically of lower predicted habitat suitability, and the rangewide ensemble SDM is not affected by this issue. Model calibration curves for the ensemble models indicated that, collectively, the models tended to predict somewhat higher probabilities than observed presences at the low end of values (<0.4), and somewhat lower probabilities than expected at the higher end (Figure S1). However, the estimates were close enough to the observed values that they would not result in mispredictions when the model threshold is applied to determine a suitability cutoff.
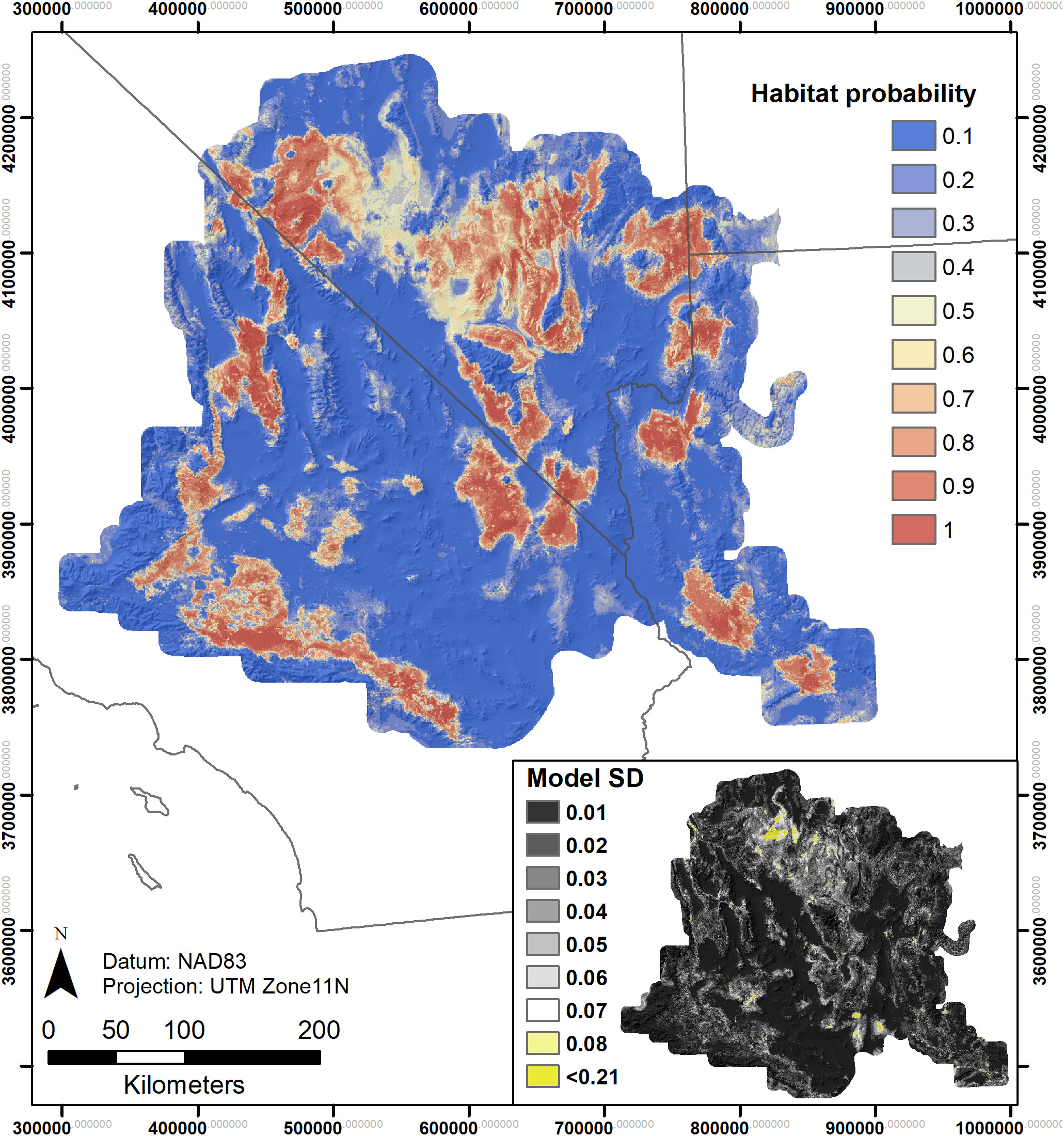
Figure 4 Rangewide species distribution model using presence and absence data for both Y. jaegeriana and Y. brevifolia – based on ensemble modeling of eight candidate standard deviations (SDs) in predicted probabilities across candidate models.
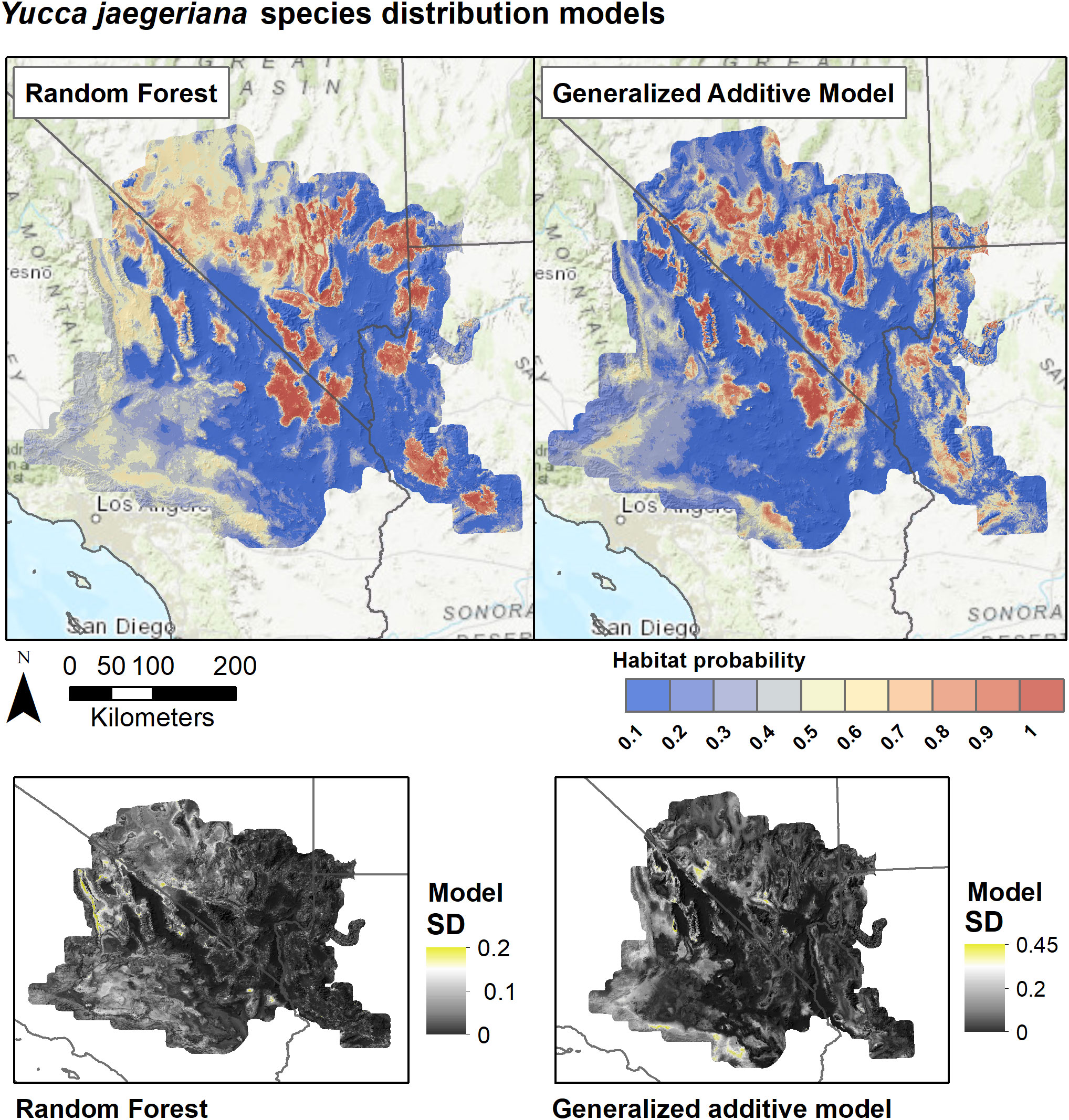
Figure 5 Species distribution models for Y. jaegeriana based on Random Forests and Generalized Additive Models. SDMs for each algorithm are derived as the weighted average of predictions from eight individual candidate models based on the True Skill Statistic. Lower panels show the standard deviation of predictions among the candidate models for each algorithm.
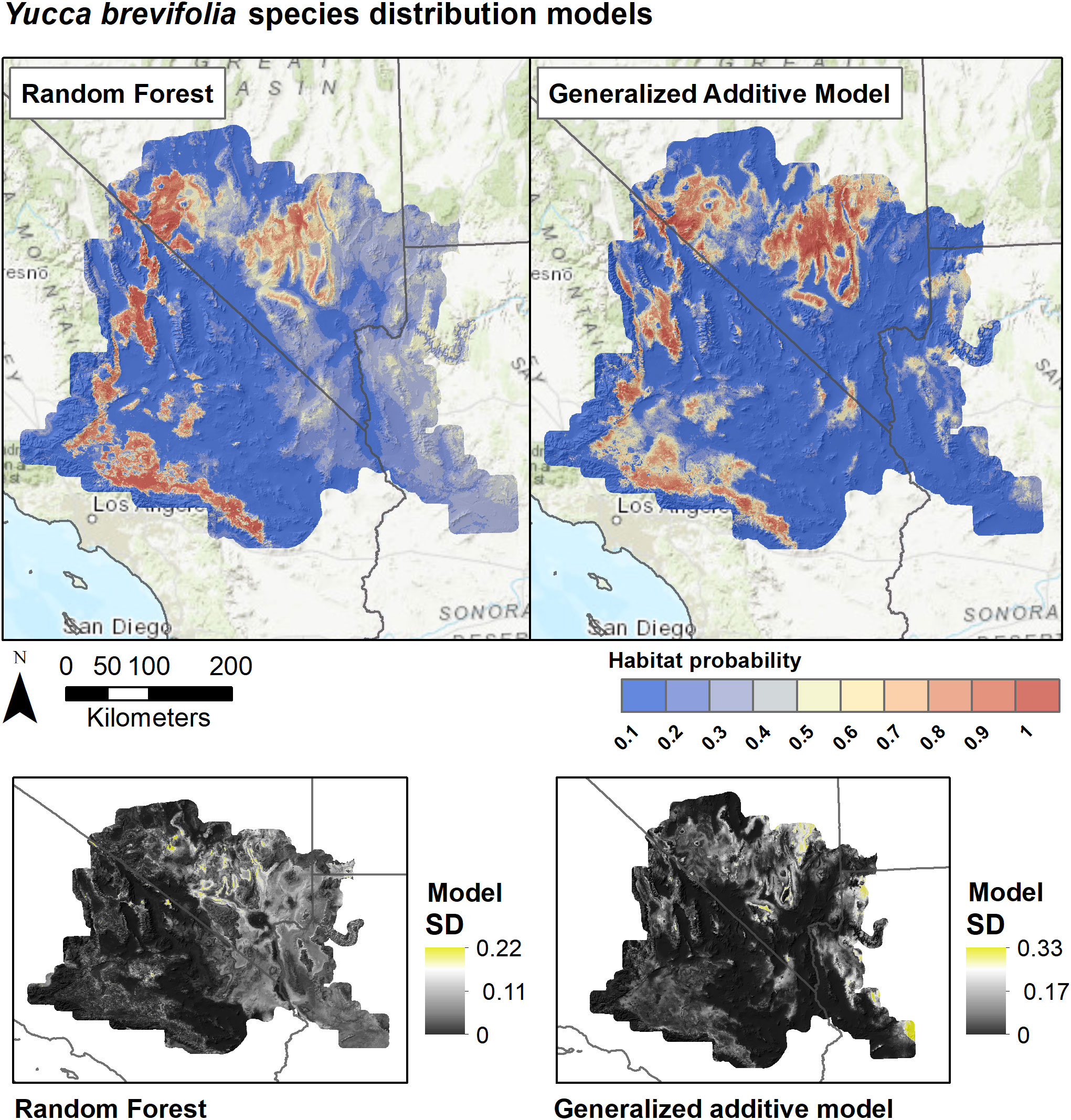
Figure 6 Species distribution models for Y. brevifolia based on Random Forests and Generalized Additive Models. SDMs for each algorithm are derived as the weighted average of predictions from eight individual candidate models based on the True Skill Statistic. Lower panels show the standard deviation of predictions among the candidate models for each algorithm.
3.5 Model selection
Climate variables were typically more important predictors in the SDMs than the vegetation or topography variables for each species. For Y. jaegeriana, the GAM model with the best (lowest) AIC was model 3, while for Y. brevifolia, the GAM with the lowest AIC was model 7 (Supplementary Table S3), and models 3 and 7 were the top two models for both Joshua tree species on this metric (Table S3). The environmental variables for these models only differed by including the climate moisture deficit (CMD for model 3), and summer maximum temperature (STMX for model 7). Despite the large differences in AIC, GAM models were largely consistent in predictive performance based on other metrics, with AUC ranging from 0.796 to 0.798 for Y. jaegeriana, and from 0.852 to 0.859 for Y. brevifolia (Table S3) among the eight models. No single GAM model scored highest across all performance metrics.
Among RF models, we observed less variation in predictive performance across models than for GAM (Table S3). For Y. jaegeriana, models 2 and 1 had the highest AUC scores, while models 2 and 5 had the highest TSS scores (Table S3). Both models 1 and 2 contained a measure of annual aridity (AHM); similarly, model 5 reflected annual precipitation and temperature variables (MAP, MAT) but not their seasonal components. However, the models varied in AUC by only 0.004 (0.859 versus 0.855). For Y. brevifolia, models 4 and 3 had the highest AUC and TSS scores respectively (Table S3). These models only differed by including either seasonal precipitation (SP, WP, Model 3) or annual precipitation (MAP, Model 4). However, as with Y. jaegeriana, AUC differed by only 0.004 between the highest and lowest performing RF models for this species (0.878 versus 0.874), suggesting similar predictive performance. The rangewide, multi-species RF models also showed consistently high predictive performance, with Models 7 and 8 showing the highest AUC scores (Table S3). These models both contained seasonal precipitation variables but varied in representing seasonal (Model 7) vs. annual (Model 8) temperature averages.
3.6 Environmental variables predicting modeled habitats in SDMs
For Y. jaegeriana, AHM and MAP were the strongest predictors of Joshua tree habitat averaged across algorithms, followed by SP, MAT, Pratio, PCV, and TSD (Table 4). For Y. brevifolia, MAT was the strongest predictor averaged across GAM and RF followed by TSD, PCV, SP, AHM, WTMN, and Pratio (Table 4). The best eight environmental predictors averaged between RF and GAM differed in rank but were largely shared between the two species, differing only with respect to STMX (for Y. jaegeriana) and MAP (for Y. brevifolia). For the rangewide RF model, AHM was the strongest predictor, followed by MAP, MAT, SP, WP, STMX, Pratio, PCV, TSD, and WTMN (Table 4).
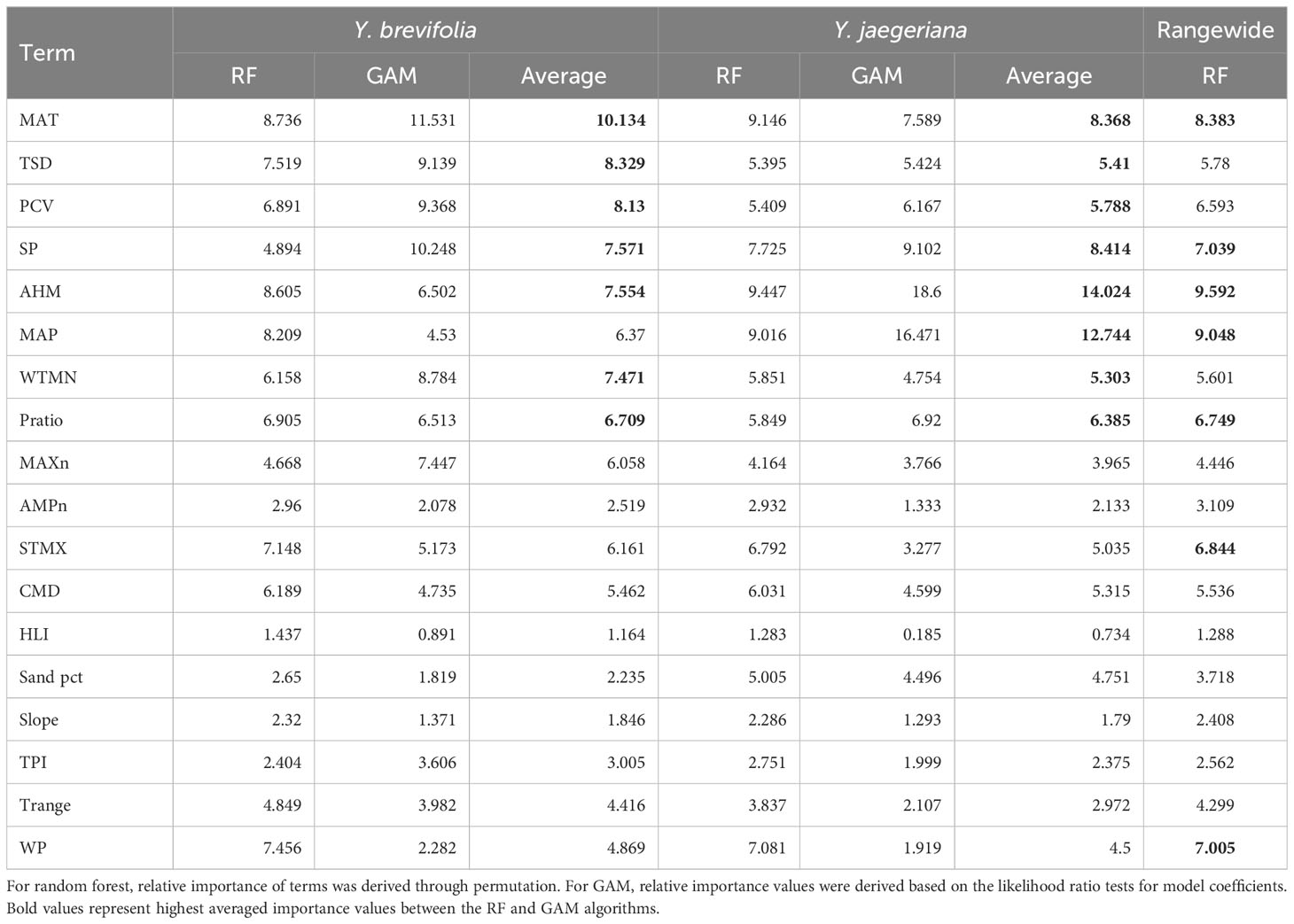
Table 4 Relative importance of environmental covariates in species distribution models for each algorithm and species.
We used partial response curves to illustrate relationships between Joshua tree modeled habitat and individual environmental variables across gradients in the landscape. Both Y. brevifolia and Y. jaegeriana have similar peaks in probability of occurrence for the annual heat/moisture index (AHM), climatic moisture deficit (CMD), and mean annual precipitation (MAP) for the GAM and RF models (Figure 7). In contrast, Y. jaegeriana are predicted to occur at somewhat higher temperatures than Y. brevifolia for both RF and GAM algorithms (Figure 7). Summer precipitation (SP) increased habitat probabilities at higher values for Y. jaegeriana in the GAM and RF models, and this relationship was also reflected in higher probability values with increasing ratio of summer to winter precipitation (Pratio). Both the RF and GAM response curves indicated that habitat probabilities for Y. brevifolia may also increase in response to a higher ratio of summer precipitation, which occurs in Y. brevifolia habitat in the vicinity of the hybrid zone and in the most northerly parts of the species range in NV. Similarly, while Y. jaegeriana typically occurs in areas with more bimodal precipitation and hence lower precipitation seasonality (PCV), response curves suggested that higher PCV values (i.e., more winter-dominated precipitation) increased habitat probability in the GAM model for Y. jaegeriana, likely reflecting the large amount of potential habitat occurring in the western Mojave that is often congruent with Y. brevifolia habitat (Figure 5). This is also illustrated in the Fort Irwin area, where the GAM model predicted higher habitat suitability values than the RF model (see Figure 5). Higher levels of temperature variability were more influential in the SDMs for Y. jaegeriana for both RF and GAM (Figure 7). Full partial response curves from GAM and RF models for each species are available in Supplementary Figures S2–S6.
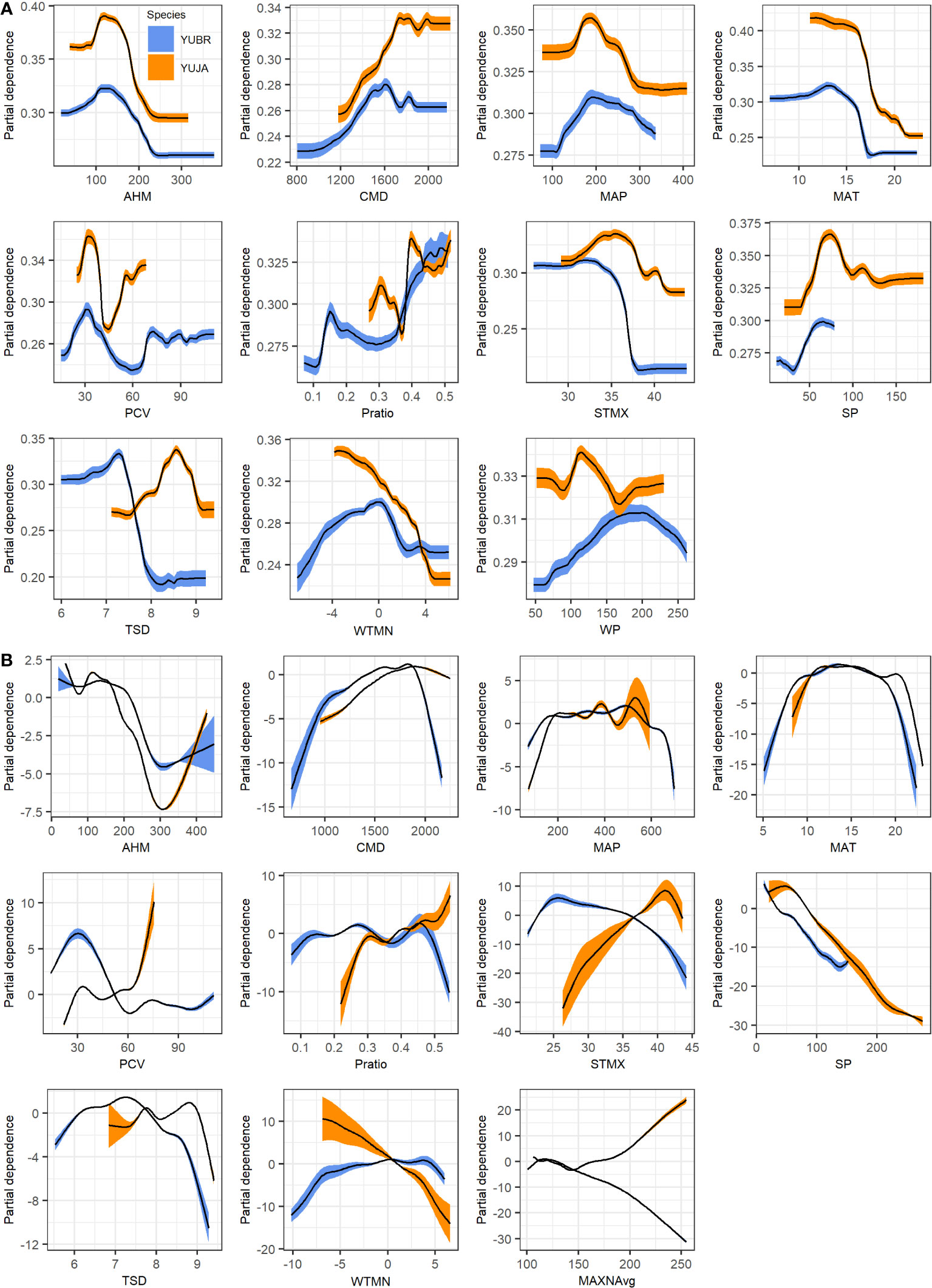
Figure 7 Partial dependence plots from (A) Random Forest and (B) GAM species distribution models for Y. brevifolia (blue curves) and Y. jaegeriana (orange curves). Curves show the marginal influence of each term on the predicted probability of habitat. 95% confidence intervals (colored bands) are derived from cross-validation.
3.7 Comparisons of modeled and potential habitat
Niche similarity statistics used on SDMs suggested considerable overlap between the two species, with D = 0.629 and I = 0.882 for the multi-algorithm ensemble predictions. However, AUC comparisons suggested that the niche similarity was asymmetric: while the Y. jaegeriana ensemble SDM predicted Y. brevifolia presences with an AUC = 0.764, the Y. brevifolia ensemble SDM predicted Y. jaegeriana presences with an AUC = 0.667. We note that SDMs extrapolated beyond the known range of a species are prone to uncertainty: we projected each species SDM into the range of the other species to illustrate where the habitat may be similar, but this does not imply occupancy of both species in these areas, and these observations are corroborated by the habitat mapping reported here.
Spatial comparisons of the ensemble SDMs for each species further illustrate that potential habitat (modeled habitat where presences were not detected in surveys) for Y. jaegeriana extends farther into the habitat of Y. brevifolia than vice versa (Figure 8). In fact, potential habitat for Y. jaegeriana extends throughout the entire range of Y. brevifolia, while potential habitat of Y. brevifolia only occurs in small patches beyond its extant range (Figures 8B, C), in addition to the area of obscured imagery where both species share potential habitat. Overall, potential habitat derived from the ensemble SDM for Y. brevifolia covers approximately 16,400 km2 and is of similar size to the area of occupied habitat (Figure 8B). However, potential habitat had a median probability value of 0.624, lower than the median of 0.726 for grid cells in occupied habitat (e.g., those overlapping with surveyed presences). Potential habitat for Y. brevifolia also overlaps with approximately 1,834 km2 of occupied Y. jaegariana habitat. Potential habitat for Y. jaegeriana covers approximately 27,638 km2 and is substantially larger than the occupied habitat for this species (Figure 8C). As observed for Y. brevifolia, potential habitat for Y. jaegeriana may be of lower current suitability, with a median habitat probability of 0.61 versus a median of 0.73 for occupied habitat. Approximately 5,410 km2 of Y. jaegeriana’s potential habitat is currently occupied by Y. brevifolia, mirroring the asymmetric niche similarity measures noted above. Overall, the two species share approximately 9,669 km2 of potential habitat that is not currently occupied by either species. Much of this area of shared potential habitat falls within the area of obscured imagery (Figure 8); hence, a caveat to the calculations presented here is that the amount of occupied habitat in the obscured imagery area is unknown.
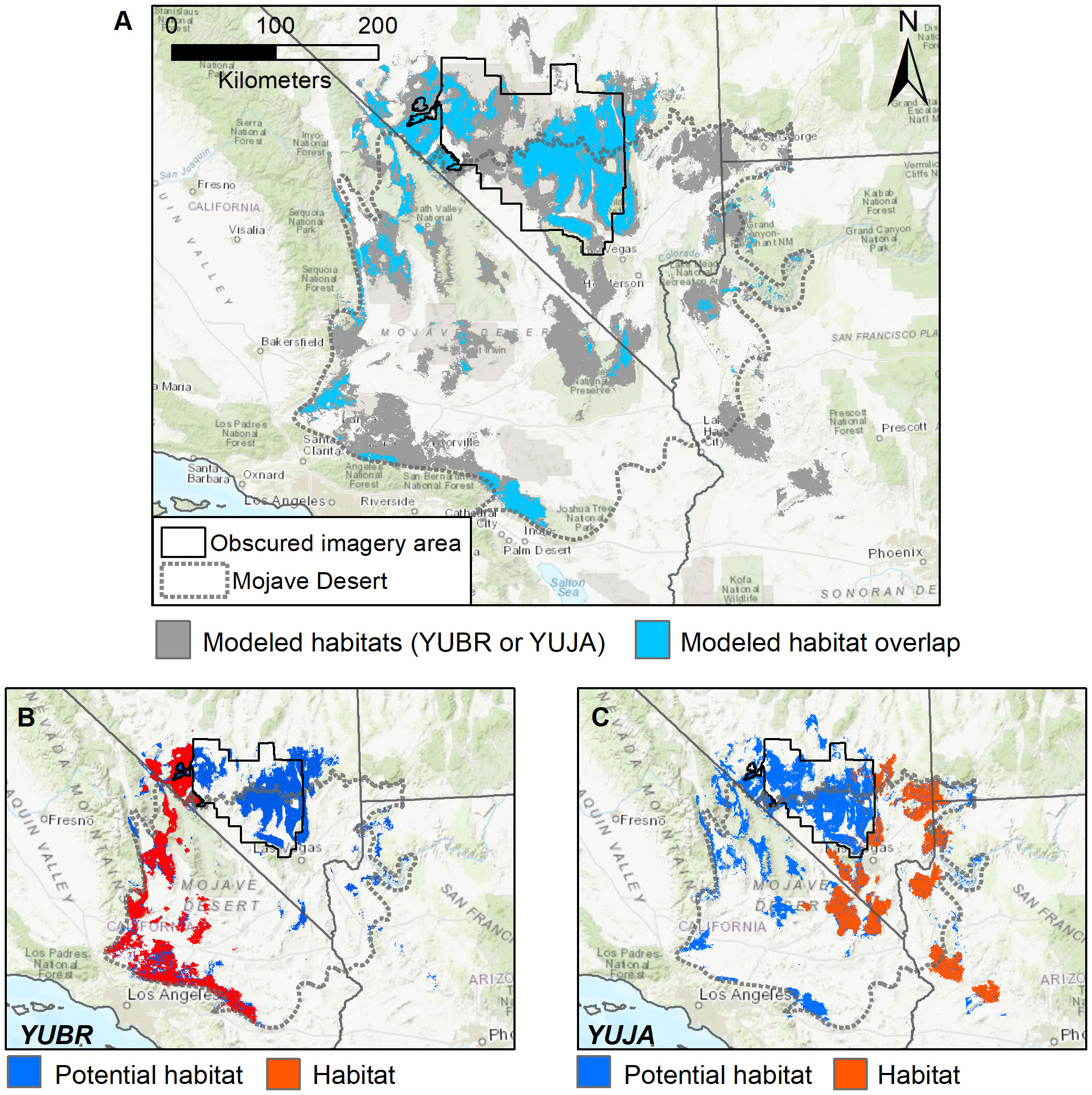
Figure 8 (A–C) Overlap in modeled habitat predicted by overlaying separate SDMs for both Joshua tree species (Y. brevifolia and Y. jaegeriana). (A) Modeled habitat overlap (blue) was determined by applying a threshold to the individual species SDMs, and then overlaying these raster layers to determine where the modeled habitat for each species overlaps. While these maps reflect areas of similar habitat characteristics, they do not imply occupancy of both Joshua tree species in areas of joint modeled habitat. (B, C) Comparison of occupied versus potential habitat for each species. Here, habitat indicates cells with an observed presence from satellite and/or ground surveys, whereas potential habitat indicates cells with modeled habitat that were not underlain by a surveyed presence.
Potential habitat predicted for both species tended to occur at higher elevations than currently occupied habitat, suggesting that SDMs did not typically extrapolate into warmer areas. For example, potential habitat for Y. brevifolia was at significantly higher elevation than occupied habitat (mean of 1459 m versus 1325 m, respectively; t = 71.07, P < 0.0001), received more annual precipitation (MAP of 206 mm versus 189 mm; t = 57.52, P < 0,0001), and had lower annual temperatures (MAT of 13.7°C versus 14.4°C; t = 61.62; P < 0.001). Similarly, potential habitat for Y. jaegeriana was higher in elevation (average of 1408 m versus 1078 m; t = 230.99, P < 0.0001) and cooler (MAT of 14.1°C versus 17.2°C; t = −295, P < 0.0001) than occupied habitat. However, precipitation was lower in Y. jaegeriana’s potential habitat (MAP of 190 mm versus 210 mm in occupied habitat; t = −86.52, P < 0.0001), reflecting a westward shift into areas receiving less summer precipitation that are more typical of Y. brevifolia habitat.
While higher elevation and lower temperatures in potential habitat suggest these areas could serve as refugia as the climate warms, accessibility to current populations could be a major constraint. For Y. jaegeriana, potential habitat that is contiguous with actual habitat is rarely more than a few kilometers deep around Y. jaegeriana stands (Figure 8). Outlying patches and diffuse cells of potential habitat are likely inaccessible for colonization under present climatic conditions because the unoccupied intervening areas do not support Joshua trees and Joshua trees seem to be dispersal limited (Vander Wall et al., 2006). While the pattern is similar for most of Y. brevifolia’s range, there are some large potential habitat patches in the southwest of the range that are largely contiguous with occupied habitat.
4 Discussion
4.1 Yucca spp. distributions
Our species distribution models are based on the most comprehensive presence and absence data yet available for Joshua trees, enabling us to evaluate the environmental variables underlying each species’ distribution. Thus, we present the first highly accurate and nearly complete empirically derived range maps for Yucca brevifolia and Y. jaegeriana. By coupling Google Earth imagery to detect adult Joshua trees with extensive field validation, we identified presences on 0.25 km2 grids across the ranges of both species. While presence data were lacking for one area of obscured imagery where national defense is a priority for the Department of Energy and the Department of Defense, we used our high-resolution presence and absence data to fit SDMs across the full range, resulting in completed rangewide distribution maps.
Identifying large, multi-stem Joshua trees using remotely sensed Google Earth data is relatively straightforward, especially in the absence of other large non-target succulent species that are similar in physiognomy. However, Joshua trees can be challenging to distinguish remotely from other large species including Mojave yucca (Y. schidigera Roezl ex Ortgies), soaptree yucca (Y. elata Engelm.), banana yucca (Y. baccata Torr.), giant saguaro cactus (Carnegiea gigantea (Engelm.) Britton & Rose), desert almond (Prunus fasciculata (Torr.) A. Gray); sugar sumac (Rhus ovata S. Watson), creosote bush (Larrea tridentata Coville), or trees such as pinyon pines (e.g., Pinus monophyla Torr. & Frém.) and junipers (e.g., Juniperus osteosperma (Torr.) Little). Clonal Joshua tree forms can also be difficult to observe remotely where they are prevalent, e.g., on the western margin of Y. brevifolia distributions. Areas scarred by wildfires can have exceptionally low densities of Joshua trees, which also makes them difficult to detect. Joshua trees that were within natural distributions, but recognizably in cultivation (i.e., planted specimens within fences, urban settings) were not included as presences. Despite these difficulties, extensive field validations of imagery-based presence and absence classifications indicated the method to be highly effective, with 87% of over 29,000 field surveyed grid cells requiring no change (Table S2). Secondary imagery-based validations of over 76,000 grid cells by experienced observers further refined our presence and absence data, resulting in reclassifications of 5.5% of grid cells and likely increased accuracy in the most challenging areas of imagery (Table S2).
Yucca jaegeriana habitat occurs in discrete well-defined and homogeneously occupied populations of the eastern Mojave Desert and neighboring ecoregions, while Y. brevifolia populations have a sprawling distribution of considerably less homogeneous patches along the western boundary of the Mojave Desert. Furthermore, empirical demographic measurements at the leading edges of Joshua tree distributions indicate that small founder trees occurring there extend less than a kilometer from the edges of established Joshua tree stands. This is consistent with observations during demographic transect surveys, in which young Joshua trees are well represented within some Joshua tree stands and taper off within a few 100 m near the stand edges (Smith, et al., unpublished data). These distributional patterns are generally consistent with previous Joshua tree range maps (Rowlands, 1978; Godsoe et al., 2009; Cole et al., 2011; Smith et al., 2011; Wilkening et al., 2020); however, the habitat we defined has 23.8% less areal coverage than the distribution identified in the most recently published distribution map (see Supplementary Table S4; Wilkening et al., 2020). Within populations we found absences of Y. jaegeriana only in small, widely scattered locations; in contrast, Y. brevifolia populations were more diffuse owing to a greater number of absences dispersed throughout the populations, especially in the southwest of the range. Based on satellite imagery some of those absences within Y. brevifolia populations appear to be the result from urban development, fire, and other cumulative disturbances.
The upper and lower elevational, latitudinal, and longitudinal limits were revised for both species where appropriate (see Figure 2). Differences we noted from previous work mostly represent the higher resolution of imagery now available and the benefits of widespread ground searches, rather than recent changes in species range limits. The northern limit for Y. jaegeriana range was increased by a few kilometers but the eastern limit that we interpreted from Rowlands (1978) was reduced by a few kilometers (P. Rowlands – pers. comm.), while the southern and western limits remain unchanged (Rowlands, 1978). The northern limit of Y. brevifolia was increased by 35 km, and the western limit was not previously well-defined, and misrepresented by a herbarium record with an erroneous locality, but is currently at the junction of Orwin Way road and the Quail Canyon Motocross road in Los Angeles, Co., CA. The eastern limit remains in Tikaboo Valley, NV (verified genetically near the hybrid zone – Starr et al., 2013) and the southern limit also remains the same as identified by Rowlands (1978).
4.2 Environmental variables and Yucca spp.
Applying SDMs to our Joshua tree presence and absence data facilitates understanding the ecological correlates of Joshua tree distributions. Clarifying the roles of abiotic and biotic ecological factors are key to understanding species distributions (Lexer and Fay, 2005), and how Joshua trees may interact with future disturbances such as climate change. In this study, climate variables were the most important environmental correlates of Joshua tree distributions compared with remotely sensed or topographic variables; however, we did not include some important biotic variables in our analyses such as pollinator biology or intraspecific genomic variation also important to Joshua tree distributional patterns (Godsoe et al., 2008; Smith et al., 2008; Royer et al., 2016; Royer et al., 2020).
Several variables highlight differences between Y. jaegeriana and Y. brevifolia in the climatographs generated from survey-based presence data, and functional response curves generated from SDMs. Variables with the greatest contrast between species were the precipitation coefficient of variation, the precipitation ratio, and temperature standard deviation (Figure 3). Climatographs showed that the overall frequency distributions of both species were similar for mean annual precipitation and winter precipitation (Figure 3), however, only Y. jaegeriana occupies regions receiving regular summer precipitation. This influence is also correlated with the distribution of the species in relation to the precipitation coefficient of variation. Yucca jaegeriana also experiences more variable temperature patterns than Y. brevifolia (Figure 3). For example, mean annual temperature had a similar amplitude between species but was clearly skewed toward higher temperatures for Y. jaegeriana and lower temperatures for Y. brevifolia which is similar to functional response curves for SDMs (Figure 7). The standard deviation for monthly mean temperatures (TSD) was also higher for Y. jaegeriana, indicating the species experiences both warmer and more variable temperatures.
Higher temperatures experienced by Y. jaegeriana are ameliorated by greater summer precipitation (SP), such that the overall aridity profile (e.g., AHM, Figure 3) is similar between species. Patterns in seasonality and amount of precipitation experienced by Y. jaegeriana contrast with those of Y. brevifolia, particularly for the most easterly populations. This contrast is the result of a regional precipitation pattern of greater winter precipitation in the west, a more even precipitation pattern central to the range and near the hybrid zone, and bi-modal precipitation in the east (Hereford et al., 2006). Central and northern populations of Y. jaegeriana share a more similar climate with Y. brevifolia populations, and SDMs suggest that Y. jaegeriana species could extend further into the western Mojave absent limits on pollination, dispersal and other unaccounted factors. However, the Mojave Desert and surrounding regions are predicted to experience rapidly changing climate over the next several decades (Dai, 2013), and local adaptation within the range of each species could lead to differences in the population’s response to future changes in climate, particularly if climate change alters the seasonality of precipitation. Recruitment of Joshua tree populations follows narrow seasonal precipitation and temperature cues (Reynolds et al., 2012). Like other large and long-lived desert plants (Steenbergh and Lowe, 1969; Steenbergh and Lowe, 1977; Jordan and Nobel, 1979), seedling and juvenile Joshua trees are more vulnerable to drought-related mortality than adults (Esque et al., 2015). Rapid shifts in the climate regime could limit a population’s ability to recruit while adult Joshua trees continue to survive in undisturbed habitat, leading to lag effects (Svenning and Sandel, 2013) that may not be detected using the methods presented here. Lags in population change for long-lived plants are already observed among the large tree-like quiver tree (Aloidendron dichotomum) of South Africa and Namibia (Foden et al., 2007; Brodie et al., 2021).
4.3 Comparison of habitat, modeled habitat, and potential habitat between species
Although the sister species of Joshua tree currently exist in allopatry, except for the narrow hybrid zone (Godsoe et al., 2009) – a pattern not uncommon to many sister species (Barton and Hewitt, 1985) – our SDM projections of potential habitat suggest this pattern may reflect ecological relationships other than habitat suitability, as previously hypothesized (Godsoe et al., 2009). Potential habitat for Y. brevifolia is closely associated with occupied habitat and does not extend far into the range of Y. jaegeriana east of the hybrid zone with a few scattered exceptions (Figure 8). Conversely, for Y. jaegeriana west of the hybrid zone (where this species does not currently exist), extensive potential habitat is predicted and closely mimics the habitat of Y. brevifolia with few exceptions. Considering this pattern, as well as the tendency for introgression of Y. jaegeriana genetic material westward into Y. brevifolia populations (Starr et al., 2013; Royer et al., 2020), one wonders why Y. jaegeriana is not the dominant species in a front moving westward across the Mojave Desert. We suggest this may be largely dependent on biological factors that we did not account for in this analysis. Such factors include but are not limited to pollination interference by mis-matched pollinators, lack of vigor in hybrids for a variety of reasons, low capability of pollinator dispersal, reproductive isolation, and low seed dispersal distances (Vander Wall et al., 2006; Smith et al., 2008; Godsoe et al., 2009; Waitman et al., 2012; Royer et al., 2016; Royer et al., 2020). As Godsoe et al. (2009) previously suggested, the natural experiment between Joshua tree species in the hybrid zone is not sufficient to understand all the dynamics driving the patterns observed there. Further experimentation using known matrilines in combination with growth chambers and common gardens to tease out differences in species performance by genetics, physiology, and phenology would be a good start toward unraveling these patterns.
5 Conclusions and future directions
One of the most compelling questions toward the conservation of Y. brevifolia and Y. jaegeriana, i.e., how they will respond to current and future climate change, is the topic of a second manuscript. This question is related to a host of ecological questions about species distributions and climate change, and the work presented here lends itself directly to answering such questions. Joshua trees may be more challenging than most in regard to climate change, because of the symbiotic relationship with their obligate pollinators (Smith et al., 2008; Starr et al., 2013). Thus, we may well understand the relationships with climate variability for the adult Joshua trees, but their responses to climate change may be influenced by how yucca moths (genus Tegeticula) will respond and interact with Joshua trees and changing climate
While the overall presence and absence data sets for Joshua trees were very robust, the area of obscured imagery in the north-central portion of the study area introduces unwanted variability and increased error for that location (Figures 5, 6). Acquiring this information would aid our understanding of the distributions of each species for management. Such an endeavor would be greatly enhanced by the acquisition of genetic samples in the same area, which is partially adjacent to the hybrid zone between the species.
A caveat to our approach of mapping Joshua tree habitats is that recent recruits to Joshua tree populations (perhaps the past 30 years – Esque et al., 2015) are mostly not visible with remote sensing because these small Joshua trees are closely tied to nurse plants which benefit the juvenile Joshua trees through crypsis from herbivores (Esque et al., 2015). Juvenile Joshua trees are typically hidden by the canopy of associated nurse plants, and rarely survive in the absence of these larger hosts (Brittingham and Walker, 2000; Esque et al., 2015). Not being able to detect recruitment for decades from remotely sensed data is a drawback of this method, because the lack of recruitment detections confounds our understanding of recent Joshua tree recruitment which is essential for understanding their population status. It will be necessary to establish demographic plots that are searched on-the-ground for juvenile Joshua trees if we are to fully understand population recruitment in coming decades. Furthermore, conditions for recruitment of Joshua trees are very specific and were not accounted for in these models (Reynolds et al., 2012). Future modelling work will undoubtedly include recruitment conditions (Diamond, 2018). However, Artificial Intellligence technicques advance rapidly; perhaps with higher resolution imagery and other technological advances mapping juvenile Joshua trees will be possible. Such advances would allow greater ability to forecast how populations will respond to variable climates in terms of distributional shifts and recruitment.
Data availability statement
The raw data supporting the conclusions of this article will be made available by the authors, without undue reservation. Requests to access the datasets should be directed to dGVzcXVlQHVzZ3MuZ292.
Author contributions
TE: Conceptualization, Data curation, Funding acquisition, Investigation, Methodology, Project administration, Supervision, Validation, Writing – original draft, Writing – review & editing. DS: Conceptualization, Formal analysis, Investigation, Methodology, Writing – original draft, Writing – review & editing. GB: Data curation, Formal analysis, Investigation, Methodology, Supervision, Validation, Writing – review & editing. FC: Conceptualization, Data curation, Formal analysis, Investigation, Methodology, Project administration, Supervision, Validation, Writing – review & editing. LD: Conceptualization, Formal analysis, Methodology, Writing – original draft, Writing – review & editing. SL: Data curation, Investigation, Methodology, Writing – review & editing. BC: Data curation, Investigation, Validation, Writing – review & editing. EG: Data curation, Investigation, Validation, Writing – review & editing. CP: Data curation, Investigation, Validation, Writing – review & editing. GG: Data curation, Investigation, Validation, Writing – review & editing. RG: Data curation, Investigation, Validation, Writing – review & editing. BG: Data curation, Investigation, Validation, Writing – review & editing. AM: Data curation, Investigation, Validation, Writing – review & editing. JY: Conceptualization, Funding acquisition, Methodology, Writing – review & editing. CS: Conceptualization, Data curation, Funding acquisition, Methodology, Writing – review & editing. KN: Conceptualization, Methodology, Writing – review & editing.
Funding
The author(s) declare financial support was received for the research, authorship, and/or publication of this article. US Fish and Wildlife Service (contact #4500139195) and US Geological Survey for providing funding to accomplish this work. During the development of this paper the authors received support from the National Science Foundation (Award #2001190 to TCE, LAD, CIS, and #201180 to JY).
Acknowledgments
P.G. Rowlands generously shared his early recollections of Joshua tree distributional interpretations to clarify our records. K. Heyduk provided helpful discussion and comments on the manuscript. US Department of the Army, Ft Irwin National Training Center provided LiDar data used to confirm presences and absences of Joshua trees. Nellis Air Force Base provided unpublished presence data from their base. W. Hodgson provided helpful discussion about range limits of Yucca jaegeriana. We thank B. Smith, C. Silva, E. Ceplecha, J. Swart, K. Forgrave, K. Davison, S. Dahl, S. Murray, and S. Thomson for their help in completing the primary surveys. As well as P. Baird, G. Olson, A. Rohr, B. Carlile, G. Lawson, J. Samuelson, J. Christie, K. Cantrell, K. Herbinson, M. Specht, M. Carlile, R. Miller, S. Zelen, and Z. Young for their additional help. Any use of trade, product, or firm names is for descriptive purposes only and does not imply endorsement by the US Government.
Conflict of interest
The authors declare that the research was conducted in the absence of any commercial or financial relationships that could be construed as a potential conflict of interest.
The reviewer CB declared a past co-authorship with the author(s) CS and JY to the handling editor.
Publisher’s note
All claims expressed in this article are solely those of the authors and do not necessarily represent those of their affiliated organizations, or those of the publisher, the editors and the reviewers. Any product that may be evaluated in this article, or claim that may be made by its manufacturer, is not guaranteed or endorsed by the publisher.
Supplementary material
The Supplementary Material for this article can be found online at: https://www.frontiersin.org/articles/10.3389/fevo.2023.1266892/full#supplementary-material
References
Allouche O., Tsoar A., Kadmon R. (2006). Assessing the accuracy of species distribution models: Prevalence, kappa and the true skill statistic (TSS). J. Appl. Ecol. 43, 1223–1232. doi: 10.1111/j.1365-2664.2006.01214.x
Bahn V., McGill B. J. (2012). Testing the predictive performance of distribution models. Oikos 122, 321–331. doi: 10.1111/j.1600-0706.2012.00299.x
Barbet-Massin M., Jiguet F., Albert C. H., Thuiller W. (2012). Selecting pseudo-absences for species distribution models: how, where and how many? Methods Ecol. Evol. 3, 327–338. doi: 10.1111/j.2041-210X.2011.00172.x
Barrows C. W., Murphy-Mariscal M. L. (2012). Modeling impacts of climate change on Joshua trees at their southern boundary—How scale impacts predictions. Biol. Conserv. 152, 29–36. doi: 10.1016/j.biocon.2012.03.028
Barton N. H., Hewitt G. M. (1985). Analysis of hybrid zones. Annu. Rev. Ecol. Systematics 16, 113–148. doi: 10.1146/annurev.es.16.110185.000553
Bean W. T., Stafford R., Brashares J. S. (2012). The effects of small sample size and sample bias on threshold selection and accuracy assessment of species distribution models. Ecography 35, 250–258. doi: 10.1111/j.1600-0587.2011.06545.x
Brittingham S., Walker L. R. (2000). Facilitation of Yucca brevifolia recruitment by Mojave Desert shrubs. Western North Am. Nat. 60, 374–383.
Brodie L. P., Grey K.-A., Bishop J. M., Midgley G. F. (2021). Broadening predictive understanding of species’ range responses to climate change: The case of Aloidendron dichotomum. Front. Ecol. Evol. 9. doi: 10.3389/fevo.2021.715702
Brown C. H., Griscom H. P. (2022). Differentiating between distribution and suitable habitat in ecological niche models: A red spruce (Picea rubens) case study. Ecol. Modeling 472, 110102. doi: 10.1016/j.ecolmodel.2022.110102
California Fish and Game Commission. (2019). Public notice of receipt of petition to list the western Joshua tree (Yucca brevifolia) as an Endangered species under state law. Available at: nrm.dfg.ca.gov/FileHandler.ashx?DocumentID=175217&inline.
Cole K. L., Ironside K., Eischeid J., Garfin G., Duffy P. G., Toney C. (2011). Past and ongoing shifts in Joshua tree distribution support future modeled range contraction. Ecol. Appl. 21, 137–149. doi: 10.1890/09-1800.1
Dai A. (2013). Increasing drought under global warming in observations and models. Nat. Climate Change 3 (1), 52–58. doi: 10.1038/nclimate1633
Daly C., Halbleib M., Smith J. I., Gibson W. P., Doggett M. K., Taylor G. H., et al. (2008). Physiographically sensitive mapping of climatological temperature and precipitation across the conterminous United States. Int. J. Climatology 28, 2031–2064. doi: 10.1002/joc.1688
DeFalco L. A., Esque T. C., Scoles-Sciulla S. J., Rodgers J. (2010). Desert wildfire and severe drought diminish survivorship of the long-lived Joshua tree (Yucca brevifolia; Agavaceae). Am. J. Bot. 97, 243–350. doi: 10.3732/ajb.0900032
Diamond S. E. (2018). Contemporary climate-driven range shifts: Putting evolution back on the table. Functional Ecology 32, 1652–1665. doi: 10.1111/1365-2435.13095
Dobrowski S. Z., Abatzoglou J., Swanson A. K., Greenberg J. A., Mynsberge A. R., Holden Z. A., et al. (2013). The climate velocity of the contiguous United States during the 20th century. Global Change Biol. 19, 241–251. doi: 10.1111/gcb.12026
Dole K. P., Loik M. E., Sloan L. C. (2003). The relative importance of climate change and the physiological effects of CO2 on freezing tolerance for the future distribution of Yucca brevifolia. Global Planetary Change 36, 137–146. doi: 10.1016/S0921-8181(02)00179-0
Elith J., Leathwick J. R. (2009). Species distribution models: Ecological explanation and prediction across space and time. Annu. Rev. Ecology Evolution Systematics 40, 677–697. doi: 10.1146/annurev.ecolsys.110308.120159
Esque T. C., Baird P. E., Chen F. C., Housman D., Holton J. T. (2020a). Using remotely sensed data to map Joshua Tree distributions at Naval Air Weapons Station China Lake, California 2018: U.S. Geological Survey Sci. Investigations Rep., 2020–5053. doi: 10.3133/sir20205053
Esque T. C., DeFalco L. A., Hodgson W., Salywon A., Puente R., Clary K. (2020b). Yucca brevifolia. IUCN Red List Threatened Species 2020, e.T117423077A117469962. doi: 10.2305/IUCN.UK.2020-3.RLTS.T117423077A117469962.en
Esque T. C., DeFalco L. A., Hodgson W., Salywon A., Puente R., Clary K. (2020c). Yucca jaegeriana. IUCN Red List Threatened Species 2020, e.T162386466A162386497. doi: 10.2305/IUCN.UK.2020-3.RLTS.T162386466A162386497.en
Esque T. C., DeFalco L. A., Tyree G. L., Drake K. K., Nussear K. E., Wilson J. S. (2021). Priority species lists to restore desert tortoise and pollinator habitats in Mojave Desert shrublands. Natural Areas J. 41, 145–158. doi: 10.3375/043.041.0209
Esque T. C., Medica P. A., Shryock D. F., DeFalco L. A., Webb R. H., Hunter R. B. (2015). Direct and indirect effects of environmental variability on growth and survivorship of pre-reproductive Joshua trees, Yucca brevifolia Engelm. (Agavaceae). Am. J. Bot. 102, 85–91. doi: 10.3732/ajb.1400257
Fielding A. H., Bell J. F. (1997). A review of methods for the assessment of prediction errors in conservation presence/absence models. Environ. Conserv. 24, 38–49. doi: 10.1017/S0376892997000088
Foden W., Midgley G. F., Hughes G., Bond W. J., Thuiller W., Hoffman M. T., et al. (2007). A changing climate is eroding the geographical range of the Namib Desert tree Aloe through population declines and dispersal lags. Diversity Distributions 13, 645–653. doi: 10.1111/j.1472-4642.2007.00391.x
Fourcade Y., Engler J. O., Rödder D., Secondi J. (2014). Mapping species distributions with MAXENT using a geographically biased sample of presence data: A performance assessment of methods for correcting sampling bias. PloS One 9 (5), e97122. doi: 10.1371/journal.pone.0097122
Franklin J. (1995). Predictive vegetation mapping: geographic modeling of biospatial patterns in relation to environmental gradients. Prog. Phys. Geogr. 19, 474–499. doi: 10.1177/030913339501900403
Franklin J. (2009). Mapping species distributions: spatial inference and prediction (Cambridge University Press, Cambridge, UK).
Godsoe W., Strand E., Smith C. I., Yoder J. B., Esque T. C., Pellmyr O. (2009). Divergence in an obligate mutualism is not explained by divergent climate factors. New Phytol. 183, 589–599. doi: 10.1111/j.1469-8137.2009.02942.x
Godsoe W., Yoder J. B., Smith C. I., Pellmyr O. (2008). Coevolution and divergence in the Joshua tree/yucca moth mutualism. Am. Nat. 171, 816–823. doi: 10.1086/587757
Greenwell B. M. (2017). Pdp: an R package for constructing partial dependence plots. R J. 9, 421–436. doi: 10.32614/RJ-2017-016
Guisan A., Thuiller W. (2005). Predicting species distribution—Offering more than simple habitat models. Ecol. Lett. 8, 993–1009. doi: 10.1111/j.1461-0248.2005.00792.x
Hereford R., Webb R. H., Longpré C. I. (2006). Precipitation history and ecosystem response to multidecadal precipitation variability in the Mojave Desert region 1893–2001. J. Arid Environments 67, 13–34. doi: 10.1016/j.jaridenv.2006.09.019
Hirzel A. H., Le Lay G., Helfer V., Randin C., Guisan A. (2006). Evaluating the ability of habitat suitability models to predict species presences. Ecol. Model. 199, 142–152. doi: 10.1016/j.ecolmodel.2006.05.017
Hu T., Sun X., Su Y., Guan H., Sun Q., Kelly M., et al. (2021). Development and performance evaluation of a very low-cost UAV-lidar system for forestry applications. Remote Sens. 13, 77. doi: 10.3390/rs13010077
Isaac N. J. B., Jarzyna M. A., Keil P., Dambly L. I., Boersch-Supan P. H., Browning E., et al. (2020). Data integration for large-scale models of species distributions. Trends Ecol. Evol. 35, 56–67. doi: 10.1016/j.tree.2019.08.006
Jordan P. W., Nobel P. S. (1979). Infrequent establishment of seedlings of Agave deserti (Agavaceae) in the northwestern Sonoran Desert. Am. J. Bot. 66, 1079–1084. doi: 10.1002/j.1537-2197.1979.tb06325.x
Kwit C., Horvitz C. C., Platt W. J. (2004). Conserving slow-growing, long-lived tree species: Input from the demography of a rare understory conifer, Taxus floridana. Conserv. Biol. 18, 432–443. doi: 10.1111/j.1523-1739.2004.00567.x
Lenz L. W. (2007). Reassessment of Yucca brevifolia and irecognition of Y. jaegeriana as a distinct species. Aliso 24, 97–104. doi: 10.5642/aliso.20072401.07
Lexer C., Fay M. F. (2005). Adaptation to environmental stress: a rare or frequent driver of speciation? J. Evolutionary Biol. 18, 893–900. doi: 10.1111/j.1420-9101.2005.00901.x
Liu C., Berry P. M., Dawson T. P., Pearson R. G. (2005). Selecting thresholds of occurrence in the prediction of species distributions. Ecography 28 (3), 385–393. doi: 10.1111/j.0906-7590.2005.03957.x
Lobo J. M., Jiménez-Valverde A., Hortal J. (2010). The uncertain nature of absences and their importance in speciesdistribution modelling. Ecography 33, 103–114. doi: 10.1111/j.1600-0587.2009.06039
Lobo J. M., Tognelli M. F. (2011). Exploring the effects of quantity and location of pseudo-absences and sampling biases on the performance of distribution models with limited point occurrence data. J. Nat. Conserv. 19, 1–7. doi: 10.1016/j.jnc.2010.03.002
Loik M. E., St. Onge C. D., Rodgers J. (2000). Post-fire recruitment of Yucca brevifolia and Yucca schidigera in Joshua Tree National Park, California, in interface between ecology and land development in California. U.S. Geological Survey Open-File Report 00-62, 2nd. Eds. Keeley J. E., Baer-Keeley M., Fotheringham C. J.. Sacramento, CA: U.S., Geological Survey, Western Ecological Research Center
McCune B., Keon D. (2002). Equations for potential annual direct incident radiation and heat load index. J. Vegetation Science. 13, 603–606. doi: 10.1111/j.1654-1103.2002.tb02087.x
McKelvey S. (1938). Yuccas of the southwestern United States (Harvard University, Jamaican Plain, MA: Arnold Museum).
Moore I. D., Gessler P. E., Nielsen G. A., Peterson G. A. (1993). Soil attribute prediction using terrain analysis. Soil Sci. Soc. America J. 57, 443–452. doi: 10.2136/sssaj1993.03615995005700020026x
Neilson R. P., Pitelka L. F., Soloman A. M., Nathan R., Midgley G. F., Fragoso J. M. V., et al. (2005). Forecasting regional to global plant migration in response to climate change. BioScience 55, 9, 749–759. doi: 10.1641/0006-3568(2005)055[0749:FRTGPM]2.0.CO;2
Omernik J. M., Griffith G. E. (2014). Ecoregions of the conterminous United States: evolution of a hierarchical spatial framework. Environ. Manage. 54 (6), 1249–1266. doi: 10.1007/s00267-014-0364-1
Pellmyr O., Segraves K. A. (2003). Pollinator divergence within an obligate mutualism: two yucca moth species (Lepidoptera: Prodoxidae). Ann. Entomological Soc. America 96, 716–722. doi: 10.1603/0013-8746(2003)096[0716:PDWAOM]2.0.CO;2
Phillips S. J., Dudík M., Elith J., Graham C. H., Lehmann A., Leathwick J., et al. (2009). Sample selection bias and presence-only distribution models: implications for background and pseudo-absence data. Ecol. Appl. 19, 181–197. doi: 10.1890/07-2153.1
Pitelka L. F., Plant Migration Workshop Group (1997). Plant Migration and Climate Change: A more realistic portrait of plant migration is essential to predicting biological responses to global warming in a world drastically altered by human activity. Am. Scientist 85 (5), 464–473.
Poggio L., de Sousa L. M., Batjes N. H., Heuvelink G. B. M., Kempen B., Ribeiro E., et al. (2021). SoilGrids 2.0: producing soil information for the globe with quantified spatial uncertainty. SOIL 7, 217–240. doi: 10.5194/soil-7-217-2021
R Core Team. (2022). R: A language and environment for statistical computing. R Foundation for Statistical Computing, Vienna, Austria. Available at: https://www.r-project.org.
Reynolds M. B. J., DeFalco L. A., Esque T. C. (2012). Short seed longevity, variable germination conditions, and infrequent establishment events provide a narrow window for Yucca brevifolia (Agavaceae) recruitment. Am. J. Bot. 99, 1647–1654. doi: 10.3732/ajb.1200099
Rowlands P. G. (1978). The vegetation dynamics of the Joshua tree (Yucca brevifolia Engelm.) in the southwestern United States of America (Riverside, CA: Dissertation, University of California).
Royer A. M., Streisfeld M. A., Smith C. I. (2016). Population genomics of divergence within an obligate pollination mutualism: Selection maintains differences between Joshua tree species. Am. J. Bot. 103, 1730–1741. doi: 10.3732/ajb.1600069
Royer A. M., Waite-Himmelwright J., Smith C. I. (2020). Strong selection against early generation hybrids in Joshua tree hybrid zone not explained by pollinators alone. Front. Plant Sci. 11. doi: 10.3389/fpls.2020.00640
SEINet. (2023). Arizona chapter. Available at: https://swbiodiversity.org/seinet/index.php.
Smith C. I., Godsoe W. K. W., Tank S., Yoder J. B., Pellmyr O. (2008). Distinguishing coevolution from covicariance in an obligate pollination mutualism: asynchronous divergence in joshua tree and its pollinators. Evolution 62, 2676–2687. doi: 10.1111/j.1558-5646.2008.00500.x
Smith C. I., Sweet L. C., Yoder J., McKain M. R., Heyduk K., Barrows C. (2023). Dust storms ahead: Climate change, green energy development and endangered species in the Mojave Desert. Biol. Conserv. 227, 109819. doi: 10.1016/j.biocon.2022.109819
Smith C. I., Tank S., Godsoe W., Levenick J., Strand E., Esque T., et al. (2011). Comparative phylogeography of a coevolved community: Concerted population expansions in Joshua trees and four yucca moths. PloS One 6 (10), e25628. doi: 10.1371/journal.pone.0025628
Starr T. N., Gadek K. E., Yoder J. B., Flatz R., Smith C. I. (2013). Asymmetric hybridization and gene flow between Joshua trees (Agavaceae: Yucca) reflect differences in pollinator host specificity. Molular Ecolology 22, 437–449. doi: 10.1111/mec.12124
State of California (2023). Western joshua tree conservation act. A table bill to the california state legislature. SECTION 1. Chapter 11.5 (commencing with section 1927) is added to division 2 of the fish and game code.
St. Clair S. B., St. Clair E. A., St. Clair S. B. (2022). Spatio-temporal patterns of Joshua tree stand structure and regeneration following Mojave Desert wildfires. Front. Ecol. Evol. 9. doi: 10.3389/fevo.2021.667635
Steenbergh W. F., Lowe C. H. (1969). Critical factors during the first years of life of the saguaro (Cereus giganteus) at Saguaro National Monument, Arizona. Ecology 50, 825–834. doi: 10.2307/1933696
Steenbergh W. F., Lowe C. H. (1977). Ecology of the Saguaro: II. Reproduction, germination, establishment, growth, and survival of the young plant. National Park Service Scientific Monograph Series No. 8. U.S. Government Printing Office, Washington, D.C. 242 pp.
Svenning J., Sandel B. (2013). Disequilibrium vegetation dynamics under future climate change. Am. J. Bot. 100 (7), 1266–1286. doi: 10.3732/ajb.1200469
Sweet L. C., Green T., Heintz J. G. C., Frakes N., Graver N., Rangitsch J. S., et al. (2019). Congruence between future distribution models and empirical data for an iconic species at Joshua Tree National Park. Ecosphere 10 (6), e02763. doi: 10.1002/ecs2.2763
USFWS (2023). Endangered and Threatened Wildlife and Plants; Petition Finding for Joshua Trees (Yucca brevifolia and Y. jaegeriana), Federal Register 88: 50 CFR Part 17, Docket No. FWS–R8–ES–2022–0165; FF09E21000 FXES1111090FEDR 234.
Valavi R., Elith J., Lahoz-Monfort J. J., Guillera-Arroita G. (2019). blockCV: An r package for generating spatially or environmentally separated folds for k-fold cross-validation of species distribution models. Methods Ecol. Evol. 10, 225–232. doi: 10.1111/2041-210X.13107
Vander Wall S. B., Esque T. C., Waitman B. A., Haines D. F., Garnett M. G. (2006). Joshua tree (Yucca brevifolia) seeds are dispersed by seed-caching rodents. Écoscience 13, 593–543. doi: 10.2980/1195-6860(2006)13[539:JTYBSA]2.0.CO;2
VanDerWall J., Shoo L. P., Graham C., Williams S. E. (2009). Selecting pseudo-absence data for presence-only distribution modeling: How far should you stray from what you know? Ecol. Model. 220, 589–594. doi: 10.1016/j.ecolmodel.2008.11.010
Veloz S. D. (2009). Spatially autocorrelated sampling falsely inflates measures of accuracy for presence-only niche models. J. Biogeography 36, 2290–2299. doi: 10.1111/j.1365-2699.2009.02174.x
Waitman B. A., Vander Wall S. B., Esque T. C. (2012). Seed dispersal and seed fate in Joshua tree (Yucca brevifolia). J. Arid Environments 81, 1–8. doi: 10.1016/j.jaridenv.2011.12.012
Wang T., Hamann A., Spittlehouse D., Carroll C. (2016). Locally downscaled and spatially customizable climate data for historical and future periods for North America. PloS One 11 (6), e0156720. doi: 10.1371/journal.pone.0156720
Warren D. L., Glor R. E., Turelli M., Funk D. (2009). Environmental niche equivalency versus conservatism: quantitative approaches to niche evolution. Evolution 62, 2868–2883. doi: 10.1111/j.1558-5646.2008.00482.x
Wenger S. J., Olden J. D. (2012). Assessing transferability of ecological models: An underappreciated aspect of statistical validation. Methods Ecol. Evol. 3, 260–267. doi: 10.1111/j.2041-210X.2011.00170.x
WildEarth Guardians (2015). Emergency petition to list the eastern Joshua tree (Yucca jaegeriana) under the Endangered Species Act. 20.
Wilkening J. L., Hoffmann S. L., Sirchia F. (2020). Examining the past, present, and future of an iconic Mojave Desert species, the Joshua tree (Yucca brevifolia, Yucca jaegeriana). Southwestern Nat. 65, 216–229. doi: 10.1894/0038-4909-65.3-4.216
Wood S. N. (2017). Generalized additive models: An introduction with R. 2nd ed (CRC Press, Boca Raton, FL, USA).
Keywords: Joshua tree, Yucca brevifolia, Yucca jaegeriana, species distribution modeling, habitat map, remote sensing, climate variability, Mojave Desert
Citation: Esque TC, Shryock DF, Berry GA, Chen FC, DeFalco LA, Lewicki SM, Cunningham BL, Gaylord EJ, Poage CS, Gantz GE, Van Gaalen RA, Gottsacker BO, McDonald AM, Yoder JB, Smith CI and Nussear KE (2023) Unprecedented distribution data for Joshua trees (Yucca brevifolia and Y. jaegeriana) reveal contemporary climate associations of a Mojave Desert icon. Front. Ecol. Evol. 11:1266892. doi: 10.3389/fevo.2023.1266892
Received: 25 July 2023; Accepted: 16 November 2023;
Published: 14 December 2023.
Edited by:
Debra Hughson, Mojave National Preserve, United StatesReviewed by:
Cameron Barrows, University of California, Riverside, United StatesTasha La Doux, University of California, Riverside, United States
Copyright © 2023 Esque, Shryock, Berry, Chen, DeFalco, Lewicki, Cunningham, Gaylord, Poage, Gantz, Van Gaalen, Gottsacker, McDonald, Yoder, Smith and Nussear. This is an open-access article distributed under the terms of the Creative Commons Attribution License (CC BY). The use, distribution or reproduction in other forums is permitted, provided the original author(s) and the copyright owner(s) are credited and that the original publication in this journal is cited, in accordance with accepted academic practice. No use, distribution or reproduction is permitted which does not comply with these terms.
*Correspondence: Todd C. Esque, dGVzcXVlQHVzZ3MuZ292
†These authors have contributed equally to this work and share first authorship
‡These authors have contributed equally to this work
§These authors have contributed equally to this work and share last authorship