- 1Ecology and Evolutionary Biology, University of Kansas, Lawrence, KS, United States
- 2Kansas Biological Survey & Center for Ecological Research, University of Kansas, Lawrence, KS, United States
- 3Environmental Studies, University of Kansas, Lawrence, KS, United States
The loss of plant productivity with declining diversity is well established, exceeding other global change drivers including drought. These patterns are most clearly established for aboveground productivity, it remains poorly understood whether productivity increases associated with diversity are replicated belowground. To address this gap, we established a plant diversity-manipulation experiment in 2018. It is a full factorial manipulation of plant species richness and community composition, and precipitation. Three and five years post-establishment, two bulk soil cores (20cm depth) were collected and composited from each plot and were processed for roots to determine belowground biomass as root standing crop. We observed a strong positive relationship between richness and aboveground production and belowground biomass, generating positive combined above and belowground with diversity. Root standing crop increased 1.4-fold from years three to five. Grass communities produced more root biomass (monoculture mean 463.9 ± 410.3g m−2), and the magnitude of the relationship between richness and root standing crop was greatest within those communities. Legume communities produced the fewest roots (monoculture mean 212.2 ± 155.1g m−2), and belowground standing crop was not affected by diversity. Root standing crops in year three were 1.8 times higher under low precipitation conditions, while in year five we observed comparable root standing crops between precipitation treatments. Plant family was a strong mediator of increased belowground biomass observed with diversity, with single family grass and aster families generating 1.7 times greater root standing crops in six compared to single species communities, relationships between diversity and aboveground production were consistently observed in both single-family and multiple family communities. Diverse communities with species from multiple families generated only 1.3 times the root standing crop compared to monoculture average root biomass. We surprisingly observe diverse single family communities can generate increases in root standing crops that exceed those generated by diverse multiple family communities, highlighting the importance of plant richness within plant family for a given community. These patterns have potential implications for understanding the interactions of multiple global change drivers as changes in both precipitation and plant community composition do alter whether plant production aboveground is translated belowground biomass.
Introduction
The loss of plant diversity globally is a challenge facing humanity (Rockstrom et al., 2009). Yet, how multiple global change drivers, specifically drought and diversity loss, interact to effect productivity remails poorly understood. The implications of plant diversity loss on aboveground plant productivity are well established (Tilman et al., 1997; Hector et al., 1999; Reich et al., 2012; Tilman et al., 2014; Wagg et al., 2022), and generally exceed drought effects (Rockstrom et al., 2009; Tilman et al., 2014). For example, a reduction in plant species from 16 to 1 can result in a reduction in biomass that is four times greater than effects observed with drought. Yet, these patterns describe aboveground effects, representing only a portion of total plant production. A community’s resistance to and ability to recover from altered precipitation is thought to depend upon plant species richness and composition (van Ruijven and Berendse, 2010; Isbell et al., 2015; Skelton et al., 2015), but again these studies focus heavily on aboveground production. Thus, understanding when aboveground patterns are reflective of those occurring belowground is necessary for understanding the implications of interacting global change drivers, i.e. plant diversity loss and drought.
Diversity effects on root biomass likely depend on the identity of the plants that comprise communities, i.e. defining which plant functional groups are present. Plants utilize different rooting strategies often corresponding with their functional groups and the traits they exhibit to acquire resources. For instance, some plants root deeply, while others invest heavily in shallow root systems (Mueller et al., 2013; Carmona et al., 2021). Some plants utilize their rooting systems to form symbiotic relationships with microbes, exchanging C for access to limiting resources, e.g. phosphorus or nitrogen (Reynolds et al., 2003; Vitousek et al., 2013), while others stimulate the activity of free living rhizosphere microbes or directly scavenge soil resources via root exudation (Phillips et al., 2011; de Vries et al., 2019). Experiments exploring the role of plant species richness in determining belowground biomass suggest plant functional group diversity governs root production increases observed with richness (Gastine et al., 2003; Mueller et al., 2013; Prommer et al., 2020; Furey and Tilman, 2021). For example, synergistic effects on belowground biomass have been observed when communities include C4 grasses and legumes (Fornara et al., 2009; Mueller et al., 2013; Yang et al., 2019). Grasses with C4 photosynthetic strategies often invest heavily in fine roots (Carmona et al., 2021), while legumes are capable of increasing access to nitrogen (N) (Vitousek et al., 2013) by forming symbiotic relationships with rhizobia (Zahran, 2001). However, disentangling the effects of richness and community composition on belowground biomass has been challenging (Gastine et al., 2003; Fornara et al., 2009; Mueller et al., 2013; Yang et al., 2019; Prommer et al., 2020; Furey and Tilman, 2021), as the longest running biodiversity experiments do not independently manipulate both the number of species and plant composition.
Furthermore, how belowground biomass responds to altered precipitation is likely determined by plant community composition, as plant species have different responses to drought and overwatering. Across regional and global scales, belowground production tends to increase with precipitation (Wu et al., 2011; Kang et al., 2013; Du et al., 2020). However, these patterns are inconsistent, and we only sometimes observe in manipulative experiments increased root biomass with precipitation (Xu et al., 2012; Evans and Burke, 2013). In many cases belowground biomass does not change (Sindhøj et al., 2000; Byrne et al., 2013; Flanagan et al., 2013; Denton et al., 2017), or is higher in communities experiencing drought (Wilcox et al., 2016; Slette et al., 2022). Environmental conditions, e.g. depth to the water table (Fan et al., 2017), can alter the rooting strategies of plants. Additionally, plant functional group and community composition may likewise determine these outcomes. For example, the presence of deep-rooted plants can increase soil moisture higher in soil profiles, alleviating stressful conditions for neighbors generating increased belowground production with diversity in systems experiencing drought (Horton and Hart, 1998; Pang et al., 2013). This represents an unexplored potential mediator of plant responses to diversity. Manipulative experiments evaluating potential interactive effects of drought, plant diversity, and community composition on belowground biomass are rare. While few studies have explored the interaction between drought, plant community composition, and diversity on root biomass, it has been observed that less abundant species can generate increased root biomass with drought (Slette et al., 2022). This suggests that community composition could govern belowground biomass responses to diversity with altered precipitation.
While diversity can generate increased belowground biomass, it remains unclear how changes in environmental conditions, such as altered precipitation regimes, and plant community composition effect these outcomes. We sought to determine whether aboveground production benefits from plant diversity are similarly observed in belowground biomass. Specifically, we asked whether plant community composition and precipitation interact with each other and plant diversity to effect root standing crops three and five years after plant establishment. To address this, we established a grassland rainfall and diversity manipulation experiment, that independently alters both plant richness and community composition, by selecting species for mixtures from the same or multiple families. This experimental design can uniquely disentangle the role plant diversity and functional group may independently play in generating benefits from diversity. We might expect aboveground production benefits from richness will be replicated by root biomass belowground, and those patterns will be greatest in families including both legumes and C4 grasses (Fornara et al., 2009; Mueller et al., 2013; Yang et al., 2019). Furthermore, we might expect root biomass will increase with precipitation, as has been observed in meta-analyses across regional and global scales (Wu et al., 2011; Kang et al., 2013; Du et al., 2020), but whether belowground root biomass responses to biodiversity increase with precipitation is unknown.
Methods
Experimental design
In spring 2018, we established an experiment manipulating Rainfall and Diversity (RaD) at the University of Kansas (KU) Field Station (39.05°N, 95.19°W, Jefferson Co., KS) on lands previously cropped and pastured (1870–1970), that since have been left fallow. Prior to 1870, this location was a tallgrass prairie with no recorded woody vegetation (Kettle et al., 2000). After 1970, the site was dominated by cool-season non-native plants (a mix of Tall Fescue and Smooth Brome), before the community transitioned to a C4 prairie grassland (dominated by Sorghastrum nutans and Andropogron gerardii). The experimental site is located at an ecotone transitioning eastern deciduous forest to tallgrass prairies, with a humid subtropical climate receiving 986mm (10th–90th quantile: 792–1197mm) of mean cumulative precipitation and N deposition rates of 9.38kg ha−1 y−1 annually (Wang et al., 2022). The experiment is a full factorial manipulation of precipitation (50% and 150% ambient rainfall), plant species richness (1, 2, 3, 5, and 6 grassland species) and plant community composition (diverse plots containing all asters, legumes, grasses, or multiple families). The experiment utilizes a paired plot design, manipulating precipitation across twelve houses, each of which contain 20 plots that vary in richness and composition in six diversity designs. Each of the twelve shelters contains 20–2.25m2 plots, totaling 240 (Figure 1). This and neighboring experiments are enclosed fencing (3m height) to exclude large herbivores. Efforts are taken to dissuade permanent establishment of small herbivores within the experiment, though they are not deliberately excluded.
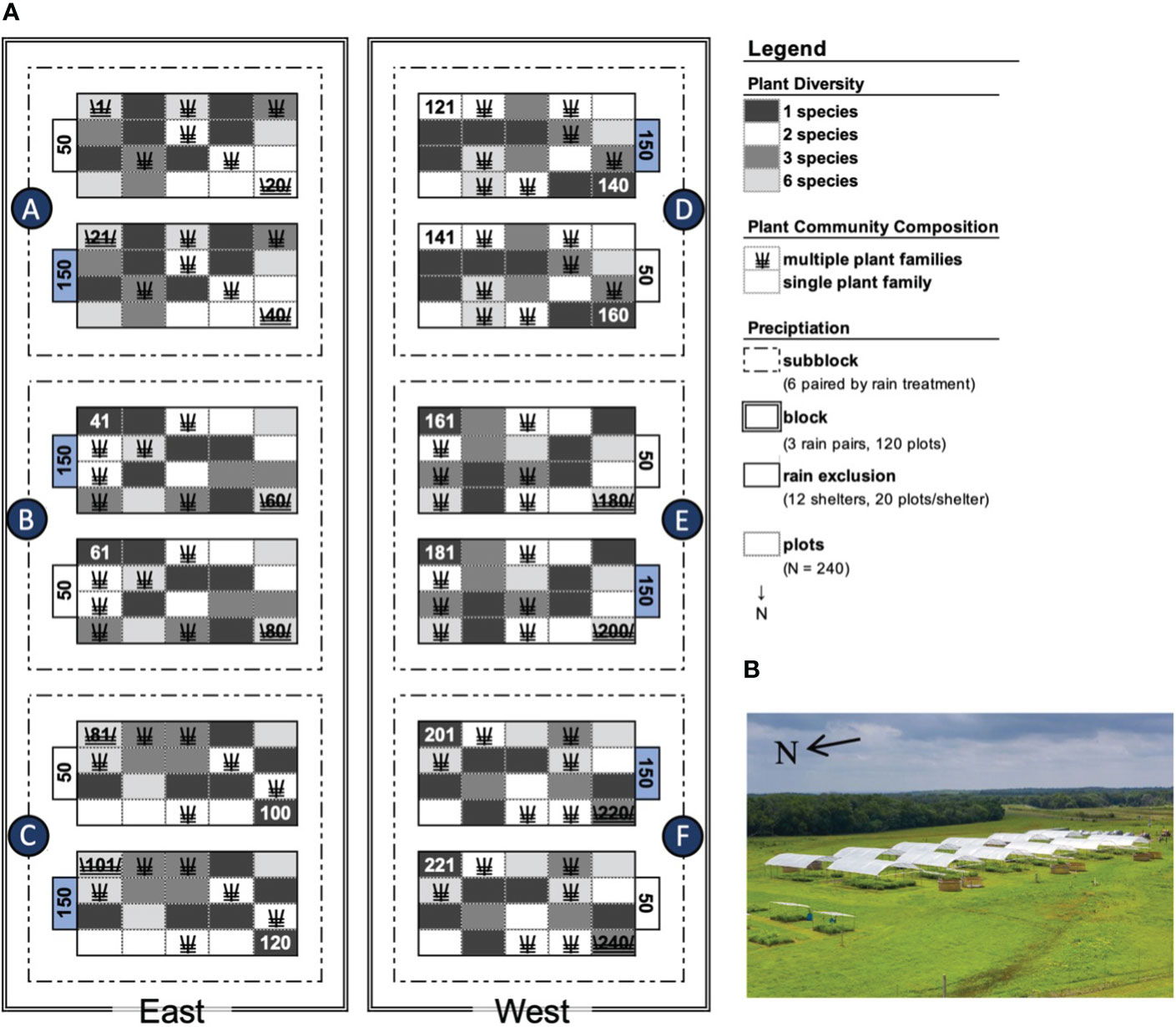
Figure 1 Plot layout and precipitation treatment. (A) Plot layout depicting plant diversity, community composition, and precipitation treatments. Species richness levels 1, 2, 3, and 6 are depicted in dark grey, white, medium, and light grey respectively. Multiple family mixtures are indicated with three lines crossed, while single family mixtures are open rectangles. Rain shelters receiving high and low watering are designated with a blue 150 and open 50. Subblocks are outlined with dot-dashed lines and a circle lettered A–F. The east and west blocks are outlined with double lines. (B) Overhead view of the RaD experiment.
To accelerate plant establishment, we added whole native tallgrass prairie soil to inoculate plots with soil microbiomes associated with established prairies. Whole prairie soil (Welda soil) was collected in January 2018 from The Nature Conservancy’s Anderson County Prairie Preserve near Welda, KS (Anderson Co., KS, 38°11’00’’N, 95°15’39’’W), from prairie designated for destruction as part of a road expansion project in the southeast corner of Anderson County Prairie Preserve Management Unit 10. That management unit is considered native prairie and was formally called Nichols Meadow. To collect Welda soils, we first removed the top 5–7cm from a 16.75m × 45.72m section adjacent highway U.S.169. The subsequent 15cm was tilled until the soils were fully homogenized before they were collected for use in the plots. Welda soils are classified by the National Resource Conservation Service (NRCS) as Kenoma-Olpe complex, which have a silty loam texture (USDA-NRCS, 2019). Our sampling procedure would have captured only soils from the A horizon, which extend to a 25.4cm depth in Kenoma-Olpe soils. RaD plots were first tilled to a depth of 15cm. We then added 4cm Welda soil (75.1L) to the center of the plots and racked Welda soils into the surface to an extent of 2m × 2m, exceeding the dimensions of the 1.5m × 1.5m plots with the goal to reduce edge effects. The plots were then tilled again to 15cm to homogenize field station soils with the Welda soil amendments. RaD soils are classified as Grundy silty clay loam (USDA-NRCS, 2019), with an Ap horizon extending to a depth of 15cm and a silty clay loam texture. Below Ap horizons, Grundy silty clay loam soils have a BA horizon, extending to a depth of 28cm which are also classified as silty clay loam in texture.
The species pool for the plant communities included 18 species, six species each from three families commonly represented in tallgrass prairies: Asteraceae, Fabaceae, and Poaceae (asters, legumes, and grasses; Supplementary Table S1). Plots include 1, 2, 3, and 6 species to alter planted richness (Supplementary Table S2). The experimental design represented each species equally in all treatment combinations. However, by year three one species of grass had not established (Koeleria macrantha), so realized richness (1, 2, 3, 5, or 6) is presented (Supplementary Table S3). All individual species used in this study are native to tallgrass prairies and likely to be observed in prairie remnants. Individual species were chosen to represent the range of life histories of species from these three families, from short-lived annuals to slow, long-lived perennial species, with the additional constraint of availability and viability of seed. In the first year, 18 plants were inoculated with native prairie soil, grown in the greenhouse, and then planted into the plots in equal proportion within a hexagonal array (Supplementary Figure S1). Additionally, the plots were seeded in equal proportion, which has continued in subsequent years. All species beside K. macrantha have persisted in the experiment.
To alter plant community composition, in 2–6 species mixtures, we selected species from the same or differing families, with plots containing all asters, legumes, grasses, or multiple families (Supplementary Table S2). In this experimental design, plant community composition can be evaluated at two levels, by comparing the performance of multiple and single-family communities (two-factor levels) or considering the performance of each single-family mixture distinctly (four-factor levels). The three families selected, are both the most common plant families in prairies, and map onto commonly conceived functional groups (grasses, legumes, forbs), providing the additional benefit of allowing tests of plant functional group diversity influence on belowground production. Each year at the end of the growing season (November) annual aboveground growth is clipped approximately 5cm from the ground and all clipped biomass is removed prior to the next year’s seed addition.
The plant diversity treatments described above were established in paired shelters that receive different water treatments, with one receiving 150% and the other receiving 50% ambient growing season rainfall (Figure 1; Supplementary Figure S2). In spring 2018, large rainfall exclusion shelters were constructed 9.14m × 10.97m and 3.66m tall to facilitate airflow (Supplementary Figure S1). These rainfall manipulation shelters collect ambient rainfall that is stored in tanks, and the water is then re-applied using a hanging rotating sprinkler system chosen to mimic rainfall. Both shelters excluded and reapply rainfall to ensure the plots are experiencing similar microclimatic conditions created by the structures (Supplementary Figure S2). Water tanks are sometimes supplemented with water from onsite Reservoir 2 when levels are low, e.g. the beginning of the season or when water levels near levels that would shut off the pump emergency stop mechanism; the reservoir’s water source is ambient precipitation. Reservoir 2 was sampled in 2018 and found to have relative low nutrient status, TN approximately 750µg L−1 and TP 25µg L−1, as well as low total suspended solids, <10 FNU (Ted Harris, personal communications). Since windy conditions can cause inefficiencies, the irrigation system is calibrated multiple times within a year for each shelter by placing 12 collection cups in the aisle junctions, running the irrigation system for at least 10 minutes, and collecting the total volume across an entire shelter (Supplementary Figure S1). The average irrigation rate is calculated among shelters and over experimental years to account for variance in weather conditions, which to date is 0.2514 ± 0.0521mm min−1. This value is used to calculate irrigation times. The gravimetric soil moisture (%) was measured to check soil moisture differences between treatments at the end of the growing season soil sampling. In the 50% and 150% treatments soil moisture was on average 20.04 ± 4.08 and 25.97 ± 3.75 in 2020, and 17.15 ± 3.89 and 20.82 ± 5.41 in 2022. The high watering treatments received 827.8mm and 1036.5mm of growing season irrigation in 2020 and 2022, while the low treatments received 272.0mm and 345.6mm of irrigation, respectively (Supplementary Table S4).
Sample collection and processing
To quantify aboveground production, in 2020 and 2022 at peak biomass, we observed planted cover and harvested biomass strips from the 0.1m2 of the plot. Biomass strips were collected from 0.1m × 1m strip using Ryobi grass shears. To determine the collection location a 1m × 1m quadrat, avoiding the edge, was placed in the center of the plot, and was divided into ten segments labeled sequentially from east to west. Segments are harvested in order skipping one segment to minimize annual disturbance. Biomass is collected and if it could not be sorted that day it is stored at 4°C for no more than 48 hours. Plants are then sorted and identified. Sorted plants are dried at 70°C for five days and massed. Plant cover is assessed concurrent with biomass harvests, observing the percent cover of all planted species within the plot with observations including all plants to the edge of the plot.
Planted cover and biomass from strips were correlated (Supplementary Figure S3; Pearson’s r > 0.76) across all plots. We then used species-specific correlations to scale biomass data to the plot level. This approach allows us to project total plot biomass while minimizing disturbance to our plots. Our plots are sufficiently sized to capture plant species interactions (Roscher et al., 2005). However, annual mid-season full-plot harvests could damage the long-term viability of diversity treatments. To limit this disturbance, we used species-specific relationships between cover to biomass, obtained by regressing species’ biomass in the strip against that species’ plot cover, to convert cover estimates to biomass estimates, which are scaled to one square meter (multiplying by 10). As the biomass strip only represents 0.1m2 of a 2.25m2 plot and an individual strip will not represent each species in proportion to its total coverage (histogram are zero inflated in Supplementary Figure S3), there is noise in the individual regression relationships. However, we are able to get statistically strong, robust, and reproducible relationships across all 240 plots (Supplementary Figure S3; Pearson’s r > 0.76). Importantly, the noise in cover-biomass relationships for individual species is not the noise in plot level biomass estimates. This approach may result in a reduction in the precision for our measurements of aboveground biomass compared a total plot harvest, but this imprecision is unbiased with respect to our treatments.
To quantify belowground root biomass, we collected root standing crops from bulk soil cores harvested in September 2020 and 2022, three and five years after experimental establishment. At both time points, two soil cores were collected to a depth of 20cm from each plot (Fornara et al., 2009; Lange et al., 2015; Yang et al., 2019), composited, and homogenized by hand in the field, before being transported on ice to the lab, where samples were stored at 4°C. The practice of hand homogenizing soils is commonly used on soils with fine textures and has been done on samples collected from areas near the location of RaD (Billings, 2006). In 2020, cores were taken using 1.905cm diameter step probe corers. In 2022, as the soils in 50% precipitation treatments became increasingly denser, we opted to use slide hammer corers, 2.54cm in diameter, which was the smallest size available, and could facilitate collection of dry soils. In both years, we thoroughly picked roots from each composited soil sample (Craig et al., 2015), either until all visible roots were removed or for 20 minutes to standardize efforts. We removed roots by thoroughly picking them from the sample rather than sieving because the majority of roots were fine in size class (<2mm) and would have passed easily through a standard sieve. Furthermore, our soils have a relatively fine texture and do not pass easily through a sieve. Since the soils collected from the cores are also used for other analyses and archived, we do not wet sieve the samples in order to preserve their physical and chemical integrity. Root biomass was then, oven dried at 70°C for three days, and then weighed (Craig et al., 2015). Root standing crop is presented on a per area basis (g m−2) to facilitate comparison with aboveground production and account for core size differences between years. Though it should be noted, for aboveground biomass we remove senesced materials annually, while for belowground root biomass samples we cannot distinguish between live or dead material, nor can we disentangle how much growth is new. We also quantify on a plot level the combined aboveground production and belowground root biomass as the sum of aboveground and belowground biomass, though the differences in measures noted above apply.
Data analysis
To explore how belowground biomass, aboveground production, and combined biomass changes with plant diversity, community composition, and precipitation, we fit linear mixed effect models to our data. To simplify potentially overfit models, we used the model selection function, ‘glmulti’ package in R v. 4.2.2 (Calcagno et al., 2020; R Core Team, 2022). The parameters surveyed by the model selection process could include any of our experimental treatments, plant diversity, community composition, and precipitation, with year and all potential interactions as potential predictors. We included plot, subblocks, and watering nested in subblock as random effects to account for non-independence of repeated sampling and of plots nested within the same shelter. ‘Glmulti’ generates all potential combinations of models, generating a suite ranked by Akaike’s Information Criterion (AIC). Candidate models are considered those that are statistically indistinguishable from the model with the lowest AIC (delta AIC < 2). Weighted model averages (Burnham and Anderson, 2004) were included to compare the likelihood that a parameter was predicting root standing crops. We present all significant candidate model parameters for root standing crop and the model with the highest likelihood of explaining patterns in aboveground biomass. We performed the model simplification process including plant community composition with two-factor levels (single family, multiple families) and four factor levels (only grass, only legumes, only asters, multiple families), then use AIC values to determine whether designating the family that makes up the community improves our ability to describe patterns in yields. We present all models and consider the model with the lowest AIC as the best simplification of our experimental treatments. Root, aboveground, and combined biomass were log10 transformed to improve normality of residuals. Models were fit in R v. 4.2.2 (R Core Team, 2022)., using the packages ‘lme4’ and ‘lmerTest’ packages (Bates et al., 2015; Kuznetsova et al., 2017) and visualized using the ‘visreg’ package (Breheny and Burchett, 2017).
Results
Root, aboveground, and combined aboveground and belowground biomass increased with plant diversity overall for both years (Figure 2; Supplementary Figure S4). Plant richness significantly increased aboveground production (Figure 2B; Table 1), and the magnitude of the relationship significantly strengthened with time (Figure 3; Table 1). Six species mixtures generated 255% more aboveground biomass than monocultures (Figure 2B). Similarly, we observed a significant positive relationship between plant species richness and root standing crop (Figure 2C; Table 2). Root standing crop increased 132% when species richness increased from one to six, and 232% from one to five species. Notably, the highest diversity level of single-family grass communities has five species and are not represented in six species richness level. Root, aboveground, and combined above and belowground biomass increased over time (Table 1). While an interaction between richness and year was present in two of the candidate models explaining root standing crop (Table 2) and in five out of the seven explaining combined above and belowground plant biomass (Supplementary Table S5), the interaction was not significant in either model (Table 2).
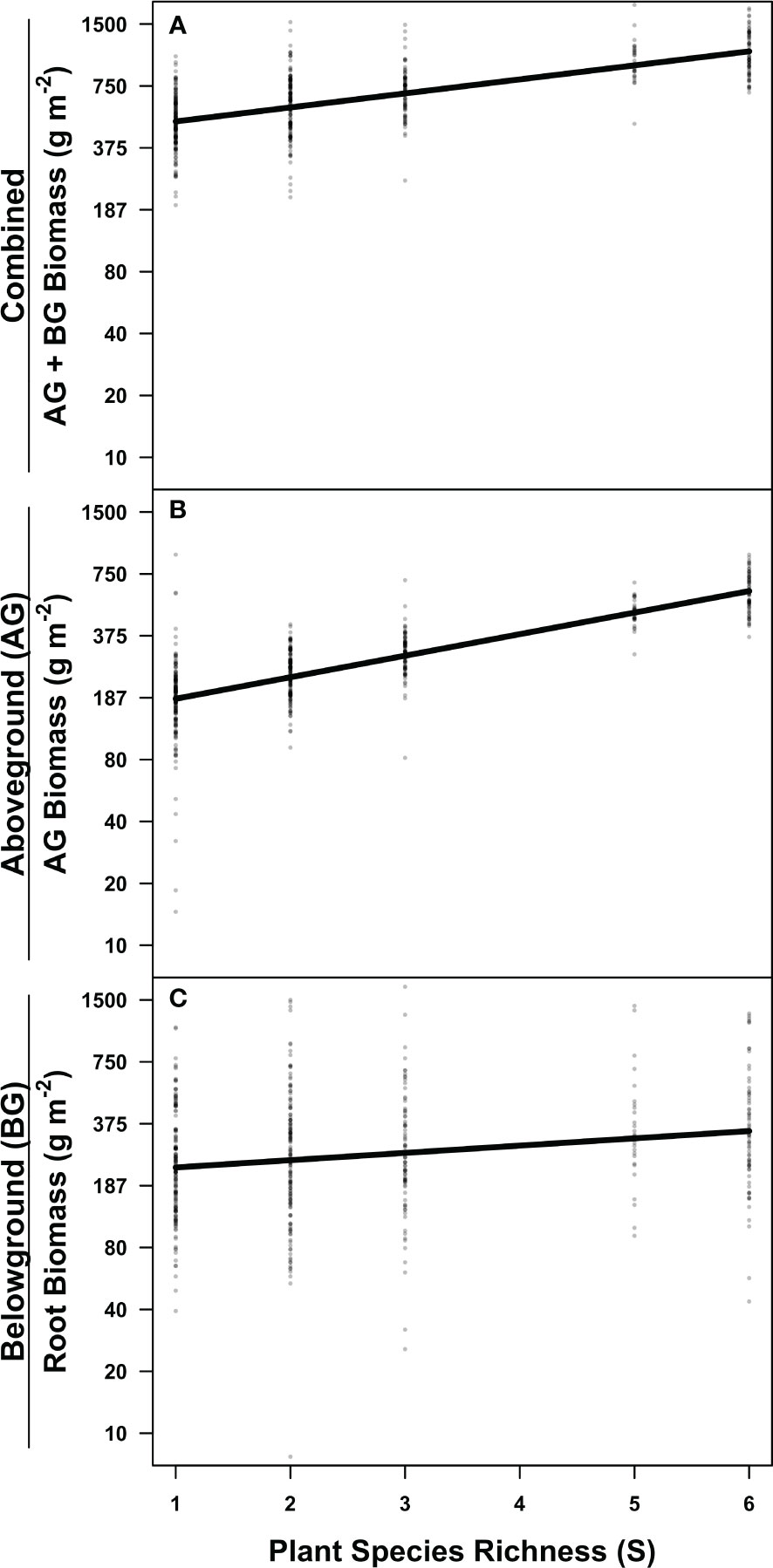
Figure 2 The relationship between productivity and plant diversity. Partial residual plots depicting the relationships between richness and (A) total, (B) aboveground, and (C) belowground plant biomass. Partial residual plots visualize linear mixed effects models while holding all other parameters constant. Statistical outputs can be found in Table 1. Note the log scale on the y-axis. N = 472.
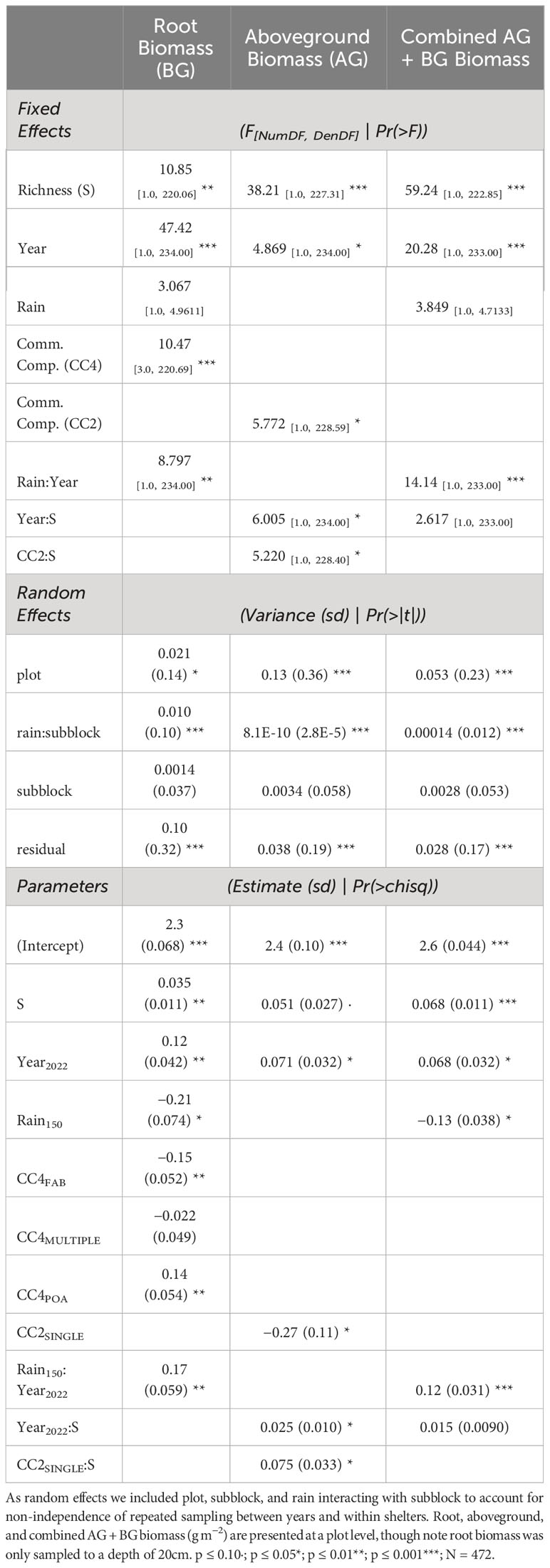
Table 1 Linear mixed effect model outputs predicting root, aboveground, and combined above and belowground biomass.
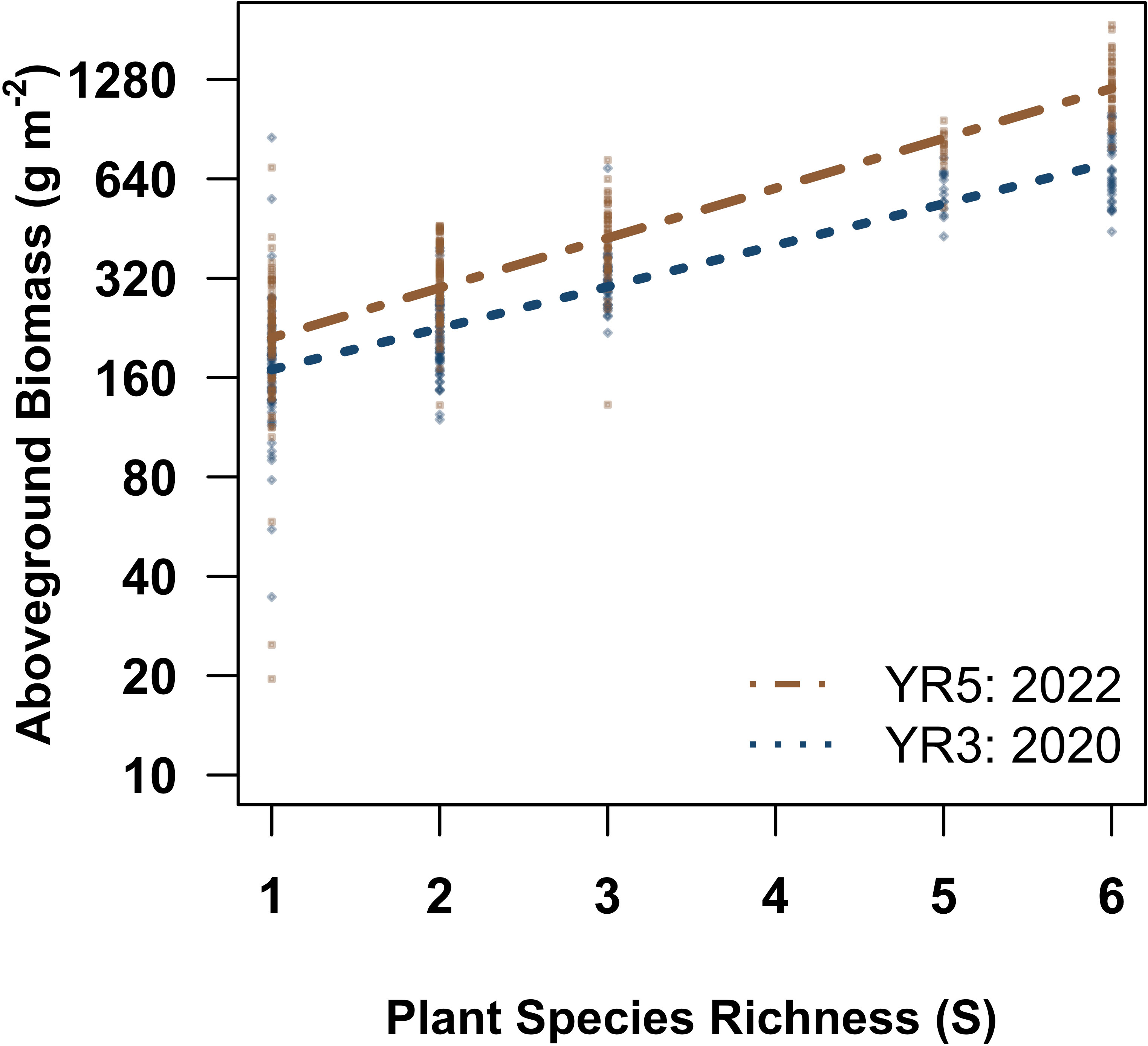
Figure 3 The relationships between aboveground biomass and richness by year. Partial residual plot visualizing the relationship between aboveground biomass and richness by year, Year five, 2022 is indicated in orange and dot dashed lines, while year three, 2020, is in blue and dotted lines. Partial residual plots visualize linear mixed effects models while holding all other parameters constant. Statistical outputs can be found in Table 1. N = 472.
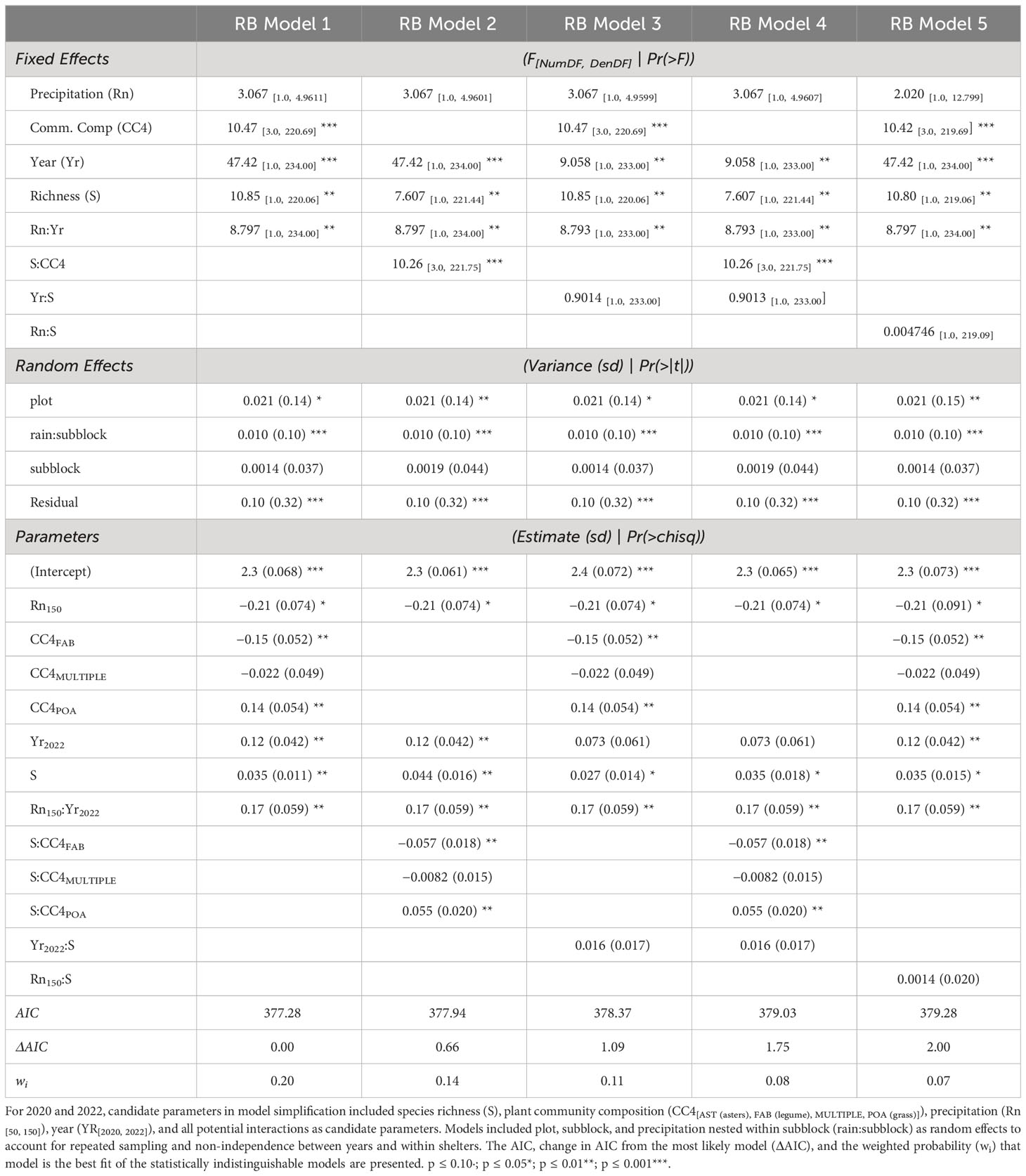
Table 2 Statistically indistinguishable models (ΔAIC ≤ 2) predicting root biomass (RB) generated from model simplification.
Plant family identity was a strong determinant of root standing crops and moderated the magnitude of the relationship between root standing crop and richness (Figure 4B; Supplementary Table S5). In three of the five candidate models explaining root standing crop, a significant main effect of plant family designations (CC4) was present (Table 2). We observed higher mean root standing crop in grass (monoculture mean 463.9 ± 410.3g m−2), lower in legume (monoculture mean 212.2 ± 155.1g m−2), and mid-level in communities comprised of all asters (monoculture mean 332.9 ± 255.6g m−2), and across richness levels average differences between families held and significantly differed (Table 2; Supplementary Figure S5; Supplementary Table S5). In the other two models, a significant interaction between plant community composition and richness was present (Table 2). Here we observed a strong positive relationship between root standing crop and richness in communities composed of all grasses, a weak positive relationship for communities composed from multiple families and all asters and a weakly negative relationship in communities composed of all legumes (Figure 4B; Table 1). We observed a 173%, 136%, and 167%, increase in root standing crops from one to six species for asters, legumes, and grass communities respectively. Though the slope was steepest in grass communities, the percent increase in standing crop was highest in aster communities because root standing crops in grass monocultures were on average high compared aster and legume monocultures. We observed the lowest percent increase from one to six species in multiple family communities, 129%, which were compared to the average root standing crop of all monocultures (Supplementary Table S6).
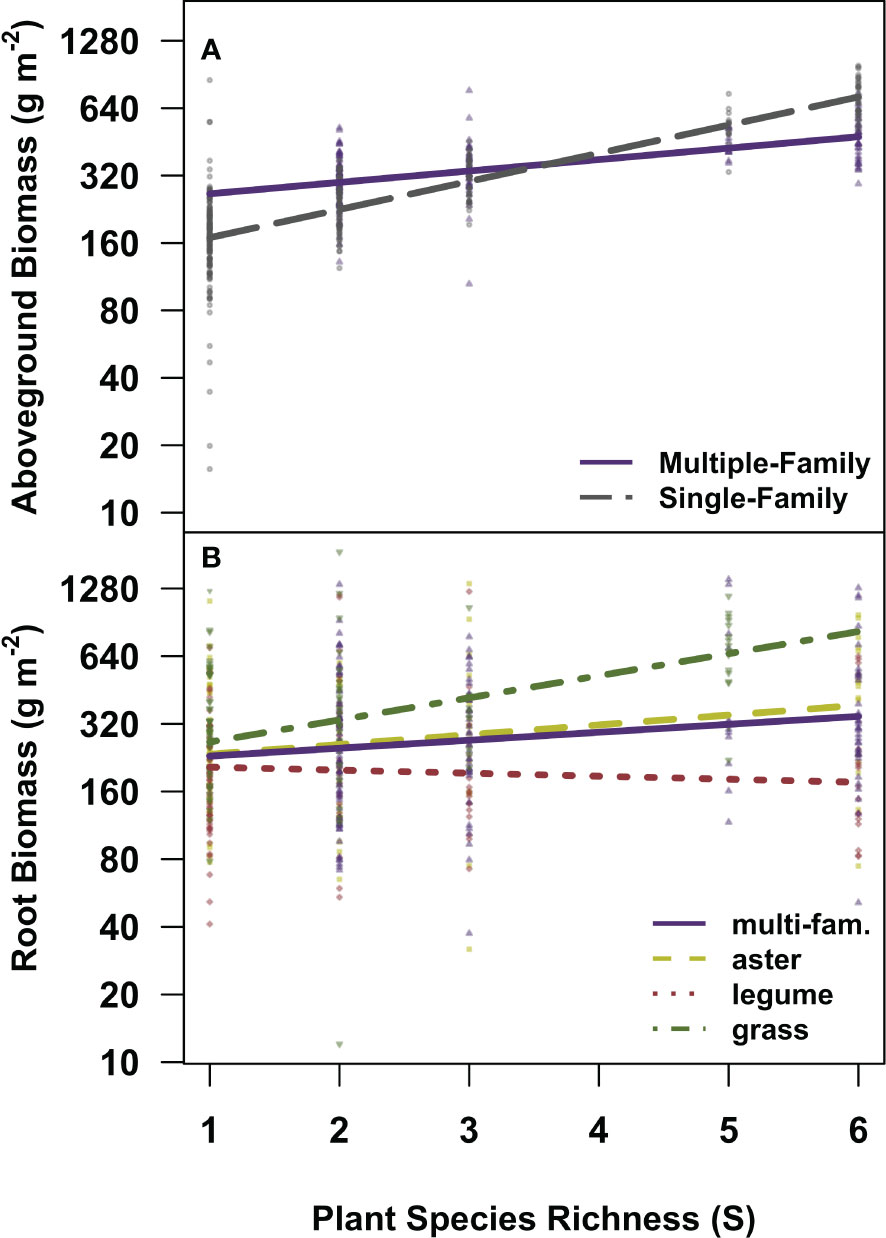
Figure 4 The relationships between above and belowground biomass by richness, and plant community composition. Partial residual plots visualizing the relationship between richness and (A) aboveground biomass by plant community composition (multiple-family in a purple solid line and single-family in grey dashed line), and (B) root biomass and plant community composition. In panel (B), multiple family communities are indicated in solid purple, asters in yellow dashed, legumes in red dotted, and grasses in green dot-dashed lines. Partial residual plots visualize linear mixed effects models while holding all other parameters constant. Statistical outputs can be found in Tables 1 and 2 (RB Model 2). Note the log scale on the y-axis. N = 472.
In contrast with root standing crops, plant family diversity (single vs multiple), but not plant family identity (grasses vs legumes vs asters), moderates aboveground production (Supplementary Tables S5, S7, S8). We observed a significant main effect of plant community composition with two levels (CC2) on aboveground biomass (Table 1). The relationship between plant richness and aboveground biomass was steeper in single family communities in comparison to multiple family communities (Figure 4A; Supplementary Table S7 see the CC2 SINGLE:S). Plant community composition was not in the most probable model predicting combined above and belowground production (Supplementary Tables S5, S9, S10). We observed a weak relationship between plant community composition with two levels (CC2) and richness in the candidate models predicting combined above and belowground biomass replicating the pattern observed in aboveground biomass (Supplementary Table S9; Supplementary Figure S6).
The effect of precipitation on root standing crop changed with time, while the precipitation treatment did not predict changes in aboveground production (Table 1). Root standing crop significantly increased overtime from 2020 to 2022 by 1.4-fold, three and five years after initial establishment (Figure 5; Table 2; Supplementary Table S11). In all candidate models, we observed a significant interaction between year and precipitation treatment (Table 2). In 2020 root biomass was significantly higher in 50% precipitation treatments generating 179% greater root standing crops than high water treatments (Supplementary Table S11). In 2022, we observed no difference between mean root standing crop in high and low precipitation (Figure 5). While rain was in models predicting aboveground production, it did not significantly affect aboveground biomass (Supplementary Tables S7, S8). The interaction between year and precipitation on combined above and belowground biomass replicated patterns observed in belowground root biomass (Supplementary Figure S8; Supplementary Tables S9, S10).
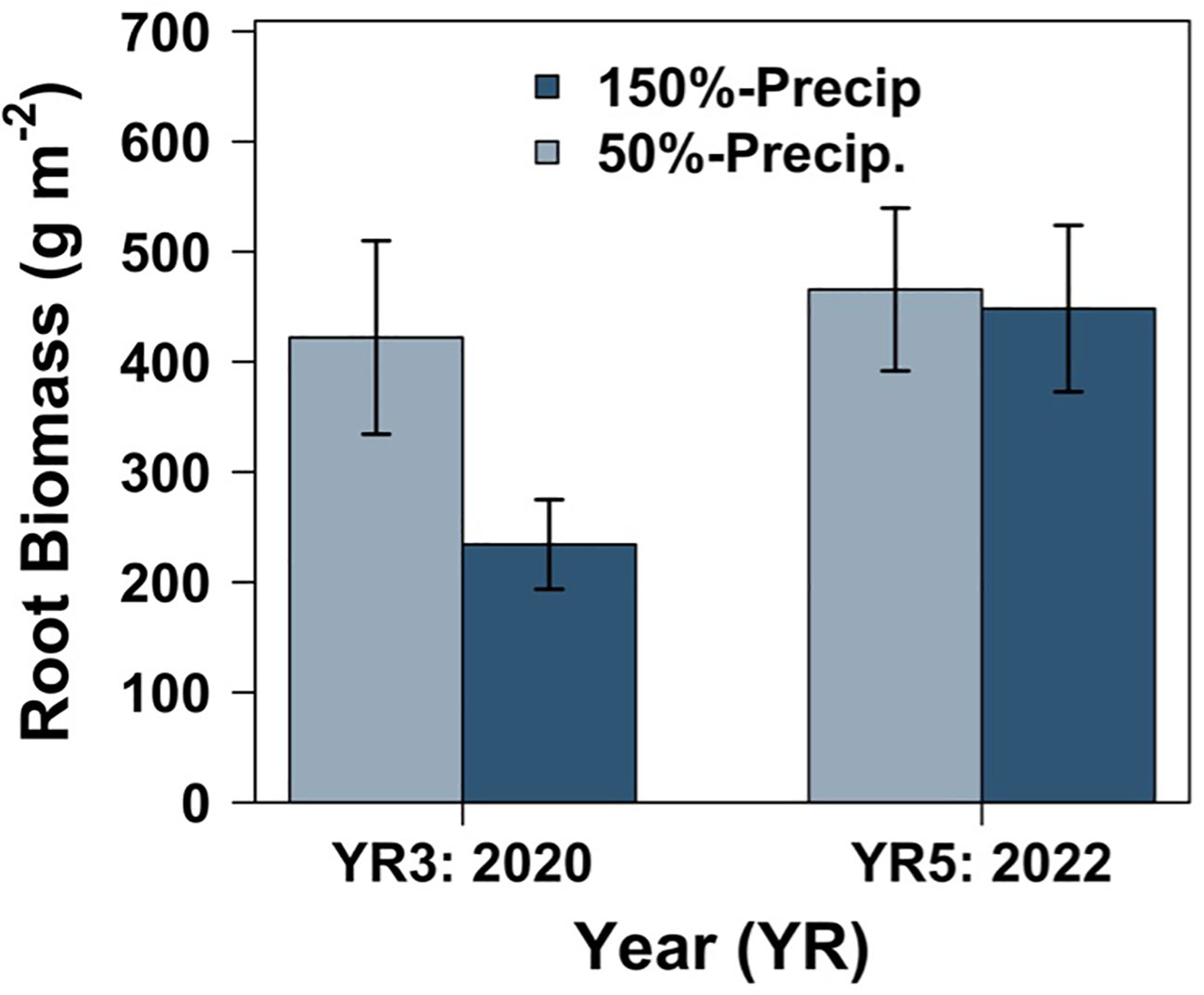
Figure 5 The relationship between root biomass and precipitation. Bar plot of mean root biomass by year with the standard error. High watering (150%-precip.) is colored in dark blue and low watering (50%-precip.) is colored in light blue. Note the differences in scale on the y-axes. N = 472.
Discussion
While it is well-established that plant diversity generates increased aboveground productivity (Tilman et al., 1997; Hector et al., 1999; Reich et al., 2012; Wagg et al., 2022), whether these patterns are replicated belowground is less certain. Moreover, whether plant diversity increases belowground biomass is dependent on plant community composition and precipitation, both of which are anticipated to change in the Anthropocene (Knapp et al., 2002; Nippert et al., 2006; Zeppel et al., 2014; Lee et al., 2021), and has broader consequences under future climate change scenarios. This critical gap needs to be resolved to understand how interactive effects of climate change and biodiversity loss, both significant global change drivers themselves (Rockstrom et al., 2009; Tilman et al., 2014), will affect total plant production with cascading effects on biodiversity and soil ecosystem function (Guerra et al., 2020). In a rainfall and grassland diversity manipulation experiment we observed strong evidence of plant diversity benefits on root standing crops (Figure 2C). Diversity benefits for root standing crops were greatest in communities composed of grasses and weakest in communities composed of all legumes (Figure 4B). We did not, however, observe increases in belowground standing crops when plots included both legumes and C4 grasses in diverse communities, and instead our diverse multiple-family mixtures underperformed mixtures with only grasses. Consistent with our expectations, plant community composition had a strong influence on belowground standing crops (Figure 4B) and root biomass increased in the plots over time (Figure 5). In year three, 2020, we observed higher root standing crops in low compared to high water treatments, while those differences dissipated two years later as root biomass increased in high precipitation treatments to levels comparable to 50% precipitation treatments. This contrasted with aboveground patterns, and our expectations, that increased precipitation generated greater yields.
Effects of precipitation on belowground root biomass changed over time. Generally, across regional to global scales root biomass increases with precipitation (Wu et al., 2011; Kang et al., 2013; Du et al., 2020). While we expected our findings to reflect this, as has been confirmed in some rainfall manipulation experiments (Evans and Burke, 2013; Xu et al., 2013), we observed patterns inconsistent with these expectations (Figure 5). In some manipulative experiments no change in root biomass is observed between precipitation treatments (Sindhøj et al., 2000; Gill et al., 2002; Byrne et al., 2013; Flanagan et al., 2013; Denton et al., 2017), which is consistent with our 2022 results. In other instances, belowground biomass increases with low watering (Wilcox et al., 2016; Slette et al., 2022), consistent with our 2020 results. We suspect time may influence the relationship between belowground root biomass and precipitation. One study found that six years after establishment, plants in a water-limited grasslands had vertically redistributed their roots to optimize water uptake (Zhang et al., 2019). After exposure to reduced precipitation, root biomass redistributed to 10–30cm and 30–50cm depths, while in high precipitation treatments more root biomass was found only at 0–10cm depths. Root redistribution may explain why we observed strong differences between drought in year three that are no longer detectable in year five. Our samples are composites of 0–20cm depths, which could mask the differences between precipitation treatments over time as roots redistribute in response to long term exposure to altered precipitation. Though notably 80% of tallgrass prairie root biomass can be found in the top 25cm of soils (Nippert and Knapp, 2007), suggesting our sampling approach likely still captured the majority of roots.
Plant community composition may likewise play a role in how root standing crops respond to changes in precipitation. For instance one study found in comparison to the most abundant C4 grass which generated similar root biomass in high and low water treatments, subdominant plants drove the overall increase in belowground biomass observed under drought, a pattern that was consistent across 0–10, 10–20, and 20–30cm depths (Slette et al., 2022). Though we did not observe an interaction between plant community composition and precipitation, it is possible these patterns will develop over time as plants change their rooting strategies in response to long-term exposure to altered precipitation.
Plant community composition was a strong determinant of belowground root biomass, while aboveground production consistently increased with diversity driving combined above and belowground biomass benefits associated with increasing diversity. A few caveats must be acknowledged combing these measures. First, where each year aboveground biomass is removed prior to the growing season, our sampling efforts belowground are unable to distinguish between live and dead roots, nor are they able to remove the previous year’s (or years’) growth. Thus, our measures aboveground represent annual growth, production, while belowground biomass represent a standing crop. Despite these discrepancies in method that are difficult to avoid within the context of the experimental design, combined the patterns still provide some insights into how plant biomass above and belowground together responds to changes in diversity precipitation. Second, combined above and belowground biomass might underestimate the contribution of deep-rooted plants to production, as roots were only sampled to 20cm. Given the sampling limitations, belowground patterns might be underrepresented in combined above and belowground biomass measures.
It is notable that communities containing all grasses had the highest belowground biomass, and their diversity had the greatest positive effect on root biomass, while legume diversity did not generate increases in belowground standing crops (Figure 4B). This could reflect well established trait differences between C4 grasses and legumes. Warm season C4 grasses, which characterizes most grass species in this experiment, invest heavily in the production of shallowly distributed fine roots. Legumes, in contrast, tend to root deeply, investing fewer resources into the generation of fine roots (Gastine et al., 2003; Prommer et al., 2020; Carmona et al., 2021; Furey and Tilman, 2021). We, unlike previous studies, observed no benefits of having both shallow and deep rooting strategies in a single community (Figure 4B). Instead, our multiple family mixtures represent essentially the average of the pool of species in the experiment, a pattern consistent with other plant diversity manipulation experiments sampled less than five years after establishment (Gastine et al., 2003), and thus in early stages of community development.
Importantly, in experiments sampled more than ten years after establishment, mixtures containing both grasses and legumes generated increased root yields (Mueller et al., 2013; Yang et al., 2019), and in some cases generated root productivity benefits in mixtures that were greater than expectations based on belowground monoculture yields (Fornara et al., 2009). This may reflect a delay in the accrual of belowground biomass from slow growing plants or it may likewise reflect delayed benefits associated with plants capable of forming symbioses with N fixers included in a community. Resource benefits may take time to accrue in plant communities as the initial disturbance required to setup the experiment may have resulted in a loss of accessible N and many plant diversity manipulation experiments are established on N deplete post-agricultural soils (Tilman et al., 1997; Hector et al., 1999). The reestablishment of N may develop in these systems over longer time periods, decadal scales, as N needs to accumulate in plant and microbial tissues and be decomposed to become available for plant use. These patterns could likewise be depth dependent, as the most diverse and productive plant communities had the deepest distribution of roots. This pattern has been attributed to the cooccurrence of C4 grasses and legumes, which have differing rooting strategies from each other in terms of rooting depths and growth form (Mueller et al., 2013). It is possible by sampling only to 20cm we did not capture the range necessary to see the effects of these differing rooting strategies especially as communities develop, though this depth range is commonly used in grassland diversity experiments (Fornara et al., 2009; Lange et al., 2015; Yang et al., 2019).
Benefits from diversity developed belowground three to five years after establishment. These patterns are consistent with other findings suggesting belowground patterns replicate increases in biomass observed aboveground (Mueller et al., 2013; Ravenek et al., 2014; Eisenhauer et al., 2017; Yang et al., 2019). The timing of the emergence of belowground biomass increases observed with diversity was consistent with a similar grassland diversity manipulation experiment (Ravenek et al., 2014). The patterns observed in our study and by Ravenek, Bessler (Ravenek et al., 2014) emerged perhaps slightly faster than those observed by others (Gastine et al., 2003), though in the latter case the underperformance of a typically dominant plant drove those patterns, which may reflect a range of factors including unfavorable environmental conditions for that species. Diverse mixtures (16 species) can generate more roots than the best performing monocultures, suggesting belowground overyielding can develop (Fornara et al., 2009; Yang et al., 2019), though this pattern has not emerged in our experiment. However, the positive relationship we observed between richness and root standing crops suggests we might expect to see strong changes in microbial community composition and function with increased plant diversity five years after planting, as root inputs have been directly linked to increased microbial activity (Eisenhauer et al., 2017). Indeed, changes in soil microbiome composition in response to plant diversity and family composition have been observed in our experiment (Burrill et al., 2023). Since root inputs are more likely to form stable and persistent forms of soil C, these patterns could represent a more direct link between plant productivity and C sequestration.
These patterns confirm that aboveground production increases associated with diversity can generally be replicated in belowground biomass. However, in contrast with aboveground patterns where productivity–diversity relationships are found consistently across different community composition types, belowground whether root standing crops increased with richness is strongly dependent on plant community composition. Perhaps most surprisingly diversity within a single family can both rival (aboveground) and exceed (belowground) biomass increases observed in diverse multiple-family communities. Furthermore, whether belowground biomass increased with diversity occurred independent of altered precipitation regimes. Overall, these patterns clarify some of the biological (i.e. plant community composition) and environmental (i.e. precipitation) conditions under which diversity is associated with increased biomass, further disentangling when patterns aboveground production are linked with belowground biomass.
Data availability statement
The raw data supporting the conclusions of this article will be made available by the authors, without undue reservation.
Author contributions
LP: Conceptualization, Data curation, Formal analysis, Funding acquisition, Investigation, Methodology, Project administration, Resources, Software, Supervision, Visualization, Writing – original draft, Writing – review & editing. MH: Data curation, Formal analysis, Investigation, Methodology, Writing – original draft, Writing – review & editing. JB: Conceptualization, Formal analysis, Funding acquisition, Methodology, Project administration, Resources, Supervision, Writing – review & editing.
Funding
The author(s) declare financial support was received for the research, authorship, and/or publication of this article. Funding was provided by National Science Foundation (NSF) grants (DEB-1738041, BII 2120153) and the United State Department of Agriculture National Institute of Food and Agriculture (USDA-NIFA) grant (AFRI-EWD 1022923, 1030634).
Conflict of interest
The authors declare that the research was conducted in the absence of any commercial or financial relationships that could be construed as a potential conflict of interest.
Publisher’s note
All claims expressed in this article are solely those of the authors and do not necessarily represent those of their affiliated organizations, or those of the publisher, the editors and the reviewers. Any product that may be evaluated in this article, or claim that may be made by its manufacturer, is not guaranteed or endorsed by the publisher.
Supplementary material
The Supplementary Material for this article can be found online at: https://www.frontiersin.org/articles/10.3389/fevo.2023.1259809/full#supplementary-material
References
Bates D., Mächler M., Bolker B., Walker S. (2015). Fitting linear mixed-effects models using lme4. J. Stat. Software 67 (1), 1–48.
Billings S. A. (2006). Soil organic matter dynamics and land use change at a grassland/forest ecotone. Soil Biol. Biochem. 38 (9), 2934–2943. doi: 10.1016/j.soilbio.2006.05.004
Breheny P., Burchett W. (2017). Visualization of regression models using visreg. R J. 9 (2), 56–71. doi: 10.32614/RJ-2017-046
Burnham K. P., Anderson D. R. (2004). Multimodel inference: understanding AIC and BIC in model selection. Sociol. Methods Res. 33 (2), 261–304. doi: 10.1177/0049124104268644
Burrill H., Wang G., Bever J. (2023). Rapid differentiation of soil and root microbiomes in response to plant composition and biodiversity in the field. ISME Commun. 3 (1), 1–13, p.31. doi: 10.1038/s43705-023-00237-5
Byrne K. M., Lauenroth W. K., Adler P. B. (2013). Contrasting effects of precipitation manipulations on production in two sites within the central grassland region, USA. Ecosystems 16 (6), 1039–1051. doi: 10.1007/s10021-013-9666-z
Calcagno V., de Mazancourt C. (2010). glmulti: An R Package for Easy Automated Model Selection with (Generalized) Linear Models. Journal of Statistical Software 34(12), 1–29. doi: 10.18637/jss.v034.i12
Carmona C. P., Bueno C. G., Toussaint A., Trager S., Diaz S., Moora M., et al. (2021). Fine-root traits in the global spectrum of plant form and function. Nature 597 (7878), 683–68+. doi: 10.1038/s41586-021-03871-y
Craig M. E., Pearson S. M., Fraterrigo J. M. (2015). Grass invasion effects on forest soil carbon depend on landscape-level land use patterns. Ecology 96 (8), 2265–2279. doi: 10.1890/14-1770.1
Denton E. M., Dietrich J. D., Smith M. D., Knapp A. K. (2017). Drought timing differentially affects above- and belowground productivity in a mesic grassland. Plant Ecol. 218 (3), 317–328. doi: 10.1007/s11258-016-0690-x
de Vries F. T., Williams A., Stringer F., Willcocks R., McEwing R., Langridge H., et al. (2019). Changes in root-exudate-induced respiration reveal a novel mechanism through which drought affects ecosystem carbon cycling. New Phytologist. 224 (1), 132–145. doi: 10.1111/nph.16001
Du Y., Wang Y. P., Su F. L., Jiang J., Wang C., Yu M. X., et al. (2020). The response of soil respiration to precipitation change is asymmetric and differs between grasslands and forests. Global Change Biol. 26 (10), 6015–6024. doi: 10.1111/gcb.15270
Eisenhauer N., Lanoue A., Strecker T., Scheu S., Steinauer K., Thakur M. P., et al. (2017). Root biomass and exudates link plant diversity with soil bacterial and fungal biomass. Sci. Rep. Uk 7, 44641. doi: 10.1038/srep44641
Evans S. E., Burke I. C. (2013). Carbon and nitrogen decoupling under an 11-year drought in the shortgrass steppe. Ecosystems 16 (1), 20–33. doi: 10.1007/s10021-012-9593-4
Fan Y., Miguez-Macho G., Jobbagy E. G., Jackson R. B., Otero-Casal C. (2017). Hydrologic regulation of plant rooting depth. P Natl. Acad. Sci. U.S.A. 114 (40), 10572–10577. doi: 10.1073/pnas.1712381114
Flanagan L. B., Sharp E. J., Letts M. G. (2013). Response of plant biomass and soil respiration to experimental warming and precipitation manipulation in a Northern Great Plains grassland. Agr. For. Meteorol. 173, 40–52. doi: 10.1016/j.agrformet.2013.01.002
Fornara D. A., Tilman D., Hobbie S. E. (2009). Linkages between plant functional composition, fine root processes and potential soil N mineralization rates. J. Ecol. 97 (1), 48–56. doi: 10.1111/j.1365-2745.2008.01453.x
Furey G. N., Tilman D. (2021). Plant biodiversity and the regeneration of soil fertility. P Natl. Acad. Sci. U.S.A. 118 (49), e2111321118. doi: 10.1073/pnas.2111321118
Gastine A., Scherer-Lorenzen M., Leadley P. W. (2003). No consistent effects of plant diversity on root biomass, soil biota and soil abiotic conditions in temperate grassland communities. Appl. Soil Ecol. 24 (1), 101–111. doi: 10.1016/S0929-1393(02)00137-3
Gill R. A., Kelly R. H., Parton W. J., Day K. A., Jackson R. B., Morgan J. A., et al. (2002). Using simple environmental variables to estimate below-ground productivity in grasslands. Global Ecol. Biogeogr. 11 (1), 79–86. doi: 10.1046/j.1466-822X.2001.00267.x
Guerra C. A., Heintz-Buschart A., Sikorski J., Chatzinotas A., Guerrero-Ramírez N., Cesarz S., et al. (2020). Blind spots in global soil biodiversity and ecosystem function research. Nat. Commun. 11 (1), 3870. doi: 10.1038/s41467-020-17688-2
Hector A., Schmid B., Beierkuhnlein C., Caldeira M. C., Diemer M., Dimitrakopoulos P. G., et al. (1999). Plant diversity and productivity experiments in European grasslands. Science 286 (5442), 1123–1127. doi: 10.1126/science.286.5442.1123
Horton J. L., Hart S. C. (1998). Hydraulic lift: a potentially important ecosystem process. Trends Ecol. Evol. 13 (6), 232–235. doi: 10.1016/S0169-5347(98)01328-7
Isbell F., Craven D., Connolly J., Loreau M., Schmid B., Beierkuhnlein C., et al. (2015). Biodiversity increases the resistance of ecosystem productivity to climate extremes. Nature 526 (7574), 574–U263. doi: 10.1038/nature15374
Kang M. Y., Dai C., Ji W. Y., Jiang Y., Yuan Z. Y., Chen H. Y. H. (2013). Biomass and its allocation in relation to temperature, precipitation, and soil nutrients in inner Mongolia Grasslands, China. PloS One 8 (7), e69561. doi: 10.1371/journal.pone.0069561
Kettle W. D., Rich P. M., Kindscher K., Pittman G. L., Fu P. (2000). Land-use history in ecosystem restoration: a 40-year study in the prairie-forest ecotone. Restor. Ecol. 8 (3), 307–317. doi: 10.1046/j.1526-100x.2000.80043.x
Knapp A. K., Fay P. A., Blair J. M., Collins S. L., Smith M. D., Carlisle J. D., et al. (2002). Rainfall variability, carbon cycling, and plant species diversity in a mesic grassland. Science 298 (5601), 2202–2205. doi: 10.1126/science.1076347
Kuznetsova A., Brockhoff P. B., Christensen R. H. B. (2017). lmerTest Package: Tests in Linear Mixed Effects Models. Journal of Statistical Software 82 (13), 1–26. doi: 10.18637/jss.v082.i13
Lange M., Eisenhauer N., Sierra C. A., Bessler H., Engels C., Griffiths R. I., et al. (2015). Plant diversity increases soil microbial activity and soil carbon storage. Nat. Commun. 6, 6707. doi: 10.1038/ncomms7707
Lee J.-Y., Marotzke J., Bala G., Cao L., Corti S., Dunne J., et al. (2021). Future Global Climate: Scenario-Based Projections and Near-Term Information (Cambridge, United Kingdom and New York, NY, USA: Cambridge University Press).
Mueller K. E., Tilman D., Fornara D. A., Hobbie S. E. (2013). Root depth distribution and the diversity-productivity relationship in a long-term grassland experiment. Ecology 94 (4), 787–793. doi: 10.1890/12-1399.1
Nippert J. B., Knapp A. K. (2007). Soil water partitioning contributes to species coexistence in tallgrass prairie. Oikos 116 (6), 1017–1029. doi: 10.1111/j.0030-1299.2007.15630.x
Nippert J. B., Knapp A. K., Briggs J. M. (2006). Intra-annual rainfall variability and grassland productivity: can the past predict the future? Plant Ecol. 184 (1), 65–74. doi: 10.1007/s11258-005-9052-9
Pang J., Wang Y., Lambers H., Lambers H., Tibbett M., Siddique K. H., et al. (2013). Commensalism in an agroecosystem: hydraulic redistribution by deep-rooted legumes improves survival of a droughted shallow-rooted legume companion. Physiol. Plant. 149 (1), 79–90. doi: 10.1111/ppl.12020
Phillips R. P., Finzi A. C., Bernhardt E. S. (2011). Enhanced root exudation induces microbial feedbacks to N cycling in a pine forest under long-term CO2 fumigation. Ecol. Lett. 14 (2), 187–194. doi: 10.1111/j.1461-0248.2010.01570.x
Prommer J., Walker T. W. N., Wanek W., Braun J., Zezula D., Hu Y. T., et al. (2020). Increased microbial growth, biomass, and turnover drive soil organic carbon accumulation at higher plant diversity. Global Change Biol. 26 (2), 669–681. doi: 10.1111/gcb.14777
Ravenek J. M., Bessler H., Engels C., Scherer-Lorenzen M., Gessler A., Gockele A., et al. (2014). Long-term study of root biomass in a biodiversity experiment reveals shifts in diversity effects over time. Oikos 123 (12), 1528–1536. doi: 10.1111/oik.01502
R Core Team (2022). R: A language and environment for statistical computing. 4.1.1 (Vienna, Austria: R Foundation for Statistical Computing).
Reich P. B., Tilman D., Isbell F., Mueller K., Hobbie S. E., Flynn D. F. B., et al. (2012). Impacts of biodiversity loss escalate through time as redundancy fades. Science 336 (6081), 589–592. doi: 10.1126/science.1217909
Reynolds H. L., Packer A., Bever J. D., Clay K. (2003). Grassroots ecology: Plant-microbe-soil interactions as drivers of plant community structure and dynamics. Ecology 84 (9), 2281–2291. doi: 10.1890/02-0298
Rockstrom J., Steffen W., Noone K., Persson A., Chapin F. S., Lambin E. F., et al. (2009). A safe operating space for humanity. Nature 461 (7263), 472–475. doi: 10.1038/461472a
Roscher C., Temperton V. M., Scherer-Lorenzen M., Schmitz M., Schumacher J., Schmid B., et al. (2005). Overyielding in experimental grassland communities - irrespective of species pool or spatial scale. Ecol. Lett. 8 (4), 419–429. doi: 10.1111/j.1461-0248.2005.00736.x
Sindhøj E., Hansson A. C., Andrén O., Kätterer T., Marissink M., Pettersson R. (2000). Root dynamics in a semi-natural grassland in relation to atmospheric carbon dioxide enrichment, soil water and shoot biomass. Plant Soil 223 (1-2), 255–265. doi: 10.1023/A:1004801718567
Skelton R. P., West A. G., Dawson T. E. (2015). Predicting plant vulnerability to drought in biodiverse regions using functional traits. P Natl. Acad. Sci. U.S.A. 112 (18), 5744–5749. doi: 10.1073/pnas.1503376112
Slette I. J., Blair J. M., Fay P. A., Smith M. D., Knapp A. K. (2022). Effects of compounded precipitation pattern intensification and drought occur belowground in a mesic grassland. Ecosystems 25 (6), 1265–1278. doi: 10.1007/s10021-021-00714-9
Tilman D., Isbell F., Cowles J. M. (2014). Biodiversity and ecosystem functioning. Annu. Rev. Ecol. Evol. S. 45, 471–493. doi: 10.1146/annurev-ecolsys-120213-091917
Tilman D., Knops J., Wedin D., Reich P., Ritchie M., Siemann E. (1997). The influence of functional diversity and composition on ecosystem processes. Science 277 (5330), 1300–1302. doi: 10.1126/science.277.5330.1300
USDA-NRCS. (2019) Web Soil Survey. Available at: https://websoilsurvey.nrcs.usda.gov/app/HomePage.htm?TARGET_APP=Web_Soil_Survey_application_sp11wo1zqfzscmxfnpqovqut.
van Ruijven J., Berendse F. (2010). Diversity enhances community recovery, but not resistance, after drought. J. Ecol. 98 (1), 81–86. doi: 10.1111/j.1365-2745.2009.01603.x
Vitousek P. M., Menge D. N. L., Reed S. C., Cleveland C. C. (2013). Biological nitrogen fixation: rates, patterns and ecological controls in terrestrial ecosystems. Philos. T R Soc. B. 368 (1621), 20130119. doi: 10.1098/rstb.2013.0119
Wagg C., Roscher C., Weigelt A., Vogel A., Ebeling A., De Luca E., et al. (2022). Biodiversity-stability relationships strengthen over time in a long-term grassland experiment. Nat. Commun. 13 (1), 7752. doi: 10.1038/s41467-022-35189-2
Wang G., Koziol L., Foster B. L., Bever J. D. (2022). Microbial mediators of plant community response to long-term N and P fertilization: Evidence of a role of plant responsiveness to mycorrhizal fungi. Global Change Biol. 28 (8), 2721–2735. doi: 10.1111/gcb.16091
Wilcox K. R., Blair J. M., Smith M. D., Knapp A. K. (2016). Does ecosystem sensitivity to precipitation at the site-level conform to regional-scale predictions? Ecology 97 (3), 561–568. doi: 10.1890/15-1437.1
Wu Z. T., Dijkstra P., Koch G. W., Penuelas J., Hungate B. A. (2011). Responses of terrestrial ecosystems to temperature and precipitation change: a meta-analysis of experimental manipulation. Global Change Biol. 17 (2), 927–942. doi: 10.1111/j.1365-2486.2010.02302.x
Xu X., Niu S. L., Sherry R. A., Zhou X. H., Zhou J. Z., Luo Y. Q. (2012). Interannual variability in responses of belowground net primary productivity (NPP) and NPP partitioning to long-term warming and clipping in a tallgrass prairie. Global Change Biol. 18 (5), 1648–1656. doi: 10.1111/j.1365-2486.2012.02651.x
Xu X., Sherry R. A., Niu S. L., Li D. J., Luo Y. Q. (2013). Net primary productivity and rain-use efficiency as affected by warming, altered precipitation, and clipping in a mixed-grass prairie. Global Change Biol. 19 (9), 2753–2764. doi: 10.1111/gcb.12248
Yang Y., Tilman D., Furey G., Lehman C. (2019). Soil carbon sequestration accelerated by restoration of grassland biodiversity. Nat. Commun. 10, 718. doi: 10.1038/s41467-019-08636-w
Zahran H. H. (2001). Rhizobia from wild legumes: diversity, taxonomy, ecology, nitrogen fixation and biotechnology. J. Biotechnol. 91 (2-3), 143–153. doi: 10.1016/S0168-1656(01)00342-X
Zeppel M. J. B., Wilks J. V., Lewis J. D. (2014). Impacts of extreme precipitation and seasonal changes in precipitation on plants. Biogeosciences 11 (11), 3083–3093. doi: 10.5194/bg-11-3083-2014
Keywords: belowground biomass, biodiversity-ecosystem function, diversity manipulation, rainfall exclusion, grasslands
Citation: Podzikowski LY, Heffernan MM and Bever JD (2023) Plant diversity and grasses increase root biomass in a rainfall and grassland diversity manipulation. Front. Ecol. Evol. 11:1259809. doi: 10.3389/fevo.2023.1259809
Received: 16 July 2023; Accepted: 14 November 2023;
Published: 27 November 2023.
Edited by:
Jie Gao, Xinjiang Normal University, ChinaReviewed by:
George Furey, Norwegian University of Life Sciences, NorwayAmber Churchill, Binghamton University, United States
Copyright © 2023 Podzikowski, Heffernan and Bever. This is an open-access article distributed under the terms of the Creative Commons Attribution License (CC BY). The use, distribution or reproduction in other forums is permitted, provided the original author(s) and the copyright owner(s) are credited and that the original publication in this journal is cited, in accordance with accepted academic practice. No use, distribution or reproduction is permitted which does not comply with these terms.
*Correspondence: Laura Y. Podzikowski, bHBvZHppa29AZ21haWwuY29t