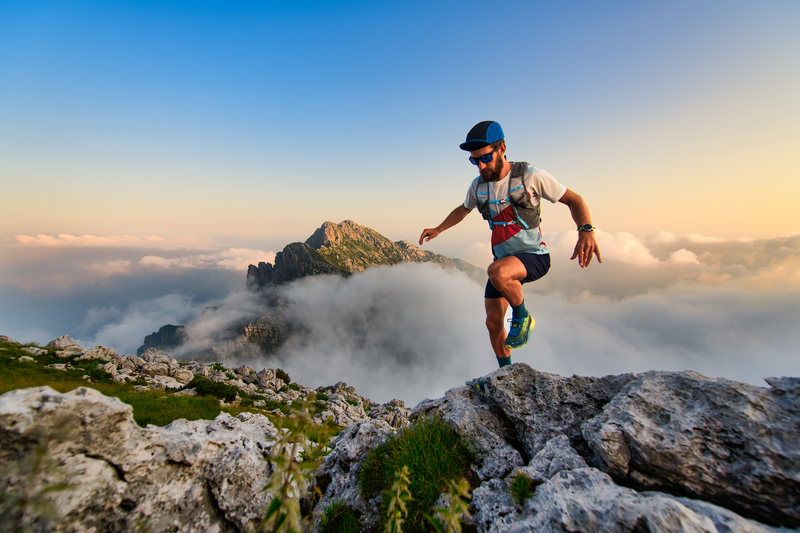
94% of researchers rate our articles as excellent or good
Learn more about the work of our research integrity team to safeguard the quality of each article we publish.
Find out more
ORIGINAL RESEARCH article
Front. Ecol. Evol. , 01 November 2023
Sec. Environmental Informatics and Remote Sensing
Volume 11 - 2023 | https://doi.org/10.3389/fevo.2023.1264870
Soil moisture (SM) serves as a vital indicator reflecting environmental water conditions, but significant uncertainties still persist regarding how human activity and climate change affect SM. In this study, we quantified the influences of human activity and climate change on growing-season SM in the Qinghai–Tibet grasslands from 2000 to 2020. Climate change led to a decline in spatially mean SM at a rate of −0.01 and −0.06 g g−1 year−1 at 0–10 and 10–20 cm, respectively. Nonetheless, climate change caused the soil to become wetter in 39.97% and 22.29% areas at 0–10 and 10–20 cm, respectively. Human activity resulted in a decline in spatially mean SM by 36% and 21% at 0–10 and 10–20 cm, respectively. Nonetheless, human activity caused soil to become wetter in 2.82% areas at 0–10 cm and 30.03% areas at 10–20 cm. Therefore, both climate change and human activity have contributed to a pattern where the whole Qinghai–Tibet grasslands became drier while specific parts became wetter during the last 20 years. In addition to temperature and precipitation change, we should also pay attention to the response of SM to radiation change.
Soil moisture (SM), as a vital water resource, plays a crucial role in plant growth (Baumann et al., 2009; Bell et al., 2009; Berdanier and Klein, 2011). Under global change scenarios, SM undergoes significant alterations, which can subsequently impact plant growth through feedback mechanisms (Brito et al., 2013; Buttler et al., 2015; Berg et al., 2017). Numerous studies have explored the response of SM to climate change or human activity and their feedback, resulting in valuable scientific research achievements (Berg et al., 2017; Rigden et al., 2020; Humphrey et al., 2021). These achievements provide crucial support for the high-level development of human society (Flanagan and Johnson, 2005; Engel et al., 2009; Craine and Gelderman, 2011). However, previous studies have certain limitations in two ways. First, they have primarily focused on the influences of climate warming/cooling and decreased/increased precipitation on SM and their feedback to terrestrial ecosystems, whereas the influence of radiation change on SM and its feedback to terrestrial ecosystems are still lacking (Barron-Gafford et al., 2011; Fu et al., 2018). Radiation change is an integral part of climate change, and under global climate change, radiation decreases (You et al., 2010). It has been proven that radiation change can have obvious effects on the nutritional quality and storage of plant herbage, species diversity, and soil pH, which is even more important than climate warming and precipitation change (Fu et al., 2022; Tian and Fu, 2022). Considering the close relationships between SM and forage nutrition quality, species diversity, soil pH, etc. (Fu et al., 2018; Fu and Sun, 2022), further studies are imperative to understand the influence of brightening/dimming on SM. Second, whether climate change or human activity leads to soil drying or wetting is still controversial, and this uncertainty might be related to the relative intensity of changes in various key factors of human activity and climate change (Yu et al., 2019; Zhang and Fu, 2021). If precipitation does not change or decrease, warming should cause a decrease in surface SM (Fu et al., 2018; Han et al., 2023). Nonetheless, the influence of climate change on SM becomes uncertain when both temperature and precipitation increase simultaneously (Fu et al., 2018; Yu et al., 2019). If the increased magnitude of SM triggered by elevated precipitation is greater than the decreased magnitude of SM triggered by climate warming, climate change will cause soil to become wet; otherwise, it will cause the soil to become dry (Shen et al., 2015). Grazing reduces transpiration by harvesting herbage from herbivores, whereas nitrogen fertilizer addition generally increases transpiration by increasing grassland productivity (Fu and Shen, 2016; Zhang and Fu, 2021). The response of SM to grazing activities differs with increasing grazing intensity and grazing season in grassland ecosystems (Sun et al., 2021; Zhang and Fu, 2021). Accordingly, it is essential to further strengthen studies on the influences of human activity and climate change on SM.
The grasslands on the Qinghai–Tibet Plateau (TP) are important parts of global grassland ecological systems. It is commonly believed that climate warming and increased precipitation on the TP led to an overall improvement of grassland ecological systems, but they also caused deterioration in some specific areas (Hu et al., 2020; Li et al., 2020). Nonetheless, many studies have demonstrated that, in comparison to precipitation, SM is more closely associated with grassland productivity and forage nutrient quality, offering a better reflection of the influence of environmental water conditions on these aspects (Baumann et al., 2009; Fu et al., 2018). Therefore, SM serves as a key limiting factor in grassland ecosystems, affecting herbage yield, nutrient quality, and the development of animal husbandry on the TP. Unfortunately, understanding its response to human activities and climate change is limited and has been mainly studied on a small scale (Fu et al., 2018; Dai et al., 2022), which hinders accurate quantification of its influence on grassland ecosystems across the region. Accordingly, it is essential to strengthen studies on the response of SM to human activity and climate change in the TP grasslands.
The objective of this study is to examine the response of growing season SM to human activity and climate change at 0–10 and 10–20 cm in the TP grasslands during the period of 2000–2020. This study is expected to provide valuable insights into managing soil water dynamics under the influence of human activity and climate change. Furthermore, agricultural sector managers can better identify soil drought by exploring the influence law of human activity and climate change on SM. We hypothesized that climate change caused soil wetting over the whole TP grasslands. Based on previous studies (Sun et al., 2023; Zhang et al., 2023), we hypothesized that human activities may alter the sensitivities of SM to climate change.
We utilized random forest models to derive monthly mean soil moisture data (SMp_0-10, SMa_0-10, SMp_10-20, and SMa_10-20) during the growing season (May–September) from 2000 to 2020 across the TP grassland region (Wang and Fu, 2023). The randomForest package of the R software was used to construct the random forest models of SM (Wang and Fu, 2023). Observed SM at 0–10 cm, air temperature, precipitation, and radiation data inside the enclosure were used to obtain the random forest models of SMp_0-10 (Wang and Fu, 2023). Observed SM at 0–10 cm, air temperature, precipitation, radiation, and normalized difference vegetation index (NDVI) data outside the enclosure were used to obtain the random forest models of SMa_0-10 (Wang and Fu, 2023). Similarly, the random forest models of SMp_10-20 and SMa_10-20 were constructed (Wang and Fu, 2023).
SMp_0-10, SMa_0-10, SMp_10-20, and SMa_10-20 referred to the potential SM at 0–10 cm, actual SM at 0–10 cm, potential SM at 10–20 cm, and actual SM at 10–20 cm, respectively. Detailed descriptions of the monthly precipitation, temperature, radiation, and/or NDVI from 2000 to 2020 were reported in our previous studies (Fu et al., 2022). We obtained growing season mean SMp_0-10, SMa_0-10, SMp_10-20, and SMa_10-20 using corresponding monthly SMp_0-10, SMa_0-10, SMp_10-20, and SMa_10-20 in 2000–2020, respectively. We also calculated growing season total precipitation, mean temperature, total radiation, and mean NDVI (GST, GSP, GSRad, and GSNDVI) using monthly total precipitation, mean temperature, total radiation, and NDVI, respectively. All these values were computed for all pixels with a spatial resolution of 1 km × 1 km across the entire TP grasslands.
The slope is often used to reflect the change trend of a specific variable (Fu et al., 2022; Wang et al., 2022; Sun et al., 2023) since we obtained the slope of GSP (ΔGSP), GST (ΔGST), GSRad (ΔGSRad), GSNDVI (ΔGSNDVI), SMp_0-10 (ΔSMp_0-10), SMa_0-10 (ΔSMa_0-10), SMp_10-20 (ΔSMp_10-20), and SMa_10-20 (ΔSMa_10-20) using the sens.slope function of the trend package. The ggscatter function of the ggpubr package was used to obtain the relationship between SMp_0-10, SMa_0-10, SMp_10-20, or SMa_10-20 and longitude, latitude, elevation, GST, GSP, GSRad, ΔGST, ΔGSP, and ΔGSRad. The ggscatter function of the ggpubr package was also used to obtain the relationship between SMa_0-10 or SMa_10-20 and GSNDVI and ΔGSNDVI. The varpart function of the vegan package was used to quantify the relative impacts of the three geographic variables (i.e., longitude, latitude, and elevation), or the three mean climate condition variables (i.e., GST, GSP, and GSRad), or the three climate change variables (i.e., ΔGST, ΔGSP, and ΔGSRad) on SMp_0-10, SMa_0-10, SMp_10-20, or SMa_10-20. We quantified the relative impacts of geographic position, mean climate conditions, and climate change on SMp_0-10, SMa_0-10, SMp_10-20, or SMa_10-20.
The RSM_0-10 was the ratio of SMa_0-10 to SMp_0-10, and the RSM_10-20 was the ratio of SMa_10-20 to SMp_10-20 (Equations 1 and 2).
Both the SMp_0-10 and SMp_10-20 were only affected by climate change, whereas both the SMa_0-10 and SMa_10-20 were simultaneously affected by climate change and human activity. In other words, both the RSM_0-10 and RSM_10-20 exclude the effects of climate change, and they are only affected by human activity. Therefore, the RSM_0-10 and RSM_10-20 can indicate the effects of human activity on SM at 0–10 and 10–20 cm, respectively. We obtained the change slope of RSM_0-10 and RSM_10-20 (i.e., ΔRSM_0-10 and ΔRSM_10-20) (Fu et al., 2022). The ggscatter function of the ggpubr package was used to obtain the relationship between RSM_0-10 or RSM_10-20, or ΔRSM_0-10 or ΔRSM_10-20 and longitude, latitude, elevation, GST, GSP, GSRad, GSNDVI, ΔGST, ΔGSP, ΔGSRad, and ΔGSNDVI. We quantified the relative impacts of the three geographic variables (i.e., longitude, latitude, and elevation), or the three mean climate condition variables (i.e., GST, GSP, and GSRad), or the three climate change variables (i.e., ΔGST, ΔGSP, and ΔGSRad) on RSM_0-10, RSM_10-20, ΔRSM_0-10, and ΔRSM_10-20. We quantified the relative impacts of geographic position, mean climate conditions + GSNDVI, and climate change + ΔGSNDVI on RSM_0-10, RSM_10-20, ΔRSM_0-10, and ΔRSM_10-20. The statistical software used was R.4.2.2.
Different regions exhibited different patterns for ΔSMp_0-10, ΔSMp_10-20, ΔSMa_0-10, and ΔSMa_10-20 (Figure 1). Spatially mean values of ΔSMp_0-10, ΔSMp_10-20, ΔSMa_0-10, and ΔSMa_10-20 were −0.01 (from −0.46 to 0.54 g g−1 year−1), −0.06 (from −0.42 to 0.27 g g−1 year−1), 0.02 (from −0.42 to 0.51 g g−1 year−1), and −0.02 g g−1 year−1 (from −0.56 to 0.49 g g−1 year−1), respectively. There were 39.97%, 22.29%, 56.60%, and 34.71% areas showing increasing trends for ΔSMp_0-10, ΔSMp_10-20, ΔSMa_0-10, and ΔSMa_10-20, respectively. In contrast, there were 60.03%, 77.67%, 43.40%, and 65.29% areas showing decreasing trends for ΔSMp_0-10, ΔSMp_10-20, ΔSMa_0-10, and ΔSMa_10-20, respectively. Longitude had higher influences on ΔSMp_0-10 and ΔSMa_0-10 than latitude and elevation (Figure S1). In contrast, latitude had higher influences on ΔSMp_10-20 and ΔSMa_10-20 than longitude and elevation (Figure S2). Compared to GST and GSRad, GSP had higher influences on ΔSMp_0-10, ΔSMp_10-20, and ΔSMa_10-20 (Figures S3, S4). In contrast, compared to GST and GSP, GSRad had a greater effect on ΔSMa_0-10 (Figure S3). The ΔGSP had the greatest effects on ΔSMp_0-10 and ΔSMa_0-10, and the ΔGST had the lowest effects on ΔSMp_0-10 and ΔSMa_0-10 among the three variables of climate change (Figure S5). In contrast, compared to ΔGST and ΔGSP, ΔGSRad had higher influences on ΔSMp_10-20 and ΔSMa_10-20 (Figure S6). The ΔGST, ΔGSP, and ΔGSRad had higher influences on ΔSMp_10-20 than ΔSMp_0-10 (Figures S5, S6). The overall effect of the three variables of climate change on the ΔSMp_10-20 was greater than that on ΔSMp_0-10 (Figure S7). The ΔGSP had greater exclusionary influences on ΔSMp_0-10 and ΔSMa_0-10, but the ΔGSRad had higher exclusionary influences on ΔSMp_10-20 and ΔSMa_10-20 among the three variables of climate change (Figures S7, S8). The GSNDVI and ΔGSNDVI significantly affected ΔSMa_0-10 and ΔSMa_10-20 (Figure S9). Climate change had the greatest exclusionary effects on ΔSMp_0-10, ΔSMa_0-10, ΔSMp_10-20, and ΔSMa_10-20 than geographical position and mean climate conditions (Figure 2). Mean climate conditions had higher exclusionary influences on ΔSMp_0-10 and ΔSMp_10-20 than geographical position (Figure 2). Mean climate conditions and geographical position had the same exclusionary effects on ΔSMa_0-10 and ΔSMa_10-20 (Figure 2).
Figure 2 Relative influences of geographical position, mean climate conditions, and climate change to (A) ΔSMp_0–10, (B) ΔSMa_0–10, (C) ΔSMp_10–20, and (D) ΔSMa_10–20.
Spatially mean values of RSM_0-10 and RSM_10-20 were 0.64 (from 0.28 to 1.45) and 0.79 (from 0.33 to 1.97), respectively (Figure 3). There were only 2.82% and 30.03% areas where RSM_0-10 and RSM_10-20 values were greater than one, respectively. In contrast, there were 97.18% and 69.97% areas where RSM_0-10 and RSM_10-20 values were lower than one, respectively. Longitude had the strongest effects on RSM_0-10 and RSM_10-20, but latitude had the least effects on RSM_0-10 and RSM_10-20 among the three geography variables (Figure S10). The GSP had the strongest effects on RSM_0-10 and RSM_10-20, but the GST had the least effects on RSM_0-10 and RSM_10-20 among the three variables of mean climate conditions (Figure S11). Compared to the three variables of mean climate conditions, the GSNDVI had higher influences on RSM_0-10 and RSM_10-20 (Figure S11). The ΔGSP had the strongest effects on RSM_0-10 and RSM_10-20, but the ΔGSRad had the least effects on RSM_0-10 and RSM_10-20 among the three variables of climate change (Figure S12). The ΔGSNDVI also had some effects on RSM_0-10 and RSM_10-20 (Figure S12). Longitude had the greatest exclusionary effects on RSM_0-10 and RSM_10-20, but elevation had the least exclusionary effects on RSM_0-10 and RSM_10-20 among the three geography variables (Figure S13). Compared to the three variables of mean climate conditions, the GSNDVI had the greater exclusionary influences on RSM_0-10 and RSM_10-20 (Figure S13). The GST had the greatest exclusionary effects on RSM_0-10 and RSM_10-20, but the GSRad had the lowest exclusionary effects on RSM_0-10 and RSM_10-20 among the three variables of mean climate conditions (Figure S13). The ΔGST had the strongest exclusionary influences on RSM_0-10, but the ΔGSP had the strongest exclusionary influences on RSM_10-20 among the three variables of climate change (Figure S13). The ΔGSRad had the lowest exclusionary effects on RSM_0-10 and RSM_10-20 among the three variables of climate change (Figure S13). The ΔGSNDVI also had some exclusionary effects on RSM_0-10 and RSM_10-20 (Figure S13). Mean climate conditions + GSNDVI had the greatest exclusionary effects on RSM_0-10 and RSM_10-20, but the geographical position had the lowest exclusionary effects on RSM_0-10 and RSM_10-20 (Figure 4).
Figure 4 Relative influences of geographical position, mean climate conditions + GSNDVI, and climate change + slope_GSNDVI to (A) RSM_0–10, (B) ΔRSM_0–10, (C) RSM_10–20, and (D) ΔRSM_10–20.
Spatially mean values of ΔRSM_0-10 and ΔRSM_10-20 were 0.0011 (from −0.0287 to 0.0263) and 0.0013 (from −0.0590 to 0.0564), respectively (Figure 3). There were 63.78% and 53.36% areas showing increasing trends for ΔRSM_0-10 and ΔRSM_10-20, respectively. In contrast, there were 36.22% and 46.64% areas showing decreasing trends for ΔRSM_0-10 and ΔRSM_10-20, respectively. The three geography variables and GSNDVI had some effects on ΔRSM_0-10 and ΔRSM_10-20 (Figures S14, S15). The GSP had the strongest effects on ΔRSM_0-10 and ΔRSM_10-20, but the GST had the lowest effect on ΔRSM_0-10 and ΔRSM_10-20 among the three variables of mean climate conditions (Figure S15). Compared to the three variables of climate change, the ΔGSNDVI had the higher influences on ΔRSM_0-10 and ΔRSM_10-20 (Figure S16). Compared to the three variables of climate change, the ΔGSNDVI had the higher exclusionary influences on ΔRSM_0-10 and ΔRSM_10-20 (Figure S17). Climate change + ΔGSNDVI had the greatest exclusionary effects on ΔRSM_0-10 and ΔRSM_10-20, but the geographical position had the lowest exclusionary effects on ΔRSM_0-10 and ΔRSM_10-20 (Figure 4).
Inconsistent with the hypothesis, this study implies that climate change resulted in soil drying trends across the entire TP grassland region during the past 20 years. The finding is likely attributed to the elevated evapotranspiration triggered by climate warming offsets and even exceeds the water increase triggered by increased precipitation (Berg et al., 2017). These findings contradict previous studies that suggested a general increase in wetness across the TP grasslands in recent years (Lu and Liu, 2010; Diffenbaugh and Field, 2013). Moreover, this study also showed that the proportion of soil drying (≥43.40%) outweighed the proportion of drought trend based on precipitation (22.57%) in the TP grassland regions during the last 20 years. These discoveries indicated that previous assessments of climate humidity trends based solely on precipitation may have underestimated the extent of drying. It is important to note that precipitation does not always effectively support plant growth, and SM may be more associated with plant growth (Fu et al., 2018; Rigden et al., 2020).
Climate warming, changes in precipitation, and radiation can significantly affect SM in the TP grasslands. This finding is consistent with previous research (Yu et al., 2019; Fu and Shen, 2022; Han et al., 2023). Notably, the exclusionary effects of radiation change on SM emphasize the necessity of considering the impacts of radiation change on grassland ecosystems in addition to climate warming and decreased/increased precipitation, particularly on the TP. These exclusionary effects of radiation change on SM also align with some previous findings that demonstrated its notable impact on vegetation carbon use efficiency, plant physiological characteristics, forage nutritional quality, and storage on the TP (Fu et al., 2016; Fu et al., 2022; Li and Fu, 2023).
This study shows that climate change had non-linear effects on SM in the TP grassland areas. This aligns with previous studies (Shen et al., 2015; Fu et al., 2018) and can be attributed to several underlying mechanisms. First, it is essential to consider that SM holding capacity is not infinite and that there exists a state of soil saturation where the soil cannot hold more water. Although increased precipitation can initially lead to an increase in SM, the point of saturation will eventually be reached, and further increases in precipitation will not result in additional gains in SM (Shen et al., 2015; Fu et al., 2018). The level of soil saturation is largely influenced by soil porosity, which, in turn, can be altered by factors such as plant growth and soil organic matter decomposition. These processes are directly or indirectly influenced by climate change (Fu et al., 2018; Zhang et al., 2022). Second, the influences of climate change on SM can be closely associated with the magnitude of climate change (Xu et al., 2013), local climate conditions (Fu and Sun, 2022), and geographical location (Zhang et al., 2021). Third, the influences of climate change on SM depend on their relative intensity of the influences of climate warming, increased/decreased precipitation, and dimming/brightening on SM. However, it is crucial to note that the influences of these factors on SM are not simply additive or subtractive effects in nature (Yu et al., 2019; Xiao et al., 2023).
This study shows that climate change had stronger negative effects on ΔSMp_10-20 than ΔSMp_0-10. This is similar to previous studies (Rui et al., 2011; Berg et al., 2017; Dai et al., 2022), and several potential reasons contribute to this phenomenon. First, the dominant climate change factors affecting SM were different between soil depths under climate change scenarios (Figure S7). Second, the influences of climate change on SM were related to local water availability and warming magnitude (Rui et al., 2011; Xu et al., 2013). Both local SM and increase in soil temperature generally decrease with the increasing soil depth (Rui et al., 2011). Third, plant roots interspersed in soil pores draw water from water-bearing soil pores and generally decline with increasing soil depth (Berg et al., 2017). Although climate warming generally stimulates plant root growth and increases root biomass, such a boosting effect varies with soil depth (Fu et al., 2015).
This study shows that climate change resulted in the spatial relocation of SM across the TP grassland areas. This finding is consistent with previous studies (Wang et al., 2021a; Fu and Sun, 2022) and can be explained by one or more of the following reasons. First, significant spatial variations in the direction and amplitude of climate change have directly led to the spatial relocation of air temperature, precipitation, radiation, and wind (Huang and Fu, 2023). Moreover, because of such spatial heterogeneity of climate change, soil temperature and vapor pressure deficit can indirectly be relocated (Wang et al., 2021b; Han et al., 2022). Increases in wind speed, soil temperature, and vapor pressure deficit can generally elevate evapotranspiration, leading to a reduction in SM (Xu et al., 2013; Fu et al., 2018). Second, different species or function groups of plants may have diverse physiological water requirements, root growth depths, and phyllosphere microbes, indicating the change in plant growth, α-diversity, and community composition can also affect SM (Lian et al., 2020; Huang et al., 2023). Climate change can result in spatial relocation of plant growth and community structure (Wang et al., 2021a; Fu and Sun, 2022; Huang and Fu, 2023).
In line with the hypothesis, this study shows that human activity strengthened the sensitivities of SM at 0–10 cm but weakened the sensitivities of SM at 10–20 cm to climate change. This is similar to previous studies (Rui et al., 2011; Zhang et al., 2020), and the underlying mechanism for this phenomenon is likely rooted in the fact human activity may have the capacity to either amplify or attenuate the influences of climate change on critical factors, including soil temperature, vapor pressure deficit, plant growth, and community structure (Wang et al., 2012; Fu and Shen, 2016; Li and Fu, 2023).
Furthermore, this study demonstrated that the influence of human activity on SM was related to soil depth. This is in agreement with previous studies (Sun et al., 2019). Several factors may account for this relationship. First, the growth of the human population has led to an increasing demand for groundwater, which serves as a vital supply of SM and is more proximate to the 10–20 cm soil layer. Second, the effects of human activity on soil temperature, bulk density, and root density are dependent on soil depth (Rui et al., 2011; Sun et al., 2019; Lai and Kumar, 2020).
In addition to soil depth, our study also indicated that the influence of human activity on SM varied from year to year (Figure 3), which aligned with findings from some previous studies (Sun et al., 2021; Zhang and Fu, 2021). This variability is likely attributable to at least one of the following factors. First, human activity can have cumulative influences on plant growth and community structure, etc. (Fu and Shen, 2016; Zhao et al., 2016; Gao and Carmel, 2020). Second, climate conditions can regulate the influence of human activity on SM (Figure S11), and climate conditions vary from year to year. Third, the intensity and extent of human activity also varied from year to year.
This study shows that human activity resulted in the spatial relocation of SM across the TP grassland ecological systems. This is in agreement with some previous studies (Zhang et al., 2020; Zhang and Fu, 2021). The underlying mechanisms for this phenomenon are likely rooted in at least one of the next causes. First, both the scope and intensity of human activity are spatiotemporal heterogeneous (Sun et al., 2019; Zha et al., 2022). For example, warm- and cold-season grazing may have diverse influences on SM (Sun et al., 2021; Zhang and Fu, 2021), and they exhibit spatial heterogeneity. Second, human activity may result in the spatial relocation of vapor pressure deficit, soil temperature, porosity, and compactness (Fu et al., 2012; Fu and Shen, 2016). Third, influences of human activity on SM were related to plant growth (Figures S11, S12), and human activity may result in spatial relocation of plant growth (Fu et al., 2022; Zha et al., 2022; Li and Fu, 2023). Moreover, human activity can also result in spatial relocation of plant α-diversity and community composition in TP grassland ecological systems (Sun et al., 2021; Zha et al., 2022).
This study represents the earliest investigation into the influences of both human activity and climate change on SM at 0–10 and 10–20 cm across the entire grasslands on the TP over the past 20 years. Our findings suggest that the impacts of human activity and climate change on SM were dynamic, varying with soil depth and geographical position. Notably, climate change resulted in topsoil drying of grassland areas on the TP as a whole, a phenomenon that previous research greatly underestimated. The effects of climate change on soil moisture are non-linear, with a stronger negative impact on SM at 10–20 cm than at 0–10 cm. Importantly, when compared to climate warming and precipitation change, radiation change exhibited significant exclusionary effects on SM, emphasizing the need for serious consideration of radiation change influences on SM. Additionally, human activity can modify the sensitivity of SM to climate change. Both human activity and climate change can contribute to the spatial relocation of SM.
The original contributions presented in the study are included in the article/Supplementary Material. Further inquiries can be directed to the corresponding author.
JX: Writing – original draft. CY: Writing – original draft. GF: Writing – original draft, Investigation, Writing – review & editing.
The author(s) declare financial support was received for the research, authorship, and/or publication of this article. This research was funded by the Chinese Academy of Sciences Youth Innovation Promotion Association (2020054), the Pilot Project of Chinese Academy of Sciences (XDA26050501), the China National Key Scientific Research Project (2021YFD1000303), the National Natural Science Foundation of China (31600432), the Science and Technology Project of Tibet Autonomous Region (XZ202301YD0012C, XZ202202YD0009C, XZ202201ZY0003N, XZ202101ZD0007G, and XZ202101ZD0003N), and the construction project of fixed observation and experimental station of first and try support system for agricultural green development in Zhongba County.
The authors declare that the research was conducted in the absence of any commercial or financial relationships that could be construed as a potential conflict of interest.
All claims expressed in this article are solely those of the authors and do not necessarily represent those of their affiliated organizations, or those of the publisher, the editors and the reviewers. Any product that may be evaluated in this article, or claim that may be made by its manufacturer, is not guaranteed or endorsed by the publisher.
The Supplementary Material for this article can be found online at: https://www.frontiersin.org/articles/10.3389/fevo.2023.1264870/full#supplementary-material
Barron-Gafford G. A., Scott R. L., Jenerette G. D., Huxman T. E. (2011). The relative controls of temperature, soil moisture, and plant functional group on soil CO2 efflux at diel, seasonal, and annual scales. J. Geophys. Res. Biogeosci 116. doi: 10.1029/2010JG001442
Baumann F., He J. S., Schmidt K., Kuhn P., Scholten T. (2009). Pedogenesis, permafrost, and soil moisture as controlling factors for soil nitrogen and carbon contents across the Tibetan Plateau. Global Change Biol. 15, 3001–3017. doi: 10.1111/j.1365-2486.2009.01953.x
Bell C. W., Acosta-Martinez V., Mcintyre N. E., Cox S., Tissue D. T., Zak J. C. (2009). Linking microbial community structure and function to seasonal differences in soil moisture and temperature in a Chihuahuan desert grassland. Microbial Ecol. 58, 827–842. doi: 10.1007/s00248-009-9529-5
Berdanier A. B., Klein J. A. (2011). Growing season length and soil moisture interactively constrain high elevation aboveground net primary production. Ecosystems 14, 963–974. doi: 10.1007/s10021-011-9459-1
Berg A., Sheffield J., Milly P. C. D. (2017). Divergent surface and total soil moisture projections under global warming. Geophys. Res. Lett. 44, 236–244. doi: 10.1002/2016GL071921
Brito P., Trujillo J. L., Morales D., Jimenez M. S., Wieser G. (2013). Soil moisture overshadows temperature control over soil CO2 efflux in a Pinus canariensis forest at treeline in Tenerife, Canary Islands. Acta Oecol. 48, 1–6. doi: 10.1016/j.actao.2013.01.006
Buttler A., Robroek B. J. M., Laggoun-Défarge F., Jassey V. E. J., Pochelon C., Bernard G., et al. (2015). Experimental warming interacts with soil moisture to discriminate plant responses in an ombrotrophic peatland. J. Veg. Sci. 26, 964–974. doi: 10.1111/jvs.12296
Craine J. M., Gelderman T. M. (2011). Soil moisture controls on temperature sensitivity of soil organic carbon decomposition for a mesic grassland. Soil Biol. Biochem. 43, 455–457. doi: 10.1016/j.soilbio.2010.10.011
Dai L. C., Fu R. Y., Guo X. W., Du Y. G., Zhang F. W., Cao G. M. (2022). Soil moisture variations in response to precipitation across different vegetation types on the northeastern Qinghai-Tibet plateau. Front. Plant Sci. 13. doi: 10.3389/fpls.2022.854152
Diffenbaugh N. S., Field C. B. (2013). Changes in ecologically critical terrestrial climate conditions. Science 341, 486–492. doi: 10.1126/science.1237123
Engel E. C., Weltzin J. F., Norby R. J., Classen A. T. (2009). Responses of an old-field plant community to interacting factors of elevated CO2 , warming, and soil moisture. J. Plant Ecol. UK 2, 1–11. doi: 10.1093/jpe/rtn026
Flanagan L. B., Johnson B. G. (2005). Interacting effects of temperature, soil moisture and plant biomass production on ecosystem respiration in a northern temperate grassland. Agr. For. Meteorol. 130, 237–253. doi: 10.1016/j.agrformet.2005.04.002
Fu G., Li S. W., Sun W., Shen Z. X. (2016). Relationships between vegetation carbon use efficiency and climatic factors on the Tibetan Plateau. Can. J. Remote Sens. 42, 16–26. doi: 10.1080/07038992.2016.1131115
Fu G., Shen Z. X., Sun W., Zhong Z. M., Zhang X. Z., Zhou Y. T. (2015). A meta-analysis of the effects of experimental warming on plant physiology and growth on the Tibetan Plateau. J. Plant Growth Regul. 34, 57–65. doi: 10.1007/s00344-014-9442-0
Fu G., Shen Z. X. (2016). Response of alpine plants to nitrogen addition on the Tibetan Plateau: A meta-analysis. J. Plant Growth Regul. 35, 974–979. doi: 10.1007/s00344-016-9595-0
Fu G., Shen Z. (2022). Asymmetrical warming of growing/non-growing season increases soil respiration during growing season in an alpine meadow. Sci. Total Environ. 812. doi: 10.1016/j.scitotenv.2021.152591
Fu G., Shen Z. X., Zhang X. Z. (2018). Increased precipitation has stronger effects on plant production of an alpine meadow than does experimental warming in the Northern Tibetan Plateau. Agr. For. Meteorol. 249, 11–21. doi: 10.1016/j.agrformet.2017.11.017
Fu G., Shen Z. X., Zhang X. Z., Zhou Y. T., Zhang Y. J. (2012). Response of microbial biomass to grazing in an alpine meadow along an elevation gradient on the Tibetan Plateau. Eur. J. Soil Biol. 52, 27–29. doi: 10.1016/j.ejsobi.2012.05.004
Fu G., Sun W. (2022). Temperature sensitivities of vegetation indices and aboveground biomass are primarily linked with warming magnitude in high-cold grasslands. Sci. Total Environ., 843. doi: 10.1016/j.scitotenv.2022.157002
Fu G., Wang J., Li S. (2022). Response of forage nutritional quality to climate change and human activities in alpine grasslands. Sci. Total Environ., 845. doi: 10.1016/j.scitotenv.2022.157552
Gao J. J., Carmel Y. (2020). A global meta-analysis of grazing effects on plant richness. Agr. Ecosyst. Environ., 302. doi: 10.1016/j.agee.2020.107072
Han F. S., Yu C. Q., Fu G. (2022). Warming alters elevation distributions of soil bacterial and fungal communities in alpine grasslands. Global Ecol. Conserv. 39. doi: 10.1016/j.gecco.2022.e02306
Han F., Yu C., Fu G. (2023). Non-growing/growing season non-uniform-warming increases precipitation use efficiency but reduces its temporal stability in an alpine meadow. Front. Plant Sci. 14. doi: 10.3389/fpls.2023.1090204
Hu Y., Wang S., Niu B., Chen Q., Zhang G. (2020). Effect of increasing precipitation and warming on microbial community in Tibetan alpine steppe. Environ. Res., 189. doi: 10.1016/j.envres.2020.109917
Huang S., Fu G. (2023). Impacts of climate change and human activities on plant species α-diversity across the Tibetan grasslands. Remote Sens. doi: 10.3390/rs15112947
Huang S., Zha X., Fu G. (2023). Affecting factors of plant phyllosphere microbial community and their responses to climatic warming – A Review. Plants 12, 2891. doi: 10.3390/plants12162891
Humphrey V., Berg A., Ciais P., Gentine P., Jung M., Reichstein M., et al. (2021). Soil moisture-atmosphere feedback dominates land carbon uptake variability. Nature 592, 65–6+. doi: 10.1038/s41586-021-03325-5
Lai L. M., Kumar S. (2020). A global meta-analysis of livestock grazing impacts on soil properties. PLoS One 15. doi: 10.1371/journal.pone.0236638
Li M., Zhang X., Niu B., Yongtao H., Wu J. (2020). Changes in plant species richness distribution in Tibetan alpine grasslands under different precipitation scenarios. Global Ecol. Conserv. 21. doi: 10.1016/j.gecco.2019.e00848
Li S., Fu G. (2023). Impacts of anthropogenic activities and climate change on forage nutrition storage in Tibetan grasslands. Plants 12, 2735. doi: 10.3390/plants12142735
Lian X., Piao S., Li L. Z., Li Y., Huntingford C., Ciais P., et al. (2020). Summer soil drying exacerbated by earlier spring greening of northern vegetation. Sci. Adv. 6. doi: 10.1126/sciadv.aax0255
Lu H. L., Liu G. F. (2010). Trends in temperature and precipitation on the Tibetan Plateau 1961-2005. Climate Res. 43, 179–190. doi: 10.3354/cr00909
Rigden A. J., Mueller N. D., Holbrook N. M., Pillai N., Huybers P. (2020). Combined influence of soil moisture and atmospheric evaporative demand is important for accurately predicting US maize yields. Nat. Food 1. doi: 10.1038/s43016-020-0028-7
Rui Y. C., et al. (2011). Warming and grazing affect soil labile carbon and nitrogen pools differently in an alpine meadow of the Qinghai-Tibet Plateau in China. J. Soil Sediment. 11, 903–914. doi: 10.1007/s11368-011-0388-6
Shen Z. X., Li Y. L., Fu G. (2015). Response of soil respiration to short-term experimental warming and precipitation pulses over the growing season in an alpine meadow on the Northern Tibet. Appl. Soil Ecol. 90, 35–40. doi: 10.1016/j.apsoil.2015.01.015
Sun J., Zhan T., Liu M., Zhang Z., Wang Y., Liu S., et al. (2019). Verification of the biomass transfer hypothesis under moderate grazing across the Tibetan plateau: a meta-analysis. Plant Soil. doi: 10.1007/s11104-019-04380-8
Sun W., Li S., Zhang G., Fu G., Qi H., Li T. (2023). Effects of climate change and anthropogenic activities on soil pH in grassland regions on the Tibetan Plateau. Global Ecol. Conserv. 45. doi: 10.1016/j.gecco.2023.e02532
Sun W., Li S., Wang J., Fu G. (2021). Effects of grazing on plant species and phylogenetic diversity in alpine grasslands, Northern Tibet. Ecol. Eng. 170. doi: 10.1016/j.ecoleng.2021.106331
Tian Y., Fu G. (2022). Quantifying plant species α-diversity using normalized difference vegetation index and climate data in alpine grasslands. Remote Sens. 14. doi: 10.3390/rs14195007
Wang S., Duan J., Xu G., Wang Y., Zhang Z., Rui Y., et al. (2012). Effects of warming and grazing on soil N availability, species composition, and ANPP in an alpine meadow. Ecology 93, 2365–2376. doi: 10.1890/11-1408.1
Wang S., Fu G. (2023). Modelling soil moisture using climate data and normalized difference vegetation index based on nine algorithms in alpine grasslands. Front. Environ. Sci. 11. doi: 10.3389/fenvs.2023.1130448
Wang J., Li M., Yu C., Fu G. (2022). The change in environmental variables linked to climate change has a stronger effect on aboveground net primary productivity than does phenological change in alpine grasslands. Front. Plant Sci. 12. doi: 10.3389/fpls.2021.798633
Wang J., Yu C., Fu G. (2021a). Warming reconstructs the elevation distributions of aboveground net primary production, plant species and phylogenetic diversity in alpine grasslands. Ecol. Indic. 133. doi: 10.1016/j.ecolind.2021.108355
Wang J. W., Yu C. Q., Fu G. (2021b). Asymmetrical warming between elevations may result in similar plant community composition between elevations in alpine grasslands. Front. Ecol. Evol. doi: 10.3389/fevo.2021.757943
Xiao J., Yu C., Fu G. (2023). Response of aboveground net primary production, species and phylogenetic diversity to warming and increased precipitation in an alpine meadow. Plants 12, 3017. doi: 10.3390/plants12173017
Xu W., Yuan w., Dong W., Xia J., Liu D., Chen Y. (2013). A meta-analysis of the response of soil moisture to experimental warming. Environ. Res. Lett. 8. doi: 10.1088/1748-9326/8/4/044027
You Q., Kang S., Flügel W. A., Sanchez-Lorenzo A., Yan Y., Huang J., et al. (2010). From brightening to dimming in sunshine duration over the eastern and central Tibetan Plateau, (1961-2005). Theor. Appl. Climatol. 101, 445–457. doi: 10.1007/s00704-009-0231-9
Yu C. Q., Wang J. W., Shen Z. X., Fu G. (2019). Effects of experimental warming and increased precipitation on soil respiration in an alpine meadow in the Northern Tibetan Plateau. Sci. Total Environ. 647, 1490–1497. doi: 10.1016/j.scitotenv.2018.08.111
Zha X. J., Tian Y., Fu G. (2022). Response of forage nutrient storages to grazing in alpine grasslands. Front. Plant Sci. doi: 10.3389/fpls.2022.991287
Zhang G., Dai E., Dawaqiongda, Luobu, Fu G. (2023). Effects of climate change and fencing on forage nutrition quality of alpine grasslands in the Northern Tibet. Plants 12, 3182. doi: 10.3390/plants12183182
Zhang H., Fu G. (2021). Responses of plant, soil bacterial and fungal communities to grazing vary with pasture seasons and grassland types, northern Tibet. Land Degrad. Dev. 32, 1821–1832. doi: 10.1002/ldr.3835
Zhang H., Li S., Zhang G., Fu G. (2020). Response of soil microbial communities to warming and clipping in alpine meadows in Northern Tibet. Sustainability 12. doi: 10.3390/su12145617
Zhang G., Shen Z., Fu G. (2021). Function diversity of soil fungal community has little exclusive effects on the response of aboveground plant production to experimental warming in alpine grasslands. Appl. Soil Ecol. 168. doi: 10.1016/j.apsoil.2021.104153
Zhang G., Shen Z., Fu G. (2022). Geo-distribution patterns of soil fungal community of Pennisetum flaccidum in Tibet. J. Fungi 8. doi: 10.3390/jof8111230
Zhao J. X., Luo T. X., Li R. C., Li X., Tian L. H. (2016). Grazing effect on growing season ecosystem respiration and its temperature sensitivity in alpine grasslands along a large altitudinal gradient on the central Tibetan Plateau. Agr. For. Meteorol. 218, 114–121. doi: 10.1016/j.agrformet.2015.12.005
Keywords: soil drying, soil quality, random forest, alpine region, data mining
Citation: Xiao J, Yu C and Fu G (2023) Influences of human activity and climate change on growing-season soil moisture in the Qinghai–Tibet grasslands from 2000 to 2020. Front. Ecol. Evol. 11:1264870. doi: 10.3389/fevo.2023.1264870
Received: 21 July 2023; Accepted: 16 October 2023;
Published: 01 November 2023.
Edited by:
Luis Gomez, University of Las Palmas de Gran Canaria, SpainCopyright © 2023 Xiao, Yu and Fu. This is an open-access article distributed under the terms of the Creative Commons Attribution License (CC BY). The use, distribution or reproduction in other forums is permitted, provided the original author(s) and the copyright owner(s) are credited and that the original publication in this journal is cited, in accordance with accepted academic practice. No use, distribution or reproduction is permitted which does not comply with these terms.
*Correspondence: Gang Fu, ZnVnYW5nQGlnc25yci5hYy5jbg==
Disclaimer: All claims expressed in this article are solely those of the authors and do not necessarily represent those of their affiliated organizations, or those of the publisher, the editors and the reviewers. Any product that may be evaluated in this article or claim that may be made by its manufacturer is not guaranteed or endorsed by the publisher.
Research integrity at Frontiers
Learn more about the work of our research integrity team to safeguard the quality of each article we publish.