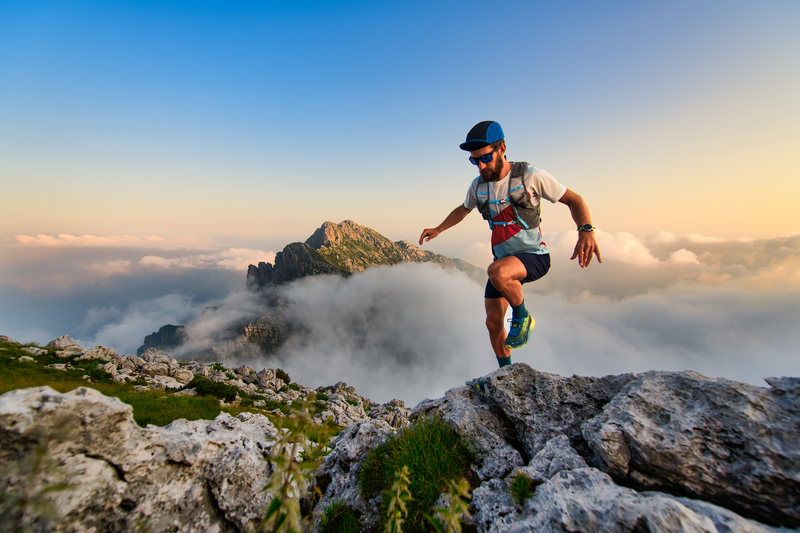
94% of researchers rate our articles as excellent or good
Learn more about the work of our research integrity team to safeguard the quality of each article we publish.
Find out more
ORIGINAL RESEARCH article
Front. Ecol. Evol. , 29 September 2023
Sec. Interdisciplinary Climate Studies
Volume 11 - 2023 | https://doi.org/10.3389/fevo.2023.1250593
This article is part of the Research Topic New Directions of Digital Economy, Energy Transition, and Climate Change in the Post-Covid-19 Era: Application of Machine Learning and Other Advanced Analytical Techniques View all 17 articles
Introduction: With the rapid development of digital technology and its deep integration with the engineering and construction field, digital construction has become an effective way for low-carbon transformation in the construction industry. However, there is a gap of empirical research between digital construction and carbon emissions.
Methods: This paper empirically investigates the impact of digital construction level on carbon emission intensity and the mechanism of action by using the two-way fixed effects model and mechanism testing based on the panel data of 52 Shanghai and Shenzhen A-share listed companies in China’s construction industry from 2015 to 2021.
Results: The findings indicate that the improvement of digital construction level can significantly decrease the carbon emission intensity of construction enterprises, and the conclusions still hold after robustness tests and discussions on endogeneity issues such as replacing core explanatory variables, replacing models, using instrumental variables method, system GMM model and difference in differences model. According to a mechanism analysis, digital construction can curb carbon emission intensity by enhancing the R&D innovation capacity and total factor productivity of enterprises. Furthermore, the heterogeneity analysis shows that the improvement of digital construction level in state-owned enterprises as well as civil engineering construction enterprises can better contribute to reducing carbon emission intensity.
Discussion: This paper will provide a reference for the synergistic optimization of digital construction development and carbon emissions reduction in construction enterprises. The research conclusions are going to promote the digital transformation of the construction industry to accelerate the achievement of the carbon peaking and carbon neutrality goals.
Since the industrial revolution, carbon dioxide (CO2) emissions have proliferated, and the global climate has gradually warmed because of the massive global consumption of fossil energy. The Paris Agreement, signed by 175 countries in 2016, aimed to limit global warming far below 2°C, ideally to 1.5°C, relative to pre-industrial levels (Hu et al., 2023). As a major carbon-emitting country, China attaches great importance to achieving peak carbon and carbon neutrality. The Chinese government’s high sense of responsibility and determination to achieve high-quality development is reflected in the pledge made by Chinese leaders to achieve China’s carbon peak and carbon neutral “3060 goals”, as well as in the Chinese Government Report in 2023, which mentioned “working toward the targets of peak carbon emissions and carbon neutrality with well-conceived and systematic steps”.
The construction industry is a pillar industry of China’s national economy, with a gross output value of 31.2 trillion dollars and an added value of 8.3 trillion dollars, accounting for 6.9% of GDP, which has made a great contribution to the high-quality development of China’s economy in 2022. However, the traditional construction industry also has the following factors and characteristics that constrain its high-quality development: first, as an energy-intensive industry, it suffers from high resource consumption and serious energy wastage; second, it has a poor construction environment, more problems with building quality, and the industry suffers from low productivity and high labor costs; and third, it has a slow process of industrialization of the construction industry, with a low degree of application of informatization and digitization of the construction process. As a consequence, the building sector has become one of the top three industries in terms of global CO2 emissions (Chen et al., 2020). The 2022 China Building Energy Consumption and CO2 Emissions Research Report shows that the proportion of the total life-cycle carbon emissions of buildings in the country is 50.9% in 2020. At the same time, the building sector also has the most significant potential for energy savings, with urban commercial buildings contributing 45% by 2050 and urban residential buildings contributing 49% (Zhou et al., 2018). Consequently, how to promote the carbon emission reduction of the construction industry and then put forward corresponding strategic suggestions is an urgent problem to be solved to achieve the “dual carbon” goal of China’s construction industry.
Meanwhile, a new generation of information technology is featured by digitalization, networking and intelligence to the subversive changes brought to the manufacturing industry, which triggered the industrial transformation is gradually affecting the traditional construction industry. However, unlike the manufacturing industry, the digital transformation of the construction industry has its own characteristics such as the manufacturing industry can usually manufacture products in the assembly line, the production tools are relatively fixed, while the location of the building in the construction industry is unchanged, the construction tools have a greater dynamic (Ding, 2020). In addition, the digitalization of the construction industry is also characterized by the following features: informatization and intelligence of the entire construction lifecycle supported by engineering software, enhanced upstream and downstream collaboration and information sharing in the construction supply chain, timely completion of key project milestones, and control of project costs and reduction of exceeding the project budget (Yilmaz et al., 2023). “A Program for the Overall Layout of China’s Digital Development” pointed out that the construction of digital China is an essential engine for promoting the Chinese path to modernization in the digital era in February 2023, and digital construction is an essential portion of the realization of digital China construction, which will effectively solve the pain points of the traditional building industry such as extensive production methods, low production efficiency, and large resource consumption. At the same time, the “Digital Building Development White Paper” published by the China Academy of Information and Communications Technology (CAICT, 2022) proposed that the global building digitization market size was about $9.8 billion in 2019. It is predicted to reach $29.1 billion by 2027, with a CAGR of 18.2%, which is in a rapid growth trend. It can be seen that the combination of engineering construction and digital technologies such as machine learning, building information modeling (BIM), blockchain, and big data will become a novel power for the transformation and high-quality development of the construction industry.
Construction enterprises play a crucial role in the use of digital construction technology and the realization of carbon emission intensity reduction as the carrier of digital construction technology implementation and the micro-unit to realize carbon peaking and carbon neutrality goals of the construction industry. From the existing literature, the carbon emission decrease effect of digital construction technology has sparked the interest of the academic community. It has been shown that Building 4.0 can improve productivity and economic efficiency (Forcael et al., 2020), promote technological progress and technological innovation, and enhance sustainability in the construction industry through new technologies and processes (Baduge et al., 2022). The overall green building analysis capability of BIM facilitates the design of sustainable buildings and the rational selection of materials (Liu et al., 2022b), and automation and robotics in intelligent construction help minimize waste in construction, providing the construction industry with opportunities to improve accuracy, keep project costs down and reduce waste, resulting in enabling efficient use of resources and reducing carbon emissions (Adepoju et al., 2022). In the articles above, instead of fully testing the impact of digital construction at a comprehensive level on CO2 emissions and mechanism, the scholars have only analyzed the impact of carbon emission with a few specific digital construction technologies.
In this context, this study aims to respond to the following questions: Can digital construction effectually reduce the CO2 emissions of construction enterprises? If digital construction can empower construction enterprises to reduce carbon emissions, what is its mechanism of action? What actions should be taken by relevant government departments and construction companies to promote the synergistic development of digital construction and carbon emission reduction?
Accordingly, the research objectives of this paper are as follows:
(1) Focusing on the construction industry, this research measures the digital construction level index scientifically and comprehensively and analyzes the impact of the digital construction level on the carbon emission of construction enterprises from the micro-enterprise level.
(2) We systematically analyze the micro-internal mechanism of digital construction affecting the carbon emission intensity of enterprises and examine the heterogeneity of the level of digital construction on carbon emission reduction in terms of the nature of business and the sub-industry types to which it belongs.
(3) We propose corresponding policy recommendations based on the theoretical analysis and empirical test results.
As a consequence, from the perspective of micro-enterprises, this paper innovatively measures the digital construction level index using the entropy weight method from the input, governance, and output dimensions of digital construction and empirically tests the influence of digital construction level on the carbon emission intensity of enterprises and its mechanism of action based on a sample of 52 listed companies in the construction industry from 2015–2021. The results of the study provide a theoretical basis for government departments to formulate leading policies on digital construction technology and carbon emission reduction policies in the construction industry and offer a reference for carbon emission reduction management decisions of construction enterprises.
The remainder of the paper is laid out as follows. Section 2 is a literature review. Section 3 presents the research hypotheses. The materials and methods are presented in Section 4. Section 5 discusses the empirical findings. Section 6 consists of conclusions and policy recommendations.
A number of scholars have studied digital technology and its development extensively, with the tremendous changes it has produced in human production and lifestyle as well as the important role it plays in helping the global process of combating climate change. According to the research theme of this paper, the literature involved is mainly reviewed from the following three aspects: the first is the application of digital technology in the area of engineering construction, that is, the related research of digital construction, the second is the research related to building carbon emissions, and the third is the study on the influence of digital technology on carbon emissions.
Disruptive digital technologies have driven the evolutionary adaptation of the construction industry through historical socio-technical processes (Woodhead et al., 2018). Digital construction is a new engineering construction mode that uses digital technology for architectural design, construction and operation under the background of a new round of scientific and technological revolution (Ding, 2020). Similarly, some scholars have drawn analogies to the theory of Industry 4.0, where the increasing automation of manufacturing environments and the creation of digital value chains derive the concept of Construction 4.0 (Craveiro et al., 2019), which can then be called a new era for construction (Chen et al., 2022). Construction 4.0 will contribute to the transformation of the construction industry into a technologically innovative industry and align it with manufacturing in terms of productivity and performance improvement (Oesterreich and Teuteberg, 2016). In view of this, scholars have conducted extensive research on how to apply digital construction technologies. Dou et al. (2023) summarized and analyzed the overall application of the top ten emerging digital technologies in the architecture, engineering and construction sectors from 2011 to 2020, including BIM, radio frequency identification, 3D printing, big data, digital twins, blockchain, IoT, virtual reality and artificial intelligence. Zhang et al. (2022) proposed a digital twin framework for building site monitoring by combining the multiple levels of detail of BIM, which can enhance the process monitoring of construction sites, improve quality, efficiency and construction safety, as well as the integration of digital twin and BIM can also support the implementation of net-zero carbon buildings (Shen et al., 2022). Regarding the future trend of digital construction, efficient construction, value-driven computational design and user-driven built environments are emerging visions for digital transformation in the building industry (Ernstsen et al., 2021).
The current research on building carbon emissions involves the calculation of carbon emissions and the path to achieve carbon reduction and carbon neutrality related to this study. Regarding the accounting of carbon emissions, at the macro level, one method is to adopt the carbon emission factor method proposed by IPCC in 1996 to measure the national carbon emissions from the construction industry (Chi et al., 2021); the other is to apply the input–output model (Leontief, 1970) and combine it with the LCA method to measure the carbon emissions of the construction industry (Onat et al., 2014). In contrast, there is no systematic methodology at the micro-firm level, which is mainly obtained directly through ESG reports etc., or estimated with the help of firm and industry main operating cost (Chapple et al., 2013). Furthermore, scholars have analyzed the building life cycle and emission reduction measures at various stages in the path to achieving carbon emissions reduction in buildings. Du et al. (2023b) pointed out that reasonable profit distribution based on carbon emission reduction is crucial to promote the implementation of low-carbon initiatives by construction supply chain firms. Zhang et al. (2019) developed the China Building Construction Model (CBCM) based on the production and transportation of building materials and on-site construction processes, indicating that it may be possible to reduce future carbon emissions associated with the building construction sector with the promotion of new low-carbon building structures and the improvement of productivity. Li et al. (2022) assessed the changes in carbon emission reductions from commercial building operations in various provinces of China, which provides a reference for local governments and other economies to improve energy efficiency during the operation phase. In addition, it can promote carbon reduction in the construction industry by rationally managing the construction waste generated in the construction process during the building demolition phase and utilizing this resource (Liu et al., 2023a).
At present, numerous studies have been undertaken to explore the influence of the digital economy or digitalization on CO2 emissions, and digital technology can provide digital and intelligent technical means for the green development of society, contributing to promoting the decrease of overall energy consumption and CO2 emissions in society (CAICT, 2021). Some scholars have studied the correlation between the digital economy and carbon emissions in various cities in China and found that the growth of the digital economy can decrease urban CO2 emissions (Yu et al., 2022) through strengthening environmental supervision and promoting green innovation (Pan et al., 2023), while positively influencing carbon emission reduction by exerting a “spillover effect” on neighboring cities (Liu et al., 2022a). Lu (2018) found that information and communication technologies significantly curbed CO2 emissions based on a sample of 12 Asian nations from 1993 to 2013. Danish (2019) also came to the same conclusion using data from 59 nations along the Belt and Road from 1990 to 2016. However, other academics contend that as digital technology depends on energy (Yang et al., 2022), using electricity will intensify the extraction and consumption of resources, resulting in more carbon emissions, but it will gradually suppress CO2 emissions with the deepening of the use of digital technology, displaying a nonlinear connection with an inverted U shape (Li et al., 2021; Miu et al., 2022). For example, Li et al. (2023b) found that the coefficient of the influence of the digital economy on 3E (energy–environment–economy) efficiency changed from negative to positive when per capita GDP exceeded the threshold based on data from 24 EU nations from 2011 to 2019, illustrating that as the continuous maturity of the digital economy, the sustainability of economic growth progressively rose and the energy intensity decreased step by step.
Further focusing on the micro-enterprise level research perspective, Shang et al. (2023) took listed companies in China from 2012 to 2020 as a sample, and revealed that company digital transformation can dramatically lower enterprise carbon emission intensity by enhancing internal control abilities and technological innovation capability. In addition, other scholars have discovered that enterprise digital transformation can also decrease corporate CO2 emissions by improving energy utilization efficiency (Yang et al., 2023), resource allocation capacity (Chen and Kim, 2023), green innovation capability (Liu et al., 2023c) and other factors.
In conclusion, it can be found that domestic and international scholars have achieved stage-by-stage results in the research related to digital construction and carbon emission in the construction field based on the organization and review of domestic and international literature. However, some problems still need to be solved:
(1) The study on the impact of digital technology on CO2 emissions is mostly concentrated at the macro level of countries, regions and industries, but there are fewer studies at the micro-enterprise level. Especially for construction enterprises, existing studies mainly focus on the specific application of digital construction technology to the various stages of the building life cycle, but few studies have measured the comprehensive level of digital construction of construction enterprises; therefore, there are fewer empirical studies on the impact on carbon emissions of enterprises.
(2) In the path of building carbon emission reduction, scholars primarily analyze emission reduction measures based on the industry level in the building life cycle and its various stages, in which digital construction provides new opportunities for low-carbon transformation for construction enterprises. However, the current research only stays in the stage of qualitative analysis, and few researches have systematically analyzed and explored the intrinsic mechanism of carbon emission reduction of construction enterprises by digital construction through empirical tests.
In view of the gaps and deviations of the above studies, this study draws on the way scholars measure the digital economy or enterprise digitization and combines the digital characteristics of engineering construction itself, to construct the digital construction level index of construction enterprises from a brand-new dimension. Then, we study its impact on corporate carbon emission reduction and the internal mechanism from both theoretical and empirical perspectives.
As an emerging construction mode in the construction industry, digital construction provides new strategic opportunities and scientific and technological support for the low-carbon transformation of the construction industry (Wang et al., 2023a), and promotes the carbon emission reduction of construction enterprises from the following aspects. To begin with, the application of digital construction technology can achieve real-time collection, monitoring, transmission and analysis of energy data and guide energy factors to realize efficient allocation (Yao et al., 2023); at the same time, according to the theory of supply and demand, it can also effectively promote the coordination of the supply and demand side of production factors in construction enterprises, so that enterprises can achieve higher energy efficiency under the same conditions (Veskioja et al., 2022; Zhao and Ren, 2023) to promote carbon emission reduction. Furthermore, in accordance with the project management life cycle theory (Ma et al., 2018), the carbon emission reduction empowered by digital construction technology is also reflected in the use of digital elements by enterprises to strengthen the control of the whole life cycle process of construction. In the project design stage, construction enterprises can virtualize the construction process by using construction virtual prototype technology and mixed reality technology, which aims to provide visual means for carbon emission prediction and minimization of construction projects so as to find methods to decrease carbon emissions by taking preventive or corrective measures before the project starts (Wong et al., 2013). During the construction phase, the materials used in the use of 3D concrete printing technology can emit 80% less CO2 than the production of traditional concrete materials (Nematollahi et al., 2018). In the construction operation and management phase, companies generate fewer carbon emissions in future construction activities by applying industrial IoT to collect past data from the construction process and using digital twin architecture to monitor real-time architectural situations (Shen et al., 2022) to compose models and predict their future behavior (Metallidou et al., 2022). Finally, the application of digital construction technology can enhance the ability of information interaction and sharing within enterprises, such as the use of data creation and sharing, cross-temporal information dissemination and other channels to transmit, flow green emission reduction technology and other aspects of information (Lyu et al., 2023), for the purpose of reducing unnecessary activities, in addition, big data technology can offer data support for enterprise carbon emission reduction by improving the integration of data information such as energy input structure and CO2 emissions (Zhang et al., 2021). Based on the above, a hypothetical H1 is proposed.
H1: The development of digital construction level can contribute to the reduction of carbon emission intensity of enterprises.
For the construction industry, the process of integrating traditional engineering construction activities with digital technology is an innovative activity (Ding, 2020), such as China State Construction Engineering Corporation Limited established the first China National Digital Construction Technology Innovation Center and independently developed AECMate domestic 3D engineering image software. On the one hand, digital innovation theory holds that firms will invest more in innovation activities among themselves in order to make better products in the digital economy (Wen et al., 2022a; Wen et al., 2022b). In order to implement innovation-driven development strategies and enhance their competitive advantages in the industry, construction enterprises will inevitably increase their R&D efforts and investments in digital construction technology and other key core technologies (Wu et al., 2021), thereby enhancing innovation output (Kim, 2019). Pecking order theory states that since the cost of external financing is higher than the cost of internal financing, the company can only rely heavily on internal financing, resulting in underinvestment (Myers and Majluf, 1984). The implementation of digital construction technology in construction enterprises also releases a benign signal to the outside world to actively respond to the national strategy, implying high-quality development in the future, which means that it is conducive to facilitating the trust of external financial backers (Bertani et al., 2020; Zhang and Dong, 2023), so as to provide financial support for enhancing R&D innovation capabilities (Ding et al., 2022). It has been shown that for China, increased R&D investment directly curbs carbon intensity (Wang and Zhang, 2020) and can play a moderating role between digitization and CO2 emissions (Ma et al., 2022).
On the other hand, transaction cost theory holds that cost reduction is an essential way for firms to obtain heterogeneous innovation resources (Hennart, 1988). Digital technologies such as the Internet and artificial intelligence have the advantage of facilitating the sharing of explicit and tacit knowledge resources, which can help enterprises break down information silos (Wu et al., 2022), alleviate information asymmetry (Liu et al., 2023b) and improve the transmission efficiency and accuracy of internal and external information and knowledge (Goldfarb and Tucker, 2019; Fang et al., 2022; Wen et al., 2022a). It can also increase the efficiency of resource allocation of companies (Sousa-Zomer et al., 2020), and allocate key resources to core technologies and innovation activities (Li et al., 2023a), thus reducing the cost of companies in the process of information acquisition and avoiding the misallocation of resources and energy waste, and reducing CO2 emissions. In addition, digital technology also helps to enhance the green development capability of enterprises when R&D cooperation and experience exchange of reducing carbon emission among companies in the construction industry (Wang et al., 2018; Zhang et al., 2021); and it promotes collaboration and knowledge sharing between enterprises and institutions such as universities and research institutes (Zhang, 2019) and open innovation of enterprises (Mubarak et al., 2021), resulting in reducing the R&D trial and error costs of digital construction technologies, shortening the R&D design cycle, and improving innovation efficiency (Shang et al., 2023). Based on the above, a hypothetical H2 is proposed.
H2: The development of digital construction level can reduce the carbon emission intensity by improving the R&D innovation capability of enterprises.
Total factor productivity is part of the output that cannot be interpreted by the number of inputs used in production, and more is the growth brought about by intangible factors such as technological progress and technological efficiency (Comin, 2010). The theory of technological progress argues that the margins of productivity factors can be raised in the identical ratio by technological progress to bring down energy intensity, thus achieving carbon emissions reduction (Cheng et al., 2021). Correspondingly, in the long run, it is increasingly becoming a consensus in Chinese society to achieve low-carbon economic development with technology (Wang et al., 2022). On the one hand, digital construction technologies can boost total factor productivity by increasing construction efficiency on the construction site; specifically, when building concrete walls with complex geometry using a robot on the construction site, productivity increases significantly compared to traditional forms of construction (García De Soto et al., 2018). Moreover, a construction site digital process platform built upon the foundation of IoT, cloud computing, and 5G mobile communication technologies can improve productivity in the construction process (Zhou et al., 2020) by addressing the complexity of the construction site environment and enhancing collaboration between different project participants such as contractors, construction workers, machinery and equipment, and material suppliers on the construction site (Oesterreich and Teuteberg, 2016). On the other hand, digital construction can also provide technical support for lean construction, such as the development of construction sites VisiLean (Dave et al., 2011) and BeaM! (Schimanski et al., 2021) production management systems integrated with BIM, IoT and lean construction, which can be applied to the entire project lifecycle, enabling the realization of lean principles from planning and design, construction and post-construction phases (Dave et al., 2016), with the aim of minimizing waste and uncertainty in the building construction process and thus increasing total factor productivity. Further, the increase in total factor productivity can yield desirable outputs such as meeting project schedules, cost and quality targets with sufficiently few construction inputs such as materials, energy and labor (Hu and Liu, 2017), which can improve energy efficiency and reduce carbon emission intensity (Amri et al., 2019; Altinoz et al., 2021; Lahouel et al., 2021). For instance, Zhu et al. (2019) found that technological progress (measured by total factor productivity) decreased the energy consumption intensity of the Chinese building industry at an average rate of 7.1% per year by constructing a model of the building process, hence contributing to carbon productivity (i.e., the inverse of carbon intensity) (Fan et al., 2021; You and Zhang, 2022). Based on the above, a hypothetical H3 is proposed.
H3: The development of digital construction level can reduce the carbon emission intensity of enterprises by improving total factor productivity.
Through the above theoretical analysis, the theoretical transmission mechanism is shown in Figure 1.
To test the effect of digital construction on the carbon intensity of construction enterprises, the following benchmark regression model is constructed in this paper, as shown in Equation (1):
where, the enterprise, t denotes the year, denotes the carbon emission intensity of the enterprise i in t year, denotes the digital construction level index of the enterprise, represents the control variable, as well as is the random error term; in addition, the model also controls the enterprise fixed effect , the year fixed effect . For the sake of making the test results more robust, this paper defaults to the clustering robustness standard error.
Enterprise carbon emission intensity (CEI) is determined by accounting for carbon emissions, which indicates the CO2 emissions produced per unit of production value, and the reduction of carbon emission intensity reflects, to some extent, the improvement of enterprises’ CO2 emission reduction ability. This paper evaluates carbon emission intensity by the proportion of enterprise CO2 emissions to main business income in light of the data accessibility, as described by Chapple et al. (2013). The amount of directly available data is very limited since the Chinese government currently does not mandate companies to disclose CO2 emissions data in their annual reports or environmental, social, and governance (ESG) reports. Hence, research uses the way of Shang et al. (2023) to estimate the carbon emissions of companies using industry carbon emissions with the help of the main business cost, which is calculated as shown in Equation (2):
where denotes enterprise CO2 emissions, denotes the main business cost of company, denotes the industry main business cost and denotes industry CO2 emissions. The carbon emission behaviors of the construction enterprise include the carbon emission generated by the process of completing the construction of various sub-elements and implementing various measures of the project, as well as the various types of energy power consumed by mechanical equipment used in the demolition phase. In other words, the CO2 emission is mainly due to the energy consumption, i.e., the combustion of fossil fuels, and therefore the industry’s CO2 emissions are measured according to the energy consumption. When we check the “China Energy Statistical Yearbook”, there are 11 main types of energy consumption involved in the construction industry, mainly including raw coal, coke, kerosene, fuel oil, gas oil, diesel oil, crude oil, LPG, natural gas, heat and electricity, of which the CO2 emissions from the depletion of the first nine types of energy are direct emissions from the combustion of fossil fuels, and the electricity and heat purchased by enterprises outside are indirect emissions. The CO2 emissions of the industry can be calculated according to Equation (3):
where, denotes CO2 emissions due to the burning of consumed fossil fuels, denotes CO2 emissions due to the burning of electricity and heat by enterprises.
Equations (4) and (5) can be used to compute CO2 emissions from fossil fuel burning using the emission factor approach described in the 2006 IPCC Guidelines for National Greenhouse Gas Inventories:
In Equation (4), denotes the quantity of fossil fuel consumed, and denotes the CO2 emission factor.
In Equation (5), stands for the average low-level calorific value of the fossil fuel, for its carbon content per unit calorific value, for its carbon oxidation rate, and ρ denotes the proportion of CO2 to the molecular weight of carbon, which is 44/12. Table 1 displays the values of each variable and data source.
For the measurement of CO2 emissions from electricity and heat, it can be calculated according to Equation (6):
where and denote the consumption of electricity and heat respectively, and denote the CO2 emission factors of electricity and heat respectively, both adopt the recommended values of the Ministry of Ecology and Environment, and the CO2 emission factors of electricity in 2015–2020 and 2021 are calculated as 0.6101 tCO2/MWh and 0.518 tCO2/GJ respectively, and the CO2 emission factor of heat is 0.11 tCO2/GJ.
How to measure the digital construction level index (DIGC) of construction enterprises is a complex and systematic difficulty. As digital construction is in the initial phase, scholars are gradually exploring and improving the concepts and theories, and there is a dearth of academic research on the measurement of the digital construction level of construction enterprises. At the same time, with the advent of the digital economy era, many scholars measure the digital economy or digital transformation of enterprises with various methods. Therefore, this paper draws on the relevant digital index measurement methods and combines the digital characteristics of engineering construction itself to make a comprehensive estimation of the digital construction level of construction enterprises.
In this paper, we will measure the digital construction level index in three dimensions: input, governance and output of construction enterprises in digital construction, whose conceptual diagram is shown in Figure 2, and synthesize the index by using the entropy value method.
(1) Referring to Xiao et al. (2022), this paper uses the proportion of digitization-related portion of intangible assets in the breakdown of intangible assets to total assets as a proxy variable for firms’ digital construction input. Specifically, the use of software in construction enterprises mainly includes BIM, engineering management information systems, virtual design and construction, simulation calculation and process planning. As a consequence, the items with intangible asset line items, including “software”, “computer software”, “computer software”, “software use rights”, “software systems”, “intelligent platforms” and other digital technology-related keywords are regarded as “digital construction intangible assets”, and then their proportion to the total assets of the year is calculated, which is the digital construction input of the enterprise.
(2) Digital construction technology, as a key point for the transformation and upgrading of construction enterprises, its characteristic information is more likely to be represented in the annual reports of enterprises. The vocabulary usage in annual reports can reflect the strategic features and future view of enterprises, and to a large extent, it also manifests the management concepts promoted by enterprises and the development path guided by this philosophy (Wu et al., 2021). Consequently, this paper refers to Wu et al. (2021) and Zhao and Wang (2021) to extract the keywords of “digital construction technology” from the annual reports of construction enterprises and conduct word frequency statistics as a variable for the digital construction governance of companies.
Following are the precise steps. In the first step, the annual reports of listed enterprises in the construction industry from 2015–2021 were collected from Giant Tide Information Network and converted to text format through Python’s “pdfplumber” function. In the second step, a feature thesaurus of digital construction technology was formed on the basis of policies and research reports such as the “Digital Construction Development White Paper (2022)” and the “Several Comments on Accelerating the Development of New Construction Industrialization”, as shown in Figure 3, with a total of 96 feature words in six dimensions. In the third step, based on the self-built feature lexicon, the Jieba function of Python was used to split words for all samples and remove intonation words, auxiliary words and punctuation that have no practical meaning in the text, making it more meaningful to do word frequency statistics. The fourth step was to search, match and count word frequencies depending on the feature thesaurus based on the annual report in the company text format. In the fifth step, because the Jieba function had certain limitations on the word segmentation function of English words, such as part of word frequency statistics of “AR”, “VR”, “AI” and “CIM”, which had nothing to do with digital construction technology, these were eliminated after identification to form the final total word frequency of digital construction of construction enterprises.
(3) The output of digital construction is expressed by using the patents applied by construction companies in the area of digital construction technology. The calculation method is as below. In the first step, obtain the IPC subdivision classification numbers of patents applied for inventions and utility models by construction enterprises for each year from the China Research Data Service Platform (CNRDS). In the second step, patents in the field of digital construction are matched according to the “International Patent Classification and National Economic Industry Classification Reference Relationship Table (2018)” (China National Intellectual Property Administration, 2018) and the “Statistical Classification of Digital Economy and Its Core Industries” issued in China in 2021.
Finally, the proportion of digital construction input, i.e., the enterprise’s investment in digital construction intangible assets is 35.6% in the establishment of the digital construction level index. The weight of digital construction governance is 16%, which may be influenced by the quality of the company’s annual report and the construction of the characteristic word database, and there may be some deviation in the results, so the weight is reasonable. The weight of digital construction output, i.e., digital construction technology patents, accounts for nearly half of the weight since the patent applications are generated by construction enterprises in the process of engineering construction, which can best reflect the digital construction level of companies. Consequently, the weights of each sub-index of the digital construction level index are scientific and reasonable.
To increase the study’s accuracy, this paper draws on previous studies (Shen et al., 2020; Zhang and Dong, 2023) and combines the research practice of this paper to add the control variables. Enterprise size (Size) and enterprise age (Age) are the basic situations of the enterprise. The larger the size of the enterprise, the more likely it is to have the energy to implement low-carbon behaviors and governance. The enterprise age may reflect the life cycle in which the firm is located, with longer-established firms likely to be in decline and less able to govern low-carbon behaviors. Reducing greenhouse gas emissions demands a great deal of additional management in day-to-day operations, as it requires a complex design of green governance processes within the company, so good corporate governance enables companies to integrate internal resources to promote carbon reduction (Kock et al., 2012). Therefore, this paper controls corporate governance factors from board size (Board), equity multiplier (EM), current ratio (CR) and operating capacity (ET), in which the board of directors makes the final decision on the extent to which the firm implements carbon reduction strategies; equity multiplier reflects the financial leverage of the enterprise, and excessive leverage is not conducive to the enterprise’s carbon emission reduction; current ratio reflects the enterprise’s solvency, and the value of which to a certain extent will affect the decision on the carbon emission reduction; and the operating capacity embodies the enterprise’s efficiency of the use of assets to support the business activities. In addition, the disclosure of environmental and sustainable development (Sus) reflects a company’s environmental awareness and social responsibility. Specific variables are defined in Table 2.
We have combed through China’s important national policies on the development of digitization and informatization in the construction industry in recent years and found that the state has been vigorously promoting digital construction-related technologies as well as the wide-scale application of digital construction technologies by construction enterprises since 2015. Therefore, in this paper, 52 listed companies in Shanghai and Shenzhen A-shares in the construction industry from 2015–2021 are used as the initial sample, and the listed companies in the construction industry are determined according to the industry categories stipulated by the China Securities Regulatory Commission in 2012. According to the needs of the study, the samples are screened as follows: (1) exclude samples listed after 2015; (2) exclude samples listed in ST, *ST and delisted from 2015–2021; (3) exclude samples undergoing major asset restructuring from 2015–2021; (4) exclude samples changing from other industries to the construction industry from 2015–2021. After the above screening, we finally obtained 364 company-annual observations. The original data used in this study are obtained from China Energy Statistical Yearbook, China City Statistical Yearbook, CSMAR Database, China Economy Information NET Database, RESSET Database and CNRDS, and the annual reports of enterprises are compiled from Giant Tide Information Network.
The results of descriptive statistics are presented in Table 3, in which the mean value of carbon emission intensity (CEI) is 46.313, indicating that construction enterprises produce 46.313 kg of CO2 emissions per 10,000 yuan of business income on average, and the standard deviation of 4.921 with the maximum values is 59.9 and the minimum values is 31.085, indicating that there are large differences in the carbon emission intensity of different enterprises. The mean value of the Digital Construction Level Index (DIGC) is 2.514, the standard deviation is 0.543, and the maximum and minimum values are 3.730 and 0.796, respectively, which means that the development level of digital construction of different construction enterprises varies greatly, some construction enterprises have a higher development level and application of digital construction, but some construction enterprises have not yet made effective use of digital construction technology. The mean value of Sus is 0.885, and the standard deviation is 0.320, indicating that most construction enterprises disclose environmental and sustainability-related information in their annual reports or ESG reports. The distribution characteristics of the remaining control variables are roughly the same as in previous studies and will not be repeated.
The benchmark regression findings of the effect of the level of digital construction on the CO2 emission intensity of construction companies are shown in Table 4. This study adopts the progressive regression method in the benchmark regression, and after gradually introducing fixed effects and control variables, the influence of digital construction on carbon intensity is always negative. Column (1) reports the regression results of the explanatory variables and core explanatory variables only, and the coefficient of the digital construction level index (DIGC) is −3.283, which passes the 1% statistical significance test, indicating that the improvement of digital construction level of construction enterprises can significantly reduce carbon emission intensity. Although column (2) controls for firm and year fixed effects based on column (1), the coefficient for DIGC is still statistically negative at the 1% level at −1.982. Columns (3) and (4) add control variables to column (1) and control for firm and year fixed effects in turn, and it can be found that the R2 of the model is increasing and the DIGC coefficient is gradually decreasing, indicating that it may be because some factors affecting the intensity of carbon emissions have been absorbed after the inclusion of control variables and fixed effects, but the significance has not changed. In conclusion, the data above demonstrates that the level of digital construction has a considerable negative effect on the intensity of carbon emissions, and that the carbon emission intensity decreases with increasing levels of digital construction in construction enterprises, and the research hypothesis H1 is supported by the empirical evidence.
In order to enhance the robustness and validity of the core findings, this paper chooses to replace the core explanatory variables and replace the model for robustness testing.
In this paper, the digital construction level of construction enterprises is remeasured in the following way: the enterprise's governance of digital construction indicator is replaced by the frequency of digital construction keywords in the annual reports of enterprises as the ratio of the total number of keyword disclosures to the total number of words in the annual report of the corresponding year, and then it is re-synthesized into the digital construction level index with the digital construction input and output indicators using the entropy value method, which is denoted as DIGC_1. The test finding is reported in column (1) of Table 5, where it is discovered that DIGC_1’s coefficient is considerably negative at the 1% level, which demonstrates that the main finding of the study still holds after changing the measurement of digital construction level, further validating the research hypothesis of this paper.
To address the possible heteroskedasticity and autocorrelation in the panel data, this paper draws on Cai et al. (2022) to estimate the model using FGLS, and the findings are shown in column (2) of Table 5, where the regression coefficient of DIGC is still dramatically negative at the 1% level.
The findings of all the aforementioned robustness tests do not differ noticeably from the sign and degree of significance of the primary explanatory variables based on the fixed-effects model, demonstrating the robustness of the estimates of the fixed-effects model.
The endogeneity problem due to reverse causality may exist in the empirical study of this article (Zhao and Wang, 2021), in other words, enterprises with low carbon emission intensity are more focused on improving digital construction. To mitigate the endogeneity problem, we control for time effects and firm effects in the baseline model, furthermore, the instrumental variables approach, the system GMM model and the difference in differences model are applied to discuss the endogenous problem.
The basic approach to solving the endogeneity problem is to select appropriate instrumental variables for the core explanatory variables, and effective instrumental variables fulfill the basic requirements of correlation and exogeneity. This study utilizes the historical data with the lagged terms of the core explanatory variables as a solution to the endogeneity problem. Specifically, this paper selects the postal and telecommunication data in 1984 of the province where the firm is located as an instrumental variable drawing on Wang et al. (2023b), Du et al. (2023a) and Xue et al. (2022). Since the post and telecommunication data are cross-sectional data and cannot be directly used in the econometric analysis of panel data, we refer to Nunn and Qian (2014), Wang et al. (2023b) and Xiao et al. (2022) for the treatment of this issue, and introduce the time-series variable of the number of Internet broadband access subscribers in the previous year to construct the panel instrumental variable. Finally, instrumental variables for the level of digital construction in this study are the interaction terms of the number of Internet broadband access subscribers in the previous year with the number of landline telephones per 100 people in the province where the construction firms were registered in 1984, respectively, as well as the first-order lagged terms of the core explanatory variables. The model is re-tested using the two-stage least squares (2SLS) method.
Theoretically, for historical postal data, on the one hand, digital construction technology is based on BIM, Internet, big data, digital twin and other digital technologies; meanwhile, post and Internet provide specific carriers for digital construction technology applications in construction enterprises, so the tool variables satisfy the relevance condition; on the other hand, the development of post and mobile Internet itself does not produce the massive CO2 emissions and will not have a direct effect on the carbon emission intensity of enterprises, in this sense, the selected instrumental variables satisfy the condition of exogeneity. For the lagged terms of the core explanatory variables, on the one hand, the core explanatory variables are correlated with their first-order lagged variables, and on the other hand, the lagged variables are exogenous because they have already occurred and are therefore “pre-determined”, and may not be correlated with the current period’s disturbance terms.
The result of the second-stage estimation of the instrumental method is shown in column (1) of Table 6. The Kleibergen-Paap rk LM statistic is 22.254, which significantly rejects the original hypothesis of non-identifiability at the 1% level. The Cragg-Donald Wald F statistic is greater than the Stock-Yogo test at 10% critical value of 19.93, rejecting the original hypothesis of weak instrumental variables. The Hansen J test p-value is 0.338, which is greater than 0.1, indicating that all instrumental variables are exogenous. The above indicators show that the instrumental variables are reasonably reliable. The coefficient of the digital construction level of the enterprise (DIGC) is extremely negative at the 1% level in the second-stage regression, indicating that the main conclusion of this paper holds again.
Next, a system GMM model is used with reference to Xie and Kuang (2020), and the level lagged terms of key variables are introduced as instrumental variables in the regressions for testing. The core explanatory variable DIGC regression coefficient is dramatically negative, according to the result in column (2) of Table 6, which is similar to the prior estimation and shows that the conclusion of this study is robust.
In this study, the difference in differences model (DID) is chosen to further overcome the endogeneity problem by referring to the studies of Wu et al. (2021) and Wang et al. (2023b). When constructing the digital construction level index using the entropy value approach, its subindex, the quantity of applications for digital construction patents, has the largest weight and best reflects the digital construction level of construction enterprises, so it is used to judge whether the digital construction technology has been effectively applied according to the digital construction patent applications of enterprises in each year. If a construction enterprise has applied for a digital construction patent during the sample period, it means that there is a substantial application of digital construction technology, and this type of enterprise is used as an experimental group (du=1); if there has been no application for a digital construction patent, it means that there is no substantial application of digital construction technology, and this type of enterprise is used as a control group (du=0); furthermore, the period dummy variable dt is set, and dt is assigned to 1 if the enterprise adopts digital construction technology in the current and subsequent years, otherwise it is 0. Accordingly, the following difference in differences model is established to examine how the level of digital construction of construction enterprises affects carbon intensity:
where reflects the change in carbon emission intensity before and after the adoption of digital construction technology by enterprises and is the parameter to be estimated for the key variable. Considering that the application of digital construction technology by construction companies is an incremental behavior with time continuity, the sample of enterprises whose digital construction level is greater than 0 in the current year but 0 in the subsequent years is excluded from this paper.
Further, to verify the robustness of the difference in differences model again, the above model is tested again after focusing on regulating for firm fixed effects and year fixed effects , as shown in Equation (8):
In columns (3) to (4) of Table 6, the empirical findings based on the difference in differences model tests are displayed. Column (3) is tested with Equation (7), and the coefficient of is −4.788, which is significantly negative at the 1% level, again indicating that the carbon intensity of construction enterprises was significantly reduced after adopting digital construction technology. Column (4) shows the results of the test in Equation (8), adding firm and year fixed effects, and finds that the coefficient of shrinks significantly to −0.714, but remains significant at the 5% level. The above findings indicate that after mitigating the endogeneity of the model using the difference in differences model, the improvement in the level of digital construction still significantly reduces the carbon emission intensity.
The difference in differences model should satisfy the parallel trend assumption condition, i.e., the carbon emission intensity of the experimental group samples and the control group samples should have the same trend of change before the implementation of digital construction by the construction enterprises. For this reason, we carry out the parallel trend test, and the test results are shown in Figure 4.
In Figure 4, current denotes the time variable of the first implementation of digital construction technology by a construction enterprise, which is taken as the time base, and pre_i and post_i denote the time variable of the ith year before and after the implementation of digital construction technology by a construction enterprise, respectively. Let pre_3 denote the time variable 3 years before and before the implementation of digital construction technology by the enterprises, and post_5 denote the time variable 5 years after and after the implementation of digital construction technology by the enterprises. As can be seen in Figure 4, the estimated coefficients for all periods are significantly around 0 before the implementation of digital construction technology in construction firms, indicating that the experimental group is not significantly different from the control group and the parallel trend assumption is satisfied. After the implementation of digital construction technology, the estimated coefficients show a significant downward trend, which indicates that digital construction has a significant inhibitory effect on the carbon emission intensity of construction enterprises.
The disincentive influence of digital construction on carbon emission intensity may vary among different types of enterprises, so this paper will classify construction enterprises according to the nature of business and the industry segment to which they belong for heterogeneity analysis.
This paper separates the sample into state-owned and non-state-owned firms based on the kind of business, and lines (1) to (2) of Table 7 analyze the carbon emission reduction impact of businesses with various business natures. The study discovers that the effect of digital construction level on carbon emission intensity in the group of state-owned enterprises is significantly negative at the 5% level, and its coefficient is −2.234; while the regression coefficient of digital construction on carbon emission intensity for the group of non-state-owned companies is also negative (−1.068), but it fails the statistical significance test, and its coefficient is also significantly lower than that of the state-owned enterprises’ group, indicating that the digital construction level of non-state-owned companies is relatively less effective in curbing carbon emission intensity compared to state-owned companies. The reason for this difference may be that, for one thing, state-owned companies need to consider social benefits in addition to economic benefits when formulating their own development strategies, and take more social responsibilities than non-state-owned companies, as well as consider the overall development of society and actively respond to the strategy of the carbon peaking and carbon neutrality goals proposed by China, so they play a greater role in carbon emission reduction in the building sector. For another thing, the implementation of digital construction technology in construction enterprises requires large-scale investment in software such as BIM, virtual design and construction and digital twin, or high-end equipment such as construction robots and intelligent factories. State-owned enterprises not only have more advantages in terms of capital and technology but also have access to more preferential policies, resource support and institutional guarantees, which can provide sufficient conditions for the rapid development of digital construction technologies, thus empowering enterprises to reduce carbon emissions.
In this paper, the sample is divided into civil engineering construction enterprises and non-civil engineering construction enterprises according to the differences in the sub-sectors and main business of construction enterprises, and the influence of digital construction on carbon emission intensity is examined for both types of enterprises, and the results are indicated in lines (3) to (4) of Table 7. It has been discovered that digital construction technology significantly decreases the intensity of carbon emissions for civil engineering construction companies (the regression coefficient is −1.900 and passes the 1% statistical significance test). In contrast, for non-civil engineering construction enterprises, including landscape, building decoration and other construction enterprises, the coefficient of DIGC does not pass the statistical significance test, indicating that civil engineering construction enterprises are more able to promote carbon emission reduction by adopting digital construction technology compared to non-civil engineering construction enterprises. The reason for this difference may be that the adoption of digital construction technology is more focused on the field of engineering construction, which can realize the refinement, wisdom and efficiency of the whole life cycle management of the construction process, such as architectural design, construction and operation, for example, the use of the IoT and intelligent construction platform can realize fine construction, so that logistics scheduling, construction scheduling and other information flow automatically, and reduce the cost and increase the efficiency of engineering projects, while for landscape, building decoration and other construction industry enterprises, the use of digital construction technology is more limited, so civil engineering construction enterprises will suppress carbon emission intensity to a greater extent based on the extensive use of digital construction technology.
On the basis of the previous theoretical analysis, the implement of digital construction in construction enterprises can decrease carbon emission intensity and promote carbon emission reduction in enterprises by improving R&D innovation capability and total factor productivity. In order to verify this mechanism of action, the following mechanism testing models are utilized to analyze the R&D innovation capacity effect and the total factor productivity effect, based on the methodology of Huang et al. (2023):
where is the mechanism variable, denoting enterprises’ R&D innovation capability (RDI) and total factor productivity (TFP), is random error terms, is firm fixed effects, and is year fixed effects.
In this study, we assess the R&D innovation capability of companies on the basis of two factors: R&D input and innovation output (Hall and Lerner, 2010), where R&D input is expressed by the annual R&D investment funds of enterprises; the innovation output of enterprises primarily consists of invention, utility model and design patents. The quantity of patent applications is a more timely indicator of an organization’s potential for innovation because patents typically take a long time to be granted (Zhang and Dong, 2023). Finally, utilizing the enterprise’s R&D expenditure and the quantity of annual patent applications, the proxy variables of the enterprise’s R&D innovation capabilities are synthesized using the entropy method. Then, the total factor productivity of companies is determined using the LP approach based on the research of Levinsohn and Petrin (2003) and Lu and Lian (2012).
The regression result in line (1) of Table 8 shows that the regression coefficient of digital construction level is significantly negative, indicating that the use of digital construction in construction enterprises can lower carbon emission levels; the use of digital construction is advantageous for enhancing the R&D innovation capability of construction firms, as shown by the regression coefficient of R&D innovation capability in line (2) of Table 8 being statistically significant in the positive direction at the 1% level. On the one hand, the use of digital construction technology prompts construction enterprises to make extensive use of emerging digital technologies and break down information silos, obtain heterogeneous innovation resources and advanced technologies and management tools from more channels, optimize the allocation of resources (Huang et al., 2023), and reduce the waste of resources, which improves the R&D and innovation capability and at the same time reduces the carbon emissions of enterprises. On the other hand, construction companies are bound to adopt more digital construction technologies, increase R&D investment, improve R&D and innovation capacity, and generate new and more productive energy efficient technologies through the innovation process (Petrović and Lobanov, 2020), in order to improve efficiency and reduce costs in the planning, design, construction and building operation phases. An increase in R&D investment reduces CO2 emissions through direct and indirect effects (rebound effects, spillover effects) (Fernández Fernández et al., 2018). As a result of the above analysis, there is a mechanism of “digital construction development → enterprise R&D innovation capability improvement → carbon emission intensity reduction”, and hypothesis H2 is verified.
According to the regression coefficient in line (3) of Table 8, which is dramatically positive at the 1% level, the growth of digital construction is favorable to increasing the total factor productivity of construction enterprises. Digital construction technology can increase total factor productivity by improving productivity and efficiency in the construction process and maximizing the elimination of waste and uncertainty of resources. The enhancement in total factor productivity implies technological progress and technological revolution, which can enable construction companies to obtain the desired output with sufficiently few construction inputs such as materials, energy and labor, and the efficiency of energy utilization has been improved, thus reducing the intensity of carbon emissions. Technological advances have been widely recognized as the most promising approach to curbing China’s current carbon emissions (Huang et al., 2020), which could lead to CO2 reductions through carbon efficiency improvements (You and Zhang, 2022). After the above analysis, there is a mechanism of “digital construction development → total factor productivity increase → carbon emission intensity reduction” in this study, and hypothesis H3 is verified.
The continuous development of digital construction provides a significant opportunity for the low-carbon transformation of the construction industry. This paper constructs the digital construction level index of construction enterprises from three dimensions, and empirically analyzes and examines the impact and mechanism of digital construction on the carbon intensity of enterprises by using the two-way fixed effects model based on the panel data of 52 listed companies in China’s building industry from 2015 to 2021. The results of the study show that:
(1) The improvement of digital construction level can significantly reduce the carbon emission intensity of construction enterprises, and for every 1 unit increase in digital construction level, the carbon emission intensity of construction enterprises will decrease by 1.426 units. The conclusions are still valid after a battery of robustness tests and discussions on endogeneity issues such as replacing core variables, replacing models and using instrumental variables method, system GMM model and difference in differences model.
(2) This paper reveals the intrinsic mechanism between digital construction and the carbon emission intensity of construction enterprises, in which digital construction can significantly reduce the carbon emission intensity by improving the R&D and innovation capability and total factor productivity.
(3) From the point of view of the nature of enterprises, state-owned enterprises are more able to curb carbon emission intensity than non-state-owned enterprises in terms of improving the level of digital construction, which is mainly due to the fact that state-owned enterprises need to assume more social responsibility and have more resources and institutional safeguards, and therefore the leading role of state-owned enterprises should be played.
(4) In terms of the industry type of the enterprises, digital construction of civil engineering construction enterprises can significantly reduce carbon emission intensity due to their more extensive application of digital construction technology, while digital construction of non-civil engineering construction enterprises is not significant to carbon emission intensity.
This study further extends the application of project management life cycle theory, digital innovation theory, transaction cost theory and technological progress theory, and makes up for the lack of research on the impact of digital transformation of the construction industry on the carbon emission intensity of enterprises. The conclusions of this paper can provide a reference for the carbon emission reduction management decisions of construction enterprises, have practical guiding significance for future construction enterprises to implement digital change and integrate digital technology into all stages of project construction, and provide theoretical guidance for promoting the transformation and upgrading of the construction industry and for the introduction of relevant policies by the government.
According to the preceding research and conclusions, this paper provides the following policy recommendations:
(1) The government ought to speed up the formulation of leading policies for digital construction technology in the construction industry, encourage to fully incorporate of digital technology and engineering construction, guide each construction enterprise to recognize that digital construction is the best path to achieve carbon emission reduction, and also introduce a series of preferential policies and incentive policies to strengthen the digital technical support efforts and financial support for non-state construction enterprises and small and medium businesses, so as to stimulate the green innovation behavior and CO2 emission reduction potential of enterprises. Similarly, it is essential to actively cultivate compound talents in digital construction and carbon management of construction enterprises, and invest more resources in talent training. To this end, we should vigorously promote the construction of composite disciplines in colleges and universities and connect with the needs of the industry. Furthermore, it is also crucial to establish and robust a system for environmental information disclosure that complies with legal requirements, as well as to encourage enterprises to do so, as a result, strengthen enterprises’ environmental awareness and consciously and proactively promote green transformation and development.
(2) Construction enterprises ought to pay attention to and make full use of digital construction technologies and engineering wisdom management platforms such as blockchain technology, BIM technology, digital twin, 3D printing, construction robots, Internet of Things, VR and construction industry Internet platforms in construction projects, integrate the vision of green development into the whole process of construction and daily management, effectively reduce resource consumption and environmental pollution, and achieve a higher level of green and low-carbon. Simultaneously, they are also supposed to play the leading role of corporate champions, such as state-owned and central enterprises in the construction field, drive other enterprises to implement digital, networked and intelligent changes, vigorously develop digital construction, enhance the sense of social responsibility and promote green development of enterprises.
(3) Construction enterprises should implement the national innovation-driven development strategy, pay attention to improving R&D innovation capacity, increase R&D investment, strengthen cooperation with higher education institutions or other enterprises, obtain information through multiple channels, break information silos, effectively integrate the advantages of all parties, and focus superior resources on breaking through the key core technologies, engineering software and major equipment of digital construction, so as to provide green transformation for the construction industry. In the meanwhile, they should also focus on improving total factor productivity, taking the implementation of digital construction technology as an opportunity to advance the technological progress and technical efficiency of companies, improve energy utilization efficiency and decrease resource waste so as to maximize the carbon emission reduction effect of digital construction.
This paper explores and innovatively investigates the impact and mechanism of digital construction level on carbon emission of construction enterprises, but there are some limitations: firstly, this study examines the causal connection between an enterprise’s level of digital construction and its ability to reduce CO2 emissions, as well as the role that R&D and innovation capacity and total factor productivity play in that relationship, but there may be other ways and mechanisms that can be further explored; secondly, as a result of the shortage of data, this article solely takes Chinese listed companies in the construction industry as the sample, but there are still a sizable number of businesses left out and they can all be included in the sample for further exploration; finally, since most construction enterprises do not disclose the detailed data of carbon emissions, this paper obtains them indirectly, and with the improvement and implementation of the system related to environmental information disclosure of enterprises, this data will be available directly in the future, so that the data will be more accurate.
The original contributions presented in the study are included in the article/Supplementary Material. Further inquiries can be directed to the corresponding author.
XY: conception, design of the study, validation, writing—review and editing, supervision and funding acquisition. GL: conception, design of the study, validation, writing—original draft preparation, data curation, visualization and software. XW: data curation, visualization, software and investigation. All authors contributed to manuscript revision, read, and approved the submitted version.
This research was supported by the 2021 Heilongjiang Province Philosophy and Social Science Research Planning Project (Grant no. 21GLB063) and 2022 Harbin University of Commerce Teachers “Innovation” Project Support Program Project (Grant no. 21GLB063).
The authors declare that the research was conducted in the absence of any commercial or financial relationships that could be construed as a potential conflict of interest.
All claims expressed in this article are solely those of the authors and do not necessarily represent those of their affiliated organizations, or those of the publisher, the editors and the reviewers. Any product that may be evaluated in this article, or claim that may be made by its manufacturer, is not guaranteed or endorsed by the publisher.
Adepoju O., Aigbavboa C., Nwulu N., Onyia M. (2022). “Construction 4.0,” in Re-skilling human resources for construction 4.0,. Eds. Chen S.-H., Prisco D. M., Vayas I. (Berlin: Springer International Publishing), 17–39.
Altinoz B., Vasbieva D., Kalugina O. (2021). The effect of information and communication technologies and total factor productivity on CO(2) emissions in top 10 emerging market economies. Environ. Sci. pollut. Res. Int. 28, 63784–63793. doi: 10.1007/s11356-020-11630-1
Amri F., Zaied Y. B., Lahouel B. B. (2019). ICT, total factor productivity, and carbon dioxide emissions in Tunisia. Technol. Forecast. Soc Change 146, 212–217. doi: 10.1016/j.techfore.2019.05.028
Baduge S. K., Thilakarathna S., Perera J. S., Arashpour M., Sharafi P., Teodosio B., et al. (2022). Artificial intelligence and smart vision for building and construction 4.0: Machine and deep learning methods and applications. Autom. Constr. 141, 104440. doi: 10.1016/j.autcon.2022.104440
Bertani F., Raberto M., Teglio A. (2020). The productivity and unemployment effects of the digital transformation: an empirical and modelling assessment. Rev. Evol. Polit. Econ. 1, 329–355. doi: 10.1007/s43253-020-00022-3
Cai Y., Gong X., Zhao X. (2022). An empirical test of influence of digital economy development on enterprise TFP. Stat. Decis. 38, 98–103. doi: 10.13546/j.cnki.tjyjc.2022.15.018
CAICT. (2021). White paper on digital carbon neutrality. (Beijing, China: China Academy of Information and Communications Technology).
CAICT. (2022). Digital building development white paper. (Beijing: China Academy of Information and Communications Technology).
Chapple L., Clarkson P. M., Gold D. L. (2013). The cost of carbon: Capital market effects of the proposed emission trading scheme (ETS). Abacus 49, 1–33. doi: 10.1111/abac.12006
Chen Y., Huang D., Liu Z., Osmani M., Demian P. (2022). Construction 4.0, industry 4.0, and building information modeling (BIM) for sustainable building development within the smart city. Sustainability 14, 10028. doi: 10.3390/su141610028
Chen P., Kim S. (2023). The impact of digital transformation on innovation performance - The mediating role of innovation factors. Heliyon 9, e13916. doi: 10.1016/j.heliyon.2023.e13916
Chen X., Shuai C., Wu Y., Zhang Y. (2020). Analysis on the carbon emission peaks of China's industrial, building, transport, and agricultural sectors. Sci. Total Environ. 709, 135768. doi: 10.1016/j.scitotenv.2019.135768
Cheng Z., Li L., Liu J. (2021). Research on China’s industrial green biased technological progress and its energy conservation and emission reduction effects. Energy Effic. 14, 42. doi: 10.1007/s12053-021-09956-x
Chi Y., Liu Z., Wang X., Zhang Y., Wei F. (2021). Provincial CO2 emission measurement and analysis of the construction industry under China’s carbon neutrality target. Sustainability 13, 1876. doi: 10.3390/su13041876
China National Intellectual Property Administration. (2018). International Patent Classification and National Economic Industry Classification Reference Relationship Table (2018) Available at: https://www.cnipa.gov.cn/art/2018/10/8/art_75_131968.html. [Accessed June 30, 2023].
Comin D. (2010). “Total factor productivity,” in Economic growth,. Eds. Durlauf S. N., Blume L. E. (London: Palgrave Macmillan), 260–263.
Craveiro F., Duarte J. P., Bartolo H., Bartolo P. J. (2019). Additive manufacturing as an enabling technology for digital construction: A perspective on Construction 4.0. Autom. Constr. 103, 251–267. doi: 10.1016/j.autcon.2019.03.011
Danish (2019). Effects of information and communication technology and real income on CO2 emissions: The experience of countries along Belt and Road. Telemat. Inform. 45, 101300. doi: 10.1016/j.tele.2019.101300
Dave B., Boddy S., Koskela L. (2011). “Visilean: Designing a production management system with lean and BIM,” in 19th Annual conference of the international group for lean construction (Lima). Lima, Peru: The International Group for Lean Construction, 477–487
Dave B., Kubler S., Främling K., Koskela L. (2016). Opportunities for enhanced lean construction management using Internet of Things standards. Autom. Constr. 61, 86–97. doi: 10.1016/j.autcon.2015.10.009
Ding L. (2020). “Digital construction framework system,” in Digital construction introduction,. Eds. Zhao X., Zhu X. (Beijing China: China Architecture & Building Press), 22–37.
Ding X., Gao L., Wang G., Nie Y. (2022). Can the development of digital financial inclusion curb carbon emissions? Empirical test from spatial perspective. Front. Environ. Sci. 10. doi: 10.3389/fenvs.2022.1045878
Dou Y., Li T., Li L., Zhang Y., Li Z. (2023). Tracking the research on ten emerging digital technologies in the AECO industry. J. Constr. Eng. Manage. 149, 03123003. doi: 10.1061/jcemd4.Coeng-12290
Du J., Shen Z., Song M., Zhang L. (2023a). Nexus between digital transformation and energy technology innovation: An empirical test of A-share listed enterprises. Energy Econ. 120, 106572. doi: 10.1016/j.eneco.2023.106572
Du Q., Zhu H., Huang Y., Pang Q., Shi J. (2023b). Profit allocation of carbon emission reduction in the construction supply chain. Environ. Dev. Sustain., 1–30. doi: 10.1007/s10668-023-03488-3
Ernstsen S. N., Whyte J., Thuesen C., Maier A. (2021). How innovation champions frame the future: three visions for digital transformation of construction. J. Constr. Eng. Manage. 147, 05020022. doi: 10.1061/(asce)co.1943-7862.0001928
Fan L. W., You J., Zhang W., Zhou P. (2021). How does technological progress promote carbon productivity? Evidence from Chinese manufacturing industries. J. Environ. Manage. 277, 111325. doi: 10.1016/j.jenvman.2020.111325
Fang Z., Razzaq A., Mohsin M., Irfan M. (2022). Spatial spillovers and threshold effects of internet development and entrepreneurship on green innovation efficiency in China. Technol. Soc 68, 101844. doi: 10.1016/j.techsoc.2021.101844
Fernández Fernández Y., Fernández López M. A., Olmedillas Blanco B. (2018). Innovation for sustainability: The impact of R&D spending on CO2 emissions. J. Clean Prod. 172, 3459–3467. doi: 10.1016/j.jclepro.2017.11.001
Forcael E., Ferrari I., Opazo-Vega A., Pulido-Arcas J. A. (2020). Construction 4.0: A literature review. Sustainability 12, 9755. doi: 10.3390/su12229755
García De Soto B., Agustí-Juan I., Hunhevicz J., Joss S., Graser K., Habert G., et al. (2018). Productivity of digital fabrication in construction: Cost and time analysis of a robotically built wall. Autom. Constr. 92, 297–311. doi: 10.1016/j.autcon.2018.04.004
Hall B. H., Lerner J. (2010). “The financing of R&D and innovation,” in Handbook of the economics of innovation,. Eds. Hall B. H., Rosenberg N. (Amsterdam: Elsevier), 609–639.
Hennart J. F. (1988). A transaction costs theory of equity joint ventures. Strateg. Manage. J. 9, 361–374. doi: 10.1002/smj.4250090406
Hu X., Liu C. (2017). Total factor productivity measurement with carbon reduction. Engineering Construction Architectural Manage. 24, 575–592. doi: 10.1108/ecam-06-2015-0097
Hu J., Zhang H., Irfan M. (2023). How does digital infrastructure construction affect low-carbon development? A multidimensional interpretation of evidence from China. J. Clean Prod. 396, 136467. doi: 10.1016/j.jclepro.2023.136467
Huang J., Chen X., Yu K., Cai X. (2020). Effect of technological progress on carbon emissions: New evidence from a decomposition and spatiotemporal perspective in China. J. Environ. Manage. 274, 110953. doi: 10.1016/j.jenvman.2020.110953
Huang Y., Hu M., Xu J., Jin Z. (2023). Digital transformation and carbon intensity reduction in transportation industry: Empirical evidence from a global perspective. J. Environ. Manage. 344, 118541. doi: 10.1016/j.jenvman.2023.118541
Kim K. (2019). Moderating effects of legitimacy on the liability- and R&D investment-innovation relationships in manufacturing SMEs. Asian J. Technol. Innov. 27, 23–45. doi: 10.1080/19761597.2019.1594321
Kock C. J., Santaló J., Diestre L. (2012). Corporate governance and the environment: what type of governance creates greener companies? J. Manage. Stud. 49, 492–514. doi: 10.1111/j.1467-6486.2010.00993.x
Lahouel B. B., Taleb L., Zaied B. Y., Managi S. (2021). Does ICT change the relationship between total factor productivity and CO2 emissions? Evidence based on a nonlinear model. Energy Econ. 101, 105406. doi: 10.1016/j.eneco.2021.105406
Leontief W. (1970). Environmental repercussions and the economic structure: An input-output approach. Rev. Econ. Stat. 52, 262–271. doi: 10.2307/1926294
Levinsohn J., Petrin A. (2003). Estimating production functions using inputs to control for unobservables. Rev. Econ. Stud. 70, 317–341. doi: 10.1111/1467-937x.00246
Li S., Gao L., Han C., Gupta B., Alhalabi W., Almakdi S. (2023a). Exploring the effect of digital transformation on Firms’ innovation performance. J. Innov. Knowl. 8, 100317. doi: 10.1016/j.jik.2023.100317
Li X., Liu J., Ni P. (2021). The impact of the digital economy on CO2 emissions: A theoretical and empirical analysis. Sustainability 13, 7267. doi: 10.3390/su13137267
Li K., Ma M., Xiang X., Feng W., Ma Z., Cai W., et al. (2022). Carbon reduction in commercial building operations: A provincial retrospection in China. Appl. Energy 306, 118098. doi: 10.1016/j.apenergy.2021.118098
Li S., Wang W., Wang L., Wang G. (2023b). Digital economy and 3E efficiency performance: Evidence from EU countries. Sustainability 15, 5661. doi: 10.3390/su15075661
Liu N., Ding X.-J., Mao J.-Q., Wei Y.-H. (2023c). Research on the influence of enterprise’s digital transformation on carbon emission intensity—A moderated mediation model. Int. Business Res. 16, 27–38. doi: 10.5539/ibr.v16n4p27
Liu J., Li Y., Wang Z. (2023a). The potential for carbon reduction in construction waste sorting: A dynamic simulation. Energy 275, 127477. doi: 10.1016/j.energy.2023.127477
Liu M., Li C., Wang S., Li Q. (2023b). Digital transformation, risk-taking, and innovation: Evidence from data on listed enterprises in China. J. Innov. Knowl. 8, 100332. doi: 10.1016/j.jik.2023.100332
Liu Z., Li P., Wang F., Osmani M., Demian P. (2022b). Building information modeling (BIM) driven carbon emission reduction research: A 14-year bibliometric analysis. Int. J. Environ. Res. Public. Health 19, 12820. doi: 10.3390/ijerph191912820
Liu J., Yu Q., Chen Y., Liu J. (2022a). The impact of digital technology development on carbon emissions: A spatial effect analysis for China. Resour. Conserv. Recycl. 185, 106445. doi: 10.1016/j.resconrec.2022.106445
Lu W.-C. (2018). The impacts of information and communication technology, energy consumption, financial development, and economic growth on carbon dioxide emissions in 12 Asian countries. Mitig. Adapt. Strateg. Glob. Change 23, 1351–1365. doi: 10.1007/s11027-018-9787-y
Lu X., Lian Y. (2012). Estimation of total factor productivity of industrial enterprises in China: 1999-2007. China Econ. Q. 11, 541–558. doi: 10.13821/j.cnki.ceq.2012.02.013
Lyu Y., Zhang L., Wang D. (2023). The impact of digital transformation on low-carbon development of manufacturing. Front. Environ. Sci. 11. doi: 10.3389/fenvs.2023.1134882
Ma Q., Tariq M., Mahmood H., Khan Z. (2022). The nexus between digital economy and carbon dioxide emissions in China: The moderating role of investments in research and development. Technol. Soc 68, 101910. doi: 10.1016/j.techsoc.2022.101910
Ma X., Xiong F., Olawumi T. O., Dong N., Chan A. P. (2018). Conceptual framework and roadmap approach for integrating BIM into lifecycle project management. J. Manage. Eng. 34, 05018011. doi: 10.1061/(ASCE)ME.1943-5479.0000647
Metallidou C., Psannis K. E., Vergados D. D., Dossis M. (2022). “Digital twin and industrial internet of things architecture to reduce carbon emissions,” in 2022 4th International Conference on Computer Communication and the Internet (ICCCI). Chiba, Japan: IEEE 185–189. doi: 10.1109/ICCCI55554.2022.9850248
Miu L., Chen J., Fan T., Lv Y. (2022). The impact of digital economy development on carbon emission:A panel data analysis of 278 prefecture-level cities. S. China Financ. 546, 45–57. doi: 10.3969/j.issn.1007-9041.2022.02.004
Mubarak M. F., Tiwari S., Petraite M., Mubarik M., Raja Mohd Rasi R. Z. (2021). How Industry 4.0 technologies and open innovation can improve green innovation performance? Manage. Environ. Qual. 32, 1007–1022. doi: 10.1108/meq-11-2020-0266
Myers S. C., Majluf N. S. (1984). Corporate financing and investment decisions when firms have information that investors do not have. J. Financ. Econ. 13, 187–221. doi: 10.1016/0304-405X(84)90023-0
Nematollahi B., Vijay P., Sanjayan J., Nazari A., Xia M., Naidu Nerella V., et al. (2018). Effect of polypropylene fibre addition on properties of geopolymers made by 3D printing for digital construction. Materials 11, 2352. doi: 10.3390/ma11122352
Nunn N., Qian N. (2014). US food aid and civil conflict. Am. Econ. Rev. 104, 1630–1666. doi: 10.1257/aer.104.6.1630
Oesterreich T. D., Teuteberg F. (2016). Understanding the implications of digitisation and automation in the context of Industry 4.0: A triangulation approach and elements of a research agenda for the construction industry. Comput. Ind. 83, 121–139. doi: 10.1016/j.compind.2016.09.006
Onat N. C., Kucukvar M., Tatari O. (2014). Scope-based carbon footprint analysis of US residential and commercial buildings: An input–output hybrid life cycle assessment approach. Build. Environ. 72, 53–62. doi: 10.1016/j.buildenv.2013.10.009
Pan M., Zhao X., Lv K., Rosak-Szyrocka J., Mentel G., Truskolaski T. (2023). Internet development and carbon emission-reduction in the era of digitalization: Where will resource-based cities go? Resour. Policy 81, 103345. doi: 10.1016/j.resourpol.2023.103345
Petrović P., Lobanov M. M. (2020). The impact of R&D expenditures on CO2 emissions: evidence from sixteen OECD countries. J. Clean Prod. 248, 119187. doi: 10.1016/j.jclepro.2019.119187
Schimanski C. P., Pradhan N. L., Chaltsev D., Pasetti Monizza G., Matt D. T. (2021). Integrating BIM with Lean Construction approach: Functional requirements and production management software. Autom. Constr. 132, 103969. doi: 10.1016/j.autcon.2021.103969
Shang Y., Raza S. A., Huo Z., Shahzad U., Zhao X. (2023). Does enterprise digital transformation contribute to the carbon emission reduction? Micro-level evidence from China. Int. Rev. Econ. Financ. 86, 1–13. doi: 10.1016/j.iref.2023.02.019
Shen K., Ding L., Wang C. (2022). Development of a framework to support Whole-Life-Cycle Net-Zero-Carbon buildings through integration of building information modelling and digital twins. Buildings 12, 1747. doi: 10.3390/buildings12101747
Shen J., Tang P., Zeng H. (2020). Does China's carbon emission trading reduce carbon emissions? Evidence from listed firms. Energy Sustain Dev. 59, 120–129. doi: 10.1016/j.esd.2020.09.007
Sousa-Zomer T. T., Neely A., Martinez V. (2020). Digital transforming capability and performance: a microfoundational perspective. Int. J. Oper. Prod. Manage. 40, 1095–1128. doi: 10.1108/ijopm-06-2019-0444
Veskioja K., Soe R.-M., Kisel E. (2022). Implications of digitalization in facilitating socio-technical energy transitions in Europe. Energy Res. Soc Sci. 91, 102720. doi: 10.1016/j.erss.2022.102720
Wang B., Chen J., Liao F., Ao Y. (2023a). Path and policy of green and low-carbon transformation of construction industry in the context of intelligent construction. Sci. Technol. Rev. 41, 60–68. doi: 10.3981/j.issn.1000-7857.2023.05.006
Wang Z., He S., Zhang B., Wang B. (2018). Optimizing cooperative carbon emission reduction among enterprises with non-equivalent relationships subject to carbon taxation. J. Clean Prod. 172, 552–565. doi: 10.1016/j.jclepro.2017.10.196
Wang J., Liu Y., Wang W., Wu H. (2023b). How does digital transformation drive green total factor productivity? Evidence from Chinese listed enterprises. J. Clean Prod. 406, 136954. doi: 10.1016/j.jclepro.2023.136954
Wang L., Wang H., Cao Z., He Y., Dong Z., Wang S. (2022). Can industrial intellectualization reduce carbon emissions? — Empirical evidence from the perspective of carbon total factor productivity in China. Technol. Forecast. Soc Change 184, 121969. doi: 10.1016/j.techfore.2022.121969
Wang Q., Zhang F. (2020). Does increasing investment in research and development promote economic growth decoupling from carbon emission growth? An empirical analysis of BRICS countries. J. Clean Prod. 252, 119853. doi: 10.1016/j.jclepro.2019.119853
Wen H., Wen C., Lee C.-C. (2022a). Impact of digitalization and environmental regulation on total factor productivity. Inf. Econ. Policy 61, 101007. doi: 10.1016/j.infoecopol.2022.101007
Wen H., Zhong Q., Lee C.-C. (2022b). Digitalization, competition strategy and corporate innovation: Evidence from Chinese manufacturing listed companies. Int. Rev. Financ. Anal. 82, 102166. doi: 10.1016/j.irfa.2022.102166
Wong J. K. W., Li H., Wang H., Huang T., Luo E., Li V. (2013). Toward low-carbon construction processes: the visualisation of predicted emission via virtual prototyping technology. Autom. Constr. 33, 72–78. doi: 10.1016/j.autcon.2012.09.014
Woodhead R., Stephenson P., Morrey D. (2018). Digital construction: From point solutions to IoT ecosystem. Autom. Constr. 93, 35–46. doi: 10.1016/j.autcon.2018.05.004
Wu F., Hu H., Lin H., Ren X. (2021). Enterprise digital transformation and capital market performance: Empirical evidence from stock liquidity. J. Manage. World 37, 130–144+10. doi: 10.19744/j.cnki.11-1235/f.2021.0097
Wu L., Sun L., Chang Q., Zhang D., Qi P. (2022). How do digitalization capabilities enable open innovation in manufacturing enterprises? A multiple case study based on resource integration perspective. Technol. Forecast. Soc Change 184, 122019. doi: 10.1016/j.techfore.2022.122019
Xiao T., Sun R., Chun Y., Jian S. (2022). Digital transformation, human capital structure adjustment and labor income share. J. Manage. World 38, 220–237. doi: 10.19744/j.cnki.11-1235/f.2022.0174
Xie F., Kuang X. (2020). Can manufacturing corporations increase profit rates by expanding financial activities? An example of Chinese A-share listed manufacturing corporations. J. Manage. World 36, 13–28. doi: 10.19744/j.cnki.11-1235/f.2020.0180
Xue Y., Tang C., Wu H., Liu J., Hao Y. (2022). The emerging driving force of energy consumption in China: Does digital economy development matter? Energy Policy 165, 112997. doi: 10.1016/j.enpol.2022.112997
Yang Z., Gao W., Han Q., Qi L., Cui Y., Chen Y. (2022). Digitalization and carbon emissions: How does digital city construction affect China's carbon emission reduction? Sustain. Cities Soc. 87, 104201. doi: 10.1016/j.scs.2022.104201
Yang G., Wang F., Deng F., Xiang X. (2023). Impact of digital transformation on enterprise carbon intensity: The moderating role of digital information resources. Int. J. Environ. Res. Public. Health 20, 2178. doi: 10.3390/ijerph20032178
Yao W., Zhang W., Li W. (2023). Promoting the development of marine low carbon through the digital economy. J. Innov. Knowl. 8, 100285. doi: 10.1016/j.jik.2022.100285
Yilmaz G., Salter L., Mcfarlane D., Schönfuß B. (2023). Low-cost (Shoestring) digital solution areas for enabling digitalisation in construction SMEs. Comput. Ind. 150, 103941. doi: 10.1016/j.compind.2023.103941
You J., Zhang W. (2022). How heterogeneous technological progress promotes industrial structure upgrading and industrial carbon efficiency? Evidence from China's industries. Energy 247, 123386. doi: 10.1016/j.energy.2022.123386
Yu Z., Liu S., Zhu Z. (2022). Has the digital economy reduced carbon emissions?: Analysis based on panel data of 278 cities in China. Int. J. Environ. Res. Public. Health 19, 11814. doi: 10.3390/ijerph191811814
Zhang X. (2019). Research on evolution of innovation model under the condition of digital economy. Economist 247, 32–39. doi: 10.16158/j.cnki.51-1312/f.2019.07.005
Zhang J., Cheng J. C. P., Chen W., Chen K. (2022). Digital twins for construction sites: concepts, LoD definition, and applications. J. Manage. Eng. 38, 04021094. doi: 10.1061/(asce)me.1943-5479.0000948
Zhang X., Dong Z. (2023). Digital transformation and corporate technological innovation: mechanism identification, safeguard condition analysis and heterogeneity test. Econ. Rev. 239, 3–18. doi: 10.19361/j.er.2023.01.01
Zhang B., Xin Q., Tang M., Niu N., Du H., Chang X., et al. (2021). Revenue allocation for interfirm collaboration on carbon emission reduction: complete information in a big data context. Ann. Oper. Res. 316, 93–116. doi: 10.1007/s10479-021-04017-z
Zhang Y., Yan D., Hu S., Guo S. (2019). Modelling of energy consumption and carbon emission from the building construction sector in China, a process-based LCA approach. Energy Policy 134, 110949. doi: 10.1016/j.enpol.2019.110949
Zhao N., Ren J. (2023). Impact of enterprise digital transformation on capacity utilization: Evidence from China. PLoS One 18, e0283249. doi: 10.1371/journal.pone.0283249
Zhao C., Wang W. (2021). How does digitalt Transformation affect the total factor productivity of enterprises? Financ. Trade Econ. 42, 114–129. doi: 10.19795/j.cnki.cn11-1166/f.20210705.001
Zhou N., Khanna N., Feng W., Ke J., Levine M. (2018). Scenarios of energy efficiency and CO2 emissions reduction potential in the buildings sector in China to year 2050. Nat. Energy 3, 978–984. doi: 10.1038/s41560-018-0253-6
Zhou H. A., Otte T., Odenthal J., Abdelrazeq A., Hees F. (2020). “Towards a digital process platform for future construction sites,” in 2020 ITU kaleidoscope: Industry-driven digital transformation (ITU K)(Ha Noi, Vietnam: IEEE) 1–7. doi: 10.23919/ITUK50268.2020.9303198
Keywords: construction enterprises, digital construction, carbon emission reduction, R&D innovation capability, total factor productivity
Citation: Yang X, Lei G and Wang X (2023) Can the development of digital construction reduce enterprise carbon emission intensity? New evidence from Chinese construction enterprises. Front. Ecol. Evol. 11:1250593. doi: 10.3389/fevo.2023.1250593
Received: 30 June 2023; Accepted: 12 September 2023;
Published: 29 September 2023.
Edited by:
Tsun Se Cheong, Hang Seng University of Hong Kong, Hong Kong SAR, ChinaReviewed by:
Huwei Wen, Nanchang University, ChinaCopyright © 2023 Yang, Lei and Wang. This is an open-access article distributed under the terms of the Creative Commons Attribution License (CC BY). The use, distribution or reproduction in other forums is permitted, provided the original author(s) and the copyright owner(s) are credited and that the original publication in this journal is cited, in accordance with accepted academic practice. No use, distribution or reproduction is permitted which does not comply with these terms.
*Correspondence: Gaowei Lei, bGd3QHMuaHJiY3UuZWR1LmNu
Disclaimer: All claims expressed in this article are solely those of the authors and do not necessarily represent those of their affiliated organizations, or those of the publisher, the editors and the reviewers. Any product that may be evaluated in this article or claim that may be made by its manufacturer is not guaranteed or endorsed by the publisher.
Research integrity at Frontiers
Learn more about the work of our research integrity team to safeguard the quality of each article we publish.